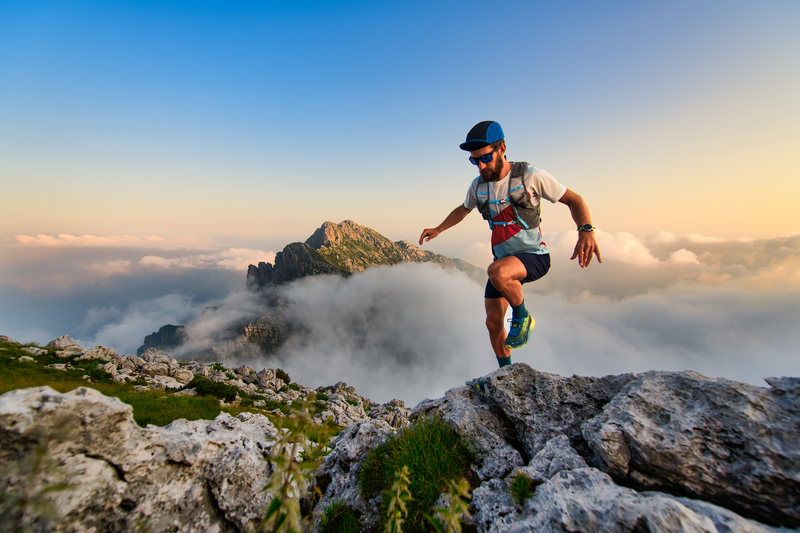
95% of researchers rate our articles as excellent or good
Learn more about the work of our research integrity team to safeguard the quality of each article we publish.
Find out more
ORIGINAL RESEARCH article
Front. Comput. Sci. , 04 June 2024
Sec. Networks and Communications
Volume 6 - 2024 | https://doi.org/10.3389/fcomp.2024.1382347
This article is part of the Research Topic Industry-Oriented Interfaces: Bridging the Gap Between Humans and Machines in the Context of Industry 4.0 in the Generative AI Era View all 5 articles
The Internet of Things (IoT) proposes to transform human civilization so that it is smart, practical, and highly efficient, with enormous potential for commercial as well as social and environmental advantages. Reliability is one of the major problems that must be resolved to enable this revolutionary change. The reliability issues raised with specific supporting technologies for each tier according to the layered IoT reliability are initially described in this research. The research then offers a complete review and assessment of IoT reliability. In this paper, various types of reliability on the IoT have been analyzed with each layer of IoT to solve the issues of failure rates, latency, MTTF, and MTBF. Each parameter has a certain classification and perception as well as enhancement in efficiency, accuracy, precision, timeliness, and completeness. Reliability models provide efficient solutions for different IoT problems, which are mirrored in the proposed study and classified with four types of reliabilities. The field of IoT reliability exploration is still in its initial phases, despite a sizable research record. Furthermore, the recent case study of CHISS is elaborated with discovered behaviors including brand-new aspects such as the multifaceted nature of evolving IoT systems, research opportunities, and difficulties.
The Internet of Things (IoT) is a brand-new ideology that changes the conventional way of living based on technology (González-Vidal et al., 2019). Some of the advancements made possible by IoT include smart homes, smart cities, smart transportation, smart energy management, and even smart industries (Ergun et al., 2020a,b). To create technologies employing IoT, several important studies and research projects have been carried out. If the potential of IoT is to be fully fulfilled, there remain a significant number of concerns and obstacles which must be resolved. These challenges as well as issues must be considered from a variety of IoT viewpoints, including uses, difficulties, technology that enables them, and repercussions on society and the environment (Sharma et al., 2020, 2021; Indira et al., 2023). The major objective of this evaluation article was to provide a thorough examination from both a technical and a sociological standpoint. The study examines many difficulties, urgent problems, design, and significant application sectors of IoT. The article similarly discusses recent literature, but it does so by demonstrating how it has influenced specific IoT components. In addition, reliability significance and case studies concerning IoT have indeed been covered (Kim, 2016; Thomas and Rad, 2017; Barak et al., 2020; Hulme et al., 2022; Kazemi and Ansari, 2022; Kholmirzayev et al., 2022; Kou et al., 2022). Readers will find it simpler to understand how the IoT works in the actual world after reading this page (Metsämuuronen, 2022; Najafzadeh et al., 2022). Figure 1 depicts the scientific design of reliability in IoT with exploratory analysis. In this current proposed study, various types of reliability on the IoT have been analyzed with each layer of IoT to solve the issues of failure rates, latency, MTTF, and MTBF. Each parameter has a certain classification and perception as well as enhancement in efficiency, accuracy, precision, timeliness, and completeness.
Several layered models exist for IoT systems. A four-layered architecture has been considered to make the talks of dependability and remedies in this article in a more convenient manner. The four standard layers are the perception or sensing layer, communication or transport layer, support layer, and application or service layer (Karthikeyan and Poongodi, 2023). Examples of these layers not only include sensors as well as sensor networks, wired and wireless networks, and cloud computing but also storage area networks (e.g., smart healthcare and home automation). The reliability of the specific technologies used throughout all of these tiers poses problems with obstacles, and the perception layer often includes numerous installed cluster heads that should carry out numerous metrics (e.g., temperature, humidity, ECG, and EMG). The IoT systems in general, including sensor nodes, are multimodal having a variety of sensing, computing, and communication, as well as coverage capabilities having characteristics, leading to a variety of failure or dependability behaviors. In addition, for certain IoT, a huge number of sensor nodes are installed. Applications make the conventional techniques for assessing network reliability difficult to use (Mavrogiorgou et al., 2018) (applicable to networks of small or moderate size). IoT devices, especially those working in challenging as well as unmanaged situations, are prone to failure because of limited resources (power, processing, storage, and communication capacity) (Catelani et al., 2021). They often use wireless networks for communication, which are similarly prone to generating errors because of disturbances, and channel fades through transmission attenuations. Missteps in IoT gadgets and network connections have a significant impact here on the architecture of IoT networks as well as communications. IoT systems are becoming more potent as well as sophisticated because of developments in numerous IoT-supporting technologies (Maratha et al., 2020, 2021). However, when the components of system cooperate as well as interact more, new potentially unidentified dependencies arise. For instance, many elements at the support layer could act in a dependent manner. In particular, in other words, it depends on the switch concerning their function (FDEP) as in an IoT storage area network location or is accessible through fiber channel switches (Mishra et al., 2023). Servers linked to switches that fail become unavailable or isolated in the event of a switch failure. A switch is known as the trigger element in this FDEP connection, whereas the servers and storage arrays are often mentioned as the components of the connection. A cloud-based RAID offers FDEP on the RAID controller for the disk arrays (redundant matrix of separate disks) in a disk drive (Stiawan et al., 2016). This program includes the FDEP behavior layer. For instance, an energy storage unit of smart home has solar panels that have FDEP (ESS); if the ESS is not functioning correctly, the energy that the panels generate is wasted. In the aforementioned scenarios, the FDEP occurs deterministically. In the IoT system, it may potentially occur probabilistically (Saini, 2016). For instance, a sensor that often broadcasts its observed information to a base station, as well as a sink node via a relay node, produces FDEP as a trigger on the relays (Nandan and Nalini, 2023; Yusof et al., 2023). If the relay node fails, its sensor might increase the signal it transmits to allow a clear relation to the ground station and the mobile. The amount of remaining battery life is what matters, however. In this case, the sensor may not necessarily become isolated when the relay fails. In both deterministic and probabilistic FDEP systems, competition between the different trigger and dependent potential error types, particularly between local trigger component failures vs. propagating dependent component failures, may occur. In contrast with a local failure, which only affects the injured component directly, a propagating failure can cause significant harm or even put the whole system to a halt (Brogi and Forti, 2017). Depending on the moment it occurs, a propagated failure in the FDEP system coming from dynamic effects might occur with a reliant component. There is a failure spreading impact, which may result in the system being crashed if it occurs before the regional collapse of the relevant trigger element. Early localized failure of the trigger isolates all the interconnected parts, avoiding the possibility of spreading failures impacting the rest of the network (either probabilistically or deterministically). For the Internet of Things, these dynamic sets of images provide unique challenges. Standby spares are frequently used for important IoT devices to improve fault tolerance and availability (Maratha and Gupta, 2019, 2022, 2023). Three alternative standby modes—cold, hot, and warm—are available based on the amount of recovery time required and resource limitation (Li et al., 2021; Baber and Young, 2022). A cold standby component is kept switched off and often has a 0% failure rate prior to usage; nonetheless, it takes a lengthy time to recover if the primary online component fails to restore system functionality. Even though it uses the same resources and therefore has a similar failure rate, a heated standby element in the base structure joins up with it to enable swift recovery. A heated backup element exhibits a reduced failure rate while still being partially powered before being triggered to replace the malfunctioning main component. The backup systems that have dynamic/changing failure rates before and following their activation are not covered by the conventional reliability models that assume static component failure rates and processes. The phased-mission characteristic applies to the IoT as well. The system may be required to perform a variety of tasks throughout a variety of periods that call for a variety of system capabilities or components. These components may be subjected to a multitude of environmental conditions, such as stress, which might result in a variety of failure rates or processes (Behera et al., 2015).
For instance, a smart home power generating system will combine conventional electricity and solar energy. As the brightness of sun changes over time, so do the capacities of solar panels to produce energy. In addition, additional energy of vitality from the solar cells is adequate to power the wiring cupboard of this particular home automation system whether it is stored or transferred toward the utility network during some hours (such as the afternoon). However, during other hours (such as the evening), these same solar panels may stop working, necessitating the use of both stored electricity and energy from the grid system to power the smart home. Another example is when an individual wears an internal sensor device to have their movements plus physiological data tracked (Agarwal et al., 2022). This person's day is divided into two phases: active daytime activity and inactive nighttime slumber. Biosensors detect just the physiological data (such as blood pressure and heart rates) during the night phase, whereas motion sensors and biosensors, respectively, track a combination of physiological and motion data, during the day phase. Different subsets of system parts (such as sensors) influence the system operation in both instances at different times, necessitating a unique dependability that can be seen in Figure 2.
In this paper, an evaluation of all the different reliabilities on the IoT based on network, device, system, and anomaly with how their work is feasible and an assessment of reliability on the IoT and reliability models has been done.
The shares of the world for the market size in the IoT have been analyzed. It is evident when compared to certain other IoT initiatives, those centered on the industry, smart cities, smart energy, and especially smart cars have a substantial share of the market. One of the most common IoT use cases is this smart city, which incorporates smart homes. IoT-enabled home appliances, a climate control system, a television, and audio media players, make up a smart home, plus security systems to provide the greatest levels of comfort, safety, and energy efficiency. The Internet is used for all the interactions. The Internet of thing is used for all the interactions which are IoT-based centralized control system. In December, the concept of a “smart city” gained popularity and attracted a great deal of study. The smart home industry is anticipated to exceed $1 trillion by 2022 (De et al., 2022). The advantages of a smart home extend beyond internal comfort and include financial savings in a variety of areas. A decreased power bill will be the outcome of less energy use (Yadav et al., 2021a,b). Together with smart homes, smart cities also feature a subcategory for modern vehicles. Most intelligent devices and sensors manage every component in contemporary cars from the lights to the motor. The IoT is committed to developing new smart car technologies that combine wireless technology among automobiles and between cars and the users of those cars to offer predictive maintenance with enjoyable and secure driving dynamics (Nõmm and Bahşi, 2018; Akhmedov, 2022).
Khajenasiri et al. (2017) conducted research on IoT networks with smart energy control to support applications in connected cities. It has been investigated that just a few application sectors have so far leveraged IoT to benefit both technology and people. Because of its vast applicability, IoT has the potential to engulf almost all industries in the near term. It has been claimed that preserving energy is one of the most crucial aspects of civilization as well as that the IoT may help create a clever power management system that might dramatically reduce usage costs. IoT design was addressed in connection with the concept of smart cities. The scientist noted how the lack of development in software and hardware for the IoT makes reaching this goal one of the more difficult tasks. It has been proposed that these problems needed that be fixed to guarantee an effective, dependable, yet user-friendly IoT system.
Alavi et al. (2018) explored the issue of city urbanization. Cities are becoming more populous because of people shifting from rural to urban areas. As a result, smarter solutions for energy, infrastructure, and healthcare, including transportation are required. The smart city is one of the most important applications for IoT developers. It examines a variety of issues, such as smart public safety solutions, smart parking, smart lighting, smart waste management, smart garbage collection, including smart air quality management. It has been investigated how hard IoT is working to solve these challenging issues. Opportunities for entrepreneurs in the field of smart city technology have been generated by increasing urbanization as well as the desire for improved infrastructure. IoT-enabled technology, according to the authors, is essential for the development of sustainable smart cities.
IoT security and privacy are major issues that need attention and in-depth research (Singh et al., 2021, 2023a,b,c,d). It was stated that client confidentiality, data identification, and security systems, including attack resilience, should all be included in business processes by private enterprises employing IoT. Weber (2010) focused on these concerns and stated that doing so would be advantageous. Works review the reliability assessment of each layer on the IoT has been shown in Table 1.
As was covered in the preceding study, there is a significant amount of quantification in the notion of dependability. As the definition of reliability states that it is not a biased science, techniques intended to measure dependability should be unbiased but quantitative. Determining yet another aspect of reliability that gains a lot of focus is the use of measures to evaluate the reliability of extra parts of the system. IoT reliability research has been done to improve reliability at many levels of the Protocol stack. The study examined important topics for improving IoT, including device and data quality, and network stability, including anomaly detection. Reliability is summarized in this study. Figure 3 demonstrates different types of reliability in the IoT. Various types of reliability issues involved in IoT such as hardware reliability ensure high-quality components, rigorous testing, redundancy where critical, continuous health monitoring, and proactive maintenance prevent hardware failures (Luo et al., 2022). Network reliability employs redundant connections, robust protocols, quality of service (QoS) mechanisms, ongoing performance monitoring, and swift resolution of network issues to maintain reliable connectivity (Li X. Q. et al., 2022; Yeh et al., 2022; Singh et al., 2023e,f). Data reliability implements encryption, access controls, data validation, and backups to ensure data integrity, with regular audits and disaster recovery plans to handle data loss or corruption. Software/firmware reliability follows best development practices, deploys updates securely, implements error handling, monitors performance metrics, and responds promptly to software/firmware issues for reliable operation. Power supply reliability utilizes reliable power sources, manages power efficiently, monitors power systems, and has backup power solutions in place to maintain continuous operation despite power disruptions. By addressing these reliability concerns comprehensively through a combination of hardware design, software development, network management, and operational strategies, IoT systems can achieve high levels of reliability, performance, and resilience in various environments and IoT use cases.
Integrating the studies of many authors on IoT devices such as dependability and integrating traditional dependability measures into IoT-focused solutions. Zin et al. (2016) measured reliability, failure rate, availability, and overall MTTR. The study showed a probabilistic model for evaluating the dependability of connected IoT devices, arguing that their failure structures follow a particular likelihood distribution. The authors claim that the likelihood that perhaps the gadget is in good working order at the interval [0, t] is the reliability measure R(t). This probabilistic function enables an estimate of predicted time to failure, availability, and overall reliability of a particular IoT device. In contrast, Mavrogiorgou et al. (2018) developed a framework enabling the collection of diverse IoT device dependability and the inclusion of mean repair time (MTTR), MTTF, MTBF, as well as accessibility metrics in their study. This method included both known and unidentified device types and attempted to distinguish between reliable and unreliable devices to gather info from trustworthy sources and disregard it from unreliable ones. The mechanism had four stages: systems identification, requirements categorization, reliability estimate, and then reliability validation. The authors were able to use this approach to rate connected fitness gadgets based on the results of defined reliability standards. Finally, Kharchenko et al. (2016) suggested a weighted methodology to assess reliability in the IoT plus employed reliability, failure rate, and recoverability in their research. The model had three quality standards: portability, functionality, and quality dependability. Metrics were created and assigned weights in line with these requirements so that a general assessment of the effectiveness of IoT application could be made by the model. The model was then evaluated in a virtual environment when scores were produced for each of the metrics. Even though this method promotes weighing, each criterion in this experiment was assigned the same amount of weight. Even though they are not completely developed and are unable to testify to dependability at all layers of the IoT architecture, these traditional metrics provide an excellent starting point in the evaluation of IoT reliability.
Going above unique dependability, lately, several novel reliability techniques have been created to be employed in investigations (Yadav et al., 2022). It provides a methodology to assess the reliability and trustworthiness of IoT systems over time (ROPT). Because of this idea similar IoT sensors may display varied predicted lifespan depending on the environment that is used in (e.g., exposed to variable degrees of humidity, temperature, and wind). To fully understand how dependable the system is, the author provides the ROPT computation for every single system, and every gateway inside the IoT system was proposed. The author also provided a trust that concludes that certain IoT applications, such as defense systems, needed greater availability levels as well as higher degrees of trust from the factor rating scale. The ability of research to accurately portray IoT reliability is constrained as only one signal was utilized to evaluate the dependability of the system. It also provided three quasi-reliability measure categories to measure the real-time data quality gathered from IoT devices. Using two actual open-source datasets, the research confirms the use of these measures. The three requirements were listed as being current, accessible, and valid. The ability of the measures to be created in real time was shown by applying them to real-world datasets, but this did not establish how well the metrics could identify poor data quality in IoT. Both Sicari et al. (2016a,b) provide a more thorough strategy for dependable quality control. This architecture is meant to gauge how well-performing various IoT devices are in terms of security. The method retrieved metadata from such an IoT node network using networked smart objects (NOS). The security-related criteria that were retrieved were integrity, privacy, secrecy, and appropriate authentication. Among the criteria utilized to assess quality were accuracy, precision, timeliness, and general completeness. An index score that varied from 0 to 1 was used to represent how well the nodes related to each parameter. The ability of the model to calculate the necessary values was evaluated using sensors from the meteorological department. This usage of quality data of the model to establish the data quality characteristics of IoT nodes is inadequate to clearly explain the degree of dependability of an IoT device. Safety meta-data offer certain details on a degree of security of a specific node in an IoT system (Sinche et al., 2018). To enhance this, anomaly detection could be used. The analysis presented in this study aids in shedding light on the dependability and susceptibility to failure of the parts of the IoT architecture. These studies provide light on the potential measurement of some of this data utilizing parameters, such as availability, MTBF, and MTTR. To address overall IoT reliability, monitoring hardware dependability is just one step in the process. These studies cannot assess the dependability of the network or the potential that the system can create anomalous data or give in to an increasing threat.
In addition, an urgent need to be able to verify the dependability of the routing protocol that serves as the foundation for IoT communication to have the ability to reason about how the IoT device functions. The findings of network tests that try to improve network QoS but those that measure network dependability measures are mostly covered in this study. The most recent, cutting-edge research on the dependability of IoT networks is presented in this area. To improve and quantify dependability for IoT applications, particularly crisis applications, Li and Huang (2017) introduced a unique IoT network QoS measure and created a light, energy-efficient routing method. All activated alerts for IoT emergency applications need a swift response. The study established AJIA, a method for assessing both route quality and packet loss (Adaptive Joint Protocol based on their Implicit ACK). The broadcast function of the protocol, which sends messages to all nearby nodes, is essential to the process. As a result, nearby nodes may “overhear” the communication in motion. Instead of using conventional ACK messages, this eavesdropping function is used to confirm the dependability of the message; thus, the credibility of each channel is evaluated using a measure known as the connectivity of the nodes using the linkage visual design (LQI), which also evaluates prior occurrences of packet loss in the network. Other QoS metrics that Kamyod (2018) monitored were delay throughput and packet loss. The network reliability indicators in a scenario including smart agriculture were tracked in this research using the optimized network engineering tools (OPNET) from Riverbed (Lyu and Yin, 2020). To get insight into the overall reliability of the end-to-end IoT system, these characteristics were monitored (Kamyod, 2018). The study found that packet delays, transmission times, and packet loss all substantially increased with network node density. Based on the fog computing paradigm, Brogi and Forti (2017) developed a general architecture for an IoT infrastructure that is QoS aware. The concept enables IoT applications to build QoS profiles as well as request certain QoS qualities from devices connected. The model can determine the potential latency and bandwidth for an application-to-object connection given the QoS profile that corresponds to each communication channel that exists in the IoT system. The only factor considered by the model is bandwidth throughput latency, which really only makes up a small fraction of the QoS requirements and does not accurately reflect the reliability of the network at any given time, including additional QoS indicators for IoT networks. A municipality QoS management framework (mQoSM), in the research by Al-Masri (2018), QoS-aware middleware was examined in connection with the actions of industrial IoT (IIoT) devices (Behera et al., 2015). The endpoints for choosing the “best” microservice among the ones that were discovered. With this knowledge, IoT architects may choose whether to implement the capability. The parameters that this framework tracks include response time, throughput, availability, dependability, and cost. Although the model has not been scaled up above services inside an IoT context, it offers a promising beginning toward developing a special awareness for the IoT system, especially concerning dependability and performance. Li and Huang suggested a method for modeling dependability using extended stochastic Petri nets (GSPN). This approach provides information on the performance of IoT devices using mathematical models at edge nodes. Time spent, response time, failure rate, and repair times were calculated measures. These metrics do not offer a comprehensive picture of IoT reliability as it has only addressed device-to-edge layer performance and therefore present a highly condensed perspective of network performance. A gateway redundancy model was suggested. In this experiment, redundancy at both the gateway (edge node) level and the Internet Service Provider (ISP) level was used. Three scenarios—an IoT infrastructure with redundant gateways, an IoT with redundant gateways, ISPs, and ISPs, and an IoT without redundant gateways—were each examined using this model. The model was evaluated using a real-world IoT testbed that utilized the I2C bus standard for device communication. The performance measure utilized to assess the efficacy of the model was return trip time (RTT). According to the study, although only by that much, the model without the need for a redundancy method increased the RTT under fault situations by 14%. It is 1% once more for redundancy models. Just the reliability of the cloud at the network level is considered in this study. As a result, it ignores the dependability of equipment and its susceptibility to failing at any moment. Moreover, the variety of IoT connectivity protocols is not taken into consideration in this research. This has proposed a TCP-based architecture to resolve the IoT reliability problems. TCP stands for transmission control protocol. The reliability calculator, reliability regulator, and reliability handler are the three parts of the framework. The framework takes advantage of the delay to identify an IoT system failure scenario. Whenever the reliability analysis detects significant amounts of delay, the reliability handler begins broadcasting and enters power-saving mode. The retransmission attempt will then be made by the reliability controller. This framework cannot accurately describe the degree of system dependability as it only considers the delayed quality of service of IoT (Nguyen et al., 2020). This study research shows that there are currently no study approaches that successfully combine device and network reliability within one framework, despite some attempts to increase reliability inside of IoT networks both through improving the QoS of network and by monitoring and calculating network reliability.
Moreover, to assess IoT system robustness, some studies have been done. Such techniques operate at a high level and do not consider the intricacies of dependability, such as the failure mechanisms of individual devices or even the network segments that cause traffic jams. A technique for modeling dependability in a service-oriented IoT has been discussed. Algorithms were especially used to evaluate the dependability of a centrally managed diverse IoT service system (CHISS). The authors suggested that to measure dependability, it may be possible to model the availability of the program required to execute the service, the accessibility of the data required for the service to run, and the serviceability of the related subsystems. To assess the algorithms, a case study of a fire detection system that was in operation at the time but was also regularly operating was employed. The algorithms may detect the program and file availability with each IoT system component. This strategy, meanwhile, disregarded the chance that malware or other danger may spread across the network or that certain IoT components could suddenly stop working and start producing inaccurate data. A technique that can warn the user of system defects before crucial decisions are taken is required to appropriately display dependability (Alam, 2018). To forecast the dependability needs of an IoT system, a Markov model was suggested. The Markov model considered a possible range of 15 states for the application, from the usual situation to total failure. The probabilistic character of the Markov model makes it possible to forecast how the system will change from one state to the next and to calculate the likelihood that it will fail at any given moment. This model is incapable of responding to novel scenarios that were not considered during model creation as it only considers the states that were defined during model design (Tsantilis et al., 2021).
Given that IoT networks are weak, their devices have limited capabilities, and these are highly mobile, any framework aiming to assess the dependability of an IoT infrastructure must be aware of the possibility that erroneous data would be included in its applications. If these abnormal data are delivered unnoticed to the protocol and utilized in crucial actuation situations, serious repercussions might follow. The latest recent studies on IoT anomaly detection are presented in this area. The need for portable solutions makes IoT-specific anomaly detection a challenging challenge given the variety of IoT devices (Bhatia et al., 2023). According to the network activity of the device, Spanos et al. (2019) suggested a smart-home outlier detection method that integrates statistical and machine learning approaches. Features are taken out of the network packet data during training, standardized, and then supplied to a clustering algorithm (Afshari et al., 2022). Then, the outcome of the soft voting is determined utilizing these cluster tags with ensemble classification techniques. The mechanical and physical degeneration of the gadgets was also acknowledged by the writers. To ascertain whether the model, in this case, applies to a larger number of devices and at scale, further information, including efficacy assessments, is needed. The research looked at techniques for identifying abnormalities in IoT time-series data. The scientist initially used the traits inferred from such models to remove outliers and aberrant patterns from the time-series data before categorizing the data into annotated categories. Although the classification part of the model made use of Random Forest as well as Association Rule Mining techniques, the time series anomaly detection component of the model made use of the ARIMA and HOT-SAX frameworks. The authors believe that these techniques might have a 90% accuracy rate. This study greatly advances the field of sensor anomaly detection, while being constrained by the fact that it needs time-series data to function.
A method for early anomaly finding via network traffic analysis was put in. This approach gathered messages from a variety of IoT devices using the Simple Network Mapping Protocol (SNMP). For further research, this traffic was then represented in graphs. In the network, the presence of an aberrant link might then be determined using thresholds based on CPU and memory use. This strategy is straightforward and appropriate for the IoT, but this does not seem to be a way to calculate a failure threshold automatically or statistically, which might lead to a lot of erroneous alerts. An IoT device-friendly anomaly detection technique with low-resource requirements was reported by Sedjelmaci et al. (2016). To enhance energy efficiency, the method combines two well-known methods for IoT intrusion detection—signature-based detection and anomaly detection. After the learning activity, to identify the anomaly by its signature as opposed to requiring to repeat the classifier to find it, the anomaly-based component creates a classification rule that is then sent to the component that detects signatures. Subsequently, to conserve even more energy, this hybrid technique used game theory, which pitted two “players”—the attacker who released the new attack signatures and the algorithm runner who noticed suspicious new signatures—against one another. When the contest is completed, previous data may be examined to determine whether a new signature is likely to appear, and this knowledge may be used to determine when anomaly detection should be carried out to generate new rules. Some well-known hybrid techniques from the academic literature were contrasted with the proposed lightweight game-theoretic strategy. Considering the predictive nature of the game-theoretic method, the study discovered that accuracy fell, as was expected. Yet, when comparing energy use, the study found that the resource-poor nature of IoT, using the lightweight technique, might save as much as 6,000 mJ of energy, so that's a substantial amount of energy. It has presented a technique for identifying abnormalities in the IoT by establishing a set of constraints again for applications utilizing property information (IoT) applications. Limits may be generated from the historical data of the data, such as the recommendation that temperature of a home should not increase over 30°C, or it may be triggered by, for instance, a motion sensor at work that ceases collecting data. Each time one of these limits is crossed, an unusual situation is created. This technique is effective for identifying blatantly strange circumstances, but it completely depends on the rule found that the sys admin develops. This restriction prevents the detection of anomalies that are not considered by the constraints. Based on the IoT fog computing architecture, Abeshu and Chilamkurti (2018) developed a deep learning technique for identifying threats. When used in mission-critical IoT applications (Herwin et al., 2022), the fog computing paradigm can drastically decrease latency when compared to the traditional cloud-centered paradigm. The research evaluated the efficacy of a deep learning model in contrast to a shallow learning model. The pre-trained stacked auto-encoder in feature engineering and SoftMax for classification were both added to the deep learning model.
According to the study, the accuracy of the deep model consistently performed better than that of the shallow model; on average, such an accuracy gap was 4%, which is a considerable difference in a scenario where precision is essential. The study also removes the threat of the network. To determine whether a node is abnormal or not, a statistical approach must be used. Further research on this strategy is required to produce a list of networking data to monitor that is more comprehensive. There is a method for identifying bot-net attacks on IoT settings (Moore et al., 2020). Before supplying the data to a classifier, a strategy looked at feature selection techniques to lessen the complexity of the data. The dataset utilized in the experiment included 115 discrete numerical characteristics produced by nine IoT devices and was drawn from a real dataset of a Mirai botnet assault. Source and destination IP, jitter, socket data, as well as other network measurements were among the features. The author used three distinct methods to lessen the dimensionality of the data: entropy, variance, and Hopkins statistics. The data were then classified using three classifiers: an IF, a one-class SVM (support vector machine), and an LOF (local outlier factor), a remote forest. The research found that utilizing only five characteristics, the IF classifier-coupled feature reduction with entropy, yielded accuracy results of 90%. This feature reduction is an effective machine learning technique for the IoT as it is far better ecologically friendly than having a classifier train and test 115 attributes. Despite its success in spotting abnormalities at the network level, this method of anomaly detection ignores the information included in packets sent out by IoT devices themselves. The accuracy of the shallower model declined by 2% when exposed to 80 or more fog nodes present; however, the deep model handled an increasing number of nodes far more easily. In their 2016 paper, Thanigaivelan et al. (2016) developed an IoT system where each node observes the behavior of its one-hop neighbors to identify abnormalities. The Metrics plus Grading Component (MGSS), the Reporting Subsystem (RSS), and the ISS are the three primary parts of the suggested system (Subsystem for Isolation). The component in charge of this task, the MGSS, assigns grades to nearby nodes depending on both packet size and data rate. Any nodes that are discovered to be abnormal must be reported to the RSS, which will subsequently alert the ISS component. The research community is unquestionably motivated to develop a more dependable IoT environment, according to the articles we have looked at currently on IoT anomaly detection. This highlights the importance of understanding that anomaly detection is a very wide area having applications within the IoT, network security, in addition to numerous computer-related domains. Although it would be difficult to review all available anomaly detection techniques within the constraints of this study, only the relevant IoT occurrences are carefully explored here. In the literature, anomaly detection techniques are examined in further detail. This study discussed a wide range of exact and interesting methods for identifying anomalies in IoT systems. Although it should be noted that the existence of an anomaly need not make it difficult or unattainable for IoT services to operate, it was stated that further research is needed to determine how anomalies truly influence the dependability of an IoT system. Anomalies, however, are a certain sign that the IoT system is no longer operating at its best. If the IoT is used to operate critical infrastructure, like security systems and important transportation networks, researchers must be able to quickly and accurately assess how reliable the system can work. The research by Maalel et al. (2013) highlights the need to pay close attention to apps that provide emergency workers and necessitate a prompt and trustworthy response. Understanding the reliability requirements for each location is also essential. A smart building system, for instance, could be able to tolerate delays of up to a few seconds. On the other hand, an industrial operation probably can only survive delays of a few microseconds. Research must address dependability across all the aforementioned vertical businesses to identify these needs and provide effective solutions. This study has shown the large variety of hardware and protocols that are accessible for use with IoT services. The creation of communication protocol standards is still going on because of efforts of several research organizations to create lighter but more efficient communication protocols. In addition, new IoT gadgets and accessories are available every day. Hence, the optimal dependability solution must be agnostic of the communication protocol, software, and hardware (Xu and Saleh, 2021).
One of the findings from the literature analysis was that even though end-to-end security scheme have been frequently used. This is no simple undertaking given the scope and that dependability several researchers had successfully addressed a specific issue, or set of difficulties, in IoT reliability research, no study had been conducted that had a thorough understanding complexity of developing IoT systems. Nevertheless, this does not imply that academics should attempt to create a dependable strategy that “fits all,” because doing so would be in opposition to the original path of investigation suggested in this paper. Instead, customized dependability guidelines tailored to each IoT sector should be developed while considering the IoT architecture as a whole. However, developing an end-to-end trustworthy IoT solution would be a significant and ground-breaking scientific discovery with the potential to significantly improve IoT end-user experience (Xing et al., 2017). IoT services anomalies have indeed been extensively researched in utilizing anomalies to integrate reliability information reported after research. Even while this effort is crucial and advantageous, it does not always lead to greater reliability without adopting additional measures. The user might not always be able to tell whether an anomaly has reduced the dependability of an IoT system. Thus, it is important to research the best way to integrate information about newly discovered problems in IoT systems with information about how dependability has been affected. For instance, if a sensor in a smart home is watching a scenario involving assisted living breaks, there may not be an existing problem right away. In contrast, there is a potential that dangerous equipment may malfunction if a temperature probe in a smart factory begins to provide misleading data (Maalel et al., 2013). Anticipate and avoid failure Current work goes into detail on the task of assessing dependability. If the research is to go beyond this goal, the role of predictive maintenance may be considered. If the researcher can quantify the reliability of a system, then consequently an accurate maintenance date can be obtained. It is also possible to further categorize this at the component level and transform it into a dynamic system that bases results on real-time effectiveness assessment, rather than relying on past failures to predict the future failure date. This research topic may have led to significant advancements in the field of IoT dependability study (Xing, 2020).
Many research initiatives recommended using high-performance computing machines or cloud platforms to deliver streaming data analytics. These streaming data analytics of frameworks are based on incremental processing and data parallelism. Data parallelism divides a huge dataset into numerous smaller datasets so that parallel analytics may be carried out on them all at once. When processing data incrementally, a stream of computing activities is used to swiftly handle a small batch of data. While these methods decrease the time it takes for the streaming automated analysis framework to respond, it has not been the ideal answer for IoT applications that must respond quickly. The requirement for data parallelism with incremental processing is less necessary by putting streaming data analytics near the data source (i.e., IoT devices or edge devices) as the amount of the data at the source enables it to be processed quickly. Fast analytics on IoT devices, however, come with their own set of difficulties, such as limited computation, storage, and power resources at the data source.
Four reliability models have been discussed so far in the current research study. Based on that, the first reliability model discusses the combination of component faults that caused the undesired event; the second is a dynamic fault tree that modeling of dynamic and dependable behavior; and the third is a binary decision diagram. Two-terminal reliability, k-terminal reliability, and all-terminal reliability became distinguished as indicated in Figure 3 and have connections with models that create an effective combinatorial framework enabling statistical analysis of fault trees to accomplish system reliability characteristics. Figure 4 demonstrates the reliability models of the IoT.
Fault trees are frequently utilized to comprehend the logical connection between a network failure and its causes. A graphical depiction of possible permutations of component defects that lead to the emergence of a predefined undesired event is called a fault tree (typically, a system error). “Gates,” which are objects that explain the logical connections among fault occurrences, are indeed the building blocks of a fault tree. Just the logic gates AND, OR, and K-out-of-N (sometimes known as a vote) are often employed in fault trees (Rajawat et al., 2022). Before the 1960s, reliability analysis often used fault tree analysis. Since the Challenger disaster in 1986, NASA has used FTA to evaluate the dependability of the system.
The typical fault tree shines at showing failure modes of a system, but when it comes to considering dynamic, highly dependent traits like functional dependency, it falls short. Dynamic fault trees (DFT) including dedicated gates have been used to mimic a variety of system dependencies (Sandelic et al., 2022). Since then, a wide range of dynamic systems throughout a wide range of industrial sectors have been represented by the DFT. The Function Dependency (FDEP) gate, one of the gates utilized in this study, simulates the occurrence of a trigger event that renders other concessionaries unavailable or useless as demonstrated in the example (Li S. et al., 2022). Figure 5 demonstrates the structure of an FDEP gate.
“An effective computational model for quantitatively analyzing fault trees to attain system reliability metrics is the binary decision diagram (BDD). A BDD is an if-then-else (ite) structured directed acyclic graph (DAG) founded upon Shannon's decomposition (1).”
If x, then F1, F2, and x must all have values of 1 or 0, respectively, according to Equation (1). A binary tree may be created to express this idea, with each node having two outgoing edges: else, expressed by a 0 edge and then symbolized by a 1 edge. It, therefore, indicates whether the relevant component is working or in a failed condition. Logical operations (AND and OR) were used to demonstrate BDD manipulations (Zhang et al., 2022). The dependability of binary governmental institutions, in which each component may exist in either a working or failing condition, has already been examined using BDD (Jia et al., 2022). After the BDD has been constructed, each path from the root up to the leaf node contains a unique mix of component failures and non-failures. A path that ends at leaf node 1 leads to system success as opposed to one that does so at leaf node 0. Right/then and left/else edges are linked to q (component failure probability) and 1 – q, respectively, on each node or component (component operating probability). To evaluate both the dependency and unreliability of the system, the probabilities for each route that leads from the root via leaf node 1 are put together.
The concept of reliability at two, k, and all terminals was defined by network probability and statistics (Ali et al., 2022). Two-terminal reliability is the possibility that two network components might contact over at least one reliable channel, whereas all-terminal reliability is indeed the likelihood that all potential pairings of network components would be able to communicate. The probability that all potential pairings of k components have been able to interact is known as k-terminal reliability. In this article, two-terminal reliability is considered in the evaluation of SAN dependability. The state-space enumeration methodology, the cut-set/tie-set method, the graph materials developed, and the BDD-based approach are indeed the four main methods of two-terminal reliability assessment. The BDD-based method may be effectively used to examine the reliability of large networks or systems.
The first step is to define specific metrics for each type of reliability (hardware, network, data, software/firmware, and power supply) that is relevant to the IoT case study. For example, in hardware reliability, metrics might include mean time between failures (MTBF), failure rate, or availability percentage. Collect data related to each reliability type from the IoT devices and systems involved in the case study. These data may include hardware failure logs, network uptime/downtime records, data integrity checks, software/firmware performance metrics, and power supply status reports. Analyze the collected data to evaluate the reliability of the IoT system across different dimensions. Use statistical methods, trend analysis, and comparative assessments against established benchmarks or industry standards to assess the reliability levels. Identify challenges or areas of concern within each type of reliability. This could involve pinpointing frequent hardware failures, network bottlenecks, data integrity issues, software bugs, or power supply vulnerabilities that affect system reliability. Conduct root cause analysis to understand why reliability issues are occurring. This may involve examining design flaws, implementation errors, environmental factors, user behaviors, or external threats that contribute to reliability challenges. Based on the findings, propose recommendations and solutions to improve reliability in each area. This could include hardware upgrades, network optimization strategies, data validation protocols, software/firmware updates, power management enhancements, or redundancy implementations (Yadav and Yadav, 2019; Yadav et al., 2019, 2021b, 2023; Yadav, 2020). Implement the proposed solutions and validate their effectiveness through testing and monitoring. This may involve simulation tests, real-world deployment trials, performance monitoring tools, and feedback mechanisms to ensure that reliability improvements are achieved. Document the entire study process, including data collection methods, analysis techniques, findings, recommendations, implemented solutions, and their impact on reliability. Prepare a comprehensive report or presentation summarizing the case study results and lessons learned. By following these steps, researchers or practitioners can systematically study and address different types of reliability challenges within an IoT case study, leading to more robust and dependable IoT systems.
The connection between the two apps is established in the first step of an IoT service reliability analysis based on a SOC case study. This research demonstrated the way the IoT may well be utilized for two subsystems: an intelligent health service and a fire detection system. This is in line with its original purpose.
Figure 5 depicts the generalized structure of the centralized heterogeneous network service system (CHISS). The IoT service system consists of two subsystems that fall under two different IoT subcategories: the intelligent fire alarm system falls under the home/personal category, and the smart healthcare system falls under the community category (Su, 2011). According to the files needed to carry out the stated services of the subsystems as needed by the end users and as demanded by the scenario, many components and their relationships of a fire alarm system are graphically displayed in subsystem 1 of Figure 6. An inclusive view of IoT subsystems considers the various components and layers that make up an IoT ecosystem, ensuring that all elements work together seamlessly to achieve the desired functionality and reliability. Various layers such as the perception layer, network layer, middleware layer, application layer, business layer, security layer, and management and control layer. This layer includes sensors, actuators, and other devices that interact with the physical world, collecting data and sending it to the IoT system for processing. The network layer consists of communication protocols and technologies that enable data transfer between devices, gateways, and the cloud. This layer ensures reliable and secure data transmission. Middleware provides a communication bridge between the network and application layers, handling data processing, device management, and protocol translation. This layer includes the applications and services that use the data collected by IoT devices to provide value to users. It also includes data analytics and machine learning algorithms for data processing. The business layer encompasses the business processes, models, and strategies that drive the IoT implementation. It includes aspects such as monetization, scalability, and sustainability of the IoT solution. Security is a critical aspect of IoT subsystems, encompassing measures such as authentication, encryption, and access control to protect data and devices from unauthorized access and cyber threats. This layer includes tools and mechanisms for managing and controlling IoT devices and systems, such as device provisioning, firmware updates, and troubleshooting. An inclusive view of IoT subsystems ensures that all these layers work together cohesively to create a reliable, secure, and efficient IoT ecosystem that meets the needs of users and organizations. The same applies to intelligent healthcare systems. The application and data required by other devices determine how a device connects to the network (Kou et al., 2022). This is an illustration of how the IoT may function in a smart home design that makes use of several sensors. The article uses a fire alarm service as an example. To do this, regular data collection from temperature sensors and smoke sensors is performed. A continuous analysis determines whether the heat recorded either by the temperature sensor is more than the threshold or whether the suggested smoke might lead to choking. A “no danger” signal is sent, and the stage is transferred back to the sensor and detector if the data analyzed by the analyzer once again falls short of the threshold for the temperature probe with a smoke detector. The analyzer turns on the fire alarm and the water sprinkler when any or both requirements or even just one are met. A water tank is connected to the sprinkler. The water sprinkler as well as the alarm starts working simultaneously after activation. The analyzer, which also keeps an eye on the temperature as well as smoke level at that time, continuously provides input to the sprinkler as well as the alarm. As it drops underneath the threshold, the water supply is automatically cut off, and the alert is turned off. Figure 6 depicts the inclusive view of IoT subsystems.
According to the previous definition, an adaptive fire alarm system may be implemented to use a common network architecture, the app team, including files that provide the indicated services to the customers. The second part of our technique was finding the association utilizing the files required by each program. Figure 7 displays six elements of a fire emergency, designated by the letters N1 through N6. TP and CP signify each component, the thread, and the case as shown separately. As such, based on Figure 7, we can infer that Thread1 as well as Case C1 is required for the temperature sensors to function properly. The abbreviations in use in Figure 7 are listed in Table 2. Table 2 shows which node in Figure 6 runs various applications as well as holds which documents.
Just at the third stage of the algorithm, we assumed that the necessary programs are always nearby, meaning that the chance of accessibility is always 1. Hence, to calculate the IoT service reliability Rs, just the value of degrees Celsius, which depends on the whole execution tree of each system, must be computed (TB). Each subsystem's geometric mean is thus the Cinal value, and the dependability of each subsystem relies just on the execution tree (Herwin et al., 2022).
The empirical case study validates the importance of reliability in IoT systems. The proposed design approach offers a practical solution for ensuring reliability. Recommendations for future research include further exploration of advanced technologies for enhancing IoT reliability. The case study demonstrates the effectiveness of the designing approach in improving reliability in IoT systems. Comparison with existing methods highlights the innovative aspects of the proposed approach. Implications for the design and development of reliable IoT systems are discussed, emphasizing the need for proactive measures. The designing approach focuses on three key components: redundancy, fault tolerance, and predictive maintenance. Implementing redundancy in critical components to ensure system operation even in case of failure building fault-tolerant mechanisms to detect and recover from faults without affecting system functionality. Using data analytics to predict potential failures and perform maintenance proactively. In the case studies, the implementation selection of IoT applications has been analyzed critically. A smart building management system was selected for the case study. Implemented redundancy in HVAC systems, fault tolerance in lighting controls, and predictive maintenance in elevator systems have been analyzed. The approach significantly improved system reliability, reducing downtime and maintenance costs. The case study demonstrates the effectiveness of the designing approach in improving reliability in IoT systems. Comparison with existing methods highlights the innovative aspects of the proposed approach. Implications for the design and development of reliable IoT systems are discussed, emphasizing the need for proactive measures. Research on IoT reliability contributes significantly to the advancement and innovation of IoT technologies in several ways such as enhanced system performance, optimized resource utilization, improved user experience, security and privacy enhancements, scalability and interoperability, business and industry impact, sustainability, and environmental benefits. Overall, the contribution and innovation of research on IoT reliability lie in creating robust, dependable, and resilient IoT systems that deliver tangible benefits to users, businesses, industries, and society as a whole. These advancements drive the evolution of IoT technology and pave the way for its widespread adoption and continued development.
In this paper, various types of reliability on the IoT have been analyzed with each layer of IoT to solve the issues of failure rates, latency, MTTF, and MTBF. Each parameter has a certain classification and perception as well as enhancement in efficiency, accuracy, precision, timeliness, and completeness. Although the end-to-end literature review was carried out, one thing has been discovered that despite the fact several researchers had successfully addressed a specific issue or set of difficulties in IoT reliability research, no study has been conducted that has a thorough understanding of the complexity of developing IoT systems and covers each problem that occurs related to reliability techniques, such as system reliability, device reliability, network reliability, and anomaly detection. This is not the same as the other approaches such as cloud computing environment, the internet of things, artificial intelligence, and machine learning. The problems with reliability extend to several other domains. A failure rate model, a dynamic failure rate model, a binary decision diagram, and a network reliability model are some of the other kinds of reliability models that have been covered. Each model contributes to the solution of a reliability challenge, such as k out of n component failure problems; dedicated gates have been utilized to imitate a range of system dependencies; and two terminal reliability evaluations have been performed. Each model resolves 90% of reliability issues with the greatest possible outcomes. A case study relating to service reliability has been carried out by using a centralized heterogeneous Network service system (CHISS), which assists in lowering the network failure rate and maintaining network devices in a range of specified states.
The raw data supporting the conclusions of this article will be made available by the authors, without undue reservation.
KS: Writing—review & editing, Writing—original draft, Investigation, Conceptualization. MY: Writing—review & editing, Writing—original draft, Investigation, Conceptualization. YS: Writing—review & editing, Writing—original draft, Investigation, Conceptualization. DB: Writing—review & editing, Writing—original draft, Investigation, Conceptualization. AS: Writing—review & editing, Writing—original draft, Investigation, Conceptualization. FM: Writing—review & editing, Writing—original draft, Investigation, Conceptualization.
The author(s) declare that financial support was received for the research, authorship, and/or publication of this article. This study was supported by the FCT—Fundação para a Ciência e a Tecnologia, I.P. [Project UIDB/05105/2020].
The authors declare that the research was conducted in the absence of any commercial or financial relationships that could be construed as a potential conflict of interest.
All claims expressed in this article are solely those of the authors and do not necessarily represent those of their affiliated organizations, or those of the publisher, the editors and the reviewers. Any product that may be evaluated in this article, or claim that may be made by its manufacturer, is not guaranteed or endorsed by the publisher.
Abeshu, A., and Chilamkurti, N. (2018). Deep learning: the frontier for distributed attack detection in fog-to-things computing. IEEE Commun. Mag. 56, 169–175. doi: 10.1109/MCOM.2018.1700332
Afshari, S. S., Enayatollahi, F., Xu, X., and Liang, X. (2022). Machine learning-based methods in structural reliability analysis: a review. Reliab. Eng. Syst. Saf. 219:108223. doi: 10.1016/j.ress.2021.108223
Agarwal, V., Tapaswi, S., and Chanak, P. (2022). Intelligent fault-tolerance data routing scheme for IoT-enabled WSNs. IEEE Internet Things J. 9, 16332–16342. doi: 10.1109/JIOT.2022.3151501
Akhmedov, B. A. (2022). Analysis of the reliability of the test form of knowledge control in cluster education. Psychol. Educ. 59, 403–418. Available online at: https://rb.gy/s8n9
Alam, T. (2018). A reliable communication framework and its use in internet of things (IoT). CSEIT1835111, 450–456. Available online at: https://ssrn.com/abstract=3619450 (accessed September 14, 2023).
Alavi, A. H., Jiao, P., Buttlar, W. G., and Lajnef, N. (2018). Internet of Things-enabled smart cities: state-of-the-art and future trends. Measurement 129, 589–606. doi: 10.1016/j.measurement.2018.07.067
Ali, M. H., Kamel, S., Hassan, M. H., Tostado-Véliz, M., and Zawbaa, H. M. (2022). An improved wild horse optimization algorithm for reliability based optimal DG planning of radial distribution networks. Energy Rep. 8, 582–604. doi: 10.1016/j.egyr.2021.12.023
Al-Masri, E. (2018). “QoS-aware IIoT microservices architecture,” in 2018 IEEE International Conference on Industrial Internet (ICII) (Seattle, WA: IEEE), 171–172. doi: 10.1109/ICII.2018.00030
Baber, C., and Young, M. S. (2022). Making ergonomics accountable: Reliability, validity and utility in ergonomics methods. Appl. Ergon. 98:103583. doi: 10.1016/j.apergo.2021.103583
Barak, D. D., Singh, K., Ahlawat, P., and Sharma, H. K. (2020). “Real time tracking system: an IoT based application,” in 5th International Conference on Next Generation Computing Technologies (NGCT-2019). doi: 10.2139/ssrn.3545226
Behera, R. K., Reddy, K. H. K., and Roy, D. S. (2015). “Reliability modelling of service oriented Internet of Things,” in 2015 4th International Conference on Reliability, Infocom Technologies and Optimization (ICRITO) (Trends and Future Directions) (Noida: IEEE), 1–6. doi: 10.1109/ICRITO.2015.7359216
Bhatia, S., Goel, A. K., Naib, B. B., Singh, K., Yadav, M., and Saini, A. (2023). “Diabetes prediction using machine learning,” in 2023 World Conference on Communication and Computing (WCONF) (IEEE), 1–6. doi: 10.1109/WCONF58270.2023.10235187
Brogi, A., and Forti, S. (2017). QoS-aware deployment of IoT applications through the fog. IEEE Internet Things J. 4, 1185–1192. doi: 10.1109/JIOT.2017.2701408
Catelani, M., Ciani, L., Bartolini, A., Del Rio, C., Guidi, G., and Patrizi, G. (2021). Reliability analysis of wireless sensor network for smart farming applications. Sensors 21:7683. doi: 10.3390/s21227683
De, P., Kar, S., Ambure, P., and Roy, K. (2022). Prediction reliability of QSAR models: an overview of various validation tools. Arch. Toxicol. 96, 1279–1295. doi: 10.1007/s00204-022-03252-y
Ergun, K., Yu, X., Nagesh, N., Cherkasova, L., Mercati, P., Ayoub, R., et al. (2020a). “Simulating reliability of IoT networks with RelIoT,” in 2020 50th Annual IEEE-IFIP International Conference on Dependable Systems and Networks-Supplemental Volume (DSN-S) (Valencia: IEEE), 25–28. doi: 10.1109/DSN-S50200.2020.00019
Ergun, K., Yu, X., Nagesh, N., Cherkasova, L., Mercati, P., Ayoub, R., et al. (2020b). “RelIoT: reliability simulator for IoT networks,” in In Internet of Things-ICIOT 2020: 5th International Conference, Held as Part of the Services Conference Federation, SCF 2020, Honolulu, HI, USA, September 18–20, 2020, Proceedings 5 (Cham: Springer International Publishing), 63–81. doi: 10.1007/978-3-030-59615-6_5
González-Vidal, A., Cuenca-Jara, J., and Skarmeta, A. F. (2019). “IoT for water management: Towards intelligent anomaly detection,” in 2019 IEEE 5th World Forum on Internet of Things (WF-IoT) (Limerick: IEEE), 858–863. doi: 10.1109/WF-IoT.2019.8767190
Herwin, H., Pristiwaluyo, T., Ruslan, R., and Dahalan, S. C. (2022). Do scoring techniques and number of choices affect the reliability of multiple-choice tests in elementary schools? Cypriot J. Educ. Sci. 17, 1258–1268. doi: 10.18844/cjes.v17i4.7149
Hulme, A., Stanton, N. A., Walker, G. H., Waterson, P., and Salmon, P. M. (2022). Testing the reliability and validity of risk assessment methods in human factors and ergonomics. Ergonomics 65, 407–428. doi: 10.1080/00140139.2021.1962969
Indira, P., Arafat, I. S., Karthikeyan, R., Selvarajan, S., and Balachandran, P. K. (2023). Fabrication and investigation of agricultural monitoring system with IoT and AI. SN Appl. Sci. 5:322. doi: 10.1007/s42452-023-05526-1
Jia, H., Peng, R., Yang, L., Wu, T., Liu, D., and Li, Y. (2022). Reliability evaluation of demand-based warm standby systems with capacity storage. Reliab. Eng. Syst. Saf. 218:108132. doi: 10.1016/j.ress.2021.108132
Kamyod, C. (2018). “End-to-end reliability analysis of an IoT based smart agriculture,” in 2018 International Conference on Digital Arts, Media and Technology (ICDAMT) (Phayao: IEEE), 258–261. doi: 10.1109/ICDAMT.2018.8376535
Karthikeyan, S., and Poongodi, T. (2023). “Secure and optimized communication in the internet of things using DNA cryptography with x.509 digital attributes. Int. J. Eng. Trends Technol. 71, 1–8. doi: 10.14445/22315381/IJETT-V71I3P201
Kazemi, M., and Ansari, M. R. (2022). An integrated transmission expansion planning and battery storage systems placement-a security and reliability perspective. Int. J. Electr. Power Energy Syst. 134:107329. doi: 10.1016/j.ijepes.2021.107329
Khajenasiri, I., Estebsari, A., Verhelst, M., and Gielen, G. (2017). A review on Internet of Things solutions for intelligent energy control in buildings for smart city applications. Energy Procedia 111, 770–779. doi: 10.1016/j.egypro.2017.03.239
Kharchenko, V., Kolisnyk, M., Piskachova, I., and Bardis, N. (2016). “Reliability and security issues for IoT-based smart business center: architecture and Markov model,” in 2016 Third International Conference on Mathematics and Computers in Sciences and in Industry (MCSI) (Chania: IEEE), 313–318. doi: 10.1109/MCSI.2016.064
Kholmirzayev, S., Akhmedov, I., Khamidov, A., Akhmedov, A., Dedakhanov, F., and Muydinova, N. (2022). Calculation of reinforced concrete structures of buildings based on the theory of reliability. Sci. Innov. 1, 1027–1032. doi: 10.5281/zenodo.7447650
Kim, M. (2016). A quality model for evaluating IoT applications. Int. J. Comput. Electr. Eng. 8:66. doi: 10.17706/IJCEE.2016.8.1.66-76
Kou, G., Yi, K., Xiao, H., and Peng, R. (2022). Reliability of a distributed data storage system considering the external impacts. IEEE Trans. Reliab. 72, 3–14. doi: 10.1109/TR.2022.3161638
Li, L., Jin, Z., Li, G., Zheng, L., and Wei, Q. (2012). “Modeling and analyzing the reliability and cost of service composition in the IoT: a probabilistic approach,” in 2012 IEEE 19th International Conference on Web Services (Honolulu, HI: IEEE), 584–591. doi: 10.1109/ICWS.2012.25
Li, S., Chi, X., and Yu, B. (2022). An improved particle swarm optimization algorithm for the reliability–redundancy allocation problem with global reliability. Reliab. Eng. Syst. Saf. 225:108604. doi: 10.1016/j.ress.2022.108604
Li, S., Cui, T., and Alam, M. (2021). Reliability analysis of the internet of things using Space Fault Network. Alex. Eng. J. 60, 1259–1270. doi: 10.1016/j.aej.2020.10.049
Li, S., and Huang, J. (2017). “GSPN-based reliability-aware performance evaluation of IoT services,” in 2017 IEEE International Conference on Services Computing (SCC) (Honolulu, HI: IEEE), 483–486. doi: 10.1109/SCC.2017.70
Li, X. Q., Song, L. K., and Bai, G. C. (2022). Recent advances in reliability analysis of aeroengine rotor system: a review. Int. J. Struct. Integr. 13, 1–29. doi: 10.1108/IJSI-10-2021-0111
Luo, C., Shen, L., and Xu, A. (2022). Modelling and estimation of system reliability under dynamic operating environments and lifetime ordering constraints. Reliab. Eng. Syst. Saf. 218:108136. doi: 10.1016/j.ress.2021.108136
Lyu, Y., and Yin, P. (2020). Internet of Things transmission and network reliability in complex environment. Comput. Commun. 150, 757–763. doi: 10.1016/j.comcom.2019.11.054
Maalel, N., Natalizio, E., Bouabdallah, A., Roux, P., and Kellil, M. (2013). “Reliability for emergency applications in internet of things,” in 2013 IEEE international conference on distributed computing in sensor systems (Cambridge, MA: IEEE), 361–366. doi: 10.1109/DCOSS.2013.40
Maratha, P., and Gupta, K. (2019). A comprehensive and systematized review of energy-efficient routing protocols in wireless sensor networks. Int. J. Comput. Appl. 44, 83–100. doi: 10.1080/1206212X.2019.1697513
Maratha, P., and Gupta, K. (2022). HFLBSC: heuristic and fuzzy based load balanced, scalable clustering algorithm for wireless sensor network. Wirel. Pers. Commun. 125, 281–304. doi: 10.1007/s11277-022-09550-z
Maratha, P., and Gupta, K. (2023). Linear optimization and fuzzy-based clustering for WSNs assisted internet of things. Multimed. Tools Appl. 82, 5161–5185. doi: 10.1007/s11042-021-11850-8
Maratha, P., Gupta, K., and Kuila, P. (2021). Energy balanced, delay aware multi-path routing using particle swarm optimisation in wireless sensor networks. Int. J. Sens. Netw. 35, 10–22. doi: 10.1504/IJSNET.2021.112885
Maratha, P., Gupta, K., and Luhach, A. K. (2020). Improved fault-tolerant optimal route reconstruction approach for energy consumed areas in wireless sensor networks. IET Wirel. Sens. Syst. 10, 112–116. doi: 10.1049/iet-wss.2019.0152
Mavrogiorgou, A., Kiourtis, A., Symvoulidis, C., and Kyriazis, D. (2018). “Capturing the reliability of unknown devices in the IoT world,” in 2018 Fifth International Conference on Internet of Things: Systems, Management and Security (Valencia: IEEE), 62–69. doi: 10.1109/IoTSMS.2018.8554720
Metsämuuronen, J. (2022). Attenuation-corrected estimators of reliability. Appl. Psychol. Meas. 46, 720–737. doi: 10.1177/01466216221108131
Mishra, A. R., Mishra, R., and Shukla, R. (2023). A cloud-centric real-time telemonitoring system for cardiac patients based on the internet of medical thing. Int. J. Eng. Trends Technol. 71, 105–119. doi: 10.14445/22315381/IJETT-V71I3P212
Moore, S. J., Nugent, C. D., Zhang, S., and Cleland, I. (2020). IoT reliability: a review leading to 5 key research directions. CCF Trans. Pervasive Comput. Interact. 2, 147–163. doi: 10.1007/s42486-020-00037-z
Najafzadeh, M., Homaei, F., and Mohamadi, S. (2022). Reliability evaluation of groundwater quality index using data-driven models. Environ. Sci. Pollut. Res. 29, 8174–8190. doi: 10.1007/s11356-021-16158-6
Nandan, R. R., and Nalini, N. (2023). An efficient approach for discovering objects in the internet of things using clue-based search engine. Int. J. Eng. Trends Technol. 71, 282–294. doi: 10.14445/22315381/IJETT-V71I3P229
Nguyen, T. A., Min, D., and Choi, E. (2020). A hierarchical modeling and analysis framework for availability and security quantification of IoT infrastructures. Electronics 9:155. doi: 10.3390/electronics9010155
Nõmm, S., and Bahşi, H. (2018). “Unsupervised anomaly based botnet detection in IoT networks,” in 2018 17th IEEE international conference on machine learning and applications (ICMLA) (Orlando, FL: IEEE), 1048–1053. doi: 10.1109/ICMLA.2018.00171
Rajawat, A. S., Bedi, P., Goyal, S. B., Shaw, R. N., and Ghosh, A. (2022). “Reliability analysis in cyber-physical system using deep learning for smart cities industrial IoT network node,” in AI and IoT for Smart City Applications, 157–169. doi: 10.1007/978-981-16-7498-3_10
Safaei, B., Monazzah, A. M. H., Bafroei, M. B., and Ejlali, A. (2017). “Reliability side-effects in Internet of Things application layer protocols,” in 2017 2nd International Conference on System Reliability and Safety (ICSRS) (Milan: IEEE), 207–212. doi: 10.1109/ICSRS.2017.8272822
Saini, N. K. (2016). “Trust factor and reliability-over-a-period-of-time as key differentiators in IoT enabled services,” in 2016 International Conference on Internet of Things and Applications (IOTA) (Pune: IEEE), 411–414. doi: 10.1109/IOTA.2016.7562762
Sandelic, M., Peyghami, S., Sangwongwanich, A., and Blaabjerg, F. (2022). Reliability aspects in microgrid design and planning: status and power electronics-induced challenges. Renew. Sustain. Energy Rev. 159:112127. doi: 10.1016/j.rser.2022.112127
Sedjelmaci, H., Senouci, S. M., and Al-Bahri, M. (2016). “A lightweight anomaly detection technique for low-resource IoT devices: a game-theoretic methodology,” in 2016 IEEE international conference on communications (ICC) (Kuala Lumpur: IEEE), 1–6. doi: 10.1109/ICC.2016.7510811
Sharma, H., Singh, K., Ahmed, E., Patni, J., Singh, Y., and Ahlawat, P. (2021). IoT based automatic electric appliances controlling device based on visitor counter. doi: 10.13140/RG.2.2.30825.83043
Sharma, H. K., Singh, K., Ahmed, M. E., Patni, J. C., Singh, Y., and Ahlawat, P. (2020). IoT based automatic electric appliances controlling device based on visitor counter. Int. J. Psychosoc. Rehabil. 24, 4186–4196. doi: 10.37200/V24I10/32891
Sicari, S., Cappiello, C., De Pellegrini, F., Miorandi, D., and Coen-Porisini, A. (2016a). A security-and quality-aware system architecture for Internet of Things. Inform. Syst. Front. 18, 665–677. doi: 10.1007/s10796-014-9538-x
Sicari, S., Rizzardi, A., Miorandi, D., Cappiello, C., and Coen-Porisini, A. (2016b). A secure and quality-aware prototypical architecture for the Internet of Things. Inform. Syst. 58, 43–55. doi: 10.1016/j.is.2016.02.003
Sinche, S., Polo, O., Raposo, D., Femandes, M., Boavida, F., Rodrigues, A., et al. (2018). “Assessing redundancy models for IoT reliability,” in 2018 IEEE 19th International Symposium on A World of Wireless, Mobile and Multimedia Networks (WoWMoM) (Chania: IEEE), 14–15. doi: 10.1109/WoWMoM.2018.8449816
Singh, K., Barak, D., and Singh, Y. (2021). IOT Chatbot in insurance. Int. J. Eng. Manag. Humanit. Soc. Sci. Paradigms 33, 12–16. doi: 10.37622/IJHSS/12.1.2022.1-12
Singh, K., Barak, D., and Singh, Y. (2023a). Reviewing the IOT systems reliability and accuracy. Pharma Innov. J. 12, 2775–2780. Available online at: https://rb.gy/sn4y
Singh, K., Singh, Y., Barak, D., and Yadav, M. (2023b). Comparative performance analysis and evaluation of novel techniques in reliability for internet of things with RSM. Int. J. Intell. Syst. Appl. Eng. 11, 330–341. Available online at: https://www.ijisae.org/index.php/IJISAE/article/view/3123
Singh, K., Singh, Y., Barak, D., and Yadav, M. (2023c). “Detection of lung cancers from ct images using a deep CNN architecture in layers through ML,” in AI and IoT-Based Technologies for Precision Medicine (Hershey, PA: IGI Global), 97–107. doi: 10.4018/979-8-3693-0876-9.ch006
Singh, K., Singh, Y., Barak, D., and Yadav, M. (2023d). Evaluation of designing techniques for reliability of Internet of Things (IoT). Int. J. Eng. Trends Technol. 71, 102–118 doi: 10.14445/22315381/IJETT-V71I8P209
Singh, K., Singh, Y., Barak, D., Yadav, M., and Özen, E. (2023e). Parametric evaluation techniques for reliability of Internet of Things (IoT). Int. J. Comput. Methods Exp. Meas. 11, 123–134. doi: 10.18280/ijcmem.110207
Singh, K., Yadav, M., Singh, Y., and Barak, D. (2023f). “Reliability techniques in IoT environments for the healthcare industry,” in AI and IoT-Based Technologies for Precision Medicine (Hershey, PA: IGI Global), 394–412. doi: 10.4018/979-8-3693-0876-9.ch023
Spanos, G., Giannoutakis, K. M., Votis, K., and Tzovaras, D. (2019). “Combining statistical and machine learning techniques in IoT anomaly detection for smart homes,” in 2019 IEEE 24th International Workshop on Computer Aided Modeling and Design of Communication Links and Networks (CAMAD) (Limassol: IEEE), 1–6. doi: 10.1109/CAMAD.2019.8858490
Stiawan, D., Idris, M. Y., Malik, R. F., Nurmaini, S., and Budiarto, R. (2016). “Anomaly detection and monitoring in Internet of Things communication,” in 2016 8th International Conference on Information Technology and Electrical Engineering (ICITEE) (Yogyakarta: IEEE), 1–4. doi: 10.1109/ICITEED.2016.7863271
Su, M. Y. (2011). Real-time anomaly detection systems for Denial-of-Service attacks by weighted k-nearest-neighbor classifiers. Expert Syst. Appl. 38, 3492–3498. doi: 10.1016/j.eswa.2010.08.137
Thanigaivelan, N. K., Nigussie, E., Kanth, R. K., Virtanen, S., and Isoaho, J. (2016). “Distributed internal anomaly detection system for Internet-of-Things,” in 2016 13th IEEE annual consumer communications and networking conference (CCNC) (Las Vegas, NV: IEEE), 319–320. doi: 10.1109/CCNC.2016.7444797
Thomas, M. O., and Rad, B. B. (2017). Reliability evaluation metrics for internet of things, car tracking system: a review. Int. J. Inf. Technol. Comput. Sci. 9, 1–10. doi: 10.5815/ijitcs.2017.02.01
Tsantilis, I., Dasaklis, T. K., Douligeris, C., and Patsakis, C. (2021). Searching deterministic chaotic properties in system-wide vulnerability datasets. Informatics 8:86. doi: 10.3390/informatics8040086
Weber, R. H. (2010). Internet of Things–New security and privacy challenges. Comput. Law Secur. Rev. 26, 23–30. doi: 10.1016/j.clsr.2009.11.008
Xing, L. (2020). Reliability in Internet of Things: current status and future perspectives. IEEE Internet Things J. 7, 6704–6721. doi: 10.1109/JIOT.2020.2993216
Xing, L., Tannous, M., Vokkarane, V. M., Wang, H., and Guo, J. (2017). Reliability modeling of mesh storage area networks for Internet of Things. IEEE Internet Things J. 4, 2047–2057. doi: 10.1109/JIOT.2017.2749375
Xu, Z., and Saleh, J. H. (2021). Machine learning for reliability engineering and safety applications: review of current status and future opportunities. Reliab. Eng. Syst. Saf. 211:107530. doi: 10.1016/j.ress.2021.107530
Yadav, M. (2020). A review on piezoelectric energy harvesting systems based on different mechanical structures. Int. J. Enhanced Res. Sci. Technol. Eng. 9, 1–7. Available online at: https://rb.gy/w8mdzx
Yadav, M., Kumar, S., Kaushik, A., and Chhabra, D. (2023). Piezo-beam structure in a pipe with turbulent flow as energy harvester: mathematical modeling and simulation. J. Inst. Eng. D 104, 739–752. doi: 10.1007/s40033-022-00440-z
Yadav, M., Kumar, S., Kaushik, A., Garg, R. K., and Chhabra, D. (2022). Modeling and simulation of piezo-beam structure mounted in a circular pipe using laminar flow as energy harvester. Int. J. Eng. Trends Technol. 71, 296–314. doi: 10.14445/22315381/IJETT-V71I2P232
Yadav, M., and Yadav, D. (2019). Micro energy generation in different kinds of water flows on lead zirconium titanite/PVDF. Int. J. R and D Eng. Sci. Manag. 9, 1–8. Available online at: https://rb.gy/nybsqd
Yadav, M., Yadav, D., Garg, R. K., Gupta, R. K., Kumar, S., and Chhabra, D. (2021a). “Modeling and optimization of piezoelectric energy harvesting system under dynamic loading,” in Advances in Fluid and Thermal Engineering: Select Proceedings of FLAME 2020 (Singapore: Springer Singapore), 339–353. doi: 10.1007/978-981-16-0159-0_30
Yadav, M., Yadav, D., Kumar, S., and Chhabra, D. (2021b). State of art of different kinds of fluid flow interactions with piezo for energy harvesting considering experimental, simulations and mathematical modeling. J. Math. Comput. Sci. 11, 8258–8287.
Yadav, M., Yadav, D., Kumar, S., Garg, R. K., and Chhabra, D. (2019). Experimental and mathematical modeling and analysis of piezoelectric energy harvesting with dynamic periodic loading. Int. J. Recent Technol. Eng 8, 6346–6350. doi: 10.35940/ijrte.C6107.098319
Yeh, W. C., Tan, S. Y., Zhu, W., Huang, C. L., and Yang, G. Y. (2022). Novel binary addition tree algorithm (BAT) for calculating the direct lower-bound of the highly reliable binary-state network reliability. Reliab. Eng. Syst. Saf. 223:108509. doi: 10.1016/j.ress.2022.108509
Yi, Y., Zhang, Z., Yang, L. T., Deng, X., Yi, L., and Wang, X. (2020). Social interaction and information diffusion in social Internet of Things: dynamics, cloud-edge, traceability. IEEE Internet Things J. 8, 2177–2192. doi: 10.1109/JIOT.2020.3026995
Yusof, Y. W. M., Mohamed, A. H., and Kassim, M. (2023). Energy monitoring system design and analysis with the internet of things on the blynk platform. Int. J. Eng. Trends Technol. 71, 345–353. doi: 10.14445/22315381/IJETT-V71I3P236
Zhang, R., Shim, B., Yuan, W., Di Renzo, M., Dang, X., and Wu, W. (2022). Integrated sensing and communication waveform design with sparse vector coding: low sidelobes and ultra reliability. IEEE Trans. Veh. Technol. 71, 4489–4494. doi: 10.1109/TVT.2022.3146280
Keywords: reliability, Internet of Things, network reliability, fault tree, CHISS
Citation: Singh K, Yadav M, Singh Y, Barak D, Saini A and Moreira F (2024) Reliability on the Internet of Things with designing approach for exploratory analysis. Front. Comput. Sci. 6:1382347. doi: 10.3389/fcomp.2024.1382347
Received: 07 February 2024; Accepted: 29 April 2024;
Published: 04 June 2024.
Edited by:
Alicia García-Holgado, University of Salamanca, SpainReviewed by:
David Fonseca, Ramon Llull University, SpainCopyright © 2024 Singh, Yadav, Singh, Barak, Saini and Moreira. This is an open-access article distributed under the terms of the Creative Commons Attribution License (CC BY). The use, distribution or reproduction in other forums is permitted, provided the original author(s) and the copyright owner(s) are credited and that the original publication in this journal is cited, in accordance with accepted academic practice. No use, distribution or reproduction is permitted which does not comply with these terms.
*Correspondence: Fernando Moreira, Zm1vcmVpcmFAdXB0LnB0
Disclaimer: All claims expressed in this article are solely those of the authors and do not necessarily represent those of their affiliated organizations, or those of the publisher, the editors and the reviewers. Any product that may be evaluated in this article or claim that may be made by its manufacturer is not guaranteed or endorsed by the publisher.
Research integrity at Frontiers
Learn more about the work of our research integrity team to safeguard the quality of each article we publish.