- 1School of Computer Science and Engineering, International University, Ho Chi Minh City, Vietnam
- 2Vietnam National University, Ho Chi Minh City, Vietnam
- 3Sussex Ingestive Behaviour Group, School of Psychology, University of Sussex, Brighton, United Kingdom
- 4Ultraleap, Bristol, United Kingdom
- 5Department of Computer Science, University College London, London, United Kingdom
Novel gustatory interfaces offer the potential to use the sense of taste as a feedback modality during the interaction. They are being explored in a wide range of implementations, from chemical to electrical and thermal stimulation of taste. However, the fundamental aspect of gustatory interaction that has yet to be explored is the Sense of Agency (SoA). It is the subjective experience of voluntary control over actions in the external world. This work investigates the SoA in gustatory systems using the intentional binding paradigm to quantify how different taste outcome modalities influence users' SoA. We first investigate such gustatory systems using the intentional binding paradigm to quantify how different tastes influence users' SoA (Experiment 1). The gustatory stimuli were sweet (sucrose 75.31 mg/ml), bitter (caffeine powder 0.97 mg/ml), and neutral (mineral water) as the outcomes of specific keyboard presses. We then investigated how SoA was altered depending on users' sweet liking phenotype, given that sweet is one of the taste outcomes (Experiment 2), and in contrast with audio as a traditional outcome. In Experiment 2, stronger taste concentrations (sweet-sucrose 342.30 g/L, bitter-quinine 0.1 g/L, and neutral) were used, with only participants being moderate sweet likers. We further contrasted tastes with audio as the traditional outcome. Our findings show that all three taste outcomes exhibit similar intentional binding compared to auditory in medium sweet likers. We also show that longer action-outcome duration improved the SoA. We finally discuss our findings and identify design opportunities considering SoA for gustatory interfaces and multisensory interaction.
1. Introduction
Our ability to taste begins with the human tongue, which has an average of 5,000 and 10,000 taste buds (Schacter et al., 2011). When a taste compound (i.e., sucrose, citric acid, or MSG) touches our tongue, those taste buds are activated and send signals to the central nervous system, which interprets those signals as tastes. Generally, experts in taste perception agree on five basic tastes (Trivedi, 2012): sweet, sour, salty, bitter, and umami, with discussions about new taste qualities [e.g., starch (Lapis et al., 2016), fat (Liu et al., 2011), and spicy (Simon and de Araujo, 2005)] are emerging. Of those five basic tastes, sour is used to detect acid elements to gauge fruit palatability. In contrast, the bitter taste is a potential indicator of poisonous food (Hupfeld and Rodden, 2012). The salty taste serves the essential function of sensing ions, required minerals, and water homeostasis, which is necessary for bodily functions (Purves et al., 2008). Sweet and umami promote the consumption of nutritive food (sweet provides an extra energy source, and umami detects essential amino acids needed for protein synthesis). All those basic tastes can be perceived anywhere on the tongue. However, the human tongue is more sensitive around its edges (the tip and two sides), with the back mainly sensitive to bitterness to avoid swallowing poisonous substances (Informedhealth.org., 2006).
In HCI, taste defines a final frontier in interaction design because it is the only sense that requires active acceptance of stimulation by the user. While vision, hearing, touch, and smell can all be stimulated externally, taste can only be stimulated by activating the taste receptors located on the human tongue (Zhao et al., 2003). Motivating users to put things in their mouths, as we were accustomed to doing as babies, is challenging unless there is a real value added to the user's experience. Therefore, the potential value of taste experiences in HCI remains largely unexplored. Nonetheless, progress has been made toward designing gustatory interfaces and comprehending taste experiences for the purpose of designing interactive systems (Obrist et al., 2014a).
Gustatory interface design and understanding user's tasting experience is an emerging field in HCI, especially in the context of augmenting gaming and virtual reality experiences, food interaction design, enabling the creation of new eating experiences (Cornelio et al., 2022a). Recently, we have seen various investigations linked to the study of food in everyday life (Comber et al., 2012; Deng et al., 2021), investigating the ecologies of domestic food consumption (Hupfeld and Rodden, 2012), product and package design (Velasco et al., 2016), but also with respect to the exploration of novel interaction concepts [e.g., shape-changing food (Wang et al., 2017), edible screen/interface (Gayler, 2017)]. Recently, Wilberz et al. (2020) developed an automatic food dispenser interface, a robot arm attached to a head-mounted display, providing localized, multi-directional and movable haptic cues to the user's face. Another taste delivery system is TastyFloats (Vi et al., 2017b) which uses acoustic levitation to deliver food morsels to the users' tongues. This work was extended to synchronized integration of levitated food with visual, olfactory, auditory, and tactile stimuli (Vi et al., 2020), enabling systematic investigations of multisensory signals around levitated food and eating experiences.
These introductions of gustatory interfaces show the potential of how the sense of taste can be used as an input modality in the field of HCI, comparison or addition to the traditional input modalities such as already well-used visual or auditory senses and the increasingly investigated sense of touch and smell. However, a question that remained unanswered with the sense of taste is if it can let users have a similar, or even better, feeling of control in interacting with the system, in comparison to the traditional senses (e.g., using audio as the system feedback). This work aims to answer this question through the sense of agency (SoA).
SoA refers to the subjective experience of voluntary control over actions in the external world. It is a critical aspect in human computer interaction as it allows users to say: “I am, who is doing this” (Coyle et al., 2012). A system without or with a low SoA will discourage users from operating it, preventing the widespread use of the system. An example of this is in Limerick et al. (2015), where they showed that a low SoA in voice command systems contributed to the low uptake of speech interfaces for interactive applications despite the availability of high-accuracy voice recognition techniques (e.g., 97.3% recognition rate). In another example, Coyle et al. (2012) showed that on-body interaction can elicit a greater sense of agency than keyboard interaction, consequently creating a wide opportunity for on-body interaction systems. We need a similar understanding of the sense of taste for gustatory interfaces to enable wider uptake of such systems.
It is generally agreed that humans have five basic tastes: sweet, bitter, sour, salty, and umami [with other potential new tastes of starch (Sclafani, 2004), fat (Besnard et al., 2015), metallic (Lawless et al., 2005)]. Previous works have focused on the characteristics of these basic tastes, such as their neural correlates and pathways (Matsumoto, 2013), taste perception characteristics (Crisinel and Spence, 2010), as well as their perceived spatial and temporal properties (Obrist et al., 2014a). Sweet and bitter tastes are the two most salient sensory percepts for humans. Due to their perceived pleasantness, sweet and bitter are often referred to as the taste of reward (sweet) and punishment (bitter) (Vi et al., 2018).
Recent works have shown a link between taste (i.e., sweet or bitter) and human cognitive functions, emotions, or moral judgments. For example, people often use taste words (i.e., in English, Chinese, and Vietnamese) to describe their emotions (sweet is positive, and bitter/sour is negative) (Zhou and Tse, 2020). In another example, physical disgust (induced via a bitter taste) elicited feelings of moral disgust (Eskine et al., 2011). Meier et al. (2012) showed that individual differences in the preference for sweet foods predicted personalities, intentions, and behaviors. Hellmann et al. (2013) showed that priming participants with a sweet taste (versus bitter taste) in their mouths led to more lenient judgement toward people who take revenge on others. Such examples emphasize the importance of a user's taste preference in taste-based and multisensory interactions.
Earlier research has demonstrated the connection between cognitive factors and the judgment of agency. For example, David et al. (2008) presented a comprehensive understanding of the sense of agency (SoA) in relation to other cognitive processes and underlying mechanisms. Osumi et al. (2019) more recently explored the time window of agency judgment and delay detection in an agency attribution task. Their results suggest that cognitive factors besides multisensory information comparison are involved in the judgment of agency. Additionally, research has shown that the emotional valence of an action outcome affects explicit and implicit SoA. Positive action outcomes are linked to an increased sense of agency in most studies (Barlas and Obhi, 2014; Gentsch et al., 2015; Hoemann et al., 2019), which has been interpreted as part of a self-serving bias in human cognition (Gentsch and Synofzik, 2014; Haggard, 2017). Conversely, having control over one's actions or feeling a degree of choice can result in a more positive or less negative affect.
As there is a clear link between taste and human emotion, it can be hypothesized that these individual differences in liking sweet tastes may impact different cognitive processes related to tastes, such as SoA.
Sweet phenotypes: Previous work has shown that people can be classified into different phenotypes depending on their individual difference in responses to basic tastes. For example, people with different sensitivity to bitters can be categorized into super-tasters (high sensitivity), medium tasters (medium sensitivity), and non-tasters (low sensitivity). Similarly, depending on their liking for sweet taste, a user can belong to one of three sweet-liker phenotypes (Iatridi et al., 2019; Armitage et al., 2021):
• Extreme sweet likers (liking increases with sweetness)—approximately 25%−30% of the population.
• Moderate sweet likers (like moderate but not intense sweetness)—approximately 50% of the population.
• Sweet dislikers (liking decreases with sweetness)—approximately 20%−25% of the population.
We conducted two user studies to understand users' SoA when interacting with gustatory interfaces. Our studies use the intentional binding paradigm, which provides an implicit and quantitative measure of the SoA. In the first experiment, we aim to quantify how different tastes (sweet: sucrose 75.31 mg/ml, bitter: caffeine powder 0.97 mg/ml, and water) influence users' SoA. In the second experiment, we contrasted SoA between audio- and taste-feedback of strong concentrations (sweet-sucrose 342.3 mg/ml, bitter-quinine 0.1 mg/ml, and mineral water) in moderate sweet likers, the largest group of the three sweet-liker phenotypes in the population.
The contributions of this manuscript are:
• We investigate agency effects for taste-based interaction for the first time.
• We demonstrate the importance of the user's taste profile in applications with taste outcomes.
• We discuss our findings and identify design opportunities considering agency in taste-based and multisensory interaction.
2. Related works
2.1. Taste as an interaction modality
Although our everyday activities involve five basic senses, our interaction with technology has mainly focused on auditory, vision, and haptic as the primary interaction modalities. However, recent research has demonstrated an uptake toward multisensory experiences in HCI with more opportunities for smell (Obrist et al., 2014b, 2017) and taste (Altarriba Bertran et al., 2019; Khot and Mueller, 2019) in user interfaces. Among those two, taste is often referred to as a final frontier in interaction design as it is the only sense that requires the user to accept the stimulation actively. Vision, hearing, touch, and smell can all be stimulated outside the human body, but taste requires the stimulation of the human tongue, where all taste receptors are located (Chandrashekar et al., 2006). Therefore, motivating users to put things in their mouths, as we were used to as babies, is not easy unless a real added value to the users' experience is provided.
Consequently, the value of taste experiences for human-computer interaction is still an under-explored field, yet initial steps toward understanding taste experiences for designing interactive systems have been made (Obrist et al., 2014a). There has been a growing interest in exploiting the sense of taste for novel gustatory systems (Maynes-Aminzade, 2005; Narumi et al., 2010; Murer et al., 2013; Khot et al., 2017; Vi et al., 2017a,b, 2018, 2020; Arnold et al., 2018). Khot et al. (2017), for instance, designed EdiPulse, which presents a 3D-printed chocolate message mapped to the user's heart rate. BeanCouter (Maynes-Aminzade, 2005) delivers jelly beans to users with different (e.g., cherry, strawberry, and lemon), with potential memory profiling and network monitoring applications. Meta Cookies (Narumi et al., 2010) delivers a simulated cookie taste in VR based on the olfactory scent. LOLLio (Murer et al., 2013) is a taste-based game controller that uses taste qualities as a human decision-making system (e.g., sweet as a reward and sour as punishment). Notably, Vi et al. (2017b) presented TastyFloats, which uses ultrasonic waves to levitate food morsels (small pieces of solid food) or droplets (liquid drops) in mid-air and deliver them to the user's tongue. The authors continued to develop LeviSense, the first integrated platform to investigate multisensory experiences with levitating food (Vi et al., 2020). The system supports a synchronized integration of levitated food with visual, olfactory, auditory, and tactile stimuli to create an immersive tasting experience.
The above examples show an increasing development of gustatory interfaces and their cover in many HCI areas such as VR, gaming, communication, and human-food interaction. However, an important question is the role of taste, as the output of a gustatory interface, on the sense of being in control (or SoA) remains unexplored.
2.2. The sense of agency
The SoA refers to the experience of being the source of one's own voluntary actions (Roessler and Eilan, 2003), influencing the world around us (Beck et al., 2017). Georgieff and Jeannerod (1998) defined this phenomenon as a “who” system that helps us identify the agent of an action and thus differentiates the self from external agents. The SoA also reflects the experience that links intentions to their external outcomes. When a person intends to create a specific outcome, the body moves under the control of the brain's voluntary motor system, and the anticipated outcome is created in the surrounding environment (Chambon et al., 2014). The match between an action's intended and actual result produces a feeling of controlling the environment (Synofzik et al., 2013). The brain mechanisms that produce this experience are efficient and familiar, so our SoA is experienced naturally and like a continuous mental background during everyday motor movements. To experience an SoA, three conditions need to occur, (1) one intends to produce an outcome through one's own action, (2) one voluntarily commands the corresponding body movement, and (3) the intended outcome occurs (see Figure 1A). This action-effect causality is particularly crucial in our interactions with technology.
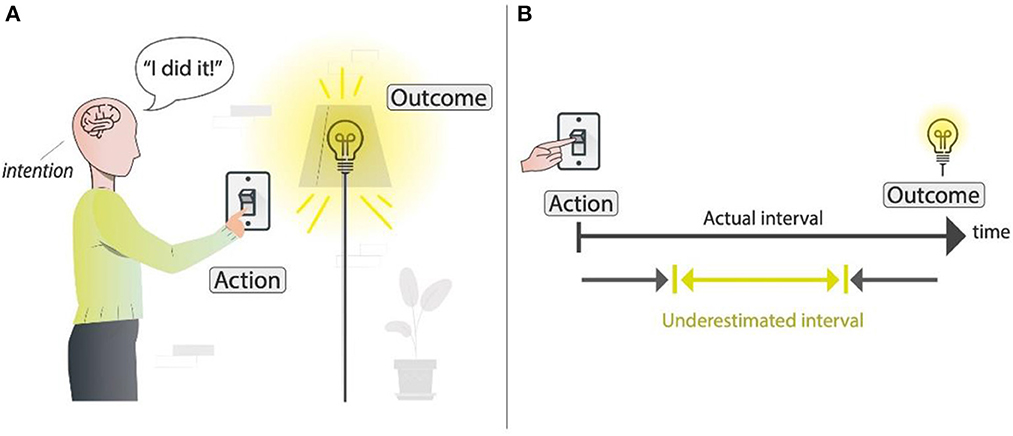
Figure 1. (A) Elements that compose the SoA—the feeling of “I did it,” An intention to produce an outcome followed by the body moving to perform the action that produces such an outcome; (B) The intentional binding effect. People underestimate the time interval between a voluntary action and its resulting outcome.
2.3. The sense of agency in HCI
The SoA is not only a crucial part of our experiences in daily life but also in our interaction with technologies that figure in Human-Computer Interaction (HCI). That is, the SoA gives the user the feeling of “I did that” as opposed to “the system did that,” thus supporting a feeling of being in control. HCI is defined as a stimulus-response interplay between humans and technology (Farooq and Grudin, 2016). In this interplay, actions are represented by user input commands, and outcomes are represented by system feedback. Input modalities thus translate the user's intentions into state changes within the system, while system feedback informs the user about the system's current state. Here, it is crucial that the SoA is not disrupted to support a feeling of being in control. For instance, when we manipulate a user interface (e.g., on a computer or smartphone), we expect the system to respond to our input commands as we want to feel we are in charge of the interaction. If this stimulus-response interplay elicits an SoA, the user will feel “I am controlling this.” A clear example of the SoA being disrupted is when there is a mismatch between what the system is expected to do and the actual sensory feedback from the system. This disruption causes a sudden interruption in the feeling of control, which can negatively affect acceptability (Berberian, 2019) and usability (Winkler et al., 2020). In another example, Haselager (2013) argues that adding intelligent devices to Brain-Computer Interfacing may make users feel insecure about whether the resulting behavior is genuinely their own, consequently affecting the user's SoA. Recently, Zanatto et al. (2023) examined the What-Whether-When model of intentional action and its effect on SoA. Their results show that when participants were told what action to perform and whether to act decreased SoA, whereas leaving them free to decide what action to take and whether to perform it did not compromise SoA. If a system does not support an SoA, the user might feel discouraged from using it (Limerick et al., 2015) and lose self-attribution to their actions' outcomes. For this reason, SoA is gaining increasing attention in the field of HCI, and researchers and practitioners are using agency measures as a way to evaluate the user's feeling of control in different interaction techniques. One particularly relevant measure is the intentional binding paradigm.
2.4. Intentional binding paradigm and interval estimation task
There are two ways to measure the SoA: explicit or implicit. Explicit judgments of the SoA are obtained by simply asking subjects whether they were the agent of a certain action or not. Implicitly measures such as the intentional binding paradigm (Haggard et al., 2002) depend on a relationship between agency experience and time perception (illustrated in Figure 1B). In this paradigm, the level of agency can be assessed as perceived differences in time between voluntary actions and their resultant outcomes. Subjects are asked to report their time perception of events using either the Libet clock method (Libet et al., 1983) or the interval estimation method (Ebert and Wegner, 2010). SoA is conceptualized as a strong link between the action and its outcome across time. It is therefore measured as compression in the perceived time interval between action and outcome. Both implicit and explicit measures of SoA have been adopted in HCI aiming to evaluate the degree of control in novel interactions techniques, such as gestural interaction (Cornelio et al., 2017), on-skin input (Bergstrom-Lehtovirta et al., 2018) and automated actions (Kasahara et al., 2019). These studies suggest agency measures as promising evaluations of the feeling of control in HCI. Indeed, it has been suggested that on-skin input produces a higher SoA than traditional button-press (Coyle et al., 2012) and touchpad (Bergstrom-Lehtovirta et al., 2018) inputs.
Interval Estimation Task: To evaluate intentional binding, we measured subjects' time perception using the interval estimation method (Ebert and Wegner, 2010). Since this method does not allow distinguishing between action and outcome binding, we obtained a single measure from subjects' reports about their perceived time interval between action and outcome in milliseconds.
In the interval estimation method, participants were asked to press a button from a keyboard. Pressing the button produced a type of outcome (a beep or a taste in their mouth) after a time delay randomly varied (see Figure 2). Then, participants were asked to estimate the interval (in milliseconds) between pressing the button (action) and the outcome. Crucially, during a training stage where participants were allowed to practice their interval estimation with feedback of the actual intervals, they were told that there were 19 possible delays between action and outcome ranging from 50 to 900 ms in intervals of 50 ms (e.g., 50 ms, 100 ms, 150 ms, 200 ms, etc.). However, during the actual experiment, we presented only three fixed intervals (100 ms, 400 ms, and 700 ms) in a counterbalanced order as in Ebert and Wegner (2010) and Coyle et al. (2012). The errors are calculated by the difference between actual and reported times in milliseconds. The mean error is thus associated with Intentional Binding.
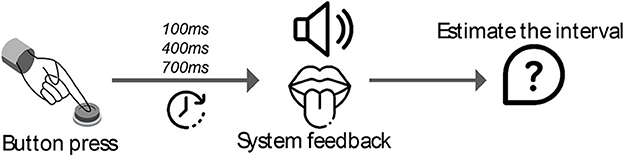
Figure 2. Goal-directed task as an example of measuring SoA using the Interval Estimation Task. Subjects moved a mouse cursor from a start area to click a target (that varies in distance and size), producing a beep after three fixed intervals (100 ms, 400 ms, and 700 ms).
3. Building a taste delivery device: TasteBud
To deliver taste to the participant's mouth, we built a gustatory interface based on the TasteBud design (Vi et al., 2018). The device is designed as an interactive and flexible interaction in gameplay using taste stimuli. The device has six peristaltic pumps controlling six bottles of aqueous stimuli (i.e., five basic tastes and one water for control; see Figure 3C). Each taste is pumped from the taste solution bottle through a Teflon tube to the participant's mouth. Multiple tastes can be delivered independently to the participant's mouth using a 3D-printed mouthpiece (see Figure 3B) mounted on a stand in front of the participant (see Figure 3D). The flow rate of each peristatic pump can be customized by controlling the pump head's rotation per minute (RPM), allowing control of the amount of taste delivered to the user's mouth. Each pump inside the TasteBuds device allows three levels of control with the command format as in Figure 3A:
• Push/pull direction of delivery.
• Flow rate (ml/s).
• Delivery duration (in s).
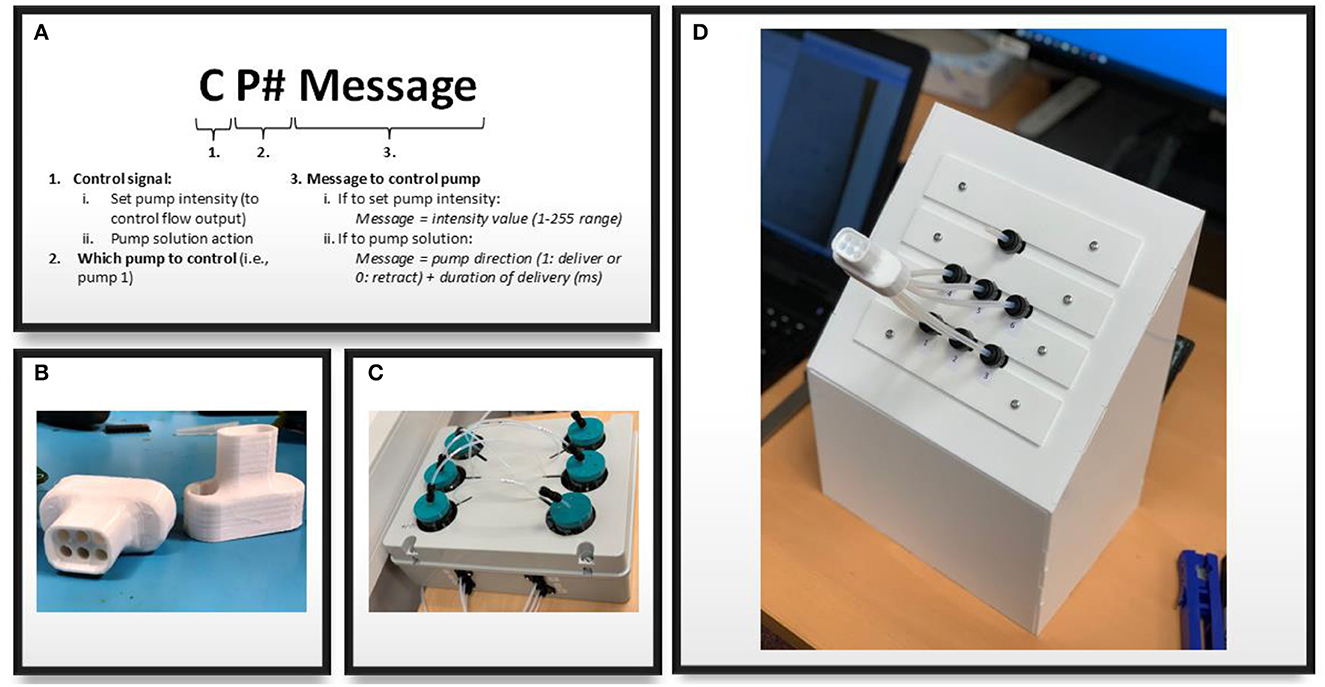
Figure 3. (A) Command message format of TasteBud; (B) Two 3D-printed mouthpieces, each can mount six taste outcomes; (C) a TasteBud device with six bottles of solutions; and (D) An interface stand mounting a taste outcome mouthpiece.
We performed three technical tests to ensure the device was competent for this study, measuring the SoA with high timing precision.
• Test 1: Measure flow outcome based on the applied voltage to a peristaltic pump.
• Test 2: Measure the differences in flow outcome between six pumps.
• Test 3: Measure the duration between the moment of command is executed on the PC and the moment a taste outcome is delivered to the user's tongue.
3.1. TEST 1: Measure flow outcome based on the applied voltage to a peristaltic pump
We tested the flow outcome of a pump depending on the applied voltage to determine the linear relationship between them. We used eight different voltage levels for the pump and measured the flow outcome for 1 s with a high-precision scale (Precisa® 1600c Precision Balance, ±0.001 g accuracy). We first measured the weight of 1 ml test solution (0.97 g), which was then used as a reference to calculate the volume of the test outcome. Eight voltage levels were used (8–15 V with 1 V increment), and each level was applied and measured 10 times. Figure 4 illustrates the volume and consistency of the pump's outcome for 1 s with different applied voltages.
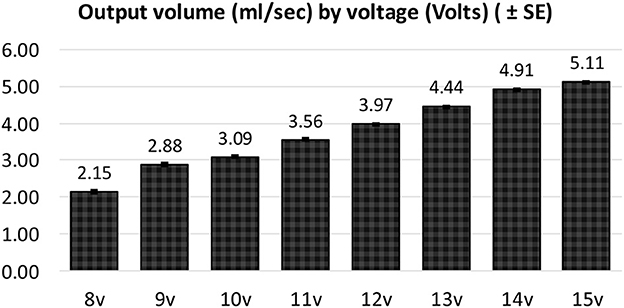
Figure 4. Pump's outcome volume (in milliliters) by applied voltage (in Volt; bars represent standard deviation SE).
3.2. TEST 2: Measure the differences in flow outcome between six pumps
With the relationship between the pump's outcome volume and the applied voltage established, we tested the difference in outcome volume between the six pumps of the TastyFloats device. Each pump had 14 V input, turned on for a 1-s duration, and had its outcome volume measured. This process was repeated 10 times for each pump. Figure 5A illustrates the average outcome volume of each pump. Repeated measures ANOVA found significant differences between the pumps' outcomes (p < 0.001). Post-hoc tests with Bonferroni correction found no significant difference between pumps (1 vs. 2), (3 vs. 5), (3 vs. 6), (4 vs. 5), and (5 vs. 6) and significant differences in other pairs.
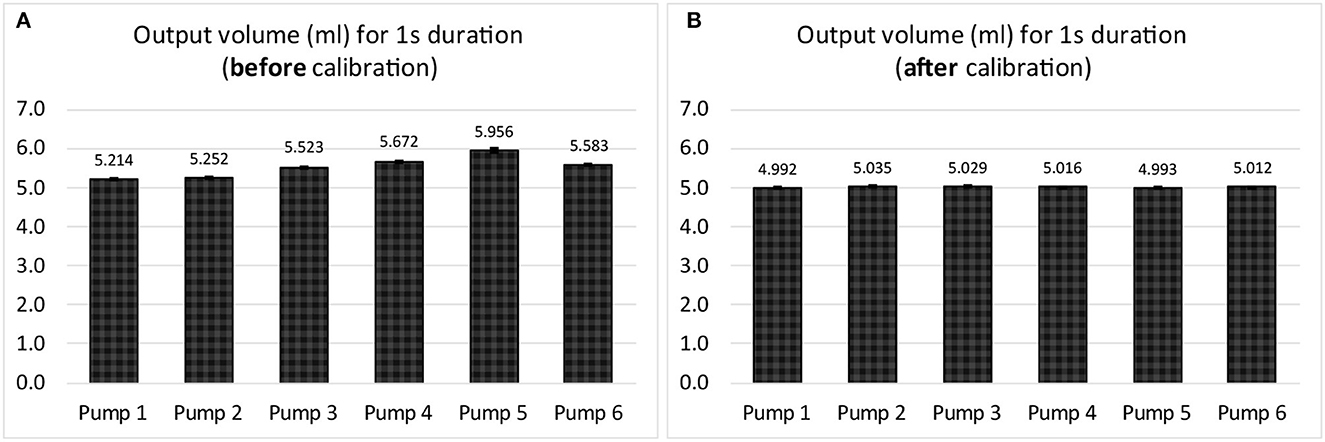
Figure 5. Pump's outcome volume (ml) for 1-second duration before- (A) and after-calibration (B) (vertical bars represent standard error of mean ± SE).
Based on these measurements, we calibrated each pump to have the same outcome of 5 ml by adjusting their intensity using the appropriate message format (as in Figure 3A). Repeated measures ANOVA was performed on the pumps' outcome volume for 1 s after this calibration and found no significant difference between the pumps (p = 0.529). Figure 5 details the average outcome volume of each pump before- and after- the calibration was applied.
3.3. TEST 3: Measure the delay between the moment a command is sent to the TasteBud device and the moment a taste outcome is delivered to the user's tongue
Imagine a simple scenario where a user clicks a button on the screen to activate a pump (i.e., push the liquid out of pump 1—moment ONE) to deliver the taste solution to the user's mouth (moment TWO). We hypothesize that there is a delay of the device defined as the difference between the two moments:
To measure this delay, we set up a simple task where a user clicked on a button (mouse up event) to send a string message to the serial port to release the aqueous outcome out of the connected tube. The tube was primed with the aqueous outcome up to the release point. When the button on the screen was clicked with a mouse, its background changed (i.e., alternating between GREEN and RED). A high-speed camera (Sony RX10 II, set at 1,000 frames per second) was placed at a place that can capture both the button and the tube outcome in its frame to record the delay between the two moments, as in Device DELAY (milliseconds) = moment TWO – moment ONE. Each pump was measured 10 times.
These values (Figure 6) must be considered to calculate the precise delivery moment of tastes on the tongue after a command is executed on the control software (i.e., execute the command 12.67 ms in advance for pump 1). In our experiments, the used pumps were all calibrated to have the same outcome of 5 ml/s and have the device delays applied.
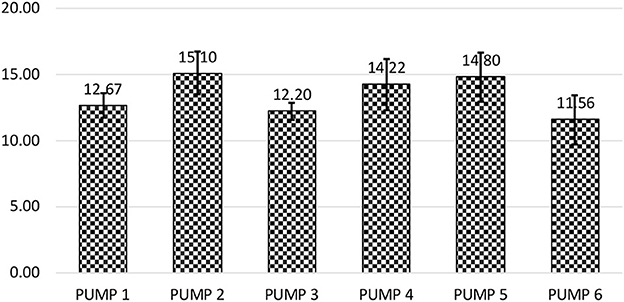
Figure 6. Device delay (in milliseconds) for each pump between the message sent moment and taste delivered moment. Vertical bars represent the standard error of the mean.
4. Experiment 1
4.1. Study design
This experiment investigates if the two most salient taste percepts (sweet and bitter) elicit SoA, measured using the interval estimation task (Haggard et al., 2002). We conducted a within-subject experiment comparing SoA in three taste feedback: Sweet (sucrose 220 mM or 75.3 g/L, table sugar), Bitter (caffeine powder 5 mM or 0.97 g/L, obtained from Sigma-Aldrich), and water (control condition). The concentration of the used taste stimuli is based on previous works using basic tastes in HCI (Vi et al., 2017b, 2018; Vi and Obrist, 2018) as well as other fields (Schoenfeld et al., 2004; Green et al., 2015; Hoogeveen et al., 2015). Participants performed three blocks of the interval estimation task, each with one taste outcome (sweet or bitter), in a counterbalanced order using a Latin square to avoid any order bias (Wakeling and MacFie, 1995).
4.2. Participants
Fifty-three participants (all females, 18–28 years old, mean age = 20.36, SD = 1.65), who were students at the local institution, volunteered to take part in this study. As the experiment used tastes as system feedback, participants were recruited based on the following criteria: not having any food allergies, not diabetic, not being pregnant, being a non-smoker, not on a weight loss diet or having an eating disorder and prescription medication free (not including contraception). In addition, participants were instructed when they signed up for the study to not eat or drink (apart from water) at least 2 h before attending the study to avoid any bias of strong flavors on the taste perception (Obrist et al., 2014a). The experiment lasted about 45 min and was approved by the local ethics committee. Participants were compensated with three credits for undergraduate students.
4.3. Procedure
Upon arrival, participants read the information sheet and signed the consent form before participating. Next, they were seated at a desk in front of an LCD monitor (set at 1,920 × 1,200 resolution and a 60-Hz refresh rate), which was connected to a Dell Precision T1600 computer with a 3.3 Hz Intel Xeon processor. Between participants and the screen was a TasteBud stand with a mouthpiece mounted conveniently for participants to hold in their mouths (see Figure 7). Participants then completed a disguised mood and appetite questionnaire to record their hunger level and a Three-Factor Eating Questionnaire (for a different study). Participants were then instructed to use a pair of noise-blocking headphones (3M PELTOR Optime III Earmuffs) to block surrounding noises and noises from the TasteBud's pumps.
In each trial of the main task, participants were instructed to place the index finger of their dominant hand on the keyboard spacebar (labeled as the ACTION key). Next, the index finger of their non-dominant hand was placed on one of the two control keys (labeled as the NEXT key to proceed between experimental instructions/ messages). After this, they were told to hold the mouthpiece connected to the taste device (see Figure 7) in their mouth and have their lips tightly sealed around the mouthpiece's tip. Doing this would make sure the taste outcome is delivered to the participant's mouth and onto their tongue. Participants were also asked to put on soundproof headphones to block any surrounding noise (i.e., made by the device when a taste is being delivered). Participants were then told that in each trial, they would make an action (pressing the keyboard spacebar) randomly at any point after a countdown of 5 s. Once the action had been made, a taste would be delivered onto their tongue after a short delay. There were 19 possible delays between action (keypress) and outcome (taste on the tongue), ranging from 50 to 950 ms. Finally, participants were asked to use a computer mouse to select their estimated delay of the current trial (as illustrated in Figure 8).
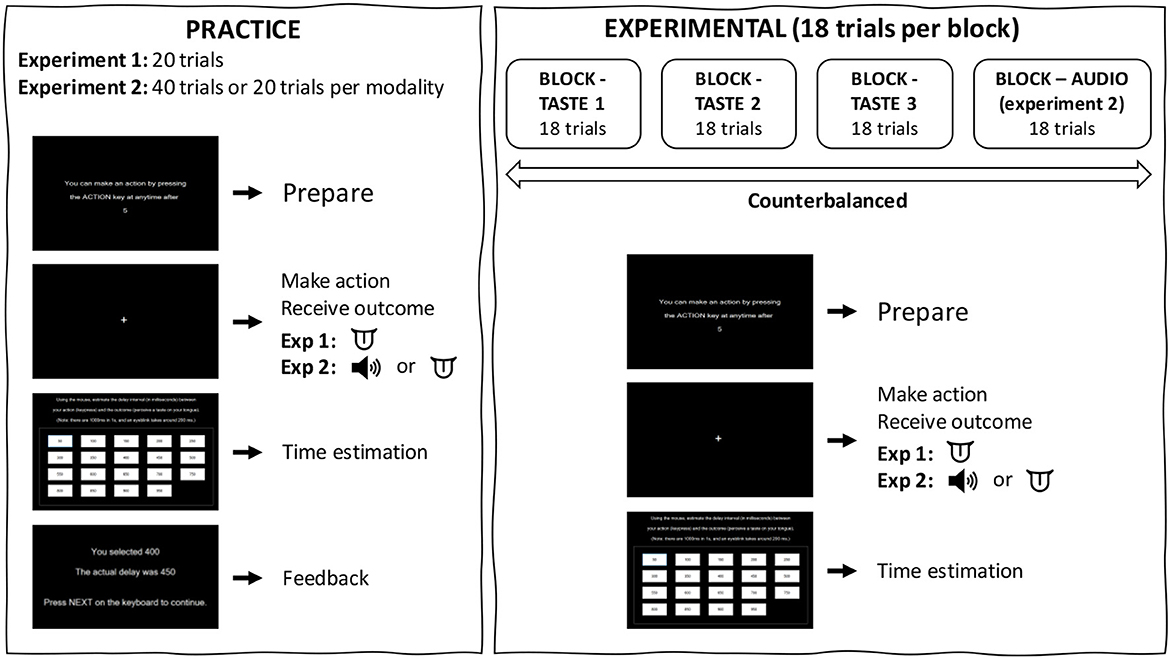
Figure 8. Experiment design: each participant completed one practice block (20 trials) of taste (neutral) and (experiment 2 only) audio outcome where they were informed of the actual delay between action (spacebar key press) and outcome (taste delivered to the mouth). They then completed three blocks of 18 trials each of taste outcomes (experiment 1) or four blocks of three taste and audio outcomes (experiment 2). Order of the outcomes was counterbalanced between participants.
Participants started with a practice block of 20 trials, and the taste outcome delivered to the participant's mouth was water. The actual delays were displayed on the screen after a selection was made. Participants then performed three blocks (18 trials each) of sweet, bitter, and neutral. Here, participants did not receive the correct estimation on the screen after making their estimation. Participants were also provided with a spit cup and asked to rinse with water and spit after each time they received a taste in their mouth. During the three blocks of taste outcomes, there were only three fixed intervals of delay (100 ms, 400 ms, and 700 ms counterbalanced between trials). Participants, however, were not made aware of this and were still presented with all 19 possible delays (see Figure 8). The experiment was coded using Microsoft Visual C# and a high-precision. NET Multimedia Timer for accurate timing (±0.1 ms on the experiment machine).
In each trial, a taste outcome (sweet, bitter, and neutral) was delivered for 200 ms using three different channels (channels 1, 2, and 3 of the TasteBud device) on the participant's tongue. All channels were calibrated to have the same outcome of 5 ml/s and had the delivery delay accounted for. We measured the amount of taste delivered in each trial per channel to ensure that an equal amount of liquid was delivered across the taste outcomes. Each channel was measured 10 times; the results are presented in Figure 9A. Repeated measures ANOVA found no significant difference between pumps [F(2, 18) = 0.24, p = 0.79]. In total, each participant ingested an average of 20.06 ml of the sweet solution (1,506.12 mg sucrose), 20.22 ml of the bitter solution (19.42 mg caffeine), and 20.02 ml mineral water.
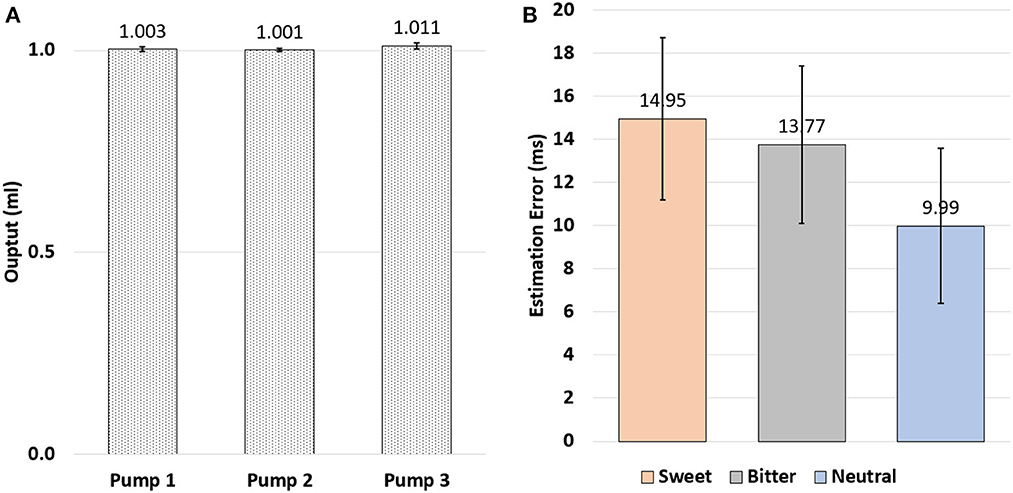
Figure 9. (A) Amount of taste (in ml) delivered to participant's mouth per trial (200 ms duration). (B) The mean interval estimation errors in milliseconds for each of the three taste outcomes. Vertical bars represent the standard error of means SE.
4.4. Results
4.4.1. Correlations between estimated and actual intervals
To determine an adequate number of participants for this experiment design, we performed a priori statistical power analysis for sample size estimation in G*Power (Faul et al., 2007). Using a repeated measures ANOVA with three taste conditions, 18 repetitions per condition, a power of 0.95, an alpha level of 0.05, and a large effect size (f = 0.25) (Faul et al., 2007; Lakens, 2013), the required sample size is ~15 participants. Thus, our number of 53 participants was adequate for the primary goal of this study. Partial eta squared (η2) is reported as a measure of effect size, according to (Wassertheil and Cohen, 1970), with a value of 0.01 as a small effect, 0.06 as a medium effect, and 0.14 or greater as a large effect size.
Of the 53 participants, two were removed from the analysis. In one case, the participant chose to withdraw, and the other case was removed due to human error. Of the remaining 51 participants, Pearson correlation test shows a significant positive correlation between the actual- and estimated-intervals (r = 0.487; p < 0.001). This result demonstrates that participants exhibited a high degree of accuracy in estimating intervals on the practice trials. For four participants, these correlations were negative, suggesting unusual difficulty with the interval estimation task, and those individuals were excluded from subsequent analyses.
4.4.2. Binding and authorship
The interval estimation error of each trial was calculated by subtracting the actual interval from the participant's estimated interval for that trial. Figure 9B and Table 1 show the mean estimation errors for each taste outcome across 846 trials (47 participants × 3 tastes × 18 trials per taste). Lower estimation errors indicate a higher SoA, and negative estimation errors indicate intentional binding, hence an SoA. Repeated measures ANOVA was performed on the estimation errors of participants across the three taste outcomes (sweet, bitter, and neutral), following Coyle et al.'s work (Coyle et al., 2012). Mauchly's Test of Sphericity indicated that the assumption of sphericity had not been violated [ = 3.73, p = 0.16]. With the sphericity assumed, we found no significant difference between the taste outcomes F(2, 844) = 0.19, p = 0.83.
Looking into more detail of each delay, a Tastes x Delays repeated measures ANOVA was conducted on participants' mean interval estimates, as in Ebert and Wegner (2010). Mauchly's Test of Sphericity indicated that the assumption of sphericity had not been violated for Tastes [ = 0.63, p = 0.73]. However, this test showed that the assumption of sphericity had been violated for Delays [ = 34.31, p < 0.001] and Tastes * Delays interaction [ = 50.59, p < 0.001] and therefore, Greenhouse-Geisser correction was used. There was no significant effect within the Tastes group [F(2, 562) = 0.152, p = 0.86]. However, confirming that participants' estimates of delays were generally accurate, the main effect of the delay was highly significant [F(2, 503.89) = 771.46, p < 0.001], with longer estimates (M = 229.14 ms) at 100 ms, intermediate estimates (M = 391.84 ms) at 400 ms, and shorter estimates (M = 617.73 ms) at 700 ms (see Figure 10). Post-hoc tests with Bonferroni correction found significant differences between any two of the three delays (p < 0.001). The Tastes * Delays interaction was not significant [F(3.67, 1031) = 2.36, p = 0.057].
4.5. Discussion
The results show that none of the three taste outcomes produced intentional binding, hence an SoA. This result was the average across all three delays (100, 400, and 700 ms). However, we also found that only the most prolonged delay (700 ms) enhanced intentional binding (see Figure 10). Previous studies have reported contradictory findings about this relationship. For example, it has been shown that longer action–effect delays have been associated with reduced SoA (Blakemore et al., 1999; Elijah et al., 2016), whereas a recent study from Evangelou et al. (2021) showed that haptic feedback increased the implicit sense of agency for the most extended action-outcome interval length. Our findings are consistent with the latter argument, perhaps because our study is the first to use taste as an outcome measure, which is considered the most intrusive of the five senses.
This study examined SoA with three different taste outcomes. However, this experiment did not consider users' sweet liking phenotype, which could impact SoA for sweetness. Users can be characterized into three phenotypes: extreme sweet likers (liking increases with sweetness), moderate sweet likers (like moderate but not intense sweetness), and sweet dislikers (liking decreases with sweetness) (Iatridi et al., 2019). Therefore, we designed another experiment, contrasting SoA between audio (1,000 Hz) and taste outcomes (sweet, bitter, and neutral) in only moderate sweet likers.
5. Experiment 2
5.1. Study design
Experiment 2 had three alterations compared to Experiment 1:
1. Users were pre-screened for their sweet-liking status, following the procedure of Iatridi et al. (2019). Those who consented to be contacted for further studies had their sweet liking status stored in a database. Only medium-sweet likers (~50% of the population) were invited to participate in Experiment 2.
2. We used higher sweet and bitter concentrations compared to Experiment 1:
• Sweet: Sucrose 342.30 g/L (the same concentration as in the sweet screening test).
• Bitter: Quinine 0.1 g/L (equivalent in intensity to the concentration of Sweet). We decided to use quinine over caffeine powder since caffeine is a psychostimulant. Increasing the concentration raises the potential for caffeine to be absorbed, and results then are confounded by potential pharmacological effects. In contrast, quinine is a purely bitter substance without any potential psychopharmacological confound.
3. We added the Audio outcome (a 1,000 Hz tone) as a control condition to compare with the three taste outcomes.
5.2. Participants
Twenty-one participants (15 females, six males, 19–32 years old, mean age = 22.00, SD = 3.52), who were students at the local institution, volunteered to participate in this study. Similar to Experiment 1, participants were recruited based on the following criteria: not having any food allergies, not being diabetic, not being pregnant, being a non-smoker, not on a weight loss diet, or having an eating disorder and prescription medication free (not including contraception). In addition, participants were instructed not to eat or drink (apart from water) for at least 2 h before attending the study to avoid any bias of strong flavors on the taste perception (Obrist et al., 2014a). The experiment lasted about 1 hour and was approved by the local ethics committee. Participants were compensated with either GBP 10 or 4 credits for undergraduate students.
5.3. Procedure
The experiment was set up similarly to Experiment 1. However, for the added Audio condition, the audio outcome was 1,000 Hz frequency, 80 dB volume, and played on a speaker (XT1 audio system, Altec Lansing Technologies—see Figure 7) for the same brief duration as a taste outcome (200 ms).
Participants started with two practice blocks (20 trials each) in a counterbalanced order between participants: one with audio and one with taste (neutral) outcome, where the actual delays were displayed to them on screen after a selection was made. Participants then performed four blocks (18 trials each) of audio, sweet, bitter, and neutral, in a counterbalanced order between participants. At the beginning of each of the three taste-outcome blocks, participants had 10 ml of the taste outcome delivered to their mouth. They were instructed to swirl the taste around their mouth for at least 10 s before swallowing it. They then provided their ratings of liking (on a 100-point horizontal Visual Analog Scale—VAS), intensity [on a vertical general Labeled Magnitude Scale—gLMS (Green et al., 1996)], and four basic taste qualities (sweet, bitter, sour, salty—in random order-−100-point VAS). They then proceeded to the task as in Experiment 1. Participants received instructions on the screen to put on the noise-blocking headphones if that block had a taste outcome (including the practice block). They were instructed to do otherwise in the block of the audio outcome.
5.4. Results
5.4.1. Taste stimuli ratings
To determine an adequate number of participants for this experiment design, we performed a priori statistical power analysis for sample size estimation in G*Power (Faul et al., 2007). Using a repeated measures ANOVA with four conditions (one audio and three tastes), 18 repetitions per condition, a power of 0.95, an alpha level of 0.05, and a large effect size (f = 0.25) (Faul et al., 2007; Lakens, 2013), the required sample size is 16 participants. Thus, our number of 21 participants was adequate for the main goal of this study. Partial eta squared (η2) is reported as a measure of effect size, according to Wassertheil and Cohen (1970), with a value of 0.01 as a small effect, 0.06 a medium effect, and 0.14 or greater as a large effect size.
We first examine the collected ratings of Liking, Intensity, Sweetness, and Bitterness of the taste stimuli used. Figure 11 illustrates the mean values of these ratings in each category. Next, we performed a MANOVA with the rating types as the dependent variable and taste outcome as the independent variable.
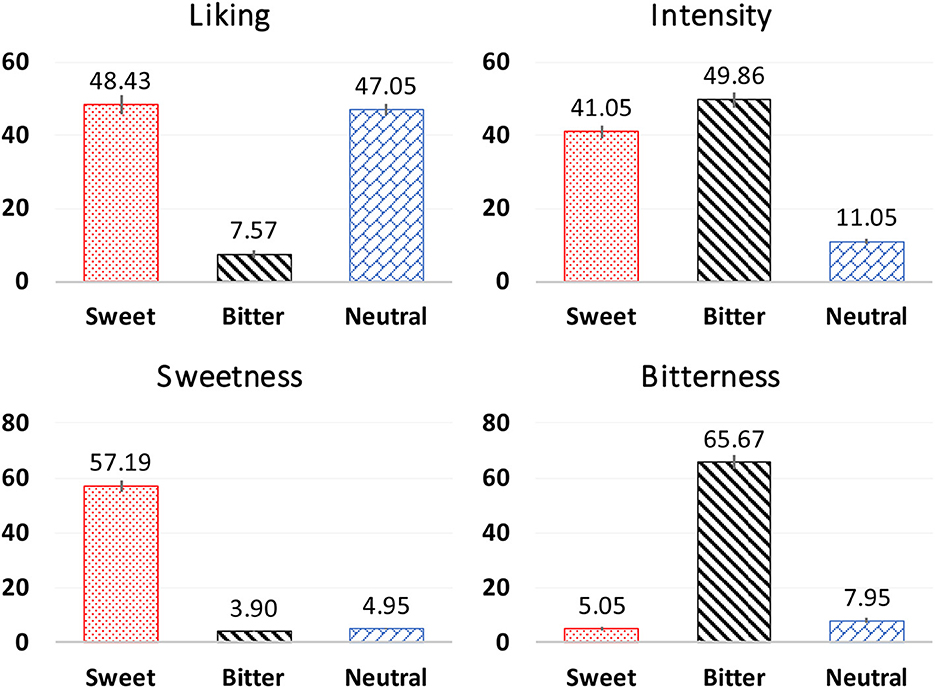
Figure 11. Mean ratings of the three taste outcomes of Liking, Intensity, Sweetness, and Bitterness. Bars represent standard errors of the mean.
Liking: We found a significant effect in Liking [F(2, 60) = 262.58, p < 0.001]. Post-hoc comparisons with Bonferroni corrections found a significant difference between (Bitter vs. Sweet) and (Bitter vs. Neutral; p < 0.001) but not (Neutral vs. Sweet; p = 1.0). This result confirms the innate nature of the tastes where sweet is liked by the participants, and bitter is an aversive taste.
Intensity: We also found a significant effect in Intensity [F(2, 60) = 40.67, p < 0.001]. Post-hoc comparisons with Bonferroni corrections found a significant difference between (Neutral vs. Sweet) and (Neutral vs. Bitter; p < 0.001) but not (Sweet vs. Bitter; p = 0.18), confirming that the two solutions were well matched in intensity. Additionally, we compared the ratings of sweetness for Sweet and bitterness for Bitter. Paired t-test found no significant difference between them [t(20) = −1.5, p = 0.15]. These results confirm that the used Sweet and Bitter stimuli were equally intense tastes.
5.4.2. Binding
Pearson correlation test shows that all participants exhibited a high degree of accuracy in estimating intervals on the practice trials (r = 0.62, p < 0.001). Figure 12 and Table 2 show the mean estimation errors for each taste outcome. Lower estimation errors indicate a higher SoA, and negative estimation errors indicate intentional binding, hence an SoA. Notably, all outcomes yielded intentional binding, indicated by the average negative estimation errors. Repeated measures ANOVA was performed on the estimation errors of participants across the four outcomes (sweet, bitter, neutral, and audio), following Coyle et al.'s work (Coyle et al., 2012). Mauchly's Test of Sphericity indicated that the assumption of sphericity had been violated [ = 40.41, p < 001], so Greenhouse-Geisser correction was used. We found no significant difference between the taste outcomes F(2.79, 1047.18) = 0.09, p = 0.97. Additionally, paired samples T-Test found no significant difference between males (M = 377.89 ms) and females [M = 356.13 ms; t(431) = 1.75, p = 0.08].
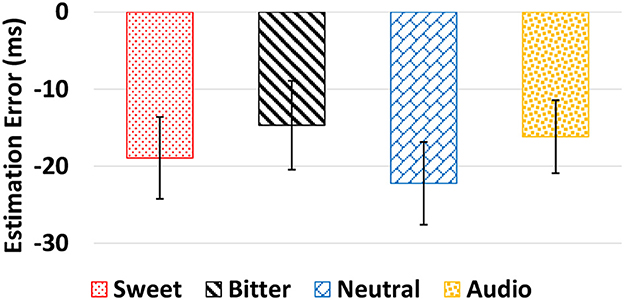
Figure 12. The mean interval estimation errors in milliseconds for each of the four outcomes. Lower estimation errors indicate a greater SoA. Vertical bars represent the standard error of the means.
Additionally, an Outcome x Delay repeated measures ANOVA was conducted on participants' mean interval estimates. Mauchly's Test of Sphericity indicated that the assumption of sphericity had been violated for delay [ = 29.26, p < 0.001] and Outcome * Delay interaction [ = 39.74, p < 0.01]; therefore Greenhouse-Geisser correction was used. The sphericity assumption was violated for Outcome [ = 10.74, p = 0.057]. The main effect of Outcome was not significant [F(3, 372) = 0.078, p = 0.97]. Confirming that participants' estimates of delays were generally accurate, the main effect of Delay was highly significant [F(1.65, 204.67) = 418.28, p < 0.001], with longer estimates at 100 ms (M = 214.40 ms, SE = 8.76), but shorter at 400 ms (M = 368.70 ms, SE = 9.20) and 700 ms (M = 566.80 ms, SE = 11.06). The Outcome * Delay interaction was also significant [F(5.46, 677.29) = 3.31, p < 0.005]. Repeated measures ANOVA was carried out at each of the three delays to examine this interaction (illustrated in Figure 13). The effect of Outcome was significant at 100 ms [F(2.82, 349.17) = 3.74, p < 0.05], with significant differences in pairs of (Audio vs Sweet, p < 0.05) and (Audio vs. Bitter, p < 0.05) but not in other pairs. However, we did not find a significant effect at 400 and 700 ms delays.
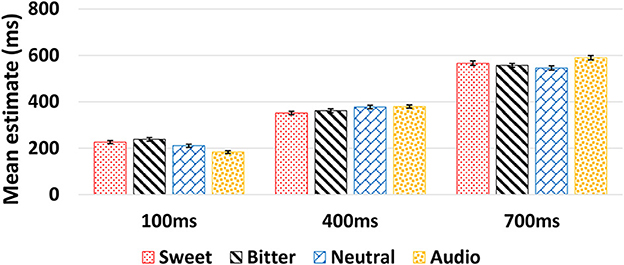
Figure 13. Mean interval estimates at each of the three delays. Error bars show the standard error of means across participants.
5.5. Discussion
Our results show that all outcomes (taste or audio) had no significant difference in yielding SoA. This result demonstrates that a gustatory interface gives users the feeling of being in control (or SoA) as much as in a system with a traditional auditory outcome.
Notably, this experiment's result differs from Experiment 1, where all three taste outcomes did not yield SoA. A possible reason is a difference in users' taste profiles. Experiment 2 had only participants who were moderate-sweet likers (like moderate but not intense sweetness). Evidence for this is in the result of the neutral outcome (mineral water). With the same setup and procedure of having mineral water as the system outcome, participants in Experiment 1 did not feel the SoA (Mean estimation error = 9.99 ms). In contrast, they did in Experiment 2 (M = −22.22 ms).
It can be argued that the higher taste concentration contributes to the change in elicit SoA. In Experiment 1, we used the same taste concentration as in Vi and Obrist (2018). In that work, sweet was rated slightly pleasant (M = 1.43 ± 0.62), and bitter was rated slightly unpleasant (M = −1.29 ± 0.59) on a 7-point Likert scale from –3: Extremely unpleasant to +3: Extremely pleasant. However, sweet and bitter intensity in Experiment 2 was rated between “Strong” and “Very strong” on the General Linear Magnitude Scale (Green et al., 1993) (as shown in Figure 11).
Similar to Experiment 1, our results show that the difference between mean estimates and actual delays is biggest at 700 ms and intermediate at 400 ms, suggesting that the long delays of taste outcome in a gustatory interface may have a greater intentional binding effect.
6. General discussion
Human–computer interaction is defined as a stimulus–response interplay between humans and technology (Farooq and Grudin, 2016). Actions are represented by user input commands, and outcomes are represented by system feedback. This action-effect causality is essential in our interactions with the technologies (Cornelio et al., 2022b). In other words, letting users feel in control of their interaction with the system is crucial in designing interactive systems and multimodal interfaces (Shneiderman, 1997). A system that gives users the feeling of “that outcome is because of my action” rather than “the system caused that outcome” can result in the uptake of such system's usage as it offers a more agentive and responsive experience. Previous studies have studied agency modulation through novel input modalities [e.g., speech (Limerick et al., 2015), gestural and mid-air haptics (Cornelio et al., 2017), on-skin interaction (Bergstrom-Lehtovirta et al., 2018), and smell (Cornelio et al., 2020)]. In this work, we have introduced taste as an interaction modality and its relationship with SoA.
Our key finding of this work is the existence of intentional binding in a taste-outcome interactive system. Furthermore, Experiment 2 demonstrated that a taste-outcome exhibited as much as intentional binding when the system outcome was auditory. Similar research in smell, another chemical sense, as an output modality demonstrates that positive emotions (i.e., pleasantness) can influence SoA (Cornelio et al., 2020).
Contrasting the two experiments, we show that HCI designers must consider the user's taste profile and characteristics of the used tastes to offer the sense of being in control of the interaction. We envision a scenario where an interactive task with a taste outcome can include a “calibration step” to ensure personalized taste experiences, akin to adjusting the brightness of a color (screen) or speaker's volume. This can be as simple as answering a rating question of a sweet solution's liking before users begin using the system. Future gustatory interfaces will become part of the digital interactive space (Cornelio et al., 2022b) and are envisioned as a part of the 6th generation of mobile communication technology (6G) (Banafaa et al., 2022).
It can be argued that the exhibited intentional bindings might be contributed partially or mainly by the haptic sensation on users' tongues, evidenced by the no significant difference between exhibited intentional binding between the three taste outcomes. It is also supported by previous works, such as Cornelio et al. (2017), showing that haptic feedback (vibrotactile or mid-air haptics) on the user's hand exhibited significantly higher intentional binding than a traditional visual outcome. However, we did not observe this in Experiment 1, which delivered the same amount of liquid for the same duration on the user's tongue. Here, the difference between the two studies, which include or exclude the two extreme groups of sweet likers and dislikers, can be a possible explanation. To further investigate this, a follow-up study can investigate the two aspects of a taste outcome concerning SoA: the perceived taste and the proprioceptive perception of an aqueous stimulus on the tongue separately and contrast between sweet liking phenotypes. Another possible direction is to manipulate the texture of delivered aqueous stimuli (i.e., thickness) to create a perception of different food types in the mouth.
6.1. Application scenarios
It has been argued that a system in a digital world where users can see, hear, smell, touch, and taste just like they do in the real world can provide sensory signals to users that facilitate the agency delegation between humans and systems (Cornelio et al., 2021). Although in such a digital environment, users' actions are usually assisted or influenced (i.e., task assistance or automation) by the system, multisensory signals can help the users to feel agency during the interaction with technology, even though they are not the agent of the action (Banakou and Slater, 2014). Here, our findings open up opportunities for HCI designers to improve users' SoA through multisensory signals, combined with other modalities that have been proven their links to SoA, such as touch (Bergstrom-Lehtovirta et al., 2018), mid-air haptic (Cornelio et al., 2017), and smell (Cornelio et al., 2020). For example, our results could be used to design a better gaming task, such as the taste-based Minesweeper (Vi et al., 2018) or CandyCrush, which offers users a fun gaming experience and a sense of responsiveness and being in control.
In another example, we can imagine that taste and smell stimuli are used in VR environments to create virtual tasting experiences that not only improve the immersiveness of the environment but also improve the user's feeling of control in the virtual space. In such a digital environment, different tastes can be used creatively [i.e., sweet for reward, bitter for punishment, sour for risk-taking (Vi and Obrist, 2018)] to manipulate SoA, creating novel multisensory experiences. This can be combined with manipulating the shape, size, and color of the taste stimuli. For example, a recent study has shown that a Bouba-shaped (rounded) taste sample can increase a user's perceived sweetness compared to a Kiki-shaped (spiky) one (Cornelio et al., 2022a). There are many more types of interactive tasks that we hope our results will inspire, consequently increasing the inclusion of taste as a novel interaction modality.
6.2. Limitations and future works
The findings of this study are constrained by the use of taste stimuli. As the TasteBud device was used to the delivered taste, the results are for stimuli in liquid form, with the abovementioned concentrations and chemical compounds (i.e., sucrose, quinine, caffeine powder). Future works can expand beyond this using a more advanced platform, such as LeviSense (Vi et al., 2020), which can deliver both liquid and solid foods and stimulate taste in a pre-defined pattern.
In the present work, we employed the intentional binding paradigm and the interval estimation task as an implicit measure of the SoA. However, previous studies have suggested that implicit and explicit agency processing systems may be separatable (Moore et al., 2012). Therefore, further research is needed to investigate the relationship between the explicit judgment of agency and intentional binding for gustatory interfaces.
Similarly, we collected quantitative data from two populations in two experiments: Experiment 1 had females as participants only, whereas experiment had males and females. Previous works have shown that genders may affect SoA using different methods (Jones et al., 2008; Hurault et al., 2020). However, it is notable that there is still a debate about the differences between methods to measure SoA. Furthermore, our work investigates, for the first time, the SoA of a system with taste as an outcome, which may not be the same as the traditional output. In addition, our Experiment 2 results suggest that there was no significant difference between genders.
7. Conclusion
In this work, we demonstrated for the first that taste, as a system outcome, elicits the user's Sense of Agency (SoA). We also illustrated the importance of considering the user's taste profile in designing interactive applications. SoA was not found when such taste profile screening was not considered. However, taste outcomes yield as much SoA as the traditional output (i.e., audio) in medium sweet likers, the largest group of users (~50% of the population), considering their hedonic liking toward sweet taste. Our findings contribute to the growing research around designing gustatory interfaces. We also provide valuable insights to designers when designing gustatory interfaces that support a sense of being in control. One can imagine how taste in a future meta-verse era could be relevant, especially in light of the Sense of Agency.
Data availability statement
The raw data supporting the conclusions of this article will be made available by the authors, without undue reservation.
Ethics statement
The studies involving human participants were reviewed and approved by Sciences & Technology Cross-Schools Research Ethics Committee (C-REC), University of Sussex. The patients/participants provided their written informed consent to participate in this study.
Author contributions
CV: methodology, coding, conducting the experiment, analyzing data pipeline, writing—original draft preparation, and review and editing. PC: methodology, interpretation, and writing—review and editing. MO and MY: supervision, interpretation, review, and editing. All authors contributed to the article and approved the submitted version.
Funding
This work has received funding from the Leverhulme Trust (Research Project Grant RPG-2018-068) and the ERC (SenseX—Starting Grant Agreement 638605).
Acknowledgments
We thank Mr. Rob Cobden for his technical help. We thank Miss Rhiannon Armitage, Miss Lydia Henke, Miss Kallina Perrou, and Miss Katie Karnif for their help in screening the participants and assisting the running of experiments. Finally, we thank all participants that participated in our studies.
Conflict of interest
PC was employed by Ultraleap.
The remaining authors declare that the research was conducted in the absence of any commercial or financial relationships that could be construed as a potential conflict of interest.
Publisher's note
All claims expressed in this article are solely those of the authors and do not necessarily represent those of their affiliated organizations, or those of the publisher, the editors and the reviewers. Any product that may be evaluated in this article, or claim that may be made by its manufacturer, is not guaranteed or endorsed by the publisher.
References
Altarriba Bertran, F., Jhaveri, S., Lutz, R., Isbister, K., and Wilde, D. (2019). “Making sense of human-food interaction,” in Conference on Human Factors in Computing Systems - Proceedings (Glasgow). doi: 10.1145/3290605.3300908
Armitage, R. M., Iatridi, V., and Yeomans, M. R. (2021). Understanding sweet-liking phenotypes and their implications for obesity: narrative review and future directions. Physiol. Behav. 235, 113398. doi: 10.1016/j.physbeh.2021.113398
Arnold, P., Khot, R. A., and Mueller, F. F. (2018). ““you better eat to survive”: exploring cooperative eating in virtual reality games,” in Proceedings of the Twelfth International Conference on Tangible, Embedded, and Embodied Interaction (Stockholm, Sweden: Association for Computing Machinery). doi: 10.1145/3173225.3173238
Banafaa, M., Shayea, I., Din, J., Hadri Azmi, M., Alashbi, A., et al. (2022). 6G mobile communication technology: requirements, targets, applications, challenges, advantages, and opportunities. Alex. Eng. J. 64, 245–274. doi: 10.1016/j.aej.2022.08.017
Banakou, D., and Slater, M. (2014). Body ownership causes illusory self-attribution of speaking and influences subsequent real speaking. Proc. Natl. Acad. Sci. USA. 111, 17678–83. doi: 10.1073/pnas.1414936111
Barlas, Z., and Obhi, S. S. (2014). Cultural background influences implicit but not explicit sense of agency for the production of musical tones. Conscious. Cogn. 28, 94–103. doi: 10.1016/j.concog.2014.06.013
Beck, B., Di Costa, S., and Haggard, P. (2017). Having control over the external world INCREASES the implicit sense of agency. Cognition 162, 54–60. doi: 10.1016/j.cognition.2017.02.002
Berberian, B. (2019). Man-machine teaming: a problem of agency. IFAC-Pap. 51, 118–123. doi: 10.1016/j.ifacol.2019.01.049
Bergstrom-Lehtovirta, J., Coyle, D., Knibbe, J., and Hornbæk, K. (2018). “I really did that: Sense of Agency with touchpad, keyboard, and on-skin interaction,” in Proceedings of the 2018 CHI Conference on Human Factors in Computing Systems (Montreal, QC: Association for Computing Machinery), 1−8. doi: 10.1145/3173574.3173952
Besnard, P., Passilly-Degrace, P., and Khan, N. A. (2015). Taste of fat: a sixth taste modality? Physiol. Rev. 96, 151–176. doi: 10.1152/physrev.00002.2015
Blakemore, S. J., Frith, C. D., and Wolpert, D. M. (1999). Spatio-temporal prediction modulates the perception of self-produced stimuli. J. Cogn. Neurosci. 11, 551–9. doi: 10.1162/089892999563607
Chambon, V., Sidarus, N., and Haggard, P. (2014). From action intentions to action effects: how does the sense of agency come about? Front. Hum. Neurosci. 8, 320. doi: 10.3389/fnhum.2014.00320
Chandrashekar, J., Hoon, M. A., Ryba, N. J. P., and Zuker, C. S. (2006). The receptors and cells for mammalian taste. Nature 444, 288–294. doi: 10.1038/nature05401
Comber, R., Ganglbauer, E., Choi, J. H-. J., Hoonhout, J., Rogers, Y., O'hara, K., and Maitland, J. (2012). “Food and interaction design: designing for food in everyday life,” in CHI '12 Extended Abstracts on Human Factors in Computing Systems (Austin, TX: Association for Computing Machinery). doi: 10.1145/2212776.2212716
Cornelio, P., Dawes, C., Maggioni, E., Bernardo, F., Schwalk, M., Mai, M., et al. (2022a). Virtually tasty: an investigation of the effect of ambient lighting and 3D-shaped taste stimuli on taste perception in virtual reality. Int. J. Gastron. Food Sci. 30, 100626. doi: 10.1016/j.ijgfs.2022.100626
Cornelio, P., de Pirro, S., Vi, C. T., and Subramanian, S. (2017). “Agency in mid-air interfaces,” in Proceedings of the 2017 CHI Conference on Human Factors in Computing Systems (Denver, CO: Association for Computing Machinery), 2426–2439. doi: 10.1145/3025453.3025457
Cornelio, P., Haggard, P., Hornbaek, K., Georgiou, O., Bergström, J., Subramanian, S., et al. (2022b). The sense of agency in emerging technologies for human–computer integration: a review. Front. Neurosci. 16, 949138. doi: 10.3389/fnins.2022.949138
Cornelio, P., Maggioni, E., Brianza, G., Subramanian, S., and Obrist, M. (2020). “SmellControl: the study of sense of agency in smell,” in Proceedings of the 2020 International Conference on Multimodal Interaction (Virtual Event, Netherlands: Association for Computing Machinery). doi: 10.1145/3382507.3418810
Cornelio, P., Velasco, C., and Obrist, M. (2021). Multisensory Integration as per technological advances: a review. Front. Neurosci. 15, 652611. doi: 10.3389/fnins.2021.652611
Coyle, D., Moore, J., Kristensson, P. O., Fletcher, P., and BLackwell, A. (2012). “I did that! Measuring users' experience of agency in their own actions,” in Proceedings of the SIGCHI Conference on Human Factors in Computing Systems (Austin, TX: Association for Computing Machinery), 2025–2034. doi: 10.1145/2207676.2208350
Crisinel, A. S., and Spence, C. (2010). As bitter as a trombone: synesthetic correspondences in nonsynesthetes between tastes/flavors and musical notes. Atten. Percep. Psychophysi. 72, 1994–2002. doi: 10.3758/APP.72.7.1994
David, N., Newen, A., and Vogeley, K. (2008). The “sense of agency” and its underlying cognitive and neural mechanisms. Conscious. Cogn. 17, 523–534. doi: 10.1016/j.concog.2008.03.004
Deng, J., Wang, Y., Velasco, C., Bertran, F. A. A., Comber, R., Obrist, M., et al. (2021). “The future of human-food interaction,” in Extended Abstracts of the 2021 CHI Conference on Human Factors in Computing Systems (Yokohama, Japan: Association for Computing Machinery). doi: 10.1145/3411763.3441312
Ebert, J. P., and Wegner, D. M. (2010). Time warp: authorship shapes the perceived timing of actions and events. Conscious. Cogn. 19, 481–489. doi: 10.1016/j.concog.2009.10.002
Elijah, R. B., Le Pelley, M. E., and Whitford, T. J. (2016). Modifying temporal expectations: Changing cortical responsivity to delayed self-initiated sensations with training. Biol. Psychol. 120, 88–95. doi: 10.1016/j.biopsycho.2016.09.001
Eskine, K. J., Kacinik, N. A., and Prinz, J. J. (2011). A bad taste in the mouth: gustatory disgust influences moral judgment. Psychol. Sci. 22, 295–299. doi: 10.1177/0956797611398497
Evangelou, G., Limerick, H., and Moore, J. (2021). “I feel it in my fingers! Sense of agency with mid-air haptics,” in 2021 IEEE World Haptics Conference (Montreal, QC: IEEE), 727–732.
Farooq, U., and Grudin, J. (2016). Human-computer integration. Interactions 23, 26–32. doi: 10.1145/3001896
Faul, F., Erdfelder, E., Lang, A.-G., and Buchner, A. (2007). G* Power 3: a flexible statistical power analysis program for the social, behavioral, and biomedical sciences. Behav. Res. Methods 39, 175–191. doi: 10.3758/BF03193146
Gayler, T. (2017). “Towards edible interfaces: designing interactions with food,” in Proceedings of the 19th ACM International Conference on Multimodal Interaction (Glasgow, UK: Association for Computing Machinery). doi: 10.1145/3136755.3137030
Gentsch, A., and Synofzik, M. (2014). Affective coding: the emotional dimension of agency. Front. Hum. Neurosci. 8, 608. doi: 10.3389/fnhum.2014.00608
Gentsch, A., Weiss, C., Spengler, S., Synofzik, M., and Schütz-Bosbach, S. (2015). Doing good or bad: how interactions between action and emotion expectations shape the sense of agency. Soc. Neurosci. 10, 418–430. doi: 10.1080/17470919.2015.1006374
Georgieff, N., and Jeannerod, M. (1998). Beyond consciousness of external reality: a “who” system for consciousness of action and self-consciousness. Conscious. Cogn. 7, 465–477. doi: 10.1006/ccog.1998.0367
Green, B. G., Dalton, P., Cowart, B., Shaffer, G., Rankin, K., Higgins, J., et al. (1996). Evaluating the ‘labeled magnitude scale' for measuring sensations of taste and smell. Chem. Senses 21, 323–334. doi: 10.1093/chemse/21.3.323
Green, B. G., Shaffer, G. S., and Gilmore, M. M. (1993). Derivation and evaluation of a semantic scale of oral sensation magnitude with apparent ratio properties. Chem. Senses 8, 683–702. doi: 10.1093/chemse/18.6.683
Green, E., Jacobson, A., Haase, L., and Murphy, C. (2015). Neural correlates of taste and pleasantness evaluation in the metabolic syndrome. Brain Res. 1620, 57–71. doi: 10.1016/j.brainres.2015.03.034
Haggard, P. (2017). Sense of agency in the human brain. Nat. Rev. Neurosci. 18, 196–207. doi: 10.1038/nrn.2017.14
Haggard, P., Clark, S., and Kalogeras, J. (2002). Voluntary action and conscious awareness. Nat. Neurosci. 5, 382–385. doi: 10.1038/nn827
Haselager, P. (2013). Did i do that? Brain–computer interfacing and the sense of agency. Minds Mach. 23, 405–418. doi: 10.1007/s11023-012-9298-7
Hellmann, J. H., Thoben, D. F., and Echterhoff, G. (2013). The sweet taste of revenge: gustatory experience induces metaphor-consistent judgments of a harmful act. Soc. Cogn. 31, 531. doi: 10.1521/soco.2013.31.5.531
Hoemann, K., Xu, F., and Barrett, L. F. (2019). Emotion words, emotion concepts, and emotional development in children: a constructionist hypothesis. Dev. Psychol. 55, 1830–1849. doi: 10.1037/dev0000686
Hoogeveen, H. R., Dalenberg, J. R., Renken, R. J., ter Horst, G. J., and Lorist, M. M. (2015). Neural processing of basic tastes in healthy young and older adults — an fMRI study. Neuroimage 119, 1–12. doi: 10.1016/j.neuroimage.2015.06.017
Hupfeld, A., and Rodden, T. (2012). “Laying the table for HCI: uncovering ecologies of domestic food consumption,” in Proceedings of the SIGCHI Conference on Human Factors in Computing Systems (Austin, TX: Association for Computing Machinery). doi: 10.1145/2207676.2207694
Hurault, J. C., Broc, G., Crône, L., Tedesco, A., and Brunel, L. (2020). Measuring the sense of agency: a French adaptation and validation of the sense of agency scale (F-SoAS). Front. Psychol. 11, 584145. doi: 10.3389/fpsyg.2020.584145
Iatridi, V., Hayes, J. E., and Yeomans, M. R. (2019). Quantifying sweet taste liker phenotypes: Time for some consistency in the classification criteria. Nutrients 11, 129. doi: 10.3390/nu11010129
Informedhealth.org. (2006). How Does Our Sense of Taste Work? Cologne: Institute for Quality and Efficiency in Health Care (IQWiG). Available online at: https://www.ncbi.nlm.nih.gov/books/NBK279408/ (accessed December 26, 2022).
Jones, S. R., de-Wit, L., Fernyhough, C., and Meins, E. (2008). A new spin on the wheel of fortune: priming of action-authorship judgements and relation to psychosis-like experiences. Conscious. Cogn. 17, 576–586. doi: 10.1016/j.concog.2007.08.008
Kasahara, S., Nishida, J., and Lopes, P. (2019). “Preemptive action: Accelerating human reaction using electrical muscle stimulation without compromising agency,” in Proceedings of the 2019 CHI Conference on Human Factors in Computing Systems (Glasgow: Association for Computing Machinery), 1−15. doi: 10.1145/3290605.3300873
Khot, R. A., Aggarwal, D., Pennings, R., Hjorth, L., and Mueller, F. (2017). “EdiPulse: investigating a playful approach to self-monitoring through 3D printed chocolate treats,” in Conference on Human Factors in Computing Systems - Proceedings (Denver, CO), 2017.
Khot, R. A., and Mueller, F. (2019). Human-food interaction. Found. Trends Hum. Comput. Interact. 12, 238–415. doi: 10.1561/1100000074
Lakens, D. (2013). Calculating and reporting effect sizes to facilitate cumulative science: a practical primer for t-tests and ANOVAs. Front. Psychol. 4, 863. doi: 10.3389/fpsyg.2013.00863
Lapis, T. J., Penner, M. H., and Lim, J. (2016). Humans can taste glucose oligomers independent of the hT1R2/hT1R3 sweet taste receptor. Chem. Senses 41, 755–776. doi: 10.1093/chemse/bjw088
Lawless, H. T., Stevens, D. A., Chapman, K. W., and Kurtz, A. (2005). Metallic taste from electrical and chemical stimulation. Chem. Senses 30, 185–194. doi: 10.1093/chemse/bji014
Libet, B., Gleason, C. A., Wright, E. W., and Pearl, D. K. (1983). Time of conscious intention to act in relation to onset of cerebral activity (readiness-potential). The unconscious initiation of a freely voluntary act. Brain. 106, 623–642.
Limerick, H., Moore, J. W., and Coyle, D. (2015). Empirical evidence for a diminished sense of agency in speech interfaces. Proceedings of the 33rd Annual ACM Conference on Human Factors in Computing Systems, ACM, 3967–3970. doi: 10.1145/2702123.2702379
Liu, P., Shah, B. P., Croasdell, S., and Gilbertson, T. A. (2011). Transient receptor potential channel type M5 is essential for fat taste. J. Neurosci. 31, 8634–8642. doi: 10.1523/JNEUROSCI.6273-10.2011
Matsumoto, I. (2013). Gustatory neural pathways revealed by genetic tracing from taste receptor cells. Biosci. Biotechnol. Biochem. 77, 1359–62. doi: 10.1271/bbb.130117
Maynes-Aminzade, D. (2005). “Edible bits: Seamless interfaces between people, data and food,” in CHI Extended Abstracts (Portland, OR).
Meier, B. P., Moeller, S. K., Riemer-Peltz, M., and Robinson, M. D. (2012). Sweet taste preferences and experiences predict prosocial inferences, personalities, and behaviors. J. Pers. Soc. Psychol. 102, 163–74. doi: 10.1037/a0025253
Moore, J. W., Middleton, D., Haggard, P., and Fletcher, P. C. (2012). Exploring implicit and explicit aspects of sense of agency. Conscious. Cogn. 21, 1748–1753. doi: 10.1016/j.concog.2012.10.005
Murer, M., Aslan, I., and Tscheligi, M. (2013). “LOLLio: exploring taste as playful modality,” in Proceedings of the 7th International Conference on Tangible, Embedded and Embodied Interaction - TEI '13 (Barcelona).
Narumi, T., Kajinami, T., Tanikawa, T., and Hirose, M. (2010). “Meta cookie,” in ACM SIGGRAPH 2010 Posters on - SIGGRAPH '10 (Los Angeles, CA: ACM) doi: 10.1145/1836845.1836998
Obrist, M., Comber, R., Subramanian, S., Piqueras-Fiszman, B., Velasco, C., Spence, C., et al. (2014a). “Temporal, affective, and embodied characteristics of taste experiences: a framework for design,” in Proceedings of the SIGCHI Conference on Human Factors in Computing Systems (Toronto, ON: Association for Computing Machinery) doi: 10.1145/2556288.2557007
Obrist, M., Gatti, E., Maggioni, E., Vi, C. T., and Velasco, C. (2017). “Multisensory Experiences in HCI,” in IEEE Multimedia. p. 24. doi: 10.1109/MMUL.2017.33
Obrist, M., Tuch, A. N., and Hornbaek, K. (2014b). “Opportunities for odor: experiences with smell and implications for technology,” in Proceedings of the SIGCHI Conference on Human Factors in Computing Systems (Toronto, ON: Association for Computing Machinery). doi: 10.1145/2556288.2557008
Osumi, M., Nobusako, S., Zama, T., Yokotani, N., Shimada, S., Maeda, T., et al. (2019). The relationship and difference between delay detection ability and judgment of sense of agency. PLoS ONE 14, e0219222. doi: 10.1371/journal.pone.0219222
Purves, D., Cabeza, R., Huettel, S. A., Labar, K. S., Platt, M. L., Woldorff, M. G., et al. (2008). Cognitive Neuroscience. Sunderland: Sinauer Associates, Inc.
Roessler, J., and Eilan, N. (2003). Agency and Self-awareness: Issues in Philosophy and Psychology. Oxford: Oxford University Press.
Schacter, D., Gilbert, D., Wegner, D., and Hood, B. M. (2011). Psychology: European Edition. London: Macmillan International Higher Education.
Schoenfeld, M. A., Neuer, G., Tempelmann, C., Schüßler, K., Noesselt, T., Hopf, J. M., et al. (2004). Functional magnetic resonance tomography correlates of taste perception in the human primary taste cortex. Neuroscience 127, 347–35. doi: 10.1016/j.neuroscience.2004.05.024
Shneiderman, B. (1997). Designing the User Interface: Strategies for Effective Human-Computer Interaction. New York, NY: Addison-Wesley Longman Publishing Co., Inc.
Simon, S. A., and de Araujo, I. E. (2005). The salty and burning taste of capsaicin. J. Gen. Physiol. 125, 531–534. doi: 10.1085/jgp.200509329
Synofzik, M., Vosgerau, G., and Voss, M. (2013). The experience of agency: an interplay between prediction and postdiction. Front. Psychol. 4, 127. doi: 10.3389/fpsyg.2013.00127
Velasco, C., Carvalho, F. R., Petit, O., and Nijholt, A. (2016). “A multisensory approach for the design of food and drink enhancing sonic systems,” in Proceedings of the 1st Workshop on Multi-sensorial Approaches to Human-Food Interaction (Tokyo: Association for Computing Machinery). doi: 10.1145/3007577.3007578
Vi, C. T., Ablart, D., Arthur, D., and Obrist, M. (2017a). “Gustatory interface: The challenges of 'how' to stimulate the sense of taste,” in MHFI 2017 - Proceedings of the 2nd ACM SIGCHI International Workshop on Multisensory Approaches to Human-Food Interaction, Co-located with ICMI 2017 (Glasgow), 29–33. doi: 10.1145/3141788.3141794
Vi, C. T., Arthur, D., and Obrist, M. (2018). “TasteBud: Bring taste back into the game,” in MHFI 2018 - 3rd Workshop on Multisensory Approaches to Human-Food Interaction (Boulder, CO). doi: 10.1145/3279954.3279955
Vi, C. T., Marzo, A., Ablart, D., Memoli, G., Subramanian, S., Drinkwater, B., et al. (2017b). Tastyfloats: a contactless food delivery system. Proceedings of the 2017 ACM International Conference on Interactive Surfaces and Spaces, ISS 2017, 161–170. doi: 10.1145/3132272.3134123
Vi, C. T., Marzo, A., Memoli, G., Maggioni, E., Ablart, D., Yeomans, M., et al. (2020). LeviSense: a platform for the multisensory integration in levitating food and insights into its effect on flavour perception. Int. J. Hum. Comput. Stud. 139, 102428. doi: 10.1016/j.ijhcs.2020.102428
Vi, C. T., and Obrist, M. (2018). Sour promotes risk-taking: an investigation into the effect of taste on risk-taking behaviour in humans. Sci. Rep. 8, 1–8. doi: 10.1038/s41598-018-26164-3
Wakeling, I. N., and MacFie, H. J. H. (1995). Designing consumer trials balanced for first and higher orders of carry-over effect when only a subset of k samples from t may be tested. Food Qual. Prefer. 6, 299–308. doi: 10.1016/0950-3293(95)00032-1
Wang, W., Yao, L., Zhang, T., Cheng, C-. Y., Levine, D., Ishii, H., et al. (2017). “Transformative appetite: shape-changing food transforms from 2D to 3D by water interaction through cooking,” in Proceedings of the 2017 CHI Conference on Human Factors in Computing Systems (Denver, CO: Association for Computing Machinery). doi: 10.1145/3025453.3026019
Wassertheil, S., and Cohen, J. (1970). Statistical power analysis for the behavioral sciences. Biometrics 26, 588. doi: 10.2307/2529115
Wilberz, A., Leschtschow, D., Trepkowski, C., Maiero, J., Kruijff, E., Riecke, B., et al. (2020). “FaceHaptics: Robot arm based versatile facial haptics for immersive environments,” in Proceedings of the 2020 CHI Conference on Human Factors in Computing Systems (Honolulu, HI: Association for Computing Machinery). doi: 10.1145/3313831.3376481
Winkler, P., Stiens, P., Rauh, N., and Franke, T. KREMS, J. (2020). How latency, action modality and display modality influence the sense of agency: a virtual reality study. Virtual Real. 24, 411–422. doi: 10.1007/s10055-019-00403-y
Zanatto, D., Bifani, S., and Noyes, J. (2023). “Constraining the sense of agency in human-machine interaction,” in International Journal of Human-Computer Interaction. p. 1–12.
Zhao, G. Q., Zhang, Y., Hoon, M. A., Chandrashekar, J., Erlenbach, I., Ryba, N. J., ZUKER, C. S., et al. (2003). The receptors for mammalian sweet and umami taste. Cell 115, 255–266. doi: 10.1016/S0092-8674(03)00844-4
Keywords: sense of agency (SoA), gustatory interfaces, human taste interaction, sweet liking phenotype, sweet dislikers, sweet likers
Citation: Vi CT, Cornelio P, Obrist M and Yeomans M (2023) “Sweet: I did it”! Measuring the sense of agency in gustatory interfaces. Front. Comput. Sci. 5:1128229. doi: 10.3389/fcomp.2023.1128229
Received: 20 December 2022; Accepted: 24 April 2023;
Published: 12 May 2023.
Edited by:
Mariella Pazzaglia, Sapienza University of Rome, ItalyReviewed by:
Kostas Karpouzis, Panteion University, GreeceAnton Nijholt, University of Twente, Netherlands
Copyright © 2023 Vi, Cornelio, Obrist and Yeomans. This is an open-access article distributed under the terms of the Creative Commons Attribution License (CC BY). The use, distribution or reproduction in other forums is permitted, provided the original author(s) and the copyright owner(s) are credited and that the original publication in this journal is cited, in accordance with accepted academic practice. No use, distribution or reproduction is permitted which does not comply with these terms.
*Correspondence: Chi Thanh Vi, dmN0aGFuaEBoY21pdS5lZHUudm4=