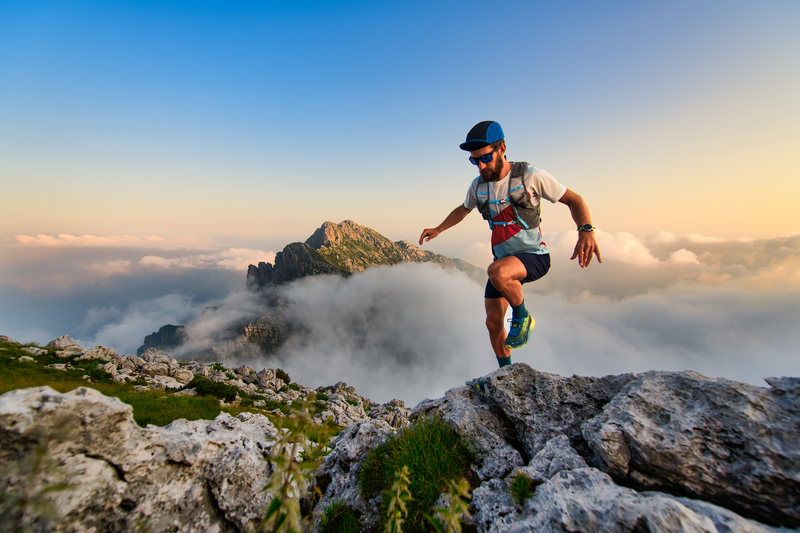
94% of researchers rate our articles as excellent or good
Learn more about the work of our research integrity team to safeguard the quality of each article we publish.
Find out more
ORIGINAL RESEARCH article
Front. Comput. Sci. , 10 May 2024
Sec. Human-Media Interaction
Volume 6 - 2024 | https://doi.org/10.3389/fcomp.2024.1387291
This article is part of the Research Topic Industry-Oriented Interfaces: Bridging the Gap Between Humans and Machines in the Context of Industry 4.0 in the Generative AI Era View all 5 articles
Introduction: Social networking websites and applications are increasingly becoming significant cultural phenomena. However, the motivation driving individuals to utilize these platforms, particularly in the Middle East, remains unclear. While numerous studies have explored technology adoption, limited research has investigated social media usage in the UAE. Therefore, this study proposes and empirically analyzes a framework based on a modified version of the Technology Acceptance Model to understand the factors influencing users’ opinions, objectives, and actual usage of social media platforms, focusing on Facebook.
Methods: To investigate these factors, we employed structural equation modeling and smart PLS techniques on a sample of 540 participants. We aimed to identify predictors such as perceived usefulness, perceived ease of use, as well as a hedonic dimension encompassing perceived enjoyment, curiosity, and perceived connectedness, in shaping users’ attitudes towards Facebook usage. Additionally, we examined the relationship between social presence and users’ intentions to use Facebook.
Results: Our analysis revealed that perceived usefulness and perceived ease of use are significant predictors of users’ attitudes towards utilizing Facebook. Furthermore, the hedonic dimension, comprising perceived enjoyment, curiosity, and perceived connectedness, also plays a crucial role in shaping users’ attitudes. Moreover, social presence was found to have a significant association with users’ intentions to use Facebook.
Discussion: These findings contribute to our understanding of the factors influencing the adoption and usage of social media platforms, particularly in the context of the UAE. By highlighting the importance of both utilitarian and hedonic aspects, this research provides insights into the complex motivations behind individuals’ acceptance of dynamic applications like Facebook. Furthermore, our empirical model lays the groundwork for exploring additional factors that may influence the uptake of information systems and social media utilities, thereby advancing attitude-behavior theories in this domain.
The proliferation of features and applications on the web has facilitated the emergence of more interactive technology that no longer just delivers information to users but rather allows them to be primary contributors and disseminators of content (Garcia and Silva, 2017; Al-Qaysi et al., 2020). The term “Web 2.0” was initially introduced in 1999 to describe the increasing trend (O’reilly, 2005). According to O’reilly (2005), the phrase “Web 2.0” refers to the technical breakthroughs that enable people to generate material, share it with other users, design user-generated material, and engage with others. The emergence of a variety of social media platforms was a key part of the Web 2.0 phenomenon (Kaplan and Haenlein, 2010; Allam et al., 2020). Individuals often use the term “social media” to describe various types of technology infrastructure that focus on community and cooperation (Joosten, 2012; Allam et al., 2019). Additionally, the phrase “social media,” which is commonly substituted for “social software” and “Web 2.0,” refers to the social networking tools that serve as sources of social interaction, collaboration, and creative expression. Similarly, the term “social software” can be substituted for the phrase “Web 2.0” (Al-Qaysi et al., 2020).
Social media platforms such as Twitter, Facebook, Instagram, and LinkedIn have been described as a selection of internet-based applications that build on the ideological and technological foundations of Web 2.0 and enable the production and sharing of user-generated content (Kaplan and Haenlein, 2010; Rauniar et al., 2014). According to Webster’s online dictionary, social media is “a type of electronic communication (e.g., websites for social networking and microblogging) in which users form online communities to exchange information, ideas, personal messages, and other material (e.g., videos) (Mcafee, 2006). Kaplan and Haenlein (2010) identified social media as blogs (e.g., Open Diary and LiveJournal), social networking sites (e.g., Facebook, Twitter, and Google), virtual social worlds (e.g., Second Life and OpenSim), collaborative projects (e.g., Wikipedia), communities (e.g., YouTube and Flicker), and virtual game worlds (e.g., Second Life and OpenSim) (e.g., World of Warcraft).
When discussing user-generated content, people often mention popular Web 2.0 applications like Facebook, WhatsApp, Twitter, YouTube, LinkedIn, Pinterest, and Instagram. These apps have had a notable effect on various areas, including commercial activities, political issues, and venture capital (Greenwood and Gopal, 2015). Not just posts; these social media networks are flooded with photo and video uploads. Recent figures indicate that Snapchat receives approximately 5 billion snaps daily, with 347 million users sharing media daily and three million pictures every second (Statista, 2022). Moreover, 50 million active businesses advertise on Facebook, while 88% of companies use Twitter for promotional activities (Lister, 2017).
Facebook is the most populous and extensively used social networking site, with over 2.9 billion monthly participants as of February 2022 (Dixon, 2024). As seen in Figure 1, Facebook is the most popular social media platform, followed by YouTube, which has 2,562 million monthly users worldwide. It is justifiable to say that Facebook is the first IT application to ever attain this magnitude of fame, showing how swiftly and extensively social media apps are becoming popular. The inquiry of what factors may have an effect on information professionals’ determinations when utilizing new technologies such as Facebook is imperative since information professionals must realize that many of their targeted users employ Facebook as a prime channel of communication, and they must be able to use it to get to and affect their audience as well. Information personnel should also be cognizant that Facebook can be a great way to stay in touch with their colleagues and a spot to share expert updates (Aharony, 2013).
Figure 1. Total social media users (in Millions of Monthly Active Users as of January 2024) (Dixon, 2024).
Users may construct a profile on Facebook and supplement it with images, text, and videos, as well as personal details and preferences. Facebook has an effective communication system and functions as a medium for information dissemination (Amala Jayanthi et al., 2018). Facebook’s social element allows individuals to form groups of like-minded people and utilize control capabilities to monitor the group’s conduct. Members of these communities can rapidly exchange and upload files, articles, links, data, and videos free of charge (Neill and Puettmann, 2013).
The burgeoning popularity of social media necessitates a re-search into uptake and user perspectives, particularly in Middle Eastern countries such as the UAE, where there is a dearth of data concerning user involvement with social platforms. It is worth noting that the United Arab Emirates comprises a number of nationalities and ethnicities, making it an opportune pick for a more thorough study on how customers embrace technology. Owing to the incessant updates of these technologies, there is still a need for empirical research on the social, psychological, and cognitive components that influence people’s adoption of SNSs. This is because there has not been much sustained, comprehensive research on what impels people to use SNSs, despite the swift increase in SNS users. The utilization and acceptance of technology by individuals have been the focus of a multitude of investigations. The Technology Acceptance Model (TAM) is one of the most common methods for understanding how people adopt and use new technologies (Davis et al., 1989). TAM implies that a person’s behavioral intention (BI) to utilize technology is determined by their attitude towards utilizing the technology and their perceived usefulness (PU) and perceived ease of use (PEOU). It is also believed that perceived usefulness is directly impacted by PEOU. Although TAM has been successful in predicting people’s technology adoption, it may not be as effective in the case of social media platforms, as TAM originated from static technology and social media technology is more interactive and dynamic. In this study, we will consider the dynamic, collaborative, and hedonic nature of the social media environment, such as perceived enjoyment (PE), curiosity, perceived connectedness (PC), perceived usefulness (PU), and social influence (SI).
The primary objectives of this research are twofold. Firstly, it will emphasize the applicability of TAM (Davis et al., 1989) to predict user behavior across multiple contexts, including the social media realm. Static systems have previously evaluated TAM because it meets the theoretical requirements of being straightforward (parsimony), amenable to data verification, and capable of predicting the usage and adoption of new technology across a multitude of disciplines (generalizability) (Rauniar et al., 2013). However, TAM has been criticized for only concentrating on organizational information systems and the tangible benefits that users will derive from them. Consequently, the second objective of this study is to incorporate more entertaining features into TAM and demonstrate that these features are critical in determining how users interact with social media platforms, specifically Facebook, in the UAE (Allam et al., 2020).
To accomplish the study’s aims, this paper has been structured as follows: The theoretical framework has been established and a conceptual model has been put forward; the conceptual model will be discussed in the subsequent section, as well as an analysis of Facebook as an example of an information system to which the proposed model could be applied and a description of the research setting. After formulating the hypotheses, which are emphasized in the subsequent parts, the methodology and research outcomes are stated. Next, we investigate the main results and relate them to earlier research findings. Finally, we discuss the essential study principles and limitations, along with potential future research opportunities.
Mark Zuckerberg unveiled ‘thefacebook.com’ on February 4, 2004, initially tailored to Harvard University students. This foundation has since grown into its current status as a ubiquitous social media platform with over two billion users globally. Daily, approximately 500 million individuals access the website and spend a collective 700 billion minutes on it in a single month (Duan et al., 2017). Every month, the website uploads three billion photographs, shares up to five billion items weekly, and creates 3.5 million events (Lee and Paris, 2016; Amala Jayanthi et al., 2018).
Countless studies have been conducted on the uptake of social media. Liebana-Cabanillas and Does-Santos (Liebana-Cabanillas and Alonso-Dos-Santos, 2017) studied the components that determined the uptake of Facebook commerce. Their findings revealed that social image, subjective norms, and usefulness ascertain the users’ end purpose. Moreover, they noticed significant disparities in Facebook usage depending on age. Shaalan (2019) researched factors influencing the adoption of Facebook in an educational setting. Their study found that perceived pleasure, usefulness, and ease of use had a positive effect on Facebook adoption. Amala Jayanthi et al. (2018) probed the sustainability of the aspects impacting Pakistani SMEs’ utilization of social media adoption, particularly Facebook. The study combined the renowned TAM with extra parameters that correspond to the Facebook environment. Particularly, they blended the TAM’s perceived usefulness with a more pragmatic notion of usefulness, displayed as increasing sales and customer factors. The empirical consequences of the study demonstrated a high correlation between Facebook usage and its perceived usefulness. Additionally, they found a strong connection between how frequently people used Facebook and how effortless they thought it was to use.
Unraveling the factors behind the widespread adoption of social media applications by billions of users is essential for better understanding and advancing any future hypotheses in the field of social media. The extensive utilization of these social media platforms demonstrates their success in terms of acceptance and implementation in users’ personal, social, and professional lives. This includes everyday engagement, photo sharing, self-presentation, and so on. Consequently, new research initiatives need to be directed towards the examination of internet-enabled media and platforms, as well as online socialization processes and activities. If the utilization of social media is mostly voluntary in nature, then the cause of this behavior must be attributed to the personal intentions and motives of individual users (Rauniar et al., 2014). As suggested by Fishbein and Ajzen (1975) and Doll and Torkzadeh (1988), such emotional dispositions and intents contribute to the actual use of the system. In this regard, Davis’s technology acceptance model (TAM) is a well-substantiated model that can be employed to evaluate the usage behavior of new technologies (Davis, 1986; Davis et al., 1989).
Beginning with Davis et al. (1989), the Technology Acceptance Model (TAM) suggests that consumers’ adoption of technology is affected by their perceived usefulness (PU) and perceived ease of use (PEOU). Both of these factors have an effect on users’ attitudes and intentions to use technology, and TAM proposes that these intentions have a positive influence on actual utilization. PU is described as “the extent to which an individual believes that utilizing a given system would boost their work performance,” while PEOU is “the degree to which a person believes that using a specific system would be straightforward” [17 p.]. Subsequent studies have incorporated other elements into TAM to assess the adoption of social media in different sectors (e.g., Allam et al., 2011, 2012; Kwon et al., 2014).
User acceptance denotes the eagerness of users to employ information technology for its proposed use (Dillon and Morris, 1996). Social media applications, like Facebook, Twitter, and Instagram, among others, have traits that set them apart from more traditional static technology. In accordance with Wattal et al. (2009), social media applications are regarded as bottom-up technologies, implying that users must be acquainted with them before their deployment within a business. Because they resemble generally used tools with which customers are already familiar, even non-technical users will have minimal difficulty utilizing these applications. Given the considerable disparities between social media tools and traditional technology tools in their reliance on users’ social interaction, it is reasonable to inquire whether formerly inspected socio-demographic characteristics applicable to earlier technologies will continue to apply to the adoption of social media tools (Wattal et al., 2009; Amala Jayanthi et al., 2018; Allam et al., 2019, 2020). Since researchers want not only acceptance but also successful sharing, contribution, and cooperation to gain the most from these tools, they need to move away from the traditional TAM to discover hidden dimensions that can be applicable to the nature of social media tools in particular. As evidenced in the previous section, this study applies TAM and supplements it with other sociological theories to take social variables pertinent to the setting of social media technologies into account. Recent studies suggest that TAM should be adjusted to reflect technological and user advancements. For instance, Legris et al. (2003) recommended that TAM’s original PU should be modified to encompass elements pertinent to human and social transformation processes.
TAM’s motivation theory is regularly employed to grasp user adoption of information technology, as noted by Deci et al. (2001). The uptake of technology by users is contingent on two types of motivation: extrinsic and intrinsic. As per Deci et al. (2001), extrinsic motivation alludes to the behaviors users take part in while interacting with IT systems to acquire a preferred outcome, such as enhancing job performance or increasing the efficiency of work-related tasks. Intrinsic motivation encompasses all acts done by users for non-extrinsic motives. Put differently, intrinsic motivation is any action that gives users a sense of satisfaction and pleasure from within, like aiding others or using an app, because it is delightful and nourishes curiosity and explorability.
By amalgamating the original TAM variables with other external components that could be successful with Facebook, this study focuses on evaluating the factors that shape face-book utilization. We include the hedonic and social aspects that match the ever-changing character of Facebook. Previous studies have alluded to the need to modify TAM to incorporate social factors that fit the dynamic nature of contemporary social media platforms. Previous research on the Technology Acceptance Model (TAM), for instance, has incorporated variables that are in line with the static nature and simplicity of older applications that do not necessitate users to socially interact with other users (Allam et al., 2019, 2020). As opposed to their fixed counterparts, today’s social media programs are animated with user interaction and have components intended to evoke feelings of pleasure and joy in today’s tech-savvy consumers. Moreover, TAM’s initial design was primarily focused on the characteristics of the system, thus omitting some crucial aspects of social interactions. Notably, TAM’s fundamental conception was that organizations leverage information technology to enhance employee efficiency. However, TAM fails to acknowledge the potential use of information systems for purposes beyond work, like leisure and pleasure. Additionally, TAM theory does not consider how other users may shape one’s opinion on social media and, consequently, how much they use it. This is a noteworthy issue, considering various psychological studies have demonstrated that people are swayed by the behavior of those around them (Rauniar et al., 2014). This proposed model of the study seeks to bridge the gap by adding contemporary elements that would give TAM a modernized makeover, so it can be applied to social media platforms such as Facebook.
Venkatesh et al. (2003) conducted an in-depth study of IT behavior that provides several valuable techniques and UTAUT deployment. Subsequent researchers could use this research as a basis to further validate and test their theories. The UTAUT model (Amala Jayanthi et al., 2018) can be used to evaluate the difficulties encountered while implementing a social media environment. According to Venkatesh et al. (2003), the UTAUT model serves as the primary theoretical framework to analyze the adaptive behavior of social media managers. Figure 1 displays the proposed research model of the factors that affect three latent variables: attitude to use (ATU), intention to use (IU), and actual use of Facebook (AU). As can be seen in Figure 2, the study hypothesizes that perceived ease of use (PEOU), perceived usefulness (PU), enjoyment (PE), curiosity (CU), and perceived connectedness (PC) have a significant impact on the attitude of Facebook users. Furthermore, the model postulates that users’ attitudes (ATU) positively affect their intention to use (IU), and that (IU) has a positive impact on the actual use (AU) of Facebook users. Every supposition will be examined, in conjunction with its pertinent literature, in the subsequent section.
Perceived Ease-of-use is the extent to which a user perceives that utilizing a certain system will be easy (Davis et al., 1989; Venkatesh et al., 2000). Hence, we define it as the degree to which someone believes that employing the Facebook platform requires no effort. Davis et al. (1989) articulated that “an application seen as simple to use is more likely to be adopted by users” (p. 2). Our model, consequently, includes a relationship between perceived ease of use and the intent to use. Substantial connections between these two variables have been identified in numerous empirical investigations of the TAM (Moore and Benbasat, 1991; Allam et al., 2012, 2020, 2022) discovered a powerful correlation between users’ perception of the simplicity of social platforms and their attitudes toward tagging resources. Similarly, Li et al. (2024) revealed that a high level of PEOU correlates with a high degree of perceived usefulness and a strong preference for tagging systems among users. In their examination of users’ acceptance of a combination social platform approach in an online knowledge forum, Qin et al. Qin, Liu, Mou, and Chen (2019) demonstrated that the more convenient the system is, the more favorably users view it. Furthermore, by employing an expansion of the TAM, Al-Ghaith (2015) studied individuals’ investment intentions, and conduct on social networking sites; he revealed that PEOU had a noteworthy effect on users’ dispositions towards utilizing such sites. These results affirm the following postulation:
H1: Perceived ease of use will have a positive impact on users’ attitudes towards using Facebook.
Perceived usefulness (PU) is a concept referring to the extent to which an individual believes that using a particular system would be beneficial in improving their job tasks (Davis et al., 1989). It has been found that a user’s view of an information system’s utility is strongly correlated to their intention to utilize technology, particularly in work-related situations Morschheuser, Hamari, Koivisto, and Maedche (2017). Recent research on the basis of TAM-based models, however, has established a connection between perceived usefulness and users’ attitude towards using social and hedonic systems Hamari and Keronen (2017) In the case of this study, “perceived usefulness” is used to describe how much a person believes that using Facebook can make it easier to find beneficial resources. In their exploration into why people utilize gaming systems Saleem, Noori, and Ozdamli (2022)uncovered a strong association between usage and the perceived benefits procured from playing games. Even though games are regularly seen as the quintessence of hedonistically-oriented platforms, the results demonstrate that both delight and utility are similarly critical in deciding their utilization (albeit their definitive job changes between game types). Subsequently, it tends to be inferred that game systems and social hedonic systems are stages that can be utilized for more than one reason and depend on hedonic parameters even when they are utilized for instrumental purposes. Consequently, we present the accompanying hypothesis:
H2: Perceived connectedness will have a positive impact on users’ attitudes towards using Facebook.
Van-der-Heijden (2004) defines perceived enjoyment as the extent to which utilizing social systems is thought to be enjoyable, regardless of its efficacy. Owing to its pleasurable nature, online users are more likely to take part in activities on social media platforms. Allam et al., 2012, 2020, 2022 and Van-der-Heijden (2004) have established a substantial link between perceived enjoyment and user adoption of technology with significant implications for hedonic systems. Additionally, Al-Azawei (2018) has discovered a robust link between perceived enjoyment and social users’ intent to make use of social media. Thus, it is hypothesized that perceived enjoyment will be related to users’ behavioral intention to utilize the tagging systems as well as positive attitudes. As a result, we posited:
H3: Perceived enjoyment will have a positive impact on users’ attitudes towards using Facebook.
We conceptualized curiosity as the notion that utilizing Facebook will satisfy one’s innate curiosity cravings. Allam et al. (2012) observed that curiosity is positively correlated with users’ attitudes toward using social media platforms, such as social tagging tools. Moreover, earlier studies have demonstrated that curiosity appears to enhance the quality of the search experience in terms of time and data resources, as well as lead to a more vigilant recall and understanding of new information (Menon and Soman, 2002; Allam et al., 2020). Moon and Kim (2001) also studied the concept of curiosity to describe the effect of perceived playfulness on the attitude of users towards utilizing online web applications. They suggested that the notion of playfulness could have many levels, one of which is curiosity, which motivates users to heighten their perceived enjoyment, which has a beneficial effect on their attitude toward utilizing online apps. Recent research on hedonic tagging systems by Allam et al. (2020) identified three dimensions of the pleasure concept. They empirically support a previously portrayed theoretical model of three-dimensional conceptions of hedonism with three components explaining the hedonistic state of people when interacting with social tagging tools: explorability, curiosity, and enjoyment. Utilizing structural equation modeling, the research suggests utilizing a more precise definition of pleasure to encompass a hedonic factor when examining intrinsic motivation by means of interactive social media applications. Specifically, they demonstrated that users click on the tags of others out of intrinsic interest to discover what resources have been labeled. Consequently, we suggest the following:
H4: Curiosity will have a positive impact on users’ attitudes towards using Facebook.
According to Venkatesh et al. (2003), social influence is the degree to which one comprehends how others feel they should utilize a new information system. Past research suggests that social influence has a bearing on an individual’s readiness to accept and use novel technologies. Together with knowledge from UTAUT and preceding studies (Amala Jayanthi et al., 2018; Salloum et al., 2018), we acknowledge how social influence significantly affects behavioral intention to employ social media technology. A recent study by Allam et al. (2020) recently discovered a noteworthy connection between social influence and the intention of users to utilize social networking sites, particularly social tagging sites. Similarly, Amala Jayanthi et al. (2018) and Salloum et al. (2018) ascertained that students’ behavioral intentions to use Facebook in the UAE are impacted by the social influence of their acquaintances who also use the network. Consequently, the following hypothesis is tested:
H6: Social influence will have a positive influence on the intentions of Facebook users.
Attitude is a person’s own assessment of how beneficial or damaging their actions may be (Ajzen and Fishbein, 1980). Therefore, a Facebook user’s attitude involves their judgment on whether or not the usage of such tools is advantageous and the degree to which they decide to apply them. Attitudes are shaped based on past experiences and accessible emotional and mental data, and it is believed that they influence people’s behavior toward a specific activity (Fishbein and Ajzen, 1975). According to TAM (Davis et al., 1989), a person’s goal to utilize a system is influenced by their attitude toward using that system. Attitude is considered a primary determinant of behavior and results in extensive IS users’ acceptance research, such as social tagging (Allam et al., 2011; Dhir, 2016; Amala Jayanthi et al., 2018), social networking services (Kwon et al., 2014), social world (Hamari and Keronen, 2017), and online purchase intention (Rana et al., 2016). Accordingly, we posit the following hypothesis:
H7: Users’ attitudes will have a positive impact on users’ attitudes towards using Facebook.
TAM postulates that beliefs or attitudes towards perceived usefulness dictate users’ intention to engage (IU) with the technology, resulting in actual usage (AU) of the technology (Davis et al., 1989; Venkatesh et al., 2000). In the context of our current research on social media, we conceptualize IU as the continuing determination to partake in social media-related activities using Facebook (Fishbein and Ajzen, 1975).
Additionally, we conceptualize AU as the frequency with which an individual interacts with Facebook. Notably, the connection between PU, IU, and AU in the TAM model was derived from the TRA theory (Ajzen and Fishbein, 1980). We posit, based on the TRA, that a social media user’s behavior on Facebook is determined by his or her intention to use it, which is dependent on his or her perceived benefit from the social media platform. Intention symbolizes a decision that a person has made on whether or not to execute a particular behavior and, thus, is established through a process of mental contemplation, conflict, and commitment that may take a considerable amount of time (Davis, 1986). Consequently, intention implies a more constant mental state of a user in terms of social media consumption behavior. IU Social media is the conscious and intentional portrayal of an individual’s eagerness to employ social media.
Consequently, in our extended technology acceptance model, we view IU social media use as the precursor of Facebook consumption. We posit that the selection of IU social media should be determined by the user’s evaluation of its advantages. The Theory of Reasoned Action states that, as a general rule, the more positive an individual’s opinion on an action, the more likely they are to indulge in that behavior. Different social media applications serve various functions. For example, LinkedIn, Twitter, Pinterest, and Digg are used for professional networking, while YouTube is utilized for sharing videos. Considering that social media is about user-generated and shared content, the amount of communication by people implies that they have a positive view of these websites. The countless Facebook posts that get comments, the millions of tweets that are retweeted, and the many YouTube videos that are uploaded and commented on demonstrate that users are participating in these activities due to their good perception of the sites. We suggest that social media users form opinions about PU derived from their experiences and actions on social media sites. The favorable outlook towards potential gain, as elucidated by Bandura (1977), Ajzen and Fishbein (1980), Davis et al. (1989), and Ajzen (1991), may thereby anticipate IU social media and stimulate its adoption. Recent studies have employed the Theory of Reasoned Action to foretell actual utilization of social media (e.g., Amala Jayanthi et al., 2018; Allam et al., 2019; Shafique et al., 2020). Hence, we propose the following hypothesis:
H8: Users’ intentions will have a positive impact on users’ attitudes toward using Facebook.
To corroborate the suggested model, Structure Equation Modeling (SEM) was applied as the data analysis method (Chin, 1998), as the aim of the research was to analyze the connection and the effect of interactions between five dimensions. SEM is a technique employed to detect and estimate linear relationship models between tangible and latent variables (Fornell and Larcker, 1981; Gefen and Straub, 2005), who recommend using SEM to measure the intensity and direction of the correlation between the theoretical components. Smart PLS was the software utilized in our study to assess the measurement and structure.
Table 3 displays the findings of the measurement framework, including average variance extracted (AVE), composite reliability (CR), variance inflation Factors (VIF) as a multi-collinearity measure, Cronbach’s alpha, and item loading. To evaluate the convergent authenticity of reflective variables, AVE is taken into account. As Table 3 demonstrates, the AVE values in this study were higher than the 0.5 cutoff point, varying from 0.581 to 0.866 (Garver and Mentzer, 1999). Concerning all latent variables over the 0.7 cutoff, the composite reliability ranged from 0.831 to 0.928, while the internal consistency reliability ranged from 0.702 to 0.879 (Hulland, 1999). The VIF figures indicate that the multi-collinearity assumption was not breached. The reliability of a single item is investigated by measuring the outer loadings, which pertain to the loadings of the reflective items with their corresponding constructs. This is shown in Table 3. If an item’s loading is 0.7 or higher, it is deemed acceptable (Urbach and Ahlemann, 2010). All items in the research had external loadings that ranged from 0.718 to 0.931, with the exception of one item that had an external loading of 0.675.
Table 3. AVE, VIF, Cronbach’s alpha, external weighing, and estimates of loads derived from the measurement framework.
The aim of the research was to analyze the connection and effect of interactions between five dimensions. Researchers employ SEM to detect and estimate linear relationship models between tangible and latent variables (Fornell and Larcker, 1981; Gefen and Straub, 2005), recommending its use to measure the intensity and direction of correlation between theoretical components. We used Smart PLS software in our study to evaluate the measurement and its structure.
Table 3 displays the findings of the measurement framework, including average variance extracted (AVE), composite reliability (CR), variance inflation factors (VIF) as a multi-collinearity measure, Cronbach’s alpha, and item loading. We consider AVE to evaluate the convergent authenticity of reflective variables. As Table 3 demonstrates, the AVE values in this study were higher than the 0.5 cutoff point, varying from 0.581 to 0.866 (Garver and Mentzer, 1999). Concerning all latent variables over the 0.7 cutoff, the composite reliability ranged from 0.831 to 0.928, while the internal consistency reliability ranged from 0.702 to 0.879 (Hulland, 1999). The VIF figures demonstrate that the multi-collinearity assumption remained intact. We investigate the reliability of a single item by measuring the outer loadings, which correspond to the loadings of reflective items with their respective constructs. This is shown in Table 3. If an item’s loading is 0.7 or higher, it is deemed acceptable (Urbach and Ahlemann, 2010). All items in the research had external loadings that ranged from 0.718 to 0.931, with the exception of one item that had an external loading of 0.675.
Moreover, we assessed discriminant validity, which refers to the extent to which the measures for each latent variable deviate from the measures of other constructs. In simpler terms, it guarantees that the items in a construct do not inadvertently measure other constructs. According to Fornell and Larcker’s (1981) criteria, for a latent variable to be deemed valid, the square root of the AVE of the latent variable should have a stronger correlation with itself than with any other construct. Table 4 demonstrated that for each latent variable, the square root of AVE exceeded the estimations of intercorrelations with other pertinent constructs (Fornell and Larcker, 1981). No relationship was found between 0.85 and bigger constructs (Kline, 2011). Therefore, we can infer that the estimations of intercorrelation across all constructs seemed to be internally consistent, which confirms that all constructs are authentic.
Next, in the structural model evaluation process is the examination of path coefficients between latent variables. Recommendations for evaluating path coefficient correlations include three checks: algebraic sign, size, and significance. The magnitude of a path coefficient reflects the strength of the association between latent variables. Paths that show signs contrary to the theoretical model do not support the hypotheses of the model. Path coefficients must be significant at the 0.05 level or above (Hair et al., 2011). Recent research (Efron and Tibshirani, 1994; Hair et al., 2011) suggests employing resampling approaches such as bootstrapping to assess the relevance of the model (Hsu and Lin, 2008). The bootstrapping method is used to evaluate the significance of the t-value. Table 5 provides a summary of the path coefficients for the tag generation and sharing model, along with their associated t-values. This study adopted a bootstrapping method in assessing the t-value, utilizing 5,000 samples with 540 observations collected for the analysis. Figure 2 displays the structural model, which features three endogenous variables (i.e., attitude, intention, and actual Facebook usage behavior). The structural model is in accordance with all eight hypotheses, with the following values being statistically significant at the 0.001 level: 2.1, 2.6, 3.4, 3.02, 4.25.5.20, 6.54, and 5.6. The ATU’s R2 of 0.65 indicates that the influencing variables account for 65% of the variance in the attitude toward using Facebook, while the intention’s R2 of 0.72 implies that attitude and social presence together explain 72% of the variation in the intention construct. Lastly, the R2 for actual use was 0.68, revealing that intention to use explains almost 68% of the variance in actual Facebook usage (Figure 3).
A theoretical model was devised to delve into the factors influencing the adoption behavior of Facebook users. To scrutinize this, a questionnaire was meticulously crafted to inquire about participants’ perceptions and experiences with Facebook. The questionnaire encompassed 28 measuring items. To align with the context of Facebook adoption, questions adapted from prior Information Systems (IS) research underwent minor adjustments. The items for Perceived Ease of Use (PEOU), Attitude (ATT), and Intention to Use (IU) were drawn from studies by Davis et al. (1989) and Liao et al. (2008). For the concept of Perceived enjoyment (PE), items based on Davis et al. (1989) and Adilla-Melendez et al. (2013) were developed. To assess perceived connectedness, items were slightly modified from Kwon et al. (2014). Additionally, items from the recent research of Allam et al. (2019) were utilized to measure the construct of Curiosity (CU). Ultimately, three items were formulated to quantify Actual Usage (AU), drawing from the works of Phang et al. (2009), Moon and Kim (2001), Hsu and Lin (2008), and Rauniar et al. (2013). The questionnaire employed a seven-point Likert scale, ranging from “Strongly Disagree” (1) to “Strongly Agree” (7), to evaluate each topic. A compilation of the specific constructs and item measurements is provided in Table 1.
We disseminated a link to the survey and a synopsis of the study over UAE Facebook groups to evaluate our proposed model. Invitees were asked to provide responses regarding their Facebook usage patterns in our online survey. To incentivize participation in the study, participants had the opportunity to enter a draw for one of 30 AED 25 Amazon gift cards. To avoid duplicate replies, we implemented browser cookies and strictly followed the survey’s progression. Email addresses were employed to detect duplicate entries, as individuals had to supply them to be in the draw. From the first announcement, a total of 559 answers were obtained. We re-posted the invitation and the survey link on the same Facebook group a week after the first announcement, resulting in an additional 170 answers. Over the 10 days that the survey link was active, 729 people responded. Hundred and eighty nine unfinished responses were excluded, leaving 540 completed, ready-to-analyze answers. Table 2 includes the attributes of the respondents in our sample.
Table 2. Descriptive statistics for variables impacting attitude, behavioral intention, and real Facebook utilization (N = 540).
Table 2 shows that 44% of survey respondents were male and 56% were female. Generally, the majority of users were in the age range of 31 to 40, followed by those aged 21 to 30. Regarding nationality, 28% were Indian, 24% were Egyptian, 18% were Pakistani, 15% were from Bangladesh, 8% were from Iraq, and 5% were from Jordan. Interestingly, despite the survey being conducted in the UAE, only 2% of Facebook users were UAE citizens. This could be attributed to Instagram or other social media platforms being more popular in the UAE than Facebook. When it comes to experience, 24 and 33% of respondents declared they had used Facebook for 3–5 years, respectively. This is likely due to the fact that most users are relatively new to Facebook. As for the average amount of time spent on Facebook, 44% of users said they use it between 1 and 2 h per day, while 35% said they use it for 2–3 h per day.
Facebook’s global ubiquity stems from its ability to foster interpersonal connections, facilitate communication, provide entertainment, and promote social interactions among its vast user base. In light of this, our research endeavors to identify the motivational drivers behind social media platform usage and explore their impact on user acceptance of Facebook. To achieve this, we have developed an integrated research model aimed at complementing the well-established Technology Acceptance Model (TAM) when applied in the dynamic context of social media.
By expanding the TAM model, our study seeks to elucidate crucial factors influencing users’ acceptance and usage behavior on social media platforms, particularly Facebook. Through empirical analysis, we have made significant theoretical advancements in understanding social media adoption and usage. Building upon prior research insights and frameworks (Rauniar et al., 2014; Al-Azawei, 2018; Allam et al., 2019, 2020; Al-Qaysi et al., 2020). our study represents a comprehensive investigation into user acceptance and actual utilization of social media within the unique context of the UAE.
The internal consistency and composite reliability of the constructs examined in our study indicate the robustness of our model and the soundness of the proposed hypotheses. As such, our research provides a solid foundation for future explorations into various social media platforms, offering valuable insights for academia and industry alike.
The proposed study posited that Perceived Ease of Use (PEOU), Perceived Usefulness (PU), Perceived Enjoyment (PE), Curiosity (CU), and Perceived Connectedness (PC) would exert a positive influence on Facebook users’ attitudes. Furthermore, the study hypothesized that users’ attitudes and social pressure would significantly impact Facebook usage, ultimately leading to increased application of Facebook. The outcomes of the factor analysis and the measurement model were instrumental in identifying the essential components of the new TAM model for social media. This represents a crucial contribution to the social media literature, enriching the TAM framework with emotional and hedonic elements tailored to the dynamics of social media platforms. The validated model holds the potential to serve as a benchmark for future researchers in this domain.
According to our research findings, perceived ease of use, usefulness, connectedness, enjoyment, curiosity, and connectedness all play significant roles in influencing the decision to utilize Facebook in the UAE. Notably, pleasure and curiosity emerged as more influential factors than perceived usefulness, contrary to previous TAM findings on static applications. Historically, research on technology acceptance has heavily emphasized utilitarian aspects, emphasizing practical advantages as the primary motivator for technology use. However, our findings suggest that the primary driver behind Facebook usage lies in hedonistic motives, such as seeking amusement and curiosity-driven exploration of novel content on other users’ profiles. This aligns with recent studies proposing hedonism as a key predictor of social media application usage, particularly when users engage with platforms out of enjoyment in browsing their content.
Additionally, our findings challenge the conventional notion that perceived ease of use is a decisive factor in determining Facebook acceptance. While users perceive social networking websites like Facebook as user-friendly and easy to navigate, our study suggests that other features such as enjoyment, curiosity, social impact, and perceived connectedness play more significant roles in motivating their usage. This aligns with previous research on Facebook and Twitter, indicating that users find these platforms easy to use due to their intuitive interfaces and basic functionalities, facilitating seamless adoption even among novices.
An intriguing discovery from our study is the prominence of perceived connectedness as a predictor of user attitudes towards using Facebook, surpassing the influence of perceived usefulness and perceived ease of use. This underscores Facebook’s role in fostering personal connections and highlights users’ inclination to utilize the platform for establishing and nurturing social relationships, ultimately leading to a sense of connectedness. For instance, features like Facebook’s “Find My Friends” functionality contribute to enhancing feelings of closeness by facilitating effortless location sharing among users, further reinforcing their social bonds.
While our findings contribute significantly to our understanding of social network adoption, particularly on Facebook, there are notable limitations in our study that should be considered when planning future research on similar topics. Firstly, our study was confined to the setting of the United Arab Emirates. Therefore, conducting a more comprehensive investigation that includes a more diverse population would enrich the pool of knowledge on this subject. Secondly, our research model did not account for individual characteristics among survey respondents. Individual attributes such as age, gender, and experience are expected to have moderating effects on the process of technology adoption.
Hence, future research could explore additional motivational factors that were not addressed in our study. For instance, Kwon et al. (2014) suggest that perceived mobility is a significant driver of Facebook and Twitter user adoption. Additionally, Salloum et al. (2018) and Sangi et al. (2018) argue that performance expectancy is strongly correlated with users’ willingness to use Facebook in the UAE. It is important to note that, as defined by Venkatesh et al. (2003), “performance expectancy” refers to the extent to which an individual believes they can achieve benefits in their job performance with the assistance of an information system.”
Furthermore, as our proposed research model only explains 65%, 72%, and 68% of the variances in attitude, intention, and actual Facebook usage, respectively, incorporating additional elements such as perceived portability (Kwon et al., 2014) and performance expectation (Amala Jayanthi et al., 2018) may enhance the model’s ability to elucidate these phenomena.
This study delved into the perceptions of UAE users regarding the adoption of social media technology, focusing specifically on Facebook, employing the Technology Acceptance Model (TAM). Recognizing the dynamic nature of social media platforms, we extended the traditional TAM framework to comprehensively investigate the factors influencing Facebook usage among UAE users, drawing on insights from previous research in the realm of social media platforms.
To gather data for our quantitative study, we utilized the survey method, capturing the perspectives and behaviors of users in the UAE. Structural Equation Modeling (SEM) was employed to assess the predictive power of our research model, which aimed to elucidate the determinants of users’ attitudes towards Facebook, subsequently influencing their motivation and actual usage.
Our proposed model integrated several key variables deemed critical in shaping users’ attitudes and behaviors on Facebook. These variables included Perceived Ease of Use (PEOU), Perceived Usefulness (PU), Perceived Enjoyment (PE), Curiosity (CU), and Perceived Connectedness (PC). We posited that these factors collectively influence users’ attitudes, intentions, and ultimately their usage behavior on Facebook.
Moreover, recognizing the inherently social nature of social media platforms, our model also incorporated the influence of social factors, predicting that social influence would play a significant role in users’ intention to use Facebook. This aspect reflects the interconnectedness and influence of social relationships within the realm of social media platforms.
Through a comprehensive empirical validation process utilizing Structural Equation Modeling (SEM) and Smart PLS, our research model demonstrated considerable explanatory power. Specifically, our model accounted for 65% of users’ attitudes, 72% of users’ intentions, and ultimately 68% of actual Facebook usage among UAE users.
Our study underscores the versatility and applicability of the Technology Acceptance Model (TAM) in the context of social media platforms. By augmenting the traditional TAM framework with relevant social and hedonistic aspects tailored to the social media environment, we were able to provide valuable insights into users’ perceptions and behaviors on Facebook within the UAE context.
The raw data supporting the conclusions of this article will be made available by the authors, without undue reservation.
HA: Conceptualization, Data curation, Investigation, Methodology, Software, Supervision, Writing – original draft, Writing – review & editing. JD: Data curation, Formal analysis, Investigation, Methodology, Project administration, Supervision, Validation, Writing – original draft, Writing – review & editing. EL: Data curation, Formal analysis, Project administration, Validation, Writing – original draft, Writing – review & editing, Conceptualization, Funding acquisition, Investigation, Methodology, Resources, Software, Supervision. CD: Project administration, Resources, Validation, Visualization, Writing – original draft, Writing – review & editing, Conceptualization, Data curation, Investigation, Methodology, Software, Supervision.
The author(s) declare that no financial support was received for the research, authorship, and/or publication of this article.
The authors declare that the research was conducted in the absence of any commercial or financial relationships that could be construed as a potential conflict of interest.
All claims expressed in this article are solely those of the authors and do not necessarily represent those of their affiliated organizations, or those of the publisher, the editors and the reviewers. Any product that may be evaluated in this article, or claim that may be made by its manufacturer, is not guaranteed or endorsed by the publisher.
Adilla-Melendez, A., Aguila-Obra, A. R., and Garrido-Moreno, A. (2013). Perceived playfulness, gender differences, and technology acceptance model in a blended learning scenario. Comput. Educ. 63, 306–317. doi: 10.1016/j.compedu.2012.12.014
Aharony, N. (2013). Factors affecting the adoption of Facebook by information professionals. Proc. Am. Soc. Inf. Sci. Technol. 50, 1–10. doi: 10.1002/meet.14505001030
Ajzen, I. (1991). The theory of planned behavior. Organ. Behav. Hum. Decis. Process. 50, 179–211. doi: 10.1016/0749-5978(91)90020-T
Ajzen, I., and Fishbein, M., Understanding attitudes and predicting social behavior. Englewood Cliffs, NJ: Prentice-Hall (1980)
Al-Azawei, A. (2018). Predicting the adoption of social media: an integrated model and empirical study on Facebook usage. Int. J. Inform. Knowledge Manag. 13, 233–258. doi: 10.28945/4106
Al-Ghaith, W. (2015). Applying the technology acceptance model to understand social networking sites (SNS) usage: impact of perceived social capital. Int. J. Comput. Sci. Inform. Technol. 7, 105–117. doi: 10.5121/ijcsit.2015.7409
Allam, H., Bliemel, M., Ali-Hassan, H., Blustein, J., and Spiteri, L. (2020). If you build it, they won’t come: what motivates employees to create and share tagged content: a theoretical model and empirical validation. Int. J. Inf. Manag. 54:102148. doi: 10.1016/j.ijinfomgt.2020.102148
Allam, H., Bliemel, M., Spiteri, L., Blustein, J., and Ali-Hassan, H. (2019). Applying a multi-dimensional hedonic concept of intrinsic motivation on social tagging tools: a theoretical model and empirical validation. Int. J. Inf. Manag. 45, 211–222. doi: 10.1016/j.ijinfomgt.2018.11.005
Allam, H., Blustein, J., Bliemel, M., and Spiteri, L., Exploring factors Impacting users’ attitude and intention towards social tagging systems, (2012)
Allam, H., Blustein, J., Spiteri, L., and Bliemel, M., Enhancing exploratory search with hedonic browsing using social tagging tools, IEEE third Int’l conference on privacy, security, risk and trust and 2011 IEEE Third Int’l Conference On Social Computing (2011).
Allam, H., Akre, V., Blustein, J., Toze, S., and Kalota, F. (2022). Factors affecting people’s adoption of facebook: a uae case study. In 2022 8th International Conference on Information Technology Trends (ITT). IEEE. 34–38.
Al-Qaysi, N., Mohamad-Nordin, N., and Al-Emran, M. (2020). Employing the technology acceptance model in social media: a systematic review. Educ. Inf. Technol. 25, 4961–5002. doi: 10.1007/s10639-020-10197-1
Amala Jayanthi, M., Swathi, S., and Lakshmana Kumar, R. (2018). Investigation on association of self-esteem and students’ performance in academics. Int. J. Grid and Utility Comput. 9, 211–219. doi: 10.1504/IJGUC.2018.10015144
Bandura, A. (1977). Self-efficacy: toward a unifying theory of behavioral change. Psychol. Rev. 84, 191–215. doi: 10.1037//0033-295X.84.2.191
Boyd, D. M., and Ellison, N. B. (2007). Social network sites: definition, his- tory, and scholarship. J. Comput.-Mediat. Commun. 13, 210–230. doi: 10.1111/j.1083-6101.2007.00393.x
Chin, W. W. (1998). The partial least squares approach to structural equation modeling. Modern Methods Busin. Res. 295, 295–336.
Davis, F. D., A technology acceptance model for empirically testing new end-user information systems: theory and results, Doctoral Dissertation, Cambridge, MA (1986)
Davis, F. D., Bagozzi, R. P., and Warshaw, P. R. (1989). User acceptance of computer technology: a comparison of two theoretical models. Manag. Sci. 35, 982–1003. doi: 10.1287/mnsc.35.8.982
Davis, F. D., Bagozzi, R. P., and Warshaw, P. R. (1992). Extrinsic and intrinsic motivation to use computers in the workplace. J. Appl. Soc. Psychol. 22, 1111–1132. doi: 10.1111/j.1559-1816.1992.tb00945.x
Deci, E. L., Koestner, R., and Ryan, R. M. (2001). Extrinsic rewards and intrinsic motivation in education: reconsidered once again. Rev. Educ. Res. 71, 1–27. doi: 10.3102/00346543071001001
Dhir, A. (2016). Why do young people avoid photo tagging? A new service avoidance scale. Soc. Sci. Comput. Rev. 35, 480–497. doi: 10.1177/0894439316653636
Dillon, A., and Morris, M. G. (1996). User acceptance of new information technology: theories and models. Available at: https://repository.arizona.edu/handle/10150/105584
Dixon, S. J., Most popular social networks worldwide as of January 2024, ranked by number of monthly active users, (2024). Available at: https://www.statista.com/statistics/272014/global-social-networks-ranked-by-number-of-users/
Doll, W. J., and Torkzadeh, G. (1988). The measurement of end-user computing satisfaction. MIS quarterly. doi: 10.2307/248851
Duan, N., Bai, Y., Sun, H., Wang, N., Ma, Y., Li, M., et al. (2017). Genome re-sequencing reveals the history of apple and supports a two-stage model for fruit enlargement. Nat. Commun. 8:249. doi: 10.1038/s41467-017-00336-7
Efron, B., and Tibshirani, R. J. (1994). An introduction to the bootstrap. Chapman and Hall/CRC. Available at: https://www.taylorfrancis.com/books/mono/10.1201/9780429246593/introduction-bootstrap-bradley-efron-tibshirani
Fishbein, M., and Ajzen, I., Belief attitude, intention and behavior: an introduction to theory and research. Reading. MA: Addison-Wesley (1975)
Fornell, C., and Larcker, D. F. (1981). Evaluating structural equation models with unobservable variables and measurement error. J. Market. Res. 18, 39–50. doi: 10.1177/002224378101800104
Garcia, L. S., and Silva, C. M. C. (2017). Differences between perceived usefulness of social media and institutional channels by undergraduate students. Interact. Technol. Smart Educ. 14, 196–215. doi: 10.1108/ITSE-01-2017-0009
Garver, M. S., and Mentzer, J. T. (1999). Logistics research methods: employing structural equation modeling to test for construct validity. J. Bus. Logist. 20:33.
Gefen, D., and Straub, D. (2005). A practical guide to factorial validity using PLS-Graph: Tutorial and annotated example. Communications of the Association for Information systems. 16:5.
Greenwood, B. N., and Gopal, A. (2015). Research note—Tigerblood: news- papers, blogs, and the founding of information technology firms. Inf. Syst. Res. 26, 812–828. doi: 10.1287/isre.2015.0603
Hair, J. F., Ringle, C. M., and Sarstedt, M. (2011). PLS-SEM. indeed a silver bullet. J. Mark. Theory Pract. 19, 139–152. doi: 10.2753/MTP1069-6679190202
Hamari, J., and Keronen, L. (2017). Why do people play games? A meta- analysis. Int. J. Inf. Manag. 37, 125–141. doi: 10.1016/j.ijinfomgt.2017.01.006
Hsu, C. L., and Lin, J. C. C. (2008). Acceptance of blog usage: the roles of technology acceptance, social influence 651 and knowledge sharing motivation. Inf. Manag. 45, 65–74. doi: 10.1016/j.im.2007.11.001
Hulland, J. (1999). Use of partial least squares (PLS) in strategic management research: a review of four recent studies. Strateg. Manag. J. 20, 195–204. doi: 10.1002/(SICI)1097-0266(199902)20:2<195::AID-SMJ13>3.0.CO;2-7
Kaplan, A. M., and Haenlein, M. (2010). Users of the world, unite! The challenges and opportunities of social media. Bus. Horiz. 53, 59–68. doi: 10.1016/j.bushor.2009.09.003
Kline, R. B. Principles and practice of structural equation modeling. (3rd ed). Guilford Press. (2011).
Kwon, K. S., Park, E., and Kim, K. J. (2014). What drives successful social networking services? A comparative analysis of user acceptance of Facebook and Twitter. Soc. Sci. J. 51, 534–544. doi: 10.1016/j.soscij.2014.04.005
Lee, W., and Paris, C. M. (2016). How your emotions on Facebook can drive your attitudes and intentions to go to the special event? Tourism Travel Res. Assoc. 17. Available at: https://scholarworks.umass.edu/ttra/2010/Oral/17/?utm_source=scholarworks.umass.edu%2Fttra%2F2010%2FOral%2F17&utm_medium=PDF&utm_campaign=PDFCoverPages
Legris, P., Ingham, J., and Collerette, P. (2003). Why do people use information technology? A critical review of the technology acceptance model. Inf. Manag. 40, 191–204. doi: 10.1016/S0378-7206(01)00143-4
Li, F., Zhu, D., Lin, M. T., and Kim, P. B. (2024). The technology acceptance model and hospitality and tourism consumers’ intention to use mobile technologies: meta-analysis and structural equation modeling. Corn. Hospitality Quar. doi: 10.1177/19389655241226
Liao, C.-H., Tsou, C.-W., and Shu, Y.-C. (2008). The roles of perceived enjoy- ment and Price perception in determining acceptance of multimedia- on-demand. Int. J. Bus. Inform. 3:1.
Liebana-Cabanillas, F., and Alonso-Dos-Santos, M. (2017). Factors that deter- mine the adoption of Facebook commerce: the moderating effect of age. J. Eng. Technol. Manag. 44, 1–18. doi: 10.1016/j.jengtecman.2017.03.001
Lister, M. (2017). Available at: http://www.wordstream.com/blog/ws/2017/01/05/social-media-marketing-statistics
Mcafee, A. P. (2006). Enterprise 2.0: the dawn of emergent collaboration. MIT Sloan Manag. Rev. 34, 38–28. doi: 10.1109/EMR.2006.261380
Menon, S., and Soman, D. (2002). Managing the power of curiosity for effective web advertising strategies. J. Advert. 31, 1–14. doi: 10.1080/00913367.2002.10673672
Moon, J., and Kim, Y. (2001). Extending the TAM for a world-wide-web context. Inf. Manag. 38, 217–230. doi: 10.1016/S0378-7206(00)00061-6
Moore, G. C., and Benbasat, I. (1991). Devlepmen an t of an instrument to measure perceptions of adopting information technology innovation. Inf. Syst. Res. 2, 192–222. doi: 10.1287/isre.2.3.192
Morschheuser, B., Hamari, J., Koivisto, J., and Maedche, A. (2017). Gamified crowdsourcing: conceptualization, literature review, and future agenda. Int. J. Hum. Comput. Stud. 106, 26–43. doi: 10.1016/j.ijhcs.2017.04.005
Neill, A. R., and Puettmann, K. J. (2013). Managing for adaptive capacity: thinning improves food availability for wildlife and insect pollinators under climate change conditions. Can. J. For. Res. 43, 428–440. doi: 10.1139/cjfr-2012-0345
O’reilly, T., (2005). Available at: http://www.oreillynet.com/1pt/a/6228
Phang, C. W., Kankanhalli, A., and Sabherwal, R. (2009). Using text mining techniques for extracting information from research articles. Intell. Natural Langu. Process.Trends Appli. 373–397.
Qin, C., Liu, Y., Mou, J., and Chen, J. (2019). User adoption of a hybrid social tagging approach in an online knowledge community. Aslib J. Inf. Manag. 71, 155–175. doi: 10.1108/AJIM-09-2018-0212
Rana, N. P., Dwivedi, Y. K., Williams, M. D., and Weerakkody, V. (2016). Adoption of online public grievance redressal system in India: toward developing a unified view. Comput. Hum. Behav. 59, 265–282. doi: 10.1016/j.chb.2016.02.019
Rauniar, R., Rawski, G., Johnson, B., and Yang, J. (2013). Social media user satisfaction—theory development and research findings. J. Internet Commer. 12, 195–224. doi: 10.1080/15332861.2013.817864
Rauniar, R., Rawski, G., Yang, J., and Johnson, B. (2014). Technology acceptance model (TAM) and social media usage: an empirical study on Facebook. J. Enterp. Inf. Manag. 27, 6–30. doi: 10.1108/JEIM-04-2012-0011
Saleem, A. N., Noori, N. M., and Ozdamli, F. (2022). Gamification applications in E-learning: a literature review. Technol. Knowl. Learn. 27, 139–159. doi: 10.1007/s10758-020-09487-x
Salloum, S. A., Al-Emran, M., Monem, A. A., and Shaalan, K. (2018). Using text mining techniques for extracting information from research articles. Intelligent natural language processing: Trends and Applications. 373–397. Available at: https://link.springer.com/chapter/10.1007/978-3-319-67056-0_18
Sangi, N., Shuguang, L., and Sangi, A. R. (2018). Robustness of factors influencing social media usage/adoption amongst SMEs in developing countries: a case of Pakistan. In Proceedings of the 9th International Conference on E-Education, E-Business, E-Management and E-Learning. 103–109.
Shaalan, M. A. (2019). Understanding the quality determinants that influence the intention to use the mobile learning platforms: a practical study. Int. J. Interact. Mobile Technol. 13:11. doi: 10.3991/ijim.v13i11.10300
Shafique, K., Khawaja, B. A., Sabir, F., Qazi, S., and Mustaqim, M. (2020). Internet of things (IoT) for next-generation smart systems: a review of current challenges, future trends and prospects for emerging 5G-IoT scenarios. IEEE Access 8, 23022–23040.
Shin, D. H. (2010). Analysis of online social networks: a cross-national study. Online Inf. Rev. 34, 473–495. doi: 10.1108/14684521011054080
Shin, D. H., and Kim, W. Y. (2008). Applying the technology acceptance model and flow theory to cyworld user behavior: implication of the web 2. 0 user acceptance. Cyberpsychol. Behav. 11, 378–382. doi: 10.1089/cpb.2007.0117
Statista (2022). Available at: https://www.statista.com/statistics/272014/global-social-networks-ranked-by-number-of-users/
Urbach, N., and Ahlemann, F. (2010). Structrual equation modeling in in- formation systems research using partial least squares. J. Inform. Technol. Theory. Appli. (JITTA), 11:2.
Van-der-Heijden, H. (2004). User acceptance of hedonic information systems. MIS Q. 28, 695–704. doi: 10.2307/25148660
Venkatesh, V., Morris, M. G., Davis, G. B., and Davis, F. D. (2003). User acceptance of information technology: Toward a unified view. MIS quarterly. eds. V. Venktatesh and F. D. Davis, 425–478. Available at: https://www.jstor.org/stable/30036540
Venkatesh, V., Speir, C., and Morris, M. G. (2000). Available at: https://onlinelibrary.wiley.com/doi/abs/10.1111/j.1540-5915.2002.tb01646.x
Keywords: Facebook, technology acceptance, perceived enjoyment, perceived connectedness, structural equation (SEM), technology adoption, perceived usefulness (PU), perceived ease of ICT usage
Citation: Allam H, Dempere J, Lazaros EJ and Davison C (2024) Empowering engagement: unveiling motivational drivers for social media adoption: a Facebook case study. Front. Comput. Sci. 6:1387291. doi: 10.3389/fcomp.2024.1387291
Received: 17 February 2024; Accepted: 27 March 2024;
Published: 10 May 2024.
Edited by:
Cesar Collazos, University of Cauca, ColombiaReviewed by:
Manuel Bolaños, University of Nariño, ColombiaCopyright © 2024 Allam, Dempere, Lazaros and Davison. This is an open-access article distributed under the terms of the Creative Commons Attribution License (CC BY). The use, distribution or reproduction in other forums is permitted, provided the original author(s) and the copyright owner(s) are credited and that the original publication in this journal is cited, in accordance with accepted academic practice. No use, distribution or reproduction is permitted which does not comply with these terms.
*Correspondence: Hesham Allam, SGVzaGFtLmFsbGFtQGJzdS5lZHU=
Disclaimer: All claims expressed in this article are solely those of the authors and do not necessarily represent those of their affiliated organizations, or those of the publisher, the editors and the reviewers. Any product that may be evaluated in this article or claim that may be made by its manufacturer is not guaranteed or endorsed by the publisher.
Research integrity at Frontiers
Learn more about the work of our research integrity team to safeguard the quality of each article we publish.