- 1Department of Linguistics, University of Wisconsin–Milwaukee, Milwaukee, WI, United States
- 2Department of Linguistics, University of California, Davis, Davis, CA, United States
Voice-AI assistants offer innovative ways for people to interact with technology, such as delivering search results through human-sounding voices. Unlike printed text, however, voices are associated with particular characteristics, such as accents, which have the potential to influence perceived credibility. Voice-AI assistants are also a relatively new phenomenon, and while people who use them frequently may be inclined to trust them, other people may not be. The current study investigated how voice accent and frequency-of-use affected users’ credibility assessments of search results delivered by voice-AI assistants. Participants, who were native speakers of American English and self-classified themselves according to how frequently they used voice-AI assistants, listened to statements produced by neural text-to-speech in either an American English or British English accent. They then rated the credibility of both the information content and the voice itself, along several dimensions. Results showed that in multiple conditions, participants perceived information delivered by British-accented voices as more credible than that delivered by American English-accented voices. Furthermore, frequency-of-use exerted a significant effect on perceived trustworthiness of a voice. These findings have implications for the ethical design of voice-AI systems, and for human-computer interaction more generally.
1 Introduction
In the early 2000s, internet technology underwent a revolution, as search queries began providing results whose quality and relevance were unprecedented. Since then, high-tech companies and researchers have produced innovations of a different and even more palpable nature: instead of being constrained to text, we can now have the experience of searching the internet through the medium of the spoken voice. With voice-AI assistants such as Amazon Alexa or Google Home, users issue queries by speaking (e.g., “Alexa, what’s the weather today?”) and listen to search results delivered by synthetic speech (“Forty degrees and sunny”).
While this hands-free interface comes with increased convenience and intuitive functionality, its deeper implications for human communication and society remain unclear. Unlike type-written text, spoken voices have qualities that we associate with individual human beings. One of the most prominent qualities is accent. By definition, every voice possesses an accent, whether it is foreign, regional, or mainstream. These accents exert an influence on the listener: indeed, decades of research on language attitudes has shown that accents shape listeners’ impressions of speakers and also impact their decision-making (Giles and Billings, 2004). Thus, when a voice-AI assistant delivers search results, we know that the effect of the voice’s accent will not be neutral – and yet we still have not pinpointed what exactly that effect will be. This is the first question that the current study seeks to address: what effect does a voice-AI accent have on listeners’ judgments of the information delivered by the device?
According to the latest Smart Audio Report, 24% of people in the United States own a voice-AI assistant (The Smart Audio Report, 2020). While this is a robust number, it also means that many people – indeed, an overwhelming majority – have never used a virtual assistant, or have done so only infrequently. Previous research on why people use voice-AI assistants has identified a variety of different and sometimes unrelated motivations, but a common factor is social interaction. People use assistants because, in doing so, they experience the presence of another social entity. Indeed, at least one study has reported that social interaction is the key element in determining whether people develop a sense of trust in voice-AI assistants (Pitardi and Marriott, 2021). Importantly, those people who do not frequently use virtual assistants are lacking this element of social interaction, which may mean that they have not developed a sense of trust. Thus, infrequent users of voice-enabled devices may respond very differently to search results than frequent users do, which is the second question that the current study investigates.
1.1 Attitudes toward accents
Unlike type-written text, spoken language conveys an additional layer of social information. When we hear a voice, we associate it with a particular individual’s gender, age, social status, and accent. Previous research has already demonstrated that these factors can influence how users perceive information. Gaiser and Utz (2023) asked participants to assign credibility ratings to internet search results delivered by type-written text versus a voice-AI assistant. Some of the search results were highly accurate (e.g., “although e-cigarettes are less harmful than traditional cigarettes, they still contain carcinogenic substances”) while others were less accurate (e.g., “the risk associated with smoking a hookah is significantly reduced compared to smoking cigarettes”). The researchers found that participants rated results from the voice-AI assistant as significantly more credible than those from text. Furthermore, although high-accuracy statements were generally rated as more credible than low-accuracy statements, the difference between these two conditions was significantly reduced when the results were delivered by voice-AI, compared to text.
Dozens of studies have demonstrated that people express clear and consistent attitudes toward spoken stimuli (Giles and Billings, 2004). Thus, even though Gaiser and Utz (2023) did not report the gender, age, or accent of the German-speaking voice that they employed (other than that it was generated by a smart speaker), it seems highly likely that these characteristics impacted the participants’ credibility assessments. As early as the 1930’s, for example, research showed that listeners formed different perceptions of an individual based upon their particular accent of British English (Pear, 1931). More recent studies have explored a direct link between accent and credibility. Lev-Ari and Keysar (2010) asked American English-speaking participants to evaluate the truth value of statements such as “A giraffe can go without water longer than a camel can.” The statements were recorded by speakers with a strong foreign accent, with a mild foreign accent, or who were native speakers of American English. Results showed significantly higher truth ratings for statements delivered in the native accent, compared to either of the foreign accent conditions. Taylor (2015) extended this paradigm to regional accents. Results showed that New Zealand English-speaking participants rated information delivered by a New Zealand speaker as most credible, while information delivered by a Scottish speaker was rated as least credible. Together, these two studies suggest that voice characteristics exert a significant effect on users’ credibility judgments. Furthermore, these effects occur not just when the voice exhibits a foreign accent, but also when it exhibits a regional accent.
However, several other studies have employed similar designs with other regional accents, yet reported null results. For example, Wetzel et al. (2021) asked Swiss French-speaking participants to assess the credibility of statements delivered in Swiss French, Quebec French, German-accented French, and Finnish-accented French. Results showed no effect of accent. Similarly, when Barcelona Spanish-speaking participants listened to statements delivered in regional accents of Latin American Spanish (Frances et al., 2018), results showed no effect of accent. Most relevant to the current study, when American English-speaking participants listened to statements delivered in British or Southern United States accents, results also showed no effect of accent (Sarkis, 2015). Taken together, these findings certainly cast doubt on the notion that accents – or at least, regional accents delivered by a native speaker from another area – affect credibility judgments (see also Souza and Markman, 2013; Stocker, 2017).
Meanwhile, not all regional accents are the same. For speakers of California or midwestern American English, for example, both British and Southern U.S. accents might be classified as “regional,” yet they are clearly associated with different social statuses. Historically, British English has been considered a standard variety, where “standard” is associated with greater socio-economic status and wider media usage (Giles and Billings, 2004). This association was clearly reflected in language attitudes in the 1980s, when Stewart et al. (1985) reported that “received pronunciation” (RP) accents of British English received the highest favorability ratings in the English-speaking world, including among speakers in the U.S. More recently, however, Bayard et al. (2001) reported that American accents were perceived as most favorable, and concluded that “the American accent seems well on the way to equaling or even replacing RP as the prestige – or at least preferred – variety” (p. 22).
However, this conclusion is far from settled. In a follow-up study that adopted different methods from those used by Bayard et al. (2001), Garrett et al. (2005) reported that American participants overwhelmingly associate British English with positively “cultured” adjectives such as intelligent, refined, and well-spoken, although these results were tempered by associations indicating negative affect, such as snobbish. More recently, Wolfram and Schilling (2016) argued that North Americans continue to place value on British accents, speculating that this may be due to “a lingering colonial effect” (p. 34). Meanwhile, van den Doel (2006) has shown that U.S.-based listeners rated pronunciation errors (such as wea[d]er for weather) very differently when they occurred in a British accent, compared to in an American accent. Altogether, then, we have plausible reason to believe that American attitudes about British English remain complex, and may manifest themselves in judgments about linguistic content. For this reason, American and British accents are the focus of the current study.
While the results for accents in human voices are decidedly mixed and potentially changing over time, synthetic voices – and voice-AI assistants in particular – present a fresh set of questions. Unlike human beings, who communicate for a wide variety of reasons, voice-AI assistants are commercial products with a circumscribed set of objectives. In addition to returning search results, for example, they could also be used to collect data about users and encourage them to purchase products. Research strongly suggests that users are aware of these practices and modulate their attitudes toward voice-AI assistants accordingly (McLean and Osei-Frimpong, 2019; Buteau and Lee, 2021; Pitardi and Marriott, 2021). Because of these differences, it is an open question as to whether users will respond to accented voice-AI assistants in a manner similar to what has been previously reported for accented human voices. A primary goal of the current study is to shed light on this question.
1.2 Choosing to use a voice-AI assistant
Although the use of voice-AI assistants has increased greatly in recent years, the majority of the population still does not use them on a regular basis. Those people who do use assistants are presumably driven by particular attitudes and motivations, which have been the subject of several recent studies. These studies vary widely in terms of their variables and participant pools, but their findings nevertheless provide some clues as to which factors might help differentiate frequent versus infrequent users.
Some of these studies examined general attitudes. Buteau and Lee (2021) conducted an online survey of 558 people. Results showed that the factors of perceived usefulness, perceived security, and personal norms had a positive relationship with attitudes toward voice-AI assistants. Perceived ease-of-use had a non-significant effect, which is notable because this has historically been an important factor in human attitudes toward technology. Note that in this study, participants’ use (or non-use) of assistants was not verified. Shao and Kwon (2021) conducted an online survey of 247 people who were verified users of assistants. Results showed that dynamic control, functional utility, and social presence were all determiners of satisfaction. (Both of these studies were conducted by researchers based in the U.S., although the location of residence for the participants was not reported).
Other studies have focused more precisely on motivations. Choi and Drumwright (2021) conducted an online survey of 256 people in the southeastern U.S. who were verified users of voice-AI assistants. Results revealed five primary motivations for using assistants: life efficiency, information, conformity, personal identity, and social interaction. Interestingly, only those users who were motivated by information were likely to consider the assistant to be a form of technology; meanwhile, those users who were motivated by social interaction were more likely to perceive the assistant as a friend and socially attractive. McLean and Osei-Frimpong (2019) surveyed 724 people in the United Kingdom who had used Amazon Echo for at least 1 month. Results showed that people were motivated to use assistants for utilitarian, symbolic, and social benefits. Social benefits referred to users’ perception that they were in the presence of another social entity (e.g., “When I interact with a voice assistant it feels like someone is present in the room”).
At least one study has focused specifically on trust, which is closely related to the current study’s focus on credibility assessments. Pitardi and Marriott (2021) surveyed 466 people in the United Kingdom who had at least some experience using voice-AI assistants. Results showed that, among the many different variables examined, social cognition and social presence were the unique antecedents for developing trust. Social cognition refers to attributes of warmth and competence (“I think my assistant is helpful”). As in previous studies, social presence refers to the perception of being in the presence of a social entity (“When I interact with my assistant I feel there is a sense of human contact”).
While it is difficult to generalize across studies that employed such different variables of analysis, one common theme is social interaction. That is, to a degree, people seem to use voice-AI assistants because doing so conjures certain aspects of communication with real humans. As McLean and Osei-Frimpong (2019) point out, the spoken conversations between people and assistants provide a human-like attribute, which encourages users to engage with voice-AI in the same way as they would with humans. Furthermore, as Pitardi and Marriott (2021) have shown, such experiences are a crucial component toward the development of trust.
Tying all of this together, we return to our question of what might differentiate frequent users of voice-AI assistants from infrequent users. It seems reasonable to suppose that infrequent users, lacking the social interactions that frequent users have experienced, may be less willing to trust the information that assistants provide, and a second goal of the current study is to examine this issue.
1.3 The current study
The current study investigates how voice accent and frequency-of-use affect users’ credibility assessments of the search results delivered by voice-AI assistants. Following the design of Gaiser and Utz (2023), participants listened to statements that were either of high accuracy (e.g., although e-cigarettes are less harmful than traditional cigarettes, they still contain carcinogenic substances) or low accuracy (e.g., the risk associated with smoking a hookah is significantly reduced compared to smoking cigarettes). The statements were produced by neural text-to-speech in either an American English or British English accent. Participants, who were native speakers of American English and self-classified themselves according to how frequently they used voice-AI assistants, gave ratings to each statement, assessing the credibility of both (a) the actual information and (b) the voice that delivered the information.
We hypothesize that participants will assign higher credibility ratings to statements produced in British English, compared to American English. As discussed above, there is credible evidence that Americans still assign prestige to British English in its human form, and we hypothesize that they will transfer this attitude to voice-AI. Furthermore, we also anticipate that participants will perceive smaller differences in credibility for high- versus low-accuracy statements produced by British accents, compared to American accents. This is based upon the findings of Gaiser and Utz (2023), where an overall effect for voice-AI versus text statements was accompanied by a significant interaction with accuracy. Finally, in light of evidence suggesting that infrequent users of voice-AI are less likely to trust the technology, we hypothesize that participants who are frequent users will assign overall higher credibility ratings than those who are infrequent users.
To preview, the results partially confirmed these hypotheses. In multiple conditions, participants perceived information delivered by British-accented voices as more credible than that delivered by American English-accented voices. Furthermore, frequency-of-use exerted a significant effect on perceived trustworthiness of a voice.
2 Methods
2.1 Stimulus materials
Following Gaiser and Utz (2023), we developed stimuli using information-seeking questions that users might pose to a search engine, such as How dangerous is smoking e-cigarettes compared to cigarettes? For each question, we generated a high-accuracy response that contained fully correct information (i.e., although e-cigarettes are less harmful than traditional cigarettes, they still contain carcinogenic substances) and a low-accuracy response that contained some incorrect information (e.g., the risk associated with smoking a hookah is significantly reduced compared to smoking cigarettes). We used eight different questions, for a total of 16 responses. Six of the responses were adapted from those used by Gaiser and Utz (2023), though we modified the lower-accuracy statements to contain even less accurate statements. The complete list of questions and responses are provided in the Supplementary Material.
For each statement, we generated recordings in eight different text-to-speech (TTS) voices using Amazon Web Services (AWS) Polly in neural TTS. Four of the voices had North American accents (designated as “U.S.”: Salli, Kimberly, Stephen, Matthew) and four of the voices had British accents (designated as “U.K.”: Emma, Amy, Arthur, Brian). Each statement was downloaded from the AWS console as an individual sound file, then amplitude normalized to 65 dB.
2.2 Participants
One hundred ninety-nine native speakers of American English (153 female, 0 non-binary/gender non-conforming, 46 male, mean age = 19.7 years old, age range 18–37) completed the experiment online via a Qualtrics survey. Participants were recruited from the UC Davis psychology subject pool and given partial course credit. This study was approved by the UC Davis Institutional Review Board and all participants completed informed consent. Participants were instructed to complete the experiment in a quiet room without distractions or noise, to silence their phones, and to wear headphones. None of the listeners reported having a hearing or language impairment.
2.3 Procedure
Participants were randomly assigned to an experimental list in which each of the eight voices was randomly assigned to a statement. Within each list, there were four high-accuracy statements and four low-accuracy statements. Accent was equally balanced between high- and low-accuracy statements, as was gender. Each participant rated a total of eight statements.
The experiment began with an audio check. Participants heard a spoken sentence (Lubricate the car with grease) and were asked to identify it from among three options, each containing phonologically similar words (Activate the car with keys, Navigate the car through streets, Lubricate the car with grease). All participants passed the check.
Next, we informed participants that they would be presented with spoken internet search results from a new smart speaker application, called SearchBot. We informed them that they would separately evaluate the information and the voice of each statement.
For each trial, the search question was displayed on the screen, e.g., You are hearing the SearchBot’s response to the following question: How dangerous is smoking e-cigarettes compared to cigarettes? Next, participants heard one of the voices produce a statement in response, once only with no option to repeat.
The participant’s task was to provide ratings. Three of the ratings focused on the information content of the statement: How {accurate | believable | authentic} is the information you just heard? Five of the ratings focused on the voice that delivered the statement: How {accurate | believable | trustworthy | competent | biased} is the voice assistant you just heard? Each rating was on a slider scale from 1 to 100, with the guidelines that 1 = describes it very badly, 100 = describes it very well.
We used previous research as a framework for selecting these adjectives, relying principally on Gaiser and Utz (2023) as well as Appelman and Sundar (2016), who asked participants to rate internet-search statements using the words “authentic,” “believable,” and “accurate.” In our study, we employed these same three adjectives for the information content, and also extended them to ratings for the voice itself. In addition, prior work investigated how the adjectives “trustworthy” and “biased” apply to AI agents (Waytz et al., 2014), and these words were therefore relevant for our investigation of voice-based AI agents. Finally, previous investigations of machine versus human interlocutors (Cowan et al., 2015), as well as studies of different AI voices (Ernst and Herm-Stapelberg, 2020), have employed the concept of “competence,” motivating its inclusion in the current study.
Finally, participants were asked: How often do you use voice-activated digital assistants (e.g., Apple’s Siri, Amazon’s Alexa, Google Assistant, Cortana)? The response options were: Never, Rarely, Once a month, Weekly, Daily.
3 Results
Participants’ responses to the usage question were coded binarily as either Frequent device usage (daily, weekly, monthly; n = 82) or Infrequent device usage (never, rarely; n = 117). The responses to each of the eight rating questions were analyzed with separate mixed effects linear regression models using the lmer function in the lme4 R package (Bates et al., 2015). Each model included fixed effects of Accuracy Level (higher vs. lower), Speaker Accent (British vs. American), and Listener Device Usage Frequency (Infrequent vs. Frequent) and as well as all possible two- and three-way interactions. Fixed effects were sum-coded.
We first fit models with maximal random effects structure, including random intercepts for topic (i.e., one of eight search queries; see the Supplementary Material), speaker (i.e., one of the eight voice options provided by AWS Polly, such as Salli, Kimberly, etc.), and participant, as well as by-participant random slopes for all the fixed effects and the interactions between them. If this resulted in a singularity error (indicating overfitting of the random effects), then the random effects structure was simplified by removing those predictors which accounted for the least amount of variance until the model fit, following Barr et al. (2013). The retained lmer syntax of all the models was the same: Rating ~ Accuracy Level * Speaker Accent * Usage Frequency + (1 + Accuracy Level + Speaker Accent | Participant) + (1 | Speaker) + (1 | Topic).
To explore significant interactions, we performed post hoc Tukey’s HSD pairwise comparisons using the emmeans() function in the emmeans R package (Lenth et al., 2021).
3.1 Ratings of the statement’s information content
The descriptive results for participants’ ratings for information content are displayed in Table 1, and discussed individually in subsequent sections.
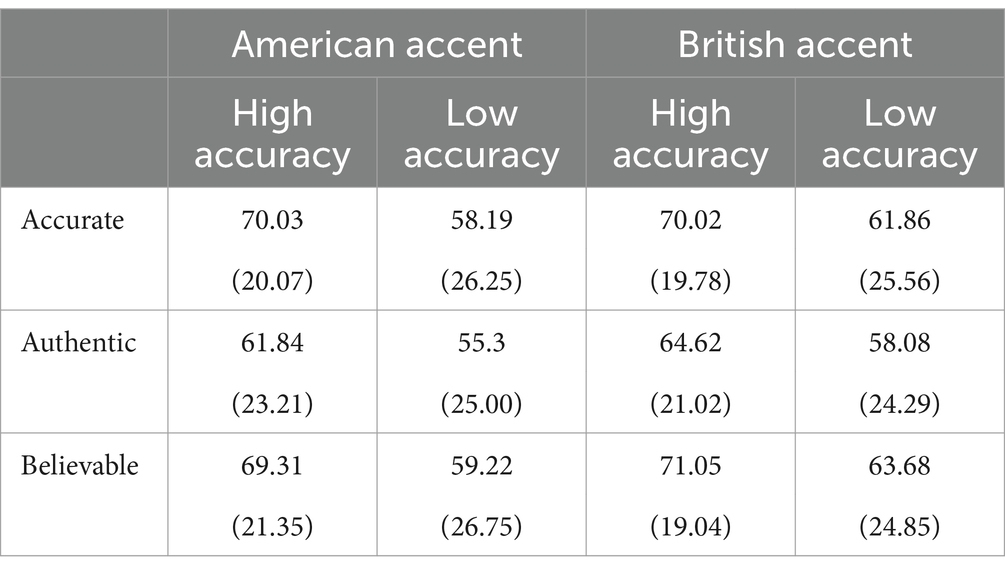
Table 1. Means (standard deviations) of participant ratings for information content, based on a scale from 1 to 100.
3.1.1 Accuracy
For Accuracy ratings, there was a significant effect of statement Accuracy Level, such that high-accuracy statements were rated as more accurate than low-accuracy statements (coef. = 5.02, SE = 0.6, t = 8.5, p < 0.001).
The interaction between Accuracy Level and Voice Accent was also significant (coef. = −0.97, SE = 1.02, t = −2.1, p < 0.05). This is depicted in Figure 1. Low- versus high-accuracy statements received significantly different ratings for accuracy when they were delivered in a British English accent (coef. = 8.1, SE = 1.5, t = 5.4, p < 0.001), but the difference in ratings was even larger when they were delivered in an American English accent (coef. = 12.0, SE = 1.5, t = 7.9, p < 0.001).
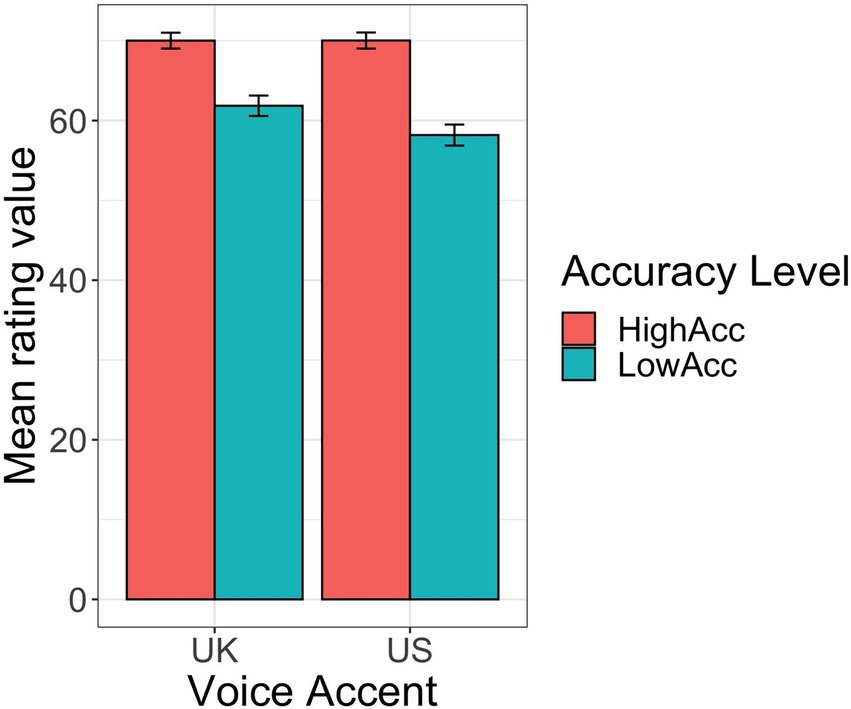
Figure 1. Results for participants’ ratings of statement accuracy, by voice accent (U.K. vs. U.S.) and statement accuracy level (Higher vs. Lower accuracy).
There was also a significant interaction between Voice Accent and Listener Usage Frequency (coef. = −1.1, SE = 0.5, t = −2.3, p < 0.05). This is depicted in Figure 2. Infrequent users of voice-AI rated British English statements as more accurate than American English statements (coef. = 3.7, SE = 1.5, t = 2.4, p < 0.05), but Frequent users of voice-AI exhibited no difference (p = 0.7).
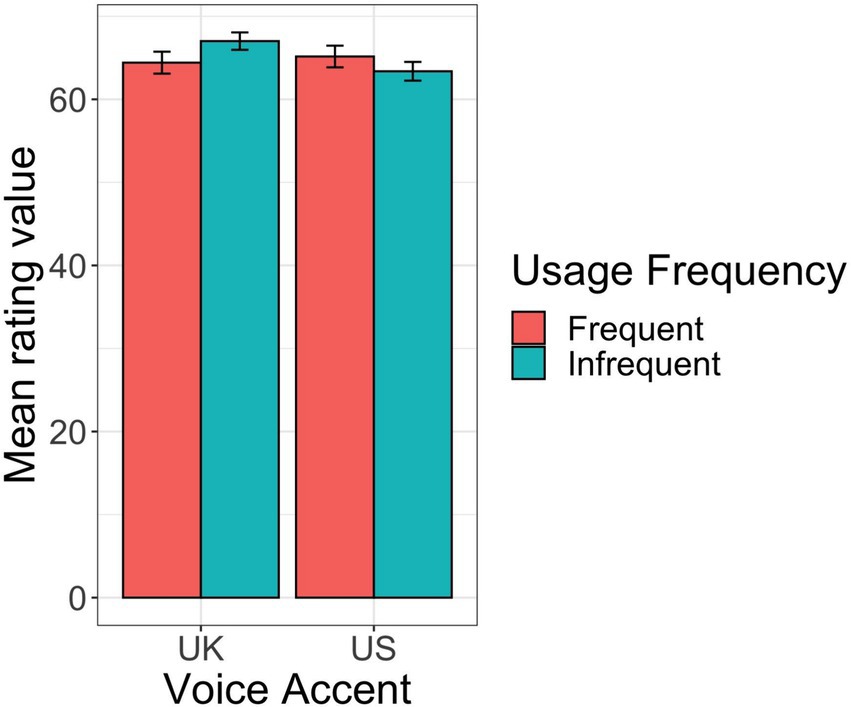
Figure 2. Results for participants’ ratings of statement accuracy, by voice accent (U.K. vs. U.S.) and participants’ reported Usage Frequency (Frequent vs. Infrequent device usage).
No other effects were significant predictors of Accuracy.
3.1.2 Believability
For believability ratings, there was a significant effect of statement Accuracy Level, such that high-accuracy statements were rated as more believable than low-accuracy statements (coef. = 4.4, SE = 0.6, t = 7.4, p < 0.001).
There was a significant interaction between Voice Accent and Listener Usage Frequency (coef. = −1.01, SE = 0.5, t = −2.0, p < 0.05). The pattern was similar to that found for accuracy ratings. Infrequent users of voice-AI rated British English statements as more believable than American English statements (coef. = 3.7, SE = 1.6, t = 2.4, p < 0.05), but Frequent users of voice-AI exhibited no difference (p = 0.7).
No other effects were significant predictors of Believability.
3.1.3 Authenticity
For authenticity ratings, there was a significant effect of statement Accuracy Level, such that high-accuracy statements were rated as more authentic than low-accuracy statements (coef. = 3.2, SE = 0.6, t = 5.7, p < 0.001). No other effects or interactions were significant.
3.2 Ratings of the voice that delivered the statement
The descriptive results for participants’ ratings for voices are displayed in Table 2, and discussed individually in subsequent sections.
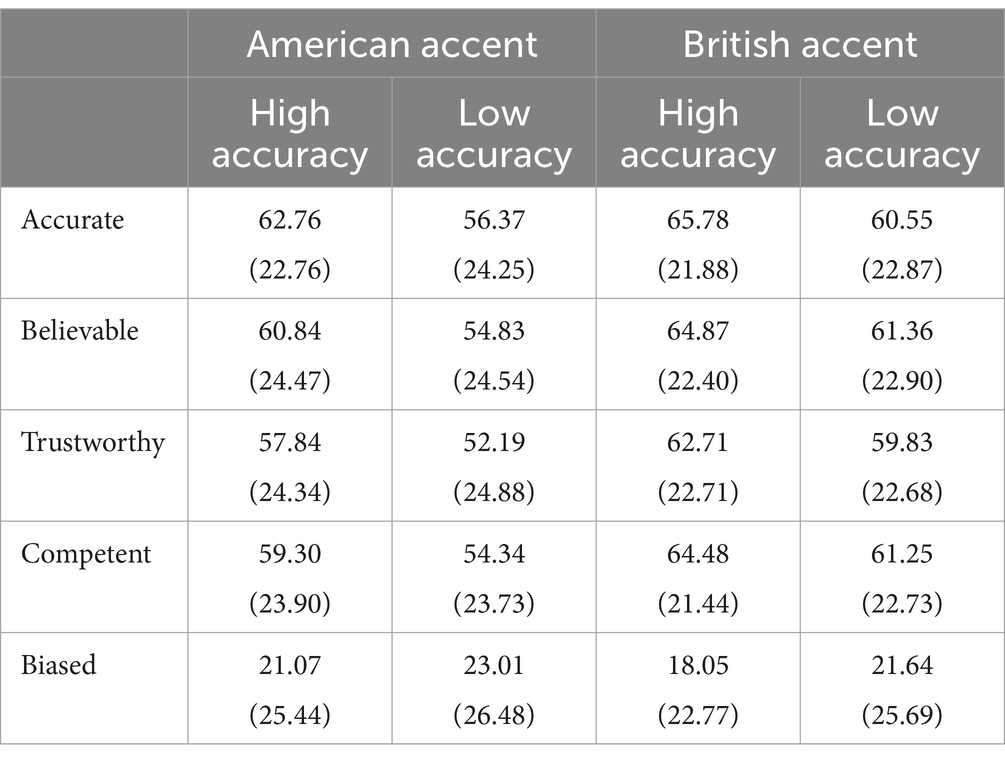
Table 2. Means (standard deviations) of participant ratings for voices, based on a scale from 1 to 100.
3.2.1 Accuracy, believability, competence, and bias
For all four of these outcome variables, there was a significant effect of statement Accuracy Level. The voices for high-accuracy statements were rated as more accurate (coef. = 2.9, SE = 0.5, t = 5.7, p < 0.001), believable, (coef. = 2.3, SE = 0.5, t = 4.5, p < 0.001) and competent (coef. = 2.0, SE = 0.5, t = 3.9, p < 0.001) than the voices for low-accuracy statements. Meanwhile, the voices for high-accuracy statements were rated as less biased (coef. = −1.4, SE = 0.5, t = −2.9, p < 0.01) than the voices for low accuracy statements. No other effects or interactions were significant.
3.2.2 Trustworthiness
For trustworthiness ratings, there was a significant effect of statement Accuracy Level, such that high-accuracy statements were rated as more trustworthy than low-accuracy statements (coef. = 2.1, SE = 0.5, t = 3.8, p < 0.001).
There was also a significant effect of Listener Device Usage Frequency, such that Frequent users of voice-AI rated the voices as more trustworthy than infrequent users (coef. = 2.02, SE = 1.0, t = 2.0, p < 0.05). This is shown in Figure 3. No other effects or interactions were significant.
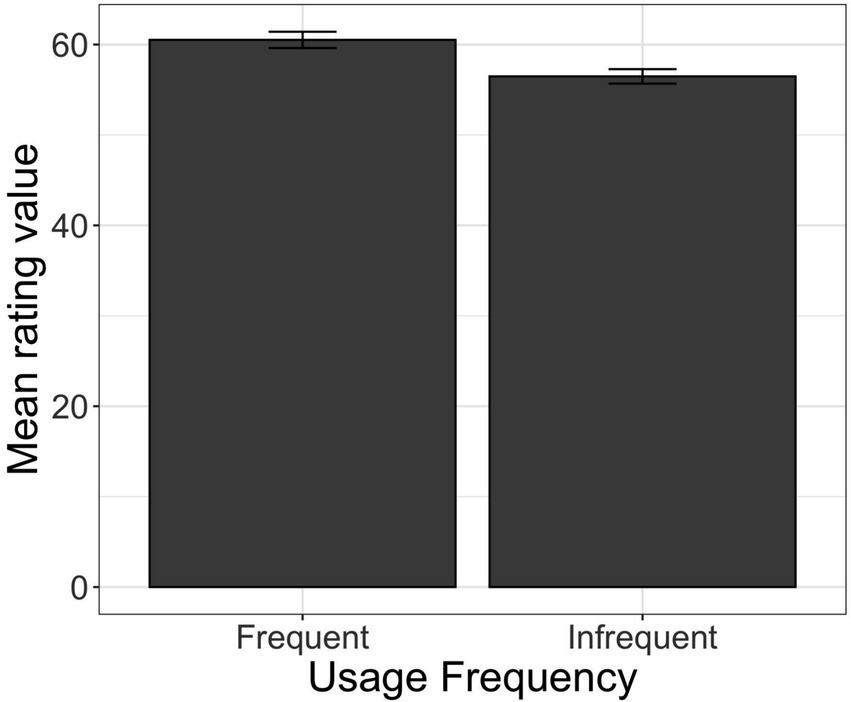
Figure 3. Results for participants’ ratings of voice assistant trustworthiness by participants’ reported Usage Frequency (Frequent vs. Infrequent device usage).
4 Discussion
We had hypothesized that (a) participants would assign higher ratings to British English statements, compared to American English statements (b) participants would be less sensitive to high- versus low-accuracy statements for British English compared to American and (c) frequent users of voice-AI would assign higher ratings than infrequent users.
Our results partially confirmed these hypotheses and revealed several key findings. First, the actual accuracy of statements had a significant effect on all three ratings for information (accuracy, believability, and authenticity) and on all five ratings for voice (accuracy, believability, competency, trustworthiness, and bias). Second, low- versus high-accuracy statements received different ratings for information accuracy when they were delivered in an American English accent, but there was a significantly smaller difference in ratings when they were delivered in a British English accent. Third, frequency of use had a significant effect on ratings for voice trustworthiness. Finally, infrequent users of voice-AI rated British English statements as more accurate and believable than American English statements, but frequent users of voice-AI exhibited no difference. In the sections that follow, we discuss each of these findings in turn.
4.1 Effect of message accuracy
The actual accuracy of statements had a significant effect on all three ratings for information content, such that high-accuracy statements received higher ratings on accuracy, believability, and authenticity than low-accuracy statements. This result is entirely expected, and demonstrates that our implementation of Gaiser and Utz’s (2023) manipulation was effective.
In addition, the actual accuracy of statements also had a significant effect on all five ratings for voice, such that high-accuracy statements received higher ratings for voice accuracy, believability, competency, trustworthiness, and lower ratings for bias, than low-accuracy statements. Overall, then, participants were more likely to rate a voice favorably when it delivered a high-accuracy statement such as “although e-cigarettes are less harmful than traditional cigarettes, they still contain carcinogenic substances,” compared to a low-accuracy statement such as “the risk associated with smoking a hookah is significantly reduced compared to smoking cigarettes.” This result is somewhat more surprising, because it indicates that information content can affect judgments about a particular speaker’s voice.
4.2 Effect of accents on information accuracy ratings
Even though participants generally rated high- versus low-accuracy statements in a veridical manner, this effect was modulated by accent. Specifically, when listening to American English accents, participants remained sensitive to the actual accuracy of the statements, giving higher information accuracy ratings to high-accuracy statements and lower ratings to low-accuracy statements. When listening to British English accents, however, this sensitivity diminished, and participants gave more similar ratings, regardless of actual accuracy. This interaction is similar to that reported by Gaiser and Utz (2023), who found that the difference between high- versus low-accuracy conditions was significantly reduced when search results were delivered by voice-AI, compared to text. In our case, the difference between conditions was reduced when results were delivered in a British accent, compared to an American one.
In this regard, our results are consistent with the most basic conclusion of Lev-Ari and Keysar (2010) and Taylor (2015), namely, that accents can exert an effect on credibility judgments. Crucially, however, our findings trend in the opposite direction. That is, Lev-Ari and Keysar (2010) and Taylor (2015) both reported a disadvantage for statements produced with an accent different from the listeners’ native accent, and argued that this effect was due strictly to comprehensibility, not social perceptions (in this case, negative perceptions). By contrast, the current study demonstrates an advantage for statements produced with a different accent, which suggests that social perceptions (in this case, positive perceptions) may be sufficient to override any difficulties in comprehensibility (cf. Lorenzoni et al., 2022 who also report an advantage for statements delivered by foreigners), at least in those cases when the accent is delivered by voice-AI.
At the same time, our work seems to contradict previous findings that had focused specifically on social perceptions of British English as it is spoken by humans. For example, Sarkis (2015) found that a British English accent exerted no effect on credibility judgments made by American English participants, while Bayard et al. (2001) reported that American accents were replacing British in terms of prestige. As we noted in Section 1.1, however, it is difficult to disentangle all of the factors influencing American attitudes toward British English, and there is still evidence that Americans view British English as culturally prestigious. The rapid adoption of voice-AI complicates the situation further. One intriguing possibility is that people judge British English differently when it is delivered by a real human voice, compared to when it is delivered by voice-AI. Such a scenario would have implications for theories of human-computer interaction (HCI), and could be pursued in a future study that directly compares credibility judgments for real voices versus AI voices (cf. Zellou et al., 2023).
Our results show that a regional accent possesses the power to diminish participants’ sensitivity to accuracy, which has important consequences for our understanding of humans and AI. It suggests that people’s credibility judgments can be easily manipulated – and moreover, they can be manipulated by a factor that is relatively new to the internet ecosystem (namely, voices), which is completely independent from the content of the statement itself.
4.3 Effect of voice-AI usage
Frequency of use had a significant effect on ratings for voice trustworthiness. Specifically, participants who frequently use voice-AI rated the voices as more trustworthy than participants who use it rarely or never. This confirms the speculation that we put forth in the Introduction, namely that because infrequent users of voice-AI have not experienced its human-like attributes and have not interacted with these devices in a social manner, they will be less likely to trust those voices.
This result is important because it shows that not all technology users are alike. In the human realm, almost all people have experiences with evaluating the trustworthiness of voices. When this same experience is transferred to the digital realm, however, people’s evaluations diverge on the basis of their technology usage.
4.4 Effect of voice-AI usage on accent credibility
Frequency of voice-AI usage also affected how participants judged accents. Infrequent users of voice-AI rated British English statements as overall more accurate and believable than American English statements. However, frequent users of voice-AI did not rate the two accents differently. This result provides additional confirmation for the conclusion that not all technology users are alike.
“Routinized” theories of HCI account for such differences by proposing that infrequent usage leads people to transfer their human-based scripts to devices, while more frequent usage leads people to develop device-specific scripts (Gambino et al., 2020). If that scenario applies to the current study, the infrequent users would presumably be following a human-based script whereby British English statements are more accurate and believable than American English statements. However, previous literature does give some reason to doubt the existence of such a script. Although the British RP accent received the highest possible favorability ratings in the mid-1980s (Stewart et al., 1985), more recent work suggests that the American accent has taken over this position of prestige (Bayard et al., 2001). It is therefore not clear if routinized theories of HCI can account for our results.
4.5 Limitations
As stimuli, this study used a total of sixteen statements (8 topics × 2 accuracy levels). This is an increase compared to Gaiser and Utz (2023), who used 12 statements (6 topics × 2 accuracy levels), although the relatively small number does limit the generalizability of our findings. From one topic to the next, the inaccurate statements may exhibit some variation in the number of detectable errors. For example, the mean accuracy ratings for information content ranged from 51.82 for Topic 4 to 70.95 for Topic 3, suggesting that listeners might have detected more errors in the inaccurate statement for Topic 4, compared to Topic 3. However, any such item effects would have been mitigated by the use of counter-balanced lists, as described in Section 2.3, and by the inclusion of Topic in the random-effects structure of our statistical models, as described in Section 3. Future work with a larger number of topics and pre-normed statements could address these limitations.
Participants indicated the frequency of their device usage in a gradient manner (daily, weekly, monthly, rarely, and never), which we coded into two binary categories, Frequent versus Infrequent. This approach can lead to a loss of power and increase the potential of Type I errors, but we used it for a couple of reasons. First, because our experimental design contains several other predictor variables, with the potential for many different interactions, the use of a binary predictor helped facilitate the interpretation of our results. Second, to our knowledge, previous work has not examined frequency of usage; indeed, the studies reviewed in Section 1.2 simply excluded participants who had never used voice-AI. Because the current study is presumably one of the first to examine this issue, we decided to pursue a very simple hypothesis in binary terms. Now that we have established the preliminary finding that frequency of use does indeed affect judgments of trustworthiness, future work can examine this variable in a more nuanced manner.
The adjectives that we employed, such as “authentic,” are open to interpretation, and we did not explicitly train our participants about how to respond to them. Thus it is possible that, from one participant to the next, somewhat different criteria were used to assign ratings. One advantage of this approach, however, is that each participant could assess what made a particular statement sound authentic (or biased, etc.) to them, which is appropriate for a study whose overall goal was to assess the credibility, broadly construed, of internet search results.
Some of the adjectives, such as “believable” and “trustworthy,” have similar definitions; meanwhile, two of the adjectives, “accurate” and “believable,” were assessed for both information content as well as for the voice itself. It is plausible that this situation produced correlations among different rating categories. Although these correlations may be examined in future work, we have not done so in the current study, because our goal was not to assess how accent and usage affected, say, the notion of believability independently of the notion of trustworthiness. Rather, our goal was to assess how accent and usage affected credibility as a whole, and the use of overlapping adjectives allowed us to characterize this concept to the fullest extent possible.
For any given statement, we did not explicitly provide participants with information about which accent they were listening to, nor did we ask whether they were aware that the statement was delivered in an American accent or a British accent. It seems unlikely that a native speaker of American English would fail to detect the presence of a British accent, although it is certainly plausible that participants had different levels of conscious awareness about it, and that these differences could affect their credibility ratings. This issue could be explored in future work. Meanwhile, participants who were already frequent users of voice-AI could theoretically do so using any accent of English, which may have affected their ratings in the current study. Although it seems unlikely that our American participants would choose British English for their regular personal use of voice-AI, it is nevertheless possible; it is also possible that some participants regularly use voice-AI in a language other than English. A future study can collect this data from participants and assess its influence.
5 Conclusion
Internet search has been part of the modern world for quite some time. It is only in recent years, however, that the ability to make and receive search results through the mechanism of the human voice has become widespread. The implications of this change are potentially enormous, because voices are not neutral: they carry information about gender, age, social status, and accent. We have shown that one of these characteristics – namely, accent – can alter how people judge the credibility of the information they receive. It seems entirely plausible that future work will demonstrate that other voice characteristics exert similar impacts, and these findings have crucial implications for the ethical development of voice-AI technology.
While millions of people have participated in the technological revolution of voice-AI, millions of others have not. We have shown that this divide is real: people treat voices very differently when they are frequent users of voice-AI, compared to when they are not. This finding suggests that an individual’s experiences may largely depend upon the extent to which they are willing – or not – to interact with new devices in a social manner. As our modern world struggles with novel questions about the veracity of our information, understanding this digital divide has never been more important.
Data availability statement
The raw data supporting the conclusions of this article will be made available by the authors, without undue reservation.
Ethics statement
The studies involving humans were approved by UC Davis Institutional Review Board. The studies were conducted in accordance with the local legislation and institutional requirements. The participants provided their written informed consent to participate in this study.
Author contributions
AP: Conceptualization, Writing – original draft, Writing – review & editing. GZ: Conceptualization, Formal analysis, Writing – original draft, Writing – review & editing.
Funding
The author(s) declare that no financial support was received for the research, authorship, and/or publication of this article.
Conflict of interest
The authors declare that the research was conducted in the absence of any commercial or financial relationships that could be construed as a potential conflict of interest.
Publisher's note
All claims expressed in this article are solely those of the authors and do not necessarily represent those of their affiliated organizations, or those of the publisher, the editors and the reviewers. Any product that may be evaluated in this article, or claim that may be made by its manufacturer, is not guaranteed or endorsed by the publisher.
Supplementary material
The Supplementary material for this article can be found online at: https://www.frontiersin.org/articles/10.3389/fcomp.2024.1411414/full#supplementary-material
References
Appelman, A., and Sundar, S. S. (2016). Measuring message credibility: construction and validation of an exclusive scale. J. Mass Commun. Quart. 93, 59–79. doi: 10.1177/1077699015606057
Barr, D. J., Levy, R., Scheepers, C., and Tily, H. J. (2013). Random effects structure for confirmatory hypothesis testing: keep it maximal. J. Mem. Lang. 68, 255–278. doi: 10.1016/j.jml.2012.11.001
Bates, D., Maechler, M., Bolker, B., Walker, S., Christensen, R. H. B., Singmann, H., et al. (2015). Package ‘lme4’. [Computer software].
Bayard, D., Weatherall, A., Gallois, C., and Pittam, J. (2001). Pax Americana? Accent attitudinal evaluations in New Zealand, Australia and America. J. Socioling. Malden, Massachusetts: Blackwell Publishers, 5, 22–49. doi: 10.1111/1467-9481.00136
Buteau, E., and Lee, J. (2021). Hey Alexa, why do we use voice assistants? The driving factors of voice assistant technology use. Commun. Res. Rep. 38, 336–345. doi: 10.1080/08824096.2021.1980380
Choi, T. R., and Drumwright, M. E. (2021). “OK, Google, why do I use you?” motivations, post-consumption evaluations, and perceptions of voice AI assistants. Telematics Inform. 62:101628. doi: 10.1016/j.tele.2021.101628
Cowan, B. R., Branigan, H. P., Obregón, M., Bugis, E., and Beale, R. (2015). Voice anthropomorphism, interlocutor modelling and alignment effects on syntactic choices in human−computer dialogue. Int. J. Hum. Comp. Stud. 83, 27–42. doi: 10.1016/j.ijhcs.2015.05.008
Ernst, C.-P., and Herm-Stapelberg, N.. (2020). Gender Stereotyping’s influence on the perceived competence of Siri and co. Proceedings of the 53rd Hawaii international conference on system sciences, Manoa, HI.
Frances, C., Costa, A., and Baus, C. (2018). On the effects of regional accents on memory and credibility. Acta Psychol. 186, 63–70. doi: 10.1016/j.actpsy.2018.04.003
Gaiser, F., and Utz, S. (2023). Is hearing really believing? The importance of modality for perceived message credibility during information search with smart speakers. J. Media Psychol. 36:93–106. doi: 10.1027/1864-1105/a000384
Gambino, A., Fox, J., and Ratan, R. A. (2020). Building a stronger CASA: extending the computers are social actors paradigm. Hum. Mach. Commun. 1, 71–85. doi: 10.3316/INFORMIT.097034846749023
Garrett, P., Williams, A., and Evans, B. (2005). Attitudinal data from New Zealand, Australia, the USA and UK about each other’s Englishes: recent changes or consequences of methodologies? Multilingua 24, 211–235. doi: 10.1515/mult.2005.24.3.211
Giles, H., and Billings, A. C. (2004). “Assessing language attitudes: speaker evaluation studies” in The handbook of applied linguistics. Eds. Davies, Alan and Elder, Catherine. (Malden, Massachusetts: John Wiley & Sons, Ltd.) 187–209.
Lenth, R., Singmann, H., Love, J., Buerkner, P., and Herve, M. (2021). Emmeans: Estimated marginal means, aka least-squares means (R package version 1.5. 1.) [Computer software]. The Comprehensive R Archive Network. Available at: https://CRAN.R-Project.Org/Package=Emmeans (Accessed September 27, 2022).
Lev-Ari, S., and Keysar, B. (2010). Why don’t we believe non-native speakers? The influence of accent on credibility. J. Exp. Soc. Psychol. 46, 1093–1096. doi: 10.1016/j.jesp.2010.05.025
Lorenzoni, A., Pagliarini, E., Vespignani, F., and Navarrete, E. (2022). Pragmatic and knowledge range lenience towards foreigners. Acta Psychol. 226:103572. doi: 10.1016/j.actpsy.2022.103572
McLean, G., and Osei-Frimpong, K. (2019). Hey Alexa … examine the variables influencing the use of artificial intelligent in-home voice assistants. Comput. Hum. Behav. 99, 28–37. doi: 10.1016/j.chb.2019.05.009
Pitardi, V., and Marriott, H. R. (2021). Alexa, she’s not human but… unveiling the drivers of consumers’ trust in voice-based artificial intelligence. Psychol. Mark. 38, 626–642. doi: 10.1002/mar.21457
Sarkis, J. (2015). The effect of sociolinguistic accent on the believability of trivia statements. Ann Arbor, Michigan: University of Michigan.
Shao, C., and Kwon, K. H. (2021). Hello Alexa! Exploring effects of motivational factors and social presence on satisfaction with artificial intelligence-enabled gadgets. Hum. Behav. Emerg. Technol. 3, 978–988. doi: 10.1002/hbe2.293
Souza, A. L., and Markman, A. B. (2013). Foreign accent does not influence cognitive judgments. Proceedings of the Annual Meeting of the Cognitive Science Society, 35. Available at: https://escholarship.org/content/qt8hd9v4ff/qt8hd9v4ff.pdf
Stewart, M. A., Ryan, E. B., and Giles, H. (1985). Accent and social class effects on status and solidarity evaluations. Personal. Soc. Psychol. Bull. 11, 98–105. doi: 10.1177/0146167285111009
Stocker, L. (2017). The impact of foreign accent on credibility: an analysis of cognitive statement ratings in a Swiss context. J. Psycholinguist. Res. 46, 617–628. doi: 10.1007/s10936-016-9455-x
Taylor, N. (2015). The influence of accent on perceptions of credibility. Christchurch, New Zealand: University of Canterbury.
The Smart Audio Report . (2020). National public media. Available at: https://www.nationalpublicmedia.com/insights/reports/smart-audioreport/
van den Doel, R. (2006). How friendly are the natives? An evaluation of native-speaker judgements of foreign-accented British and American English. Utrecht, Netherlands: Netherlands Graduate School of Linguistics (LOT).
Waytz, A., Heafner, J., and Epley, N. (2014). The mind in the machine: anthropomorphism increases trust in an autonomous vehicle. J. Exp. Soc. Psychol. 52, 113–117. doi: 10.1016/j.jesp.2014.01.005
Wetzel, M., Zufferey, S., and Gygax, P. (2021). Do non-native and unfamiliar accents sound less credible? An examination of the processing fluency hypothesis. J. Article Support Null Hypo. 17:61–69.
Wolfram, W., and Schilling, N. (2016). American English: Dialects and variation. 3rd Edn. Malden, Massachusetts: Wiley-Blackwell.
Keywords: voice-AI, speech perception, accents and dialects, trustworthy AI, credibility
Citation: Pycha A and Zellou G (2024) The influence of accent and device usage on perceived credibility during interactions with voice-AI assistants. Front. Comput. Sci. 6:1411414. doi: 10.3389/fcomp.2024.1411414
Edited by:
Antonio Benítez-Burraco, University of Seville, SpainReviewed by:
Susanne Brouwer, Radboud University, NetherlandsAllard Jongman, University of Kansas, United States
Copyright © 2024 Pycha and Zellou. This is an open-access article distributed under the terms of the Creative Commons Attribution License (CC BY). The use, distribution or reproduction in other forums is permitted, provided the original author(s) and the copyright owner(s) are credited and that the original publication in this journal is cited, in accordance with accepted academic practice. No use, distribution or reproduction is permitted which does not comply with these terms.
*Correspondence: Georgia Zellou, Z3plbGxvdUB1Y2RhdmlzLmVkdQ==