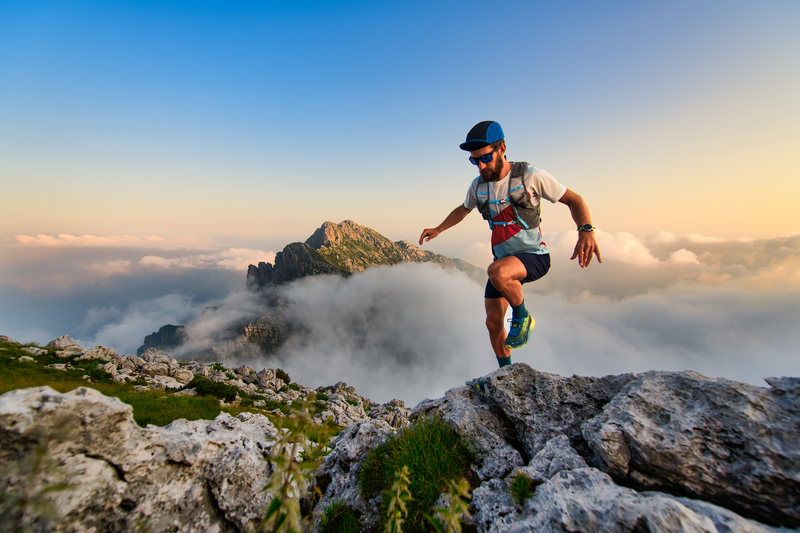
95% of researchers rate our articles as excellent or good
Learn more about the work of our research integrity team to safeguard the quality of each article we publish.
Find out more
EDITORIAL article
Front. Comput. Sci. , 25 February 2022
Sec. Human-Media Interaction
Volume 4 - 2022 | https://doi.org/10.3389/fcomp.2022.860619
This article is part of the Research Topic Brain-Computer Interfaces for Non-clinical (Home, Sports, Art, Entertainment, Education, Well-being) Applications View all 12 articles
Editorial on the Research Topic
Brain-Computer Interfaces for Non-clinical (Home, Sports, Art, Entertainment, Education, Well-Being) Applications
In this decade Brain-Computer Interface (BCI) technology has entered mainstream human-computer interaction (HCI) research for non-clinical applications. BCI has become part of multimodal interaction research as an additional interaction modality for a user of a technological system. BCI has also become part of research in which neurophysiological data provides a system with information about a user's affective and mental state, making it possible to adapt system, task, and interaction to a particular user, online (Fairclough, 2022). Currently, there is a market for inexpensive electroencephalographic (EEG) devices and software kits that capture voluntarily and involuntarily evoked brain activity and allow this activity to be translated into control and communication commands for environments and devices. Moreover, research on the use of deep networks for BCI applications has increased recently and promises to increase the accuracy of BCI systems (Craik et al., 2019). Overall, the availability of low cost non-invasive neurotechnology poses some ethical and regulatory challenges at the intersection of medical and consumer neurotechnologies.
Although EEG-based BCIs are limited in robustness and bandwidth, they are still, by far, the most accessible type of BCI to explore its potential use in domains such as games, entertainment, education, and art. While much of BCI research in clinics is increasingly relying on invasive recordings, such methods are most likely decades away from non-medical applications.
HCI researchers interest in BCI is increasing because the technology industry is expanding into application areas where efficiency is not the main goal of concern. Domestic or public space use of information and communication technology raise awareness of the importance of affect, comfort, family, community, or playfulness, rather than efficiency. Therefore, in addition to non-clinical BCI applications that require efficiency and precision, this Research Topic also addresses the use of BCI for various types of domestic, entertainment, educational, sports, and well-being applications. These applications can relate to an individual user as well as to multiple cooperating or competing users. We also see a renewed interest of artists to make use of such devices to design interactive art installations that know about the brain activity of an individual user or the collective brain activity of a group of users, for example, an audience (Contreras-Vidal et al., 2019). Hence, this Research Topic also addresses how BCI technology influences artistic creation and practice, and the use of BCI technology to manipulate and control sound, video, and virtual and augmented reality (VR/AR).
This Research Topic is composed of 11 accepted papers: seven dedicated to original research, a perspective, a mini review and two opinion pieces, and are dedicated to various themes and perspectives. These contributions address the multi-faceted nature of non-clinical BCIs, ranging from ethical ramifications of these neurotechnologies, applications to the arts, education, communication, wellbeing, and sports to the readiness of BCI deployment for gaming.
A first original research paper by Xie et al. focuses on the use of EEG-BCI technology as an objective evaluation method. The authors suggest using EEG and machine learning in order to assess car sound quality and show relationships between sound intensity and EEG energy.
Guillermo Bernal, Sean Montgomery and Pattie Maes discuss the future of BCI systems and how they may have an impact on productivity and efficiency, and/or augment the human experience by enhancing expressivity, understanding and empathy Bernal et al.. Artists and do-it-yoursef-ers (DIYers) are provided as examples of BCI users that deploy such systems beyond the intended9D use (for additional discussion, the reader is referred to Paek et al., 2020). Current challenges on data and code: sharing, transparency (e.g., preprocessed data and closed-source algorithms typically used by commercial systems), lack of interpretability and explainability (e.g., black box systems), and security are recognized as barriers to democratizing BCI systems. Their manuscript concludes with a discussion of the impact of closed loop BCI systems for generative content and augmented cognition when coupled with VR/AR systems.
Currently, the P300 component of the event-related potential (ERP) certainly is one of the most reliable EEG patterns to be used to control a so-called reactive BCI. P300-BCIs are also relevant for fast stimulus recognition as both the amplitude and latency of this EEG component are linked to cognitive processes potentially triggered by those stimuli. In their original research paper, Sutaj et al. design and evaluate a novel P300-based real-time image ranking BCI. Their work demonstrates the relevance of BCIs for stimulus sorting and brings insights on the influence of stimulus-related cognitive processes on the associated EEG patterns.
Emotional reactions monitoring and classification is an important part of many real life applications involving physiological and affective computing technologies. Li et al. describe their open-source software toolbox MindLink-Eumpy that aims at recognizing emotions by integrating EEG and facial expression information. Besides online experiment conducted, the offline validation was done using DEAP (Koelstra et al., 2011) and MAHNOB-HCI (Soleymani et al., 2011) datasets. As expected, the results show that multimodal methods outperform single-modal methods in both offline and online experiments.
With the expansion of the BCI field comes the development of numerous applications. While they could be relevant and re-used, both for research purposes and out-of-the-lab applications (e.g., entertainment, rehabilitation, etc.), those applications most often remain confidential. Efforts to provide these application open-source, as in Woo et al., will be most valuable for the community.
Recently, functional near-infrared spectroscopy (fNIRS) has been for brain studies outside the lab or indoor conditions involving user physical activity like walking, as it is more robust to movement artifacts than EEG-based imaging. The study by de With et al. focused on fear of heights in VR exposure therapy (VRET) settings using head-mounted display. It focused on the question to what extent fNIRS can differentiate users with and without anxiety disorders. Two experimental groups involved controls and pre-screened individuals with fear of heights (based on Acrophobia Questionnaire). While results showed limited statistical significance, the experimental group showed stronger reactions to fear inducing stimuli. The study demonstrates ecological validity of combining fNIRS measurements and VRET, encouraging further work on BCI-based therapy applications.
Another fNIRS study by Slutter et al. addresses phenomenon of choking under pressure in soccer players just seconds before the actual penalty kick on the football field. Results showed that when experienced players were feeling anxious, their left temporal cortex activation increased. While the work was methodologically challenging (e.g., 59% of the collected data has been removed due to artifacts), it demonstrates an important trend of moving out-of-the-lab to get ecologically valid brain imaging results. In should be also noted, that due the study design and the sports topic, this work received high media coverage, with journalists often overinterpreting the original results.
Scott and Raftery's perspective contribution addresses the potential impact of integrating brain-computer interfaces (BCI) and creative art therapy approaches to promote rehabilitative and therapeutic interactions while increasing patient engagement Scott and Raftery. The authors rightly argue that the application of BCI systems informed by creative expression—as a new form of digital health tool—may be extended to promote emotional and physiological healing and recovery. However, it remains to be seen how artistic/creative BCIs can be personalized and prescribed for health and well-being for use individually or in social contexts (see recent collection of works on BCI for artistic expression in Nijholt, 2019).
In their mini-review, Joan Belo, Maureen Clerc and Daniele Schn discuss emergent applications of passive BCIs and EEG-based auditory attention detection (AAD) in education and art Belo et al.. Of interest is the proposed use of an AAD module, which monitors the source and location of the user's attended auditory sources, to guide BCI control, for example, of external sound generating instruments.
Affective brain-computer music interface technology is used for mood enhancement by providing users with suggestions for the music they like and modulating the users affective states. In an opinion piece by Hildt, social and ethical aspects of the technology are discussed with a focus on the role of the brain and considerations about responsibility for controlling one's affective state, neural profiling, and privacy-related aspects.
In the second opinion paper, Cattan discusses the readiness of BCI game interfaces for the general public. The paper has critical reflections on the common limitations discussed in the literature, such as low transfer rate, cost and encumberment of materials, and the lack of game design and graphics compared with video games available on the market. In his opinion, there should be more focus on qualitative aspects of the interaction with BCI games, where BCI should be limited to aspects that cannot be achieved by traditional inputs.
The picture emerging from the contributions to this Research Topic, with its wide range and different approaches, is one that highlights the emergent challenges and opportunities for non-clinical BCI systems and their potential impact on society. Clearly, this is an area of research that is gaining considerable attention and that is expected to reach the public sooner than clinical BCI systems, which require careful evaluation of risks and benefits to the end users, and approval by regulatory agencies. The reader is cautioned that deployment or modifications of non-clinical BCI systems by end-users may lead to unintended consequences that are currently poorly understood. This is particularly critical for closed-loop or neurofeedback systems that may alter or adapt cognitive-emotional states in healthy individuals or in person with mental or cognitive disabilities, or brain injury. Clearly more research is needed on the ethical and thrust-worthy application of BCI systems outside the clinic.
All authors listed have made a substantial, direct, and intellectual contribution to the work and approved it for publication.
Partial support from the IUCRC BRAIN to JC-V is gladly recognized (NSF Award number 1650536). CJ has received partial support from the French National Agency (grant nos. ANR-20-CE38-0008-01—ABCIS project and ANR-20-CE37-0012-02—BETAPARK project). AV received partial support from the Estonian Research Council grant PUT1518.
JC-V is the Site Director for the IUCRC BRAIN at the University of Houston-an industry-university cooperative research center that is funded by the National Science Foundation and industrial partners.
The remaining authors declare that the research was conducted in the absence of any commercial or financial relationships that could be construed as a potential conflict of interest.
All claims expressed in this article are solely those of the authors and do not necessarily represent those of their affiliated organizations, or those of the publisher, the editors and the reviewers. Any product that may be evaluated in this article, or claim that may be made by its manufacturer, is not guaranteed or endorsed by the publisher.
To the always helpful Frontiers team whose organizational skills and understanding made this Research Topic possible.
Contreras-Vidal, J. L., Robleto, D., Cruz-Garza, J. G., Azorín, J. M., and Nam, C. S. (2019). Mobile Brain-Body Imaging and the Neuroscience of Art, Innovation and Creativity. Cham: Springer.
Craik, A., He, Y., and Contreras-Vidal, J. L. (2019). Deep learning for electroencephalogram (eeg) classification tasks: a review. J. Neural Eng. 16, 031001. doi: 10.1088/1741-2552/ab0ab5
Fairclough, S. H.. (2022). “Designing human-computer interaction with neuroadaptive technology,” in Current Research in Neuroadaptive Technology (London: Academic Press), 1–15.
Koelstra, S., Muhl, C., Soleymani, M., Lee, J.-S., Yazdani, A., Ebrahimi, T., et al. (2011). Deap: a database for emotion analysis; using physiological signals. IEEE Trans. Affect. Comput. 3, 18–31. doi: 10.1109/T-AFFC.2011.15
Paek, A. Y., Brantley, J. A., Evans, B. J., and Contreras-Vidal, J. L. (2020). Concerns in the blurred divisions between medical and consumer neurotechnology. IEEE Syst. J. 15, 3069–3080. doi: 10.1109/JSYST.2020.3032609
Keywords: brain-computer interfaces, EEG, human-computer interaction, affective computing, non-clinical applications, fNIRS
Citation: Nijholt A, Contreras-Vidal JL, Jeunet C and Väljamäe A (2022) Editorial: Brain-Computer Interfaces for Non-clinical (Home, Sports, Art, Entertainment, Education, Well-Being) Applications. Front. Comput. Sci. 4:860619. doi: 10.3389/fcomp.2022.860619
Received: 23 January 2022; Accepted: 07 February 2022;
Published: 25 February 2022.
Edited and reviewed by: Egon L. Van Den Broek, Utrecht University, Netherlands
Copyright © 2022 Nijholt, Contreras-Vidal, Jeunet and Väljamäe. This is an open-access article distributed under the terms of the Creative Commons Attribution License (CC BY). The use, distribution or reproduction in other forums is permitted, provided the original author(s) and the copyright owner(s) are credited and that the original publication in this journal is cited, in accordance with accepted academic practice. No use, distribution or reproduction is permitted which does not comply with these terms.
*Correspondence: Anton Nijholt, YS5uaWpob2x0QHV0d2VudGUubmw=
Disclaimer: All claims expressed in this article are solely those of the authors and do not necessarily represent those of their affiliated organizations, or those of the publisher, the editors and the reviewers. Any product that may be evaluated in this article or claim that may be made by its manufacturer is not guaranteed or endorsed by the publisher.
Research integrity at Frontiers
Learn more about the work of our research integrity team to safeguard the quality of each article we publish.