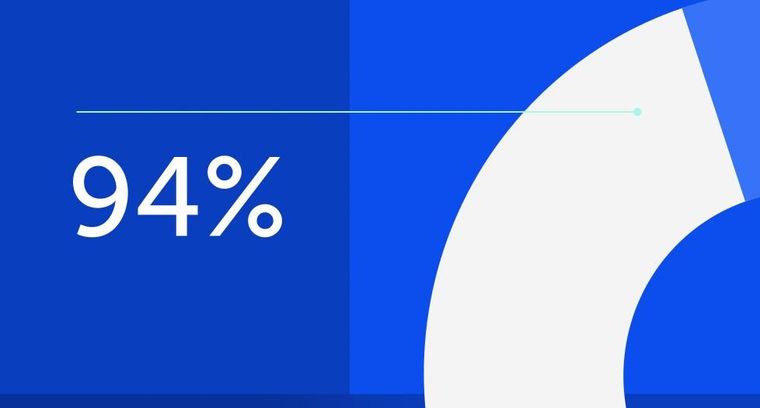
94% of researchers rate our articles as excellent or good
Learn more about the work of our research integrity team to safeguard the quality of each article we publish.
Find out more
SYSTEMATIC REVIEW article
Front. Comput. Sci., 02 June 2023
Sec. Human-Media Interaction
Volume 5 - 2023 | https://doi.org/10.3389/fcomp.2023.1187299
This article is part of the Research TopicResponsible AI in Healthcare: Opportunities, Challenges, and Best PracticesView all 5 articles
Introduction: Artificial intelligence (AI) technologies are increasingly applied to empower clinical decision support systems (CDSS), providing patient-specific recommendations to improve clinical work. Equally important to technical advancement is human, social, and contextual factors that impact the successful implementation and user adoption of AI-empowered CDSS (AI-CDSS). With the growing interest in human-centered design and evaluation of such tools, it is critical to synthesize the knowledge and experiences reported in prior work and shed light on future work.
Methods: Following the Preferred Reporting Items for Systematic Reviews and Meta-Analyses (PRISMA) guidelines, we conducted a systematic review to gain an in-depth understanding of how AI-empowered CDSS was used, designed, and evaluated, and how clinician users perceived such systems. We performed literature search in five databases for articles published between the years 2011 and 2022. A total of 19874 articles were retrieved and screened, with 20 articles included for in-depth analysis.
Results: The reviewed studies assessed different aspects of AI-CDSS, including effectiveness (e.g., improved patient evaluation and work efficiency), user needs (e.g., informational and technological needs), user experience (e.g., satisfaction, trust, usability, workload, and understandability), and other dimensions (e.g., the impact of AI-CDSS on workflow and patient-provider relationship). Despite the promising nature of AI-CDSS, our findings highlighted six major challenges of implementing such systems, including technical limitation, workflow misalignment, attitudinal barriers, informational barriers, usability issues, and environmental barriers. These sociotechnical challenges prevent the effective use of AI-based CDSS interventions in clinical settings.
Discussion: Our study highlights the paucity of studies examining the user needs, perceptions, and experiences of AI-CDSS. Based on the findings, we discuss design implications and future research directions.
Over the past decade, we have witnessed an unprecedented speed of the development of artificial intelligence (AI) techniques, such as deep neural networks and knowledge graph. An essential application area of AI is the clinical domain. Seminal work has reported their efforts in utilizing state-of-the-art AI techniques to empower clinical decision support systems (CDSS)—electronic systems designed to generate and present patient-specific assessments or recommendations to help clinicians make fast and accurate diagnostic decisions (Bright et al., 2012; Erickson et al., 2017; Jiang et al., 2017; Lindsey et al., 2018). It is expected that AI-empowered CDSS interventions (AI-CDSS) will change the landscape of clinical decision-making and profoundly impact patient-provider relationship (Patel et al., 2009; Jiang et al., 2017; Shortliffe and Sepúlveda, 2018).
Despite the technical advancement that has truly boosted AI-CDSS, it is well recognized that human factors (e.g., explainability, privacy, and fairness) as well as social and organizational dimensions of medical work are equally important in determining the success of AI-CDSS implementation (Cheng et al., 2019; Magrabi et al., 2019; Knop et al., 2022). For instance, end-users (i.e., clinicians in this context) may resist accepting or using AI-CDSS due to the lack of trust in and understanding of AI-CDSS's capability (Hidalgo et al., 2021). A possible reason is that many AI-CDSS function as a “black box,” as such, how they generate the recommendations remains opaque to clinicians (Zihni et al., 2020; Schoonderwoerd et al., 2021). These issues could lead to limited user acceptance and system uptake (Strickland, 2019). Given these potential challenges, there is a general consensus that research is urgently needed to understand how to appropriately design AI-CDSS to meet user needs and align with clinical workflow (Tahaei et al., 2023). Without involving users in rigorous system design and evaluations, it becomes an almost impossible mission to successfully implement and deploy AI-CDSS in complex and dynamic clinical contexts (Antoniadi et al., 2021).
With the growing awareness of the critical role of human and social factors in AI-CDSS implementation (Tahaei et al., 2023), there is a need to synthesize the knowledge and experiences in human-centered design and evaluation of AI-CDSS. To that end, we conducted a systematic review on the studies focusing on user needs, perceptions, and experiences when using AI-CDSS in clinical settings. Our specific research questions are as follows: (1) what are the general characteristics of prior research on human-centered design and evaluation of AI-CDSS? (2) How is the system designed, used, and evaluated? (3) What are the perceived benefits and challenges of using AI-CDSS in clinical practice? These research questions guided our literature search, screening, and analysis. Our work contributes to the intersection of AI and human-computer interaction fields by (1) providing an in-depth analysis and synthesis of prior research on the design and evaluation of AI-CDSS from eventual users' perspective; (2) further highlighting the criticality of considering user experience, social and contextual factors when implementing AI-CDSS; and (3) discussing design implications and future research on how to design AI-CDSS for better workflow integration and user acceptance.
In the following of the paper, we first describe the methods we used to screen and review relevant articles (Section 2). Then we describe the major findings of this systematic review in Section 3, including the general characteristics of selected studies, system features, system design and evaluation details, and user perceptions. We conclude this paper by discussing the implications of this review, including AI-CDSS design and future research directions (Section 4).
In this section, we describe the specific process we followed to retrieve, screen, and identify relevant articles for this systematic review.
As a key first step of our literature search, we first discussed with an experienced librarian about the search time frame, search terms, and databases. After iterative refinement of search terms, we used AI-related terms such as “artificial intelligence” and “machine learning,” along with decision support keywords such as “clinical decision support” or “healthcare decision support” to perform a literature search for articles published between January 2011 and May 2022. The latter marks the time our literature search process started. We selected this time frame to capture the evolvement of the AI technology. We chose five databases to cover research within both health care and computer science, including ACM Digital Library, IEEE Xplore, Web of Science, Ovid MEDLINE, and Scopus. The database searches were set to include only studies published in peer-reviewed journals and conference proceedings in English. Dissertations, posters, brief reports, and extended abstracts were excluded from the database search. The search process returned a total of 19,874 articles as shown in Table 1. The retrieved citations were stored and managed using Covidence1—a web-based collaboration software platform that streamlines the production of systematic and other literature reviews.
As shown in Figure 1, this systematic review was conducted following the Preferred Reporting Items for Systematic Reviews and Meta-Analyses (PRISMA) guidelines (Moher et al., 2009). Multiple researchers were involved in this process. More specifically, two researchers (LW and JL) used Covidence and independently screened all retrieved articles and selected relevant papers for inclusion. Two senior researchers (ZZ and DW) oversaw the whole article screening and selection process. Any disagreements in article selections were resolved through discussion among researchers during weekly research meetings. The inclusion criteria were peer-reviewed articles that reported human-centered use, design, and evaluation of AI-CDSS interventions in clinical or hospital settings. That is, end-users (e.g., clinicians) must be engaged in the system design and/or evaluation process. Articles were excluded if they only reported the technology development or implementation (e.g., technical details) without examining user needs or experience, or if they described a tool that was not used in clinical settings [e.g., patient-facing, AI-driven self-diagnosis chatbots (Fan et al., 2021)]. Other systematic review papers were also excluded.
Figure 1 outlines the number of records that were identified, included, and excluded through different phases. More specifically, out of the 19,874 articles that were retrieved through database search, 17,247 (86.78%) of them were included for screening after removing duplicates. We then screened the article titles and abstracts consecutively to identify relevant articles. In the title and abstract screenings, 16,215 and 965 articles were excluded, respectively. This screening process left 67 articles for full-text review. After reviewing the full text of these 67 articles, 20 of them were deemed eligible for this systematic review.
Guided by our research questions, two authors (LW and ZZ) used a Microsoft Excel spreadsheet to extract data from the included studies, such as the country where the study was conducted, year of publication, study objectives and scope, clinical setting, system evaluation, technology specifics, perceived benefits and challenges, and a summary of study findings. The research team met regularly to discuss the extracted data. As suggested by prior work (Lavallée et al., 2013), we performed the data analysis in an iterative manner, i.e., going back and forth to refine the extracted data as more knowledge was obtained.
In this section, we report the main themes that are derived from the reviewed articles, including the general characteristics of selected studies (e.g., country, clinical focus), system features, system design and evaluation details, and perceived benefits and barriers. The main findings and more details about each study are reported in Table 2.
As shown in Figure 2A, of the 20 studies, eleven were conducted in the United States (Stevens et al., 2012; Yang et al., 2016, 2019; Cai et al., 2019a,b; Chiang et al., 2020; Kumar et al., 2020; Romero-Brufau et al., 2020; Sendak et al., 2020; Jacobs et al., 2021; Lee et al., 2021), two each in China (Jin et al., 2020; Wang et al., 2021), Canada (Benrimoh et al., 2021; Tanguay-Sela et al., 2022), and Thailand (Hoonlor et al., 2018; Beede et al., 2020), and one each in Spain (Caballero-Ruiz et al., 2017), United Kingdom (Abdulaal et al., 2021), and Austria (Jauk et al., 2021). The reviewed articles were published between 2012 and 2022. As illustrated in Figure 2B, it is noticeable that the number of studies related to user experience assessment of AI-CDSS has drastically increased since 2019. In particular, six of the reviewed studies were published in 2020 (Beede et al., 2020; Chiang et al., 2020; Jin et al., 2020; Kumar et al., 2020; Romero-Brufau et al., 2020; Sendak et al., 2020) and another set of six articles was published in 2021 (Abdulaal et al., 2021; Benrimoh et al., 2021; Jacobs et al., 2021; Jauk et al., 2021; Lee et al., 2021; Wang et al., 2021). We only found one article (Tanguay-Sela et al., 2022) published in 2022 because our literature search was performed up to May 2022.
Figure 2. (A) The distribution of countries where the reviewed studies were conducted. (B) The distribution of reviewed articles over the years.
The application areas of AI-CDSS in the reviewed studies varied. As summarized in Table 3, four studies focused on general medicine (Chiang et al., 2020; Jin et al., 2020; Kumar et al., 2020; Wang et al., 2021), three studies each focused on diabetes (Caballero-Ruiz et al., 2017; Beede et al., 2020; Romero-Brufau et al., 2020) and depressive disorders (Benrimoh et al., 2021; Jacobs et al., 2021; Tanguay-Sela et al., 2022), while two studies each focused on cancer diagnosis (Cai et al., 2019a,b) and heart pump implant (Yang et al., 2016, 2019). The remaining studies focused on intensive care unit (ICU) monitoring (Stevens et al., 2012), sepsis (Sendak et al., 2020), rehabilitation (Lee et al., 2021), delirium (Jauk et al., 2021), snake envenomation (Hoonlor et al., 2018), and COVID-19 (Abdulaal et al., 2021).
Regarding the main utility of AI-CDSS (Table 3), they are primarily used to provide diagnostic support (e.g., predict critical events and diagnosis) (Caballero-Ruiz et al., 2017; Cai et al., 2019b; Beede et al., 2020; Jin et al., 2020; Jacobs et al., 2021; Jauk et al., 2021; Lee et al., 2021; Wang et al., 2021), generate intervention and treatment recommendations (Yang et al., 2016, 2019; Caballero-Ruiz et al., 2017; Hoonlor et al., 2018; Jacobs et al., 2021; Wang et al., 2021), identify and alert patients at risk (Stevens et al., 2012; Romero-Brufau et al., 2020; Sendak et al., 2020; Abdulaal et al., 2021), recommend clinical orders (Chiang et al., 2020; Kumar et al., 2020), predict treatment compliance by patients (Benrimoh et al., 2021; Tanguay-Sela et al., 2022), and facilitate the search of medical images and information (Cai et al., 2019a; Wang et al., 2021). It is worth mentioning that some systems are designed with the intent to fulfill several purposes while others are used for a specific purpose. For example, the system reported in Wang et al. (2021) not only provide diagnostic support and recommend treatment options but also allow clinicians to search for similar patient cases and other medical information. In contrast, the system prototype created in two studies conducted by Yang and her colleagues (Yang et al., 2016, 2019) is specifically designed to support the decision-making of heart pump implants (e.g., determining whether or not implanting a heart pump for a particular patient).
All the systems reported in the reviewed articles utilized machine learning techniques [e.g., deep neural network (DNN)] for automatic decision support generation. As summarized in Figure 3A, out of the reviewed 20 studies, only seven studies reported a fully implemented AI-CDSS intervention which was usually integrated with electronic health record (EHR) in real clinical settings (Caballero-Ruiz et al., 2017; Beede et al., 2020; Jin et al., 2020; Romero-Brufau et al., 2020; Sendak et al., 2020; Jauk et al., 2021; Wang et al., 2021). Among these seven studies, two examined a commercial product (Romero-Brufau et al., 2020; Wang et al., 2021), while three evaluated a system developed by the research team (Caballero-Ruiz et al., 2017; Jin et al., 2020; Sendak et al., 2020). The remaining studies (n = 13) either examined user needs and system design requirements with (Cai et al., 2019b; Jacobs et al., 2021) or without (Yang et al., 2016) a low-fidelity system prototype, or evaluated a functional, high-fidelity system prototype in a controlled environment without the involvement of real patients (Stevens et al., 2012; Hoonlor et al., 2018; Cai et al., 2019a; Yang et al., 2019; Chiang et al., 2020; Kumar et al., 2020; Abdulaal et al., 2021; Benrimoh et al., 2021; Lee et al., 2021; Tanguay-Sela et al., 2022).
Figure 3. (A) AI-CDSS implementation details and tested contexts. (B) An overview of the number of users involved in the system design and evaluation process (x-axis represents the number of users, the y-axis represents the number of studies involving a specific number range of users).
As the application area and utilization of AI-CDSS varied among the reviewed studies, it is difficult to synthesize common features across all the interventions. However, we were able to examine and identify themes in relation to how the predictions and recommendations of AI-CDSS were presented to users (Table 4). The majority of the studies (n = 19) reported what types of system output or information were presented to the user with only one exception (Yang et al., 2016). More specifically, 13 out of those 19 studies only displayed predictions or recommendations (e.g., patient diagnosis, treatment choice, the patient at risk of developing critical symptoms) (Stevens et al., 2012; Caballero-Ruiz et al., 2017; Hoonlor et al., 2018; Cai et al., 2019a,b; Yang et al., 2019; Beede et al., 2020; Chiang et al., 2020; Kumar et al., 2020; Romero-Brufau et al., 2020; Sendak et al., 2020; Abdulaal et al., 2021; Wang et al., 2021). Other studies (n = 6) provided additional information (e.g., explanations) along with the displayed prediction, such as the key patient-specific information or variables that informed each prediction (Benrimoh et al., 2021; Jauk et al., 2021; Lee et al., 2021; Tanguay-Sela et al., 2022), comparison with the baseline population data used to train the model (Jin et al., 2020; Benrimoh et al., 2021), and validation report on the tool (Jacobs et al., 2021).
Another interesting observation is that a couple of studies provide clinicians with additional features to refine the results (Cai et al., 2019a; Lee et al., 2021). For example, Cai et al. (2019a) describe an AI-CDSS for diagnostic support of cancer patients, allowing pathologists to refine the results based on visually similar medical images retrieved through “refine-by-region” (e.g., users crop a region to emphasize its importance in the image search), “refine-by-example” (e.g., users can pin examples from search results to emphasize its importance), or “refine-by-concept” (e.g., users increase or decrease the presence of clinical concepts by sliding sliders) features.
Lastly, the use of visual (e.g., colors, icons, charts) and auditory (e.g., alarming sounds) elements in AI-CDSS is common. For example, in the ICU monitoring study (Stevens et al., 2012), the researchers used colors in the patient's vital signs monitor and graduated alarm sounds to convey the urgency and nature of the patient's critical condition. In another study related to medical image search and retrieval (Cai et al., 2019b), prostate cancer grade predictions (e.g., grade 3, grade 4, and grade 5) were shown as colored overlays on top of the prostate tissue to facilitate the reading and interpretation of clinicians.
The reviewed studies focus on evaluating different aspects of AI-CDSS, including system effectiveness, user needs, user experience, and others. These studies engaged a varying number of participants (Figure 3B), ranging between 6 clinicians (Beede et al., 2020) and 81 staff members at the clinics (Romero-Brufau et al., 2020), with most studies (n = 13) having a range of 10 to 30 participants (Stevens et al., 2012; Yang et al., 2016; Hoonlor et al., 2018; Cai et al., 2019a,b; Jin et al., 2020; Sendak et al., 2020; Abdulaal et al., 2021; Benrimoh et al., 2021; Jacobs et al., 2021; Jauk et al., 2021; Wang et al., 2021; Tanguay-Sela et al., 2022). The details regarding the focal aspects, outcome measurements, and research methods are summarized in Table 5 and then elaborated on in the following sections.
Of the 20 reviewed studies, 7 (35%) evaluated the effectiveness of AI-CDSS interventions. As the clinical application areas varied, the measurements used to evaluate the system's effectiveness were also different in these studies. However, a few outcome measurements were used by more than one study, such as improvement in patient assessment and management (Caballero-Ruiz et al., 2017; Jin et al., 2020; Romero-Brufau et al., 2020; Lee et al., 2021), information retrieval performance (Cai et al., 2019a; Chiang et al., 2020; Kumar et al., 2020; Lee et al., 2021), time spent on patient care (Chiang et al., 2020; Kumar et al., 2020), and clinical appropriateness of suggested interventions or treatment orders (Caballero-Ruiz et al., 2017; Kumar et al., 2020). For example, Kumar et al. (2020) used the primary outcome (e.g., the clinical appropriateness of orders placed) and secondary outcomes (e.g., the information retrieval performance of order search methods assessed by precision and recall, and the time spent on completing order entry for each patient case) to evaluate whether the AI-driven order recommender system could significantly improve the workflow of clinical order entry.
The commonly used methodology is to conduct an experimental study to compare care performance and other metrics between the intervention condition (e.g., using AI-CDSS) and the control condition (e.g., using old or baseline systems, or conventional and manual practices) (Stevens et al., 2012; Caballero-Ruiz et al., 2017; Chiang et al., 2020; Kumar et al., 2020; Lee et al., 2021). Other methods include case studies (Jin et al., 2020), clinicians' self-rating of results (Cai et al., 2019a), compliance analysis (Caballero-Ruiz et al., 2017), and survey questionnaires (Romero-Brufau et al., 2020). For example, one study (Caballero-Ruiz et al., 2017) conducted a randomized controlled trial to assess the effectiveness of remote monitoring of diabetic patients using an AI-driven telemedical care system in comparison to the conventional care process. The effectiveness was assessed by several dimensions, such as the number of automatically generated prescriptions that were accepted, rectified, postponed, and rejected by clinicians, and the patient's compliance to use the system to measure and report physiological data (e.g., blood glucose), and the time spent on each patient case by clinicians.
A few studies (n = 5, 25%) also emphasized on user needs in designing AI-CDSS, including the information needs for enhancing the transparency and trust of AI-CDSS interventions (Cai et al., 2019b) and technology needs for supporting clinical decision making (Yang et al., 2016; Cai et al., 2019a; Jin et al., 2020; Jacobs et al., 2021). For example, Cai et al. (2019b) interviewed 21 pathologists to understand the key types of information medical experts desire when they are first introduced to a diagnostic AI system for prostate cancer diagnosis. They found that in addition to the local, case-specific reasoning behind any model decision, medical experts also desired upfront information about basic, global properties of the model, such as (1) capabilities and limitations (e.g., AI's particular strengths and limitations under specific conditions), (2) functionality (e.g., what information the AI has access to and how it uses that information to make a prediction), (3) medical point-of-view (e.g., whether AI tends to be more liberal or conservative when generating a prediction), (4) design objective (e.g., what AI has been optimized for), and (5) considerations prior to adopting or using an AI-CDSS (e.g., the impact on workflows, accountability, and cost of use).
The main method used for eliciting user needs in these five studies was interviews. In particular, three studies also used low-fidelity prototypes as a study probe during interviews to obtain more in-depth insights, asking participants to reflect on the prototypes by considering which aspects they found helpful or unhelpful, what features they would change, and what new features or ideas they would like to add (Cai et al., 2019a,b; Jacobs et al., 2021). Another noteworthy observation is that the user needs assessment was often done in a multi-phase process. For example, Jin et al. (2020) first conducted interviews with physicians and then created and used prototypes to further elicit and refine the system requirements, while Cai et al. (2019b) completed their user needs assessment with three phases (“pre-probe,” “probe,” and “post-probe”).
Most studies (n = 15, 75%) examined the user experience of AI-CDSS (Caballero-Ruiz et al., 2017; Hoonlor et al., 2018; Cai et al., 2019a; Yang et al., 2019; Chiang et al., 2020; Jin et al., 2020; Kumar et al., 2020; Romero-Brufau et al., 2020; Sendak et al., 2020; Abdulaal et al., 2021; Benrimoh et al., 2021; Jauk et al., 2021; Lee et al., 2021; Wang et al., 2021; Tanguay-Sela et al., 2022). The most frequently measured outcome was overall satisfaction, which represented the level of clinicians' satisfaction with the performance of AI-CDSS (Caballero-Ruiz et al., 2017; Cai et al., 2019a; Yang et al., 2019; Chiang et al., 2020; Kumar et al., 2020; Romero-Brufau et al., 2020; Abdulaal et al., 2021; Benrimoh et al., 2021; Jauk et al., 2021; Wang et al., 2021). The second most used outcome measurement was the ease of use of the system, which was often measured by the task completion time, the number of clicks needed to complete the task, the easiness of finding needed information, and the System Usability Scale (SUS) (Chiang et al., 2020; Jin et al., 2020; Kumar et al., 2020; Abdulaal et al., 2021; Jauk et al., 2021; Wang et al., 2021). The system's trustworthiness was another critical element that was specifically assessed by five studies (Caballero-Ruiz et al., 2017; Cai et al., 2019a; Sendak et al., 2020; Lee et al., 2021; Wang et al., 2021). This critical aspect was usually assessed by questionnaires; one study (Cai et al., 2019a) reported that they used Mayer's dimensions of trust (Mayer et al., 1995) to measure the trustfulness of their system because this instrument has been widely used in prior studies on trust.
Other measured outcomes included workload (e.g., the efforts required for using the system) (Caballero-Ruiz et al., 2017; Cai et al., 2019a; Lee et al., 2021), willingness to use (e.g., the user's intent to use the system in the future) (Lee et al., 2021; Tanguay-Sela et al., 2022), workflow integration (e.g., how well the system aligned with and was integrated into clinical workflow) (Yang et al., 2019; Wang et al., 2021), and understandability and learnability (e.g., how easy it is for users to understand the information and prediction provided by the system) (Hoonlor et al., 2018).
Regarding the methods for assessing user experience, interviews (Cai et al., 2019a; Yang et al., 2019; Jin et al., 2020; Abdulaal et al., 2021; Benrimoh et al., 2021; Jauk et al., 2021; Lee et al., 2021; Wang et al., 2021; Tanguay-Sela et al., 2022), survey questionnaires (Caballero-Ruiz et al., 2017; Cai et al., 2019a; Chiang et al., 2020; Kumar et al., 2020; Romero-Brufau et al., 2020; Abdulaal et al., 2021; Benrimoh et al., 2021; Jauk et al., 2021; Lee et al., 2021; Tanguay-Sela et al., 2022), and observation (Hoonlor et al., 2018; Benrimoh et al., 2021; Wang et al., 2021; Tanguay-Sela et al., 2022) were primarily used by researchers. A few studies also used existing instruments that had been validated in prior work, such as the NASA-TLX questionnaire for assessing workload (Hart and Staveland, 1988), the System Usability Scale (SUS) questionnaire for assessing usability (Bangor et al., 2008), and Mayer's dimensions of trust for assessing users' trust (Mayer et al., 1995). Another interesting finding is that many studies used more than one method to either corroborate research findings or collect more user insights. For example, Benrimoh et al. (2021) and Tanguay-Sela et al. (2022) relied on questionnaires, scenario observations, and interviews to collect user experience data, while Jauk et al. (2021) conducted focus groups with experts before and during the system pilot and administered the Technology Acceptance Model (TAM) questionnaire for data collection.
We identified three studies examining the impact of AI-CDSS on clinical workflow (Caballero-Ruiz et al., 2017; Chiang et al., 2020; Kumar et al., 2020). The methods used for this critical evaluation included survey questionnaires (Chiang et al., 2020; Kumar et al., 2020) and healthcare service usage analysis (Caballero-Ruiz et al., 2017). For example, in the study of evaluating an AI-empowered telemedicine platform to automatically generate diet prescriptions and detect insulin needs for better monitoring of gestational diabetes (Caballero-Ruiz et al., 2017), the researchers not only evaluated the effectiveness and user acceptance of the system but also examined the system's impact on patient-provider interaction, i.e., through calculating the face-to-face visit frequency and duration with vs. without the AI-CDSS.
Additionally, two studies investigated the sociotechnical factors affecting the use of AI-CDSS, mainly through interviews and observation (Beede et al., 2020; Sendak et al., 2020). More specifically, Beede et al. (2020) examined factors influencing the performance of a deep learning system in detecting diabetic retinopathy and found that the ability to take gradable pictures and internet speed and connectivity would impact the system's performance in a clinical setting. In another study (Sendak et al., 2020), researchers reflected on the lessons learned from the development, implementation, and deployment of an AI-driven tool that assists hospital clinicians in the early diagnosis and treatment of sepsis. They highlighted that instead of focusing solely on model interpretability to ensure fair and accountable AI systems, it is utmost of importance to treat AI-CDSS as a socio-technical system and ensure their integration into existing social and professional contexts (Sendak et al., 2020). They also suggested key values and work practices that need to be considered when developing AI-CDSS, including rigorously defining the problem in context, building relationships with stakeholders, respecting professional discretion, and creating ongoing feedback loops with stakeholders (Sendak et al., 2020).
In this section, we report our synthesized results regarding the users' perceived benefits and challenges of using AI-CDSS. A summary of user perceptions can be found in Table 6.
Overall, the reviewed studies reported that clinicians had a positive attitude toward AI-CDSS. Many clinicians believed that AI-CDSS could improve patient diagnosis and management by suggesting differential diagnosis and treatment choices, as well as preventing adverse events (Stevens et al., 2012; Cai et al., 2019a; Yang et al., 2019; Chiang et al., 2020; Jin et al., 2020; Abdulaal et al., 2021; Jauk et al., 2021; Lee et al., 2021; Wang et al., 2021; Tanguay-Sela et al., 2022). For example, the ability to suggest different treatment options and their potential outcomes were often considered a “cool and useful” feature to support making a prognosis (Jin et al., 2020; Tanguay-Sela et al., 2022). When there is a meaningful disagreement between the clinician's judgment and the AI-CDSS prediction of the patient's condition, the system could encourage clinicians to take a closer look at the patient's case or gather more information to assess the situation (Yang et al., 2019). Even when the AI-CDSS prediction perfectly aligns with the clinician's view, the system outputs could enhance the clinician's confidence in their clinical evaluation (Abdulaal et al., 2021; Tanguay-Sela et al., 2022). As such, AI-CDSS interventions were often perceived by clinicians as a useful adjunct to their clinical practice (Abdulaal et al., 2021; Wang et al., 2021; Tanguay-Sela et al., 2022).
Another perceived benefit of AI-CDSS by clinicians, which was also confirmed through quantitative analysis, is its capability in increasing clinicians' work efficiency while reducing their workload (Caballero-Ruiz et al., 2017; Cai et al., 2019a; Chiang et al., 2020; Kumar et al., 2020; Tanguay-Sela et al., 2022). For example, in the studies examining the impact of a clinical order entry recommender system (Chiang et al., 2020; Kumar et al., 2020), the researchers reported that after using the system for a while, more than 90% of the participating clinicians agreed or strongly agreed that the system would be useful for their workflows, 89% agreed or strongly agreed that the system would make their job easier, and 85% felt that it would increase their productivity. Through statistical analyses, Caballero-Ruiz et al. (2017) reported the time devoted by clinicians to patients' evaluation was reduced by almost 27.4% after the use of an AI-driven telemedicine system, and Cai et al. (2019a) reported their participants experienced less effort using their system than the conventional interface.
AI-CDSS was also reported to enhance the patient-provider relationship by increasing patient understanding of and trust in clinicians' treatment (Benrimoh et al., 2021), facilitating the discussion of treatment options between clinicians and patients (Tanguay-Sela et al., 2022), or reducing unnecessary clinical visits without compromising patient monitoring (Caballero-Ruiz et al., 2017). Additionally, AI-CDSS could also transform the interaction and communication among clinical team members. For example, one highlighted benefit was that the AI-CDSS promoted team dialog about patient needs (Romero-Brufau et al., 2020). In another study (Beede et al., 2020), the AI-CDSS tool was found to have the potential to shift the asymmetrical power dynamics between physicians and nurses—a teamwork and organizational issue which often leads to the clinical opinions and assessments of nurses being undervalued or dismissed by physicians (Okpala, 2021)—by providing outputs that nurses can use as a reference to prove their judgement about the patient status and demonstrate their expertise to more senior clinicians.
Finally, it is interesting to see that AI-CDSS was considered a useful tool for providing on-the-job training opportunities to clinicians (Beede et al., 2020; Wang et al., 2021). For example, Wang et al. (2021) reported some features of the examined AI-CDSS (e.g., searching similar patient cases) could help clinicians, especially less experienced junior clinicians, self-educate and gain new knowledge and experience. In a similar vein, participants in another study (Beede et al., 2020) used the system for diabetic retinopathy detection as a learning opportunity—improving their ability to make accurate diabetic retinopathy assessments themselves.
The reviewed studies reported a variety of challenges, issues, and barriers in using and adopting AI-CDSS in clinical environments, which were grouped into six high-level categories, including technical limitations, workflow misalignment, attitudinal barriers, informational barriers, usability issues, and environmental barriers. We describe each category of perceived challenges in detail below.
Technical limitations are a major perceived challenge reported by several studies (n = 5) (Beede et al., 2020; Jin et al., 2020; Romero-Brufau et al., 2020; Abdulaal et al., 2021; Wang et al., 2021). A major user concern is that the outputs or predictions generated by AI-CDSS may be inaccurate (Beede et al., 2020; Romero-Brufau et al., 2020; Wang et al., 2021). In such cases, medical experts would be concerned about the consequences for patients if the system produced a wrong prediction, including the additional burden to follow up on a referral, the cost of handling a wrong diagnosis, and the emotional strain a wrong prediction (e.g., false positive) could place on them (Beede et al., 2020; Wang et al., 2021).
Another related concern is that many AI-CDSS interventions failed to consider and capture subtle, contextual factors that are vital in the diagnostic process but can only be recognized by medical providers who have a face-to-face conversation with the patient (Romero-Brufau et al., 2020; Wang et al., 2021). This technical limitation prevents AI from considering the “whole person” as medical providers can, and as such, impacting the adequacy and accuracy of the recommendations (Romero-Brufau et al., 2020).
The final commonly listed technical barrier is related to the quality of the training data for AI-CDSS. There were concerns that “generalizability would be difficult” if the data were accrued from a single center (Abdulaal et al., 2021). Also, predictions would be more accurate if they are based on the statistics of a very large collection of patients over a very long period of time; when the underlying data are not rich enough to represent the rich variety of outcomes that a patient could face, the generated recommendations could become questionable and less useful (Jin et al., 2020). This issue could be more evident for those hospitals or healthcare organizations that were just starting to use EHR systems to collect and curate data (Jin et al., 2020).
Seamless integration of AI-CDSS within clinical workflows is an important step for leveraging developed AI algorithms (Juluru et al., 2021). However, it has always been a challenging task, as six reviewed articles illustrated (Beede et al., 2020; Kumar et al., 2020; Abdulaal et al., 2021; Jauk et al., 2021; Wang et al., 2021; Tanguay-Sela et al., 2022). For instance, both Beede et al. (2020) and Wang et al. (2021) examined the use of AI-CDSS in clinics in developing countries (China and Thailand, respectively) and revealed that with high patient volume already a burden, medical experts were concerned that following the protocol of using AI-CDSS would increase their workload and lead to clinician burnout. Even with reasonable patient volume, Jauk et al. (2021) reached a similar conclusion—using AI-CDSS could potentially increase the workload of clinicians, which was considered a major reason for the low system usage during their pilot study. The added workload could lead to interference in the physician-patient relationship, as clinicians were distracted by AI-CDSS from the time they were supposed to spend with patients (Tanguay-Sela et al., 2022).
Another issue related to workflow integration is the interoperability between AI-CDSS and existing clinical systems (Kumar et al., 2020; Abdulaal et al., 2021; Wang et al., 2021). When AI-CDSS is not integrated with other systems that can provide various forms of data input for AI-CDSS, their capability in generating accurate and reliable predictions could be affected. For example, Wang et al. (2021) reported that because the evaluated AI-CDSS tool was not integrated with the hospital's pharmaceutical system, the medication prescriptions recommended by AI were not available in the hospital's pharmacy. Similarly, the COVID-19 mortality prediction app evaluated in Abdulaal et al. (2021) was a standalone app, which could only be used in isolation rather than in conjunction with other clinical data, leading to the concerns about accuracy of the predictions.
Clinicians' attitudes and opinions about AI-CDSS is another critical factor in user adoption and uptake. Even though many studies suggested that clinicians generally had a positive attitude toward the use of AI-CDSS, a few studies (n = 3) reported completely opposite results (Yang et al., 2016, 2019; Romero-Brufau et al., 2020). For example, in the studies of designing decision support for heart pump implants (Yang et al., 2016, 2019), the researchers found that clinicians had no desire to use an AI-CDSS, indicated no need for data support, and expressed resistance toward the idea of showing personalized predictions. The primary reasons reported in these studies were that clinicians considered themselves knowing how to effectively factor patient conditions into clinical decisions, and preferred knowing facts such as the statistics from previous similar cases (in comparison to showing probability of a prediction). Romero-Brufau et al. (2020) also reported that only 14% of their clinician participants would recommend the tested AI-CDSS to other clinicians. Collectively, all these findings revealed a conservative attitude of clinicians toward the adoption of AI-CDSS.
Another related attitudina barrier is that clinicians tended not to trust the outputs yielded by AI-CDSS—an issue that has been widely explored by prior work (Stone et al., 2022)—leading to limited or no use of the system. One study (Wang et al., 2021) cited several reasons for the lack of trust in AI-CDSS suggestions: clinicians (rather than AI-CDSS) being held accountable for medical errors, lack of explanation on how the recommendations were generated, lack of in-depth training for the use of AI-CDSS, and clinicians' professional autonomy being challenged by AI-CDSS. Additionally, if clinicians have negative experience with current decision support tools and models, it is unlikely for clinicians to use any AI-CDSS until they trust these systems can deliver value, as stated in Yang et al. (2016).
A mismatch between clinicians' information needs and AI-CDSS outputs could also be a barrier to the adoption of and the trust building in AI-CDSS (Yang et al., 2016; Cai et al., 2019b; Tanguay-Sela et al., 2022). An example (Yang et al., 2016) is that clinicians need support for determining whether to perform a treatment or not, to do it now or to “wait and see”; however, AI-CDSS tools only predicted outcomes of conducting a procedure now, with little actionable information about waiting and seeing.
More broadly, the “black box” nature of AI causes many AI-CDSS interventions lack transparency; it is usually unclear to the clinicians how the AI predictive model functions, how the AI arrives at its predictions and suggestions, what clinical data the AI model is trained on, and whether or not the model is validated by clinical trials. With a limited understanding of these aspects of AI-CDSS, clinicians expressed the difficulty they had in determining whether they should trust and use the system outputs (Cai et al., 2019b; Tanguay-Sela et al., 2022).
Not surprisingly, the usability issues of AI-CDSS were also reported in three studies (Yang et al., 2016; Jin et al., 2020; Wang et al., 2021). These issues could be attributed to the lack of consideration of or misalignment with clinical workflow. For example, one major usability issue is related to the input methods of AI-CDSS. In Wang et al. (2021), one interaction mechanism for AI-CDSS required clinicians to manually click through a set of screens with questions to provide input (e.g., symptoms, medical history, etc.); however, this interaction approach took a lot of time to complete a diagnosis process even though it was designed to make clinicians' work easier. As it was not aligned well with the clinical workflow (e.g., clinicians were required to complete a patient case within a few minutes given the high volume of waiting patients), clinicians rarely used this interaction approach. Two other studies (Yang et al., 2016; Jin et al., 2020) highlighted that due to clinicians' constant moving and frequent changing of protective gloves and clothes in clinical environments, it is challenging to use current WIMP (windows, icons, menus, pointer) interaction style to interact with AI-CDSS.
The learning curve of AI-CDSS was also raised by two studies (Jin et al., 2020; Wang et al., 2021). For instance, clinicians complained that learning how to use the system and how to read complex visualizations or patient views took essential time away from them. These issues were exacerbated by the lack of in-depth training and the onboarding process (Wang et al., 2021).
Three studies also pointed out the unique restrictions posed by the clinical environments where AI-CDSS was deployed (Yang et al., 2016; Beede et al., 2020; Wang et al., 2021). For example, the AI-CDSS that was designed for the detection of diabetic eye disease required high-quality fundus photos that were not easy to produce in locations with low resources (e.g., non-darkening environment, low internet speed, and low quality of camera) (Beede et al., 2020). Yang et al. (2016) highlighted a few other environmental factors that could impact the effective use of AI-CDSS; for instance, clinicians are constantly on the move and need to log in and out on different computers, posing challenges to easy access to AI-CDSS in dynamic clinical environments.
In this work, we conducted a systematic review of studies focusing on the user experience of AI-CDSS. Of the 17,247 papers included for screening, only 20 (0.11%) met our inclusion criteria, highlighting the paucity of studies examining the user needs, perceptions, and experiences of AI-CDSS. However, we found that there has been a growing awareness of and interest in assessing user experience and needs of AI-CDSS, especially since 2019. This observation is consistent with (Tahaei et al., 2023) stating that researchers, especially in the Human-Computer Interaction (HCI) community, have increasingly emphasized human factor-related issues in AI (e.g., explainability, privacy, and fairness).
We found a large variation regarding the application areas of AI-CDSS. But in general, these interventions were mainly used for providing diagnostic support to clinicians, recommending intervention and treatment options, alerting patients at risk, predicting treatment outcomes, and facilitating medical information searches. To enhance the user experience (e.g., trust, understandability, usability) of AI-CDSS tools, a set of reviewed studies utilized such strategies as providing explanations for each prediction, using visual and auditory elements, and allowing users to refine the outputs. These strategies were deemed useful by clinicians.
The reviewed studies assessed different aspects of AI-CDSS, including effectiveness (e.g., improved patient evaluation and work efficiency), user needs (e.g., informational and technological needs), user experience (e.g., satisfaction, trust, usability, workload, and understandability), and other dimensions (e.g., the impact of AI-CDSS on workflow and patient-provider relationship). In most studies, AI-CDSS was found to be capable of improving patient diagnosis and treatment, preventing adverse events, increasing clinicians' work efficiency, enhancing the patient-provider and provider-provider relationship, and serving as a great opportunity for clinician training. In addition, those systems received positive user feedback in general, indicating a high level of user acceptance and perceived usefulness. Despite so, our review identified a number of challenges in adopting and using AI-CDSS in clinical practices, which were further synthesized and categorized into six high-level categories, including technical limitations, workflow misalignment, attitudinal barriers, informational barriers, usability issues, and environmental barriers. In the next section, we discuss the design implications of these findings and strategies that can potentially improve the user experience of AI-CDSS.
Prior research has pointed out that the “black box” nature of AI could cause issues related to user trust, attitude, and understandability, among many others (Caballero-Ruiz et al., 2017; Shortliffe and Sepúlveda, 2018). For example, clinicians may not understand how AI-CDSS generates recommendations for a given patient case and how reliable those recommendations are, all of which could affect clinicians' trust in the system. Several reviewed articles argued that providing additional information about AI-CDSS could help address these critical issues (Cai et al., 2019a; Yang et al., 2019; Romero-Brufau et al., 2020; Sendak et al., 2020; Jacobs et al., 2021; Wang et al., 2021; Tanguay-Sela et al., 2022). For example, Cai et al. (2019a) highlighted the importance of providing a holistic, global view of the AI-CDSS to users during the onboarding process, such as the system's capabilities, functionality, medical point-of-view, and design objective. These types of information, with careful design of presentation and visualization, could not only help clinicians understand whether or not they should trust and use the AI-CDSS, but also how they can most effectively partner with it in practice (Schoonderwoerd et al., 2021).
It is also worth noting that one study (Jacobs et al., 2021) explicitly examined when to provide additional information (e.g., explanations) about the predictions generated by AI-CDSS. This study challenged the idea of using explanations to encourage users to make determinations of trust for each prediction as medical experts usually don't have sufficient time to review additional information for every decision. Therefore, for time-constrained medical environments, rather than providing all evidence in support of a prediction, it will have a higher user acceptance to provide on-demand explanations when an AI prediction is diverging from existing guidelines or expert knowledge.
Numerous studies have revealed that failure to integrate HITs into clinical workflow could result in limited user adoption (Sittig and Singh, 2015). The primary reason is that newly introduced HIT interventions could disrupt current clinical work practice, causing not only frustrations for medical providers but also patient safety issues (Harrington et al., 2011). When this problem occurs, medical providers need to bypass the new technology and adopt informal, low-tech, potentially unsafe workarounds (Vogelsmeier et al., 2008; Beede et al., 2020).
Not surprisingly, our reviewed papers also identified workflow misalignment as a major issue for the successful implementation of AI-CDSS (Yang et al., 2016, 2019; Cai et al., 2019a; Beede et al., 2020; Sendak et al., 2020; Jacobs et al., 2021; Wang et al., 2021). They all highlighted the urgent need of designing AI-CDSS in a way that can seamlessly fit into the local clinical context and workflow. For example, Yang et al. (2019) suggested making the AI-CDSS “unremarkable”; that is, embedding the system into the point of decision-making and the infrastructure of the healthcare system in an unobtrusive way. To achieve this goal, it is critical to thoroughly examine and understand the decision-making process to determine how best to integrate AI-CDSS into the workflow and how to strategically present AI outputs to eventual users (Yang et al., 2016, 2019; Cai et al., 2019a; Beede et al., 2020; Jacobs et al., 2021; Wang et al., 2021). As illustrated in the reviewed studies, ethnographic research methods, such as interviews and in situ observation, are suitable for examining and gaining an in-depth understanding of the clinicians' fine-grained work practices (Spradley, 2016a,b; Fetterman, 2019).
Another important consideration is integrating AI-CDSS with existing clinical systems to generate more accurate predictions based on the data collected from various systems (Sendak et al., 2020; Jacobs et al., 2021; Wang et al., 2021). Even more, AI-CDSS should be designed to allow clinicians to manually input more contextual data (e.g., patient's emotional and socioeconomic status) to produce more adequate recommendations (Jin et al., 2020; Wang et al., 2021). These strategies could empower AI-CDSS to gain access to a variety of patient data (e.g., historical medical data and contextual data) to achieve the goal of considering the “whole patient.”
Our reviewed studies underscore the limits of solely relying on model interpretability to ensure transparency and accountability in practice, and point out the criticality of accounting for social, organizational, and environmental factors in achieving the values of trustworthiness and transparency in AI-CDSS design (Beede et al., 2020; Sendak et al., 2020; Jacobs et al., 2021). In Sendak et al. (2020), the researchers shared the lessons learned when they were designing and implementing an AI-CDSS. For instance, it is suggested to build accountable relationships with hospital leadership, engage stakeholders early and often, rigorously define the problem in context, make the system an enterprise-level solution by involving other departments and units (e.g., security and legal departments), and create an efficient communication mechanism with frontline healthcare providers (Sendak et al., 2020).
Given the essential role of non-technical factors, two reviewed studies (Sendak et al., 2020; Jacobs et al., 2021) suggest conceptualizing AI-CDSS as a sociotechnical system, which allows researchers and system designers to understand and take into account various social and organizational components that are equally important as technical components in determining the success of AI-CDSS implementation in a complex health care organization. These suggestions align well with prior work which recognizes the importance of designing HIT interventions through the lens of a sociotechnical perspective (Or et al., 2014; Sittig and Singh, 2015; Zhang et al., 2022).
A few reviewed articles highlight the need to allow clinicians freely operate their professional judgment and decision-making in patient care without any interference (Romero-Brufau et al., 2020; Sendak et al., 2020; Wang et al., 2021). In particular, they suggest that AI-CDSS should be designed to augment human intelligence and capabilities and work with clinicians as an adjunct or a diagnostic aid, instead of performing tasks in place of humans. The clinicians should rely on their expertise and the support of AI-CDSS to make final diagnostic decisions and be responsible for their decisions. These suggestions align with the paradigm of human-AI collaboration advocated by many researchers (Amershi et al., 2019); that is, human workers (e.g., clinicians) and AI systems should work together to complete critical tasks. With this new paradigm in clinical settings, several urgent research questions are worth exploration, such as how to draw a clear distinction of responsibility between AI and clinicians, what kinds of regulation and policy need to be in place to address the accountability issue of AI-CDSS, and how to design a “cooperative” AI-CDSS (Goodman and Flaxman, 2017; Wang et al., 2021; Bleher and Braun, 2022).
Taken together, the reviewed articles along with many other studies have highlighted the importance of employing a human-centered approach to design AI-CDSS interventions to ensure their effective use and better integration into the clinical workflow (Tahaei et al., 2023). This approach requires researchers to engage end-users in the system design process early and often (Sendak et al., 2020). More specifically, it is a useful strategy to create ongoing feedback loops with users and stakeholders. By doing so, researchers and system designers can continuously and iteratively collect user feedback to inform the design of AI-CDSS (Sendak et al., 2020; Abdulaal et al., 2021; Lee et al., 2021). For example, Sendak et al. (2020) conducted many meetings and information sessions as a communication vehicle to convey information to and collect feedback from users and stakeholders, while Lee et al. (2021) used a “human-in-the-loop” approach to engage medical experts in improving an imperfect AI-CDSS.
The reviewed articles also reported other user suggestions and lessons learned to address barriers in AI-CDSS adoption, such as providing in-depth training to clinicians to flatten the learning curve (Jin et al., 2020; Wang et al., 2021), designing AI-CDSS as a multi-user system to better engage patients in decision-making (Caballero-Ruiz et al., 2017; Jacobs et al., 2021), expanding the application of AI-CDSS tools to a variety of clinical scenarios (Jin et al., 2020; Wang et al., 2021), and examining the data quality for potential bias and fairness issues in AI-CDSS (Jin et al., 2020).
Our study reveals several research gaps that could inform future research directions. First, the reviewed articles primarily focused on evaluating the effectiveness and user experience of AI-CDSS interventions. However, other important factors that could play a vital role in AI-CDSS adoption received little attention. For example, the economic impact of AI-CDSS could be an important factor for many healthcare organizations to consider. Two of our reviewed papers (Chiang et al., 2020; Kumar et al., 2020) reported that the physicians place slightly more orders per case, i.e., 1.3 and 1 additional orders, respectively, compared to the priori situations. This observation contradicts to various earlier studies that standardized medical order systems as part of CDSS promote cost-effectiveness (e.g., Ballard et al., 2008). However, a careful economic outcomes evaluation of an AI-CDSS involves many more perspectives, including a more comprehensive set of measurements of introduced interventions, total amount of lab tests changes prior and after, as well as the average length of hospital stay based on the AI-CDSS recommendations (Fillmore et al., 2013; Lewkowicz et al., 2020). Indeed, as Chen et al. (2022) pointed out, the research on economic impact of CDSS intervention and cost-effectiveness measurement are varied and inconclusive, due to the various study contexts, the quality of reporting, and evaluation methods. Future work is needed to systematically evaluate the cost-effectiveness of AI-CDSS implementation using a comprehensive set of measurements.
Second, only a few reviewed articles (Beede et al., 2020; Sendak et al., 2020; Jacobs et al., 2021) took into consideration the social, organizational, and environmental factors in AI-CDSS design and implementation. Such factors are essential in determining AI-CDSS adoption, but they could get overlooked easily. As such, future work should take a more holistic, sociotechnical approach to determine how to implement AI-CDSS in a complex health care organization. For example, as Sittig and Singh (2015) recommended, implementation of complex healthcare systems should consider at least the following dimensions: hardware and software computing infrastructure, clinical content, human-computer interface, people, workflow and communication, internal organizational policies, procedures and culture, external rules, regulations and pressures, and finally, system measurement and monitoring. Conceptualizing AI-CDSS implementation as a sociotechnical process could help the development team foresee potential non-technical issues and tackle them as early as possible.
Third, the studies were mostly conducted in the United States and other high-income countries (e.g., Canada, United Kingdom, Spain, and Austria), with only a few studies being conducted in locations with low resources where such technology can provide significant support to patient care (e.g., Thailand and China). This finding reveals the gap in AI-CDSS implementation and research in low- and middle-income countries (Sambasivan et al., 2012). Despite some low-resource countries having increased their investment in health information technologies (HITs), these countries often face much greater challenges in implementing AI-CDSS interventions compared to high-income countries (Tomasi et al., 2004; Ji et al., 2021; Wang et al., 2021). For example, as one reviewed study mentioned (Jin et al., 2020), the implementation of EHR systems in China is still very limited, creating a barrier to gathering comprehensive patient data for AI-CDSS to generate accurate recommendations. The limited access to and integration with HITs has slowed down the implementation and adoption of AI-CDSS in low- and middle-income countries (Sambasivan et al., 2012; Li et al., 2020). Future work can examine social and economic factors that influence the success of AI-CDSS introduction in low-resource countries and the best strategies and practices that can be utilized to overcome barriers and challenges faced by these locations.
Lastly, patients' attitude about using AI-CDSS in their diagnostic process is another important factor to consider (Caballero-Ruiz et al., 2017; Zhang et al., 2021). Indeed, using AI-CDSS could affect patient-provider interaction and communication as highlighted by several reviewed articles (Caballero-Ruiz et al., 2017; Tanguay-Sela et al., 2022). Given the increased focus on enhancing patients' participation in the shared decision-making process with clinicians, it is critical to make sure that AI-CDSS is designed as a multi-user system to better engage patients in decision-making (Stiggelbout et al., 2012). A few of our reviewed studies touched upon this topic (Caballero-Ruiz et al., 2017; Jacobs et al., 2021), but more work is needed to investigate how to accomplish this design objective.
Several study limitations need to be noted. First, defining the search keywords and databases was difficult. To generate a comprehensive and relevant list of keywords, we iteratively discussed the search keywords among researchers and with librarians. We also decided to use multiple databases to cover research within both health care and computer science fields, even though this approach resulted in a significant number of retrieved papers for screening (n = 19,874). Second, biases may exist when articles were reviewed and analyzed. To address this issue, we have at least two researchers independently screened the articles and extracted details from each paper. They met regularly to compare and merge the results. Any disagreements were resolved through discussion among all researchers. Lastly, we did not assess the quality of the study results from the reviewed articles. A meta-analysis was not feasible because of the heterogeneity of the study designs and results.
The advent of artificial intelligence techniques has a tremendous potential to transform the clinical decision-making process at an unprecedented scale. Despite the importance of engaging users in the design and evaluation of AI-CDSS, the research focus on this aspect has been far outpaced by the efforts devoted to improving the AI's technical performance and prediction accuracy. Aligned with on-going research trend on human-centered AI, we highlighted the numerous sociotechnical challenges in AI-CDSS adoption and implementation that couldn't be easily addressed without an in-depth investigation of clinical workflow and user needs. To better integrate AI-CDSS into clinical practice and preserve human autonomy and control, as well as avoid unintended consequences of AI systems on individuals and organizations, the design of AI-CDSS should be human-centric. We drew the results of the reviewed articles and situated them in prior work to discuss design implications to enhance the user experience and acceptance of AI-CDSS interventions.
The original contributions presented in the study are included in the article/supplementary material, further inquiries can be directed to the corresponding author.
LW, JL, and WC worked with librarians to retrieve articles. LW, JL, ZZ, and DW were involved in article screening, analysis, and synthesisations. All authors contributed to the study conceptualization, paper writing, met regularly to discuss the project's progress, results, contributed to the article, and approved the submitted version.
We thank the librarian (Shan Yang) for supporting the literature search process.
The authors declare that the research was conducted in the absence of any commercial or financial relationships that could be construed as a potential conflict of interest.
All claims expressed in this article are solely those of the authors and do not necessarily represent those of their affiliated organizations, or those of the publisher, the editors and the reviewers. Any product that may be evaluated in this article, or claim that may be made by its manufacturer, is not guaranteed or endorsed by the publisher.
1. ^Covidence systematic review software, Veritas Health Innovation, Melbourne, Australia. Available at www.covidence.org.
Abdulaal, A., Patel, A., Al-Hindawi, A., Charani, E., Alqahtani, S. A., Davies, G. W., et al. (2021). Clinical utility and functionality of an artificial intelligence–based app to predict mortality in COVID-19: mixed methods analysis. JMIR Format. Res. 5, e27992. doi: 10.2196/27992
Amershi, S., Weld, D., Vorvoreanu, M., Fourney, A., Nushi, B., Collisson, P., et al. (2019). “Guidelines for human-AI interaction,” in Proceedings of the 2019 CHI Conference on Human Factors in Computing Systems,1–13.
Antoniadi, A. M., Du, Y., Guendouz, Y., Wei, L., Mazo, C., Becker, B. A., et al. (2021). Current challenges and future opportunities for XAI in machine learning-based clinical decision support systems: a systematic review. Appl. Sci. 11, 5088. doi: 10.3390/app11115088
Ballard, D. J., Ogola, G., Fleming, N. S., Heck, D., Gunderson, J., Mehta, R., et al. (2008). “The impact of standardized order sets on quality and financial outcomes,” in Advances in Patient Safety: New Directions and Alternative Approaches (vol. 2: culture and redesign).
Bangor, A., Kortum, P. T., and Miller, J. T. (2008). An empirical evaluation of the system usability scale. Intl. J. Hum. Comput. Interact. 24, 574–594. doi: 10.1080/10447310802205776
Beede, E., Baylor, E., Hersch, F., Iurchenko, A., Wilcox, L., Ruamviboonsuk, P., et al. (2020). “A human-centered evaluation of a deep learning system deployed in clinics for the detection of diabetic retinopathy,” in Proceedings of the 2020 CHI Conference on Human Factors in Computing Systems, 1–12.
Benrimoh, D., Tanguay-Sela, M., Perlman, K., Israel, S., Mehltretter, J., Armstrong, C., et al. (2021). Using a simulation centre to evaluate preliminary acceptability and impact of an artificial intelligence-powered clinical decision support system for depression treatment on the physician–patient interaction. BJPsych Open 7, e22. doi: 10.1192/bjo.2020.127
Bleher, H., and Braun, M. (2022). Diffused responsibility: attributions of responsibility in the use of AI-driven clinical decision support systems. AI Ethics 2, 747–761. doi: 10.1007/s43681-022-00135-x
Bright, T. J., Wong, A., Dhurjati, R., Bristow, E., Bastian, L., Coeytaux, R. R., et al. (2012). Effect of clinical decision-support systems: a systematic review. Ann. Intern. Med. 157, 29–43. doi: 10.7326/0003-4819-157-1-201207030-00450
Caballero-Ruiz, E., García-Sáez, G., Rigla, M., Villaplana, M., Pons, B., Hernando, M. E., et al. (2017). A web-based clinical decision support system for gestational diabetes: Automatic diet prescription and detection of insulin needs. Int. J. Med. Inform. 102, 35–49. doi: 10.1016/j.ijmedinf.2017.02.014
Cai, C. J., Reif, E., Hegde, N., Hipp, J., Kim, B., Smilkov, D., et al. (2019a). “Human-centered tools for coping with imperfect algorithms during medical decision-making,” in Proceedings of the 2019 CHI Conference on Human Factors in Computing Systems, 1–14.
Cai, C. J., Winter, S., Steiner, D., and Wilcox, L. (2019b). “Hello AI”: uncovering the onboarding needs of medical practitioners for human-AI collaborative decision-making. Proc. ACM Hum. Comput. Interact. 3, 1–24. doi: 10.1145/3359206
Chen, W., Howard, K., Gorham, G., O'Bryan, C. M., Coffey, P., Balasubramanya, B., et al. (2022). Design, effectiveness, and economic outcomes of contemporary chronic disease clinical decision support systems: a systematic review and meta-analysis. J. Am. Med. Inform. Assoc. 29, 1757–1772. doi: 10.1093/jamia/ocac110
Cheng, H. F., Wang, R., Zhang, Z., O'Connell, F., Gray, T., Harper, F. M., et al. (2019). “Explaining decision-making algorithms through UI: Strategies to help non-expert stakeholders,” in Proceedings of the 2019 CHI Conference on Human Factors in Computing Systems, 1–12.
Chiang, J., Kumar, A., Morales, D., Saini, D., Hom, J., Shieh, L., et al. (2020). Physician usage and acceptance of a machine learning recommender system for simulated clinical order entry. AMIA Summits Translat. Sci. Proc. 2020, 89.
Erickson, B. J., Korfiatis, P., Akkus, Z., and Kline, T. L. (2017). Machine learning for medical imaging. Radiographics 37, 505–515. doi: 10.1148/rg.2017160130
Fan, X., Chao, D., Zhang, Z., Wang, D., Li, X., Tian, F., et al. (2021). Utilization of self-diagnosis health chatbots in real-world settings: case study. J. Med. Internet Res. 23, e19928. doi: 10.2196/19928
Fillmore, C. L., Bray, B. E., and Kawamoto, K. (2013). Systematic review of clinical decision support interventions with potential for inpatient cost reduction. BMC Med. Inform. Decis. Mak. 13, 1–9. doi: 10.1186/1472-6947-13-135
Goodman, B., and Flaxman, S. (2017). European Union regulations on algorithmic decision-making and a “right to explanation”. AI Magazine 38, 50–57. doi: 10.1609/aimag.v38i3.2741
Harrington, L., Kennerly, D., and Johnson, C. (2011). Safety issues related to the electronic medical record (EMR): synthesis of the literature from the last decade, 2000-2009. J. Healthcare Manage. 56, 31–44. doi: 10.1097/00115514-201101000-00006
Hart, S. G., and Staveland, L. E. (1988). Development of NASA-TLX (Task Load Index): results of empirical and theoretical research. Adv. Psychol. 52, 139–183. doi: 10.1016/S0166-4115(08)62386-9
Hidalgo, C. A., Orghian, D., Canals, J. A., and Almeida, D. E. (2021). How Humans Judge Machines. Cambridge, MA: MIT Press.
Hoonlor, A., Charoensawan, V., and Srisuma, S. (2018). “The clinical decision support system for the snake envenomation in Thailand,” in 2018 15th International Joint Conference on Computer Science and Software Engineering (JCSSE). IEEE, 1–6.
Jacobs, M., He, J., Pradier, F., Lam, M., Ahn, B., McCoy, A. C., et al. (2021). “Designing AI for trust and collaboration in time-constrained medical decisions: a sociotechnical lens,” in Proceedings of the 2021 CHI Conference on Human Factors in Computing Systems, 1–14.
Jauk, S., Kramer, D., Avian, A., Berghold, A., Leodolter, W., Schulz, S., et al. (2021). Technology acceptance of a machine learning algorithm predicting delirium in a clinical setting: a mixed-methods study. J. Med. Syst. 45, 1–8. doi: 10.1007/s10916-021-01727-6
Ji, M., Chen, X., Genchev, G. Z., Wei, M., and Yu, G. (2021). Status of AI-enabled clinical decision support systems implementations in China. Methods Inf. Med. 60, 123–132. doi: 10.1055/s-0041-1736461
Jiang, F., Jiang, Y., Zhi, H., Dong, Y., Li, H., Ma, S., et al. (2017). Artificial intelligence in healthcare: past, present and future. Stroke Vasc. Neurol. 2, 4. doi: 10.1136/svn-2017-000101
Jin, Z., Cui, S., Guo, S., Gotz, D., and Sun, J. (2020). Carepre: an intelligent clinical decision assistance system. ACM Trans. Comput. Healthcare 1, 1–20. doi: 10.1145/3344258
Juluru, K., Shih, H.-H., Murthy, K. N. K., Elnajjar, P., El-Rowmeim, A., et al. (2021). Integrating Al algorithms into the clinical workflow. Radiol. Artif. Intelligence 3, e210013. doi: 10.1148/ryai.2021210013
Knop, M., Weber, S., Mueller, M., and Niehaves, B. (2022). Human factors and technological characteristics influencing the interaction of medical professionals with artificial intelligence–enabled clinical decision support systems: literature review. JMIR Human Factors 9, e28639. doi: 10.2196/28639
Kumar, A., Aikens, R. C., Hom, J., Shieh, L., Chiang, J., Morales, D., et al. (2020). OrderRex clinical user testing: a randomized trial of recommender system decision support on simulated cases. J. Am. Med. Inform. Assoc. 27, 1850–1859. doi: 10.1093/jamia/ocaa190
Lavallée, M., Robillard, P-., and Mirsalari, R. (2013). Performing systematic literature reviews with novices: an iterative approach. IEEE Trans. Educ. 57, 175–181. doi: 10.1109/TE.2013.2292570
Lee, M. H., Siewiorek, D. P., Smailagic, A., Bernardino, A., and Badia, S. (2021). “A human-ai collaborative approach for clinical decision making on rehabilitation assessment,” in Proceedings of the 2021 CHI Conference on Human Factors in Computing Systems, 1–14.
Lewkowicz, D., Wohlbrandt, A., and Boettinger, E. (2020). Economic impact of clinical decision support interventions based on electronic health records. BMC Health Serv. Res. 20, 1–12. doi: 10.1186/s12913-020-05688-3
Li, D., Chao, J., Kong, J., Cao, G., Lv, M., Zhang, M., et al. (2020). The efficiency analysis and spatial implications of health information technology: a regional exploratory study in China. Health Informatics J. 26, 1700–1713. doi: 10.1177/1460458219889794
Lindsey, R., Daluiski, A., Chopra, S., Lachapelle, A., Mozer, M., Sicular, S., et al. (2018). Deep neural network improves fracture detection by clinicians. Proc. Nat. Acad. Sci. 115, 11591–11596. doi: 10.1073/pnas.1806905115
Magrabi, F., Ammenwerth, E., McNair, J. B., Keizer, D. E., Hyppönen, N. F., Nykänen, H., et al. (2019). Artificial intelligence in clinical decision support: challenges for evaluating AI and practical implications. Yearb. Med. Inform. 28, 128–134. doi: 10.1055/s-0039-1677903
Mayer, R. C., Davis, J. H., and Schoorman, F. D. (1995). An integrative model of organizational trust. Acad. Manage. Rev. 20, 709–734. doi: 10.2307/258792
Moher, D., Liberati, A., Tetzlaff, J., Altman, D. G., and Group PRISMA. (2009). Preferred reporting items for systematic reviews and meta-analyses: the PRISMA statement. Ann. Intern. Med. 151, 264–269. doi: 10.7326/0003-4819-151-4-200908180-00135
Okpala, P. (2021). Addressing power dynamics in interprofessional health care teams. Int. J. Healthcare Manage. 14, 1326–1332. doi: 10.1080/20479700.2020.1758894
Or, C., Dohan, M., and Tan, J. (2014). Understanding critical barriers to implementing a clinical information system in a nursing home through the lens of a socio-technical perspective. J. Med. Syst. 38, 1–10. doi: 10.1007/s10916-014-0099-9
Patel, V. L., Shortliffe, E. H., Stefanelli, M., Szolovits, P., Berthold, M. R., Bellazzi, R., et al. (2009). The coming of age of artificial intelligence in medicine. Artif. Intell. Med. 46, 5–17. doi: 10.1016/j.artmed.2008.07.017
Romero-Brufau, S., Wyatt, K. D., Boyum, P., Mickelson, M., Moore, M., Cognetta-Rieke, C., et al. (2020). A lesson in implementation: a pre-post study of providers' experience with artificial intelligence-based clinical decision support. Int. J. Med. Inform. 137, 104072. doi: 10.1016/j.ijmedinf.2019.104072
Sambasivan, M., Esmaeilzadeh, P., Kumar, N., and Nezakati, H. (2012). Intention to adopt clinical decision support systems in a developing country: effect of Physician's perceived professional autonomy, involvement and belief: a cross-sectional study. BMC Med. Inform. Decis. Mak. 12, 1–8. doi: 10.1186/1472-6947-12-142
Schoonderwoerd, T. A., Jorritsma, W., Neerincx, M. A., and Van Den Bosch, K. (2021). Human-centered XAI: Developing design patterns for explanations of clinical decision support systems. Int. J. Hum. Comput. Stud. 154, 102684.
Sendak, M., Elish, M. C., Gao, M., Futoma, J., Ratliff, W., Nichols, M., et al. (2020). ““The human body is a black box” supporting clinical decision-making with deep learning,” in Proceedings of the 2020 Conference on Fairness, Accountability, and Transparency, 99–109.
Shortliffe, E. H., and Sepúlveda, M. J. (2018). Clinical decision support in the era of artificial intelligence. JAMA 320, 2199–2200. doi: 10.1001/jama.2018.17163
Sittig, D. F., and Singh, H. (2015). “A new socio-technical model for studying health information technology in complex adaptive healthcare systems,” in Cognitive Informatics for Biomedicine: Human Computer Interaction in Healthcare, 59–80.
Stevens, N., Giannareas, A. R., Kern, V., Viesca, A., Fortino-Mullen, M., King, A., et al. (2012). “Smart alarms: multivariate medical alarm integration for post CABG surgery patients,” in Proceedings of the 2nd ACM SIGHIT International Health Informatics Symposium, 533–542.
Stiggelbout, A. M., Van der Weijden, T., Wit, D. E., Frosch, M. P. T., Légaré, D., et al. (2012). Shared decision making: really putting patients at the centre of healthcare. BMJ 344, e256. doi: 10.1136/bmj.e256
Stone, P., Brooks, R., Brynjolfsson, E., Calo, R., Etzioni, O., Hager, G., et al. (2022). Artificial intelligence and life in 2030: the one hundred year study on artificial intelligence. arXiv preprint. 52.
Strickland, E. (2019). IBM Watson, heal thyself: How IBM overpromised and underdelivered on AI health care. IEEE Spectrum 56, 24–31. doi: 10.1109/MSPEC.2019.8678513
Tahaei, M., Constantinides, M., and Quercia, D. (2023). Toward human-centered responsible artificial intelligence: a review of CHI research and industry toolkits. arXiv preprint. doi: 10.1145/3544549.3583178
Tanguay-Sela, M., Benrimoh, D., Popescu, C., Perez, T., Rollins, C., Snook, E., et al. (2022). Evaluating the perceived utility of an artificial intelligence-powered clinical decision support system for depression treatment using a simulation center. Psychiatry Res. 308, 114336. doi: 10.1016/j.psychres.2021.114336
Tomasi, E., Facchini, L. A., and Maia, M. F. S. (2004). Health information technology in primary health care in developing countries: a literature review. Bull. World Health Organ. 82, 867–874.
Vogelsmeier, A. A., Halbesleben, J. R., and Scott-Cawiezell, J. R. (2008). Technology implementation and workarounds in the nursing home. J. Am. Med. Inform. Assoc. 15, 114–119. doi: 10.1197/jamia.M2378
Wang, D., Wang, L., Zhang, Z., Wang, D., Zhu, H., Gao, Y., et al. (2021). ““Brilliant AI doctor” in rural clinics: challenges in AI-powered clinical decision support system deployment,” in Proceedings of the 2021 CHI Conference on Human Factors in Computing Systems, 1–18.
Yang, Q., Steinfeld, A., and Zimmerman, J. (2019). “Unremarkable AI: fitting intelligent decision support into critical, clinical decision-making processes,” in Proceedings of the 2019 CHI Conference on Human Factors in Computing Systems, 1–11.
Yang, Q., Zimmerman, J., Steinfeld, A., Carey, L., and Antaki, J. F. (2016). “Investigating the heart pump implant decision process: opportunities for decision support tools to help,” in Proceedings of the 2016 CHI Conference on Human Factors in Computing Systems, 4477–4488.
Zhang, Z., Genc, Y., Wang, D., Ahsen, M. E., and Fan, X. (2021). Effect of ai explanations on human perceptions of patient-facing ai-powered healthcare systems. J. Med. Syst. 45, 64. doi: 10.1007/s10916-021-01743-6
Zhang, Z., Ramiya Ramesh Babu, N. A., Adelgais, K., and Ozkaynak, M. (2022). Designing and implementing smart glass technology for emergency medical services: a sociotechnical perspective. JAMIA Open 5, ooac113. doi: 10.1093/jamiaopen/ooac113
Keywords: artificial intelligence, clinical decision support system, human-centered AI, user experience, healthcare, literature review
Citation: Wang L, Zhang Z, Wang D, Cao W, Zhou X, Zhang P, Liu J, Fan X and Tian F (2023) Human-centered design and evaluation of AI-empowered clinical decision support systems: a systematic review. Front. Comput. Sci. 5:1187299. doi: 10.3389/fcomp.2023.1187299
Received: 15 March 2023; Accepted: 17 May 2023;
Published: 02 June 2023.
Edited by:
Marko Tkalcic, University of Primorska, SloveniaReviewed by:
Elena Cardillo, National Research Council (CNR), ItalyCopyright © 2023 Wang, Zhang, Wang, Cao, Zhou, Zhang, Liu, Fan and Tian. This is an open-access article distributed under the terms of the Creative Commons Attribution License (CC BY). The use, distribution or reproduction in other forums is permitted, provided the original author(s) and the copyright owner(s) are credited and that the original publication in this journal is cited, in accordance with accepted academic practice. No use, distribution or reproduction is permitted which does not comply with these terms.
*Correspondence: Zhan Zhang, enpoYW5nQHBhY2UuZWR1
Disclaimer: All claims expressed in this article are solely those of the authors and do not necessarily represent those of their affiliated organizations, or those of the publisher, the editors and the reviewers. Any product that may be evaluated in this article or claim that may be made by its manufacturer is not guaranteed or endorsed by the publisher.
Research integrity at Frontiers
Learn more about the work of our research integrity team to safeguard the quality of each article we publish.