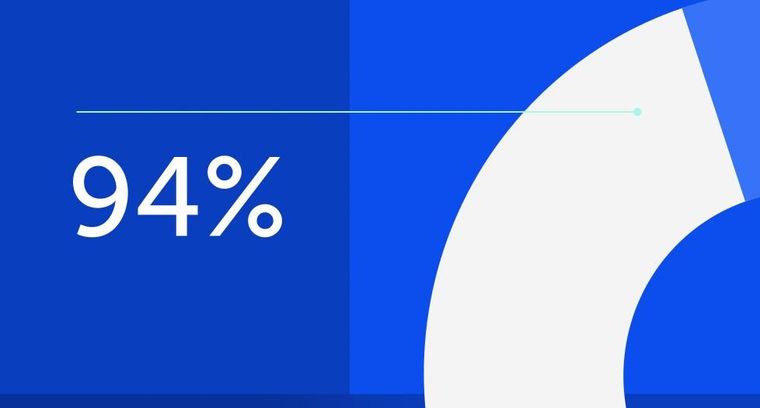
94% of researchers rate our articles as excellent or good
Learn more about the work of our research integrity team to safeguard the quality of each article we publish.
Find out more
ORIGINAL RESEARCH article
Front. Comput. Sci., 05 November 2024
Sec. Human-Media Interaction
Volume 6 - 2024 | https://doi.org/10.3389/fcomp.2024.1461131
As AI technology develops, trust in agents (including robot, AI and anthropomorphic agent) is becoming more important for more AI applications in human society. Possible ways to improve the trust relationship include empathy, success-failure series, and capability (performance). Appropriate trust is less likely to cause deviations between actual and ideal performance. In this study, we focus on the agent's empathic behavior and success-failure series to increase trust in agents. We experimentally examine the effect of empathic behavior from agent to person on changes in trust over time. The experiment was conducted with a two-factor mixed design: empathic behavior (available, not available) and success-failure series (phase 1 to phase 5). An analysis of variance (ANOVA) was conducted using data from 200 participants. The results showed an interaction between the empathic behavior factor and the success-failure series factor, with trust in the agent repairing when empathic behavior was present. This result supports our hypothesis. This study shows that designing agents to be empathic behavior is an important factor for trust and helps humans build appropriate trust relationships with agents.
Humans live in society and use a variety of tools, but AI is sometimes relied upon more than humans. Indeed, today's AI issues concern the trustworthiness and ethical use of AI technology. Ryan (2020) focused on trustworthiness and discussed AI ethics and the problem of people anthropomorphizing AI. He determined that even complex machines that use AI should not be viewed as trustworthy. Instead, he suggested that organizations that use AI and the individuals within those organizations should ensure that they are trustworthy. Hallamaa and Kalliokoski (2022) discussed bioethics as a point of reference for weighing the metaethical and methodological approaches adopted in AI ethics. Also, they proposed that AI ethics could be made more methodologically solid and substantively more influential if the resources were enriched by adopting tools from fields of study created to improve the quality of human action and safeguard its desired outcomes. Kaplan et al. (2023) aimed to identify important factors that predict trust in AI and examined three predictive categories and subcategories of human characteristics and abilities, AI performance and attributes, and contextual challenges from data from 65 articles. Based on the results of several previous studies, all of the categories were decided (human factors, AI factors, and shared contextual factors). All of the categories examined were significant predictors of trust in AI.
Previous research on trust assumes that they have mutual interests during the trust relationship. While this is an important perspective in reliability trust (Gambetta and Gambetta, 1988), it is not necessarily to assume mutual benefit in decision trust (McKnight and Chervany, 1996). JOSANG summarized and explained the definitions for each type of trust. Reliability trust is the subjective probability by which an individual, A, expects that another individual, B, performs a given action on which its welfare depends. Decision trust is the extent to which one party is willing to depend on something or somebody in each situation with a feeling of relative security, even though negative consequences are possible.
The previous studies mentioned above were informed by discussions of ethical issues in using AI and meta-analyses to predict trust in AI, suggesting a future relationship between people and AI. However, the more AI is used in human society, the more trust in AI is discussed, and it is an important issue that failure to establish appropriate trust relationships can lead to overconfidence and distrust of AI agents, which in turn reduces task performance.
As we build trust in AI, we can think of ways to repair trust in AI. To this end, research on trust games and trust repair conducted among humans may be effective for AI as well. Masuda and Nakamura (2012) analyzed a trust game with two fixed roles of trustee (i.e., seller) and investor (i.e., buyer) and studied the equilibrium and replicator dynamics of this game. They showed that the reputation mechanism enables cooperation between unacquainted buyers and sellers under generous conditions, even when such a cooperative equilibrium coexists with an asocial equilibrium in which buyers do not buy and sellers cheat. Bagdasarov et al. (2019) conducted an experimental study using a scenario-based paradigm to examine the empathy of distrustful parties in trust repair, their specific responses to violations based on integrity (apology vs. denial), the nature of consequences (individual vs. organizational), and the value of their interaction. Results showed that empathy of the distrusted party functions better in trust repair than in its absence and, when combined with denial of fault, significantly enhances the offender's perception of integrity.
Reed et al. (2020) modeled the combined effects of smiles and personality pathology on trust; in two experiments, participants read vignettes depicting either borderline personality disorder, antisocial personality disorder, or a person without personality pathology. Taken together, the results of the two experiments suggest that information on both the current emotional state and the personality traits of the other person is important for generating trust. Kähkönen et al. (2021) systematically reviewed research on trust repair conducted over the past 20 years, providing researchers and managers with comprehensive insights and directions for future research. The review suggested that early use of trust repair strategies for small violations can prevent these violations from escalating into larger violations and, in turn, increase the efficiency and effectiveness of trust repair with employees. Using insights gained from focus group discussions on a newly created set of trust, mistrust, and distrust questions, Bunting et al. (2021) identified how citizens perceive these different concepts and how these perceptions are gendered. They then used the new survey data collected to examine how the focus group results influenced survey responses and which survey items were most likely to effectively measure the three concepts.
With the emphasis on research on trust in human relationships, research on trust in AI agents has also received attention. In a study of trustworthy AI agents, Maehigashi et al. (2022a) investigated how beeps emitted by a social robot with anthropomorphic physicality affect human trust in the robot. They found that (1) sounds just prior to a higher performance increased trust when the robot performed correctly, and (2) sounds just prior to a lower performance decreased trust to a greater extent when the robot performed inaccurately. To determine how anthropomorphic physicality affects human trust in agents, Maehigashi et al. (2022b) also investigated whether human trust in social robots with anthropomorphic physicality is similar to trust in AI agents and humans. Also, they investigated whether trust in social robots is similar to trust in AI agents and humans. The results showed that the participants in this study formed trust in the social robot that was neither similar to the AI nor human and settled between them before and during the tasks. The results showed a possibility that manipulating anthropomorphic features would help assist human users in appropriately calibrating trust in an agent.
Previous studies have shown that people trust agents, but it is also known that people have empathy in addition to trust. One reason why people have trust and empathy toward agents is that humans are known to tend to treat artifacts as if they were humans in the media equation (Reeves and Nass, 1996). However, some humans do not accept these agents (Nomura et al., 2006, 2008, 2016). Empathy is closely related to trust. As agents permeate society in the future, it is hoped that they will have elements acceptable to humans.
In addition, the following several prior studies are well-known definitions of empathy in the field of psychology. Omdahl (1995) roughly classifies empathy into three types: (1) affective empathy, which is an emotional response to the emotional state of others, (2) cognitive understanding of the emotional state of others, which is defined as cognitive empathy, and (3) empathy including the above two. Preston and de Waal (2002) suggested that at the heart of the empathic response was a mechanism that allowed the observer to access the subjective emotional state of the target. They defined the perception-action model (PAM) and unified the different perspectives in empathy. They defined empathy as three types: (a) sharing or being influenced by the emotional state of others, (b) assessing the reasons for the emotional state, and (c) having the ability to identify and incorporate other perspectives.
Although there has been active research on empathy and trust between humans, few studies have focused on people and anthropomorphic agents. Among them, previous studies have focused directly on the relationship between people and agents, but there is a need to investigate empathy and trust repair toward observed agents. In particular, when an agent is entrusted with a task, trust in the agent should fluctuate depending on whether the agent succeeds or fails at the task. Therefore, our research questions are twofold: RQ1: Does the agent's empathic behavior affect the repair of trust in the agent? RQ2: Does an agent's successful completion of a task after a task failure restore trust in the agent?
In this study, we experimentally investigate whether the effect of task success - failure remains significant in repairing trust between people and agents who have a weak relationship with each other. We focus on decision trust and investigate whether the success or failure of a task on the agent's part in the absence of mutual benefit affects trust in the agent. And then, in this study, we design the agent to have the ability to empathize with people so that trust in the agent can remain appropriate. Therefore, an agent's empathy in this study makes a person perceive that an agent is capable of empathy. Specifically, the agent performs gestures and statements of personal information as empathic abilities. This empathic capacity is defined as empathic behavior, and participants are surveyed by a questionnaire to determine whether the agent has empathic capacity. To investigate the possible influence of empathy on changes in trust over time, we also investigate trust in the agent for each phase in five phases. Our goal is to elucidate temporary changes in trust in person-agent interactions that do not continue the relationship and to stabilize trust in the agent through empathic behavior.
While there are many recent papers on trust in AI, we summarized our related prior research on the impact of trust in AI on AI performance and relationships. Kumar et al. (2023) noted that new technological advances with the use of AI in medicine have not only raised concerns about public trust and ethics, but have also generated much debate about its integration into medicine. They reviewed current research investigating how to apply AI methods to create smart predictive maintenance. Kahr et al. (2023) focused on trust in appropriate AI systems and how trust evolves over time in human-AI interaction scenarios. Results showed significantly higher trust in the high accuracy model, with behavioral trust not decreasing and subjective trust increasing significantly with higher accuracy. Kirtay et al. (2023) showed that robot trust based on computational/cognitive load within a sequential decision framework leads to effective partner selection and scaffolding between robots. The results indicate that the computational load generated by the robot's cognitive processing may serve as an internal signal for assessing the trustworthiness of interaction partners.
Ma et al. (2023) proposed promoting appropriate human trust on the basis of the correctness likelihood of both sides at the task instance level. Results showed that the correctness likelihood utilization strategy promotes more appropriate human trust in the AI compared to using only trust in the AI. Sweeney (2023) argued that a more robust account of the ability and willingness to trust social robots is needed. She demonstrated that existing accounts of trust in social robots are inadequate and argued that the features of pretense and deception inherent in social robot interactions both promote trust and risk undermining it. Silva et al. (2023) introduced the first comprehensive user study testing a broad approach to explainable machine learning. They provided the first large-scale empirical evidence of the impact of explainability on human-agent teaming. Their results highlight the benefits of counterfactual explanations and the drawbacks of explainability confidence scores, helping to guide the future of explainability research.
Maehigashi (2022) experimentally investigated the nature of human trust in communication robots compared to trust in other people and AI systems. Results showed that trust in robots in computational tasks that yield a single solution is essentially similar to that in AI systems, and partially similar to trust in others in emotion recognition tasks that allow multiple interpretations. Noting that lack of trust is one of the main obstacles standing in the way of taking full advantage of AI, Gillath et al. (2021) focused on increasing trust in AI through emotional means. Specifically, they tested the association between attachment style, an individual difference that describes how people feel, think, and act in relationships, and trust in AI. Results showed that increasing attachment insecurity decreased trust, while increasing attachment security increased trust in AI.
Lee and Rich (2021) investigated the role of distrust of the human system in people's perceptions of algorithmic decisions. Their online experiment and interview results suggested that participants who mistrust human medical providers such as doctors and nurses perceive healthcare AI as equally untrustworthy and as unfair as human medical providers. Focusing on clinicians as the primary users of AI systems in healthcare, Asan et al. (2020) presented the factors that shape trust between clinicians and AI. They posit that the following factors should be incorporated into the development of AI to achieve an optimal level of trust: fairness, transparency, and robustness.
We summarized previous studies on trust repair for agents and robots, as well as theoretical models of trust repair and strategies for repair. To better understand how individual attitudes influence trust repair strategies, Esterwood and Robert (2022) proposed a theoretical model based on the theory of cognitive dissonance: 100 participants were assigned to one of four repair strategies (apology, denial, explanation, or promise) and subjected to three trust violations. Esterwood and Robert (2023a) examined the effects of four different trust repair strategies (apology, denial, explanation, and promise) on overall trustworthiness and its sub-dimensions, competence, benevolence, and integrity, after repeated trust violations. The results showed that none of the repair strategies fully restored trustworthiness in two of its sub-dimensions, competence and integrity, after repeated trust violations. Also, Esterwood and Robert (2023b) conducted a study of 400 participants to determine whether mental perception affects the effectiveness of three different repair strategies (promise, denial, or apology). Results indicated that, overall, individual differences in perception of mind were an important consideration when attempting to implement effective apologies and denials between humans and robots. Results of this study indicated that overall, individual differences in perception of mind are vital considerations when seeking to implement effective apologies and denials between humans and robots.
Zhang et al. (2023) developed three categories of technical failures commonly observed in human-robot interaction (HRI) (logical, semantic, and syntactic failures) and investigated them along with four trust repair strategies (internal attribution apology, external attribution apology, denial, and no repair). According to the Results, semantic and syntax failures were perceived as competence-based trust violations. Also, an internal-attribution apology outperformed denial but not an external-attribution apology. Furthermore, denial was more harmful than no repair attempts for competence-based trust violations. Lyons et al. (2023) investigated how trust, reliability, and responsibility attribution are affected by observing robot teammates deviate from expected behavior. Results showed that trust and trustworthiness (competence, compassion, and integrity) decreased following the unexpected behavior.
Alhaji et al. (2021) conducted a two-stage lab experiment in which 32 participants worked with a virtual CoBot to disassemble a traction battery in a mixed-reality environment in a recycling context. The results revealed that trust dynamics were stronger during dissipation than during accumulation, highlighting different relevant factors (dependability, reliability, predictability, and faith) as more interactions occurred. Besides, the factors that showed relevance as trust accumulates differ from those appear as trust dissipates. They detected four factors while trust accumulates (perceived reliability, perceived dependability, perceived predictability, and faith) which do not appear while it dissipates. This points to an interesting conclusion that depending on the stage of the collaboration and the direction of trust evolvement, different factors might shape trust. Okamura and Yamada (2020a) proposed a method for adaptive trust calibration that consists of a framework for detecting inappropriate calibration conditions by monitoring the user's trust behavior and cognitive cues, called “trust calibration cues,” that prompt the user to resume trust calibration. They focused on key trust-related issues to consider when developing AI systems for clinical use.
Okamura and Yamada (2020b) focused their research on trust alignment as a way to detect and mitigate inappropriate trust alignment, and they addressed these research questions using a behavior-based approach to understanding calibration status. The results demonstrate that adaptive presentation of trust calibration cues can facilitate trust adjustments more effectively than traditional system transparency approaches. Oksanen et al. (2020) reported the results of a study that investigated trust in robots and AI in an online trust game experiment. The trust game manipulated the hypothetical opponents that were described as either AI or robots. These were compared with control group opponents using only a human name or a nickname. According to the Results, robots and AI were not less trusted than the control group, which indicates that people are becoming more trusting of new technology, at least in contexts where one needs to be able to trust the cognitive abilities and fairness of advanced technology. Also, They found an interaction effect indicating that those who had robot use experience and high robot use self-efficacy gave lower sums of money to AI and robot opponents than those who did not have experience with robots. This interaction also reveals that despite being familiar with robotics, people might be skeptical of the intentions of robots and AI with higher skills.
Sebo et al. (2019) investigated trust restoration between humans and robots in a competitive game context, where robots attempt to restore human trust after broken promises, using trust violation framings of either competence or honesty and trust restoration strategies of either apology or denial. Results indicated that participants interacting with robots using honesty trust violation framing and denial trust restoration strategies were significantly more likely to exhibit retaliatory behavior toward the robots. de Visser et al. (2016) considered anthropomorphism to be an important variable in resolving this apparent contradiction in the trust formation, violation, and repair stages. Participants received progressively less reliable advice from a computer, an avatar, and a human agent. Results showed that (a) anthropomorphic agents were associated with resistance to trust breakdown, (b) these effects were greater with greater uncertainty, and (c) incorporating human-like trust repair behavior nearly eliminated differences between agents.
We also considered the design of empathic behavior factor from previous studies of anthropomorphic agents using empathy. Tsumura and Yamada (2023a) focused on self-disclosure from agents to humans in order to enhance human empathy toward anthropomorphic agents, and they experimentally investigated the potential for self-disclosure by agents to promote human empathy. They found that the appearance factor did not have a main effect, and self-disclosure that was highly relevant to the scenario used facilitated more human empathy with a statistically significant difference. They also found that no self-disclosure suppressed empathy. Tsumura and Yamada (2023b) also focused on tasks in which humans and agents engage in a variety of interactions, and they investigated the properties of agents that have a significant impact on human empathy toward them. To investigate the effects of task content, difficulty, task completion, and an agent's expression on human empathy, the experiment were conducted. The results showed that human empathy toward the agent was difficult to maintain with only task factors, and that the agent's expression was able to maintain human empathy. In addition, a higher task difficulty reduced the decrease in human empathy, regardless of task content.
To clarify the empathy between agents/robots and humans, Paiva represented the empathy and behavior of empathetic agents (called empathy agents in HAI and HRI studies) in two different ways: targeting empathy and empathizing with observers (Paiva et al., 2004; Paiva, 2011; Paiva et al., 2017). Rahmanti et al. (2022) designed a chatbot with artificial empathic motivational support for dieting called “SlimMe” and investigated how people responded to the diet bot. They proposed a text-based emotional analysis that simulates artificial empathic responses to enable the bot to recognize users' emotions. Perugia et al. (2020) investigated which personality and empathy traits were related to facial mimicry between humans and artificial agents. Their findings showed that mimicry was affected by the embodiment that an agent has, but not by its humanness. It was also correlated with both individual traits indicating sociability and empathy and traits favoring emotion recognition.
Asada (2015) proposed “affective developmental robotics,” which produces more truly artificial empathy. The design of artificial empathy is one of the most essential issues in social robotics, and empathic interaction with the public is necessary to introduce robots into society. There are several previous studies that have investigated the relationship between trust and empathy. Johanson et al. (2023) investigated whether the use of verbal empathic statements and nods from a robot during video-recorded interactions between a healthcare robot and patient would improve participant trust and satisfaction. Results showed that the use of empathic statements by the healthcare robot significantly increased participants' empathy, trust, and satisfaction with the robot and reduced their distrust of the robot. Spitale et al. (2022) investigated the amount of empathy elicited by a social assistance robot storyteller and the factors that influence the user's perception of that robot. As a result, the social assistance robot narrator elicited more empathy when the object of the story's empathy matched that of the social assistance robot narrator.
The protocol was approved by the ethics committee of the National Institute of Informatics (13, April, 2020, No. 1). All studies were carried out in accordance with the recommendations of the Ethical Guidelines for Medical and Health Research Involving Human Subjects provided by the Ministry of Education, Culture, Sports, Science and Technology and Ministry of Health, Labor and Welfare in Japan. Written informed consent was provided by choosing one option on an online form: “I am indicating that I have read the information in the instructions for participating in this research. I consent to participate in this research.” All participants gave informed consent. After that, they were debriefed about the experimental procedures.
The purpose of this study is to investigate whether empathic behavior factor and the success or failure of recognition over time can induce human trust when agents perform image recognition. This objective is crucial for fostering human-agent cooperation in society. The following hypotheses have been formulated for this study. If these hypotheses are supported, this study will be valuable in developing agents that are more acceptable to humans.
On the basis of the above, we considered two hypotheses. These hypotheses were inspired by related studies. In particular, H1 was based on the results of Johanson et al. (2023) and Spitale et al. (2022). Their findings show that agents' empathic behavior increases trust and that agents' comments on task success or failure may be empathic. H2 was inspired by the results of Kahr et al. (2023) and Ma et al. (2023). Their findings show that the success of an agent's task over time may inhibit overconfidence in the agent from agent task failures while increasing trust in the agent. Experiments were conducted to investigate these hypotheses.
H1: When the agent has empathy, trust is more stable than when it does not have empathy.
H2: Trust is restored by the agent's subsequent success after an agent's mistake.
The H1 hypothesis investigates whether the agent's empathic behavior is associated with stabilizing trust values for the agent. If this hypothesis is supported, then it indicates that the agent's empathic behavior is effective for the trust relationship between person and agent. The H2 hypothesis investigates whether trust repair after an agent's mistake is significantly influenced by subsequent task success. If this hypothesis is supported, then it suggests that the decrease in trust due to an agent's mistake may be temporary.
In this experiment, a total of five phases (phase 1 to phase 5) were conducted for the image recognition task, with a trust questionnaire administered at the end of each phase. Additionally, nonverbal information in the form of gestures and verbal information in the form of the agent's self-evaluation statements were prepared as empathic behavior factor. The experiments were conducted in an online environment. The online environment used in this experiment has already been used as one experimental method (Davis, 1999; Tsumura and Yamada, 2023a,b).
The image recognition task in this experiment was prepared about the phenomenon that when noise is included in an image, which is considered problematic in image recognition research, images that a person could easily correct also fail. This is appropriate for our study, which is to investigate trust in agents with whom we have no direct relationship. Although the participants are not affected by the success or failure of the agent's task, investigating observers' trust in the agent is an important issue in the social use of agents.
A flowchart of this experiment is shown in Figure 1. Participants performed five phase tasks. In addition, all tasks involved watching a video of the agent's image recognition. Below, we describe the tasks. In this study, the agent's response is made by the empathic behavior factor (available, not available) in each phase. In each phase, the agents were quizzed to guess the animals in images, and in one phase, they saw three images of animals. The percentage of correct answers within a phase was standardized; for example, in phase 1, the agent would correctly recognize all three animal images, and in phase 2, the agent would fail to recognize all three. A total of 15 animal images were recognized, and the order in which the images were displayed was unified in all conditions. At the end of each phase, a questionnaire was administered to investigate trust in the agent. After the completion of the task, a questionnaire was also administered to investigate the agent's ability to empathize in order to confirm that the empathic behavior factor was understood by the participants.
Thus, the experiment was conducted in a two-factor mixed design. The independent variables were empathic behavior factor (available, not available) and success-failure series (phase 1 to phase 5). The dependent variable was trust in the agent. In total, there were 10 levels, but because of the within-participant factor, participants were only required to participate in one of the two types of experiments.
In this study, we used a questionnaire related to trust that has been used in previous psychological studies. To measure cognitive trust, the Multi-Dimensional Measure of Trust (MDMT) (Ullman and Malle, 2019) was used. MDMT was developed to measure a task partner's reliability and competence corresponding to the definition of cognitive trust. The participants rated how much the partner AI fit each word (reliable, predictable, dependable, consistent, competent, skilled, capable, and meticulous) on an 8-point scale (0: not at all–7: very). Moreover, for emotional trust, we asked participants to answer how much the partner AI fit each word (secure, comfortable, and content) on a 7-point scale (1: strongly disagree - 7: strongly agree) as in the previous study (Komiak and Benbasat, 2006). In our study, we removed the matching 0 scale of cognitive trust, bringing it to the same 7 scale as emotional trust. The trust questionnaire used in this study was the one used by Maehigashi et al. (2022a).
To investigate the characteristics of empathy, we modified the Interpersonal Reactivity Index (IRI) (Davis, 1980) to be an index for anthropomorphic agents. The main modifications were changing the target name. In addition, the number of items on the IRI questionnaire was modified to 12; for this, items that were not appropriate for the experiment were deleted, and similar items were integrated. Since both of the questionnaires used were based on IRI, a survey was conducted using a 5-point Likert scale (1: not applicable, 5: applicable).
The questionnaire used is shown in Table 1. Since Q4, Q9, and Q10 were reversal items, the points were reversed during analysis. Q1 to Q6 were related to affective empathy, and Q7 to Q12 were related to cognitive empathy. Participants answered a questionnaire after completing the task.
In this experiment, to make the agent appear empathetic, we used gestures as nonverbal information and the agent's? self-evaluated statements as verbal information. This agent was run on MikuMikuDance (MMD).1 MMD is a software program that runs 3D characters.
Figures 2, 3 show linguistic and non-linguistic information in the tasks. The purpose of preparing the empathic behavior factor was to investigate one of our hypotheses, that is, that overconfidence and distrust are mitigated when an empathic behavior factor is present. The agent's gestures were joyful when successful, and it displayed disappointment when unsuccessful. The agent's self-evaluations expressed confidence when it succeeded and made excuses when it failed.
To treat empathy as a factor in this study, participants were surveyed after the experiment on a questionnaire about whether the agent had empathy. A T-test was conducted on the sum of the 12 items of affective and cognitive empathy in Table 1. The results showed a main effect for the empathic behavior factor (t(196)=2.679, p=0.0040, d=0.3809). Participants felt higher empathy for the agent with empathic behavior (mean = 29.90, S.D. = 8.149) than without empathic behavior (mean = 26.87, S.D. = 7.760).
In this experiment, participants watched a total of five agent image-recognition quiz videos. In phase 1, the agent successfully recognized the images and gave correct answers for the three animal images. After this, a questionnaire of trust in the agent was administered, and the value of trust at phase 1 was used as the baseline.
The agent then failed at image recognition in phase 2 and phase 4 and succeeded in phase 3 and phase 5. This allowed for an equal survey of trust in the agent after successful and unsuccessful tasks. This allowed us to test our hypothesis that “Trust is less likely to increase after an agent makes a mistake and more likely to increase after an agent succeeds.”
We used Yahoo! Crowdsourcing to recruit participants, and we paid 62 yen (= 0.44 dollars US) to each participant as a reward. We created web pages for the experiments by using Google Forms, and we uploaded the video created for the experiment to YouTube and embedded it. All participants were able to understand Japanese. There were no criteria or requirements for participants other than the ability to understand Japanese.
There were a 200 (empathy-available: 99, empathy-not available: 101) participants in total. The average age was 46.46 years (S.D. = 11.52), with a minimum of 19 years and a maximum of 77 years. In addition, there were 101 males and 99 females. After that, as a result of using Cronbach's α coefficient for the reliability of the trust questionnaire, the coefficient was determined to be 0.9400 to 0.9793 under all conditions. Also, as a result of using Cronbach's α coefficient for the reliability of the empathy questionnaire, the coefficient was determined to be 0.8491 to 0.8635 under two conditions.
By Cronbach's α, the reliability of the trust and empathy questionnaire was high. Therefore, confirmatory factor analysis was used to investigate the validity of the trust and empathy questionnaires after the experiment. The resulting factor loadings for the trust questionnaire are shown in Table 2, with model applicability as follows: χ2(43)=398.3, p < .001, CFI=0.9823, RMSEA = 0.0909, AIC = 25,303, BIC = 25,470. Based on the above results, the validity of the questionnaire is sufficient because the model applicability is also high.
On the other hand, the confirmatory factor analysis of the empathy questionnaire was analyzed with 2 and 4 factors, respectively. Table 3 shows the factor loadings of the empathy questionnaire in 2 factors (emotional and cognitive empathy), and the model applicability was as follows: χ2(53) = 391.3, p < 0.001, CFI = 0.7510, RMSEA = 0.1786, AIC = 6,119, BIC = 6,241. Based on these results, the model applicability is low when the empathy questionnaire is analyzed with two factors, and the questionnaire may not have sufficient validity. Table 4 shows the factor loadings of the empathy questionnaire on the four factors (on each scale), and the model applicability was as follows: χ2(48)= 175.8, p < 0.001, CFI = 0.9059, RMSEA = 0.1154, AIC = 5,913, and BIC = 6,052. Based on these results, the empathy questionnaire's four-factor analysis shows high model applicability and adequate questionnaire validity. Also, Table 5 results from the correlations between the factors.
We employed an ANOVA for a two-factor mixed-plan. ANOVA has been used frequently in previous studies and is an appropriate method of analysis with respect to the present study. The between-participant factors were two levels of empathy. There were five levels for the within-participant factor, success-failure series.
From the results of the participants' questionnaires, we investigated how empathic behavior and success-failure series affected as factors that elicit human trust. The values of trust aggregated in the task were used as the dependent variable. Due to the unbalanced data, Type III Sum of Squares (SS) was applied. R (ver. 4.1.0), statistical software, was used for the ANOVA and multiple comparisons in all analyses in this paper.
In this study, we considered cognitive and emotional trust jointly as trust. Table 6 shows the means and S.D. for each condition. A difference-adjusted Loftus-Masson confidence interval was used for the confidence intervals. Table 7 presents the ANOVA results for the 11-item trust questionnaire for agents. Also shown are ANOVA results for the trust categories cognitive trust (Qt1-Qt8) and emotional trust (Qt9-Q11). Because this study conducted two-way mixed ANOVA, we adjusted for the degrees of freedom of the within-participant factor by Mendoza's multisample sphericity test. In this paper, even if a main effect was found, if the interaction was significant, the analysis of the main effect was omitted, and the results were summarized. For multiple comparisons, Holm's multiple comparison test was used to examine whether there were significant differences.
The results of each questionnaire showed significant differences in the interaction between the two factors of empathic behavior and success-failure series. The results of the interaction are shown in Figure 4. In all conditions, there was no main effect for the empathic behavior factor. Since an interaction was found, a discussion of the main effect is omitted below. Table 8 shows the results of the multiple comparisons for the 11-item questionnaire.
Figure 4. All graphs of interaction between empathic behavior and success-failure series. Error bars indicate standard deviation. (A) Trust (Qt1-11). (B) Cognitive trust (Qt1-8). (C) Emotional trust (Qt9-11).
The results of Mendoza's multisample sphericity test were rejected with p < 0.000, so the degrees of freedom were adjusted with Greenhouse-Geisser's ϵ = 0.4481. The results for trust (Qt1-11) showed an interaction between the empathic behavior factor and success-failure series. Since an interaction was observed, Mendoza's multiple-sample sphericity test was performed before the simple main effect. Since it was rejected with p < 0.000, the degrees of freedom were adjusted with Greenhouse-Geisser's ϵ = 0.4137 for those with empathic behavior and ϵ = 0.4792 for those without empathic behavior. Multiple comparisons revealed that the simple main effect of the success-failure series factor with empathic behavior showed multiple significant differences among the five-level combinations, as shown in Figure 5A. The simple main effects of the success-failure series factor without empathic behavior also showed multiple significant differences among the five-level combinations, as shown in Figure 5B.
Figure 5. Results for success-failure series for empathic behavior factor on trust scale. Red lines are medians, and circles are outliers. (A) Empathic behavior. (B) No empathic behavior.
The simple main effects of the empathic behavior factor over time showed significant differences from phase 2 to 5, except for phase 1. Using trust in phase 1 as the criterion, these results indicate that trust was more stable when the agent had empathic behavior than when he did not. On the other hand, the results of significant differences over time showed that the success or failure of the image recognition task between phases had no effect on the trust values, and the evaluation of trust in the agent varied depending on the success or failure of each phase. The results of the post hoc analysis indicate that the empathic behavior factor is effective in building appropriate trust.
The results of Mendoza's multisample sphericity test were rejected with p < 0.000, so the degrees of freedom were adjusted with Greenhouse-Geisser's ϵ = 0.4557. Similarly trust, the results for cognitive trust (Qt1-8) showed an interaction between the empathic behavior factor and success-failure series. Since an interaction was observed, Mendoza's multiple-sample sphericity test was performed before the simple main effect. Since it was rejected with p < 0.000, the degrees of freedom were adjusted with Greenhouse-Geisser's ϵ = 0.4336 for those with empathic behavior and ϵ = 0.4748 for those without empathic behavior. Multiple comparisons revealed that the simple main effect of the success-failure series factor with empathic behavior showed multiple significant differences among the five-level combinations, as shown in Figure 6A. The simple main effects of the success-failure series factor without empathic behavior also showed multiple significant differences among the five-level combinations, as shown in Figure 6B.
Figure 6. Results for success-failure series for empathic behavior factor on cognitive trust. Red lines are medians, and circles are outliers. (A) Empathic behavior. (B) No empathic behavior.
The simple main effects of the empathic behavior factor over time showed significant differences from phase 2 to 5, except for phase 1. Using trust in phase 1 as the criterion, these results indicate that cognitive trust was more stable when the agent had empathic behavior than when he did not. On the other hand, the results of significant differences over time showed that the success or failure of the image recognition task between phases had no effect on cognitive trust, and the evaluation of cognitive trust in the agent varied depending on the success or failure of each phase. The results of the post hoc analysis indicate that the empathic behavior factor effectively builds appropriate trust.
The results of Mendoza's multisample sphericity test were rejected with p < 0.000, so the degrees of freedom were adjusted with Greenhouse-Geisser's ϵ = 0.4696. Similarly trust and cognitive trust, the results for emotional trust (Qt9-11) showed an interaction between the empathic behavior factor and success-failure series. Since an interaction was observed, Mendoza's multiple-sample sphericity test was performed before the simple main effect. Since it was rejected with p < 0.000, the degrees of freedom were adjusted with Greenhouse-Geisser's ϵ = 0.4184 for those with empathic behavior and ϵ = 0.5118 for those without empathic behavior. Multiple comparisons revealed that the simple main effect of the success-failure series factor with empathic behavior showed multiple significant differences among the five-level combinations, as shown in Figure 7A. The simple main effects of the success-failure series factor without empathic behavior also showed multiple significant differences among the five-level combinations, as shown in Figure 7B.
Figure 7. Results for success-failure series for empathic behavior factor on emotional trust. Red lines are medians, and circles are outliers. (A) Empathic behavior. (B) No empathic behavior.
The simple main effects of the empathic behavior factor over time showed significant differences from phase 2 to 4, except for phase 1. Phase 5 was not statistically significant. Using trust in phase 1 as the criterion, these results indicate that emotional trust was more stable when the agent had empathic behavior than when he did not. On the other hand, the results of significant differences over time showed that the success or failure of the image recognition task between phases had no effect on the emotional trust, and the evaluation of emotional trust in the agent varied depending on the success or failure of each phase. The results of the post hoc analysis indicate that the empathic behavior factor is effective in building appropriate trust.
The way to properly build trust between a human and an agent is to achieve a level of trust appropriate to the agent's performance. This idea is supported by several previous studies. Trust in agents is a necessary component for agents to be utilized in society. If trust in agents can be made constant with an appropriate approach, humans and agents can build a trusting relationship.
In this study, an experiment was conducted to investigate the conditions necessary for humans to trust agents. We focused on empathy from the agent to the human and the disclosure of the agent's capabilities over time as factors that influence trust. The purpose of this study was to investigate whether empathic behavior and success-failure series factors can control trust in interactions with trusting agents. To this end, two hypotheses were formulated, and the data obtained from the experiment were analyzed.
The results of the experiment showed an interaction between the empathic behavior factor and the time lapse factor, and multiple comparisons revealed that trust was stable from phase 2 to phase 5, based on phase 1, when the empathic behavior factor was present. In all of the analyses of trust, cognitive trust, and emotional trust, there was no significant trust due to the agent's empathic behavior factor in Phase 1. Still, across all levels from Phase 2 to Phase 5, when the agent's empathic behavior is present, a significant difference was found compared to when the agent's empathic behavior was absent.
When the agent fails the task, trust is higher when the agent's empathic behavior is present than when it is absent. When the agent succeeded in the task, trust was lower when the agent's empathic behavior was present than when the agent's empathic behavior was absent. Based on these results, it is clear that the swing of trust was smaller when the agent's empathic behavior was present than when the agent's empathic behavior was absent. These results support H1, that is, that when the agent has empathic behavior, trust is more stable than when it does not have empathic behavior.
Furthermore, H2, that is, that Trust is restored by the agent's subsequent success after an agent's mistake, was supported by the experiment. In the experiment, participants' trust changed significantly after each phase, with participants gaining greater trust by the agent getting it right even if it had made a mistake just before. In this experiment, the success or failure of the immediately preceding task is the trust at that time point.
One of the strengths of this study is that we were able to adjust trust in the agent to statistical significance through empathic behavior. In the case of failed image recognition, trust without empathic behavior was significantly lower than trust with empathic behavior, based on phase 1. This indicates that the initial perceived trust in the agent was reduced by the failed image recognition more. Also, the case of successful image recognition without empathic behavior showed significantly higher trust than the case with empathic behavior, based on phase 1. However, adequate trust is not achieved simply by judging from the success or failure of each phase.
This suggests that participants are prone to overconfidence and distrust by believing only in the results immediately prior to each phase. In contrast to this situation, the empathic behavior factor smoothed out the change in trust toward the agent. This result is a point revealed by this study. Few studies have used empathic behavior to promote an appropriate state of trust in agents, and by investigating changes in trust in agents over time and task success or failure, we were able to demonstrate a means of mitigating overconfidence and distrust in agents, which can be problematic when using agents in society.
The definition of trust in this study was treated as decision trust. This differs from trust in mutually beneficial cooperative games or joint tasks, where the outcome of the agent's task has no direct effect on the person. In real society, people and agents may not have a direct relationship. Previous studies have focused on trust in reliability because they assumed a joint task between a person and an agent. However, it was not applied to trust in relationships where the agent's task is observed in the surroundings. Therefore, this study focused on trust between people and agents that are not related to the task when the agent is used in society. The results showed that, when not task-related, trust is affected by the outcome of the previous task's success or failure.
A limitation of this study is that participants observed the trust agent's image recognition task by watching a video. Reliability trust is difficult to discuss in this study and may not apply to relationships that include mutual benefit relationships. The results of this study are not sufficient because the depth of the relationship between the participants and the agent was different from that of the actual introduction of agents into society. In addition, scenarios involving physical or cooperative interactions may yield different results when deep relationships are enhanced. Future research should be conducted in an environment where participants and agents actually perform image recognition tasks.
In addition, although the empathic behavior factor was used in the current study, the average value of the questionnaire on whether the agents thought they could empathize with the participants indicated that their empathy ability was low. This may be due to the fact that the appearance of the robot made it difficult to read facial expressions. Therefore, it is possible that providing an appearance with a recognizable facial expression may have a further effect on the trust relationship by making it easier to feel empathy from the agent.
As a trust in this study, based on the simple main effect results in Table 8, there was no significance in the empathic behavior factor at Phase 1. Therefore, the trust in phase 1 was treated as initial trust and trust repair could be discussed. However, depending on participants' background information (e.g., knowledge of AI and related occupations), the results may differ from those of this study. Similarly, while this study focuses on short-term relationships, other factors should be considered when considering long-term relationships.
The results of the confirmatory factor analysis of the trust and empathy questionnaire used in this study indicate that some questions may not be appropriate (Qe9). In addition, the low model applicability of the two-factor empathy questionnaire is due to the fact that the correlations among the factors in the four-factor empathy questionnaire indicate that empathic concern is highly positively correlated with perspective taking and Fantasy scale, indicating that it is difficult to separate affective and cognitive empathy. Future research should consider the most appropriate questionnaire items for classifying empathy.
To solve the problem of overconfidence and distrust in trust for agents, the development of appropriate trust relationships between anthropomorphic agents and humans is an important issue. When humans share tasks with agents, we expect that appropriate trust relationships will allow agents to be more utilized in human society. This study is an example of an investigation of the factors that influence trust in agents. The experiment was conducted in a two-factor mixed design, with empathic behavior as the between-participant factor and success-failure series as the within-participant factor. The number of levels for each factor was empathic behavior (available, not available) and success-failure series (phase 1 to phase 5). The dependent variable was confidence in the agent. The results showed an interaction between empathic behavior and success-failure series. It was shown that when the agent had empathic behavior, the confidence values were restored to the same level of confidence values as in Phase 1. These results support our hypothesis. This study is an important example of how empathic behavior and success-failure series (including agent competence) work when humans trust agents. Future research could examine cases of strengthening or weakening specific trust for cognitive and emotional trust to develop trust agents for a variety of situations.
The original contributions presented in the study are included in the article/Supplementary material, further inquiries can be directed to the corresponding author.
The studies involving humans were approved by the Ethics Committee of the National Institute of Informatics (13, April, 2020, No. 1). The studies were conducted in accordance with the local legislation and institutional requirements. The participants provided their written informed consent to participate in this study.
TT: Conceptualization, Formal analysis, Funding acquisition, Investigation, Methodology, Project administration, Validation, Visualization, Writing – original draft, Writing – review & editing. SY: Funding acquisition, Supervision, Validation, Writing – review & editing.
The author(s) declare financial support was received for the research, authorship, and/or publication of this article. This work was partially supported by JST, CREST (JPMJCR21D4), Japan. This work was also supported by JST, the establishment of university fellowships toward the creation of science technology innovation, Grant Number JPMJFS2136.
The authors declare that the research was conducted in the absence of any commercial or financial relationships that could be construed as a potential conflict of interest.
All claims expressed in this article are solely those of the authors and do not necessarily represent those of their affiliated organizations, or those of the publisher, the editors and the reviewers. Any product that may be evaluated in this article, or claim that may be made by its manufacturer, is not guaranteed or endorsed by the publisher.
The Supplementary Material for this article can be found online at: https://www.frontiersin.org/articles/10.3389/fcomp.2024.1461131/full#supplementary-material
Alhaji, B., Prilla, M., and Rausch, A. (2021). Trust dynamics and verbal assurances in human robot physical collaboration. Front. Artif. Intellig. 4:703504. doi: 10.3389/frai.2021.703504
Asada, M. (2015). Towards artificial empathy. Int. J. Soc. Robot. 7, 19–33. doi: 10.1007/s12369-014-0253-z
Asan, O., Bayrak, A. E., and Choudhury, A. (2020). Artificial intelligence and human trust in healthcare: focus on clinicians. J. Med. Internet Res. 22:e15154. doi: 10.2196/15154
Bagdasarov, Z., Connelly, S., and Johnson, J. F. (2019). Denial and empathy: partners in employee trust repair? Front. Psychol. 10:19. doi: 10.3389/fpsyg.2019.00019
Bunting, H., Gaskell, J., and Stoker, G. (2021). Trust, mistrust and distrust: A gendered perspective on meanings and measurements. Front. Polit. Sci. 3:642129. doi: 10.3389/fpos.2021.642129
Davis, M. H. (1980). A multidimensional approach to individual difference in empathy. In JSAS Catalog of Selected Documents in Psychology, page 85.
Davis, R. (1999). Web-based administration of a personality questionnaire: comparison with traditional methods. Behav. Res. Methods, Instrum. Comp. 31, 572–577. doi: 10.3758/BF03200737
de Visser, E. J., Monfort, S. S., McKendrick, R., Smith, M. A. B., McKnight, P. E., Krueger, F., et al. (2016). Almost human: Anthropomorphism increases trust resilience in cognitive agents. J. Exp. Psychol.: Appl. 22, 331–349. doi: 10.1037/xap0000092
Esterwood, C., and Robert, L. P. (2022). “Having the right attitude: How attitude impacts trust repair in human–robot interaction,” in 2022 17th ACM/IEEE International Conference on Human-Robot Interaction (HRI) (Sapporo: IEEE), 332–341. doi: 10.1109/HRI53351.2022.9889535
Esterwood, C., and Robert, L. P. (2023a). Three strikes and you are out!: The impacts of multiple human–robot trust violations and repairs on robot trustworthiness. Comput. Human Behav. 142:107658. doi: 10.1016/j.chb.2023.107658
Esterwood, C., and Robert, L. P. (2023b). The theory of mind and human-robot trust repair. Sci. Rep. 13:9877. doi: 10.1038/s41598-023-37032-0
Gambetta, D., and Gambetta, P. (1988). Trust: Making and Breaking Cooperative Relations. Oxford: B. Blackwell.
Gillath, O., Ai, T., Branicky, M. S., Keshmiri, S., Davison, R. B., and Spaulding, R. (2021). Attachment and trust in artificial intelligence. Comput. Human Behav. 115:106607. doi: 10.1016/j.chb.2020.106607
Hallamaa, J., and Kalliokoski, T. (2022). Ai ethics as applied ethics. Front. Comp. Sci. 4:776837. doi: 10.3389/fcomp.2022.776837
Johanson, D., Ahn, H. S., Goswami, R., Saegusa, K., and Broadbent, E. (2023). The effects of healthcare robot empathy statements and head nodding on trust and satisfaction: a video study. J. Human-Robot Interact. 12:1. doi: 10.1145/3549534
Kähkönen, T., Blomqvist, K., Gillespie, N., and Vanhala, M. (2021). Employee trust repair: A systematic review of 20 years of empirical research and future research directions. J. Busin. Res. 130, 98–109. doi: 10.1016/j.jbusres.2021.03.019
Kahr, P. K., Rooks, G., Willemsen, M. C., and Snijders, C. C. (2023). “It seems smart, but it acts stupid: Development of trust in ai advice in a repeated legal decision-making task,” in Proceedings of the 28th International Conference on Intelligent User Interfaces (IUI) (Sydney, NSW: Association for Computing Machinery), 528–539. doi: 10.1145/3581641.3584058
Kaplan, A. D., Kessler, T. T., Brill, J. C., and Hancock, P. A. (2023). Trust in artificial intelligence: meta-analytic findings. Human Fact. 65, 337–359. doi: 10.1177/00187208211013988
Kirtay, M., Hafner, V. V., Asada, M., and Oztop, E. (2023). Trust in robot–robot scaffolding. IEEE Trans. Cognit. Dev. Syst.15, 1841–1852. doi: 10.1109/TCDS.2023.3235974
Komiak, S. Y. X., and Benbasat, I. (2006). The effects of personalization and familiarity on trust and adoption of recommendation agents. MIS Quarte. 30, 941–960. doi: 10.2307/25148760
Kumar, P., Chauhan, S., and Awasthi, L. K. (2023). Artificial intelligence in healthcare: review, ethics, trust challenges & future research directions. Eng. Appl. Artif. Intell. 120:105894. doi: 10.1016/j.engappai.2023.105894
Lee, M. K., and Rich, K. (2021). “Who is included in human perceptions of ai?: Trust and perceived fairness around healthcare ai and cultural mistrust,” in Proceedings of the 2021 CHI Conference on Human Factors in Computing Systems (Yokohama: Association for Computing Machinery), 1–14. doi: 10.1145/3411764.3445570
Lyons, J. B., aldin Hamdan, I., and Vo, T. Q. (2023). Explanations and trust: What happens to trust when a robot partner does something unexpected? Comput. Human Behav. 138:107473. doi: 10.1016/j.chb.2022.107473
Ma, S., Lei, Y., Wang, X., Zheng, C., Shi, C., Yin, M., et al. (2023). Who should i trust: Ai or myself? Leveraging human and AI correctness likelihood to promote appropriate trust in AI-assisted decision-making,” in Proceedings of the 2023 CHI Conference on Human Factors in Computing Systems, 1–19.
Maehigashi, A. (2022). “The nature of trust in communication robots: Through comparison with trusts in other people and ai systems,” in 2022 17th ACM/IEEE International Conference on Human-Robot Interaction (HRI) (Sapporo: IEEE), 900–903.
Maehigashi, A., Tsumura, T., and Yamada, S. (2022a). “Effects of beep-sound timings on trust dynamics in human-robot interaction,” in Social Robotics, International Conference on Social Robotics (Florence: Springer), 652–662. doi: 10.1007/978-3-031-24670-8_57
Maehigashi, A., Tsumura, T., and Yamada, S. (2022b). “Experimental investigation of trust in anthropomorphic agents as task partners,” in Proceedings of the 10th International Conference on Human-Agent Interaction (HAI) (Christchurch: Association for Computing Machinery), 302–305. doi: 10.1145/3527188.3563921
Masuda, N., and Nakamura, M. (2012). Coevolution of trustful buyers and cooperative sellers in the trust game. PLOS ONE 7, 1–11. doi: 10.1371/journal.pone.0044169
McKnight, D., and Chervany, N. (1996). “The meanings of trust,” in Technical report, Technical Report MISRC Working Paper Series 96–04, University of Minnesota, Management Information Systems Research Center.
Nomura, T., Kanda, T., Kidokoro, H., Suehiro, Y., and Yamada, S. (2016). Why do children abuse robots? Interact. Stud. 17, 347–369. doi: 10.1075/is.17.3.02nom
Nomura, T., Kanda, T., and Suzuki, T. (2006). Experimental investigation into influence of negative attitudes toward robots on human-robot interaction. AI Soc. 20, 138–150. doi: 10.1007/s00146-005-0012-7
Nomura, T., Kanda, T., Suzuki, T., and Kato, K. (2008). Prediction of human behavior in human-robot interaction using psychological scales for anxiety and negative attitudes toward robots. IEEE Trans. Robot. 24, 442–451. doi: 10.1109/TRO.2007.914004
Okamura, K., and Yamada, S. (2020a). Adaptive trust calibration for human-AI collaboration. PLoS ONE 15:e0229132. doi: 10.1371/journal.pone.0229132
Okamura, K., and Yamada, S. (2020b). Empirical evaluations of framework for adaptive trust calibration in human-ai cooperation. IEEE Access 8, 220335–220351. doi: 10.1109/ACCESS.2020.3042556
Oksanen, A., Savela, N., Latikka, R., and Koivula, A. (2020). Trust toward robots and artificial intelligence: an experimental approach to human–technology interactions online. Front. Psychol. 11:568256. doi: 10.3389/fpsyg.2020.568256
Paiva, A. (2011). Empathy in social agents. Int. J. Virtual Real. 10, 1–4. doi: 10.20870/IJVR.2011.10.1.2794
Paiva, A., Dias, J., Sobral, D., Aylett, R., Sobreperez, P., Woods, S., et al. (2004). “Caring for agents and agents that care: building empathic relations with synthetic agents,” in Autonomous Agents and Multiagent Systems, International Joint Conference on 2, 194–201.
Paiva, A., Leite, I., Boukricha, H., and Wachsmuth, I. (2017). Empathy in virtual agents and robots: a survey. ACM Trans. Interact. Intell. Syst. 7:2912150. doi: 10.1145/2912150
Perugia, G., Paetzel, M., and Castellano, G. (2020). “On the role of personality and empathy in human-human, human-agent, and human-robot mimicry,” in Social Robotics, International Conference on Social Robotics, 120–131.
Preston, S. D., and de Waal, F. B. M. (2002). Empathy: Its ultimate and proximate bases. Behav. Brain Sci. 25, 1–20. doi: 10.1017/S0140525X02000018
Rahmanti, A. R., Yang, H.-C., Bintoro, B. S., Nursetyo, A. A., Muhtar, M. S., Syed-Abdul, S., et al. (2022). Slimme, a chatbot with artificial empathy for personal weight management: System design and finding. Front. Nutr. 9:870775. doi: 10.3389/fnut.2022.870775
Reed, L. I., Meyer, A. K., Okun, S. J., Best, C. K., and Hooley, J. M. (2020). In smiles we trust? Smiling in the context of antisocial and borderline personality pathology. PLOS ONE 15:e0234574. doi: 10.1371/journal.pone.0234574
Reeves, B., and Nass, C. (1996). The Media Equation: How People Treat Computers, Television, and New Media like Real People and Places (Cambridge: Cambridge University Press).
Ryan, M. (2020). In ai we trust: Ethics, artificial intelligence, and reliability. Sci. Eng. Ethics 26, 2749–2767. doi: 10.1007/s11948-020-00228-y
Sebo, S. S., Krishnamurthi, P., and Scassellati, B. (2019). “I don't believe you: Investigating the effects of robot trust violation and repair,” in 2019 14th ACM/IEEE International Conference on Human-Robot Interaction (HRI) (Daegu: IEEE), 57–65. doi: 10.1109/HRI.2019.8673169
Silva, A., Schrum, M., Hedlund-Botti, E., Gopalan, N., and Gombolay, M. (2023). Explainable artificial intelligence: Evaluating the objective and subjective impacts of xai on human-agent interaction. Int. J. Human–Comp. Inter. 39, 1390–1404. doi: 10.1080/10447318.2022.2101698
Spitale, M., Okamoto, S., Gupta, M., Xi, H., and Matarić, M. J. (2022). Socially assistive robots as storytellers that elicit empathy. J. Human-Robot Inter. 11:3538409. doi: 10.1145/3538409
Tsumura, T., and Yamada, S. (2023a). Influence of agent's self-disclosure on human empathy. PLoS ONE 18:e0283955. doi: 10.1371/journal.pone.0283955
Tsumura, T., and Yamada, S. (2023b). Influence of anthropomorphic agent on human empathy through games. IEEE Access 11, 40412–40429. doi: 10.1109/ACCESS.2023.3269301
Ullman, D., and Malle, B. F. (2019). “Measuring gains and losses in human-robot trust: Evidence for differentiable components of trust,” in 14th ACM/IEEE International Conference on Human-Robot Interaction (HRI) (Daegu: IEEE), 618–619. doi: 10.1109/HRI.2019.8673154
Keywords: human-agent interaction, empathy agent, empathy, trust, trust repair
Citation: Tsumura T and Yamada S (2024) Making a human's trust repair for an agent in a series of tasks through the agent's empathic behavior. Front. Comput. Sci. 6:1461131. doi: 10.3389/fcomp.2024.1461131
Received: 07 July 2024; Accepted: 16 October 2024;
Published: 05 November 2024.
Edited by:
Stefan Kopp, Bielefeld University, GermanyReviewed by:
Desrina Yusi Irawati, Universitas Katolik Darma Cendika, IndonesiaCopyright © 2024 Tsumura and Yamada. This is an open-access article distributed under the terms of the Creative Commons Attribution License (CC BY). The use, distribution or reproduction in other forums is permitted, provided the original author(s) and the copyright owner(s) are credited and that the original publication in this journal is cited, in accordance with accepted academic practice. No use, distribution or reproduction is permitted which does not comply with these terms.
*Correspondence: Takahiro Tsumura, dGFrYWhpcm8udHN1bXVyYUBpbmlhZC5vcmc=
Disclaimer: All claims expressed in this article are solely those of the authors and do not necessarily represent those of their affiliated organizations, or those of the publisher, the editors and the reviewers. Any product that may be evaluated in this article or claim that may be made by its manufacturer is not guaranteed or endorsed by the publisher.
Research integrity at Frontiers
Learn more about the work of our research integrity team to safeguard the quality of each article we publish.