- 1School of Interactive Arts and Technology, Simon Fraser University, Burnaby, BC, Canada
- 2School of Interactive Games and Media, Rochester Institute of Technology, Rochester, NY, United States
Music therapy is used to treat stress and anxiety in patients for a broad range of reasons such as cancer treatment, substance abuse, addressing trauma, and just daily stress in life. However, access to treatment is limited by the need for trained music therapists and the difficulty of quantitatively measuring efficacy in treatment. We present a survey of digital music systems that utilize biosensing for the purpose of reducing stress and anxiety with therapeutic use of music. The survey analyzes biosensing instruments for brain activity, cardiovascular, electrodermal, and respiratory measurements for efficacy in reduction in stress and anxiety. The survey also emphasizes digital music systems where biosensing is utilized to adapt music playback to the subject, forming a biofeedback loop. We also discuss how these digital music systems can use biofeedback coupled with machine learning to provide improved efficacy. Lastly, we posit that such digital music systems can be realized using consumer-grade biosensing wearables coupled with smartphones. Such systems can provide benefit to music therapists as well as to anyone wanting to treat stress from daily living.
1. Introduction
Stress and anxiety are considered a health epidemic in the twenty-first century, according to the World Health Organization (WHO). Both stress and anxiety have been estimated to cost $300 billion worldwide per year in costs (Fink, 2016). There are numerous ways to cope with and address stress and anxiety, but one often overlooked tool is the therapeutic use of music. Music has been used as a healer and form of therapy across cultures for centuries and has evolved as a methodology since the 19th century. Musicians, physicians, philosophers, and religious leaders, such as priests, have attempted to identify efficacy (Kramer, 2000). Notable figures from the 19th century include Czech physician Leopold Raudnitz, who conducted work with patients in a Prague insane asylum, and clergyman and musician Frederick Kill Harford who is considered a pioneer of music therapy (Kramer, 2000).
The therapeutic use of music addresses the psychophysiological relationship between body and mind, which is crucial knowledge given that stress and anxiety can lead to comorbidity (Johnson, 2019) and increase the likelihood of anxiety disorders later in life (Essau et al., 2018). Studies have demonstrated the positive effects of using music as a therapeutic tool, including a reduction in cortisol levels, heart rate, and arterial pressure (de Witte et al., 2022b). A meta-study examining the use of music therapy for stress and anxiety treatment found significant effects across various dimensions, including psychological and physiological impacts, individual vs. group therapy settings, the use of treatment protocols, and the tempo and beat choices of the music (de Witte et al., 2022b).
However, the success of therapeutic use of music has limits in scale of treatment and research. Any activity involving participants also requires a trained music therapist to guide the session. A music therapist's role is multi-faceted, being witness, sound engineer, producer, and co-creator (Stensæth and Magee, 2016). This limits therapy options and research to a restricted environment involving subject matter experts that can prepare the environment, control the session, and perform analysis on data collected. This makes it more difficult for subjects to find therapy opportunities.
Music therapists and researchers have traditionally used questionnaires before and after a therapeutic music session to gauge efficacy. Scales such as the Visual Analog Scale for Anxiety (VAS-A) and State-Trait Anxiety Inventory (STAI) are available to therapists and researchers. But questionnaires provide limited quantitative data when researching emotions—such as stress and anxiety. This limitation has been linked to the ordinal nature of emotions, where variance from participants can affect accuracy (Kersten et al., 2012; Yannakakis et al., 2021).
A more recent option has been to use medical-grade biosensing equipment to record psychophysiological data. For example, an Electrocardiogram (ECG) machine can record heart rate and other cardiovascular measurements. And an Electroencephalogram (EEG) headset can record brain waves. Both of these types of devices are useful in helping researchers detect and measure stress and anxiety (Lampert, 2015; Katmah et al., 2021). But these types of medical-grade equipment cost thousands of dollars, furthering the limitation in accessibility of therapeutic use of music.
With the recent advent of consumer-grade biosensing wearables such as Apple Watch for heart rate and the BrainBit headband for brain waves, music therapists and researchers now have more options. These devices are relatively inexpensive and are coupled with easy-to-use software. From a music therapist's perspective, these digital systems can act as non-human co-therapists, or “cooperative elements” (Kjetil, 2021).
And these consumer-grade biosensing devices are paired, via Bluetooth, to smartphones–Android phones or Apple iPhones. This allows portable recording of real-time biosensing data and provides music therapists and researchers with more accessible technology for therapeutic use of music. Another recent direction with therapeutic use of music is digital systems that utilize Machine Learning (ML) to classify biosensing data. Using ML can allow a digital music system to adapt to a subject's individual preferences such as tempo, music genre, and other characteristics—based on the biosensing data.
If inexpensive biosensing wearables and more-advanced AI are paired with smartphones, what relationship will this have with music therapy? Thus, our research questions for this survey are:
(1) Can therapeutic use of music be conducted with wearable biosensing computing devices?
(2) Can digital music intervention systems yield results that can address stress and anxiety?
Lastly, we discuss how digital music therapy is evolving with solutions that can detect and treat stress and anxiety. As well, we analyze how these digital music systems utilize biosensing to detect effects of music stimuli. We finish with a posit of the future of therapeutic music systems where ML and consumer-grade biosensing wearables provide the public with easily accessible solutions to address stress and anxiety.
2. Background
In this section we present key concepts related to therapeutic use of music. The first topic examines the subtle differences between stress and anxiety, followed by a clear distinction between music therapy and music medicine. The section also covers the general results of music interventions, highlighting the importance of using digital music systems. Furthermore, we delve into the role of binaural beats in addressing stress and anxiety and explore how instruments and measurements can be valuable in a therapeutic music environment. Lastly, we discuss biosensing technology in recent methodologies for therapeutic use of music.
2.1. Stress vs. anxiety and the emotion model
In the digital music systems that we surveyed, the terms stress and anxiety are treated synonymously. And while they do share overlap in psychophysiological effects on the body, there are differences, one being their duration in time. Stress is caused by external factors such as conflict, pressure (e.g., time constraint) or a challenge imposed. These external factors are known as stressors. Response to stressors is relatively short-lived (e.g., minutes, or hours) and goes away once a stressor is no longer present.
Anxiety is an internal reaction from previous events, such as stressors just mentioned (Spielberger and Sarason, 1991; Adwas et al., 2019). Unlike stress's immediate effect—and dissipation, anxiety manifests well after any initial external factor and may not dissipate over time. Anxiety can affect a patient over a period of months or even years. However, since the digital music systems that we surveyed treat stress and anxiety synonymously, we will use the term stress to refer to both.
The emotional response to stress has been used in studies where researchers are interested in psychophysiological changes in emotion (e.g., distressed, upset). A common emotion model is Dr. James Russell's emotional state wheel, known as the Circumplex Model, shown in Figure 1 (Russell, 1980). Dr. Russell's emotion model comprises of a two-dimensional wheel where the x axis is valence, and the y axis is arousal. Low valence is unpleasantness and high valance is pleasantness. The positive y is arousal and negative y is deactivation.
The circumplex model has been used in therapeutic use of music with results from brain activity via Electroencephalogram (EEG) headset electrodes. In one experiment, researchers utilized an EEG headset with a fractal-based emotion recognition algorithm to record participants' emotions while listening to soundscape sounds from the International Affective Digitized Sounds (IADS) database (Sourina et al., 2012). Researchers were able to detect emotions in real-time. In another research project, researchers changed various facets of music playback—including tempo, pitch, amplitude, and harmonic mode. Participants wore EEG headsets and brainwave data was used to influence the generated music playback. While no significance was found, the study demonstrated work toward users' ability to change music based on their emotion (e.g., happy or sad) (Ehrlich et al., 2019).
2.2. Music therapy vs. music medicine
There are two approaches when it comes to therapeutic use of music: (1) Music Therapy (MT) and (2) Music Medicine (MM). We note the distinctions specifically between MT and MM to avoid ambiguity and misrepresentation since using the terms synonymously has been considered diluting of MT (Wheeler et al., 2019) and showing insensitivity (Gold et al., 2011).
While both are considered helpful for symptom management of stress (Bradt et al., 2015), MT requires a credentialed music therapist to be present (Bradt et al., 2015; Monsalve-Duarte et al., 2022). The therapist's role is to provide opportunities for intervention—a core characteristic of MT (Gold et al., 2011). These opportunities include a wide range of activities such as singing, playing musical instruments, composing, and song writing. When conducting sessions, a music therapist can choose either active music therapy or receptive music therapy. In active music therapy, subjects are involved in the performance of the music (e.g., singing, playing instruments or both). In receptive music therapy, the subject plays a passive role, listening to music only.
MM uses more of a “scientific evaluation” approach with a focus on medical research and may not contain a “systematic therapeutic process” (i.e., methodology) that requires a customized plan for the patient (Bradt et al., 2015). With a more research-based centricity, MM relies more heavily on generated music. This is in contrast with MT's reliance on a credentialed music therapist to perform any singing or instrument playing. However, MM can also be used to compliment music therapy (Wheeler et al., 2019).
Both MM and MT involve some form of Music Intervention (MI) that involves listening, singing, or making of music within a therapeutic context. If a subject self-administers a MI, it is defined as “music as medicine” (de Witte et al., 2020).
2.3. General music interventions
The therapeutic use of music is antiquitous and spans cultures, continents, and even millennia of human history where speculation of practices goes back to paleolithic times (West, 2000). There are historic texts such as the Jewish Old Testament where David plays the harp for the first King of Israel, Saul (ca. 1120 BCE). The playing of the harp offers Saul “relief”, makes Saul “feel better” by purging any “evil spirit” (New International Version, 1 Samuel 16:14–23). This early reference to music having a healing aspect is not alone. The thirteenth century saw an Islamic brotherhood in Meknes, Morocco, known as the Isawiyya, create music called Gnawa that was used to perform exorcisms (Shiloah, 2000), another example of music as a healer.
There's also traditional medicine from the Indian culture known as Suśrutasaṃhitā (ca. first millennium BCE). One of the prescribed practices within the Suśrutasaṃhitā was for individuals to “enjoy soft sounds, pleasant sights and tastes” after eating (Katz, 2000). The goal being to help facilitate digestion by listening to calming music. More recently, in 1840, the Czech physician Leopold Raudnitz published a book on music therapy. In his writings, he accounts experiences at a Prague insane asylum where patients suffering from delirium would “cease to babble” and patients with delusion demonstrated “marked improvement” (Kramer, 2000) after listening to music. And in 1891 a clergyman and musician, Frederick Kill Harford, organized a group of “musical healers”. They were named the Guild of St. Cecilia. The purpose of Harford's work was to “alleviate pain and relieve anxiety” (Tyler, 2000). Harford is considered a pioneer in music therapy. Over the last 100 years, therapeutic use of music has become more formalized with different approaches in intervention.
2.3.1. Music intervention overview
MT and MM have roots in other disciplines such as health science, psychology, and music education (Ole Bonde, 2019). Because of the multidisciplinary nature of MT and MM, therapists and researchers can tailor treatments in specific ways based on the patient. The approach can be curative or palliative and attempt to correct behaviors or provide personal growth. They can emphasize more artistic expression or provide a more scientific approach (Ole Bonde, 2019).
A common MI session requires the music therapist in MT or researcher in MM to prepare material needed for the subject. This includes preparing song lists, tuning instruments, playing live music, and preparing any pre-session and post-session surveys, all the while remaining neutral and keeping an emotional distance from the patient (Nygaard Pedersen, 2019). Playing live music for a patient based on request is called Patient Preferred Live Music (PPLM) (Silverman et al., 2016). Accredited music therapists are generally expected to have music backgrounds and thus be able to play musical instruments (Reimnitz and Silverman, 2020).
The music therapist's role in live performance requires musical training and education since their involvement in PPLM can involve playing guitar (Reimnitz and Silverman, 2020), singing, humming, or playing a body tambura (Kim S. et al., 2018). Various techniques with subtle changes to the performance can require the music therapist to slow the music tempo (de Witte et al., 2020) or utilize a technique known as dynamic rhythmic entrainment. Dynamic rhythmic entrainment entails bi-directional synchronization performed between “two rhythmic events” (Kim S. et al., 2018). In the context of music therapy, the therapist could change the tempo of their performance to match the patient's breathing. How therapists approach a MI may also vary.
2.3.2. Therapeutic models for music intervention
The use of MIs has a long history, but the creation of formalized models has only recently been developed. In 1999, the 9th World Congress of Music Therapy convened in Washington, USA, with the theme “Five Internationally Known Models of Music Therapy”. The five recognized models were Guided Imagery and Music (GIM), analytical-oriented music therapy, Nordoff-Robbins music therapy, Benenzon music therapy, and cognitive-behavioral music therapy (Jacobsen et al., 2019). Although there are other models in use, our survey paper focuses on these five models presented by the World Congress of Music Therapy.
The GIM model was developed by Helen Lindquist Bonny in the 1970s while working at the Maryland Psychiatric Research Center in the USA. This model utilizes classical music chosen by the therapist for playback while patients deeply relax, lying down with their eyes closed. During this process, the therapist helps the patient imagine scenarios of their choosing and guides calming thoughts around the scenario. After the session, which lasts up to 50 min, the patient is asked about their experience and how they feel. GIM has broad applications for patients struggling with addiction, trauma, and cancer (Bonny, 1989; Jacobsen et al., 2019).
Analytical oriented music therapy (AOM) is based on Analytical Music Therapy (AMT) by Mary Priestley (Aigen et al., 2021). AOM is a widely accepted approach in Europe and is a form of active music therapy. Music can be played tonally (i.e., using major or minor keys) or atonally (i.e., lacking tonality). There is importance placed on the relationship between the therapist, the patient, and the music. Both the intra-psychological and inter-psychological relationships are analyzed—specifically any transference between the patient and the musical instruments. Play rules are agreed upon which may include associating notes or chords to represent a specific emotion. A patient may decide to beat a drum to express frustration in this model.
The Nordoff-Robbins music therapy model is an improvisational method, created by Paul Nordoff and Clive Robbins (Kim, 2004). Nordoff, an American composer and pianist, and Robbins, a special educator, collaborated to create a model that was initially meant to treat children with learning disabilities. Focus is on allowing the patient to express themselves with various instruments such as drums, wind instruments, and various string instruments. Assessments include rating scale questionnaires to track progress as well as therapists taking notes (Jacobsen et al., 2019).
The Benenzon music therapy model requires patients to identify their “Musical Sound Identity,” which refers to the body sounds and other nonverbal communication that define their psychological state (Benenzon, 2007). Founded by Rolando Benenzon in 1966 in Buenos Aires, Argentina, the model originally focused on patients with autism and vegetative states. The process involves three stages: (1) warming up, (2) perception and observation, and (3) sonorous dialogue, which involves full, loud sounds. This model can be used on an individual basis or in group settings (Jacobsen et al., 2019).
Last, the Cognitive Behavioral Therapy (CBT) music therapy model has roots from the Second World War as therapists treated veterans with the goal of cognitive or behavior modification. Music stimuli is played, and participants can either participate (i.e., active music therapy) or listen (i.e., receptive music therapy) (Cognitive-Behavioural Music Therapy, 2019). The cognitive behavioral music therapy model has been used with children, patients with Parkinson's disease, autism, and eating disorders. Unlike the improvisational models, this model is structured and is a form of Cognitive Behavioral Therapy (CBT) where unwanted behaviors (e.g., destructive) are identified and the therapist helps the patient work toward changing the behaviors. The music stimuli are used to modify the behavior and results are measured by the therapist during a music therapy session.
2.3.3. Self-reported music intervention measurements
When treating stress for patients, questionnaire feedback helps direct the music therapist through an effective therapeutic process. Using questionnaires such as the Visual Analog Scale for Anxiety (VAS-A) and State-Trait Anxiety Inventory (STAI) have proven to be effective in treating stress. Both VAS-A (Hayes and Patterson, 1921) and STAI-Y1 (Spielberger et al., 1983) are psychometric measuring instruments that record participant stress rating and can be administered pre-experiment and post-experiment. VAS-A has a longer history, stemming from 1921. While they are both meant to measure stress, they do have some differences. Questions for the VAS-A questionnaire (see Table 1) use a continuous (analog) scale rather than Likert. Participants are presented with a short number of questions—typically between one and 10 questions. STAI-YI on the other hand uses Likert questions representing discreet choices. There are a few variations of STAI with Y1 presenting 20. Other versions of STAI can contain up to 40 questions.
VAS-A may be considered less accurate due to less questions being presented to participants (Labaste et al., 2019). However, VAS-A is shorter and so it is referred in heightened moments where a time-consuming questionnaire could be abandoned due to pain or anxiety (Ducoulombier et al., 2020). Despite their differences, there is significant correlation between the two types of measuring instruments (Delgado et al., 2018; Ducoulombier et al., 2020; Lavedán Santamaría et al., 2022).
2.3.4. Music intervention results for stress
MT and MM have both been used to treat a wide range of health issues, disabilities, and physical and psychological stressors. MIs have been used in addressing stress in cancer patients receiving blood or marrow transfusions (Reimnitz and Silverman, 2020), stress reduction in patients going through rehabilitation for Total Knee Arthroplasty (TKA) (Leonard, 2019), and lowering stress in various other settings such as organ transplants and chemotherapy (Silverman et al., 2016). Other areas where MI is being used include hospital psychiatry, patients with developmental disabilities, brain injuries, and palliative care patients (Jacobsen et al., 2019). MIs can address Generalized Anxiety Disorder (GAD) which goes beyond normal levels of stress that occur in day-to-day lives (Gutiérrez and Camarena, 2015). Patients of GAD have “chronic and persistent worry” (Stein and Sareen, 2015), insomnia (Wittchen and Hoyer, 2001), and susceptibility to substance abuse (Stein and Sareen, 2015).
Music interventions (MI) have been studied in specific health contexts and stressors, but they can also provide benefits to healthy patients and treat cases of daily stress in patients (Thoma et al., 2013; de Witte et al., 2022a,b). MI can help strengthen family relationships, including those with children with disabilities or mental instability, and can provide opportunities for bonding (Jacobsen et al., 2019). MIs can also be used to treat daily levels of stress, offer coaching, and be used in sessions for stress prevention (Daniels Beck, 2019). Subjects seeking personal growth can also benefit from MIs (Pedersen and Bonde, 2019).
2.3.5. Music interventions with live music
MT emphasizes the therapist playing live music vs. using pre-recorded playback. This is due to consensus within the music therapy industry that live performances are more effective in addressing and reducing stress (de Witte et al., 2020). Live music involves a combination of the therapist and the patient and is used in improvisational music therapy models and where active MIs are utilized.
Live music can be beneficial in group settings, especially when multiple patients are participating in the session. In a case involving a 27-year-old woman, Carla, with bipolar disorder, live music was used to help identify moments of mania during her rapid xylophone playing. The therapist responded with piano motifs, and other group members noticed Carla mumbling during her playing. This allowed Carla to identify one of her problems: having too many thoughts at once. After her sessions, Carla's resistance and psychotic behaviors had lessened (Nolan, 1991).
Live music also helps when patients are too young to play instruments or prefer songs familiar to them. One case study involved a 9-year-old boy, Robbie, with “severe emotional problems” due to being in foster care all his life. He was prone to violent reactions and remained distant. Robbie's sessions included singing various children's songs with the therapist, and in moments of stress, the therapist would play music to calm Robbie. Over 120 sessions were held and the analysis of the therapist included increased attention span, less disruptive behavior, and his verbal communication became more succinct (Herman, 1991).
2.3.6. Music interventions with pre-recorded music
While many MIs involving MT utilize improvisation and patient participation, there are situations where live music is impractical. An example case study involved a 22-year-old patient named Jerry, with autism. He liked to dance to music from radio and his record collection. The music therapist used Jerry's favorite recordings over several weeks. The results reported from the therapist showed that Jerry transformed from withdrawn and prone to violent outbursts, to having confidence in dancing and playing musical instruments (Clarkson, 1991).
Another example where it is more practical to use pre-recorded music is during moments of hospital care—such as going through labor during childbirth. A case involving a 30-year-old woman, named Annie, involved the process of a MI session during childbirth. Eight 90-min cassette tapes were prepared with classical music. The tapes were used before and during childbirth. Questionnaires were given before and after each session. The results from the questionnaires found that music therapy was effective in pain management (Allison, 1991).
2.3.7. Music as self-administered medicine
With limited access to music therapists and with increased consumer-grade biosensing wearables and smartphones available, individuals may simply choose to self-medicate. Smartphone users have application choices for therapeutic use of music. Table 2 shows apps that are addressing MI for relaxation, meditation, and stress. This table only includes applications for iPhone and may change quickly as this is a relatively new type of app and technology.
Most of the current apps offering therapeutic use of music do not include biosensing or self-administered feedback questionnaires. Current focus offers artists whose music can be downloaded based on a subscription rather than a digital music system that responds to biosensing coupled with ML. An app named Endel shown in Table 2 does offer biosensing (HR) and other inputs such as counted steps, current weather, time-of-day, and location. This application uses inputs to generate music that is contextual to the user's environment and biosensing capabilities of the device (McGroarty, 2020; Kleć and Wieczorkowska, 2021).
But a lack of adaptivity in feedback doesn't negate efficacy in self-administered MIs. Listening to classical music or self-selected songs reduces stress. Results from studies that have used either questionnaires such as STAI or biosensing such as heart rate and respiratory rate show self-administered MIs can reduce stress (Knight and Rickard, 2001; Burns et al., 2002; Labbé et al., 2007). Even in daily life, listening to music for the purpose of stress reduction has shown efficacy (Linnemann et al., 2015). However, in self-reported studies, the amount of time subjects listened to music was important. Listening to music for relaxation for more than 20 minutes showed significance whereas <5 min increased stress (Linnemann et al., 2018).
2.3.8. Binaural beats
Binaural beats share a relationship with acoustic beats which happen when a pair of sound waves with slightly different frequencies (e.g., 122 and 128 Hz) are played together from two different sound sources (e.g., speakers). The result is the difference between these two frequencies (e.g., 128–122 Hz = 6 Hz) and creates an auditory pulsating sound, known as the beat frequency.
These two soundwaves create both constructive and destructive interference—depending on where the two crest (i.e., high points) and where their troughs (i.e., low points) meet. Constructive interference is the result of the two soundwave crests meeting in the same place in time. This causes an amplification of the combined soundwave crests. Destructive interference is the outcome of one soundwave crest meeting the other soundwave trough which results in cancellation of the two with little or no audible sound.
Acoustic beats exist in the air around us and are detected by our brains. Binaural beats involve listening to two different frequencies played in each ear through headphones, and the brain identifies the difference. Research has shown that entraining the frequency of binaural beats with the brain can have a psychophysiological effect. Binaural beats in the beta range (13–30 Hz) have been shown to decrease negative mood (Lane et al., 1998), while those in the delta (0.1–4 Hz) or theta (4–8 Hz) ranges can reduce anxiety (Le Scouarnec et al., 2001; Krasnoff, 2021). Roughly 30% of surveyed systems use binaural beats for these purposes.
2.4. Biosensing instruments and measurements
MIs with therapists utilize pretest and posttest questionnaires such as VAS-A or STAI-YI. However, measurements may be subject to variance if too much time has passed between the session and questionnaires. Biosensing offers a finer granularity of analysis by recording real-time data from the patient as they interact with and experience a MT session.
The systems we surveyed used eight types of biosensing measurements: cardiovascular, brain activity, electrodermal (i.e., skin measurements), respiratory, and optical measurements. Figure 2 shows these types in the second column, labeled “Categories”. For biosensing measurements, there are different types of instruments that are used to acquire measurement data. It is important to identify the relationships between instruments and measurements are not one-to-one. For example, respiratory measurements (i.e., breathing data) can be extracted from a Respiratory Inductance Plethysmography (RIP) instrument, Electrocardiogram (ECG) electrodes, or a Photoplethysmography (PPG) sensor. When looking at cardiovascular measurements, some papers mention the R-R interval instead of Heart Rate (HR). R-R interval refers to the interval between heart beats in milliseconds whereas HR uses minutes. HR is obtained via ECG electrodes, a PPG sensor, a Seismocardiogram (SCG) instrument, or a Gyrocardiography (GCG) instrument.
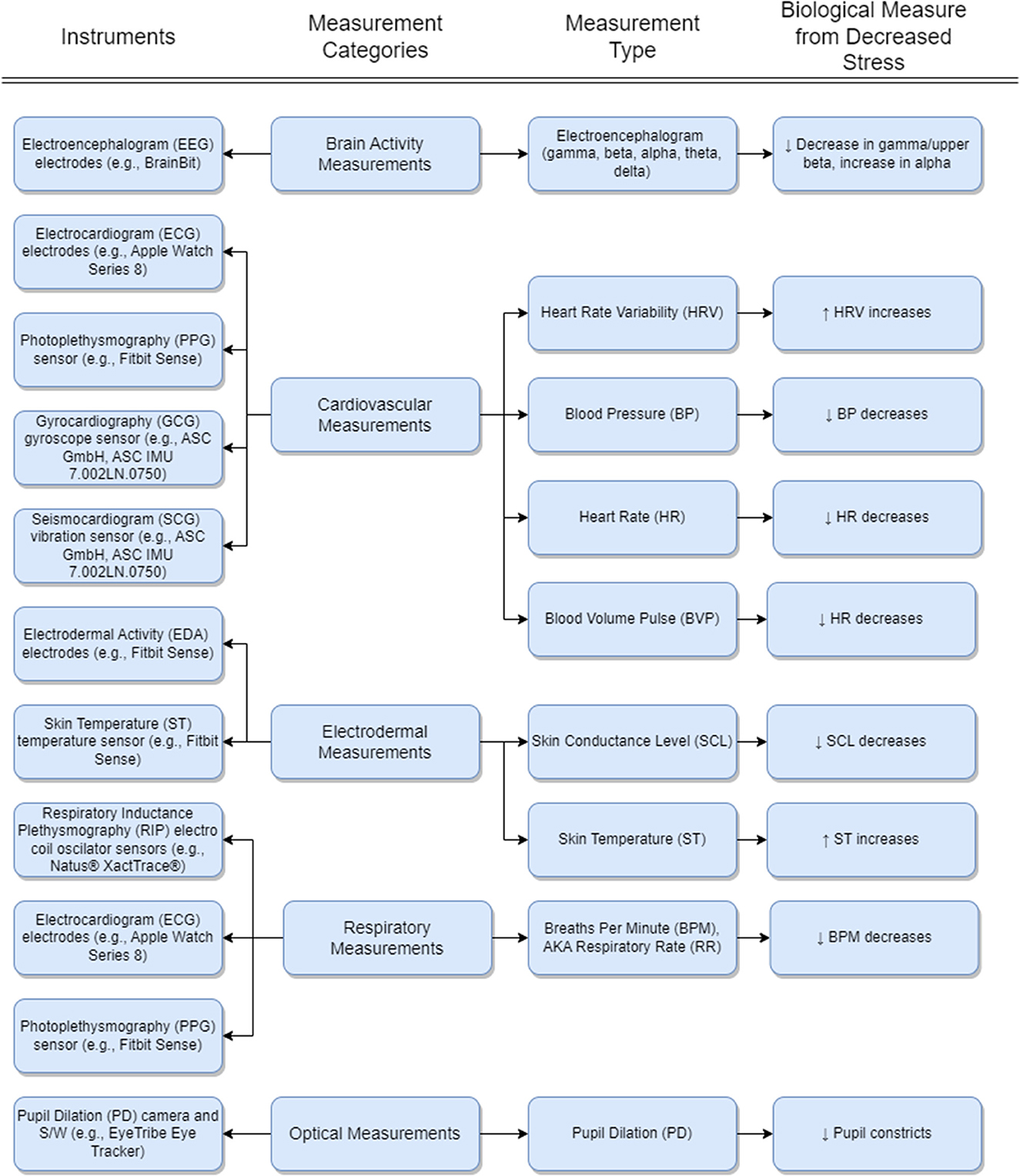
Figure 2. Biosensing instruments, measurements, and state-and-effect with music intervention. The up arrow ↑ represents an increase in biological measure with decreasing stress and the down arrow ↓ represents a decrease in biological measure with decreasing stress. Electrodermal Activity (EDA), Galvanic Skin Response (GSR), Electrodermal Response (EDR), and Psychogalvanic Reflex (PGR) are all considered synonymous where EDA is more recent and common to use.
And in the last 5 years, instrument technology has become less expensive and more accessible. Some instruments that have historically been accessible only by medical professionals are now readily available for consumer purchase. This includes portable consumer-grade Electrocardiogram (ECG) devices such as the LOOKEE® Personal ECG and Apple Watch Series 8. Electroencephalogram (EEG) devices such as the BrainBit headband and Interaxon's Muse 2 are readily ordered online for researchers and consumers alike. And the Natus® XactTrace® Respiratory Inductance Plethysmography (RIP) respiratory effort belt for respiratory measurements is also obtainable—in terms of availability and price.
Of the 10 surveyed digital music systems that we looked at, five used cardiovascular measurements, five used brain activity measurements, five used electrodermal measurements, two used respiratory measurements, and one used optical (i.e., pupil measurements). Lastly, five digital music systems used two or more types of measurements concurrently. Table 3 lists the biosensing measurements in the “Types of Feedback” column.
We note that not all wearable devices contain the same capabilities. Both the Apple Watch and the Fitbit Sense use PPG to capture HRV data. However, the Fitbit Sense can also capture Electrodermal Activity (EDA) and Skin Temperature (ST) data, whereas the Apple Watch does not. And Apple Watch Series 4 through 8 offer both a PPG sensor and an ECG electrode for cardiovascular measurements. In the following sub-sections, we discuss these instruments and measurements.
2.4.1. Brain activity instruments and measurements
To record brain activity, Electroencephalogram (EEG) electrodes are placed on a subject's scalp. Devices such as the consumer-grade BrainBit headband provide four electrodes that record electrical activity from the brain. The electrical signals are divided into five bands: gamma, beta, alpha, theta, and delta (see Table 4).
Brain activity is present in all bands although some may be more prominent than others based on a subject's mental state. The gamma band exists in the range between 30 and 45 Hz (Sanei and Chambers, 2007; Neurohealth, 2013) and is used for reading emotion (Li et al., 2018). It is active during learning, information processing, and cognitive function (Abhang et al., 2016). The beta band is between 13 and 30 Hz and associated with alertness and concentration (i.e., lower beta: 12–18 Hz) and to agitated or stressed states (i.e., higher beta: 18–30 Hz) (Garg et al., 2022).
Following is the alpha band between 8 and 13 Hz. Here, the brain is not actively processing information (Sanei and Chambers, 2007; Neurohealth, 2013) and is the range when a subject's eyes are closed but not sleeping. The theta band is between 4 and 8 Hz and is associated with intuition, creativity, daydreaming, and fantasizing (Sanei and Chambers, 2007; Neurohealth, 2013). Lastly the delta band, is between 0.1 and 4 Hz (Sanei and Chambers, 2007; Neurohealth, 2013). This band is most prominent when a subject is in deep and restorative healing sleep.
2.4.2. Cardiovascular instruments and measurements
Of our surveyed systems, the following cardiovascular measurements were used in detecting stress: HR, HRV, and Blood Volume Pulse (BVP) with HR and HRV being the more commonly used. And the following types of instruments are used: ECG, PPG, GCG, and SCG.
2.4.2.1. Cardiovascular instruments
Electrocardiogram (ECG or EKG) is the most common instrument used to record cardiovascular data. It senses the heart's electrical output through electrodes that record the depolarization wave generated as the heart beats. These waves result from the loss of negative charges inside the cells of the heart during each beat. The ionic currents generated in the body are converted into electrical signals that can be processed for various purposes (Bonfiglio, 2014, p. 5).
PPG is different from ECG as it uses a photodetector and green LED to measure heart rate and HRV. When a green light is shone at the skin, the photodetector detects the light, which is absorbed by blood due to its red color. The photodetector measures the resulting “volumetric variations of blood circulation” (Castaneda et al., 2018), also called BVP, which changes due to the heartbeat. As a result, PPG measures heart rate and HRV indirectly through changes in blood pressure and is sometimes used interchangeably with BVP.
The last two types of instruments are Seismocardiogram (SCG) devices, which detect vibrations from the beating of the heart at skin surface level, and Gyrocardiography (GCG) devices (Jafari Tadi et al., 2017) which use gyroscopic sensors to record heart motions (Sieciński et al., 2020). We focus only on ECG and PPG since they are the instruments used in the surveyed papers. GCG and SCG are mentioned for reference only.
2.4.2.2. Cardiovascular measurements
HRV and HR both use the beats-per-minute (i.e., heart contractions) whereas BPV and BP use the force exerted by the blood through the arteries. While HR and PR are not always identical, for most healthy subjects, the two are meant to measure the same thing: how many times per minute the heart beats. HR is based on the number of heart beats per minute—much like a metronome and HRV (shown in Figure 3) is the variations between the peaks of the R – R intervals over time. R-wave peak represents one heartbeat.
The intervals can be affected by many physical factors overtime including processes in the Sympathetic Nervous System (SNS) such as breathing (Shaffer and Ginsberg, 2017). When a subject inhales, HR increases and when a subject exhales, HR decreases. However, psychological effectors such as stress can also activate the SNS and therefore affect HR and HRV (Ziegler, 2004). Healthy subjects with little-to-no stress will have a higher HRV ratio due to HR being low when they are at rest or asleep. A subject that has high levels of stress have their SNS overriding the at-rest heart rate and therefore have an elevated HR. This lowers the HRV ratio (Kim H.-G. et al., 2018).
2.4.3. Electrodermal instruments and measurements
Electrodermal Activity (EDA) devices also utilize electrodes. In the case of EDA, the electrical signals are emitted from the device at a low constant voltage making it too low for human nerve detection. This low voltage helps measure the skin's conductance level over time which changes due to sweating.
Sweating is controlled by the SNS and affected by physical influences (e.g., temperature) as well as psychological effectors such as stress (Geršak, 2020). The psychologically induced sweating is independent of thermoregulatory sweating responses and a direct effect from emotional responses. Emotions such as fear, anxiety, and agitation can induce the psychologically sweating response (Geršak, 2020). EDA is used to measure Skin Conductance Level (SCL) which is measured over a period of seconds and minutes. Another electrodermal measurement is Skin Temperature (ST) which detects changes in surface skin temperature. ST lowers during stressors due to the SNS reducing peripheral vasoconstriction.
2.4.4. Respiratory instruments and measurements
The Respiratory Inductance Plethysmography input (RIP) device is an instrument used to measure pulmonary ventilation (i.e., breathing). The RIP measures volumetric changes in the rib cage and abdomen via wire coils in adhesive elastic bands that are connected to an oscillator. This wearable band is placed around the subject's rib cage. As the subject inhales, the circumference changes and affects the frequency in the wire coils. These changes are recorded via computer hardware and software.
Using a RIP to measure breathing is cumbersome as the subject is required to wear a band around their rib cage—which restricts movement and activity. ECG as well as PPG can provide respiratory data due to the changes in heart rate from breathing (mentioned previously with HRV). Both utilize software that can extract the breathing rate from the HR since the HR is affected by breathing controlled by the SNS. Breaths Per Minute (BPM), which is also known as the Respiratory Rate (RR), increases with exposure to stressors and decreases during relaxation (Widjaja et al., 2013).
2.4.5. Optical instruments and measurements
A pupilometer is the traditional device used for measuring Pupil Dilation (PD). PD is the widening of the pupil within the eye. Recent digital camera technology is used to replace a pupilometer due to the ubiquitous availability of inexpensive high-resolution digital cameras and software that records the changes.
Like HR and breathing, PD is affected by both physical responses (e.g., light with PD) and physiological effectors (e.g., stress), both under control of the SNS (Mokhayeri and Akbarzadeh, 2011). Eye tracking software that supports recording PD can record the diameter and the Pupil Dilation Acceleration (PDA) and perform interpolation for blinking. Both PD and PDA are useful measurements for tracking effectors such as stress (Zhai and Barreto, 2006) where stressors increase PD (Sege et al., 2020).
3. Surveyed systems and typology
We focused our survey on three main criteria for system inclusion. Systems that: (1) utilize music interventions in addressing stress—or a future ability to do so, (2) incorporate one or more forms of biosensing, and (3) use biosensing in an adaptive biofeedback loop, or with potential provisions to do so in the future.
Figure 4 shows the process that our surveyed systems either conform to or demonstrate the potential to meet. A session begins with the digital music system reading participant biosensing data where the data is any of the previously mentioned types. Data is preprocessed where down sampling, de-noising, or removing of unwanted artifacts is performed. In the case of EEG data (i.e., brainwave), when a participant blinks, talks, or moves parts of their body, artifacts occur and must be removed.
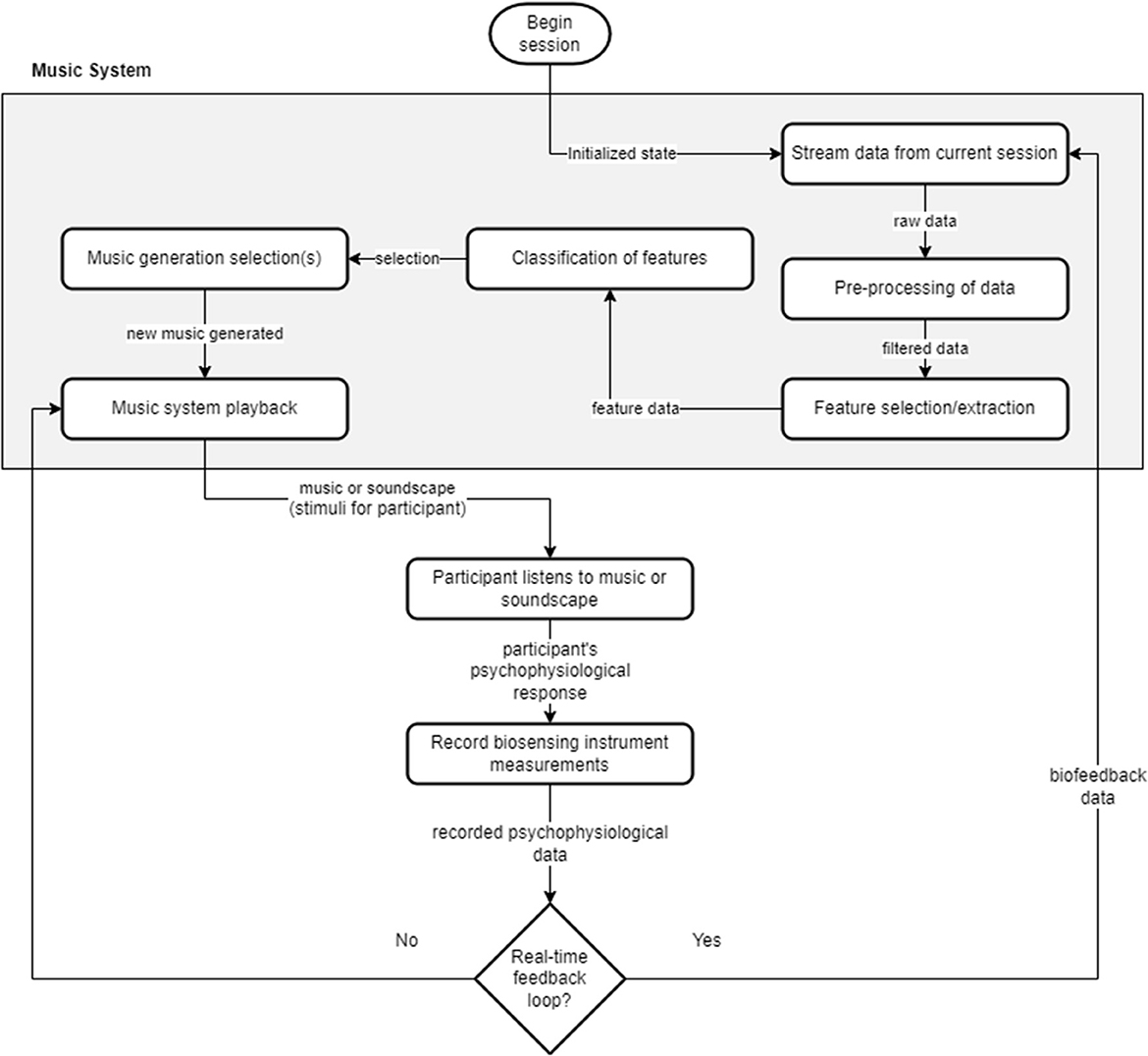
Figure 4. Digital music biofeedback loop. Rectangles inside of the digital music system represent components of the system. Rectangles outside of the music system represent external processes.
The preprocessed data is forwarded to the software component that chooses feature selection and extraction. An example of feature selection with EEG data would be choosing only beta and gamma bands, discarding information in the other three bands. Feature extraction in EEG data could capture statistical features such as mean, median, and standard deviation of a particular brainwave band (Stancin et al., 2021). Once features are selected or extracted—or both—the classification process can distinguish between the changes in features over time. With EEG data, this could involve comparing time series samples of the alpha band mean to determine whether generated music has affected participant brainwaves.
The resulting differences allow a selection of changes to be made (e.g., change track, alter tempo, add a new melody). This real-time feedback loop takes the biosensing data and turns it into a biofeedback loop. In the surveyed systems that we analyzed, the following types of changes in music playback were made: change in track, change in genre, pitch, tone, and amplitude.
When looking at digital music systems, we chose more recent papers within the last 5 years due to a couple of factors. First, as mentioned in the introduction, the advent of consumer-grade biosensing wearables has only become commonplace within the last 5 years. Second, we wish to concentrate on digital music systems that either use Machine Learning (ML) techniques for addressing stress or offer future potential for adding this feature. To the best of our knowledge, we've included all digital music systems that meet our three main criteria.
Table 2 shows the systems in our survey. The first column contains a circled number. We use these numbers to reference each of the systems throughout the rest of this paper (e.g., ① for system one, ② for system two, etc.). Column six identifies whether the system uses biosensing to create a biofeedback loop. Next, we analyze how each of the systems compares to our three main criteria. We divide the systems into two main sections: those using biosensing only, and systems that complete the biofeedback loop.
3.1. Music interventions with biosensing only
System ① employs yoga videos with yoga chakras to induce relaxation and reduce stress while recording HR, HRV, and GSR biosensing data. This five-step experiment consisted of the following phases: (1) baseline, (2) task one, (3) rest, (4) task two, and (5) post rest. Both groups watched the yoga video with ambient music for task one. For task two, Group A watched only the yoga video and Group B listened to just the ambient music. System ① did not replicate the same findings with HRV that have been found in previous research (Kim H.-G. et al., 2018): relaxation increases HRV and cognitive load or stress decreases it. Their study does show consistent results in GSR changes: relaxation decreases GSR. The researchers attribute their failure to find significance with HRV due to their protocol being short in duration (i.e., 3.5 min per task).
This system used pre-extracted video and audio YouTube videos which were not changed during the experiment and no biofeedback loop was created. After the experiment, all data was run through the following Matlab “Classification Learner” classifiers: “tree, linear discriminant, quadratic discriminant, logistic regression, support vector machine (SVM) and k-nearest neighbor (KNN)” (Wu et al., 1996; Nick and Campbell, 2007; Kramer, 2013; Suthaharan, 2016; Tonacci et al., 2020). The results from the classifiers showed significance with HR and GSR but not HRV. While this system demonstrates the ability of the ML classifiers to determine changes in participant biosensing data as they listen and watch YouTube videos, the system would require a biofeedback loop as shown in Figure 4.
Researchers with system ③ used two Generative Adversarial Networks (GANs) that play Harmonic Music Intervals (HMIs) Wang et al. (2017). HMIs are sets of notes that are played simultaneously to create harmony. Participants listened to 24 HMIs in random order which were created from 12 synthetic harmonic sounds. These were played for 10 seconds each to subjects.
GAN one's discriminator is given human generated HMIs, along with the HMIs created from the generator. The generator uses HRV data from human subjects (via two ECG electrodes) as input. The generator in GAN one reached an accuracy of 0.53 for real data and 0.52 for generated, meaning its HMIs are comparable to the human create data. Thus, using HRV data as input from subjects can be used to create HMIs. And GAN two's generator used audio data as input to generate HRV data for the discriminator, with the discriminator using HRV data from subjects. GAN two's discriminator reached an accuracy of 0.56 for real data and 0.51 for generated data.
Due to the complexity of this study, the researchers did not complete determining whether the system could raise HRV from a lower state as they were not able to test GAN-to-GAN. However, their contribution demonstrates a working model and architecture that can be used in the management of stress. And GAN one could use a set point to decide whether the loop increases the HRV and thus induces relaxation where the HRV data is either generated by GAN two or input from subjects in real-time.
The researchers that built system ⑦ utilize EEG signals from participants. During the experiment, participants watched pre-recorded video, listened to pre-recorded meditative music, or used their own thoughts to elicit an emotion from four categories: angry, calm, happy, and sad, following the circumplex emotion model (Figure 1). Classifiers, such KNN were used to identify emotions that participants were feeling—based on their EEG data. One of their measures of success was finding 15 out of 20 EEG signals from participants successfully transformed to the relaxed brain wave band: alpha.
While not real-time in delivery, or generation of music, this system demonstrates a strong ML component that shows potential for creating a digital music system using biofeedback with EEG. Participant EEG data is used with several classifiers where the results are compared against what emotion state participants stated that they are in. The classifiers chosen for this system were: KNN, a Convolutional Neural Network (CNN), a Recurrent Neural Network (RNN) (Jain and Medsker, 1999), and a Deep Neural Network (DNN). The KNN classifier was the most accurate.
This study would benefit by using a corpus of MER data such as PMEmo or DEAM since participant stated emotion may not be as accurate. MER, PMEmo, and DEAM are datasets that map song excerpts to emotions based on the Circumplex Model (Figure 1). This could be used to either generate the music or to help a music recommender component provide music based on the emotion that the participant wishes to feel, providing a unique User Experience (UX).
System ⑧ incorporates classical, instrumental, and pop music for its playback. Instrumental music was paired with binaural beats, instrumental rock was coupled with gamma binaural beats (i.e., 30–45 Hz), and instrumental jazz was coupled with alpha binaural beats (i.e., 8–12 Hz). Analysis of EDA, HR (via BVP), ST, and PD were recorded from participants during the experiment. Participants listened to 12 pieces of music that were played for ~4 min each. Like systems ⑤ and ⑦, system ⑧ employed the circumplex model (Figure 1).
Participants filled out a questionnaire where they rated each song with a series of Likert scale questions pertaining to the emotion of the song (e.g., sad to happy, unpleasant to pleasant, etc.). Both the questionnaire data and the biosensing data were used to classify a song's genre. And both provided significance in doing so. While system ⑧did not use biosensing to reduce participant stress, their focus was on the ability to classify songs where participant feedback rates songs as being helpful for reducing stress.
The researchers used a KNN and a SVM for classification of biosensing data offline. However, they also used a unique visual approach they called “Gingerbread Animation” (Rahman et al., 2021). A two-dimensional figure of a gingerbread man was filled in with color from the EDA, HR, and ST biosensing data and updated over time: EDA represented as red, HR as blue, and ST as green. These colors overlap, creating other colors (e.g., purple, orange, etc.). If the biosensing data values are not updated (due to lack of change in biosensing data), their color intensity diminishes, like ripples in a pond.
These animated changes of the gingerbread figure were used as input for a CNN where the CNN determined changes in emotion based on the changes in color. The researchers found that that the CNN performed best within their experiment. This digital music system provides a unique approach using a CNN for classification of emotion change and could use a custom dataset for better accuracy.
3.2. Music interventions with biofeedback loop
In system ②, researchers played a combination of music and binaural beats in the 10 Hz range (i.e., alpha range) to treat stress while recording EEG data. The experiment consisted of four phases: (1) music only, (2) music and alpha wave binaural beats, (3) music and theta wave binaural beats, and (4) music and a customized binaural beat. Each of the phases lasted 5 min. The fourth phase was chosen by the digital music system based on which of the two wave sets increased while the participant listened: either alpha or theta bands. The results were concordant with previous studies that utilized similar test procedures: alpha wave binaural beats increase participant brain alpha wave activity (Capili et al., 2018) and therefore promote a relaxed state.
Since the biosensing data was used to select binaural beat wavelengths, this digital music system has a biofeedback loop. There are several ways in which ML could be employed with system ②. Rather than update within the last phase of the session, real-time ML can be used to update the binaural beat band every minute as well as change the played song or transition from one song to another. To support real-time, a data set such as MER including PMEmo (Zhang et al., 2018) is needed with the ML to select musical choices to offer a more personalized relaxation experience.
System ④ plays ambient music in blocks of time for the treatment of stress. The experiment consisted of seven time-blocks in total, each lasting ~ 7 min. During all seven time-blocks, biosensing was recorded and participants were required to perform the task of pressing a key on a computer keyboard when hearing an “alarming short buzzing sound” (Leslie et al., 2019). The first four time-blocks were used for control and the last three time-blocks were music interventions: (1) fixed tempo, (2) personalized tempo to breathing, and (3) personalized amplitude to breathing.
For the biofeedback loop, only the BPM was used although HRV, EEG, and EDA data were recorded for later analysis. HRV data did not demonstrate significance between baseline and intervention phases while EDA, EEG, and respiratory biosensing did. The respiratory biofeedback's calming effect of breathing was validated by using z-score as a baseline. Z-score for respiratory biofeedback is calculated by how many Standard Deviations (SDs) from the mean BPM where the mean BPM is calculated based on age, gender, and height.
The ambient music was pre-recorded, but their digital music system changed the tempo during playback based on the interventions. Two ways in which breathing changed the ambient music: (1) personalized tempo, and (2) personalized envelope. For the breathing changes for personalized tempo, the amplitude was calculated at 75% of the participant BPM with a limit placed on potentially high breathing rates at 15 beats per minute. The personalized envelope offered a more real-time approach where inhaling and exhaling produced notes generated. Since EEG and HR were utilized in this digital music system, a ML component could compare this biosensing data against datasets to determine if the entrainment also reduces HR and places participants into alpha or theta bands. This would require HR, HRV, and EEG datasets for real-time biofeedback.
System ⑤ contains a recommender digital music system that uses song samples from the PMEmo Dataset for Music Emotion Recognition (MER) (Zhang et al., 2018) which consists of various tracks—mostly from current pop-music. This study was unique in that their focus was to detect stress rather than detect relaxation. During song playback, participants' EEG and HR (via ECG) were analyzed and compared against the circumplex model (Figure 1). The participant biosensing data was compared to the PMEmo dataset. By comparing participant EEG to the PMEmo dataset, they were able to detect certain playback songs that became stressors for participants: EEG moved from theta or alpha to upper beta.
Like system ①, system ⑤ compares classifiers within a recommender digital music system. Participant EEG, HR, and GSR data is compared against MER datasets (PMEmo and DEAM). SVM and random forest classifiers are used for emotion classification to detect stress. With the ability of this digital music system to detect whether a song creates stress, this study could be adapted to perform real-time changes during user playback based on the biosensing data that the researchers recorded to nudge user state into one of relaxation.
System ⑨ plays binaural beats, ambient music, and monk chants to address anxiety while recording participant EEG data. The survey paper included the digital music system for the purpose of an exhibition rather than a research study. The 38 participants were each given 6 min and 45 s per session where they listened to music and watched an animated mandala (i.e., circular symmetric geometrical shape) atop a milky way galaxy background in Virtual Reality (VR).
The biosensing data (EEG) in system ⑥ changes both the mandala visuals and the audio; the breathing drives the patterns. While the EEG data was used to influence the visuals as well as the audio (binaural beats were not influenced), the researchers did not measure significance in whether the music changed brain waves from beta to alpha or theta. This, however, was mentioned as a future consideration for their digital music system.
The digital music system was implemented in Unity using a plugin known as chucK script. This script allows programmers to manipulate audio in real time such as volume, create sine oscillators, and create reverb effects. The researchers used chucK to affect the volume levels of both the monk chants as well as the ambient music. This was performed by using average values of the EEG brainwave data. Because chucK has many other parameters that are configurable, the EEG biosensing data could be controlled by classifiers that use the circumplex model to control the music and the monk chants. For example, if the ML component detects participant anxiety (based on the arousal and valence emotion model), the volume of the music, monk chants, or both, could be reduced.
System ⑨ provides ambient music playback and nature soundscapes. HR, SCR, BPM, and HRV biosensing data were recorded. Participants were put into either a Control Group (CG) or an Experimental Group (EG) and run through three phases: (1) baseline phase (i.e., rest and relax), (2) stress phase (i.e., mentally challenging task), and (3) a relaxation phase. The CG listened to both soundscape, then soundscape and music together—with no adaptivity. The EG listened to soundscape, then soundscape and music together where the respiratory biosensing data was used to control the amplitude of the wind creating a biofeedback loop. At the end of the session both groups of participants filled out State-Trait Anxiety Inventory (STAI) and Rumination Response Scale (RRS) surveys. Results from the experiment demonstrated that HRV was raised, and BPM was decreased.
Because the researchers use a PPG sensor for cardiovascular measurements, BPM biosensing data was obtained and used by the music system to affect the amplitude of the generated wind sound. Since significance was found with both BPM and HRV this biofeedback system could incorporate a form of ML such as a classifier and compare against a dataset for real-time updates to not only change the wind amplitude, but also generation of ambient music as well.
Like system ⑥, system ⑩ was created for exhibition purposes. Ambient sounds came in the form of quartal and quintal piano harmonies which can avoid tensions in tonal harmony (Tatar et al., 2019). It utilized BPM biosensing data from participants and immersed them in a VR environment where breathing controlled their altitude within a 3D environment with ocean waves below. Lower BPM also decreased the calmness of the ocean waves, encouraging slower respiratory rate, and thus a relaxed state. Because this digital music system encourages slower breathing, it provides insight into how future digital music systems can help encourage relaxation from stress using ambient music and VR.
The exhibition digital music system in ⑩ also uses breathing to affect the music that it generates: quartal and quintal piano harmonies. Because this system only uses respiratory measurement, the addition of other biosensing data could help offer more real-time parameter changes. And the BPM is obtained by a device that measures BVP so that both breathing as well as HR and HRV could be utilized for other parameters. This would allow for comparison against a dataset of heart data to offer real-time changes based on a digital music system using ML.
3.3. Software adaptivity approaches to biofeedback
We saw two main approaches that researchers either chose or posited would work with their systems: (1) provide a non-ML algorithm that uses biosensing data as stimuli to change music delivery, or (2) utilize ML techniques that are used offline.
Both approaches have their merits. The first approach provides immediate results that can validate the sometimes-complicated workflow when acquiring biosensing data. An example of this workflow requires researchers to first ensure no environmental stimuli will invalidate the acquisition of data (e.g., hot temperature skews GSR data, neck movement or blinking affects EEG data, etc.). Next, the data stream may include some denoising or filtering. Without any ML involved, any algorithms used provide basic choices—such as whether participant alpha or theta bands increased more than the other—as found in system ②.
The second approach can demonstrate effectiveness of a particular ML strategy where processing may include denoising, down-sampling, filtering of data, time-synchronizing with other biosensing data, feature extraction, and finally ML such as classification. Other choices include which ML to use: classifiers, Generative Adversarial Networks (GANs) as in system ③, or even a Genetic Algorithm (GA)—found in system ⑧. Once a chosen approach is made, researchers may need some time to acquaint themselves with any frameworks, configuration, and hardware requirements. Finally, researchers need to choose a training set or budget in time to make their own. Seeing the amount of effort required to design each of these two approaches provides insight into why many research endeavors currently choose one approach or another rather than both.
4. Conclusion
The digital music systems that we've looked at show promise in providing therapeutic use of music to subjects who seek MIs. By processing biosensing data within a digital music system, the biofeedback loop provides real-time adaptivity with a promise of increased efficacy if the digital music system can provide immediate tailoring of a subject's music needs.
If this technology is implemented on mobile computing devices such as smartphones and consumer-grade wearables with biosensing data instruments, music therapists and researchers can use this technology—both during therapy as well as outside of therapy. Subjects can self-administer a MI session in a place and time of their convenience. This would help address limited access to music therapists and researchers. But there are challenges and questions that need to be addressed.
4.1. Current digital music system approaches and limitations
Of the digital music systems that we surveyed, only six out of the ten were adaptive. And of the six systems that were adaptive, only one utilized ML classifiers. None offered a real-time ML-based adaptive solution. Further, of the 10 systems that we analyzed, none were implemented on smartphones connected to consumer-grade biosensing wearables.
Moving in that direction, we see two limited or emerging areas that hinder ubiquity of using a mobile-computing, real-time ML-based adaptive digital music system for MIs for treatment of stress: (1) training sets and data for digital music systems, and (4) consumer-grade biosensing data instruments.
4.1.1. Limited training sets for digital music systems
Training sets such as the MediaEval Database for Emotional Analysis of Music' (DEAM), PMemo, and the MIREX like Mood dataset for emotion classification are relatively new and do not contain the vast amounts of data that other branches of AI have already employed such as Chat Generative Pre-Trained Processor (ChatGPT).
There are older projects that have also explored the relationship between music or sound, biosensing data, and emotion. Database for Emotion Analysis using Physiological Signals (DEAP) has been used for emotion analysis and contains data from 32 participants watching 40 one-minute snippets of music videos. The data collected includes EEG, peripheral physiological signals (e.g., GSR, respiration amplitude, ST, ECG, etc.), and Multimedia Content Analysis (MCA) data [e.g., Hue, Color, Value (HSV) average and standard deviation, etc.] (Koelstra et al., 2012). Unfortunately, DEAP has not been updated since 2012.
This limited support of pretrained data is no better demonstrated than by looking at how many of these digital music systems utilize pretrained datasets. Of the 10 systems that we surveyed, only system ⑤ used existing pretrained data sets: DEAM and PMemo. The rest of the systems provided their own training sets.
4.1.2. Limited consumer-grade biosensing instrumentation
Many of the consumer-grade biosensing data wearables are relatively new in both features as well as in developer support. For recording cardiovascular and respiratory data, Apple provides their Apple Watch series currently at series 8. Samsung, the top manufacturer of Android smartphones, has their Galaxy Watch5, and company Fitbit offers their Versa 4 device. All devices provide recording of HR, HRV, and respiratory data using either PPG or ECG.
For EEG wearables, the bio-tech company EMOTIV has offered the Muse EEG headset with the Muse 2 being the most recent. Unfortunately, since 2020 EMOTIV restricted developer access to the Software Development Kit (SDK) that allows reading data from the Muse EEG headsets. This has made it difficult for developers and researchers to utilize the power of the four electrode EEG headset. Another recent bio-tech company, BrainBit, also offers a four-electrode wearable EEG headband that is comparable with EMOTIV's Muse 2. Unlike EMOTIVE's Muse 2, the BrainBit SDK is not restricted, and developers and researchers are free to download their SDK.
4.2. The ethics of self-medicating
Allowing subjects to initiate their own MI after being onboarded by a music therapist does present a potential ethical dilemma. Are we taking jobs away from trained therapists and replacing them with AI? This is quite a topical concern where AI technologies such as ChatGPT can perform human tasks (e.g., author research papers, write poems, write program code, etc.) just as well, or better than humans—as well as faster. By providing a mobile computing solution with biosensing data support that uses ML for the adaptivity of the digital music system, patients may decide that a music therapist is too expensive or difficult to schedule with and simply download the app and treat themselves. Self-treatment may not provide the efficacy that a trained professional provides.
This not only presents a potential threat of replacement of music therapists, but it also creates a danger that without the expertise of a music therapist, a patient may not successfully treat themselves in a manner that meets their needs. Currently, music therapists are accredited by degree programs. An app and technology without direction from a Subject Matter Expert (SME) could be detrimental to patient health. However, if these systems are used in tandem with a music therapist, or used to address stress in daily lives, then there are benefits to this digital music system.
4.3. Future direction
With the Internet of Things (IoT), ubiquity of Internet connectivity, and consumer-grade biosensing wearables, mobile apps can send all relevant session data to a cloud service for further processing. This allows music therapists and researchers to remotely monitor subject progress. This could help alleviate the restricted availability of music therapists and researchers.
The wearable apps that provide biosensing, such as a smartwatch and EEG headset would communicate biosensing data in real-time to the smartphones digital music system app. The app incorporates ML techniques that were discussed in Section 3 and shown in Figure 4. After each session, data summaries are sent to a central server where researchers or music therapists can analyze the results via a web interface. This digital music system currently does not exist and many of the hardware and software features that we've discussed in this section are emerging and continually changing.
In the introduction, our survey research questions we posited were as follows:
(1) Can therapeutic use of music be conducted with wearable biosensing computing devices?
(2) Can digital music intervention systems yield results that can address stress and anxiety?
Both questions (1) and (2) may be answered soon by utilizing the current offering of consumer-grade biosensing wearables for brain, cardiovascular, electrodermal, and respiratory data. Working together, researchers and music therapists could create a standardized framework with ample training-set data for successful classification of stress across biosensing instruments. The training sets would allow for low-shot ML (Hu et al., 2021), significantly decreasing the computing time of a digital music system. This could allow a mobile app to perform the ML locally rather than utilizing server-side cloud services which require Internet connectivity. With more formalized and larger datasets, it may be easier to identify which algorithms are more accurate and efficient at providing efficacy in MIs for addressing stress.
Lastly, how music is being ontologized is changing as well. Less importance is being placed on music genres with more emphasis on music's intended purpose. This approach has roots going back to the early 1930s from a company known as Muzak where the purpose was to affect the listener. Music could be used to relax patients waiting in a doctor's office by playing soft melodies.
This approach is known as functional music and complements what we've already discussed with music successfully being used to improve relaxation and lower stress. Of the studies that we looked at, ambient, meditative, and soundscape genres offered successful results as did binaural beats. Their function is to relax and offer a meditative mood for participants. Further research is needed to see if there are any other genres that fit into the same functional music classification and which combinations provide the best efficacy. Applying all these changes together may provide a digital music system that generates music to complement the expertise of music therapists.
Author contributions
AF wrote the paper. PP provided guidance, editing, feedback, direction, and mentorship. CC provided guidance and feedback. All authors contributed to the article and approved the submitted version.
Funding
This work was supported by the Natural Sciences and Engineering Research Council of Canada (NSERC).
Conflict of interest
The authors declare that the research was conducted in the absence of any commercial or financial relationships that could be construed as a potential conflict of interest.
Publisher's note
All claims expressed in this article are solely those of the authors and do not necessarily represent those of their affiliated organizations, or those of the publisher, the editors and the reviewers. Any product that may be evaluated in this article, or claim that may be made by its manufacturer, is not guaranteed or endorsed by the publisher.
References
Abhang, P. A., Gawali, B. W., and Mehrotra, S. C. (2016). “Chapter 3 - Technical aspects of brain rhythms and speech parameters,” in Introduction to EEG- and Speech-Based Emotion Recognition, eds P. A. Abhang, B. W. Gawali, and S. C. Mehrotra (Cambridge: Academic Press), 51–79.
Adolfsson, A., Bernal, J., Ackerman, M., and Scott, J. (2019). “Musical mandala mindfulness: A generative biofeedback experience,” in Proceedings of the 6th International Workshop on Musical Metacreation (Charolette, NC).
Adwas, A., Jbireal, J., and Azab, A. (2019). Anxiety: insights into signs, symptoms, etiology, pathophysiology, and treatment. S. Afr. J. Med. Sci. 2, 80–91.
Aigen, K., Harris, B. T., and Scott-Moncrieff, S. (2021). The inner music of analytical music therapy. Nordic J. Music Ther. 30, 195–196. doi: 10.1080/08098131.2021.1904667
Allison, D. (1991). “Music therapy at childbirth,” in Case Studies in Music Therapy, ed K. E. Bruscia (Gilsum, NH: Barcelona Pub), 529–546.
Benenzon, R. O. (2007). The Benenzon model. Nordic J. Music Ther. 16, 148–159. doi: 10.1080/08098130709478185
Bonfiglio, A. (2014). “Physics of physiological measurements,” in Comprehensive Biomedical Physics, ed A. Brahme (Oxford: Elsevier), 153–165.
Bonny, H. L. (1989). Sound as symbol: guided imagery and music in clinical practice. Music Ther. Perspect. 6, 7–10. doi: 10.1093/mtp/6.1.7
Bradt, J., Potvin, N., Kesslick, A., Shim, M., Radl, D., Schriver, E., et al. (2015). The impact of music therapy versus music medicine on psychological outcomes and pain in cancer patients: a mixed methods study. Support. Care Cancer 23, 1261–1271. doi: 10.1007/s00520-014-2478-7
Burns, J. L., Labb,é, E., Arke, B., Capeless, K., Cooksey, B., Steadman, A., et al. (2002). The effects of different types of music on perceived and physiological measures of stress. J. Music Ther. 39, 101–116. doi: 10.1093/jmt/39.2.101
Capili, J., Hattori, M., and Naito, M. (2018). Computational Music Biofeedback for Stress Relief . Santa Clara, CA: Santa Clara University.
Castaneda, D., Esparza, A., Ghamari, M., Soltanpur, C., and Nazeran, H. (2018). A review on wearable photoplethysmography sensors and their potential future applications in health care. Int. J. Biosens. Bioelectron. 4, 195–202. doi: 10.15406/ijbsbe.2018.04.00125
Clarkson, G. (1991). “Music therapy for a nonverbal autistic adult,” in Case Studies in Music Therapy, ed K. E. Bruscia (Phoenixville, PA: Barcelona Pub), 373–386.
Cognitive-Behavioural Music Therapy (2019). A Comprehensive Guide to Music Therapy, 2nd Edn. London: Jessica Kingsley Publishers.
Daniels Beck, B. (2019). “Music therapy for people with stress,” in A Comprehensive Guide to Music Therapy, 2nd Edn, eds I. Nygaard-Pedersen, S. L. Jacobsen, and L. O. Bonde (London: Jessica Kingsley Publishers), 76–92.
de Witte, M., Knapen, A., Stams, G. J., Moonen, X., and van Hooren, S. (2022a). Development of a music therapy micro-intervention for stress reduction. Arts Psychother. 77, 101872. doi: 10.1016/j.aip.2021.101872
de Witte, M., Pinho, A., Stams, G. J., Moonen, X., Bos, A. E. R., and van Hooren, S. (2022b). Music therapy for stress reduction: a systematic review and meta-analysis. Health Psychol. Rev. 16, 134–159. doi: 10.1080/17437199.2020.1846580
de Witte, M., Spruit, A., van Hooren, S., Moonen, X., and Stams, G. J. (2020). Effects of music interventions on stress-related outcomes: a systematic review and two meta-analyses. Health Psychol. Rev. 14, 294–324. doi: 10.1080/17437199.2019.1627897
Delgado, D. A., Lambert, B. S., Boutris, N., McCulloch, P. C., Robbins, A. B., Moreno, M. R., et al. (2018). Validation of digital visual analog scale pain scoring with a traditional paper-based visual analog scale in adults. J. Am. Acad. Orthop. Surg. 2, e088. doi: 10.5435/JAAOSGlobal-D-17-00088
Ducoulombier, V., Chiquet, R., Graf, S., Leroy, B., Bouquet, G., Verdun, S., et al. (2020). Usefulness of a visual analog scale for measuring anxiety in hospitalized patients experiencing pain: a multicenter cross-sectional study. Pain Manage. Nurs. 21, 572–578. doi: 10.1016/j.pmn.2020.03.004
Ehrlich, S. K., Agres, K. R., Guan, C., and Cheng, G. (2019). A closed-loop, music-based brain-computer interface for emotion mediation. PLoS ONE 14, 1–24. doi: 10.1371/journal.pone.0213516
Essau, C. A., Lewinsohn, P. M., Lim, J. X., Moon-ho, R. H., and Rohde, P. (2018). Incidence, recurrence and comorbidity of anxiety disorders in four major developmental stages. J. Affect. Disord. 228, 248–253. doi: 10.1016/j.jad.2017.12.014
Fink, G. (2016). Stress: The Health Epidemic of the 21st Century. Research. Elsevier SciTech Connect. Available online at: https://scitechconnect.elsevier.com/stress-health-epidemic-21st-century (accessed April 26, 2016).
Garg, A., Chaturvedi, V., Kaur, A. B., Varshney, V., and Parashar, A. (2022). Machine learning model for mapping of music mood and human emotion based on physiological signals. Multimed. Tools Appl. 81, 5137–5177. doi: 10.1007/s11042-021-11650-0
Gold, C., Erkkilä, J., Bonde, L. O., Trondalen, G., Maratos, A., and Crawford, M. J. (2011). Music therapy or music medicine? Psychother. Psychosom. 80, 304–304. doi: 10.1159/000323166
Gutiérrez, E. O. F., and Camarena, V. A. T. (2015). Music therapy in generalized anxiety disorder. Arts Psychother. 44, 19–24. doi: 10.1016/j.aip.2015.02.003
Hayes, M. H. S., and Patterson, D. G. (1921). Experimental development of the graphic rating method. Psychol. Bull. 18, 98–99.
Herman, F. (1991). “The boy that nobody wanted: creative experiences for a boy with severe emotional problems,” in Case Studies in Music Therapy, ed K. Bruscia (Gilsum: Barcelona Pub), 99–108.
Hu, Y., Chapman, A., Wen, G., and Hall, W. (2021). What can knowledge bring to machine learning? - A survey of low-shot learning for structured data. arXiv [Preprint]. arXiv: 2106.06410. Available online at: https://arxiv.org/pdf/2106.06410.pdf
Idrobo-Ávila, E., Loaiza-Correa, H., Muñoz-Bolaños, F., van Noorden, L., and Vargas-Cañas, R. (2022). Development of a biofeedback system using harmonic musical intervals to control heart rate variability with a generative adversarial network. Biomed. Sign. Process. Cont. 71, 103095. doi: 10.1016/j.bspc.2021.10309
Jacobsen, S. L., Pedersen, I. N., and Bonde, L. O. (2019). A Comprehensive Guide to Music Therapy, 2nd Edn. London: Jessica Kingsley Publishers.
Jafari Tadi, M., Lehtonen, E., Saraste, A., Tuominen, J., Koskinen, J., Teräs, M., et al. (2017). Gyrocardiography: a new non-invasive monitoring method for the assessment of cardiac mechanics and the estimation of hemodynamic variables. Sci. Rep. 7, 6823. doi: 10.1038/s41598-017-07248-y
Jain, L., and Medsker, L. (1999). Recurrent Neural Networks: Design and Applications, 1st Edn. CRC Press, Inc.
Johnson, H. M. (2019). Anxiety and hypertension: is there a link? A literature review of the comorbidity relationship between anxiety and hypertension. Curr. Hypertens. Rep. 21, 66. doi: 10.1007/s11906-019-0972-5
Katmah, R., Al-Shargie, F., Tariq, U., Babiloni, F., Al-Mughairbi, F., and Al-Nashash, H. (2021). A review on mental stress assessment methods using EEG signals. Sensors 21, 5043. doi: 10.3390/s21155043
Katz, J. B. (2000). “Music therapy: Some possibilities in the indian tradition,” in Music as Medicine The History of Music Therapy Since Antiquity, 1st Edn, ed P. Horden (London: Routledge), 84–102.
Kersten, P., Küçükdeveci, A. A., and Tennant, A. (2012). The use of the visual analogue scale (VAS) in rehabilitation outcomes. J. Rehabilit. Med. 44, 609–610. doi: 10.2340/16501977-0999
Kim, H.-G., Cheon, E.-J., Bai, D.-S., Lee, Y. H., and Koo, B.-H. (2018). Stress and heart rate variability: a meta-analysis and review of the literature. Psychiatry Investig. 15, 235–245. doi: 10.30773/pi.2017.08.17
Kim, S., Gäbel, C., Aguilar-Raab, C., Hillecke, T. K., and Warth, M. (2018). Affective and autonomic response to dynamic rhythmic entrainment: mechanisms of a specific music therapy factor. Arts Psychother. 60, 48–54. doi: 10.1016/j.aip.2018.06.002
Kim, Y. (2004). The early beginnings of Nordoff-Robbins music therapy. J. Music Ther. 41, 321–339. doi: 10.1093/jmt/41.4.321
Kimmatkar, N. V., and Babu, B. V. (2021). Novel approach for emotion detection and stabilizing mental state by using machine learning techniques. Computers 10, 37. doi: 10.3390/computers10030037
Kjetil, J. (2021). Music technology tools – a therapist-in-a-box?” Voices World Forum Music Ther. 21. doi: 10.15845/voices.v21i2.3308
Kleć, M., and Wieczorkowska, A. (2021). “Music and healthcare recommendation systems,” in Recommender Systems for Medicine and Music, eds Z. W. Ras, A. Wieczorkowska, and S. Tsumoto (Cham: Springer International Publishing), 187–195.
Knight, W. E. J., and Rickard, N. S. (2001). Relaxing music prevents stress-induced increases in subjective anxiety, systolic blood pressure, and heart rate in healthy males and females. J. Music Ther. 38, 254–272. doi: 10.1093/jmt/38.4.254
Koelstra, S., Muhl, C., Soleymani, M., Lee, J.-S., Yazdani, A., Ebrahimi, T., et al. (2012). DEAP: a database for emotion analysis; using physiological signals. IEEE Trans. Affect. Comput. 3, 18–31. doi: 10.1109/T-AFFC.2011.15
Kramer, C. (2000). “Music as cause and cure of illness in nineteenth-century Europe,” in Music as Medicine The History of Music Therapy Since Antiquity, 1st Edn, ed P. Horden (London: Routledge), 338–352.
Kramer, O. (2013). “K-nearest neighbors,” in Dimensionality Reduction with Unsupervised Nearest Neighbors (Berlin; Heidelberg: Springer), 13–23. doi: 10.1007/978-3-642-38652-7_2
Krasnoff, E. (2021). Effects of auditory binaural beats on consciousness and the human nervous system: A transdisciplinary review of the evidence. Brain Stimulat. J. 14, 1702. doi: 10.1016/j.brs.2021.10.365
Labaste, F., Ferré, F., Combelles, H., Rey, V., Foissac, J.-C., Senechal, A., et al. (2019). Validation of a visual analogue scale for the evaluation of the postoperative anxiety: a prospective observational study. Nursing Open 6, 1323–1330. doi: 10.1002/nop2.330
Labbé, E., Schmidt, N., Babin, J., and Pharr, M. (2007). Coping with stress: the effectiveness of different types of music. Appl. Psychophysiol. Biofeedback 32, 163–168. doi: 10.1007/s10484-007-9043-9
Lampert, R. (2015). ECG signatures of psychological stress. J. Electrocardiol. 48, 1000–1005. doi: 10.1016/j.jelectrocard.2015.08.005
Lane, J., Kasian, S., Owens, J., and Marsh, G. (1998). Binaural auditory beats affect vigilance performance and mood. Physiol. Behav. 63, 249–252. doi: 10.1016/S0031-9384(97)00436-8
Lavedán Santamaría, A., Masot, O., Velez, O. C., Botigué, T., Sánchez, T. C., and Roca, J. (2022). Diagnostic concordance between the visual analogue anxiety scale (VAS-A) and the state-trait anxiety inventory (STAI) in nursing students during the COVID-19 pandemic. Int. J. Environ. Res. Public Health 19, 7053. doi: 10.3390/ijerph19127053
Le Scouarnec, R. P., Poirier, R. M., Owens, J. E., Gauthier, J., Taylor, A. G., and Foresman, P. A. (2001). Use of binaural beat tapes for treatment of anxiety: a pilot study of tape preference and outcomes. Altern. Ther. Health Med. 7, 58–63.
Leonard, H. (2019). Live music therapy during rehabilitation after total knee arthroplasty: a randomized controlled trial. J. Music Ther. 56, 61–89. doi: 10.1093/jmt/thy022
Leslie, G., Ghandeharioun, A., Zhou, D., and Picard, R. W. (2019). “Engineering music to slow breathing and invite relaxed physiology,” in Institute of Electrical and Electronics Engineers, 2019 8th International Conference on Affective Computing and Intelligent Interaction (ACII), 1–7.
Li, J., Zhang, Z., and He, H. (2018). Hierarchical convolutional neural networks for EEG-based emotion recognition. Cognit. Comput. 10, 368–380. doi: 10.1007/s12559-017-9533-x
Linnemann, A., Ditzen, B., Strahler, J., Doerr, J. M., and Nater, U. M. (2015). Music listening as a means of stress reduction in daily life. Psychoneuroendocrinology 60, 82–90. doi: 10.1016/j.psyneuen.2015.06.008
Linnemann, A., Wenzel, M., Grammes, J., Kubiak, T., and Nater, U. M. (2018). Music listening and stress in daily life—a matter of timing. Int. J. Behav. Med. 25, 223–230. doi: 10.1007/s12529-017-9697-5
McGroarty, B. (2020). Wellness Music. Global Wellness Summit. Available online at: https://www.globalwellnesssummit.com/2020-global-wellness-trends/wellness-music/ (accessed January 8, 2020).
Mokhayeri, F., and Akbarzadeh, T. M. (2011). “Mental stress detection based on soft computing techniques,” in 2011 IEEE International Conference on Bioinformatics and Biomedicine, 430–433.
Monsalve-Duarte, S., Betancourt-Zapata, W., Suarez-Cañon, N., Maya, R., Salgado-Vasco, A., Prieto-Garces, S., et al. (2022). Music therapy and music medicine interventions with adult burn patients: a systematic review and meta-analysis. Burns 48, 510–521. doi: 10.1016/j.burns.2021.11.002
Neurohealth (2013). The Science of Brainwaves - The Language of the Brain. Neurohealth Associates. Biology. Neurohealth. Available online at: https://nhahealth.com/brainwaves-the-language/ (accessed May 29, 2013).
Nick, T. G., and Campbell, K. M. (2007). “Logistic regression,” in Topics in Biostatistics, ed W. T. Ambrosius (Totowa, NJ: Humana Press), 273–301.
Nolan, P. (1991). “Group improvisation therapy for a resistant woman with bipolar disorder – manic,” in Case Studies in Music Therapy, ed K. E. Bruscia (Phoenixville, PA: Barcelona Pub), 451–464.
Nygaard Pedersen, I. (2019). “Classical psychoanalysis,” in A Comprehensive Guide to Music Therapy, 2nd Edn, eds I. Nygaard-Pedersen, S. L. Jacobsen, and L. O. Bonde (London: Jessica Kingsley Publishers), 76–92.
Ole Bonde, L. (2019). “Definitions of music therapy,” in A Comprehensive Guide to Music Therapy, 2nd Edn, eds I. Nygaard-Pedersen, S. L. Jacobsen, and L. O. Bonde (London: Jessica Kingsley Publishers), 29–35.
Pedersen, I. N., and Bonde, L. (2019). “Music therapy and personal growth,” in A Comprehensive Guide to Music Therapy, 2nd Edn, eds I. Nygaard-Pedersen, S. L. Jacobsen, and L. O. Bonde (London: Jessica Kingsley Publishers), 374–390.
Rahman, J. S., Gedeon, T., Caldwell, S., Jones, R., and Jin, Z. (2021). Towards effective music therapy for mental health care using machine learning tools: human affective reasoning and music genres. J. Artif. Intellig. Soft Comput. Res. 11, 5–20. doi: 10.2478/jaiscr-2021-0001
Reimnitz, L., and Silverman, M. J. (2020). A randomized pilot study of music therapy in the form of patient-preferred live music on fatigue, energy and pain in hospitalized adult oncology patients on a blood and marrow transplant unit. Arts Health 12, 154–168. doi: 10.1080/17533015.2018.1534251
Russell, J. (1980). A circumplex model of affect. J. Pers. Soc. Psychol. 39, 1161–1178. doi: 10.1037/h0077714
Sanei, S., and Chambers, J. A. (2007). “Introduction to EEG,” in EEG Signal Processing (Cardiff: John Wiley and sons), 1–34.
Sege, C. T., Bradley, M. M., and Lang, P. J. (2020). Motivated action: pupil diameter during active coping. Biol. Psychol. 153, 107885. doi: 10.1016/j.biopsycho.2020.107885
Shaffer, F., and Ginsberg, J. P. (2017). An overview of heart rate variability metrics and norms. Front. Public Health 5, 258. doi: 10.3389/fpubh.2017.00258
Shiloah, A. (2000). “Jewish and muslim traditions of music therapy,” in Music as Medicine The History of Music Therapy Since Antiquity, 1st Edn, ed P. Horden (London: Routledge), 69–83.
Sieciński, S., Kostka, P. S., and Tkacz, E. J. (2020). Heart rate variability analysis on electrocardiograms, seismocardiograms and gyrocardiograms on healthy volunteers. Sensors 20, 4522. doi: 10.3390/s20164522
Silverman, M. J., Letwin, L., and Nuehring, L. (2016). Patient preferred live music with adult medical patients: a systematic review to determine implications for clinical practice and future research. Arts Psychother. 49, 1–7. doi: 10.1016/j.aip.2016.05.004
Sourina, O., Liu, Y., and Nguyen, M. K. (2012). Real-time EEG-based emotion recognition for music therapy. J. Multimodal User Interfaces 5, 27–35. doi: 10.1007/s12193-011-0080-6
Spielberger, C., Gorsuch, R., Lushene, R., Vagg, P. R., and Jacobs, G. (1983). Manual for the State-Trait Anxiety Inventory (Form Y1 – Y2), Vol. IV. Palo Alto, CA: Consulting Psychologists Press.
Spielberger, C. D., and Sarason, I. G. (1991). Stress and Anxiety, Vol. 13. New York, NY: CRC Press.
Stancin, I., Cifrek, M., and Jovic, A. (2021). A review of EEG signal features and their application in driver drowsiness detection systems. Sensors 21, 3786. doi: 10.3390/s21113786
Stein, M. B., and Sareen, J. (2015). Generalized anxiety disorder. New Engl. J. Med. 373, 2059–2068. doi: 10.1056/NEJMcp1502514
Stensæth, K., and Magee, W. L. (2016). The Future of Technology in Music Therapy: Towards Collaborative Models of Practice. Available online at: http://hdl.handle.net/20.500.12613/6289 (accessed September 23, 2022).
Suthaharan, S. (2016). “Support vector machine,” in Machine Learning Models and Algorithms for Big Data Classification: Thinking with Examples for Effective Learning (Boston, MA: Springer US), 207–235. doi: 10.1007/978-1-4899-7641-3_9
Tatar, K., Prpa, M., and Pasquier, P. (2019). Respire: Virtual reality art with musical agent guided by respiratory interaction. Leonardo Music J. 29, 19-24. doi: 10.1162/lmj_a_01057
Thoma, M. V., Marca, R. L., Brönnimann, R., Finkel, L., Ehlert, U., and Nater, U. M. (2013). The effect of music on the human stress response. PLoS ONE 8, e70156. doi: 10.1371/journal.pone.0070156
Tonacci, A., Dellabate, A., Dieni, A., Bachi, L., Sansone, F., Conte, R., et al. (2020). Can machine learning predict stress reduction based on wearable sensors' data following relaxation at workplace? A pilot study. Processes 8, 448. doi: 10.3390/pr8040448
Tyler, H. M. (2000). “The music therapy profession in Modern Britain,” in Music as Medicine The History of Music Therapy Since Antiquity, 1st Edn, ed P. Horden (London: Routledge), 375–395.
Wang, K., Chao, G., Yanjie, D., Yilun, L., Xinhu, Z., and Fei-Yue, W. (2017). “Generative Adversarial Networks: Introduction and Outlook.” IEEE/CAA Journal of Automatica Sinica. 4 588–98. doi: 10.1109/JAS.2017.7510583
West, M. (2000). “Music therapy in antiquity,” in Music as Medicine The History of Music Therapy Since Antiquity, 1st Edn, ed P. Horden (London: Routledge), 51–68.
Wheeler, B., Lesiuk, T., Burns, D., Hanser, S., Rossetti, A., and Cassity, M. (2019). Music therapy and music medicine studies in oncology: part I: a comparison. Music Med. 11, 145. doi: 10.47513/mmd.v11i3.671
Widjaja, D., Orini, M., Vlemincx, E., and Huffel, S. V. (2013). Cardiorespiratory dynamic response to mental stress: a multivariate time-frequency analysis. Comput. Math. Methods Med. 2013, 451857. doi: 10.1155/2013/451857
Wittchen, H.-U., and Hoyer, J. (2001). Generalized anxiety disorder: nature and course. J. Clin. Psychiatry 62, 15–19.
Wu, W., Mallet, Y., Walczak, B., Penninckx, W., Massart, D. L., Heuerding, S., et al. (1996). Comparison of regularized discriminant analysis linear discriminant analysis and quadratic discriminant analysis applied to NIR data. Analytica Chimica Acta. 329, 257–265. doi: 10.1016/0003-2670(96)00142-0
Yannakakis, G. N., Cowie, R., and Busso, C. (2021). The ordinal nature of emotions: an emerging approach. IEEE Trans. Affect. Comput. 12, 16–35. doi: 10.1109/TAFFC.2018.2879512
Yu, B., Funk, M., Hu, J., and Feijs, L. (2018). Unwind: A musical biofeedback for relaxation assistance. Behav. Infm. Technol. 37, 800–814.
Zhai, J., and Barreto, A. (2006). “Stress Recognition Using Non-Invasive Technology,” in FLAIRS Conference, eds G. Sutcliffe and R. Goebel (AAAI Press), 395–401.
Zhang, K., Zhang, H., Li, S., Yang, C., and Sun, L. (2018). “The PMEmo dataset for music emotion recognition,” in Proceedings of the 2018 ACM on International Conference on Multimedia Retrieval. ICMR '18 (New York, NY: Association for Computing Machinery), 135–142.
Keywords: therapeutic music systems, music therapy, biofeedback, machine learning, ECG, EEG, binaural beats
Citation: Ferguson A, Castellanos C and Pasquier P (2023) Digital music interventions for stress with bio-sensing: a survey. Front. Comput. Sci. 5:1165355. doi: 10.3389/fcomp.2023.1165355
Received: 14 February 2023; Accepted: 16 May 2023;
Published: 26 June 2023.
Edited by:
Yuxuan Zhou, Nanjing Medical University, ChinaReviewed by:
Gennaro Tartarisco, National Research Council (CNR), ItalyHiroki Tanaka, Nara Institute of Science and Technology (NAIST), Japan
Copyright © 2023 Ferguson, Castellanos and Pasquier. This is an open-access article distributed under the terms of the Creative Commons Attribution License (CC BY). The use, distribution or reproduction in other forums is permitted, provided the original author(s) and the copyright owner(s) are credited and that the original publication in this journal is cited, in accordance with accepted academic practice. No use, distribution or reproduction is permitted which does not comply with these terms.
*Correspondence: Arron Ferguson, YXJyb25fZmVyZ3Vzb24mI3gwMDA0MDtiY2l0LmNh; Philippe Pasquier, cGFzcXVpZXImI3gwMDA0MDtzZnUuY2E=