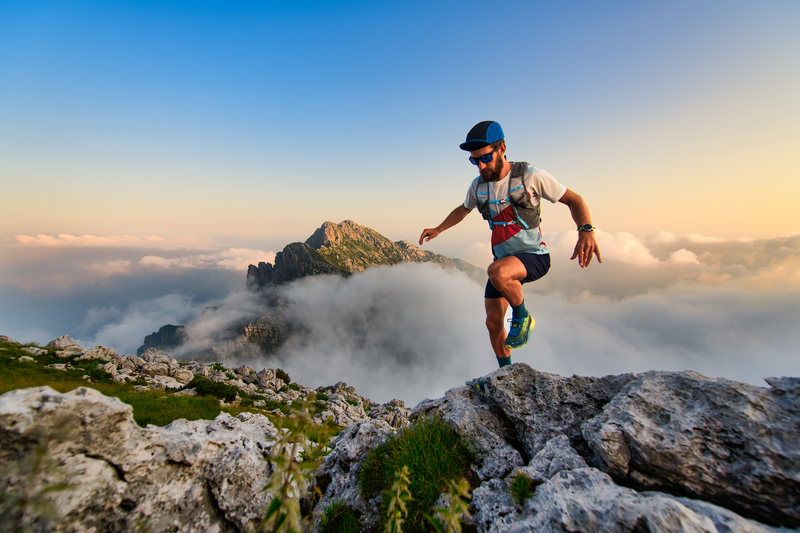
94% of researchers rate our articles as excellent or good
Learn more about the work of our research integrity team to safeguard the quality of each article we publish.
Find out more
ORIGINAL RESEARCH article
Front. Comput. Sci. , 28 May 2021
Sec. Human-Media Interaction
Volume 3 - 2021 | https://doi.org/10.3389/fcomp.2021.682982
This article is part of the Research Topic Towards Omnipresent and Smart Speech Assistants View all 12 articles
Conversational agents and smart speakers have grown in popularity offering a variety of options for use, which are available through intuitive speech operation. In contrast to the standard dyad of a single user and a device, voice-controlled operations can be observed by further attendees resulting in new, more social usage scenarios. Referring to the concept of ‘media equation’ and to research on the idea of ‘computers as social actors,’ which describes the potential of technology to trigger emotional reactions in users, this paper asks for the capacity of smart speakers to elicit empathy in observers of interactions. In a 2 × 2 online experiment, 140 participants watched a video of a man talking to an Amazon Echo either rudely or neutrally (factor 1), addressing it as ‘Alexa’ or ‘Computer’ (factor 2). Controlling for participants’ trait empathy, the rude treatment results in participants’ significantly higher ratings of empathy with the device, compared to the neutral treatment. The form of address had no significant effect. Results were independent of the participants’ gender and usage experience indicating a rather universal effect, which confirms the basic idea of the media equation. Implications for users, developers and researchers were discussed in the light of (future) omnipresent voice-based technology interaction scenarios.
Conversational Agents (CAs) have grown in popularity over the last few years (Keynes, 2020). New devices such as smart speakers (Perez, 2019) or application such as chatbots or virtual assistants (Petrock, 2019) have become part of everyday technology usage. The voice-controlled operation of technology offers a variety of features and functions such as managing a calendar or controlling the lights. They promise to simplify daily life, while their operation is convenient and low threshold (Cannon, 2017). CAs are utilizable in situations in which users need their hands for something else than handling a device. Inexperienced or less skilled users are capable to operate CAs (Sansonnet et al., 2006) resulting in increasing numbers of user groups. Moreover, CAs have become the object of user-centered scientific research, which analyzes the human users’ reactions towards the device, the underlying usage motivations or the effects of usage [e.g., CHI 2019 Workshop by Jacques et al. (2019)]. Research in this area so far mostly focuses on the standard scenario of technology usage: a single person operates a certain device. However, the usage of conversational agents expands this user-device dyad. A more social scenario will unfold if the user speaking to an CA is observed by others or if the device is used jointly by couples or families (for an overview: Porcheron et al., 2017). Consequently, speech operation widens the scientific perspective on technology usage. Not only the users themselves but also co-users and observers come into view. For example, research analyzing the verbal abuse of CAs impressively demonstrates need for research widening its focus: 10 to approximately 40% of interactions include aspects of abusive language or misuse of the device (Chin et al., 2020). As these interactions might be observed by others, by partners or children, the impact of the abusive behavior clearly exceeds the user-device-dyad.
Referring to increasingly social usage scenarios, the present study focuses on the effects of observing user-technology interactions and asks: How are observers of a rude interaction between a CA and a user affected? Do observers experience empathetic reactions towards a smart speaker, which is treated rudely?
Until recently, the ability to understand and apply spoken language was regarded as a fundamentally and exclusively human characteristic (Pinker, 1994). Interacting with ‘talking’ devices and ‘having a conversation’ with CAs constitutes a new level of usage relevant for both components of HCI, the perspective on ‘humans’ as well as on ‘computers’ (Luger and Sellen, 2016).
Conversational Agents–also referred to as voice assistants, vocal social agents, voice user interfaces or smart speakers–are among the most popular devices used to run voice-controlled personal assistants (Porcheron et al., 2018). The best-selling smart speakers worldwide are Amazon Echo and Google Home running Alexa or Google Assistant, respectively (BusinessWire, 2018). In the US, 66.4 million people own a smart speaker already, with a 61% market share of Amazon Echo and 24% share of Google Home (Perez, 2019). In 2019, 20% of United Kingdom households had a smart speaker already, while Germany passed the 10% mark (Kinsella, 2019). Statistics predict that the global increase will continue to 1.8 billion users worldwide by the end of 2021 (Go-Gulf, 2018).
Within the last 60 years, natural language-processing applications and the number of services supporting voice commands have evolved rapidly. McTear, Callejas and Griol (2016) summarize the history of conversational interfaces beginning back in the 1960s, when the first text-based dialogue systems answered questions and the first chatbots simulated casual conversation. About 20 years later, speech-based dialogue systems and spoken dialogue technology evolved, which were soon transferred to commercial contexts. In subsequent years, conversational agents and social robots came into the picture. Hirschberg and Manning (2015), p. 261 ascribe the recent improvements to four key factors: the progress in computing power, machine learning, and in the understanding of the structure and the deployment of human language. Additionally, it can be ascribed to the large amounts of linguistic data available. As a result, today’s CA technology is much easier to use than earlier voice recognition systems, which allowed only very restricted phrases and word patterns. From a user-centered perspective, human-computer interaction has never resembled human-human interaction that closely. CAs are on their way to become everyday interaction partners. We will learn how to interact with them, how to pronounce and how to phrase our speech commands properly–also by observing others. Children will grow up observing their parents interacting with smart speakers before interacting with them themselves (Hoy, 2018).
Questions about the effects of these new characteristics of usage arise from multiple disciplines with developers, researchers, users and the society as a whole being involved. First studies reveal ethical challenges (Pyae and Joelsson, 2018). By demonstrating the ‘submissiveness (of AI software) in the face of gender abuse,’ West et al. (2019), p. 4 raise issues that CAs, which are projected as young women (‘Alexa,’ ‘Siri,’ ‘Cortana’), potentially perpetuate gender biases. To gain deeper insights into the impact of ‘speaking’ technology on humans, the media equation approach offers a fruitful theoretical framework.
The ‘Computers As Social Actors’ Paradigm and the Media Equation Approach
In the early 1990s, Clifford Nass and Byron Reeves introduced a new way of understanding electronic devices. They conceptualized computers as ‘social actors’ to which users automatically react as if they were human beings (Reeves and Nass, 1996). Their empirical studies revealed that users tend to (unconsciously) interpret cues sent by computers as social indicators of a human counterpart to ‘whom’ they react accordingly. Literature provides various explanations for this phenomenon with the evolutionary perspective offering a framing theoretical perspective (Nass et al., 1997; Nass and Gong, 2000; Kraemer et al., 2015; Carolus et al., 2019a). Like our bodies, the human brain is adapted to our early ancestors’ world in which every entity one perceived was a real physical object and every entity communicating as a human being sure enough was a human being (Buss and Kenrick, 1998). ‘Mentally equipped’ in this way, we encounter today’s new media and technology sending various cues, which would have indicated a human counterpart back in the days of our evolutionary ancestors. Unconsciously, ‘evolved psychological mechanisms’–neurocognitive mechanisms evolved to efficiently contribute to the adaptive problems of our ancestors’ world (here: interacting with other human beings)–are triggered (Cosmides and Tooby, 1994). Thus, the computer’s cues are interpreted as a communicative act and therefore as a human interaction resulting in the individual to behave accordingly, showing social reaction originally exclusive for human-human interaction (Tooby and Cosmides, 2005; Dawkins, 2016).
Research analyzing these phenomena followed an experimental approach, which Nass et al. (1994) referred to as the paradigm ‘Computers As Social Actors’ (CASA). Findings of social science describing social dynamics of human-human interactions were transferred to the context of human-computer interactions. In laboratory studies, one of the human counterparts of the social dyad was replaced by a computer sending certain allegedly ‘human’ cues. For example, they anticipated research on CAs when they analyzed participants interacting with computers, which appeared to be voice controlled talking in either a female or a male voice. Results revealed that participants transferred gender stereotypes to these devices. A domineering appearance of a computer ‘speaking’ with a male voice was interpreted more positively as the exact identical statements presented by a computer with a female voice (Nass et al., 1997). Considering that these experiments took place more than 20 years ago, when devices were much bulkier and their handling was a lot more difficult, the revealed social impact of technology on their users was remarkable. Particularly, when considering that participants reported to know they were interacting with a computer–and not with a male or female person. They consciously knew that they were interacting with technology, but they (unconsciously) ascribed gender stereotypical characteristics.
Additional studies revealed further indication of gender stereotyping (Lee et al., 2000; Lee and Nass, 2002; Morishima et al., 2002) and further social norms and rules to be applied to computers, e.g., politeness (Nass et al., 1999) or group membership (Nass et al., 1996). More recently, studies transferred this paradigm to more recent technology (Carolus et al., 2018; Carolus et al., 2019a). Rosenthal-von der Puetten et al. (2013) widened the focus and analyzed observers’ empathetic reactions toward a dinosaur robot (Ugobe’s Pleo). They showed that witnessing the torture of this robot elicits empathetic reactions in observers. Similarly, Cramer et al. (2010) as well as Tapus et al. (2007) revealed different forms of empathy on users’ attitudes toward robots in Human-Robot Interactions [see also: Rosenthal-von der Puetten et al. (2013)]. However, the experimental manipulation to observe animal like or humanlike robots being tortured invites contradiction. Empathetic reactions might be triggered by effects of anthropomorphism (Riek et al., 2009) as the objects and their treatment highlighted the anthropomorphic (or animal like) character of the devices. Social robots looked like living creatures and reacted to physical stimulus, accordingly. Moreover, the incidents participants observed are of limited everyday relevance. They were invited to a laboratory to interact (or watch others interacting) with devices, which are far away from everyday experiences. Consequently, the explanatory power regarding everyday technology usage outside of scientists’ laboratories is limited.
Modern CAs can ‘listen’ and respond to the users’ requests in ‘natural and meaningful ways’ (Lee et al., 2000, p. 82) which Luger and Sellen refer to as ‘the next natural form of HCI’ (Luger and Sellen, 2016, p. 5286). From the media equation perspective, voice assistants represent a new form of a ‘social actor’: CAs adopt one of the most fundamentally human characteristics resulting in research questions regarding their social impact on human users–those actively speaking to them and those listening (Purington et al., 2017).
While processing language is a salient, humanlike feature of CAs, the outward appearance of smart speakers, for example, is distinctly technological. In contrast to embodied agents or social robots, they are barely anthropomorphic but look like portable loudspeakers. Google’s Home Mini and Amazon’s Echo Dot resemble an oversized version of a puck, their larger devices (Echo and Home) come in a cylinder shape with some colored light signals. Consequently, smart speakers are regarded as a promising research object to analyze the effects of speech–independent of further humanoid or anthropomorphistic cues such as the bodily or facial expressions of a social robot, for example. Moreover, they bridge the gap of everyday relevance as they are increasingly popular and are used by an increasing number of average users outside of scientific contexts. Finally, because ‘speaking is naturally observable and reportable to all present to its production’ (Porcheron et al., 2017, p. 433), smart speakers allow realistic social usage scenarios. Devices are designed for multiple simultaneous users. Amazon Echo and Google Home, for example, use multiple microphones and speakers facilitating conversations with multiple users. Consequently, bystanders of interactions–children, partners or other family members–watch, listen, or join the conversation and might be affected by its outcomes (Sundar, 2020).
A huge body of literature focuses on empathy resulting in various attempts to define the core aspects of the concept with Cuff et al. (2016) presenting 43 different definitions. Back in 1872, the German philosopher Robert Vischer introduced the term ‘Einfuehlung’ which literally means ‘feeling into’ another person (Vischer, 1873). Taking the perspective of this other person aims for an understanding of ‘what it would be like to be living another body or another environment’ (Ganczarek et al., 2018). A few years later, Lipps (1903) argued that an observer of another person’s emotional state tends to imitate the emotional signals of the other person ‘inwardly’ by physically adapting body signals. Macdougall (1910) and Titchener (1909) translated ‘Einfuehlung’ as ‘Empathy’ and introduced the term still used today. Today, empathy is broadly referred to as ‘an affective response more appropriate to someone else’s situation than to one’s own’ (Hoffman, 2001, p. 4). Moreover, modern conceptualizations distinguish two main perspectives on ‘empathy’: the rational understanding and the affective reaction to another person’s feelings or circumstances (De Vignemont and Singer, 2006). The cognitive component refers to the recognition and understanding of the person’s situation by including the subcomponents of perspective-taking (i.e., adopting another’s psychological perspective) and identification (i.e., identifying with the other character). The affective component includes the subcomponent of empathic concern (e.g., sympathy, compassion), pity (i.e., feeling sorry for someone) and personal distress (i.e., feelings of discomfort or anxiety) into the process of sympathizing (Davis, 1983; for an overview of empathy from a neuro scientific, psychological, and philosophical perspective see Rogers et al. (2007). Conceptually and methodologically, Davis (1983, p. 168) widens the perspective on empathy when distinguishing between state empathy and trait empathy. State empathy describes an affective state and is a result of a ‘situational manipulation’ (Duan and Hill, 1996). Trait empathy is defined as a dispositional trait resulting in enduring interindividual differences (Hoffman, 1982), which is a significant predictor of empathetic emotion (Davis, 1983).
Users’ emotional reactions towards technology has become an increasingly important area of research Misuse or abusive treatment have been reported for various forms of technological artifacts or social agents, with a substantial body of research focusing on graphically represented (virtual) agents and robots (Brahnam and De Angeli, 2008; Paiva et al., 2017). Due to their relative novelty, little empirical research has been done regarding abusive interactions with smart speakers. Although not entirely comparable, robots and smart speakers are regarded as intelligent agents interacting with its users through a physical body. Thus, the literature review focuses on literature on robots as ‘empathetic agents’ (Paiva et al., 2017).
Both anecdotal and scientific examples reveal incidents of aggressive and abusive behavior towards robots (Bartneck and Hu, 2008). Salvini et al. (2010) reported on a cleaning robot abused by bypassers. Brscic et al. (2015) told about children abusing a robot in a mall (for an overview see: Tan et al. (2018)). A more recent study refers to another perspective: people react empathetically to robots which are attacked by others (for an overview: Leite et al. (2014)). For example, Vincent (2017) reported on a drunken man, who attacked a robot in a car park resulting in empathetic reactions with the robot. Empathy with social robots has been studied increasingly in the last years to understand humans’ empathetic reactions towards them, to prevent abusive behavior or to develop robots, which users perceive as being empathetic agents (e.g., Salvini et al., 2010; Nomura et al., 2016; Bartneck and Keijsers, 2020). As the abuse even of technology raises ethical questions (and economic questions due to resulting destruction), most of the studies avoid encouraging the participants to physically harm the device. Instead, paradigms involving reduced radical variations of abuse were established (Paiva et al., 2017; Bartneck and Keijsers, 2020). Rosenthal-von der Puetten et al. (2013) introduced a paradigm, which allows to study more radical interactions. Their participants were shown pre-recorded videos of a dinosaur robot, which was tortured physically. Observing torture resulted in increased physiological arousal and self-reports revealed more negative and less positive feelings. In sum, measures revealed participants’ empathy with the robot–a finding that literature review confirms (Riek et al., 2009; Kwak et al., 2013; Paiva et al., 2017). In a follow-up study, Rosenthal-von der Puetten et al. (2014) compared participants’ neural activation and self-reports when watching a video of a human or a robot being harmed. In the human-torture condition, neural activity and self-reports revealed higher levels of emotional distress and empathy. Further studies asked for the characteristics of the entities that elicit emotional reactions revealing that participants were rather empathetic with robots, which were more humanlike in terms of anthropomorphistic appearances perceived agency and the capacity to express empathy themselves (Hegel et al., 2006; Riek et al., 2009; Cramer et al., 2010; Gonsior et al., 2012; Leite Iolanda et al., 2013; Leite et al., 2013b). Additionally, Kwak et al. (2013) emphasized the impact of physical embodiment. Participants’ empathy was more distinct toward a physically embodied robot (vs. a physically disembodied robot).
As an interim conclusion, literature research on empathy with technological devices focused on robots and revealed that humanlike cues (e.g., outward appearance and empathy toward their human counterparts) increased the level of elicited empathy in humans. However, robots send various social cues (e.g., outward appearance, move, facial expression, verbal and nonverbal communication), which evolve into meaningful social signals during an interaction (for an overview: Feine et al. (2019). Analyzing interactions with these technological entities will result in confounding regarding the underlying cues of elicited empathy. Consequently, the present study takes a step back to refine the analysis. Following the taxonomy of social cues (Feine et al., 2019, p. 30), we distinguish between verbal, visual, auditory and invisible cues that CAs could present. With or focus on an interaction with a smart speaker we concentrate on verbal cues keeping visual cues reduced (simple cylindric shape of the device, no facial or bodily expression). Therefore, smart speakers offer the externally valid option to narrow down the magnitude of social cues and study the effects of (mainly) verbal cues only. Moreover, pre-recorded videos were found to constitute a promising approach to study the perspective of bystanders or witnesses of interactions with technology.
To elaborate empathetic reactions to technology, potential interindividual differences need to be considered. De Vignemont and Singer (2006) analyzed modulatory factors, which affect empathy. Two factors of the appraisal processes are of interest for the context of technology usage: the 1) ‘characteristics of the empathizer’ and 2) ‘his/her relationship with the target’ (De Vignemont and Singer, 2006, p. 440; see also; Anderson and Keltner, 2002). Referring to the first aspect, Davis (1983) showed that gender had an impact: female participants reported greater levels of empathy than male participants. In contrast, (Rosenthal-von der Puetten et al., 2013) did not find a significant effect of gender on empathy towards their robot. They indicated a rather inconsistent state of research, which they ascribed to different definitions of empathy studies referred to, as well as to different methods and measures studies used (Rosenthal-von der Puetten et al., 2013, p. 21). In sum, there are open questions left, which further research needs to elaborate on.
Secondly, regarding the relationship with the target, Rosenthal-von der Puetten et al. (2013) focused on ‘acquaintance’ operationalized as two forms of ‘prior interaction’ with the robot: before the actual experiment started, the experimental group had interacted with the robot for 10 min, while the control group had no prior contact. Results revealed that ‘prior interaction’ had no effect on the level of participants’ empathy. In contrast to research on a certain robot, studies analyzing voice assistants need to reconsider the operationalization of prior contact. Considering that voice assistants can be regarded as a state-of-the-art technology, which has become part of the everyday lives of an increasing number of users, we suggest that the ‘relationship with the target’ seems to be more complex compared to the analysis of social robots, which are still barely used outside of laboratories. Smartphones might serve as a model. Carolus et al. (2019b) argued that smartphones constitute ‘digital companion,’ which ‘accompany their users throughout the day’ with the result that they could be more to their users than just a technological device. By transferring characteristics and outcomes known from human-human relationships to the smartphone-user relationship, they introduced the idea of a ‘digital companionship’ between smartphone users and their devices. In their study, they offered empirical support for their theoretical conceptualization, concluding their concept of companionship to be a ‘fruitful approach to explain smartphone-related behaviors’ (p. 915). The present study carries forward their idea and considers voice assistants to also be a potential ‘digital companion.’ Consequently, characteristics constituting this kind of relationship are to be focused on. From the various aspects characterizing a relationship, the way the interaction partners address each other is regarded as a first indicator offering valuable insights. The style of address, the form of greeting and the pronouns used refer to a complex system within communication, facilitating social orientation. Social relationships are expressed in the way conversational partners address each other, for example (e.g., Ervin-Tripp, 1972). In this context, the name refers to a certain individual and indicates a certain familiarity with the use of the forename strengthening the process. In this regard, names refer to a “nucleus of our individual identity” (Pilcher, 2016). Technology groups adopt these principles and give their human forenames. Amazon’s Echo is better known as ‘Alexa,’ which is actually not the name but a wake word of the assistant. Although there are more options of wake words (Echo, Amazon or Computer) ‘Alexa’ has become the popular address of the device, again indicating human preferences for an allegedly human counterpart.
Consequently, analyzing potential empathetic reactions to CAs requires considering both the users’ interindividual differences and indicators of the relationship the users might have with the device.
To summarize, the present paper refers to the concept of media equation and the idea of computers constituting social actors, which trigger social reactions in their human users originally exclusive for human-human interaction. Considering the technological progress and digital media devices pervading our lives, the cognitive, emotional as well as conative reactions to this state-of-art technology are of great scholarly and practical interest. Furthermore, empathy as a constituting factor of social cooperation and prosocial behavior and resulting social relationships is a significant focus of research, offering insights into both how technology affects humans and how these human users react, in return. Studies so far provided valuable contributions to the field but focused on objects of research, which do not closely represent current usage of digital technology and which involve a variety of social cues resulting in confounding effects. The present paper continues the analysis of the empathetic impact of technology but identifies smart speakers to be the more externally valid research object. First, they constitute ‘the next natural form of HCI’ as voice controlling adopts a basic principle of humanity. Second, voice assistants have become increasingly popular, offering a variety of applications, which end-consumers are already using in everyday life. Third, this new way of using technology affects not only the single user but results in a social usage situation as other persons present become observers or parallel users of the human-technology interaction.
To analyze an observer’s empathetic reactions to a voice assistant, this study adopts the basic idea of Rosenthal-von der Puetten et al. (2013). Thus, the first hypothesis postulates a difference between the observation of neutral and rude treatment of a voice assistant. Because the observers’ general tendency to empathize has been found to be a significant predictor of elicited empathetic reactions, this interindividually different predisposition, henceforth referred to as trait empathy, needs to be considered. Consequently, the postulated difference of the first hypothesis needs to be controlled for trait empathy:
Hypothesis 1: While controlling for the observers’ trait empathy, watching the voice assistant being treated rudely results in significantly more empathy with the assistant than watching it being treated neutrally. Following the three dimensions of empathy introduced by Rosenthal-von der Puetten et al. (2013) we distinguished three sub-hypotheses focusing on one of the three dimensions of empathy: While controlling for the observers’ trait empathy, watching the voice assistant being treated rudely results in significantly more…
H1a: …pity for the assistant than watching it being treated neutrally.
H1b: …empathy with the assistant than watching it being treated neutrally.
H1c: …attribution of feelings to the assistant than watching it being treated neutrally.
The form of address has been introduced to constitute an important characteristic of a social relationship and to contribute to social orientation. Calling the technological entity by an originally human forename is a core aspect of operating voice assistants such as Amazon Echo, which is named ‘Alexa.’ Consequently, hypothesis 2 postulates that the way the device is addressed influences an observer’s empathetic reaction–while trait empathy is controlled for again. In line with hypothesis 1, three sub-hypotheses are postulated to account for the three dimensions of empathy.
Hypothesis 2: While controlling for trait empathy, watching the voice assistant being called ‘Alexa’ results in significantly more…
H2a: …pity for the assistant than it being called ‘Computer.’
H2b: …empathy with the assistant than it being called ‘Computer.’
H2c: …attribution of feelings to the assistant than it being called ‘Computer.’
Furthermore, two explorative questions are posed referring to influencing factors for which research so far has revealed contradicting results. Inconsistent results of the role of the subjects’ gender regarding empathy with technology leads to Research Question 1: Do men and women differ regarding empathy with an assistant being treated rudely or neutrally?
The relationship with the target has been argued to be a potentially modulatory factor. However, to describe social relationships adequately a variety of variables would need to be considered. Hypothesis 2 focuses on the form of address as one constituting characteristic. Furthermore, prior contact with the device is regarded as an additional indicator. Contradicting this assumption, Rosenthal-von der Puetten et al. (2013) did not find an effect resulting from prior interaction with their research object. However, they operationalized prior interaction as a 10-min period of interaction. Focusing on voice assistants, which are more common in everyday life, allows a more externally valid operationalization when asking for prior experience in real life and outside of the laboratory. Consequently, Research Question 2 asks for the effects of experience on empathetic reactions: Does prior experience influence the empathy toward an assistant being treated rudely or neutrally?
A total of 140 participants engaged in an online experiment, ranging in age from 17 to 79 years (M = 30.14, SD = 13.9), with 64% women. Most participants were highly educated: 43% were students in higher education, 34% had finished university and 13% had finished vocational training. Regarding smart speaker experience, 51% have interacted occasionally and 40% have never interacted with one before. Only 13 participants reported to own a smart speaker (12 participants owned the Amazon device, 1 owned Google Home).
The online experiment started with a brief instruction about the broad purpose of the study and the ethical guidelines laid out by the German Psychological Association. Afterward, in a 2 × 2 experimental design, participants were randomly assigned to one out of four conditions to watch a video showing a man who prepares a meal while interacting with Amazon Echo. When his commands resulted in error messages, the man reacted either rudely or neutrally (factor 1: treatment). Furthermore, he addressed the agent by ‘Alexa: or by ‘Computer’ (factor 2: form of address).
In line with the 2 × 2 design presented above, four pre-recorded videos were used as stimulus material. In the videos, a man was preparing a meal in his kitchen. Simultaneously, he talked to Amazon Echo, which was standing on the table right in front of him (see Figure 1). He instructs the device to do several tasks like booking a hotel, asking for a train connection and sending messages. To minimize any possible influence of sympathy or antipathy, the face of the protagonist was never visible in the videos. Only his upper body was filmed. To ensure comparability of the four video conditions, the videos were produced as equally as possible regarding script, camera angle and film editing. Hence, the video set and the actor were the same across conditions. Four cameras were used to shoot, and the camera settings were not changed during or between the shooting of the different videos. In post-production, video editing was kept constant across all conditions. To ensure a controlled dialogue, voice outputs of the device were pre-programmed. We used the chat platform Dexter (https://rundexter.com/) to create a skill involving the sequences of the dialogue, which was implemented using Amazon Web Services (https://aws.amazon.com/). In contrast to the videos of previous studies, we avoided a rather unrealistic or extreme story but were guided by common usage scenarios of smart speakers (Handley, 2019).
In all four videos, the plots were basically identical. During preparation, the man’s commands became more and more complex. His rather vague commands became difficult to execute. Consequently, more and more commands failed, which allowed us to implement the treatment-factor: the man either proceeded with his neutral commands (neutral condition) or he got angry when commands failed and acted rudely (rude condition). In the neutral condition, the man recognizes his operating errors and corrects himself. He speaks calmly using neutral language. In the rude condition, the man increasingly furiously during the interaction. He scolds the device using foul names and starts yelling. Finally, the man shoves away the assistant. The form of address-factor was realized by implementing two different forms of address: either ‘Alexa’ (‘Alexa’ condition) or ‘Computer’ (‘Computer’ condition). The length of the four resulting videos were kept constant, varying 2 s only (4:49–4:51 min). In terms of content, the outlined minimal changes in the script resulted in minimal adjustments of the storyline (see Table 1). For example, the foul name the man used to address the device in the ‘computer’ condition was changed from ‘snipe’ into ‘tin box.’ To warrant a conclusive storyline the reaction of the device needed to be adapted resulting in slight differences of the interaction in the rude condition compared to the neutral condition. In a preliminary study, these differences were analyzed to warrant the comparability of the stimulus material.
A pretest was conducted to ensure 1) the assignment of the ‘rude’ and the ‘neutral’ treatment and–besides that postulated difference-2) the comparability between these two videos. 89 participants (82% women) engaged in an online experiment, ranging in age from 18 to 36 years (M = 20.57, SD = 2.57). They were evenly distributed among the four conditions. 14 participants reported to own a smart speaker.
First and to ensure rudeness vs. neutrality, a two-sided t-test for independent samples revealed significant differences in the evaluations of the man, who interacted with the device. In line with our postulated assignment, the man was rated significantly less attractive (t(87) = −12.66, p < 0.001) in the rude (M = 3.31; SD = 0.91) than in the neutral condition (M = 5.76; SD = 0.91) (Schrepp et al., 2014). The general positivity (Johnson et al., 2004) differed significantly between the rude (M = 3.68; SD = 0.81) and the neutral condition (M = 6.40; SD = 0.94), t(87) = 14.57, p < 0.001. Moreover, four more single items (see Supplementary Material for the detailed list) confirmed the postulated differences: the ‘rude condition’ was rated to be more unobjective (t(87) = 11.80, p < 0.001), impolite (t(87) = 14.71, p < 0.001.), aggressive (t(87) = 15.71, p < 0.001.) and violent (t(87) = 11.8, p < 0.001.).
Second and to ensure the comparability between these two videos, participants evaluated the device. The exact same measures used to evaluate the man were used again (see Supplementary Material). Comparing the ‘Alexa’ and the ‘computer’ condition, the evaluations of the device did not differ regarding attractiveness (t(87) = 0.15, p = 0.883) and the general positivity towards to the device (t(87) = 0.68, p = 0.500). Likewise, the semantic differentials revealed no significant differences regarding unobjectiveness (t(87) = −7.88, p = 0.433), impoliteness (t(87) = −1.34, p = 0.184), aggressiveness (t(87) = −1.21, p = 0.230) and violence (t(87) = −1.55, p < 0.125.).
To summarize, the pretest ensures the validity of the stimulus material. The rude condition did significantly differ regarding perceived rudeness, which can be ascribed to differences in the man’s behavior. Evaluations of the device, however, did not differ significantly between the ‘rude’ and the ‘neutral’ condition.
After watching the video, participants answered a questionnaire asking for 1) the empathy with the voice assistant, 2) their trait empathy, 3) their prior experience with smart speakers and 4) demographic information.
To assess empathy with the voice assistant, 22 items, based on the items used by Rosenthal-von der Puetten et al. (2013) were presented. According to the affective component of empathy, the scale includes items addressing ‘feelings of pity’ (e.g., ‘I felt sorry for the voice assistant.‘’). To assess the cognitive component, the scale incorporated items asking for ‘empathy’ (e.g., ‘I could relate to the incidents in the video’). Furthermore, an attribution of feelings to the device was assessed by ten items (e.g., ‘I can imagine that … the voice assistant suffered.’). Since we did not focus on a quantitative graduation of observers’ responses in our study, arousal was not assessed. The items were answered on a 5-point Likert scale ranging from 1 (strongly disagree) to 5 (strongly agree). Items were averaged so that higher values indicated higher levels of empathy with the assistant. Internal consistency of the scale was α = 0.82.
Trait empathy was measured using the Saarbruecker Personality Questionnaire (SPF), a German version of the Interpersonality Reactive Index (IRI) by Paulus (2009). In sum, 21 items were answered on a 5-point Likert scale (i.e., ‘I have warm feelings for people who are less well off than me.‘’). Again, items were averaged with higher values indicating higher levels of trait empathy. Internal consistency of the scale was α = 0.83.
Prior experience with voice assistants was assessed by asking if the participant had ‘ever interacted with a voice assistant’ and if he/she uses ‘a voice assistant at home.’ The answering options were ‘never,’ ‘a few times’ and ‘regularly.’ Finally, participants were asked about their age, gender and education.
To analyze the impact of treatment (factor 1: rude vs. neutral) and the impact of form of address (factor 2: ‘Alexa’ vs. ‘Computer’) on participants’ empathy with the voice assistant, three two-way ANCOVAs were conducted controlling for participants’ trait empathy as the covariate. To test hypothesis 1, while controlling trait empathy, the impacts of the rude vs. the neutral condition on empathy with the assistant were compared–with H1a focusing on pity, H1b on empathy and H1c on attribution of feelings. Regarding H1a, the covariate trait empathy was not significantly related to the intensiveness of pity with the voice assistant, F(1,135) = 1.72, p = 0.192, partial η2 = 0.01. In line with H1a, participants who observed the assistant being treated rudely reported a significantly higher level of pity with the device than participants of the neutral condition, F(1, 135) = 27.13, p < 0.001, partial η2 = 0.17 with partial eta-squared indicating a large effect (Cohen, 1988). Figure 2 shows the results. The results of H1b showed that the covariate trait empathy was again not significant, F(1,135) = 0.47, p = 0.496, partial η2 < 0.001. According to hypothesis 1b, there was again a significant main effect of the factor treatment, F(1,135) = 10.04, p = 0.002, partial η2 = 0.07, indicating a medium effect of rude vs. neutral treatment on the subscale empathy (see Figure 3).
FIGURE 2. Effects of treatment and form of address on pity for the CA (controlled for trait empathy).
FIGURE 3. Effects of treatment and form of address on empathy subscale with the CA (controlled for trait empathy).
Finally, H1c again revealed a non-significant covariate F(1,135) = 2.03, p = 0.157, partial η2 = 0.015. The way the device was treated again resulted in significant differences, F(1,135) = 4.51, p = 0.036, partial η2 = 0.032, which is interpreted as a small effect on the subscale attribution (see Figure 4).
FIGURE 4. Effects of treatment and form of address on attribution of feelings to the CA (controlled for trait empathy).
In sum, all subscales of the empathy-scale revealed significant results in line with the expectations. Rude treatment led to more empathy compared to neutral treatment.
In contrast, as Figures 2–4 show, the two different forms of address (hypothesis 2) did not result in significant differences (pity subscale: F(1,135) = 0.03, p = 0.861, partial η2 < 0.001; empathy subscale: F(1,135) = 0.02, p = 0.882, partial η2 < 0.001; attribution subscale: F(1,135) = 0.063, p = 0.429, partial η2 = 0.01). All three ANCOVAs conducted showed no significant interaction terms of the two factors treatment and form of address, neither for pity (F(1,135) = 2.43, p = 0.122, partial η2 = 0.02), nor for the empathy subscale (F(1,135) = 0.59, p = 0.444, partial η2 < 0.001) and the attribution subscale (F(1,135) = 0.88, p = 0.350, partial η2 = 0.01). Consequently, all three sub-hypotheses 2a–2c were rejected. Although the effect was not significant, participants of the rude condition reported the highest level of pity when the voice assistant was called ‘Alexa’ (see Figure 2).
To analyze potential differences between male and female participants (research question 1), three two-way ANCOVAs were conducted, again. However, factors were different than in the analyses reported before: Because the form of address was shown not to result in significant effects, it was eliminated from the following analyses. Instead, participants’ gender was analyzed as the second factor, treatment was kept as the first factor and trait empathy as the covariate. Figure 5 gives an overview of the results. Regarding pity, results revealed no significant main effect of gender, F(1,135) = 0.19, p = 0.662, η2 < 0.001. Moreover, the covariate was not significant, F(1,135) = 0.96, p = 0.329, η2 = 0.01; but the main effect of treatment was, F(1,135) = 27.14, p < 0.001, η2 = 0.17. Similarly, when analyzing the empathy subscale, the main effect of gender was not significant, F(1,135) = 2.72, p = 0.101, η2 = 0.02. Again, the covariate trait empathy was not significant F(1,135) = 0.002, p = 0.962, η2 < 0.001; but the main effect of treatment was F(1,135) = 9.14, p = 0.003, η2 = 0.06. Finally, attribution of feelings for the assistant again revealed no significant gender effect, F(1,135) = 0.09, p = 0.772, η2 < 0.001. Again, the covariate was not significant, F(1,135) = 1.74, p = 0.189, η2 = 0.01. In contrast to previous results, the effect of treatment was not significant F(1,135) = 2.46, p = 0.119, η2 = 0.02. Moreover, the interaction term almost reached significance, F(1,135) = 3.30, p = 0.07, η2 = 0.02, indicating that in the rude condition only female but not male participants reported higher attributions of feelings to the assistant. Nevertheless, to summarize results of question 1, men and women do not differ regarding all three subtypes of empathy with the assistant.
FIGURE 5. Effects of treatment and participant’s gender on empathy with the CA (controlled for trait empathy).
Research question 2 asked for the effect of prior experience with voice assistants on the reported empathy with the assistant watched in the video. In line with the preceding analyses, the approach of three ANCOVAs was retained. Furthermore, factor 1 (treatment) was retained and prior experience was added as factor 2. Again, trait empathy was kept as the covariate. Figure 6 gives an overview of the results. Regarding pity, results revealed no significant main effect of prior experience, F(1,135) = 0.3, p = 0.338, η2 = 0.01. While the covariate was not significant, F(1,135) = 1.48, p = 0.226, η2 = 0.01, the main effect of treatment was, F(1,135) = 25.49, p < 0.001, η2 = 0.16. Similarly, when analyzing the subscale empathy, the main effect of prior experience was not significant, F(1,135) = 0.58, p = 0.448, η2 = 0.02. Again, the covariate was not significant, F(1,135) = 0.38, p = 0.539, η2 < 0.01 but the main effect of treatment was, F(1,135) = 9.57, p = 0.002, η2 = 0.07. Finally, attribution of feelings for the assistant again revealed no significant effect of prior experience, F(1,135) = 1.08, p = 0.302, η2 = 0.01. Once again, the covariate was not significant, F(1,135) = 2.17, p = 0.143, η2 = 0.02, but the main effect of treatment was, F(1,135) = 4.63, p = 0.033, η2 = 0.03. To conclude, participants with or without prior experience with voice assistants do not differ regarding all three subtypes of empathy with an assistant they watched in the video.
FIGURE 6. Effects of treatment and participant’s prior experience on empathy with the CA (controlled for trait empathy).
The present study focuses on empathetic reactions to smart speakers, which have become everyday technology for an increasing number of users over the last few years. Following the approach of ‘computers as social actors’ introduced in the 1990s, we argue that the basic idea that ‘media equals real life’ is amplified by voice-based operation of devices. Voice control refers to a basic principle of humanity, which has been exclusive for human-human interactions until recently. With the adaptation of this feature, shared commonalities of human-human interactions and interactions with CAs were derived. Just like interhuman conversations can be listened to, attendees of voice-based operations within households can pay attention, for example. Therefore, research on the emotional impact of conversational agents expands its perspective and involves the impact on further attendees’ cognitive, emotional and conative reactions. Empathetic reactions as a constituting factor of social cooperation, prosocial behavior, and resulting social relationships were shown to be a promising empirical starting point (e.g., Rosenthal-von der Puetten et al., 2013; Kraemer et al., 2015). To bridge the research gap regarding 1) more real usage scenarios and everyday-relevant technology as well as 2) devices with reduced anthropomorphistic cues, this study focuses on attendees’ empathetic reaction to an interaction between a user and a smart speaker.
There are two key findings of the present research. First, compared to neutral treatment, treating the CA rudely resulted in higher ratings of empathy. Second, all the other factors analyzed did not have significant effects. Neither the participants’ characteristics (trait empathy, gender, prior experience) nor the way the assistant was addressed (‘Alexa’ vs. ‘Computer’) influenced the observers’ empathy with the device. Referring to the basic idea of Reeves and Nass (1996), who postulate that media equation ‘applies to everyone, it applies often, and it is highly consequential’ our results indicate that CAs elicit media equation effects, which are ‘highly consequential’ in terms of its independence of every influencing factor analyzed in this study. These results contradict our theoretical explanations considering both the impact of participants’ individual characteristics and the relationship people might have with technological devices. However, post-hoc explorative analyses revealed three partial results, which seem to be worth noting as they could be carefully interpreted as possibly indicating further implications. 1) Participants of the rude condition would report the highest level of pity if the voice assistant was called “Alexa” (see Figure 2). This might indicate a (non-significant) impact of the form of address. 2) Within the rude condition, only female but not male participants reported higher attributions of feelings to the CA indicating a (non-significant) impact of gender. 3) The effects on the subscale of pity were stronger than for the empathy subscale followed by only small effects for the attribution subscale, indicating that the stimuli took effect on different aspects of empathy.
Although not statistically significant, the explorative results suggest a need for further research on interindividual differences between the participants (i.e., gender) and on interindividually different relationships people have with their devices (i.e., address). Future studies need to draw more heterogenous samples to analyze these potential effects more profoundly. Moreover, the variables the present study focused on need to be further elaborated. Regarding interindividual differences beyond gender, Kraemer et al. (2015) compiled further characteristics relevant to consider. Age, computer literacy and the individual’s personality were shown to be potential influencing factors, which future research needs to transfer to users interacting with modern voice-based devices. Regarding interindividually different relationships, this study took ‘prior experience’ as a first indicator. Differentiating between no prior use vs. prior use is only the first step on the way to a comprehensive assessment of the postulated social relationship with a voice assistant stemming from interactions over time. However, referring to the concept of a ‘digital companionship’ (Carolus et al., 2019b), prior experience needs to be assessed in a more detailed way involving variables such as closeness to the voice assistant, trust in the assistant and preoccupation with it seem to be relevant characteristics of the relationship. Further constituting outcomes could be stress caused by the assistant as well as the potential to cope with stressful situations with the support of the assistant. Future research needs to incorporate these variables to analyze the effects of the relationship users have with their devices. Consequently, future research needs to reflect upon the target device the participants are confronted with. Arguing from the perspective of an established user-device relationship, future studies could use the participant’s own device as the target device–or at least a CA like the participant’s own device as similarity has been shown to be an important condition of empathy (Serino et al., 2009). Presenting a video with a rather random device a foreigner is interacting with does not fulfill these requirements convincingly. According to measures, different aspects of empathy might be activated when observing a technological device being treated rudely, compared to observing a human and animal. In addition, the level of emotional reaction (e.g., arousal) might be different. Finally, as this study did only focus on participants feelings for the technological part of the observed interaction, future research could also ask for the human counterpart. Being confronted with a device, which seems to not work properly, and which does not express empathy with the user’s struggle, future studies could also analyze the empathy participants have with the unsuccessful user of the device.
Interpretations of the results presented are faced with further methodological limitations of the study. 1) Participants only watched a video but did not observe a real-life interaction between another person and the device. Moreover, the interaction observed was not a real-life interaction but was performed by an actor resulting in questionable realism. Our ongoing development of the approach took this shortcoming into account and applied the approach to an experimentally manipulated real-life scenario. 2) To gain first insights into potentially influencing variables we controlled for trait empathy, gender and prior experience. However, research we have presented in this paper argues that further variables need to be considered. As outlined before, future studies need to include the variables operationalizing ‘characteristics of the empathizer’ and ‘his/her relationship with the target’ in a more detailed way (De Vignemont and Singer, 2006, p. 440). Furthermore, from a psychological perspective, psychological variables relevant in the context of social relationships and social interactions need to be considered, e.g., the need for affiliation, loneliness as well as affectivity or the participant’s emotional state 3) In addition, more aspects of empathy (e.g., the cognitive component according to Davis (1983); Rogers et al. (2007)). and the level of reactions should be considered by addressing a more diverse methodical approach (e.g., arousal measures, visual scales). Moreover, we limited ourselves to the analysis of empathy as the dependent variable. What we regard as a first promising starting point needs to be expanded in future studies. Especially in view of the social aspect of the use of voice assistants introduced, there are various effects to focus on, e.g., envy or jealousy, which may be elicited by the device as well as affection or attachment. 4) Lastly, and regarding the briefly introduced issue of gender stereotypes, manipulating the characteristics of the voice assistant itself are to be focused on. Changing the female voice into a male voice or changing the ‘personality’ of the device (e.g., neutral vs. rude answers given by the device itself) will be the subject of future studies.
Despite the outlined limitations, our results suggest several theoretical and practical implications. To put the study presented in a nutshell: People watching users treating voice assistants badly will empathize with the device. What sounds irrational at first, can be explained in the light of media equation and becomes a valuable insight for developers, researchers and users. First, developers should be aware of the human the final consumers’ psychological processes. Devices do not need to have anthropomorphic features to elicit social reactions in their human counterpart. Although they are consciously recognized as technological devices, they might trigger social reactions. Therefore, we argue that psychological mechanisms regulating human social life (norms, rules, schemata) are a fruitful source for developers and programmers when designing the operation of digital devices. Knowing how humans tend to react offers possibilities to manipulate these reactions–in a positive as well as in a negative way. For example, knowing that users empathize can be used to increase acceptance of misunderstandings or mistakes of devices. Furthermore, knowing that users feel for their CA can be used to counteract abusive. Developers could intentionally address the users’ tendency to transfer social rules originally established for human-human interactions to digital devices. Or, when the observer empathizes and reminds the user of an appropriate behavior. However, knowing users’ psychology also allows to manipulate them in a less benevolent way. Companies can adopt the psychological mechanism to bind users to their services and products and to maximize their profits. Second, researchers are encouraged to adopt the results presented to further analyze interwoven effects of the users’ psychology and the processing and functioning of the technological equipment. Together with further societal actors, conclusions should be drawn regarding educational programs which enable users to keep pace with technological progress and to develop media literacy skills. Competent users are key factors of our shared digital future. Third, and as a consequence of the developers’ as well as the researchers’ responsibilities, users need to accept and adopt the opportunities and be prepared for the challenges of the digital future which has already started.
The raw data supporting the conclusions of this article will be made available by the authors, without undue reservation.
Ethical review and approval was not required for the study on human participants in accordance with the local legislation and institutional requirements. The patients/participants provided their written informed consent to participate in this study.
AC: Conceptualization, Methodology, Writing-Original Draft, Writing - Review and Editing, Supervision, Project administration. CW: Formal analysis, Data Curation, Writing - Review and Editing. AT: Conceptualization, Methodology, Investigation. TF: Conceptualization, Methodology, Investigation. CS: Conceptualization, Methodology, Investigation. MS: Conceptualization, Methodology, Investigation.
This publication was supported by the Open Access Publication Fund of the University of Wuerzburg.
The authors declare that the research was conducted in the absence of any commercial or financial relationships that could be construed as a potential conflict of interest.
The Supplementary Material for this article can be found online at: https://www.frontiersin.org/articles/10.3389/fcomp.2021.682982/full#supplementary-material
Anderson, C., and Keltner, D. (2002). The Role of Empathy in the Formation and Maintenance of Social Bonds. Behav. Brain Sci. 25 (1), 21–22. doi:10.1017/s0140525x02230010
Bartneck, C., and Hu, J. (2008). Exploring the Abuse of Robots. Is 9 (3), 415–433. doi:10.1075/is.9.3.04bar
Bartneck, C., and Keijsers, M. (2020). The Morality of Abusing a Robot. J. Behav. Robotics 11 (1), 271–283. doi:10.1515/pjbr-2020-0017
Brahnam, Sheryl., and De Angeli, Antonella. (2008). Special Issue on the Abuse and Misuse of Social Agents. Oxford, UK: Oxford University Press.
Brščić, Dražen., Kidokoro, Hiroyuki., Suehiro, Yoshitaka., and Kanda, Takayuki. (2015). “Escaping from Children’s Abuse of Social Robots. In Proc. Tenth Annu. Acm/Ieee Int. Conf. Human-Robot Interaction, 59–66.
BusinessWire, (2018). Strategy Analytics: Google Home Mini Accounts for One in Five Smart Speaker Shipments Worldwide in Q2 2018, September 19, 2018. Available at: https://www.businesswire.com/news/home/20180919005089/en/Strategy-Analytics-Google-Home-Mini-Accounts-for-One-in-Five-Smart-Speaker-Shipments-Worldwide-in-Q2-2018.
Buss, D. M., and Kenrick, D. T. (1998). Evolutionary social psychology. The handbook of social psychology. Editors D. T. Gilbert, S. T. Fiske, and G. Lindzey (McGraw-Hill), 982–1026.
Cannon, A. (2017). 20 Ways Amazon’s Alexa Can Make Your Life Easier.” Wise Bread. Available at: https://www.wisebread.com/20-ways-amazons-alexa-can-make-your-life-easier (Accessed March 17, 2021).
Carolus, A., Binder, J. F., Muench, R., Schmidt, C., Schneider, F., and Buglass, S. L. (2019b). Binder, Ricardo Muench, Catharina Schmidt, Florian Schneider, and Sarah L. Buglass.Smartphones as Digital Companions: Characterizing the Relationship between Users and Their Phones. New Media Soc. 21 (4), 914–938. doi:10.1177/1461444818817074
Carolus, A., Muench, R., Schmidt, C., and Schneider, F. (2019a). Impertinent Mobiles - Effects of Politeness and Impoliteness in Human-Smartphone Interaction. Comput. Hum. Behav. 93, 290–300. doi:10.1016/j.chb.2018.12.030
Carolus, Astrid., Schmidt, Catharina., Muench, Ricardo., Mayer, Lena., and Schneider, Florian. (2018). “Pink Stinks-At Least for Men.” In International Conference on Human-Computer Interaction. Springer, 512–525.
Chin, Hyojin., Molefi, Lebogang. Wame., and Yi, Mun. Yong. (2020). Empathy Is All You Need: How a Conversational Agent Should Respond to Verbal Abuse, Proc. 2020 CHI Conf. Hum. Factors Comput. Syst. (New York, NY: Association for Computing Machinery), 1–13. doi:10.1145/3313831.3376461
Cohen, J. (1998). Statistical Power Analysis for the Behavioral Sciences. New York, NY, United States: Routledge Academic.
Cosmides, Leda., and Tooby, John. (1994). Origins of Domain Specificity: The Evolution of Functional Organization. Citeseer.
Cramer, Henriette., Goddijn, Jorrit., Wielinga, Bob., and Evers, Vanessa. (2010). “Effects of (In) Accurate Empathy and Situational Valence on Attitudes towards Robots.” In 2010 5th ACM/IEEE International Conference on Human-Robot Interaction (HRI). IEEE, 141–142. doi:10.1145/1734454.1734513
Cuff, B. M. P., Brown, S. J., Taylor, L., and Howat, D. J. (2016). Empathy: A Review of the Concept. Emot. Rev. 8 (2), 144–153. doi:10.1177/1754073914558466
Davis, M. H. (1983). Measuring Individual Differences in Empathy: Evidence for a Multidimensional Approach. J. Personal. Soc. Psychol. 44 (1), 113–126. doi:10.1037/0022-3514.44.1.113
De Vignemont, F., and Singer, T. (2006). The Empathic Brain: How, when and Why? Trends Cogn. Sci. 10 (10), 435–441. doi:10.1016/j.tics.2006.08.008
Duan, C., and Hill, C. E. (1996). The Current State of Empathy Research. J. Couns. Psychol. 43 (3), 261–274. doi:10.1037/0022-0167.43.3.261
Ervin-Tripp, Susan. M. (1972). “Sociolinguistic Rules of Address,” in Sociolinguistics. Editors J. B. Pride, and J. Holmes (Harmondsworth: Penguin), 225–240.
Feine, J., Gnewuch, U., Morana, S., and Maedche, A. (2019). A Taxonomy of Social Cues for Conversational Agents. Int. J. Human-Computer Stud. 132, 138–161. doi:10.1016/j.ijhcs.2019.07.009
Ganczarek, Joanna., Hueünefeldt, Thomas., and Belardinelli, Marta. Olivetti. (2018). From “Einfühlung” to Empathy: Exploring the Relationship between Aesthetic and Interpersonal Experience. Springer.
Go-Gulf, (2018). “The Rise of Virtual Digital Assistants Usage – Statistics and Trends.” GO-Gulf. (Blog). April 27, 2018. Available at: https://www.go-gulf.com/virtual-digital-assistants/.
Gonsior, Barbara., Sosnowski, Stefan., Buß, Malte., Wollherr, Dirk., and Kühnlenz, Kolja. (2012). “An Emotional Adaption Approach to Increase Helpfulness towards a Robot.” In 2012 IEEE/RSJ International Conference on Intelligent Robots and Systems. IEEE, 2429–2436.
Handley, Lucy. (2019). “More Than 50% of People Use Voice Assistants to ‘ask Fun Questions.’ Here’s what Else They Do.” CNBC. February 26, 2019. Available at: https://www.cnbc.com/2019/02/26/what-do-people-use-smart-speakers-for-asking-fun-questions.html.
Hegel, Frank., Torsten Spexard, , Wrede, Britta., Horstmann, Gernot., and Vogt, Thurid. (2006). “Playing a Different Imitation Game: Interaction with an Empathic Android Robot.” In 2006 6th IEEE-RAS International Conference on Humanoid Robots. IEEE, 56–61.
Hirschberg, J., and Manning, C. D. (2015). Advances in Natural Language Processing. Science 349 (6245), 261–266. doi:10.1126/science.aaa8685
Hoffman, Martin. (2001). Empathy and Moral Development: Implications for Caring and Justice. Cambridge University Press.
Hoffman, Martin. L. (1982). “Development of Prosocial Motivation: Empathy and Guilt.” In The Development of Prosocial Behavior. Elsevier, 281–313.
Hoy, M. B. (2018). Alexa, Siri, Cortana, and More: An Introduction to Voice Assistants. Med. Reference Serv. Q. 37 (1), 81–88. doi:10.1080/02763869.2018.1404391
Jacques, Richard., Følstad, Asbjørn., Gerber, Elizabeth., Grudin, Jonathan., Luger, Ewa., Monroy-Hernández, Andrés., et al. (2019). “Conversational Agents: Acting on the Wave of Research and Development.” In Extended Abstracts of the 2019 CHI Conference on Human Factors in Computing Systems, 1–8.
Johnson, D., Gardner, J., and Wiles, J. (2004). Experience as a Moderator of the Media Equation: The Impact of Flattery and Praise. Int. J. Human-Computer Stud. 61 (3), 237–258. doi:10.1016/j.ijhcs.2003.12.008
Keynes, Milton. (2020). Strategy Analytics: New Record for Smart Speakers as Global Sales Reached 146.9 Million in 2019, February 13, 2020. Available at: https://www.businesswire.com/news/home/20200213005737/en/Strategy-Analytics-New-Record-for-Smart-Speakers-As-Global-Sales-Reached-146.9-Million-in-2019.
Kinsella, Bret. (2019). Over 20% of UK Households Have Smart Speakers while Germany Passes 10% and Ireland Approaches that Milestone. Voicebot.Ai. October 11, 2019. Available at: http://voicebot.ai/2019/10/11/over-20-of-uk-households-have-smart-speakers-while-germany-passes-10-and-ireland-approaches-that-milestone/.
Kraemer, Nicole. C., AstridRosenthal-von der Pueütten, M., and Hoffmann, Laura. (2015). Social Effects of Virtual and Robot Companions. Handbook Psychol. Commun. Tech. 32, 137.
Kwak, Sonya. S., Kim, Yunkyung., Kim, Eunho., Shin, Christine., and Cho, Kwangsu. (2013). “What Makes People Empathize with an Emotional Robot?: The Impact of Agency and Physical Embodiment on Human Empathy for a Robot.” In 2013 IEEE RO-MAN. IEEE, 180–185. doi:10.1109/roman.2013.6628441
Lee, E.-J., and Nass, C. (2002). Experimental Tests of Normative Group Influence and Representation Effects in Computer-Mediated Communication. Hum. Comm Res. 28 (3), 349–381. doi:10.1111/j.1468-2958.2002.tb00812.x
Lee, Eun. Ju., Nass, Clifford., and Scott, Brave. (2000). “Can Computer-Generated Speech Have Gender? an Experimental Test of Gender Stereotype.” In CHI’00 Extended Abstracts on Human Factors in Computing Systems, 289–290.
Leite, I., Castellano, G., Pereira, A., Martinho, C., and Paiva, A. (2014). “Empathic Robots for Long-Term Interaction. Int. J. Soc. Robotics 6 (3), 329–341. doi:10.1007/s12369-014-0227-1
Leite, Iolanda., Henriques, Rui., Martinho, Carlos., and Paiva, Ana. (2013a). Sensors in the Wild: Exploring Electrodermal Activity in Child-Robot Interaction.” In 2013 8th ACM/IEEE International Conference on Human-Robot Interaction (HRI). IEEE, 41–48. doi:10.1109/hri.2013.6483500
Leite, I., Pereira, A., Mascarenhas, S., Martinho, C., Prada, R., and Paiva, A. (2013). The Influence of Empathy in Human-Robot Relations. Int. J. Human-Computer Stud. 71 (3), 250–260. doi:10.1016/j.ijhcs.2012.09.005
Lipps, Theodor. (1903). Ästhetik (Psychologie Des Schönen Und Der Kunst). Hamburg, Germany: L. Voss.
Luger, Ewa., and Sellen, Abigail. (2016). “Like Having a Really Bad PA” the Gulf between User Expectation and Experience of Conversational Agents.” In Proceedings of the 2016 CHI Conference on Human Factors in Computing Systems. 5286–5297.
Macdougall, Robert. (1910). Lectures on the Experimental Psychology of the Thought Processes. JSTOR.
McTear, M., Callejas, Z., and Griol, D. (2016). The Conversational Interface. Cham: Springer, 51–72. m2016. doi:10.1007/978-3-319-32967-3_4 Conversational Interfaces: Past and Present.
Morishima, Y., Bennett, C., Nass, C., and Lee, K. M. (2002). Effects of (Synthetic) Voice Gender, User Gender, and Product Gender on Credibility in E-Commerce. ” Unpublished Manuscript, Stanford, CA: Stanford University.
Nass, C., Fogg, B. J., and Moon, Y. (1996). Can Computers Be Teammates?. Int. J. Human-Computer Stud. 45 (6), 669–678. doi:10.1006/ijhc.1996.0073
Nass, C., and Gong, L. (2000). Speech Interfaces from an Evolutionary Perspective. Commun. ACM 43 (9), 36–43. doi:10.1145/348941.348976
Nass, Clifford., Steuer, Jonathan., Ellen, R., and Tauber, . (1994). “Computers Are Social Actors.” In Proceedings of the SIGCHI Conference on Human Factors in Computing Systems, 72–78.
Nass, C., Moon, Y., and Carney, P. (1999). Are People Polite to Computers? Responses to Computer-Based Interviewing Systems1. J. Appl. Soc. Pyschol 29 (5), 1093–1109. doi:10.1111/j.1559-1816.1999.tb00142.x
Nass, C., Moon, Y., and Green, N. (1997). Are Machines Gender Neutral? Gender-Stereotypic Responses to Computers with Voices. J. Appl. Soc. Pyschol 27 (10), 864–876. doi:10.1111/j.1559-1816.1997.tb00275.x
Nomura, T., Kanda, T., Kidokoro, H., Suehiro, Y., and Yamada, S. (2016). Why Do Children Abuse Robots? Is 17 (3), 347–369. doi:10.1075/is.17.3.02nom
Paiva, A., Leite, I., Boukricha, H., and Wachsmuth, I. (2017). Empathy in Virtual Agents and Robots. ACM Trans. Interact. Intell. Syst. 7 (3), 1–40. doi:10.1145/2912150
Paulus, Christoph. (2009). “Der Saarbrücker Persönlichkeitsfragebogen SPF (IRI) Zur Messung von Empathie: Psychometrische Evaluation Der Deutschen Version Des Interpersonal Reactivity Index. Available at: http://psydok.sulb.uni-saarland.de/volltexte/2009/2363/.”
Perez, Sarah. n. d. (2019). Over a Quarter of US Adults Now Own a Smart Speaker, Typically an Amazon Echo.” TechCrunch (Blog).Accessed November 17, 2019. Available at: https://social.techcrunch.com/2019/03/08/over-a-quarter-of-u-s-adults-now-own-a-smart-speaker-typically-an-amazon-echo/. doi:10.1037/e613692010-001
Petrock, Victoria. n. d. (2021). US Voice Assistant Users 2019.” Insider Intelligence. Accessed March 17, 2021, Available at: https://www.emarketer.com/content/us-voice-assistant-users-2019. doi:10.21236/ada463921
Pilcher, J. (2016). Names, Bodies and Identities. Sociology 50 (4), 764–779. doi:10.1177/0038038515582157
Porcheron, Martin., Fischer, Joel. E., McGregor, Moira., Brown, Barry., Luger, Ewa., Candello, Heloisa., et al. (2017). “Talking with Conversational Agents in Collaborative Action.” In Companion of the 2017 ACM Conference on Computer Supported Cooperative Work and Social Computing, 431–436.
Porcheron, Martin., Fischer, Joel. E., Reeves, Stuart., and Sarah Sharples, . (2018). “Voice Interfaces in Everyday Life.” In Proceedings of the 2018 CHI Conference on Human Factors in Computing Systems, 1–12.
Purington, Amanda., Taft, Jessie. G., Sannon, Shruti., Bazarova, Natalya. N., and Taylor, Samuel. Hardman. (2017). “Alexa Is My New BFF” Social Roles, User Satisfaction, and Personification of the Amazon Echo.” In Proceedings of the 2017 CHI Conference Extended Abstracts on Human Factors in Computing Systems, 2853–2859.
Pyae, Aung., and Joelsson, Tapani. N. (2018). “Investigating the Usability and User Experiences of Voice User Interface: A Case of Google Home Smart Speaker.” In Proceedings of the 20th International Conference on Human-Computer Interaction with Mobile Devices and Services Adjunct, 127–131.
Reeves, Byron., and Nass, Clifford. (1996). The Media Equation: How People Treat Computers, Television, and New Media like Real People. UK: Cambridge university press Cambridge.
Riek, Laurel. D., Rabinowitch, Tal-Chen., Chakrabarti, Bhismadev., and Robinson, Peter. (2009). “Empathizing with Robots: Fellow Feeling along the Anthropomorphic Spectrum.” In 2009 3rd International Conference on Affective Computing and Intelligent Interaction and Workshops, 1–6. IEEE.
Rogers, K., Dziobek, I., Hassenstab, J., Wolf, O. T., and Convit, A. (2007). Who Cares? Revisiting Empathy in Asperger Syndrome. J. Autism Dev. Disord. 37 (4), 709–715. doi:10.1007/s10803-006-0197-8
Rosenthal-von der, P., Astrid, M., Kraeämer, Nicole. C., Hoffmann, Laura., Sobieraj, Sabrina., and Eimler, Sabrina. C. (2013). An Experimental Study on Emotional Reactions towards a Robot. Int. J. Soc. Robotics 5 (1), 17–34.
Rosenthal-Von Der, P., Astrid, M., Schulte, Frank. P., Eimler, Sabrina. C., Sobieraj, Sabrina., Hoffmann, Laura., et al. (2014). Investigations on Empathy towards Humans and Robots Using FMRI. Comput. Hum. Behav. 33, 201–212.
Salvini, Pericle., Ciaravella, Gaetano., Yu, Wonpil., Ferri, Gabriele., Manzi, Alessandro., Mazzolai, Barbara., et al. (2010). How Safe Are Service Robots in Urban Environments? Bullying a Robot.” In 19th International Symposium in Robot and Human Interactive Communication. IEEE, 1–7.
Sansonnet, J.-P., Leray, D., and Martin, J.-C. (2006). “Architecture of a Framework for Generic Assisting Conversational Agents.” In International Workshop on Intelligent Virtual Agents, 145, 156–56. Springer. doi:10.1007/11821830_12
Schrepp, Martin., Hinderks, Andreas., and Thomaschewski, Jörg. (2014). International Conference of Design, User Experience, and Usability. Springer, 338–392.Applying the User Experience Questionnaire (UEQ) in Different Evaluation Scenarios.
Serino, Andrea., Giovagnoli, Giulia., and Làdavas, Elisabetta. (2009). I Feel what You Feel if You Are Similar to Me. PLoS One 4 (3), e4930. doi:10.1371/journal.pone.0004930
Sundar, Harshavardhan. (2020). Locating Multiple Sound Sources from Raw Audio. Amazon Science, April 27, 2020, Available at: https://www.amazon.science/blog/locating-multiple-sound-sources-from-raw-audio.
Tan, Xiang. Zhi., Vázquez, Marynel., Carter, Elizabeth. J., Morales, Cecilia. G., and Steinfeld, Aaron. (2018). “Inducing Bystander Interventions during Robot Abuse with Social Mechanisms.” In Proceedings of the 2018 ACM/IEEE International Conference on Human-Robot Interaction, 169–177.
Tapus, A., Mataric, M., and Scassellati, B. (2007). Socially Assistive Robotics [Grand Challenges of Robotics]. IEEE Robot. Automat. Mag. 14 (1), 35–42. doi:10.1109/mra.2007.339605
Titchener, Edward. Bradford. (1909). Lectures on the Experimental Psychology of the Thought-Processes. Macmillan. doi:10.1037/10877-000
Keywords: conversational agent, empathy, smart speaker, media equation, computers as social actors, human-computer interaction
Citation: Carolus A, Wienrich C, Törke A, Friedel T, Schwietering C and Sperzel M (2021) ‘Alexa, I feel for you!’ Observers’ Empathetic Reactions towards a Conversational Agent. Front. Comput. Sci. 3:682982. doi: 10.3389/fcomp.2021.682982
Received: 19 March 2021; Accepted: 05 May 2021;
Published: 28 May 2021.
Edited by:
Benjamin Weiss, Hanyang University, South KoreaReviewed by:
Iulia Lefter, Delft University of Technology, NetherlandsCopyright © 2021 Carolus, Wienrich, Törke, Friedel, Schwietering and Sperzel. This is an open-access article distributed under the terms of the Creative Commons Attribution License (CC BY). The use, distribution or reproduction in other forums is permitted, provided the original author(s) and the copyright owner(s) are credited and that the original publication in this journal is cited, in accordance with accepted academic practice. No use, distribution or reproduction is permitted which does not comply with these terms.
*Correspondence: Astrid Carolus, YXN0cmlkLmNhcm9sdXNAdW5pLXd1ZXJ6YnVyZy5kZQ==
Disclaimer: All claims expressed in this article are solely those of the authors and do not necessarily represent those of their affiliated organizations, or those of the publisher, the editors and the reviewers. Any product that may be evaluated in this article or claim that may be made by its manufacturer is not guaranteed or endorsed by the publisher.
Research integrity at Frontiers
Learn more about the work of our research integrity team to safeguard the quality of each article we publish.