- Faculty of Health, Nutrition and Sports, Nutrition and Dietetics Department, The Hague University of Applied Sciences, The Hague, Netherlands
People tend to adapt the amount of their food intake to that of others around them. This so-called social modeling of eating has been extensively studied over the past decades. The current study complements these experiments and aims to investigate social modeling of healthy food intake using a video paradigm in which a virtual model consumed either a small or a large portion of apple. In addition, it was tested whether modeling effects of a virtual female confederate were equally strong in male and female participants. Thirty participants (13 female, 17 male) completed the low norm condition in which a virtual model consumed 30 g of apple. Another 30 participants (17 female, 13 male) were allocated to the high norm condition in which a virtual model consumed 100 g of apple. Participants completed an irrelevant task, after which their own intake of apple was measured. Average intake in the low norm condition was 3 g, average intake in the high norm condition was 26 g (p < 0.001). In conclusion, participants adapted their intake to that of the virtual model. This effect was found irrespective of gender; female and male participants equally adapted their intake to that of a female virtual model. Stimulating food intake via a virtual model in people who have difficulty to meet dietary requirements or inhibiting food intake in people who tend to consume too much would be interesting new steps in the development of healthcare applications.
Introduction
The consumption of food is a social practice and thus often shared with other people (Rozin, 2005). Surprisingly, these other people seem to have a large influence on our food intake (Herman et al., 2003; Suwalska and Bogdański, 2021). People tend to observe others to gain information about the appropriate amount of food to consume, an effect that has been described as social modeling of eating: people consume less when their co-eater eats less and more if their company eats more, compared to when eating alone (Herman and Polivy, 2005). This phenomenon has been extensively studied over the past decades (see for a review: Cruwys et al., 2015 and Suwalska and Bogdański, 2021) and turns out to be a robust phenomenon. Social modeling of eating takes place irrespective of being hungry or not (Goldman et al., 1991), of body weight (Romero et al., 2009) and of restraint status (Leone et al., 2007), amongst other things.
Social modeling can even take place when the model (the person who is being modeled) is not physically present in the room. Feeney et al. (2011) demonstrated that confronting participants with a list of food and fictional indicators of how much of the food was eaten by other (non-existing) participants had the same effect as a real-life model: in both conditions participants equally adapted their intake to the model (Feeney et al., 2011).
Others have used a video paradigm, in which the model was “present” via a digital screen (Romero et al., 2009; Hermans et al., 2012; McGeown and Davis, 2018). These latter studies gave mixed results: Hermans et al. (2012) failed to find modeling effects using videos (probably because of the large difference between the contexts in which the model and participants were consuming their food), Romero et al. (2009) did demonstrate social modeling via videos in children, and McGeown and Davis (2018) demonstrated social modeling mainly in participants with a lower BMI compared to those with a higher body weight.
According to the review of Cruwys et al. (2015) social modeling studies have largely focused on the intake of high-energy-dense and palatable foods, i.e., snacks. The same applies to the aforementioned studies using a video paradigm: the test products consisted of wine gums and M&M's (Hermans et al., 2012), cookies (Romero et al., 2009), and potato chips (McGeown and Davis, 2018). Social modeling of healthy eating (i.e., less palatable food) has been demonstrated as well, although with an actual model in the room (e.g., Howland et al., 2012; Robinson and Higgs, 2013) and with modeling effects that appear to be smaller (Hermans et al., 2009) or even absent (Pliner and Mann, 2004).
Less is known about social modeling effects of healthy food intake using a video paradigm. Horne et al. 's (2004) intervention on fruit and vegetable intake, involved showing videos to primary school children, featuring appealing fun characters (“food dudes”) eating fruit and vegetables. This approach resulted in a significant increase in children's food and vegetable intake as the children mirrored the characters eating behaviors. However, in this study participants probably looked up to the characters who featured in the videos, i.e., the characters were portrayed as cool and heroic, which makes this study rather different from other social modeling experiments in which the model is more equal to the participant.
Therefore, the current study sets out to investigate social modeling of healthy food intake in adult participants using a video paradigm. The outcomes of this study are relevant to the field as insights in modeling effects using a virtual confederate could be useful for the development of new technologies, for instance remote virtual assistance to promote healthy intake for people who have difficulty to meet dietary requirements or who have difficulty estimating a healthy portion size.
Since social modeling effects are stronger when the participant identifies with the individual who features in the social modeling paradigm (Cruwys et al., 2015), it was additionally tested whether possible modeling effects of a virtual female confederate were equally strong in male and female participants.
Methods
Participants
Participants were students at The Hague University of Applied Sciences in the Netherlands and were personally approached in the public spaces of the University with the request to participate in a short experiment. They were not accepted in the study if they were lecturers or allergic to apple (the test product).
Research Design
Participants were randomly allocated to either the low norm condition (in which a virtual model consumed only a small amount of apple: 30 g) or the high norm condition (in which a virtual model consumed a large amount of apple: 100 g) (see Figure 1). This was based on other modeling experiments (Roth et al., 2001; Pliner and Mann, 2004; Hermans et al., 2009): the amount in the low norm condition consisted of 30% of the amount in the high norm condition. Conditions were randomized across morning and afternoon sessions (to avoid the impact of time of day on the results).
Apple was chosen based on a pre-test amongst 387 other students who were asked to pick their most preferred healthy snack food among 14 options (e.g., apple, banana, melon, cucumber, carrots, etc.). Apple was chosen 199 times and was therefore clearly the preferred healthy snack.
Procedure
After giving their informed consent, participants were told a fictional story regarding their participation in the study, i.e., they were told that they were participating in a customer satisfaction study for a well-known furniture store. They were instructed to carefully watch a video clip (Figures 1, 2A) about the foundation of the company, its core values, its headquarters and the process of designing new furniture1. The parts on food were deleted from the original video, such that these could not have any influence on participants' food intake. The video was ~5 min long.
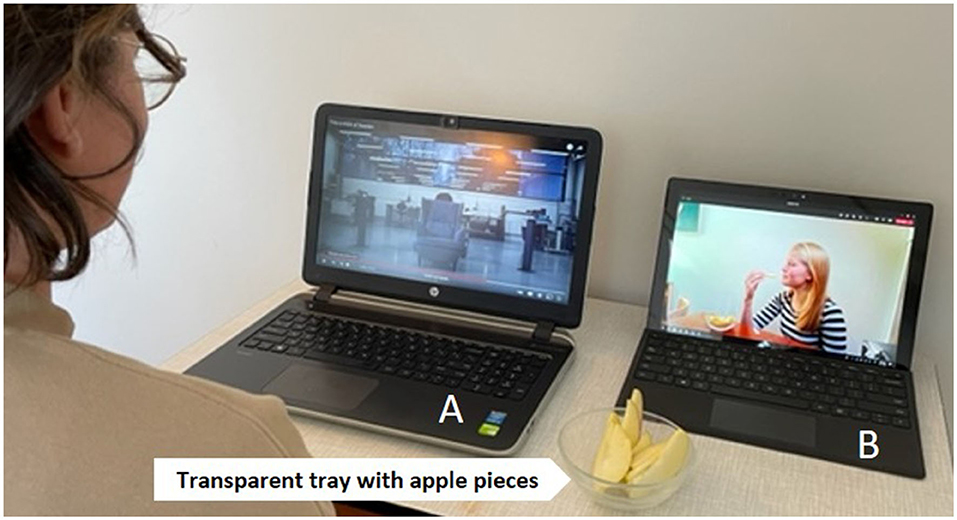
Figure 2. The experimental set-up: participants sat in front of two laptops and a transparent tray with apple pieces. Laptop (A) depicted the video and in turn the questionnaire. At the same time, laptop (B) depicted the virtual model who was either consuming a small amount (in the low norm condition) or a large amount (in the high norm condition) of apple from a comparable transparent tray.
After watching the video, participants were instructed to fill out a questionnaire about the content of the video (Figures 1, 2A). The general questions in this questionnaire were relevant for the purpose of this study: age, gender, length, and weight. All other questions about the video content only served the purpose of keeping participants busy for another 5 min after finishing watching the video. Answers to these questions were not analyzed.
During the study (10 min in total), participants were allowed to consume apple pieces from a transparent tray (Figure 2). At the start, the experimenter indicated that the participant was free to take some if he/she felt like it, but did not put a lot of emphasis on this instruction. The tray contained 100 g of apple (20 pieces of ~5 g each).
Next to the computer screen on which the furniture-store-video and questionnaire were presented, a second screen showed a person who was also consuming apple pieces from a transparent tray (Figure 2B): in the low norm condition the person on the screen consumed 30 g and in the high norm condition the person consumed 100 g. The person on the video was a female student. Participants were told that the person on the screen was a student who was also participating in the study. The screen with the virtual model was placed in such a way that participants did not need to focus on the screen, but that the movements made by the virtual person were still visible in the periphery of participants visual fields (i.e., every time the model took a piece of apple from the tray, this was clearly visible).
Analysis
Each tray with apple pieces was weighed before and after completion of the experiment, using a Silvercrest digital kitchen scale (readability 0.1 g), to determine the dependent variable Amount eaten in grams (between 0 and 100 g). In addition, the number of pieces of apples consumed (between 0 and 20) was documented. In turn, an ANOVA was conducted with dependent variable Amount eaten in grams and fixed factors Condition (Low vs. High norm) and Gender (Male vs. Female participants). The same analysis was conducted for the variable Number of pieces consumed.
Results
Participants
Table 1 shows participant characteristics for the low and high norm conditions, separately described for female and male participants. Both conditions contained a total of 30 participants. There were no differences between the low and high norm conditions and between male and female participants in Age and BMI (calculated based on length and weight of the participants) (all p's: n.s.).

Table 1. Participant characteristics (n, Age, and BMI), separately depicted per Condition (Low vs. High) and Gender (Female vs. Male participants).
Main Analysis
There was a main effect of Condition on the dependent variable Amount eaten in grams [MeanLownorm = 3.03, SD = 4.72; MeanHighnorm = 26.1, SD = 26.45; F(1, 60) = 20.86; p < 0.001]. There was no main effect of Gender on the Amount eaten in grams [MeanFemale = 12.7, SD = 21.24; MeanMale = 16.4, SD = 23.19; F(1, 60) = 0.02, p = 0.899] and no interaction between Condition and Gender [F(1, 60) = 0.18, p = 0.670]. The main effect of Condition and the absence of a Gender effect is demonstrated in Figure 3. A main effect of Condition was also observed for the dependent variable Number of pieces consumed [MeanLownorm = 0.60, SD = 0.93; MeanHighnorm = 5.23, SD = 5.34; F(1, 60) = 20.72; p < 0.001]. The effect of Gender and the interaction between Gender and Condition were not significant (p = 0.882 and p = 0.681, respectively). To control for a time of day effect, both ANOVA's were conducted again including the fixed factor Time of Day (morning or afternoon). There was no effect of Time of Day for both the dependent variables Amount eaten in grams and Number of apple pieces consumed (p = 0.396 and p = 0.409, respectively).
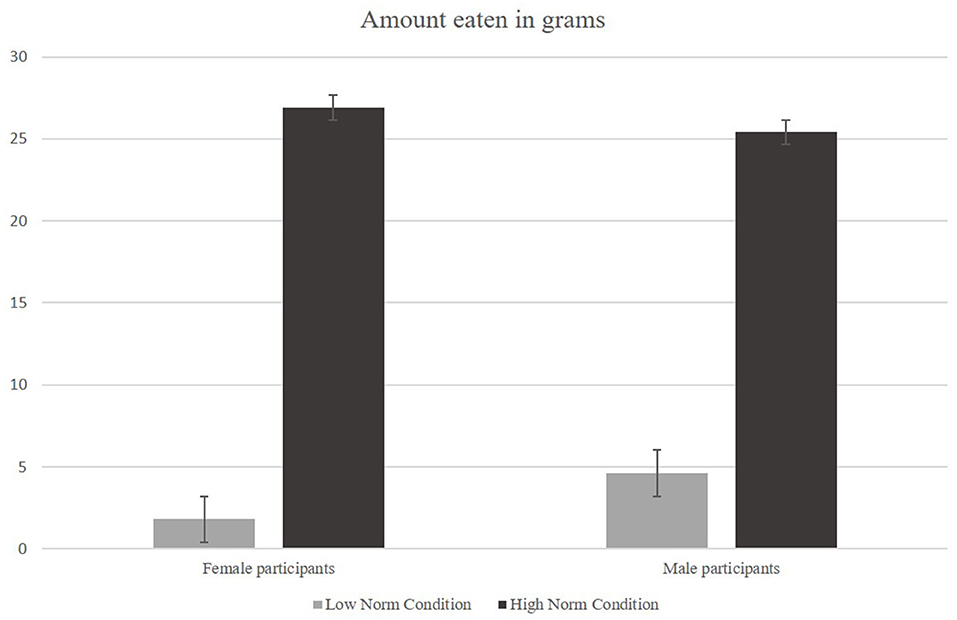
Figure 3. Amount eaten in grams after being exposed to a virtual model that consumed a small portion (Low Norm Condition) or a larger portion (High Norm Condition), separately depicted for Female and Male participants.
Discussion
This experiment demonstrated a strong social modeling effect of healthy food intake. Participants in the study adapted their intake to that of the model: more apple was consumed when the confederate consumed a large amount as opposed to the condition in which the confederate consumed a small amount.
Social modeling occurred irrespective of gender: female and male participants equally adapted their intake to that of a female virtual person. Apparently, perceived similarity between the model and the participant was high (Cruwys et al., 2015), irrespective of gender. A potential explanation could lie in the instruction that was given by the experimenter. The presence of the virtual confederate was explained as “another student who also takes part in this study,” which might have emphasized the resemblance between the participants and the model and decreased the perceived differences.
Although there was a clear difference in intake between the two conditions of ~23 g (or 4.5 pieces), the intake itself was lower than that of the virtual model. Participants consumed only 10 and 26 percent of what the confederate consumed in the low and high norm conditions, respectively (i.e., 3 of the 30 g and 26 of the 100 g). A substantial lower intake than that of the model was also found in a study by Hermans et al. (2009). Participants in that study were exposed to one of three conditions: a (live) model who did not consume any pieces of vegetables (the control condition), a model who consumed three pieces (the low norm) and a model who consumed 10 pieces (the high norm; test food: carrot and cucumber). Participants consumed a mean amount of 1.39 pieces in the control condition 1.48 pieces in the low norm condition, and 2.71 pieces in the high norm condition. Again, although participants' intake was significantly influenced by the model, the intake itself was small compared to the intake of the model.
A potential explanation of this lower intake could lie in the fact that participants were exposed to an unfamiliar model. According to research conducted by Clendenen et al. (1994) familiarity of the co-eater(s) is of substantial influence. In their experiment, participants consumed 44% more when they were eating together with friends compared to eating with unfamiliar individuals. Herman (2015) hypothesizes that this inhibition effect might be the result of increased self-awareness while eating with unfamiliar others, leading to the setting of an unconscious upper-limit to make sure a persons' intake is not perceived as excessive. On the other hand, a more recent study of Kaisari and Higgs (2015) did not demonstrate a moderating factor of familiarity on modeling effects. So the impact of familiarity remains uncertain, especially in the context of virtual modeling.
Because Hermans et al. (2009) included a control condition in which the model did not consume any food, they could clearly conclude that participants in the high norm condition were stimulated to consume more pieces of vegetables, whereas there was no inhibition in the low norm condition when comparing intake to the non-eating condition. The lack of a clear control condition in the current study makes it impossible to make inferences on whether the virtual confederate was able to stimulate or inhibit eating, or potentially do both.
Understanding the distinction between inhibition and stimulation effects is important for the design of potential applications, since both effects could be tremendously beneficial in healthcare contexts. Stimulating food intake via a virtual model in people who have difficulty to meet dietary requirements or inhibiting food intake in people who tend to consume too much would be interesting new steps in the development of healthcare applications. So far, technology in which virtual assistance is provided shows promising results in for instance elderly and people with dementia (Osvath et al., 2018), in people with diabetes (Ramchandani, 2019) and in overweight adolescents (LeRouge et al., 2016). In most of these examples, virtual assistance comprises of remote monitoring of health status or stimulating healthy behavior, sometimes using virtual “avatars” or “buddies.” However, modeling virtual healthy food intake in a real-life healthcare setting has not been studied before.
In the current study, participants' hunger status was not recorded, while hunger might have had an influence on the amount of apple participants consumed. Previous studies demonstrated that hunger had no impact on modeling effects (Goldman et al., 1991; Cruwys et al., 2015) but it is unclear whether this also applies to healthy food and to modeling via a digital screen. To randomize conditions over the day and to check for an impact of time of day, it was recorded whether participants conducted the experiment in the morning or the afternoon session. There was however no effect of the time of the day on the outcome variables amount eaten in grams and number of apple pieces consumed. Still, in future experiments hunger status should be recorded to further investigate potential mediating effects on modeling.
Similarly, participants' liking for apple was not registered. Although a pre-test was conducted to pick the most preferred healthy snack amongst students, the participants in the current study might have had a different personal taste. In future experiments, liking scores for the test food are essential to make sure that these do not interfere with the results.
The current study focused on modeling the amount eaten by the virtual model. An additional factor that should be further studied is the potential of virtual models influencing food choice behavior. Modeling effects on food choice have been demonstrated before (Prinsen et al., 2013; Salmon et al., 2014), but results seem to be less strong and robust (Pliner and Mann, 2004; Cruwys et al., 2015). An explanation for this difference has been sought in the fact that people are less uncertain about their food preferences as opposed to the appropriate quantity to consume, making food choices less sensitive for social influences (Pliner and Mann, 2004). Whether this applies to modeling virtual confederates is still to be explored.
Finally, consuming food together with people on “the other side of the screen” has an entire new connotation since the COVID-19 pandemic. Whereas, Niewiadomski et al. (2019) and Spence et al. (2019) were already reviewing insights related to the social and pleasurable aspects of remote dining (i.e., commensality) before the COVID-19-outbreak, Ceccaldi et al. (2020) investigated the phenomenon of remote eating during the pandemic: People apparently seek connectedness and belonging when they decide to eat together via video-conference-calls. Whether social modeling occurs during these circumstances should be further investigated. The social modeling effect may be enhanced during remote dining with friends and family, as familiarity increases social modeling effects. Nevertheless, other factors may also play a role in food intake during remote dining, such as how pleasurable people perceive the experience (which may increase the duration of the meal) and whether people are distracted by conversations. Both factors may increase food intake (Herman et al., 2003; Ogden et al., 2013).
Since many of our day-to-day interactions occur via screens nowadays, it might be highly relevant to further develop technological innovations to fulfill both social and pleasurable desires related to “eating together apart” as well as healthy intake requirements. This field provides many opportunities for future research and new applications.
Data Availability Statement
The raw data supporting the conclusions of this article will be made available by the authors, without undue reservation.
Ethics Statement
Ethical review and approval was not required for the study on human participants in accordance with the local legislation and institutional requirements. The patients/participants provided their written informed consent to participate in this study.
Author Contributions
PB and SS: study conception and design, draft manuscript preparation, analysis, and interpretation of results. All authors reviewed the results and approved the final version of the manuscript.
Funding
This study was provided by the Nutrition and Dietetics department of The Hague University.
Conflict of Interest
The authors declare that the research was conducted in the absence of any commercial or financial relationships that could be construed as a potential conflict of interest.
Publisher's Note
All claims expressed in this article are solely those of the authors and do not necessarily represent those of their affiliated organizations, or those of the publisher, the editors and the reviewers. Any product that may be evaluated in this article, or claim that may be made by its manufacturer, is not guaranteed or endorsed by the publisher.
Acknowledgments
The authors would like to thank Hanneke Kros for her assistance in collecting the data for this study and Simone Hackett for an extensive language check.
Footnotes
References
Ceccaldi, E., Huisman, G., Volpe, G., and Mancini, M. (2020). Guess who's coming to dinner? Surveying digital commensality during Covid-19 outbreak, in Companion Publication of the 2020 International Conference on Multimodal Interaction, 317–321.
Clendenen, V. I., Herman, C. P., and Polivy, J. (1994). Social facilitation of eating among friends and strangers. Appetite 23, 1–13. doi: 10.1006/appe.1994.1030
Cruwys, T., Bevelander, K. E., and Hermans, R. C. (2015). Social modeling of eating: a review of when and why social influence affects food intake and choice. Appetite 86, 3–18. doi: 10.1016/j.appet.2014.08.035
Feeney, J. R., Polivy, J., Pliner, P., and Sullivan, M. D. (2011). Comparing live and remote models in eating conformity research. Eat. Behav. 12, 75–77. doi: 10.1016/j.eatbeh.2010.09.007
Goldman, S. J., Herman, C. P., and Polivy, J. (1991). Is the effect of a social model on eating attenuated by hunger? Appetite 17, 129–140. doi: 10.1016/0195-6663(91)90068-4
Herman, C. P. (2015). The social facilitation of eating. A review. Appetite 86, 61–73. doi: 10.1016/j.appet.2014.09.016
Herman, C. P., and Polivy, J. (2005). Normative influences on food intake. Physiol. Behav. 86, 762–772. doi: 10.1016/j.physbeh.2005.08.064
Herman, C. P., Roth, D. A., and Polivy, J. (2003). Effects of the presence of others on food intake: a normative interpretation. Psychol. Bull. 129:873. doi: 10.1037/0033-2909.129.6.873
Hermans, R. C., Larsen, J. K., Herman, C. P., and Engels, R. C. (2009). Effects of social modeling on young women's nutrient-dense food intake. Appetite 53, 135–138. doi: 10.1016/j.appet.2009.05.004
Hermans, R. C., Salvy, S. J., Larsen, J. K., and Engels, R. C. (2012). Examining the effects of remote-video confederates on young women's food intake. Eat. Behav. 13, 246–251. doi: 10.1016/j.eatbeh.2012.03.008
Horne, P. J., Tapper, K., Lowe, C. F., Hardman, C. A., Jackson, M. C., and Woolner, J. (2004). Increasing children's fruit and vegetable consumption: a peer-modelling and rewards-based intervention. Eur. J. Clin. Nutr. 58, 1649–1660. doi: 10.1038/sj.ejcn.1602024
Howland, M., Hunger, J. M., and Mann, T. (2012). Friends don't let friends eat cookies: effects of restrictive eating norms on consumption among friends. Appetite 59, 505–509. doi: 10.1016/j.appet.2012.06.020
Kaisari, P., and Higgs, S. (2015). Social modelling of food intake. The role of familiarity of the dining partners and food type. Appetite 86, 19–24. doi: 10.1016/j.appet.2014.09.020
Leone, T., Pliner, P., and Herman, C. P. (2007). Influence of clear versus ambiguous normative information on food intake. Appetite 49, 58–65. doi: 10.1016/j.appet.2006.11.005
LeRouge, C., Dickhut, K., Lisetti, C., Sangameswaran, S., and Malasanos, T. (2016). Engaging adolescents in a computer-based weight management program: avatars and virtual coaches could help. J. Am. Med. Inf. Assoc. 23, 19–28. doi: 10.1093/jamia/ocv078
McGeown, L., and Davis, R. (2018). Social modeling of eating mediated by mirror neuron activity: a causal model moderated by frontal asymmetry and BMI. Behav. Brain Res. 338, 66–75. doi: 10.1016/j.bbr.2017.10.009
Niewiadomski, R., Ceccaldi, E., Huisman, G., Volpe, G., and Mancini, M. (2019). Computational Commensality: from theories to computational models for social food preparation and consumption in HCI. Front. Robot. AI 6:119. doi: 10.3389/frobt.2019.00119
Ogden, J., Coop, N., Cousins, C., Crump, R., Field, L., Hughes, S., et al. (2013). Distraction, the desire to eat and food intake. Towards an expanded model of mindless eating. Appetite 62, 119–126. doi: 10.1016/j.appet.2012.11.023
Osvath, P., Kovacs, A., Boda-Jorg, A., Tenyi, T., Fekete, S., and Voros, V. (2018). The use of information and communication technology in elderly and patients with dementia. J. Gerontol. Geriatr. Res. 7:2. doi: 10.4172/2167-7182.1000475
Pliner, P., and Mann, N. (2004). Influence of social norms and palatability on amount consumed and food choice. Appetite 42, 227–237. doi: 10.1016/j.appet.2003.12.001
Prinsen, S., de Ridder, D. T., and de Vet, E. (2013). Eating by example. Effects of environmental cues on dietary decisions. Appetite 70, 1–5. doi: 10.1016/j.appet.2013.05.023
Ramchandani, N. (2019). Virtual coaching to enhance diabetes care. Diabetes Technol. Ther. 21:S2–S48. doi: 10.1089/dia.2019.0016
Robinson, E., and Higgs, S. (2013). Food choices in the presence of ‘healthy' and ‘unhealthy' eating partners. Br. J. Nutr. 109, 765–771. doi: 10.1017/S0007114512002000
Romero, N. D., Epstein, L. H., and Salvy, S. J. (2009). Peer modeling influences girls' snack intake. J. Am. Diet. Assoc. 109, 133–136. doi: 10.1016/j.jada.2008.10.005
Roth, D. A., Herman, C. P., Polivy, J., and Pliner, P. (2001). Self-presentational conflict in social eating situations: a normative perspective. Appetite 36, 165–171. doi: 10.1006/appe.2000.0388
Rozin, P. (2005). The meaning of food in our lives: a cross-cultural perspective on eating and well-being. J. Nutr. Educ. Behav. 37, S107–S112. doi: 10.1016/S1499-4046(06)60209-1
Salmon, S. J., Fennis, B. M., de Ridder, D. T., Adriaanse, M. A., and De Vet, E. (2014). Health on impulse: when low self-control promotes healthy food choices. Health Psychol. 33:103. doi: 10.1037/a0031785
Spence, C., Mancini, M., and Huisman, G. (2019). Digital commensality: Eating and drinking in the company of technology. Front. Psychol. 10:2252. doi: 10.3389/fpsyg.2019.02252
Keywords: social modeling, eating behavior, food intake, virtual, social norm, health
Citation: Bulsing PJ and Salmon SJ (2022) Social Modeling of Virtual Healthy Food Intake. Front. Comput. Sci. 4:832996. doi: 10.3389/fcomp.2022.832996
Received: 10 December 2021; Accepted: 14 February 2022;
Published: 09 March 2022.
Edited by:
Gijs Huisman, Delft University of Technology, NetherlandsReviewed by:
Guido Camps, Wageningen University and Research, NetherlandsEleonora Ceccaldi, University of Genoa, Italy
Copyright © 2022 Bulsing and Salmon. This is an open-access article distributed under the terms of the Creative Commons Attribution License (CC BY). The use, distribution or reproduction in other forums is permitted, provided the original author(s) and the copyright owner(s) are credited and that the original publication in this journal is cited, in accordance with accepted academic practice. No use, distribution or reproduction is permitted which does not comply with these terms.
*Correspondence: Patricia Johanna Bulsing, UC5KLkJ1bHNpbmdAaGhzLm5s