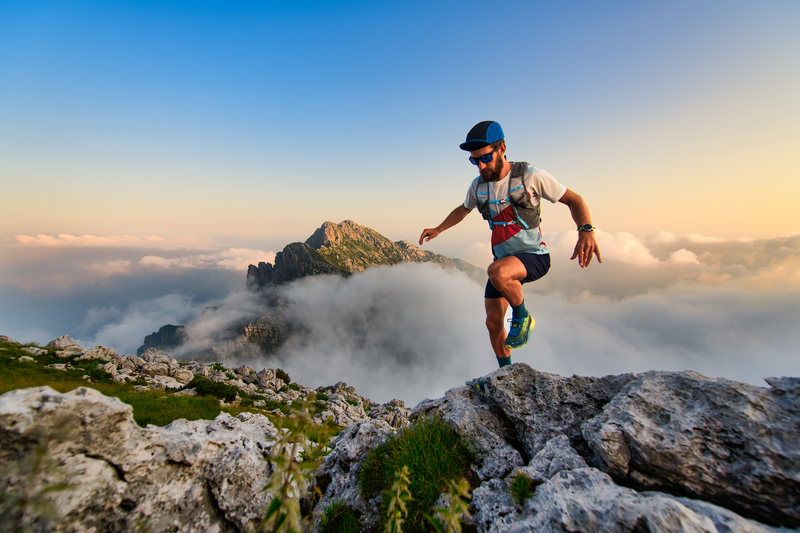
95% of researchers rate our articles as excellent or good
Learn more about the work of our research integrity team to safeguard the quality of each article we publish.
Find out more
EDITORIAL article
Front. Comput. Sci. , 07 August 2024
Sec. Mobile and Ubiquitous Computing
Volume 6 - 2024 | https://doi.org/10.3389/fcomp.2024.1465793
This article is part of the Research Topic Wearable Computing, Volume II View all 6 articles
Editorial on the Research Topic
Wearable computing, volume II
This editorial summarizes the content of the Research Topic Wearable computing, volume II. We thank the authors for their exceptional contributions.
The rapid proliferation of wearable technology has opened up unprecedented opportunities for monitoring, tracking, and influencing human behavior, physiology, and performance. From fitness trackers to smartwatches, wearable devices have become an integral part of modern life, promising to revolutionize the way we approach health, wellness, and daily activity. As the wearable market continues to expand, researchers and developers are working tirelessly to overcome the technical challenges and limitations that stand between us and a future where wearables are seamlessly integrated into our daily lives.
In this Research Topic, we accepted five articles in cutting-edge research in wearable computing, highlighting innovative solutions that are transforming our understanding of human physiology, behavior, and daily life. The five papers featured in this Research Topic demonstrate the vast potential of wearable technology to improve healthcare outcomes, enhance human performance, and revolutionize the way we interact with the world around us. From novel data solutions for measuring physiologic stress variables in clinical trials to advanced methods for tracking joint angle kinematics and detecting eye blinks, these papers showcase the latest advancements in wearable sensing, computing, and analytics. The Research Topic also explores critical challenges in wearable technology, including sensor selection, marker-based and markerless tracking, and synchronization of inertial measurement units (IMUs).
Collectively, these contributions underscore the enormous potential of wearable computing to transform healthcare, fitness, and daily life. They demonstrate how wearables can be harnessed to monitor and manage chronic conditions, enhance athletic performance, and improve our overall wellbeing. The energy-efficient, low-latency, and non-contact solutions presented in this Research Topic pave the way for widespread adoption of wearable technology, enabling seamless integration into our daily lives.
Uhlenberg and Amft validated the open-source OpenSense framework for estimating joint angles using IMU data, and its ability to select optimal sensors and positioning during daily activities was analyzed. The results showed that the framework can accurately estimate joint kinematics with median errors of < 2° across all joint angles, and that sensor selection is most important for complex joints like the shoulder. The study found that a minimum of 5–8 sensors is required to achieve the lowest estimation error across all daily activities involving upper and lower limbs.
Ray et al. proposed DrapeMoCapBench (DMCB+), an extended benchmark designed to evaluate the performance of both optical marker-based and markerless motion capture techniques across various loose garment types. The benchmark includes a nuanced analysis of advanced marker-based and markerless MoCap techniques, highlighting their strengths and weaknesses across distinct scenarios, including 3D physics simulations with six drape levels, three motion intensities, and six body-gender combinations. The results show that markerless MoCap outperforms marker-based alternatives in everyday activities with basic and swift motions, making it an advantageous and economical choice for wearable studies.
Gilbert et al. developed a new method for synchronizing multiple wireless IMU sensors using their onboard magnetometers. The method involves creating a known event using an external electromagnetic pulse, which is then measured by the magnetometer to synchronize the devices. The improved synchronization method reduces the maximum error from 40 ms per hour to 8 ms, making it suitable for synchronizing multi- person on-body sensors while they are being worn.
Liu et al. demonstrated a novel non-contact eye blink detection solution using capacitive sensing technology, integrated into standard glasses with a copper tape electrode on the frame. The system detects eye blinks by analyzing the capacitance-related signal generated when the eyelid moves with efficient user-independent machine learning models. The technology has potential for real-world applications requiring precise and unobtrusive eye blink detection.
Gerwin et al. showcased an algorithm called HRVEST to process large volumes of physiological data from wearable smart garments to measure heart rate variability (HRV) for emergency healthcare providers during the COVID-19 pandemic. HRVEST can automatically process biometric data in under 15 minutes, making it feasible for future studies and potentially allowing for real-time feedback to reduce physician stress. The algorithm's noise-filtering capabilities enable precise measurements of HRV, which could improve emotional wellbeing, clinical decision-making, and patient outcomes by providing optimal break-taking or meditation sessions to lower heart rate.
BZ: Writing – original draft, Writing – review & editing. CZ: Writing – review & editing. BI: Writing – review & editing.
The author(s) declare that no financial support was received for the research, authorship, and/or publication of this article.
The authors declare that the research was conducted in the absence of any commercial or financial relationships that could be construed as a potential conflict of interest.
All claims expressed in this article are solely those of the authors and do not necessarily represent those of their affiliated organizations, or those of the publisher, the editors and the reviewers. Any product that may be evaluated in this article, or claim that may be made by its manufacturer, is not guaranteed or endorsed by the publisher.
Keywords: activity recognition, machine learning, wearable technologies, motion capture, sensing, empirical studies
Citation: Zhou B, Zhang C and Islam B (2024) Editorial: Wearable computing, volume II. Front. Comput. Sci. 6:1465793. doi: 10.3389/fcomp.2024.1465793
Received: 16 July 2024; Accepted: 22 July 2024;
Published: 07 August 2024.
Edited and reviewed by: Kristof Van Laerhoven, University of Siegen, Germany
Copyright © 2024 Zhou, Zhang and Islam. This is an open-access article distributed under the terms of the Creative Commons Attribution License (CC BY). The use, distribution or reproduction in other forums is permitted, provided the original author(s) and the copyright owner(s) are credited and that the original publication in this journal is cited, in accordance with accepted academic practice. No use, distribution or reproduction is permitted which does not comply with these terms.
*Correspondence: Bo Zhou, Ym8uemhvdUBkZmtpLmRl
Disclaimer: All claims expressed in this article are solely those of the authors and do not necessarily represent those of their affiliated organizations, or those of the publisher, the editors and the reviewers. Any product that may be evaluated in this article or claim that may be made by its manufacturer is not guaranteed or endorsed by the publisher.
Research integrity at Frontiers
Learn more about the work of our research integrity team to safeguard the quality of each article we publish.