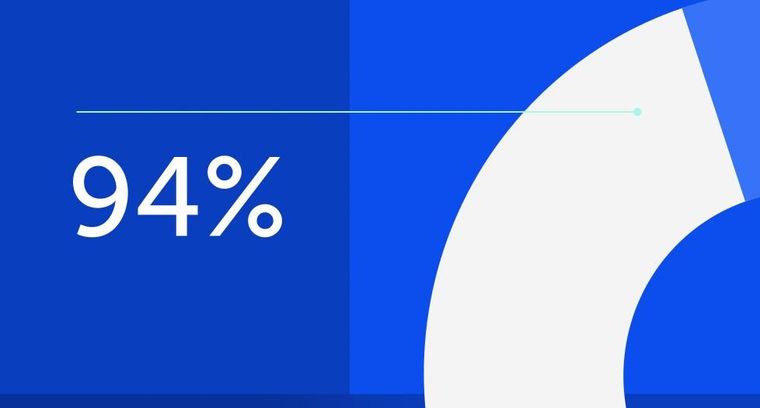
94% of researchers rate our articles as excellent or good
Learn more about the work of our research integrity team to safeguard the quality of each article we publish.
Find out more
ORIGINAL RESEARCH article
Front. Comput. Sci., 24 May 2022
Sec. Human-Media Interaction
Volume 4 - 2022 | https://doi.org/10.3389/fcomp.2022.873437
This article is part of the Research TopicGovernance AI EthicsView all 9 articles
The increasingly pervasive role of Artificial Intelligence (AI) in our societies is radically changing the way that social interaction takes place within all fields of knowledge. The obvious opportunities in terms of accuracy, speed and originality of research are accompanied by questions about the possible risks and the consequent responsibilities involved in such a disruptive technology. In recent years, this twofold aspect has led to an increase in analyses of the ethical and political implications of AI. As a result, there has been a proliferation of documents that seek to define the strategic objectives of AI together with the ethical precautions required for its acceptable development and deployment. Although the number of documents is certainly significant, doubts remain as to whether they can effectively play a role in safeguarding democratic decision-making processes. Indeed, a common feature of the national strategies and ethical guidelines published in recent years is that they only timidly address how to integrate civil society into the selection of AI objectives. Although scholars are increasingly advocating the necessity to include civil society, it remains unclear which modalities should be selected. If both national strategies and ethics guidelines appear to be neglecting the necessary role of a democratic scrutiny for identifying challenges, objectives, strategies and the appropriate regulatory measures that such a disruptive technology should undergo, the question is then, what measures can we advocate that are able to overcome such limitations? Considering the necessity to operate holistically with AI as a social object, what theoretical framework can we adopt in order to implement a model of governance? What conceptual methodology shall we develop that is able to offer fruitful insights to governance of AI? Drawing on the insights of classical pragmatist scholars, we propose a framework of democratic experimentation based on the method of social inquiry. In this article, we first summarize some of the main points of discussion around the potential societal, ethical and political issues of AI systems. We then identify the main answers and solutions by analyzing current national strategies and ethics guidelines. After showing the theoretical and practical limits of these approaches, we outline an alternative proposal that can help strengthening the active role of society in the discussion about the role and extent of AI systems.
Artificial intelligence is becoming pervasive in our societies at an increasing rate. Multiple actors in several domains from healthcare to warfare have introduced AI based technologies in order to improve the analysis and processing of large amounts of data. The widespread application of AI technology raises questions about its societal impacts. Alongside the increasingly polarized aspects related to the impact of controversial technologies, like robots and mass surveillance algorithms, scholars have raised the attention on current side effects of AI-based systems, suggesting that the development of concrete governance of AI cannot be longer delayed (Pasquale, 2015; O'Neil, 2016; Crawford, 2021).
Accordingly, these concrete risks and threats, together with the necessity to regulate the trajectories of AI research, have generated a proliferation of national strategies documents (Dutton, 2018; Berryhill et al., 2019; Misuraca and Van Noordt, 2020; Van Roy et al., 2021) as well as guidelines listing fundamental moral principles to be observed when designing AI-based technologies. For instance, scholars and practitioners have frequently highlighted the necessity to respect fundamental values of privacy, personal freedom, and respect (Floridi et al., 2018; European Commission, 2020). Concurrently, number of scholars have suggested various AI governance frameworks and operationalization of them in public administration (Wallach and Marchant, 2018; Winfield and Jirotka, 2018; Clarke, 2019; Sun and Medaglia, 2019; Ulnicane et al., 2021).
However, despite a number of ethical guidelines and governance frameworks utilized in policymaking, less attention has been dedicated to the concrete application of the trajectories and objectives that AI-based technologies should follow in order to respect value-based norms. In fact, although the appeals to mechanisms like that of accountability and transparency, and principles such as fairness are widely shared, it is not clear how they should be implemented, particularly when considering the plurality of actors, values and interests present in the current societal constellation. It has been argued that these indications suffer from indeterminacy and abstractness, puzzling designers and developers about their implementation (Hagendorff, 2020). Furthermore, their fuzziness together with an overproduction of overlapping documents has raised some doubts about the real intentions behind their adoption in terms of ethics washing (Bietti, 2020). While there are also suggestions to operationalize ethical principles (Yeung et al., 2019; de Almeida et al., 2021; Stix, 2021; Sigfrids et al., 2022), to our knowledge, there are yet no empirical evidence, pilots or experimentations on suggested solutions.
On the one hand, we argue that ethics guidelines are based on an ideal model, assuming that individuals can pursue ethically sound processes by following universal principles. This conception stands on the presumption of a well-informed abstract subject that shares and apply those principles without considerations about complexities of her/his social environment. The resolution of ethics into a set of fixed principles overlooks their relationship with a socio-economic environment formed by a plurality of contextual values, power asymmetries, interests and material conditions necessary to implement AI-based technologies (Crawford, 2021). In addition, social contexts are in constant change, making any precise predictions even more difficult. Such abstractness, together with the arbitrary way of selecting those principles (it is not clear, why exactly one particular set of principles should be selected over another set of principles and followed as a basis for AI policy) for the deployment and implementation of AI systems, witnesses a gap between the establishment of acceptable norms and their acceptance in plural and complex societies, which raises questions about the legitimacy and efficacy of current ethics guidelines.
On the other hand, the political ambitions reflected in the torrent of published national AI strategies and policies predominantly emphasize the necessity to preserve the general wellbeing of society and focus on political and economic objectives in the implementation of AI, without providing further indications on how such objectives are identified according to a democratic process and public engagement of civil society stakeholders, nor do they discuss how these objectives would match societal challenges identified by citizens. Instead, the governance approaches presented in the national AI strategies appear to rely on the above-mentioned ethical guidelines and abstract moral principles, thus lacking concrete approaches toward responsible and ethical civil society engagement. The suggested governance frameworks, in turn, tend to remain relatively abstract and ambiguous regarding, for instance, policy related aspects of power.
If both national strategies and ethics guidelines appear to be neglecting the necessary role of a democratic scrutiny for identifying challenges, objectives, strategies and the appropriate regulatory measures that such a disruptive technology should undergo, the question is then, what suggestions can we advocate that are able to overcome such limitations? In other words, considering the necessity to operate holistically with AI as a social object, what theoretical framework can we adopt in order to implement a model of governance (Ostrom, 2005)? What conceptual methodology shall we develop that is able to offer fruitful insights to governance of AI?
While we are starting to find suggestions amongst scholars to engage stakeholders and citizens in the process of governing AI implementation (Yeung et al., 2019; Delacroix and Wagner, 2021; Stix, 2021), these are not mirrored in official documents delineating the regulatory framework of AI. We argue that a truly democratic approach to AI cannot be enforced if contextual actors are not included in the discussion about what values, objectives and challenges the AI systems should address. Drawing on the insights of classical pragmatist scholars like John Dewey and Mead, we propose a framework of democratic experimentation based on the method of social inquiry. Furthermore, we will show how such a democratic theory has a concrete ethical value as it builds on the reflexive cooperation amongst individuals that can generate a significant development of freedom, equality, and solidarity. Therefore, to be able to avoid technocratic and arbitrary approaches, we argue that it is necessary to take a step back and adopt forms of governance that are able to offer cooperative and participatory solutions.
In this article, we first summarize some of the main points of discussion around the potential societal, ethical and political issues of AI systems. We then identify the main answers provide by public and private actors by analyzing current national strategies and ethics guidelines. After showing the theoretical and practical limits of these approaches, we delineate an alternative proposal that can help strengthening the active role of society in the discussion about the role and extent of AI systems.
Applications of AI are growingly adopted in different walks of life like health, finance, security, agriculture, and transport. The volume of data that AI systems can analyze is unprecedented and can provide with new knowledge in a timely manner. The importance of being among the protagonists of the emerging AI society has been portrayed as a “race” (Cave and ÓhÉigeartaigh, 2018) amongst states toward amassing investments from national and supranational institutions in order to achieve global technological supremacy (Saran et al., 2018).
The immediate benefits generated by the adoption of AI systems are of economic and social nature. Some popular examples of the opportunities entailed by AI can be identified in autonomous cars, robots, online customer support and the automatization of repetitive and alienating tasks (Makridakis, 2017). In economics, Koehler (2018) has shown how AI can strengthen business models by optimizing parameters and minimizing classification errors, improving the process in terms of data collection, prediction, decision, and action. Other tangible examples of these positive outcomes concern the time consumption in hiring processes or venue selections, in sectors like tourism (Johnson et al., 2020). In the health sector AI has proven beneficial to improve the diagnostic phase, operational accuracy, and patient care as well as bringing an overall cost reduction (Hague, 2019; Yeasmin, 2019). In agriculture the creation of digital twins or big data collection through sensors has significantly impacted the management processes and the productivity of this field (Smith, 2018). Lazic (2019) has also highlighted the strategic role of AI in the identification of obscuration processes in terms of cybersecurity. AI can also increase objectivity in juridical evaluations when assessed with non-ideal human decision-making scenarios (Green and Chen, 2019; Yu and Du, 2019).
Moreover, AI can entail long-term political effects by strengthening the role of certain groups or countries. For instance, Allen (2019) has highlighted the effort made by the Chinese government to enhance national competitiveness and protect national security. Although Toll et al. (2019) have shown that the discourse around AI benefits in the public sector might be over optimistic given the current state of the art, it is true that public institutions and governments are investing a considerable amount of resources in the future development of AI (Saran et al., 2018).
Concurrently, AI technology has raised several concerns because of aspects related to its autonomy and uncertain side-effects. Some of the main issues are of moral nature and relate to privacy, discrimination and responsibility. First, it is not always clear what data are collected and what are the exact purposes of such processing. Although regulations like GDPR help avoiding mishandling of data collection, the process of consent is often too complex and socially driven, disregarding the necessary and actual freedom that individuals should have when sharing their data. Wachter et al. (2017), have also questioned the ambiguity of the General Data Protection Regulation 2016/679 (GDPR) indications about automated decision-making and the right to explanation.
Secondly, AI is designed following existing socio-technical knowledge and economic interests that are contextually formed and that can inadvertently be integrated into an algorithm (Hagendorff, 2020). Consequently, algorithms tend to reflect the specific perspectives of the designers. It is highly possible that instead of correcting human arbitrariness toward increasing objectivity, AI can reproduce and reinforce various existing subjective perspectives. The fact that machines are often invested with an aura of technical infallibility can result in decreased critical scrutiny. Recent examples have shown how investigations and assessments relying too strongly on automatic processing can become discriminatory. This has become particularly evident in the field of facial recognition used, amongst other applications, in hiring processes. Raji et al. (2020) for instance have argued that the automatic selection to reduce discriminatory effects on the basis of ethnicity can increase racial biases instead of reducing them. They have shown that the demographic benchmark necessary to inform the algorithm can often prioritize groups or profiles that are easier to categorize. Benthall and Hynes (2019) have highlighted that although “ethnicity” is an attribute that can be identified with racial categories, these are themselves social constructs, grouping individuals with different phenotypic traits and cultural backgrounds. Consequently, AI can then legitimize stereotypical evaluations and disregard non-conforming individuals.
This leads to the third point concerning the responsibility for the potential negative effects generated or perpetrated by an AI system. It is challenging to identify the appropriate responsible parties in a process that involves ‘many hands' and that evolves as AI are many times self-learning systems. If the autonomous systems do not fall under the broader mechanisms of responsibility, then their autonomy appears questionable and even dangerous.
These three specific aspects have raised discussions on the overall opacity of AI processing systems, as well as its immediate and longer terms objectives. Furthermore, from a more philosophical point of view, debates have also rotated around the new ontology potentially emerging with AI, raising questions about autonomy (Calvo et al., 2020), the relation between subjects, objects, and new moral (Russia, 2019) and juridical categories under which AI should fall (Bennett and Daly, 2020). In the next sections, we shortly identify and analyze the main answers that have been provided by public institutions and private actors to address these concerns.
The field of AI governance has developed around the need to understand, control, govern, steer and shape AI technology as well as the institutions and contexts around which it is built (Dafoe, 2018, p. 5). Therefore, the research contributions and suggestions on public AI governance have proliferated during the last few years (Cath, 2018; Cath et al., 2018; e.g., Taeihagh, 2021), with the role of public sector being under particular scrutiny (de Sousa et al., 2019; Kuziemski and Misuraca, 2020; e.g., Zuiderwijk et al., 2021). It is possible to divide these contributions on public AI governance into three categories (see Sigfrids et al., 2022).
The first category includes contributions suggesting comprehensive governance frameworks aiming to create an overall understanding of the wide systemic socio-technical phenomenon and suggest broader sets of integrated approaches and tools to govern such a phenomenon. There are some differences among the contributions for instance in terms of how much weight they give to so-called soft governance mechanisms (not lawfully binding steering e.g., with information) and hard governance (binding regulation). In some contributions, more room is given to self-organization of AI developers, while public governance provides the general statutory framework in which the development should take place (Wirtz et al., 2020; de Almeida et al., 2021). In others, all AI systems should be subjected to relatively strict regulation and enforcement (Wallach and Marchant, 2018; Yeung et al., 2019). Overall, the contributions in this first category are concerned with the societal impacts of AI and see that negative impacts should be avoided with informed policy, which is supported among others by impact assessments and stakeholder consultations (Sigfrids et al., 2022).
The second category focuses on the processes by which public governance of AI should be conducted. The specific attention in these contributions is on the practical governance processes and principles by which such processes should work. Attention has been paid to the questions on how stakeholder engagement and public deliberation should be organized (Sun and Medaglia, 2019; Buhmann and Fieseler, 2021; Ulnicane et al., 2021), about the nature of regulation and steering processes, and with regard to the need of long-term and adaptive governance strategies and practices (Liu and Maas, 2021). It has been also suggested that a specific regulatory agency or relevant administrative bodies should have responsibility of approval of algorithms, supervision of AI developers, impact assessments, as well as certification and testing of algorithms (Bannister and Connolly, 2020; Dignam, 2020). In general, the contributions of this category argue that governance should support the operationalization of principles of good governance and AI ethics (Sigfrids et al., 2022).
The third category deals specifically with the question of how ethics and human rights principles could be operationalized to the policy-making process with concrete tools and mechanisms. This third category overlaps to some extent with the second category. The contributors (Yeung et al., 2019; e.g., Stix, 2021) of the third category emphasize that a major challenge for the suggested AI ethics principles and frameworks is their limited uptake in the actual AI policy and development practices. This is in spite of the number of relatively concrete and operational governance tools and mechanisms that have been suggested, including the relatively detailed proposals on various technical tools, development of legal and coordination procedures, instruments and institutions, as well as financial incentives for ethical principles and procedures (Wallach and Marchant, 2018; Sun and Medaglia, 2019; Tsamados et al., 2021). Additionally, in order to ensure compliance with the operationalized ethics and human rights principles, concrete administrational structures for the monitoring and controlling compliance as well as sanction mechanisms have also been presented (Wallach and Marchant, 2018; Sun and Medaglia, 2019; Tsamados et al., 2021; see Sigfrids et al., 2022).
However, when AI ethics guidelines and frameworks are utilized as a basis for governance, there are often political issues that can be left out of the scope of discussion. Crawford (2021) has recently pointed out that ethics guidelines tend to shift the attention away from issues such as working conditions, environmental footprint, and deep cultural changes. These are only three examples of the manifold repercussions that AI is generating that are not explicitly addressed by the ethical guidelines. These aspects are the expression of power dynamics present in our societies, which may not be mitigated but rather reinforced through AI-based technologies. Jobin et al. (2019) for instance, have shown that ethics codes are mostly produced by economically developed countries leaving little space of discussion to less powerful actors. Accordingly, as Crawford (2021) concludes, AI ethics is necessary but not sufficient to deal with dynamics of power entrenched in AI development.
Furthermore, apart from the current legislation that to some extent concerns AI and steers its implementation (e.g., GDPR and the general principles of good public governance), recently proposed legislation on AI1, and some program or organization based initiatives to our knowledge, there is yet no systematic empirical or real-life experiment on putting these suggestions systematically and comprehensively into practice. Thus, for the time being, the suggested models have been more or less hypothetical, based on rational scrutiny and aimed at facilitating policy and AI governance development (Sigfrids et al., 2022).
Thus, the existing governance and policy-making seems to reside at an arm's length from the suggested ethics guidelines and governance frameworks, leaving room for continuing discussion on the actual use of power and democratic mechanisms in the policy-making and governance of AI. In the following, we focus on this aspect by asking what are the current policy frameworks for utilizing AI, and how a more democratic approach could be supported with ideas from social philosophy.
Thus far, the most important policy attempts to define societal aims and frameworks for the utilization of AI can be found in the national AI strategies that several governments around the world have promulgated in the last 5 years. In the following section we will shorty analyze the main objectives and visions of the societal utilization of AI and measures to achieve them.
Given the crucial importance of AI for future economic and political positioning, Nation-states around the world are seeking to develop their capacity to harness and steer AI development and deployment toward their particular national ends (Radu, 2021). One manifestation of this trend has been the fast-paced global proliferation of National AI strategies from 2016 onwards (Dutton, 2018). As Ulnicane et al. (2020, p. 165) observe, the framing of AI as a separate strategic technological priority in need of a dedicated national strategy, is an important fact in itself. The simultaneous publication of dozens of national strategies constructed around a single technology (Dutton, 2018, p. 4), reveals the role of AI as the “key sociotechnical institution” of our age (Bareis and Katzenbach, 2021, p. 22).
Despite their relative recent origin, there have been various studies performed on National AI strategies (Dexe and Franke, 2020; Viscusi et al., 2020; Fatima et al., 2021; Saariluoma and Salo-Pöntinen, 2021; Saveliev and Zhurenkov, 2021; Wilson, 2022). National AI strategies have been analyzed for example in terms of the sociotechnical imaginaries (Bareis and Katzenbach, 2021) and political imaginaries (Paltieli, 2021) that they contain. Fatima et al. (2020) have analyzed over 30 national strategies produced during the last 5 years in order to understand national-level strategic actions in the field of AI. Although the strategies offer distinct national imaginaries, priorities and approaches (Bareis and Katzenbach, 2021), they are also remarkably similar in many ways (Fatima et al., 2020). These similarities and differences in the national AI strategies have also been outlined in various analyses and reports (Dutton, 2018; Saran et al., 2018; Berryhill et al., 2019; Bradley and Wingfield, 2020; Misuraca and Van Noordt, 2020; e.g., Van Roy et al., 2021).
In terms of governance, national strategies are a key document class that outlines political aims and broader governance objectives in the field of AI. National AI strategies are a way of signaling the particular AI pathway or a rationale (Fatima et al., 2021) of a state in the field of global AI development, or “AI race” (Cave and ÓhÉigeartaigh, 2018). Besides this rationale, the strategies also contain various expectations, visions and narratives of AI technology, constituting sociotechnical imaginaries (Jasanoff and Kim, 2009) and imagined futures (Beckert, 2016) that are embedded in the national AI strategies (Bareis and Katzenbach, 2021).
Following Wilson (2022, p. 2), we analyze National AI strategies as “a consolidation mechanism in AI governance.” The public discourse around AI utilizes various metaphors, myths and rhetoric, which guide the societal discussion and future visions of AI (Campolo and Crawford, 2020). Through national strategies, governments allocate resources and governance functions according to various sociotechnical imaginaries (Bareis and Katzenbach, 2021, p. 4). Thus, AI governance can be analyzed as the mechanism that coordinates between the different competing visions for the development, deployment and regulation of AI, whereas national strategies consolidate the normative elements in the public discourse (Wilson, 2022). Indeed, national AI strategies have been aptly described as “a hybrid of policy and discourse that offers imaginaries, allocates resources, and sets rules” (Bareis and Katzenbach, 2021, p. 2). Moreover, because of their hybrid nature, AI strategies have specific democratic function in shaping the visionary potential for political agency by describing the relationship between citizens and governments (Paltieli, 2021).
As long-term strategic plans, national AI strategies provide valuable information on how states perceive the development of AI (Fatima et al., 2020). Moreover, in the absence of strong global AI regulation, the strategic documents outline important measures and objectives of nation states in the field of AI governance (Saveliev and Zhurenkov, 2021). National AI strategies inspire public attention, mobilize societal resources and direct coordination and steering efforts of the state (Misuraca and Van Noordt, 2020).
For the purposes of this article, we have conducted a qualitative content analysis of selected national AI strategies. In order to select suitable strategies for analysis, we have consulted various available databases (Future of Life, 2020; OPSI, 2020; Zhang et al., 2021) as well as reports and lists concerning national AI strategies (Dutton, 2018; Berryhill et al., 2019; Misuraca and Van Noordt, 2020; Van Roy et al., 2021). We began by searching for published AI strategies and their official or unofficial English language translations. Secondly, we compared the different lists and databases in order to form a comprehensive picture of the field of national AI strategies. The most prominent database that we used was the “The Observatory of Public Sector Innovation” (OPSI, 2020), which lists over 50 countries that have either been published or are in the process of drafting a national AI strategy (Berryhill et al., 2019).
It is important to note that most national AI strategies originate from high income countries around the world and particularly in Europe. Therefore, we aimed to select a dataset that would be a representative, yet also diverse, sample of the global landscape of National AI strategies. The selection criteria included geographical location, Gross National Product (GDP)2 as well as Digitalization Adoption Index (DAI)3. Table 1 outlining the selection criteria.
Besides the aforementioned criteria, there were other guiding factors in terms of the scope and focus of the analysis as well. While the AI policies of different countries are outlined in various national policy measures, instruments and documents related to AI, this analysis focuses solely on published national AI strategy documents. National AI strategy is therefore defined as a published document that presents the state's coordinated approach toward maximizing the societal benefits of AI and minimizing its risks (Dutton, 2018, p. 5). Accordingly, various papers and documents that focus on innovation policy, public sector transformation or digitalization are left out of the scope of analysis (Dutton, 2018). Moreover, our focus is solely on the strategic documents themselves and not the processes preceding them.
The selected sample of national AI strategies was chosen by cross-referencing various available databases and lists of strategies. The dataset consists of national strategies that were published before the chosen cut-off date August 2021. In the end, we chose to analyze the strategies of nine countries: China, Denmark, Germany, Japan, Russia, Singapore, United Kingdom, United States and Uruguay. Table 2 listing the selected National AI Strategies.
These countries were selected by applying the following criteria, including: the representation of major global AI technology developers; diversity of geographical location; and variance in the socio-economic background. After a tentative analysis on the sample of strategies, we concluded that no additional value would be provided by adding new strategies to the sample as the qualitative data was saturated. The final selection of countries considered the availability of published national AI strategies, the availability of trustworthy translations and the match of the strategy to our specific definition of national AI strategy. The selection methodology is outlined in Figure 1.
Figure 1. Methodology for selecting national AI strategies for the qualitative and thematic content analysis.
After the selection of the dataset, we analyzed the strategies thematically by paying specific attention to the following elements: What are the stated objectives and visions of the strategy? What are the perceived and stated challenges related to AI technology? What are the proposed or envisioned governance frameworks for AI? What are the stated ethical principles and values?
On the basis of our analysis, AI entails a great value in three different but interrelated areas. Firstly, it is fundamental in terms of national sovereignty and strategic geopolitical power through technological leadership (China, 2017; Russia, 2019; United States, 2019). Secondly, AI can attract international talents and businesses (United Kingdom, 2018, p. 16; Japan, 2019, p. 4) as well as creating new business models (Singapore, 2017, p. 5), strengthening industrial production and competitiveness (Germany, 2018, p. 8; Japan, 2019, p. 5). Thirdly, national strategies place an emphasis on wider societal transformation, adaptation and progress attained through the development and deployment of AI (Germany, 2018, pp. 9, 29–30; China, 2017, pp. 5,6; Japan, 2019, pp. 1–3).
In general, AI technology is perceived as a potential tool that can be utilized in the effort to alleviate or even solve societal challenges from low birthrates (Japan, 2019, p. 34) to environmental challenges (China, 2017, p. 20). The German AI strategy emphasizes that AI is a tool that can unleash human potential by reorienting it from repetitive tasks toward more creative tasks (Germany, 2018, p. 25). Japanese strategy envisions a thorough transformation of the Japanese society to a new age of AI through the concept of Society 5.0 (Japan, 2019, p. 3). Countries such as Uruguay and Denmark focus specifically on developing a leadership position in the ethical and responsible development and use of human-centered AI (Denmark, 2019, p. 8) particularly in the public sector (Uruguay, 2019, pp. 2–8).
However, countries are also aware of the potential upheavals entrenched in AI implementation especially if forms of “general” or “broad” AI are developed (Russia, 2019, pp. 4–5; Denmark, 2019, p. 5). The often-mentioned challenge related to the use of AI systems is their potential nature as “black-boxes,” whose decision-making logic can be opaque from the point of view of the end-users and citizens (Germany, 2018, p. 16). The Russian AI strategy notes that the lack of understanding concerning AI-based decision-making can become a hindrance to the development and deployment of AI in the society (Russia, 2019, p. 5). Moreover, because of the seemingly inherent complexity and opacity of AI-systems, their deployment can produce unintended and unforeseen impacts (Uruguay, 2019, p. 13).
The role of governments in the strategies is to shape and steer the fast-paced development of AI toward particular national goals (Radu, 2021). The ways to steer such process so that the risks inherent in its implementation can be mitigated are entrusted onto a set of objective standards, be those shared global norms, technical standards or ethical guidelines (Denmark, 2019, pp. 26,27, 30; United States, 2019, pp. 3967–3970). Indeed, all of the selected strategies mention at least some of the globally shared values of AI development and use, which form principles and normative operational tools such as trustworthiness, transparency, controllability, accountability, privacy, responsibility, safety and security (China, 2017; Singapore, 2017; Germany, 2018; United Kingdom, 2018; Denmark, 2019; Japan, 2019; Russia, 2019; United States, 2019; Uruguay, 2019). Uruguay has nine “general principles” by which it seeks to shape responsible AI (Uruguay, 2019, p. 9) while Japan has three “social principles” that form the approach of human-centered AI (Japan, 2019, p. 3). Germany advocates for an “Ethics by, in and for design” framework for AI and aims to create public-private auditing bodies for the assessment of algorithmic decision-making, as well as experimental sandboxes for the development of AI (Germany, 2018, pp. 16, 23, 26). Technologically advanced countries such as Singapore and Denmark are also keen to create ethical frameworks based on human-centered approach (Singapore, 2017 pp. 64–66), as well as responsible ethical frameworks for AI development and use (Denmark, 2019, p. 25).
However, as Jobin et al. (2019) note, the contextual expressions of these shared principles can vary substantially across different cultures. Indeed, some documents emphasize that the principles of AI development and use must reflect cultural values (Japan, 2019, pp. 59), alongside issues such as national security and civil rights (United States, 2019, pp. 3967–3971). Countries such as Germany and Denmark make a step further by emphasizing that the development and use of AI should respect democratic values and processes in order to advance the common good of the society (Germany, 2018, pp. 9,10; Denmark, 2019, pp. 8, 26). In countries such as Russia and China, the state is the key actor that governs AI by regulating and guiding its interactions through ethical rules, standards, and principles (Russia, 2019, pp. 16–18; China, 2017, p. 25).
Based on our investigation, it is possible to identify a common trait that all national strategies share: an emphasis on the importance of partnership between government, businesses and academia. The US for example, highlights the importance of advancing scientific progress, economic competitiveness and national security by retaining the lead in AI development both in academia and industry as well as on the federal levels (United States, 2019, pp. 3967–3968). Moreover, countries such as Singapore and Denmark are developing public-private partnerships in the field of data sharing by improving the accessibility of public datasets for private use (Denmark, 2019, p. 40; Singapore, 2017, p. 62), while the UK AI strategy emphasizes the role of AI Council, which gathers people from different sectors within government, business and academia (United Kingdom, 2018, p. 10).
According to our analysis, and confirmed by the relevant literature, most of the national AI strategies tend to lack concrete governance frameworks as well as measures to attain their stated goals (Fatima et al., 2020, pp. 180–182, 192; Saveliev and Zhurenkov, 2021, pp. 671–672). As Misuraca and Van Noordt (2020) note, national AI strategies often refer to an intent to create various ethical frameworks, principles and guidelines for the governance of AI in the public sector (2020, pp. 54–56), while the measures to create legislation and regulation are evidently more limited (2020, pp. 82–85). Indeed, despite the fact that AI is often framed as a novel strategic technology with potentially revolutionary implications for society, governments have been reluctant to draft specific AI related legislation and regulation (Misuraca and Van Noordt, 2020, pp. 82–83). Instead, most countries have been content to take a more “reflexive” approach on AI governance (Radu, 2021) by focusing on “soft” policy instruments such as training, education and awareness raising campaigns (Fatima et al., 2020; Misuraca and Van Noordt, 2020). The lack of strong legislative or regulatory approaches to AI (Wirtz et al., 2020, p. 826) is partially explained by the supposed unwillingness of governments to propose policy actions that could stifle the development of AI innovations (Radu, 2021, p. 188).
These developments mirrors Radu (2021) analysis arguing that governments have tended to prioritize an ethics orientation instead of pushing for strict regulatory approaches to AI governance. Ethics-oriented governance combined with soft policy instruments (Misuraca and Van Noordt, 2020, pp. 82–85) provide a flexible way of ensuring that the development of AI technology is not unduly regulated (Fatima et al., 2020, pp. 180–182, 192). Indeed, according to Radu (2021), the rhetoric and discourse dealing with governance in the national AI strategies has been impacted by the idea of voluntary self-regulation, which is the prominent approach to AI governance in the private sector. As a result, the focus of AI governance is oriented toward the creation of various ethical frameworks, guidelines, and codes of conduct.
The implementation of the ethics-oriented approach to AI governance is often supported by the creation of various national ethical Councils, Centers and Committees that monitor and analyze the global development of AI (Van Roy et al., 2021, pp. 15,16; Wirtz et al., 2019). Examples of these new institutions and bodies are on display in many of the AI strategies (Radu, 2021). Denmark has created a Data-Ethics Council (Denmark, 2019, p. 29), while the German federal government works with a Data Ethics Commission (Germany, 2018, p. 9). In Singapore, an industry-led Council assists both private and public sectors by providing guidance for the ethical utilization of AI (Singapore, 2017, p. 64). In a similar fashion, the UK advances the development and deployment of safe and ethical AI through its new data ethics bodies and councils (United Kingdom, 2018, p. 10).
These novel bodies consist of governmental, academic and industrial actors, which emphasizes both the increasing “hybridity” of AI governance (Radu, 2021), as well as the importance of Triple-Helix cooperation in AI governance. Hybrid governance describes the blurring boundaries between the various societal actors and their identities, while often favoring market-oriented approaches in governance (Radu, 2021, pp. 190,191). What is notable in this hybrid “Triple-Helix approach” to AI governance is the fact that it tends to overshadow the role of civil society and public engagement in governance.
Indeed, while the national AI strategies are rife with references to public engagement, there is little evidence of specific and concrete public engagement mechanisms and activities (Misuraca and Van Noordt, 2020, p. 82). Instead, the public is still often relegated to a mere passive user of AI technology or as the recipient of various government activities, communications and services. According to Wilson (2022, pp. 7,8), the role of society in AI governance can be accurately characterized as an “afterthought or a rhetorical gesture.” The National AI strategies are more focused on traditional issues such as economic and strategic competitiveness (Ulnicane et al., 2020, p. 161; Fatima et al., 2020, p. 241).
In summary, the national AI strategies consolidate an ethics approach toward AI governance, which is implemented through the cooperation between the public sector, industry and academia, in the practical form of various voluntary mechanisms such as guidelines, codes of conduct and best practices. What is lacking in these approaches to the governance of AI are more concrete mechanisms for public engagement and the inclusion of civil society.
Since the ethical and societal aspects of AI governance seem to have been strongly delegated to voluntary ethical approaches such as ethics guidelines developed by actors belonging to the triple helix model, in the next section we will analyze the ethical guidelines in order to evaluate their legitimacy and efficiency in regulating AI.
Besides and triggered by national strategies, ethics guidelines have been increasingly considered an efficient measure to prevent or reduce harms caused by AI because of their flexibility and potentially higher capillarity. In contrast, hard regulations can often be too rigid to deal with fast-paced technologies or they can represent an obstacle to technological and economic innovation. Floridi et al. (2018) have argued that regulating AI through soft measures can entail a double advantage, because it can prevent counterproductive unintended side-effects, while increasing the correspondence of AI systems to end-users needs.
Flexibility represents a core value in AI development due to the constantly evolving aspects of AI and the need to learn. If on the hand AI systems can autonomously improve their accuracy on the basis of their investigations, on the other hand they can also adapt or change after the interaction between end-users and third parties (Rochet and Tirole, 2003), which increases the unpredictability entailed in the overall ecosystem (Zittrain, 2006; Floridi et al., 2018). de Reuver et al. (2020) argue that knowledge about the medium and long-term effects of AI can never be fully predicted, suggesting that its epistemic unpredictability can potentially mutate into an ontological shift.
Although there are different interpretations about the exact reasons, it is evident that public and private bodies have tended to embrace an approach which advocates for self-regulatory and flexible approaches to the regulation of AI. According to the AI Index Report4, it is possible to identify over 100 ethics documents that have been published between 2015 and 2020, with a recently growing contribution of private organizations (Crawford, 2021, pp. 223–224).
Amongst the different actors in charge of implementing AI strategies, it is possible to identify a widespread consistency about the ethical relevance of AI technology and systems. At the public level, apart from the indications present in national strategies, some of the most significant examples are the requirements defined by the European Commission's “Ethics Guidelines for Trustworthy AI” (European Commission, 2020), and the European Parliament's resolution for AI, robotics and related technologies5 Research organizations like the Institute for Electrical and Electronics Engineers (IEEE) have started to design a set of indications for the “Ethically Aligned Design” of AI that can suit different designers (IEEE, 2019). Finally, the attempts made by private companies like Facebook6, Google7 and Microsoft8 or the multi-stakeholder forum like the Partnership on AI (PAI)9 confirm the great interest of private actors to ensure the ethically sound development of AI.
Although the number of documents is constantly increasing, and the different values might appear variegated at first, in their analyses of 84 documents, Jobin et al. (2019) have identified several overlaps in the list of suggested values and principles. The most common principles are those of transparency (73), justice (68) and non-maleficence (60); the less present ones are those of dignity (13) and solidarity (6). Hagendorff (2020) has highlighted that concerns about accountability, privacy and fairness are present in nearly 80% of AI ethical guidelines. Although the specific terms might be different, the concerns and principles seem to be very similar. For example, the EU High-Level Expert Group on the ethics of AI, have defined the profile of a trustworthy AI according to four main principles: respect for human autonomy, prevention of harm, fairness, and explicability. The OECD identifies five complementary principles and tools, namely sustainability, fairness, transparency, safety, and accountability. Google is promoting a design process where AI systems will avoid creating or reinforcing bias, be accountable and safe, implement privacy by design methods, and strive to be socially beneficial. Microsoft has chosen the six aspects of fairness, reliability and security, privacy, inclusiveness and transparency.
At a first glance these analyses and the related principles appear widely shareable, and it is difficult to deny the importance of ethics guidelines to regulate AI-based technologies given the general concerns about its actual and potential effects. However, scholars have started to share some doubts and criticisms about their explicit or subtle limitations.
One line of argument questions the limits inherent in the distance that a set of principles overlooks in terms of legitimacy and application. In other words, skepticism stems from the difficulties in translating principles and operational normative tools, such as transparency or fairness, into concrete measures.
The most immediate concern is that these guidelines are often hard to operationalize without the appropriate knowledge regarding context of application of AI (Haas and Gießler, 2020). Furthermore, engineers and designers might lack adequate training about the ethical aspects and implications of their work (Hagendorff, 2020). Ethical principles rarely come with detailed instructions. Crawford (2021) denounces the absence of professional governance structures or standards that can be followed.
Accordingly, scholars have highlighted that despite widespread agreement about the necessity to follow ethical regulations, measures like that of transparency are hardly present in the actual operationalization of AI processes of private companies (Article19, 2019). The reasons are identified again in the supposedly deliberate abstractness and the innate lack of accountability mechanisms in ethics (Hagendorff, 2020), which may contribute to maintaining the adoption of ethical principles as a means of avoiding public criticism (Wagner, 2018; Bietti, 2020).
The difficulty in applying ethical principles also raises the question about the identification and selection of some principles with respect to others. Indeed, the process that leads to the selection of one set of principles over another one is often unclear. However, it is possible to identify a widespread tendency in the fact that most of the ethics guidelines are the result of discussions among experts, involving also private actors (e.g., European Commission, 2020), with only a marginal role for civil society. One might wonder then about the ethical and democratic legitimacy of such choice of principles and the overall objectives that are meant to facilitate.
The practical suspicions about the distance between intended and effective objective of ethics guidelines leads us to a second line of arguments which addresses the social epistemology of the principles listed in these documents.
Beside the difficulty of translating aspects of justice into technical commands, the intrinsic abstractness of principles can overlook important contextual aspects as well. For instance, it is possible that in certain domains specific ethics values will be more salient than others or that the interpretation of values can differ according to the socio-cultural traits of a given context. Furthermore, the necessity to answer to some ethical principles can clash with other needs or moral be those framed in terms of the environment, labor rights, or equality.
This line of argument helps us by unveiling the epistemic assumptions at the basis of current ethics guidelines. A common trait of these documents seems to be the process of identification and selection of a set of potentially universal principles that everyone can agree upon without an adequate reflection on their translation. Operational normative principles such as transparency, accountability are conceptually hard to reject when evaluating the ethical respondence of technical processes. The overall underlying argument highlights a tendency to consider principles, normative tools and requirements independently from their context of application, overlooking that the implementation of AI takes place in different socio-technical environments.
AI, like all technologies, is not independent of its socio-cultural background. Instead, the development of its various features is dependent partially on different situated scenarios in which it is deployed. Even a technically refined design of AI can hardly determine all of its potential usages prior to deployment to an external environment. Because of this context-specificity of potential AI functions, the autonomy of self-learning technologies often develops in ways that are not entirely predictable.
A clear example of the gap between acceptable principles and their contextual acceptance is expressed by the most recurring principle of ethics guidelines for AI, transparency. Transparency appears intuitively beneficial for increasing ethical compliance as it allows external actors to evaluate the appropriateness of a process. However, Ananny and Crawford (2016) have argued that the operational normative principle of transparency is severely limited for regulating algorithmic systems. The implicit misunderstanding is that AI consists of an algorithm that can be evaluated on paper beforehand. However, an algorithm is often not a fixed, isolated code but a relational assemblage of human and non-human factors like institutions, norms, and practices (Ananny, 2016; Fazelpour and Lipton, 2020). The possibility to see parts of the process does not necessarily entail the capacity to understand how the system works, because learning requires a dynamic interaction with the systems within a specific environment (Resnick et al., 2000). On a similar note, one might also wonder how realistic is to implement mechanisms of accountability, another protagonist of ethics guidelines, in a process that is by definition handled by multiple actors over a long period of time, and with a significant role of autonomous algorithms for which current regulations is still under consideration.
To summarize, ethics guidelines can be considered beneficial for the fact that they are a clear cornerstone in seeking to provide answers and solutions to some of the main concerns about the unintended effects of AI systems. They address controversial aspects in a contextual and therefore flexible way. However, guidelines may fall short in doing so if their relationship with socio-technical environments is disregarded by the adoption of abstract principles that have not been made object of public scrutiny.
Firstly, from an ethical and epistemological point of view, the basic assumption that characterizes most ethics guidelines seems to concern an abstract or ideal individual able to implement a responsible model of AI thanks to access to information or through references to key aspects such as transparency and fairness. However, this vision of ethics appears reductive if put in relation to both a disruptive technological apparatus such as AI, and if placed in pluralistic value and normative contexts. The model of man underlying the ethics guidelines seems isolated and endowed with rational capacities that need only be activated. What is missing, in our opinion, are references to shared practices for understanding and selecting the values that are required to make AI a responsible technology.
Secondly, from a political and democratic perspective the lack of public scrutiny sheds a grim light on the legitimacy of the ethics guidelines because of the apparent arbitrariness of their selection. Accordingly, ethics guidelines for instance tend to leave aside the question of power in our societies and the consequent necessity to democratically open the space of discussion (Wagner, 2018; Bietti, 2020).
The conceptual framework through which we have carried out our analysis, places very strong emphasis on the connections between the ethical and political dimensions in the development of individual freedoms. The pragmatist model in fact indicates the possibility for an agent to realize his or herself in the ability that an institutional system offers to take an active part in social problem-solving practices. The basic assumption is that an individual does not live in isolation but, on the contrary, his or her successful self-realization occurs through his or her insertion within a social context where he or she can meaningfully take part in interactions of different kinds. In this sense, an ethical approach is to be understood as the possibility of interacting in democratic forms with other members of a given context. Democracy and ethics are in this sense closely intertwined in an attitude that John Dewey called a way of life.
In the next section we will define more in detail the features and the advantages of such theoretical framework.
Finding or creating an appropriate framework for AI governance is particularly challenging because of its nature as a strategic level multi- or omni-use technology (Saran et al., 2018) which raises issues in terms of their viability and adaptability in the face of rapid technological developments (Liu et al., 2020, p. 2). Moreover, the multi-level extension of AI as a national asset embedded in global interactions makes it comparable to revolutionary technologies like steam power and electricity (Trajtenberg, 2018).
Indeed, the issue of AI governance is conditioned by the broader public concerns about the positive benefits and risks of AI development and deployment. The role of governments in AI governance has focused on managing the potential negative externalities of AI development and deployment in the private sector in order to safeguard societal cohesion and values such as safety (Ulnicane et al., 2020, pp. 166–167). However, as noted earlier, most attention around AI development and deployment has focused on the “ethical framing” of AI technology (Ulnicane et al., 2020, p. 161), whereas less emphasis has been given on the “political” or “democratic” framings of AI. The problem in terms of governance is that ethical frameworks and principles lack mechanisms to implement societally desired normative goals (Ulnicane et al., 2021, p. 77), because they do not address fundamental questions of political power structures (Misuraca and Van Noordt, 2020, p. 50).
Another problem in AI governance has been the closed nature of the development of AI technology, which has resulted in power imbalances and various biases (Crawford and Whittaker, 2016). As mentioned earlier, rhetorical gestures and references toward public engagement are common, but there is little concrete evidence of mechanisms aiming for increased civil society participation in AI governance (Wilson, 2022). Instead, the public is demoted to a passive user of AI technology or as the recipient of various government activities, communications, and services, thus framing AI as a depoliticized technology.
Scholars such as Jasanoff (2016) deny the idea of AI as a non-political technology by arguing that technological solutions and choices are always political in nature. Indeed, there has been an increasing emphasis in the literature for substantial societal inquiry, public engagement practices, diverse stakeholder inclusion, participatory mechanisms in AI development and governance (Ulnicane et al., 2020, 2021; Wilson, 2022). During the last decade, governance frameworks have tended to assume an increasingly reflexive and collaborative posture. De Schutter and Lenoble (2010) for instance argue that governance models should tend toward learning opportunities for the actors involved. Learning could assume the form of benchmarking of best practices, monitoring and evaluation, participation and consultation notwithstanding that all these tools have become central aspects of main governance theories. Furthermore, given the fast pace at which technological progress and its transversal application to plural societies happen, it appears important to adopt a governance theoretical model that can benefit from a strong flexibility in terms of context and plurality of knowledge and values.
The integration of AI into society is a substantial societal and political question, which requires a thorough deliberation on the fundamental questions around AI. It is thus important to pay attention to the ways in which existing governance approaches provide room for or limit democratic and societal inputs to AI governance. In particular, long-term perspectives such as imaginaries and future narratives can either limit or broaden democratic participation. In order to take into account the role of civil society in AI development, the processes of AI development and deployment should be further democratized. The responsible development of AI governance requires more human agency and the democratization of the political imagination (Jasanoff, 2016). The inclusion of broader civil society would open the field of future imaginaries for more people, giving an opportunity to shape questions of power and influence. The focus should not be solely on the experts who are developing AI technology, but rather on the level of the end-users and citizens who have to deal with the impacts of AI systems (Veale, 2020). Framing AI technology through the lens of deliberative and democratic politics could provide new possibilities for a more societally oriented AI.
In order to translate the necessity of establishing a framework that can aspire to obtain a balance between the necessary efficiency of the process with its legitimacy, we believe that we need to adopt a methodology that can increase the role of society in a scientific way. A promising methodology to respond to these needs is the one inspired by John Dewey's pragmatism, which has recently regained momentum because of its advantages to deal with technological progress in democratic ways.
In order to overcome the limits embedded in current regulatory policies we need to adopt a theoretical model of governance that can overcome the limitation of current ethical guidelines, namely arbitrariness, abstractness, and power asymmetries by strengthening collaborative processes. If Stoker (1998) defines governance as an operation that could not be done by actors independently, we believe that governance is an operation that should not be done outside of a cooperative framework. Although we are aware of the existing asymmetries present in terms of knowledge, we argue that their current negative effects can be mitigated through the integration of processes of social experimentation in the overall regulatory framework of AI. In order to do so we need to establish a cooperative approach across different social groups based on reciprocal learning. A promising framework to translate these theoretical assumptions into a concrete model are those advocated in different ways by scholars orbiting around the pragmatist tradition.
In the Public and its Problems (1991) John Dewey portrayed a picture of the state of health of democracies in relation to the impact of science and technology that is extraordinarily relevant today. Concerned by the discredit experienced by democracy, mostly caused by the effects of technological and economic changes on society, he investigated the reasons and provided a methodological suggestion to address it. The pace at which economic and technological changes occurred in democratic societies was so fast that they lost sight of their societal nature, responding to technical or sectorial imperatives. In fact, in order for social changes, emerging in the technological or economic sector for instance, to assume the dignity of progresses, Dewey believed that they needed to represent an answer to societal claims and needs, and not just to fulfill criteria of technical efficiency or profitability. Accordingly, progress for Dewey was represented by the capacity of scientific and technological innovations to favor moral flourishing in individuals as part of a community.
The problem for Dewey was basically that of an epistemic reductionism that was instrumental to maintaining power asymmetries.
It is surprising how topical and similar Dewey's critique of the allegedly determinist approach to science is to the limits we have found in the current management of AI. Like the criticisms that have recently questioned the uptake of AI, Dewey identified the the abstractness of regulatory frameworks as the main cause responsible for incapacity of democratic systems to integrate technology through a social perspective. At the time when Dewey wrote his masterpiece, democracies were legitimized by references to abstract principles. If their moral value was hardly questionable, the possibility of their translation became more difficult in light of social contexts that were increasingly plural and multifaceted. As these principles were abstract and the object of what Dewey called a metaphysical approach, they were not questioned and often served to preserve power relations that despite their presumptions, were far from being universally acceptable. justification necessary to institutionalize any change in society, was identified in universal and supposedly neutral principles.
Dewey believed that such abstractness was partly instrumental in order to favor groups in power or political representatives. All fields of knowledge suffered, according to Dewey, from a technocratic or oligopolistic approach, whereby assumptions and policies were determined by a small group of people on the basis of sectorial references and interests. Abstractness and arbitrariness were then mutually reinforcing each other to the detriment of democratic engagement.
According to Dewey, this reductionist perspective affected the general understanding of democracy. If in terms of contents its principles were relegated to an undisputable set of moral ideals detached from social reality, methodologically, democratic processes were reduced to a simple exercise of expressing a preference during political elections. Neither was democracy implemented according to more significant interactions like deliberation, nor was it extended to domains other than politics like science, work or education.
For Dewey, the potential solution to this reductionism resided in science itself and the great contribution of science to humanity, namely in its experimental methodology. By experimenting and evaluating the validity of assumptions in practice, science had been able to generate enormous progress in natural sciences. However, this fertile methodology had not been integrated into other equally important fields of associated life. It was surprising for Dewey to notice that experimental approaches had not been applied to ethical theories or democratic approaches. He identified a double effect of such reductionism in terms of deliberation and cooperation. On the one hand, individuals did not develop the habit of deliberative exchanges, which reinforced social fragmentation, isolation and political mistrust. On the other hand, by overshadowing the advantages of societal cooperation, it weakened the awareness in the population that democratic scrutiny could and should have been extended to other fields of associated life.
Now, Dewey tells us that the situation does not have to remain as it is, but in order to change it, one cannot think of remaining within the same democratic method with its outdated and limited assumptions. Dewey responded to these ethical and epistemic deficits by proposing an experimentalist democratic model of a scientific and social nature. Dewey's aim was to propose a method that could overcome abstractness and consequently arbitrariness. The abstractness can be avoided through a method of inquiry that would put under scrutiny any assumptions emerging from social tensions. This in consequence would generate a facility, a habit to scrutinize different aspects of a particular phenomena together with other individuals through processes of deliberation and cooperation. Dewey was convinced that a more receptive attitude toward social perspectives would increasingly raise the common awareness of the interconnection and equal importance of the different social spheres.
The solution Dewey proposed was to explore ways to extend the scientific method of inquiry to all aspects of associated life in a democratic way. Associations are necessary to the functioning of societies. But, in order to make those associations meaningful for the fulfillment of moral principles proper of democratic systems, individuals should be able to develop an awareness about their purposes and their historical adequacy. This shift from having an impersonal role in the overall division of labor, to becoming aware of our own role as members of a community, should be established through reciprocal communication and publicity of discussion with scientists and policy-makers.
The overall result that can be obtained through these two aspects takes the form of that method of democratic experimentation known as social inquiry, which must extend its functions to all areas of associated life and thus take on the guise of an ethical way of life where everyone participates and contributes to common challenges (Honneth, 1998; Bernstein, 2010, ch. 3).
Accordingly, throughout the years Dewey formulated and described his method of social inquiry that can represent a fertile solution for abandoning what he defined objective approaches to ethics and reductive democratic theories in favor of democratic experimentalism (Dewey, 1990).
This method involves two main underlying factors. The first one is that all the theoretical aspects necessary to any systematic knowledge should be “shaped and tested as tool of inquiry.” The second factor is that policies for social action should “be treated as working hypotheses, not as programs to be rigidly adhered to and executed.” On the contrary, Dewey was firmly convinced that social policies should be subjected to constant scrutiny once applied and be easily changeable/adapted.
More specifically the method of social inquiry consists of different steps which should be understood as part of an on-going process of experimentation (Kolb, 2015). The triggering factor for social inquiry is the emerging incapacity of individuals to deal with aspects of their social interactions and the quest for a change (Mead, 2020). Usually, when this societal sufferance persists or is particularly widespread in society it implies that existing institutions are not perceived as able or willing to respond to these claims (Marres, 2007). Accordingly, the first step of a social inquiry is the recognition of a problem, its indeterminacy and the subsequent formation of a public requesting action.
The second step in the process of inquiry is the scientific formulation of the problem which requires an analysis of the different factors at stake, holistic and plural, and the potential causes of the problem through a communicative process amongst those who are concerned (Dewey, LW 12).
The third step is the formulation of hypotheses, ideas and ways forward to address the problem (LW 8, p. 203). Here the possibilities for the adequate formulation of a working hypothesis often depends on different scientific methodologies but also on the specific field of application. This part of the process builds on the necessary creativity of actors involved as one of the main factors of social inquiry.
The fourth step is the consideration about the context in which these actions are going be implemented. This is a crucial aspect of democratic experimentalism as it aims at integrating a whole set of conditions that are usually excluded by objective types of ethics. Although Dewey's method is strongly oriented toward change, he is firm in highlighting that experience does not happen in a void but in a context (Dewey, 1991; LW, 13, pp. 1–62).
Every experience is the combination of psychological and social factors that are specific to a context. And the context is also the result of a series of values, standards and cultural features that we have inherited, in which we were raised, and that contribute to form our habits. As argued by Cefaï (2020), ethics entertains a deep relation with psychology and sociology for it addresses the relation between the character, habits, motivations and dispositions of individuals, as well as the social and technological conditions in which they live. This particular attention to experience as experience of life implies that in order to be able to set forth viable and meaningful solutions, the processes of reflection and deliberation cannot prescind from existing dynamics of power as well as a realistic understanding of feasible solutions (Mead, 2020).
The learning aspect that this step entails for individuals is expressed by the pragmatists belief that personal identity is not innate but the result of a socialization (Zimmermann, 2020). By reflecting about the context in which they are called to act upon the individuals can also learn more about themselves as part of a society. By wanting and being able to transform society, individuals also transform themselves (Cefaï, 2020).
The last step of this process of social inquiry focuses on the active contextualization of some actions that will provide the material for the evaluation of previous hypotheses. Integration into a specific context is the only way to understand if specific policies or action function for the purposes for which they have been selected (Lenoble and Maesschalck, 2016)
This methodology of experimentation depends on three main assumptions that it develops through a social, experimental and public framework. First, it must be social in the sense that different drivers, different actors as well as different aspects of experience can be considered, confronted and valued in the analysis and evaluation of outcomes. Plurality, diversity and complexity should be integrated under the common social objective of all modes of organization, which was for Dewey the core moral aspect of democratic experimentation. Second, it must be experimental in nature, meaning that the whole method should be open for scrutiny without predetermining its assumptions or its outcomes (LW 11, p. 292–93; cp. LW 8, p. 206). Third, it should be public in its outcomes enabling other members of society to learn and to build a sense of trust and acceptance of the process. According to Dewey by assuming these three conditions we can obtain for example the following advantages:
- We can raise the awareness about the interconnections across different modes of associations that a just division of labor should entail in terms of objectives.
- We can unveil the asymmetries of power present in society
- We can trigger a process of learning that can give back to individuals a meaning of being part of a society by understanding their role and value.
- We can increase the democratic level of our societies by strengthening the involved participants in the process, but also by questioning democracy as an experimental process of social inquiry.
Although this theoretical model is experimental and needs to be operationalized to verify its value, it is possible to identify an increasing use of approaches based on this model that appear promising. Examples of the application of “social inquiries” can be found in many fields where research and innovation are intertwined with ethical and social aspects10.
One of the recognized problems in AI development and deployment is the relatively narrow nature of the process. Because the national AI strategies are largely shaped by geopolitical, economic and scientific-technological considerations, they tend to emphasize the importance of Triple-Helix partnerships between government, industry and academia, as well as hybrid governance approaches in developing and deploying AI systems, whereas the role of civil society stakeholders is often overlooked. These “locked-in” and “path-dependent” visions of AI utilization can limit the space for democratic imagination of AI futures (Bareis and Katzenbach, 2021, pp. 21,22). Indeed, the public has not been able to actively engage with the design, development and deployment of AI technology (Crawford and Whittaker, 2016). On the contrary, the national strategies tend to emphasize the imperative of adapting societies to the needs of the new AI-driven economy. Similarly, the issue of societal trust is often framed in the strategies as an instrumental value that ensures the integration of AI systems into society, and not its precondition. In sum, the current framing of AI technology narrows the discussion around AI toward technical and technocratic fixes (Misuraca and Van Noordt, 2020, pp. 49–50) and draws attention away from existing structural problems and their root causes, which often have a profound effect on the development and use of AI (Ulnicane et al., 2020, p. 171).
The general reliance on ethics guidelines does not appear sufficient to deal with the significant impacts that AI-based technologies will generate. Ethics guidelines are built on a sort of epistemological optimism that overlooks a series of contextual aspects that are fundamental in dealing with the dynamic nature of AI. Furthermore, ethics guidelines tend to divert the attention from the necessity to form and include different publics in the definition of AI's role for our future societies.
The solution proposed by scholars like Dewey has the double advantage of representing a powerful tool to diagnose the health of our democratic systems in addition to being able to hold together the different societal perspectives concerning the emergence of disruptive technologies such as AI. In this sense his criticism about a reductive and metaphysical perspective on the ethos of democratic life appears highly relevant when applied to the analysis of the governance of AI. Moreover, it also represents a fruitful approach toward addressing the limits of current theoretical models by offering an empirical and open methodology, which aims to strengthen the role of society in shaping our future.
Over the past decade AI has become one of the most important but also one of the most controversial technologies due to the unpredictability of its developments and applications. It is hard to find a sector today where AI-systems of some variety would not represent the main technical tool for data collection and management. Its increasing importance has led various public and private actors to define strategic guidelines for its development in the space of a few years.
Among the various aspects that governments seem to have considered, there is a strong preponderance of the economic and geopolitical imperatives. However, awareness of the potential side effects of AI has forced the various public and private bodies to adopt ethical guidelines to prevent or mitigate the negative externalities of AI. In this sense, the production of hundreds of AI-related policy documents in a short period of time testifies to this urgency but also to the fragmentation and the absence of larger democratic processes in the selection of ethical principles. The evident absence of concrete references to the role of civil society in the discussion on the objectives and necessary limits of AI raises questions in terms of the adequacy of these ethical guidelines. Indeed, although most of the ethical principles are difficult to contest as such, they can appear abstract if not sometimes arbitrary when they are not supported by broader deliberations. More importantly, the application of ethical principles in concrete contexts is certainly more difficult than is often presented to be the case. This also raises questions about the effectiveness of ethical guidelines in terms of their ability to provide responsive action and solutions to various social needs. In other words, as AI continues to have a radical influence on the development of our democratic societies, it is important to point out the lack of broader participatory processes around AI governance.
In this sense, the purpose of this paper was twofold. On the one hand, we highlighted the limits of the current approaches to AI governance by showing their distance from the public and society at large. On the other hand, we provided a conceptual framework that could go beyond these limits without losing the economic and strategic potential that AI represents for any socio-political context. Through the theoretical model developed by John Dewey, we have highlighted the benefits of a participatory and experimental approach to the governance of AI. Accordingly, the innovativeness of our theoretical model resides in its suggestion to extend an experimental method to the domains of politics and ethics of technology. In so doing, our approach offers the advantage of strengthening the legitimacy of AI-related policies but also their effectiveness because they are enriched by the cement that results from increased interaction with the civil society. Finally, our proposal can contribute to the habit of a broad dialogue among citizens, thus improving the health of democratic systems.
The original contributions presented in the study are included in the article/supplementary material, further inquiries can be directed to the corresponding author/s.
All authors listed have made a substantial, direct, and intellectual contribution to the work and approved it for publication. The order of the writers reflects the relative contribution of the authors in the text.
The RG acknowledges that the research has received funding from the European Union's Horizon 2020 research and innovation programme under Grant Agreement 962547. The SL and MN wish to acknowledge the project Ethical AI for the Governance of the Society (ETAIROS, grant number 327356), funded by the Strategic Research Council at the Academy of Finland.
Any opinions, findings, and conclusions or recommendations expressed in this material are those of the authors and do not necessarily reflect the views of the funding agencies.
The authors declare that the research was conducted in the absence of any commercial or financial relationships that could be construed as a potential conflict of interest.
All claims expressed in this article are solely those of the authors and do not necessarily represent those of their affiliated organizations, or those of the publisher, the editors and the reviewers. Any product that may be evaluated in this article, or claim that may be made by its manufacturer, is not guaranteed or endorsed by the publisher.
1. ^https://eur-lex.europa.eu/legal-content/EN/TXT/?uri=CELEX:52021PC0206
2. ^World Economic Outlook Database, IMF, 2020. https://www.imf.org/en/Publications/WEO/weo-database/2020/April.
3. ^Digital Adoption Index, World Bank, 2016. https://www.worldbank.org/en/publication/wdr2016/Digital-Adoption-Index.
4. ^https://aiindex.stanford.edu/wp-content/uploads/2021/03/2021-AI-Index-Report-_Chapter-5.pdf
5. ^https://www.europarl.europa.eu/doceo/document/TA-9-2020-0275_EN.html
6. ^https://ai.facebook.com/blog/facebooks-five-pillars-of-responsible-ai/
7. ^https://ai.google/principles
8. ^https://www.microsoft.com/en-us/ai/responsible-ai?activetab=pivot1%3aprimaryr6
9. ^https://partnershiponai.org
10. ^For an example of the application of such methodology, see https://newhorrizon.eu/social-labs/.
Allen, G (2019). Understanding China's AI Strategy: Clues to Chinese Strategic Thinking on Artificial Intelligence and National Security. Center for a New American Security. Available online at: https://s3.us-east-1.amazonaws.com/files.cnas.org/documents/CNAS-Understanding-Chinas-AI-Strategy-Gregory-C.-Allen-FINAL-2.15.19.pdf?mtime=20190215104041&focal=none
Ananny, M (2016). Towards an ethics of algorithms: convening, observation, probability, and timeliness. Sci. Technol. Human Values 41, 93-117. doi: 10.1177/0162243915606523
Ananny, M., and Crawford, K. (2016). Seeing without knowing: Limitations of the transparency ideal and its application to algorithmic accountability. New Media Soc. 20, 973–989. doi: 10.1177/1461444816676645
Article19 (2019). Governance With Teeth: How Human Rights Can Strengthen FAT and Ethics Initiatives on Artificial Intelligence. Available online at: https://www.article19.org/resources/governance-with-teeth-how-human-rights-can-strengthen-fat-and-ethics-initiatives-on-artificial-intelligence/ (accessed February 1, 2022).
Bannister, F., and Connolly, R. (2020). Administration by algorithm: a risk management framework. Inform. Polity, 25, 471–490. doi: 10.3233/IP-200249
Bareis, J., and Katzenbach, C. (2021). Talking AI into being: the narratives and imaginaries of national AI strategies and their performative politics. Sci. Technol. Human Values. 21, 7. doi: 10.1177/01622439211030007
Bennett, B., and Daly, A. (2020). Recognising rights for robots: Can we? Will we? Should we? Law Innov. Technol. 12, 60–80. doi: 10.1080/17579961.2020.1727063
Benthall, S., and Hynes, B. (2019). FAT* '19: Proceedings of the Conference on Fairness, Accountability, and Transparency, January 2019, pp. 289–298
Berryhill, J., Heang, K. K., Clogher, R., and McBride, K. (2019). “Hello, World: Artificial intelligence and its use in the public sector,” in OECD Working Papers on Public Governance (Paris: OECD Publishing), p. 36.
Bietti, E (2020). “From ethics washing to ethics bashing,” in FAT* '20: Proceedings of the 2020 Conference on Fairness, Accountability, and Transparency, (Spain: Barcelona), p. 10.
Bradley, C., and Wingfield, R. (2020). National Artificial Intelligence Strategies and Human Rights: A Review. Global Digital Policy Incubator. Available online at: https://www.gp-digital.org/wp-content/uploads/2020/04/National-Artifical-Intelligence-Strategies-and-Human-Rights–A-Review_April2020.pdf (accessed February 1, 2022).
Buhmann, A., and Fieseler, C. (2021). Towards a deliberative framework for responsible innovation in artificial intelligence. Technol. Society 64, 101475. doi: 10.1016/j.techsoc.2020.101475
Calvo R. A Peters D Vold K Ryan R. M. (2020). "Supporting human autonomy in AI systems: A framework for ethical enquiry," in Ethics of Digital Well-Being: A Multidisciplinary Approach, eds C. Burr and L. Floridi (Cham: Springer), 31–54. doi: 10.1007/978-3-030-50585-1_2
Campolo, A., and Crawford, K. (2020). Enchanted determinism: power without responsibility in artificial intelligence. Engaging Sci. Technol. Soc. 6, 1–19. doi: 10.17351/ests2020.277
Cath, C. . (2018). Governing artificial intelligence: ethical, legal and technical opportunities and challenges. Philosophic. Trans. 18, 376. doi: 10.1098/rsta.2018.0080
Cath, C., Wachter, S., Mittelstadt, B., Taddeo, M., and Floridi, L. (2018). Artificial Intelligence and the ‘good society': The US, EU, and UK approach. Sci. Eng. Ethics 24, 505–528. doi: 10.1007/s11948-017-9901-7
Cave, S., and ÓhÉigeartaigh, S. (2018). “An AI race for strategic advantage: rhetoric and risks,” in Proceedings of the 2018 AAAI/ACM Conference on AI, Ethics, and Society (AIES '18) (New York, NY: Association for Computing Machinery), pp. 36–40.
Cefaï, D (2020). La naissance de l'experimentation démocratique quelques hypothèses de travail du pragmatisme. Pragmata 3, 270–355. Available online at: https://revuepragmata.files.wordpress.com/2021/04/pragmata-2020-3-7-cefai.pdf (accessed February 1, 2022).
China (2017). “Next Generation AI Development Plan,” in State Council of the People's Republic of China. Available online at: https://chinacopyrightandmedia.wordpress.com/2017/07/20/a-next-generation-artificial-intelligence-development-plan/ (accessed February 1, 2022).
Clarke, R (2019). Regulatory alternatives for AI. Comput. Law Secur. Rev. 35, 398–409. doi: 10.1016/j.clsr.2019.04.008
Crawford, K., and Whittaker, M. (2016). The AI Now Report. The Social and Economic Implications of Artificial Intelligence Technologies in the Near-Term. New York, NY: AI Now Institute.
Dafoe, A (2018). AI Governance: A Research Agenda. Governance of AI Program. Future of Humanity Institute. University of Oxford: Oxford, UK. Available online at: https://www.fhi.ox.ac.uk/wp-content/uploads/GovAI-Agenda.pdf (accessed December 1, 2021).
de Almeida, P. G. R., dos Santos, C. D., and Farias, J. S. (2021). Artificial Intelligence Regulation: a framework for governance. Ethics Inform. Technol. 23, 505–525. doi: 10.1007/s10676-021-09593-z
de Reuver, M., van Wynsberghe, A., Janssen, M., and van de Poel, I. (2020). Digital platforms and responsible innovation: expanding value sensitive design to overcome ontological uncertainty. Ethics Inform. Technol. 22, 257–267. doi: 10.1007/s10676-020-09537-z
De Schutter, O., and Lenoble, J. (2010). Reflexive Governance: Redefining the Public Interest in a Pluralistic World. Oxford: Hart.
de Sousa, W. G., de Melo, E. R. P., De Souza Bermejo, P. H., Sousa Farias, R. A., and Gomes, A. O. (2019). How and where is artificial intelligence in the public sector going? A literature review and research agenda. Govern. Inform. Q. 36, 0740-624. doi: 10.1016/j.giq.2019.07.004
Delacroix, S., and Wagner, B. (2021). Constructing a mutually supportive interface between ethics and regulation. Comput. Law Secur. Rev. 40, 520. doi: 10.1016/j.clsr.2020.105520
Denmark (2019). “National strategy for artificial intelligence,” in Ministry of Finance and Ministry of Industry, Business and Financial Affairs. Available online at: https://en.digst.dk/media/19337/305755_gb_version_final-a.pdf (accessed February 1, 2022).
Dewey, J (1990). The Later Works of John Dewey, 1924–1953. Carbondale, Ill.: Southern Illinois University Press.
Dexe, J., and Franke, U. (2020). Nordic lights? National AI policies for doing well by doing good. J. Cyber Policy 5, 332–349. doi: 10.1080/23738871.2020.1856160
Dignam, A (2020). Artificial intelligence, tech corporate governance and the public interest regulatory response. Cambridge J. Reg. Econ. Soc. 13, 37–54. doi: 10.1093/cjres/rsaa002
Dutton, T (2018). Building an AI world: Report on national and regional strategies. CIFAR. Available online at: https://www.cifar.ca/cifarnews/2018/12/06/building-an-ai-world-report-on-national-and-regional-ai-strategies (accessed December 6, 2018).
European Commission (2020). High-Level Expert Group on AI. Ethics Guidelines for Trustworthy AI. Brussels: Shaping Europe's digital future. Available online at: https://ec.europa.eu/digital-single-market/en/news/ethics-guidelines-trustworthy-ai (accessed February 1, 2022).
Fatima, S., Desouza, K. C., and Dawson, G. S. (2020). National strategic artificial intelligence plans: A multi-dimensional analysis. Econ. Anal. Policy 67, 178–194. doi: 10.1016/j.eap.2020.07.008
Fatima, S., Desouza, K. C., Denford, J. S., and Dawson, G. S. (2021). What explains governments interest in artificial intelligence? A signaling theory approach. Econ. Anal. Polic. 71, 238–254. doi: 10.1016/j.eap.2021.05.001
Fazelpour, S., and Lipton, Z. C. (2020). Algorithmic Fairness From a Non-Ideal Perspective. London: Scopus, pp. 57–63.
Floridi, L., Cowls, J., Beltrametti, M., Chatila, R., Chazerand, P., Dignum, V., et al. (2018). AI4People— An ethical framework for a good AI society: opportunities, risks, principles, and recommendations. Minds Mach. 28, 689–707. doi: 10.1007/s11023-018-9482-5
Future of Life (2020). Future of Life. National and International AI Strategies. Future of Life Institute. Available online at: https://futureoflife.org/national-international-ai-strategies/
Germany (2018). “Federal Government's Artificial Intelligence Strategy,” in Federal Ministry for Economic Affairs and Energy, Federal Ministry of Education and Research and Federal Ministry of Labour and Social Affairs. Available online at: https://www.de.digital/DIGITAL/Redaktion/EN/Standardartikel/artificial-intelligence-strategy.html (accessed February 1, 2022).
Green, B., and Chen, Y. (2019). “Disparate interactions: an algorithm-in-the-loop analysis of fairness in risk assessments,” in Proceedings of the Conference on Fairness, Accountability, and Transparency—FAT*' 19, 90–99. Atlanta, GA: ACM Press.
Haas, L., and Gießler, S. (2020). AI Ethics Global Inventory. Available online at: https://inventory.algorithmwatch.org/
Hagendorff, T (2020). The Ethics of AI Ethics: an evaluation of guidelines. Minds Mach. 30, 99–120, doi: 10.1007/s11023-020-09517-8
Hague, D (2019). Benefits, pitfalls, and potential bias in health care AI. C Med J. 80, 219–223. doi: 10.18043/ncm.80.4.219
Honneth, A (1998). Democracy as reflexive cooperation. Politic. Theory 26, 763–783. doi: 10.1177/0090591798026006001
IEEE. (2019) “Ethically aligned design,” in A Vision for Prioritizing Human Well-being with Autonomous and Intelligent Systems (IEEE). Available online at: https://standards.ieee.org/content/dam/ieee-standards/standards/web/documents/other/ead1e.pdf (accessed February 2 2022)
Japan (2019). “AI Strategy 2019”. Artificial Intelligence Technology Strategy Council. Available online at: https://www.kantei.go.jp/jp/singi/ai_senryaku/pdf/aistratagy2019en.pdf (accessed February 1, 2022).
Jasanoff, S. . (2016). The Ethics of Invention: Technology and the Human Future. New York: W.W. Norton.
Jasanoff, S., and Kim, S-H. (2009). Containing the atom: sociotechnical imaginaries and nuclear power in the United States and South Korea. Minerva 47, 119. doi: 10.1007/s11024-009-9124-4
Jobin, A., Ienca, M., and Vayena, E. (2019). The global landscape of AI ethics guidelines. Nat. Mach. Intell. 1, 389–399. doi: 10.1038/s42256-019-0088-2
Johnson, R., Stone, D., and Lukaszewski, K. (2020). The benefits of eHRM and AI for talent acquisition. J. Tour. Fut. 7, 13 doi: 10.1108/JTF-02-2020-0013
Koehler, J (2018). Business Process Innovation with Artificial Intelligence: Levering Benefits and Controlling Operational Risks. Euro. Bus. Manage. 4, 55–66. doi: 10.11648/j.ebm.20180402.12
Kolb, D. A (2015). “Experiential learning,” in Experience as the Source of Learning and Development, 2nd Edn (Upper Saddle River, NJ: Pearson Education).
Kuziemski, M., and Misuraca, G. (2020). AI governance in the public sector: three tales from the frontiers of automated decision-making in democratic settings. Telecommun. Policy, 44, 76. doi: 10.1016/j.telpol.2020.101976
Lazic, L (2019). “Benefit from AI in cybersecurity,” in The 11th International Conference on Business Information Security (BISEC-2019).
Lenoble, J., and Maesschalck, M. (2016). Democracy, Law and Governance. London and New York: Routledge.
Liu, H. Y., and Maas, M. M. (2021). ‘Solving for X?' Towards a problem-finding framework to ground long-term governance strategies for artificial intelligence. Futures 21, 126. doi: 10.1016/j.futures.2020.102672
Liu, H. Y., Maas, M. M., Danaher, J., Scarcella, L., Lexer, M., and Van Rompaey, L. (2020). Artificial intelligence and legal disruption: a new model for analysis. Law Innov. Technol. 12, 205–258. doi: 10.1080/17579961.2020.1815402
Makridakis, S (2017). The forthcoming Artificial Intelligence (AI) revolution: its impact on society and firms. Futures 90, 46–60. doi: 10.1016/j.futures.2017.03.006
Marres, N (2007). The issues deserve more credit: Pragmatist contributions to the study of public involvement in controversy. Soc. Stud. Sci. 37, 759–780. doi: 10.1177/0306312706077367
Mead, G. H (2020). L'Hypothèse de travail dans la réforme sociale (1899). Pragmata 3, 356–362. Available online at: https://revuepragmata.files.wordpress.com/2021/04/pragmata-2020-3-8-mead.pdf (accessed February 1, 2022).
Misuraca, G., and Van Noordt, C. (2020). AI Watch - Artificial Intelligence in public services, EUR 30255 EN. Luxembourg: Publications Office of the European Union.
O'Neil, C (2016). Weapons of Math Destruction, How Big Data Increases Inequality and Threatens Democracy. New York, NY: Broadway Books,.
OPSI (2020). OPSI AI strategies, and public sector components. Obs. Public Sect. Innov. 2020, 12. Available online at: https://oecd.ai/en/ (accessed May 5, 2022).
Ostrom, E (2005). Understanding Institutional Diversity. Princeton, NJ: Princeton University Press. Paper presented at the international conference on electronic government, San Benedetto del Tronto, Italy.
Paltieli, G (2021). The political imaginary of National AI Strategies. AI and Soc. 21, 258. doi: 10.1007/s00146-021-01258-1
Radu, R (2021). Steering the governance of artificial intelligence: national strategies in perspective. Policy Soc. 40, 178–193. doi: 10.1080/14494035.2021.1929728
Raji, I. D., Gebru, T., Mitchell, M., Buolamwini, J., Lee, J., and Emily, D. (2020). “Saving face: investigating the ethical concerns of facial recognition auditing,” in Proceedings of the 2020 AAAI/ACM Conference on AI, Ethics, and Society (New York, NY: AIES '20).
Resnick, M., Berg, R., and Eisenberg, M. (2000). Beyond black boxes: bringing transparency and aesthetics back to scientific investigation. J. Learn. Sci. 9, 7–30. doi: 10.1207/s15327809jls0901_3
Rochet, J. C., and Tirole, J. (2003). Platform competition in two-sided markets. J. Euro. Econ. Assoc. 1, 990–1029. doi: 10.1162/154247603322493212
Russia (2019). “Decree of the President of the Russian Federation on the Development of Artificial Intelligence in the Russian Federation”. President of the Russian Federation. Available online at: https://cset.georgetown.edu/publication/decree-of-the-president-of-the-russian-federation-on-the-development-of-artificial-intelligence-in-the-russian-federation/ (accessed February 1, 2022).
Saariluoma, P., and Salo-Pöntinen, H. (2021). “Lost people: how national ai-strategies paying attention to users,” in Human Interaction, Emerging Technologies and Future Applications IV. IHIET-AI 2021. Advances in Intelligent Systems and Computing, eds Ahram T., Taiar R., Groff F. (Cham: Springer), p. 75.
Saran, S., Natarajan, N., and Srikumar, M. (2018). In Pursuit of Autonomy: AI and National Strategies. November 2018. Observer Research Foundation. Available online at: https://orfonline.org/wp-content/uploads/2018/11/Ai_Book.pdf
Saveliev, A., and Zhurenkov, D. (2021). Artificial intelligence and social responsibility: the case of the artificial intelligence strategies in the United States, Russia, and China. Kybernetes 50, 656–675. doi: 10.1108/K-01-2020-0060
Sigfrids, A., Nieminen, M., Leikas, J., and Pikkuaho, P. (2022). How should public administrations support ethical and sustainable development and use of Artificial Intelligence? A systematic review of proposals for developing governance of AI. Unpublished manuscript.
Singapore (2017). “AI Singapore”. Smart Nation and Digital Government Office. Available online at: https://www.nrf.gov.sg/programmes/artificial-intelligence-r-d-programme (accessed February 1, 2022).
Smith, M (2018). Getting value from artificial intelligence in agriculture. Anim. Product. Sci. 60, 46–54, doi: 10.1071/AN18522
Stix, C (2021). Actionable principles for artificial intelligence policy: three pathways. Sci. Eng. Ethics 27, 1–17. doi: 10.1007/s11948-020-00277-3
Stoker, G (1998). Governance as theory: five propositions. Int. Soc. Sci. J. 50, 17–28. doi: 10.1111/1468-2451.00106
Sun, T. Q., and Medaglia, R. (2019). Mapping the challenges of Artificial Intelligence in the public sector: evidence from public healthcare. Gov. Inform. Q. 36, 368–383. doi: 10.1016/j.giq.2018.09.008
Taeihagh A. (2021): Governance of artificial intelligence. Policy Soc. 40, 137–157, 10.1080/14494035.2021.1928377.
Toll, D., Lindgren, I., Melin, U., and Madsen, C.Ø. (2019). Artificial Intelligence in Swedish Policies: Values, Benefits, Considerations and Risks.
Trajtenberg, M (2018). AI as the Next Gpt: A Political-Economy Perspective. NBER Working Paper No. w24245. Available online at: http://www.nber.org/papers/w24245 (accessed February 1, 2022).
Tsamados, A., Aggarwal, N., Cowls, J., Morley, J., Roberts, H., Taddeo, M., et al. (2021). The ethics of algorithms: key problems and solutions. AI Soc. 21, 302. doi: 10.2139/ssrn.3662302
Ulnicane, I., Eke, D. O., Knight, W., Ogoh, G., and Stahl, B. C. (2021). Good governance as a response to discontents? Déjà vu, or lessons for AI from other emerging technologies. Interdisciplin. Sci. Rev. 46, 71–93. doi: 10.1080/03080188.2020.1840220
Ulnicane, I., Knight, W., Leach, T., Stahl, B. C., and Wanjiku, W. G. (2020). Framing governance for a contested emerging technology: insights from AI policy. Policy Soc. 40, 158–177. doi: 10.1080/14494035.2020.1855800
United Kingdom (2018). “Artificial intelligence sector deal,” in Office for Artificial Intelligence; Department for Business, Energy and Industrial Strategy and Department for Digital, Culture, Media and Sport. Available online at: https://www.gov.uk/government/publications/artificial-intelligence-sector-deal/ai-sector-deal (accessed February 1, 2022).
United States (2019). “Executive order on maintaining American leadership in artificial intelligence,” in US President Donald Trump. Available online at: https://trumpwhitehouse.archives.gov/presidential-actions/executive-order-maintaining-american-leadership-artificial-intelligence/ (accessed February 1, 2022).
Uruguay (2019). “Artificial intelligence strategy for the digital government,” in Office of the President. Available online at: https://www.gub.uy/agencia-gobierno-electronico-sociedad-informacion-conocimiento/comunicacion/publicaciones/ia-strategy-english-version/ia-strategy-english-version (accessed February 1, 2022).
Van Roy, V., Rossetti, F., Perset, K., and Galindo-Romero, L. (2021). AI Watch - National strategies on Artificial Intelligence: A European perspective. Luxembourg: EUR 30745 EN, Publications Office of the European Union.
Veale, M (2020). A Critical Take on the Policy Recommendations of the EU High-Level Expert Group on Artificial Intelligence. Europ. J. Risk Regulat. 11, 24. doi: 10.1017/err.2019.65
Viscusi, G., Rusu, A., and Florin, M.-V. (2020). Public strategies for artificial intelligence: which value drivers? Computer 53, 38–46, doi: 10.1109/MC.2020.2995517
Wachter, S., Mittelstadt, B., and Floridi, L. (2017). Why a right to explanation of automated decision-making does not exist in the General Data Protection Regulation. Int. Data Privacy Law 7, 76–99. doi: 10.1093/idpl/ipx005
Wagner, B (2018). “Ethics as an Escape from Regulation: From ethics-washing to ethics-shopping?” in Being Profiled. Cogitas ergo sum, ed M. Hildebrandt (Amsterdam: Amsterdam University Press), p. 18.
Wallach, W., and Marchant, G. E. (2018). An agile ethical/legal model for the international and national governance of AI and robotics. Assoc. Adv. Artific. Intell. 18, 77. Available online at: https://www.aies-conference.com/2018/contents/papers/main/AIES_2018_paper_77.pdf (accessed February 1, 2022).
Wilson, C (2022). Public engagement and AI: a values analysis of national strategies. Govern. Inform. Q. 39, 52. doi: 10.1016/j.giq.2021.101652
Winfield, A. F., and Jirotka, M. (2018). Ethical governance is essential to building trust in robotics and artificial intelligence systems. Philosophic. Trans. Royal Soc. A Math. Physic. Eng. Sci. 376, 20180085. doi: 10.1098/rsta.2018.0085
Wirtz, B. W., Weyerer, J. C., and Geyer, C. (2019). Artificial intelligence and the public sector-applications and challenges. Int. J. Public Administr. 42, 596–615. doi: 10.1080/01900692.2018.1498103
Wirtz, B. W., Weyerer, J. C., and Sturm, B. J. (2020). The dark sides of artificial intelligence: an integrated AI governance framework for public administration. Int. J. Public Administr. 43, 818–829. doi: 10.1080/01900692.2020.1749851
Yeasmin, S (2019). Benefits of Artificial Intelligence in Medicine,” in 2019 2nd International Conference on Computer Applications and Information Security (ICCAIS), pp. 1–6.
Yeung, K., Howes, A., and Pogrebna, G. (2019). “AI governance by human rights-centred design, deliberation and oversight: an end to ethics washing,” in The Oxford Handbook of AI Ethics, eds Dubber, M., Pasquale, F. (New York, NY: Oxford University Press).
Yu, M., and Du, G. (2019). Why are Chinese courts turning to AI?' The Diplomat. 19 January 2019. Available online at: https://thediplomat.com/2019/01/why-are-chinese-courts-turning-to-ai/
Zhang, D., Mishra, S., Brynjolfsson, E., Etchemendy, J., Ganguli, D., Grosz, B., et al. (2021). “The AI Index 2021 annual report,” in AI Index Steering Committee, Human-Centered AI Institute, (Stanford, CA: Stanford University). Available online at: https://aiindex.stanford.edu/report/ (accessed February 1, 2022).
Zimmermann, B (2020). Capabilités et développement de l'individualité. De Dewey à Sen, la voie d'un pragmatisme critique. Pragmata, 3, p. 134-175. https://revuepragmata.files.wordpress.com/2021/04/pragmata-2020-3-4-zimmermann.pdf
Zittrain, J. L (2006). The Generative Internet. Harvard Law Rev. 1974, 119. doi: 10.1145/1435417.1435426
Keywords: Artificial Intelligence, governance, ethics, democracy—citizen, National Strategy for Artificial Intelligence, pragmatism
Citation: Gianni R, Lehtinen S and Nieminen M (2022) Governance of Responsible AI: From Ethical Guidelines to Cooperative Policies. Front. Comput. Sci. 4:873437. doi: 10.3389/fcomp.2022.873437
Received: 10 February 2022; Accepted: 26 April 2022;
Published: 24 May 2022.
Edited by:
Kostas Karpouzis, Panteion University, GreeceReviewed by:
Stavroula Tsinorema, University of Crete, GreeceCopyright © 2022 Gianni, Lehtinen and Nieminen. This is an open-access article distributed under the terms of the Creative Commons Attribution License (CC BY). The use, distribution or reproduction in other forums is permitted, provided the original author(s) and the copyright owner(s) are credited and that the original publication in this journal is cited, in accordance with accepted academic practice. No use, distribution or reproduction is permitted which does not comply with these terms.
*Correspondence: Robert Gianni, ci5naWFubmlAbWFhc3RyaWNodHVuaXZlcnNpdHkubmw=
Disclaimer: All claims expressed in this article are solely those of the authors and do not necessarily represent those of their affiliated organizations, or those of the publisher, the editors and the reviewers. Any product that may be evaluated in this article or claim that may be made by its manufacturer is not guaranteed or endorsed by the publisher.
Research integrity at Frontiers
Learn more about the work of our research integrity team to safeguard the quality of each article we publish.