- 1WellthLab, Electronics and Computer Science, University of Southampton, Southampton, United Kingdom
- 2Herbert Wertheim School of Public Health and Human Longevity Sciences, University of California, San Diego, CA, United States
This paper presents the Experiment in a Box (XB) framework to support interactive technology design for building health skills. The XB provides a suite of experiments—time-limited, loosely structured evaluations of health heuristics for a user-as-experimenter to select from and then test in order to determine that heuristic’s efficacy, and to explore how it might be incorporated into the person’s life and when necessary, to support their health and wellbeing. The approach leverages self-determination theory to support user autonomy and competence to build actionable, personal health knowledge skills and practice (KSP). In the three studies of XB presented, we show that with even the short engagement of an XB experiment, participants develop health practices from the interventions that are still in use long after the intervention is finished. To situate the XB approach relative to other work around health practices in HCI in particular, we contribute two design continua for this design space: insourcing to outsourcing and habits to heuristics. From this analysis, we demonstrate that XB is situated in a largely under-explored area for interactive health interventions: the insourcing and heuristic oriented area of the design space. Overall, the work offers a new scaffolding, the XB Framework, to instantiate time-limited interactive technology interventions to support building KSP that can thrive in that person, significantly both post-interventions, and independent of that technology.
Introduction
In this paper, we present the Experiment in a Box framework, or XB for short, for designing interactive tools to support a person building knowledge skills and practice (KSP) to maintain their own health and wellbeing. By knowledge, we mean awareness, understanding, and wisdom related to the one’s body as it constantly adapts across time and in context toward enabling a person to move toward desired adaptive states (e.g., being fit, reducing stress, maintaining a healthy weight, etc). By skills, we are referring to one’s capacity to regulate and “tune” oneself in and to context toward desired adaptive states (schraefel and Hekler, 2020). By practice, we are referring to two senses of the term: repetition and commitment. Practice in the first sense is the number and frequency of the repetitions of a skill necessary to become adept at the knowledge and skills necessary in this case to build and maintain health. That repetition, in the second sense, as a formal practice frames this repetition as an ongoing part of one’s life, of actively, continually and deliberately engaging in developing and refining desired knowledge and skills toward desired states. The XB, as we detail in Related Work below, addresses a gap in the research around how to design interactive technology to help people build these resilient (Feldman, 2020) adaptable health KSP that can be actioned across health-challenging contexts, without requiring that technology to be perpetually necessary. In other words, we want to design technology to support building health KSP so people can get off that technology, and thrive with those KSP skills. Drawing on recent work that suggests framing health technology within a continuum from outsourcing to insourcing health skills (schraefel et al., 2020), we show that most of the current research and commercial interactive health technology supports the outsourcing end of health tech design—that is, giving the problem to a third party to manage, including especially around health, to automated interactive technology devices and services, from step counters or follow along workouts and their associated data capture and visualisations, to coordinated product and service delivery.
Further, within the increasingly pervasive discourse of behavior change/habit building frame, many of the current technologies not only seek to outsource habit-building but to then also seek to cultivate a person’s reliance not just on the technology but also context to drive these habits-as-healthy behaviour-change. For example, it is increasingly common to outsource one’s exercise to a follow-along program, or in some cases to programs that also monitor various biometrics, where tools suggest strategies based on that data for making progress (“TrainingPeaks | New Year. New Focus.” n. d.; ‘The Sufferfest: Complete Training App for Cyclists and Triathletes’ n. d.). While there is great potential for such outsourced, habit-formation-oriented technologies, to date, the hope has not been observed in reality. (Riley et al., 2011; West et al., 2012; Lunde et al., 2018; McKay et al., 2018). A key reason for this gap is that an outsourced, habit-formation orientation to behavior change asks for a very heavy lift for both the technology and for context to drive a person into desired adaptive states. This is difficult based on the inherent complexity of human behavior and health, which has been summarized elsewhere in terms of recognizing three factors that impact behavior and health: context, timing, and individual differences (Hekler et al., 2019; Chevance et al., 2020; Hekler et al., 2020; ; ). These dynamics require what we frame as constant adaptivity in context (schraefel 2020). These complexities establish the need for outsourced, habit-formation oriented technologies to be highly adaptive to context and changing circumstances—which is somewhat the antithesis of habits as context specific, context dependent. Most current systems are not broad enough or expert enough to be sufficiently adaptive, which, we contend, is the primary reason behind the gap between the hope and reality. For instance, most tools cannot be responsive and supportive to a simple question like what to do when it’s too late to go for the planned run; what are options when there are no stairs to climb? These current outsourced systems are also high cost in terms of their subscriptions, the associated special gear (Ji et al., 2014), and the need for complex, often data invasive “personalization” algorithms (Hekler et al., 2018; Hekler et al., 2020). Thus, while there is possibility and promise, considerable work is needed before this form of responsive health practices could become a reality.
An alternative to outsourced health practices/health behavior change is emerging under the auspices of personal science (Wolf and De Groot, 2021) or “self-study” (Nebeker et al., 2020). This approach emphasizes what we call insourcing, meaning the cultivation of KSP within the person, thus establishing (and supporting) the person as the driver and lead for achieving desired states, not the technology. The dominant way in which personal science/self-study are being supported via technology, particularly in the human-computer interaction (HCI) literature, is through extensions of randomized control trial logic into N-of-1 cross-over trials, which involve randomizing, within person and across time, the delivery or not of an intervention relative to some meaningful comparator (Karkar et al., 2017). These approaches focus on gathering quantifiable data to refine an outsourced prescription for health activities, ranging from workout plans (Agapie et al., 2016) to sleep strategies (Daskalova et al., 2016). Implicit in many of these approaches still remains an emphasis on either a single action behavior change, such as avoiding certain foods that appear linked with undesired symptoms, or cultivating a prescribed habit and routines, such as specific sleep hygiene techniques.
Our goal with the XB framework is to extend personal science and insourcing by shifting emphasis from behavior change/habit formation to, instead, cultivation of KSP and what we frame as heuristics. By heuristics, we mean a set of general principles, grounded in prior knowledge from both science and lived experience, that can guide effective adaption of the person, across contexts, toward desired adaptive states. The XB framework seeks to cultivate personal health heuristics by blending the broad categories of outsourced health and wellbeing plans and programs (prior knowledge based on science) as initial generic heuristics with the insourcing of personal evaluation found in N-of-1 studies in order for a person to test and customize those heuristics for themselves, to work across contexts.
The focus of the XB framework is, therefore, to help build the necessary health KSP so that a person 1) can answer the question “how do you feel” as a meaningful self-diagnostic and 2) from this self-diagnostic, apply associated health and wellbeing KSP toward selecting appropriate actions in context to feel better. As pointed to above, we frame this ability to select and adapt appropriate KSP to build and maintain health and wellbeing across contexts as tuning (schraefel and Hekler, 2020). The term draws on the analogy of tuning as an instrument or machine: aligning the components of a mechanism so that they resonate together, reinforcing each other toward better performance. The strings of a guitar, for example, are tuned to particular pitches, harmonically, relative to each other enabling them to produce music within the frames of those harmonics; that instrument itself may be tuned to a standard frequency so that it resonates harmonically with other instruments as well. In a tuned engine, all components are likewise synchronized, thus producing more power, more efficiency.
Our goal in presenting the XB framework here is two-fold. First, we seek to expand the design space of health technologies to suggest the possibility for solutions that work across both the spectrums of outsourcing to insourcing, and across the dimensions of cultivating behavior change/habit formation to cultivating personal health heuristics via building and testing associated health KSP. Second, with the XB framework specifically, our goal is to help designers and individuals develop interventions that will support dynamic self-tuning for one’s healthful resilience, across contexts. In the following sections, we present a more detailed discussion of the related work motivating the XB approach. We then present three exploratory studies developed to help triangulate on the set of features that are the fundamentals of the XB framework.
These three studies were conducted explicitly to explore the XB model, framed via the ORBIT model (Czajkowski et al., 2015) notion of “proof-of-concept” studies. In particular, we follow the ORBIT approach of Defining, Refining, and Testing for a meaningful signal. Note that, as discussed in ORBIT, this is an appropriate methodology for this stage of the research process present here. From the studies reported below, we can say convincingly that we appear to be getting a clear signal across these three studies. In accordance with the ORBIT model, this finding can be used to then justify more rigorous evaluation relative to a meaningful compactor. Our studies were explicitly conducted iteratively and consecutively to enable exploration and refinement of the XB approach. The fact that similar conclusions were being drawn even across disparate samples, with the first focused on an ad agency, the second on a university setting, and the third with an open call on social media, point toward some degree of consilience and, thus, increased confidence in the value of continuing this line of work toward more formal and rigorous testing, such as randomized controlled trials.
We conclude by summarizing our findings and identifying opportunities for future work that offer some reflections on open questions about XB phases, and also challenges for use in supporting health exploration for group/cultural health practice and infrastructure, beyond the individual. Overall, the XB framework offers a set of novel parameters, such as insourcing health knowledge skills and practice thus opening new areas in the HCI health design space to be explored.
Related Work
Supporting health and wellbeing is a burgeoning research area in HCI, from addressing depression, anxiety, or bipolar health issues to new models of digitally enabled healthcare delivery e.g., (Blandford, 2019; Sanches et al., 2019). In the following sections situating our work relative to this context, we further develop both the insourcing to outsourcing design continuum (schraefel et al., 2020) (schraefel et al., 2021) and the habits to heuristics continuum outlined above as new vectors for contextualizing the health design space.
Insourcing to Outsourcing Lens of Interactive Health Tech
Outsourcing (Troacă, 2012) refers to the subcontracting out of expertize or production to reduce costs and improve efficiencies in industry. Tim Ferris’s book, The Four Hour Workweek (Ferriss 2009) made the concept more personal: one could outsource to a third party tasks from managing one’s calendar to one’s laundry to free up one’s time for more time-valuable processes. Outsourcing also outsources oversight of these processes, which can lead to issues from cost overruns and poorer quality to abuse, from overpriced contracts and substandard services (Brooks, 2020), to suicides at outsourcing factories (Heffernan, 2013) and inmate abuse, motivating the recent shutting down in the United States of outsourced federal prison services (Sabrina and sadie, 2021).
As a complement to outsourcing, we proposed “insourcing” (schraefel et al., 2020) to consider bringing tasks in-house, restoring them to the entity-as-owner. Such insourcing in physical activity would include a person undertaking to learn how to build skills for themselves to achieve and maintain fitness, rather than relying on a trainer to manage programming their fitness experience. Insourcing also has costs: time and money to gain skills, funds for associated equipment, time and resources to practices skills; risks that one’s expertize may not be sufficient on its own to support good strategies for any associated goals. On the plus side, insourced knowledge skills and practice can enable one to be more responsive to challenges, for much longer. A recent workshop call (schraefel et al., 2021), focusing on exploring the insourcing end of the insource-outsource continuum makes clear that there is not a value judgment in insourcing or outsourcing. Insourcing<→Outsourcing is, instead, a continuum: we often flow between these points. For example, most of us outsource production, management and distribution of vegetables from field to home to grocery stores—we outsource that expertize, time and risk and accept its limitations in choice and quality. We may also insource some small part of that process from time to time, as amateur farmers, working on urban community gardens, or growing a tomato in a pot over the summer, sufficient to dress the occasional salad.
This continuum of insourcing to outsourcing is one lens we can use to interpret emphasis in health tech both commercially and in interactive tech for health research. As we show in the following section, while there is a spread across the continuum, the preponderance of work clusters around outsourcing. Through the pandemic, such outsourcing has only escalated: sales of Peleton bikes and subscriptions to associated follow-along anytime-workouts have significantly increased since pre-COVID (Griffith, 2020). Subscription-based guided health services from yoga to meditation have also risen (Lerman, 2020). A trait also strongly associated with these approaches is quantitative data tracking, where sensors capture biometric information while associated apps translate these into scores or performance ratings. Step counters like Fitbit hardware and associated apps are a great example of this approach: the user tacitly agrees to follow the advice of the device which offers a simple, understandable directive: “get X steps on this counter within a day”. It is easy for a person to determine success or failure. Over the past 5 years in particular, more sensors have been integrated into single devices, from phones to watches, gathering even more types of biometric data, less obtrusively: one watch can capture heart rate, standing/moving time, sleep time movement pace, incorporated of course with where and when each beat and step is taken1. These measures offer a happy blending between computer scientist competence and the medical communities’ methodologies. As computer scientists, we are very good at creating ever cheaper, lower power, longer-lasting finer grained sensors. In the medical and sports science communities, we regularly make our cases on health prescription by connecting quantified measures with outcomes: blood pressure, heart rate, age, girth correlate to outcomes from disease prevention approaches [e.g. (Barry et al., 2014; Stevens et al., 2016)] to athlete training programs (Allen et al., 2019).
Beyond the off-the-shelf apps and tools like sports watches and health tracking apps that clearly define what can be counted and correlated, the Quantified Self (QS) communities have demonstrated the value of being able to correlate an amazing variety of measures to see more consistent data to both understand and adapt more varied practices to support desired outcomes (Prince, 2014; Barcena et al.), from room temperature to blood glucose, heart rate, weight as part of deliberate self-study. This QS community is heterogeneous. While some QS-oriented people collect more or less all kinds of data (Ajana, 2017), and some have more specific goals, such as monitoring sports performance over years, to help reflect on their practice or tune it (Dulaud et al., 2020; Heyen, 2020), others carry out more deliberate experiments, usually to understand a possible correlation. That is: a formal protocol is developed; it is executed over a specific time; the data is gathered; and then analyzed toward a conclusion (Choe et al., 2015a).
In more formal research in HCI, the outcome of such exploration has often been to support informing an outsourced outcome: a specific health prescription (Consolvo et al., 2008), supporting data-derived insights (Bentley et al., 2013) and/or using self-experimentation [e.g. (Hekler et al., 2013; Agapie et al., 2016; Duan et al., 2013; Daskalova et al., 2016; Pandey et al., 2017; Lee et al., 2017; Kravitz et al., 2020)] to support a decision to adopt a practice. Early days in this research (circa 2008) provided largely prescriptive interventions, with research focused on persuasive technology approaches of how to design the interaction to support translating a person’s actions with the intervention into a clearly prescribed habit, such as “walk 10 k steps”; “stand every hour”; “go to the gym” [e.g. Ubifit (Consolvo et al., 2008)]. Over the past decade, inspired by QS, personal informatics and n-of-1 studies, health practice designs in self-study have moved toward focusing increasingly on using one’s own data for personal sense-making. Bentley et al.’s (Bentley et al., 2013) Health Mashups attempts to find and highlight possible correlations between quantified representations of a person’s activities to help a person see in the data where they may need or want to do things differently for their health. Intriguingly, one is responding to the data picture of one’s self rather than necessarily a perceived or felt experience.
Once one identifies something to do, such as going to the gym when a pattern shows one doesn’t move, Hekler and colleagues’ DIY’ing strategies (Hekler et al., 2013) help develop and assess making the practice work in one’s life, while Agapie et al.’s workout system crowdsources tailored versions of that activity, again telling someone what to do (Agapie et al., 2016), and Daskalova et al.’s work helps assess and tune it (Daskalova et al., 2017). In related, but more disease-oriented health care, Duan and colleague’s (Duan et al., 2013) work uses formalized experimental N-of-1 designs to find triggers for negative effects in order to eliminate them. Work lead by Panday (Pandey et al., 2017) translates this methodology into a generalized architecture to carry out individually driven, but widely distributed formal N-of-1 studies within any area of human performance to answer “does X do Y” ? There is a strong emphasis, among this self-experimentation side, for producing rigorous statistical estimates (Lee et al., 2017; Phatak et al., 2018; Kravitz et al., 2020)—but that level of rigor is not always appropriate, particularly for early practices when a person is in effect, simply trying to determine even if they want to try out a given health behavior, little own commit to it for the rest of their lives.
By looking at work in HCI in one exemplar health area, sleep self-study, we see these clusters more sharply. Here, most work has focused on blending what to do in terms of sleep practice via tracking and analysis of associated sleep data [SleepTight (Choe et al., 2015)], building peripheral awareness of a recommended sleep enhancing protocol [ShutEye (Bauer et al., 2012)], and making recommendations of how to refine a sleep protocol based on external expert data analysis/pattern matching [SleepCoacher (Daskalova et al., 2016)]. In other words, the person outsources what to do about their sleep to an expert, data-driven guidance system. The prescription seems personalized based on one’s own data, and the success or failure is measured by adherence and improved performance based on measured data. In what is framed as “autoethnography” around sleep, Lockton and colleagues recently focused on participants designing probes that would let them reflect on aspects of sleep important to them, individually (Lockton et al., 2020). Here, the emphasis of the work is on reflection on current practice rather than changing it to sleep better. But even here, the majority of designs in the concluding “insights” section come back to how to re-present data already generated in sleep trackers, for instance, to look at patterns of sleep in different contexts. These approaches tend to assume these data are valuable, informative, sufficient, trustable. We let the data tell us how we are doing, and for many who adopt these approaches, these assessments are taken very seriously. Research around sleep tracking shows increased anxiety around sleep performance as a result is not uncommon (Baron et al., 2017). But research in sleep trackers also shows us how inaccurate they are (Ameen et al., 2019; Tuominen et al., 2019; Louzon et al., 2020). This is a conundrum for design, raised previously in a discussion about the “accuracy” of scales for supporting weight management (Kay et al., 2013): are we achieving what we hope to achieve for health with this data-led approach? Do we need to better qualify what the data presented is actually able to tell users about their experience, and their progress in a health journey?
Research in HCI has also shown that not everyone who might benefit from health support from interactive technology finds these quantification/tracking data-dominant approaches appealing (Epstein et al., 2016). This efficacy seems to be mostly for people already committed to exploring either their general health (Clawson et al., 2015) or to address a very specific health need (Karkar et al., 2016) and that even within that population, abandonment of trackers and self-tracking is high (Sullivan and Brown 2013) (Stawarz et al., 2015). Thus, there are at least two communities where the potential benefit of interactive technological interventions has not created benefit: those who find quantification a non-starter; and those who abandon it—without necessarily building a sustainable practice with it. There is also a related problem; even among ardent trackers, the way these technologies are designed sets up the very high risk that, if/when the technologies are removed, the desired change will also go away. This general pattern was shown in prior analogous work related to gym membership (Hekler et al., 2013). In particular, a previous physical activity intervention, which was shown to increase physical activity relative to a control condition in a randomized controlled trial (Buman et al., 2011), included the option of gym memberships, though not everyone in the study used the gym membership. While there were people who increased physical activity both using the gym and not using the gym, it was only the people who did not use the gym who maintained their physical activity when the intervention was over and the gym membership was taken away (Hekler, 2013). Returning to fitness programs and trackers, what happens on day 91, after the 90-days fitness follow along? This question of course reveals a concern dominant in this paper: what do we want our interactive health technologies to enable? To support building device reliance or independence? Another way of framing this question may be: does outsourcing to health technology make us more cyborg (Haraway, 1991) than human? Again, that continuum—raw to cyborg—is not meant as a value judgment, but as a way to be, perhaps, more deliberate and specific in our design choices.
For those who have abandoned or do not engage in data-dominant health support, we suggest that the tracking/quantification may not itself be the main issue for resistance, but rather the outsourcing of a practice to a quantifiable data driven approach, a point that is implied with Wolf and DeGroot’s framing on personal science [note, Wolf was one of the originators of the notion of Quantified Self and, in this piece, he is signaling a shift away from the emphasis on quantification (Wolf and De Groot, 2021)]. The tools, in the end, can be perceived to be too brittle or narrow at this point in their evolution to be more generally useful. Outsourcing is not just about giving monitoring of biometrics over to devices; it also needs the data-driven system to tell one how well they are doing and, what to do. In the authors’ work with athletes, it’s not uncommon to hear of dissonance between an athlete’s watch telling them about their recovery state and how they themselves think they are feeling. In the sleep trackers, it’s not uncommon for people who wake feeling ok, to be frustrated and therefore not seeing their deep sleep change. We suggest it may be that the perceived quality of the assessed states or recommended practices are not sufficiently responsive for the cost of perpetual tracking. Periodicity, messo, macro, micro cycles familiar in sports science is also largely absent. An implicit quality of most health devices is that they have no time limits, the implicit design being, the intervention will last forever. That too has been noted (Churchill and schraefel, 2015) as a frustrating assumption in such outsourced health tech design.
How Do You Feel How You Feel vs. What Does the Data Say?
There is a challenge with insourcing-oriented designs: how to support internalizing awareness and knowledge of personal state? When we rely on outsourcing, on data to tell us how we are performing, we do not have to determine how we feel; we do not have to focus on building the pathways between our own in bodied sensors and the machines’. Building up these lived connections—to be able to answer “how do you feel how you feel” is non-trivial on at least two levels. First, some people have not, at least in recent memory, had the experience of, for example, a good night’s rest: they wake every day under-slept, roused by an alarm, instead of waking on their own. If we do not know what it feels like to be well-rested, or we cannot perceive these differences in terms of affect (Zucker et al., 1997; Erbas et al., 2018) then we lack a yardstick both to judge actions against, and make decisions about the perceived cost. Without this internalized yardstick and sense of difference, any information provided on plausible difference (you’ll be well-rested) is a purely intellectual value. Thus, the plausible benefits one would experience are not part of the calculations for choosing different health actions when insourcing is not present (Vandercammen et al., 2014). Second, while humans have incredibly sensitive dynamic sensing systems, some people do not have the connection between that sensory input and their experiences well-formulated. They may be interoceptively (Tsakiris and Critchley, 2016; Murphy et al., 2019) weak. That is, without the lived allostatic experience (Kleckner et al., 2017) that connects, for example, positive movement with good mood, or nutritious food with cognitive effectiveness, the person does not have, literally, a mental map to help navigate toward healthful practices and associated experiences. Those pathways have not been built. This is critical based on new theories around subjective states and our understanding on what we feel and why. For example, there is increasing evidence suggesting that emotions are not innate to our biology or brain but, instead, appear to be a psychological construction of the interaction between interoception and context and history (Lindquist et al., 2012). The implication of this is that, if one does not develop a robust practice of interoceptive awareness in context, one quite literally, will not be able to meaningfully feel (Lindquist et al., 2012). As classic work in neuroscience and social psychology illustrates, this is critically important as, there is increased recognition that it ultimately our experience of emotions that guide on in the actions we take (Spence, 1995; Haidt, 2012). Thus, training on how to feel, meaning how to meaningfully experience and interpret the signals that flag up our state across contexts, is a critically important and, as of yet, under-studied area of work for advancing health. This is the domain that we are exploring in the XB framework.
Heuristics as Complement to Habits
Just as computer science and medicine synergize around capture and use of quantified data for health interventions, psychology and computer science in HCI meet at Behavior Change2. A key embodiment for behavior change in HCI is the habit: identifying (Pierce et al., 2010; Oulasvirta et al., 2012), breaking (Pinder et al., 2018), and especially forming (Consolvo et al., 2008; Stawarz et al., 2015) habits are strong focii of that engagement. The focus on habits is understandable: while the space of sustaining a health practice is multifaceted, including “motives, self-regulation, resources (psychological and physical), habits, and environmental and social influences” (Kwasnicka et al., 2016), habits themselves are highly amenable to what computational devices can support: context triggered cues, just in time reminders and compliance tracking (Rahman et al., 2016; Lee et al., 2017). For context, in psychology, habits are described as behaviors that, once developed, are enacted automatically, that is without much conscious thought, and within specific contexts (Wood and Rünger, 2016). This context-based automation is valuable and important as habits can facilitate appropriate rhythms for achieving meaningful health targets. For instance, “at night while watching the TV, floss teeth”. The TV, the night, the floss container at hand, all contribute to the cues to trigger that practice. Such habits are extremely helpful: who wants to think deeply every day about the precise practice of dental hygiene? But what if the context changes? If the context switches, such as while traveling, or the floss runs out? Habits, by their very specificity, do not support adaptivity, yet many health contexts require a broader range to support the goals of a practice. For instance, if the intention is to maintain fitness, and a device only supports “go for a walk after breakfast” what happens when one is where that walk is not an option? Where are alternatives? To address these challenges, we propose expanding “habit” as a single design point, into a continuum that includes heuristics.
Heuristics, like habits, are also well known in HCI, specifically as a method of usability evaluation where a set of general processes or principles (the heuristics) are provided to be used by an evaluator inspecting an interaction for those processes, like “visibility of system status” and “recognition rather than recall” (Nielsen and Molich, 1990). Heuristics like habits can be internalized, but here rather than specific autonomous actions, they are integrated as functional principles to be instantiated based on personal knowledge and skills that guide a person’s practice in any given moment. As such, they are a useful mechanism for insourcing dynamic, responsive, healthful practices. For example, in strength training, there is a heuristic: when in doubt, choose compound movements. Such movements work most of the body and are therefore “go to” work for general physical preparedness (Verkhoshansky et al., 2009). As part of building an insourced practice, one then builds up experience with a lexicon of movements that will support that heuristic: pull ups, squats, rows, deadlifts and so on. Similarly, survivalist Bear Grylls is famous for his heuristic: “Please Remember What’s First: protection, rescue, water, food” (Kearney, 2018). Success with this life-saving mnemonic depends however, on knowing what protection is in the desert vs. the jungle. Likewise, one may need practice with the skills to make a fire to melt snow for water in the tundra, ward of other creatures, or be noticed for rescue.
Deliberate Choice in Habit-Heuristic Continuum
In weighing design choices for health at one side of this continuum, habit formation requires intention (Prochaska and Velicer, 1997; Hashemzadeh et al., 2019), what we might frame as insourcing of motivation, to put the effort into repeating the task sufficiently for it to become automated/habituated. Assuming that motivation exists, the triggers for firing habits as per the references above can be supported by technology (as per Stawarz et al., 2015), and thus their development may be effectively outsourced. However, like the data-prescriptive outsourced approaches discussed above, habits do not deliberately build knowledge skills or practice for executing options either. Heuristics do focus on building those skills. While heuristics are more adaptive, as more context-agnostic than habits, they also require potentially more resources to insource in order to develop the variety of experiences and expertizes to be independent of external guides. Thus, the habit-heuristic continuum opens up a way to let designers ask more explicitly both what they are trying to instantiate in a person, and what the costs/trade-offs of either approach may be.
Behavior Change vs. Knowledge Skills and Practice in Continua
Another difference between habits and heuristics that interactive health tech may leverage is that, while habits are strongly associated with behavior change, heuristics are not. Heuristics are more associated with knowledge building and application: to have a general rule on how to approach a problem is different than a need to change in a very particular manner. While the ends may often be similar, the pathways, and thus design processes open to explore, are different, offering HCI additional options for designing toward successful health engagement.
XB—Experiment in a Box
Based on the above related work, we see considerable open space to explore the design and development of insourcing health heuristics to support building knowledge, skills and practice for personal health resilience. The following work presents XB as just such an exploration of this space. In the next sections we present an overview of the XB approach, several exploratory studies to define and confirm its key features, and then present an XB framework to enable general XB development by others that incorporates insights from these exploratory studies.
The XB approach provides a structure for translating a general health heuristic into a personal, practicable, robust health heuristic. For many, generic health heuristics are known, such as sleep hygiene rules, but they can also be hard for people to apply in their own lives. The self-determination theory postulates that to make the effort—to have the motivation—to engage in a health practice, a person’s autonomy, competency and relatedness must be supported, and when they are, increased feelings of vitality ensue (Stevens et al., 2016). Therefore, with the XB approach we can create simple, short-duration experiences, “experiments” that empower people to test out a health heuristic and to build competency through the testing process. By experiment, we are explicitly using the word in a colloquial sense, which we will unpack more momentarily. Experiments, in a colloquial sense, are what we want to inspire. Through experiments, one can not only learn a potentially new health skill; they also learn the meta skill of how to explore and find strategies for health that can work for them (i.e., experimentation and trial and error on self). Based on this, we see the XB model as a skills-building approach for supporting self-study practice around health heuristics that cultivates both self-regulatory skills in the form of self-study and skills in doing specific actions that produce desired across contexts. It is in line with the personal science framework (Wolf and De Groot, 2021), but simplified in terms of providing initial heuristics to work off of, compared to more of a blank slate implied in the personal science framework. We help participants share their experiences both through talking about their experiences as well as sharing anonymized data of their experiences for open science (Bisol et al., 2014).
To reinforce this exploratory approach, we frame each instantiation of the XB model as an “experiment in a box”, an XB. By experiment, we are explicitly not referring to the current health science use of that word, which connotes the use of randomization to enable a robust causal inference. As said, we use “experiment” in a colloquial sense as the colloquial concept aligns with the experience we offer. This framing as “experiment” has several SDT related advantages: it reinforces the autonomy of the Experimenter as the one in control, not the one being controlled or told what to do. The nature of an experiment is also time-limited, thus enabling a person to “try it out before committing” to a particular health protocol. That approach is in contrast to the dominant paradigm that assumes one will choose and follow a strategy forever. The “box” part of the Experiment in a Box is the guidance the XB provides to test and evaluate the heuristic and to translate it in the process from a general heuristic to personalized health knowledge skills and practice (KSP). The box is where the key distinction from the more general personal science framework occurs.
KSP is important in the XB model. Knowledge is prior information learned by others or oneself relative to the relationship between actions and outcomes, both positive and negative. Skills are strategies, approaches, and actions one actively engages in to achieve desired goals. These skills can be far ranging from the concrete development of one’s physical ability to do an action (e.g., shoot a basketball into a hoop) to ones’ self-regulatory capacity to define, enact, monitor, and adjust one’s actions in relation to a desired state. Practice has two associations, as pointed to in the introduction. The first is simply the repetitions necessary to be able to use a skill, apply knowledge effectively. The second is the incorporation of these skills as something that is deliberately, actively and consciously part of one’s life.
In the first case practice involves cultivating personal knowledge, which includes both that which can be expressed, and also what may be tacit knowledge—that is, “known” by the person but may not be conveyable via language (e.g., how to kick a football or play a chord on a guitar; building one’s capacity to know how to adapt appropriately in various contexts). For example, one general health heuristic for a healthy sleep practice might encapsulates actionable knowledge as: “ensure that the environment where you sleep is dark when sleeping”. The skill is the ability to execute that knowledge in diverse contexts. It might include something as simple as ensuring lights are off or blocking light leaks from windows. The more diverse the sleep contexts one experiences where they solved the dark room challenge, the broader the options are to draw upon to execute this heuristic across contexts. By extension, a person’s robust practice across contexts leads to enacting practice agility—a significant component for “resilient” (Feldman, 2020) health practices. As a general health heuristic becomes tested and refined by/for each person across contexts, it becomes an increasingly more personal and robust health heuristic, hence a personal health heuristic. For example, one may find that 1) dark rooms improve their sleep and 2) their best path to a dark room at home is a sleeping mask, but on the road, traveling with a clothespin/peg to shutter a hotel curtain, is essential. XBs are designed to help people develop this KSP experience/expertise.
Practice in the second sense is also a way of framing an ongoing engagement with knowledge and skill work. One may have a professional practice, a spiritual practice, a physical practice. The concept of practice in this way situates the interaction as deliberate, intentional, and continuous. It also inherits, at least in part, the sense of practice as part of continual skill refinement. This sense of practice further differentiates our focus on heuristics from habits. A habit’s great strength is to be able to “set it and forget it” like “brush your teeth every day on waking.” A heuristic-as-template on the other hand helps guide and instantiate knowledge, and apply skills, in diverse contexts. Thus we practice skills like a tennis serve, at first to learn the technique and build the coordination until the skill is automated (Luft and Buitrago, 2005)—where it actually changes where that pattern sits in our brain. After this, we practice the skill literally to keep those neural pathways cleared and strong (Olszewska et al., 2021). But the practice of the practice is to deliberately work to add nuance to the skill, to operate better, faster, across contexts. Such ongoing refinement is necessary, for example, around how we eat. What diet supports muscle building in year one needs to be refined in years two, as the body adapts, circumstances change. Thus, one’s physical practice is both regular, one might say habituated, but critically, is also conscious, deliberately open to refinement to support continual tuning. We will see this latter sense of a practice in KSP as part of the insourcing we have been developing the XB framework to help establish and support.
Experiment in a Box Exploratory Studies: Toward the Experiment in a Box Framework
The above section lays out our general thinking that guided of how we designed our initial explorations into XB. In this section we present how we iteratively refined and tested our approach over three studies. We had several goals in these studies. A key goal is to see that health skills tested in an XB are effectively insourced. We define that in terms of a recognition that the specific health skill a person experimented is used over time. Further, we also sought to see if people insourced the more general skill of self-experimentation. Do people report continuing to try out different generic heuristics they hear of in their lives, as is suggested they do in the XB framework? In addition to the behaviors we were seeking to observe, we also wanted to gain an insight on perceived benefit. As our focus is on insourcing and interoceptive awarenessness, we asked an intentionally broad question: does a person feel better? Further, do they feel more capable in managing their wellbeing?
Our study approach, which involved three studies over time with increasing levels of rigor, was grounded in the classic scientific logic of assumption articulation and testing (i.e., iteration) coupled with triangulation toward consilience. Specifically, based on the stage of this approach, which as we argue, is still vary nascent, we explicitly started highly exploratory in our approach, to enable experience to guide our next steps. As we learned from experience, we refined our testing protocols. While no one study of this sequence provides definitive evidence of utility of the XB approach, the similar patterns we saw across different populations, ways of measuring, and ways of supporting an XB experience (i.e., consilience), we contend, is suggestive of the value of further study and exploration into the XB framework.
In addition, our iterative study approach was inspired by the ORBIT model (Czajkowski et al., 2015) for behavioral intervention development. In particular, study one and two were both variations of a proof-of-concept trial, with study one used to help refine XB (i.e., the end of phase I of the ORBIT model), and then to gather evidence of real-world impact relevant to the outcome of interest in study I, which is the start of phase II of the ORBIT model, focused on justifying the need for a more rigorous clinical trial. And even in our third study, we used more of a proof-of-concept formalism for evaluation, which explicit does not use statistical analyses between groups. The reason why we used iterative proof-of-concept studies is because, both, this is the stage of development we are at (define, refine, test for a meaningful signal), and because this approach is increasingly recognized as a more appropriate method for exploration than the increasingly debunked strategy of running underpowered randomized controlled trials and conducting corresponding “limited efficacy” analyses of between-group differences. Indeed, under-powered pilot-efficacy-trials explicitly are increasingly being shown to produce poor evidence that actually stymies scientific progress (Freedland, 2020a; Freedland, 2020b). The short reason is that, with such under-powered trials, any effect size estimates gleaned from such trials is highly untrustworthy and, thus, largely not interpretable. To avoid these traps, a series of proof-of-concept studies, which we conducted, fits with emerging best practices for work focused on defining, refining, and testing for a signal of an intervention. Further, when looked at together, they provide increased confidence, via the notion of triangulation and consilience, that the XB approach has been specified with sufficient rigor to enable limited replicability across populations. As illustrated in ORBIT, this does not mean that any claims of efficacy or effectiveness relative to some meaningful comparator can be made at that time, but, again, that was not our purpose. Our purpose was to define, refine, and test for signal (i.e., phase I and the start of Phase II of ORBIT). More details on each proof-of-concept study variation below.
Study One presents a low-fi exploratory study with a 12-member participant group to see simply if these short experiments were positively received, experienced as useful, and in particular, lead to lasting use after the study intervention ended. The results were overall positive, and we present a set of design insights from that study that formed the basis of a more formal interrogation in Study Two.
In Study Two, we translated the insights from study one into a stand-alone application to be used by individuals, independent of a group context. Using a within-subjects design, we sought to assess if such an implementation was sufficiently robust to create a positive effect during the study, and could likewise instill lasting knowledge skills and practice, as indictive of the creation of a personal health heuristic, beyond the end of the main study.
The outcomes of the study were overall positive. In Study Three, therefore, we sought to test our assertions on the key elements of XB—the framing of an experiment; the support to regularly evaluate and reflect on its utility—actually enabled XB success beyond simply making good practice guidance readily accessible. To this end, we ran a formative between-subjects randomized study to test our hypothesis that an XB approach would be more effective at promoting engagement with health practices compared to a version that mirrors standard mechanisms of offering health support. For our test we reused the SleepBetter XB from Study Two and created a similar app that offers knowledge (i.e., information about sleep) and details sleep health behavior skills (i.e., sleep hygiene), and invites a person to try any one of these practices for a week but does not provide the self-regulatory skills exploration operationalized by the XB model, nor a structure that guides experimenting with one’s practice. While we imagined that the more supportive approach would be better than the current standard for sharing health practice building, the strength of the responses between the two conditions underpinned the value of the XB.
Study One: Exploring Experiments for Sustainable Health Practices
In Study One, we worked with 12 participants from a national advertising organization over 6 weeks. As creativity is an essential quality for advertisers, the motivation for participants was their interest in improving their health to improve their creativity. As measuring creativity directly is notoriously messy (Thys et al., 2014), we drew on related work that shows both: 1) how cognitive executive functioning areas of the brain map to associated areas for creativity; and 2) that fitness practices enhance those areas of the brain (schraefel, 2014). From that, we postulate the use of validated cognitive executive functioning tasks to demonstrate quantitative benefit, along with participants’ qualitative self-reports of their experience. Participants carried out several of these tests prior to beginning the formal study and after each week. These included stroop tests, tapping tests, memory tests (Chan et al., 2008).
In this exploratory study, we focused on offering participants a weekly “taster” of five fundamental health practices for wellbeing we called the “in five” of move, eat, engage, cogitate, and sleep, detailed in (schraefel, 2019). In the six-week engagement, week one was a preparatory week to learn about the study and how it would run each week. In each of the 5 weeks following, the group ran an experiment on one of the in five. At the start of each week, the team manager sent around a document we co-wrote, with descriptions of the experiments and instructions for the protocol, asking people to track their practice/experiences at least once a day. We did not specify how to do this tracking; we were interested in what people would find important to meet that observation requirement. The manager was very good about sending out reminders every other day to make sure people had logged something about their experience for that study. To align these experiments as much as possible with the group’s motivation around creativity and design, we also named these experiments with colors rather health concepts. For example, the Black Box was the Sleep protocol; the Green box was for Eat, the Blue Box was for Cogitate, Red Box for Engagement and Yellow was for Move. Table 1 lists each of the experiments, and their associated protocols.
In this first study, the experiments for each week were predefined, but we emphasized choice in the variety of ways the heuristic could be implemented. For example, in the Green Box, the heuristic was “up your green and red” green being green vegetables; red being any protein. The experimental protocol was: “eat any time you wish, as many times in a day as you wish, but any time you eat, for the five-day work week, have some green veg and some kind of protein before you eat anything else, any time you eat”. As a group, prior to the Green Box start, we reviewed what constituted a “green veg” and what a protein was. To reduce any sense of sacrifice around eating in the experiment, the approach was also deliberately additive rather than subtractive: “as long as a green and protein are present, add anything else after that; and also have the green and protein before eating anything else you add”. We also acknowledged that this was not likely how people would continue to eat after the experiment, but that as an experiment this was to ensure dose effect in the given time.
At the end of each week, we asked participants to capture: 1) what did you learn/experience? 2) based on the effect experienced, how would you turn this into a part of your life—how would you create a personal health practice (a personal health heuristic) from this experiment? 3) what are any questions you have right now about implementing that practice? In our Black Box for sleep experiment, the kinds of questions we would get were: “Sleeping till I wake feels great; how do I do this on days when I want to go out with my friends?” This led us to discuss sleep debt, recovery, and also balancing values like social interaction for wellbeing with rest and recovery. In the Yellow Box, we learned that people were reluctant to be seen leaving their desks so frequently lest their colleagues perceived them to be slacking off. This response offered a powerful insight for managers in terms of the interventions needed to support movement as a cultural practice to be encouraged.
At the conclusion of each week’s experiment, we met online via Skype to reflect together on outcomes from that week and to prep for the week coming up. In-between weekly meetings, we used an open-source online bulletin board to share observations, respond to questions, and add reminder requests to record experiences. As noted, participants were also invited to re-run their creativity assessments once a week, post each experiment.
In this study, we did not ask participants for their logs or their creativity assessment data: that we agreed at the outset was personal, and we were keen for as many people on the team to feel as safe as possible working with us as co-explorers, not subjects. We agreed on sharing material on the forum with all of us in our weekly meetings—including the three questions asked each week, above. Our final interviews would be used for our analysis. To this end we asked participants to share as much of their insights as they felt comfortable, to help inform the design process.
Design Insights From Study One
Overall, the consensus was that the process was interesting and surprising in positive ways. The group even made a 3-min video to reflect on the experience (Ogilvy Consulting UK, 2014). From the forum posts and our weekly interviews, we gathered the following key points around factors affecting the utility of the XB approach:
1) Small repeatable doable doses; big effects. In developing the experiments, we were very careful to ensure that an effect could be felt within the week of its practice. We drew on our own professional backgrounds in health and sports science, consultations with colleagues who coach health practices professionally, and associated literature reviews to develop these protocols. Despite this preparation, we were surprised by the reported size of the experience based on the conservative nature of the protocol. For example, in the Green Box, we were particularly concerned that any food intervention would have a noticeable effect within a week, as the usual report in the literature had been 14 days to show measurable results. It seems experiential results may be sooner: each participant reported even before the week was up about changes in energy; about the surprise as they thought it would be hard to get a green and a protein in all the time, but how easy it actually was. It seems also that dose size afforded by an experiment is also valuable: that each time a person ate, they focused on the green/protein combination. This meant they were paying attention to this practice and its effect—both on immediate eating experience but also in reflection—having many data points to draw upon by the end of that week. Thus, short, simple, multiple doses that are readily achievable (with preparation) and that will also create an experienceable effect within the period seem to be key.
2) Beyond knowing: creating space to do the practice. Participants also commented on the importance of direct experience of a practice they “knew” of but had not directly tried. For example, in our Black Box for sleep week, we worked with the Group’s management to request permission to let participants test the heuristic “wake up when you wake up” and thus to sleep as long as they needed, and to come into the office when they were up and ready, without penalty. Intriguingly, it took more effort from the manager to confirm with participants that this was OK, than it was to get that support in the first place. Once they had the space to embrace what became known as “kill the alarm”, they did. Again, they reported value around being asked to actually “test” the concept in the experiment. As one participant reported, “I’d always heard that 8 h of sleep was beneficial, but until I actually tried it, I had no idea how much of a difference it made.”
3) Unanticipated benefits—While some people expected they may have more energy or gain more motivation to stick with a health practice, they were surprised to find how quickly their mood also seemed to improve; and how easy these practices were to make a regular practice. One person’s surprise was in their colleagues, especially after the Red Box on Engagement where they were asked to take 3 min every day to listen to a colleague, without interruption. “I used to hate that person, but after listening to her and others, it was amazing, she became so much nicer; she stopped bothering me.” There may be an opportunity here for design to explore more deliberately unanticipated positive side effects associated with experimental experiences.
4) Overall effects appeared to be cumulative—Each week people reported positive experiences; after week three, most were reporting they were starting to notice positive differences in their mood, sense of wellbeing, energy, creativity and for some, their weight. This progressive experience across experiments may be another side effect that can be explored more deliberately in design: to cue up that there may not only be benefits from the time given to one experiment, but in spending this period—in this case six consecutive weeks—on health practices; there may be additional benefit just in the exploration of health for those several weeks. This experience in itself of having the permission space and support to focus on health KSP—irrespective of experimental outcomes—may foster a foundation for lasting health engagement.
5) Short exposure may lead to lasting practice—In follow ups—3, 8 and 13 months after the study, participants were still using skills they had learned—translating them into practices that were working for them. For example, one participant shared that they attempt to get full nights’ sleep for them at least four nights a week so they can have “guilt-free socializing time” with their colleagues on weekends. The participant claimed the XB experience helped them explore and build a sleep-recovery approach to support their wellbeing. This approach was not taught by the Black Box, but the Experiment aspect of the study itself encouraged the person to keep testing practices to work for them.
6) Skills Debugging—Related to ongoing use of both skills and experimental “test the approach”, we found that, without prompting, participants used the skills from an XB to debug and retest their practices. One participant reaffirmed her initial results with one experiment that for her, a diet high in greens with sufficient protein feels good on numerous levels. She told us: “My parents visited for a week, and I just stopped doing Green and Red (a green and a protein with each feeding), and I ate what I’m like when I’m at home. Lots of bread, lots of pasta—no greens, very little red. I felt stressed; I reverted to type. When they left, I felt crappy and bloated and no energy. I decided to go back to red and green; the weight I put on had gone and I feel so much better. Maybe the stress is gone because the visit is over, but I think it’s a lot about the food.”
7) Preparation This result is inferred by participants’ discussions about their practice: in many cases the way they described carrying out a practice drew from the preparation for it in the week preceding it. For the Blue Box, the experiment was “learn new skills you can practice daily, many times, for a total of at least 20 min”. Here, we heard about how people had put together tools they would need, for orienteering (“I’m directionally impaired”) or sketching materials and where tools would need to be when setting up their “lab box” for the experiment. Likewise, for the Green Box, how they would have containers and prepare vegetables for the week to come again to have their lab ready to go. Preparation also helped illuminate challenges that we had not considered and needed to address on the design side. In the Yellow Box, we asked people to move away from their desk twice an hour and to keep moving for a few minutes each time. Operationalizing this was not a problem; fear about how their colleagues not in the study would feel about them walking away so frequently was a challenge we were able to mitigate by working with management ahead of that box’s week.
8) Diversity/Choice In this study, the diversity of ways to explore health was also seen as a benefit; it let us convey how health is dynamic and that there are multiple options within multiple paths to approach it. Enabling people to determine how they would choose to implement a heuristic was also well received: “We could eat whatever green we wished—I learned about so many veg I’d never tried—it was great.”; “I wanted to learn how to play guitar—I didn’t know that was good for my brain, too.”; “after moving and sleeping, I just felt so much more creative.”
Study One Summary
In sum, our work in this first exploration showed us that guided exploration of health-KSP in even short periods provided a basis for participants to build their own health heuristics. These short, focused activities led to enduring use without any further technical support.
Study Two: SleepBetter—Many Paths to Health
Based on the richness of our results from our first study, our goal in this phase of development was to explore how we could translate that lo-tech, guided XB approach into a stand-alone digital intervention that could potentially reach and benefit more people. There was also growing concern at our University for students’ wellbeing, and the correlation of poor sleep with mental health challenges reported to be on the rise in this group (Milojevich and Lukowski, 2016; Dinis and Bragança, 2018). Therefore, we decided to explore how an XB could be redeployed to focus on one health attribute, in this case Sleep, while still building a sense of exploration and skills development. As well, we saw this XB implementation as an opportunity to complement the predominantly outsourcing-oriented work around sleep in HCI, as overviewed above, with a study of an insourcing approach.
In this single-focus XB, we were also able to shorten the engagement with the XB from 5 weeks across five topics to 15 days on one, where participants carried out 3, 5-days sleep experiment cycles. We facilitated agency by offering participants a suite of ten experiments to choose from to test sleeping better (Table 2). Further, participants could choose to carry out the same experiment for each cycle or choose a different one, from the set of ten.
In research, self-perceived sleep quality is considered to be a more important marker of success rather than duration (quantity) (Choe et al., 2015a) alone (Mary et al., 2013; Mander et al., 2017; Manzar et al., 2018). Thus, our heuristics/experiments focused on practices that were assessed on a qualitative and experiential basis, rather than the currently dominant and possibly inaccurate (Ameen et al., 2019; Tuominen et al., 2019; Louzon et al., 2020) tracking approach of hours slept, number of interruptions, time assumed to be in a particular sleep state and so on. Our focus was: after trying this heuristic, how do you feel?
Apparatus: SleepBetter Experiment in a Box App
We developed SleepBetter, an Android OS smartphone XB application (Figures 1–3). The application includes 1) an experiment selection area; 2) self-reflection aids 3) a FAQ to provide information about the rationale/science behind each experimental protocol.
The application provides ten experiments for sleep improvement (also known as “sleep hygiene”) listed in Table 2. Each experiment is grounded in sleep research drawing on: guidelines from the National Health Service (NHS) United Kingdom (Sherwin et al., 2016) the Mayo Clinic, United States (Ogilvy Consulting UK, 2014), and related research (Bechara et al., 2003; Espie et al., 2014; Schlarb et al., 2015; Agapie et al., 2016; Daskalova et al., 2016). We also reviewed this set with sleep researchers.
Each day at the same time, participants were asked to respond to a short questionnaire (Figure 1) about their sleep experience the previous night, including: how they felt when they woke up, how they felt before going to sleep, their mood, and their perceived concentration. Each day they were also asked, summatively, “Do you feel better or worse than yesterday?” (Figure 2). Each question was set on a five-point Likert scale. Figures 1, 2 show examples of these representations.
A free-form reflection diary supported text entry for any annotations participants wished to make each day. Drawing on Locke and Latham’s approach to goal setting for motivation (Loke and Schiphorst, 2018) we called this space a Goal Diary so that participants could use this space to note a desired outcome from each experiment and reflect on effect. Participants could see graphs of daily progress based on their questionnaire answers; they also had a calendar (Figure 3) with circles around dates to show completed experiments. Circles were also colour-coded against a selected Mood state: greens (better); reds (worse).
Participants
As noted, with our focus on university students’ wellbeing, our inclusion criteria were for university students. To connect with this group, we used social media to recruit a convenience sample of the 25 final participants. The mean age was 21.8 (SD = 1.28). All participants identified sleep as an interest for themselves and agreed they wished to participate in the study either to learn more about sleep practices or because they wanted to improve their own sleep. A limitation of the study group is that, in this convenience sample, all participants identified as male.
Procedures
This is a within-participants study with three Phases: A, B, C. Phase A includes initial sign up, consent gathering, apparatus set up and baselining questionnaire that took place over a 6-day period. Phase B is the XB intervention itself, which lasted for 15 days and was broken into three, 5-days “experiment” phases. At the end of phase B, one-on-one tape-recorded interviews took place, pending participant schedules, within 10 days after the intervention. Phase C is a post-intervention survey covering self-report of wellbeing and sleep practices as well as reflections on the XB experience 7 months after Phase B, when all use of the application had ceased for 6 months. We also note that out of the 25 participants who completed the study, we only contacted 16 for Phase C who deliberately stated (in Phase B) that they agree to being contacted with a follow-up at a later date. On completion of Phase A, Phase B started. Via the app, participants were presented with the list of experiments from Table 2 and were informed they could choose any experiment from the set but would not be able to change it once selected, until the 5-day period for each experiment was complete. To reduce the burden of having to remember to engage with the app, the app sent reminders to complete a questionnaire for the day. The reminder encouraged exploration of the FAQ for questions about the experiments in general or sleep in particular.
Measurement
The main measurements during Phase B were the daily questionnaires which had two parts: protocol questions and state questions. The protocol questions were specific to each experiment. For example, for experiment C1 (Table 2) the questions included: “What time was your last coffee?” and “What time did you go to bed?” State questions focus on general sleep performance, such as: ease of falling asleep, sleepiness or alertness during the day, factors in mood, appetite and concentration ratings—all factors affected by sleep. As this study is exploratory rather than confirmatory, we drew on questions where the effect of a sleep intervention has been documented within days. These questions were drawn from established sleep assessments (Espie et al., 2014; Ibáñez et al., 2018) to map to the brevity of our intervention period.
Changing Their Experiments
After each of the three 5-days experiments, participants were offered the opportunity to choose a new experiment or re-run their current experiment. They were also given an additional questionnaire on day five. This interaction explored:
1) What attracted them to the experiment they just completed;
2) Their experience, prompting them to reflect on its perceived effectiveness in terms of how they felt—and if they were changing or sticking with their current experiment for the next iteration;
3) What factors seemed interesting to them about staying with or changing experiments.
We emphasize that our goal in analyzing the data we captured from each of these questions was not to be confirmative, per se, but exploratory, to see the ways in which our design approach supported our aspirations to support connecting brief practice with a felt sense of benefit. To that end, we present findings and discussion, below.
Findings
Thirty five participants were initially recruited, out of which: 25 completed the study, four dropped out after signing up when they could not run the app on their particular phone’s OS version; two reported that due to circumstances changing they could not participate; two gave no reason, and two dropped out when they changed phones during week one of the study. In sum, eight people dropped out during the pre-study set up phase, and two within the first 2 days of the study, having effectively null engagement with the intervention. As such, they have not been included in the reported data set. The overall change or benefit of the XB can be assessed in two ways: 1) as a perceived effect from pre-experiment assessment (what we call here Phase A) to post-experiment assessment (Phase B); and 2) by uptake and ongoing use of protocols over time, post-experiment (Phase C).
Phase A to B Effect
There was a statistically observed difference between A to B. Based on 13 questions used in sleep study assessments about perceived personal state and sleep quality, with each question based on a five-point score, where lower score was better than a higher score for a maximum score of four per state (Figure 4, Y axis), the Phase A results were 33.4, with SD = 6.52. The mean of the final questionnaire at the end of the study is 25.44 with a SD = 7.04, t = 5.452 (with p < 0.001). Cohen’s d formula to evaluate an appropriate effect size for our means is 1.17, thus the measured effect for the difference between the means is strong. Results are presented in Table 3, where questions with a significant effect are highlighted.
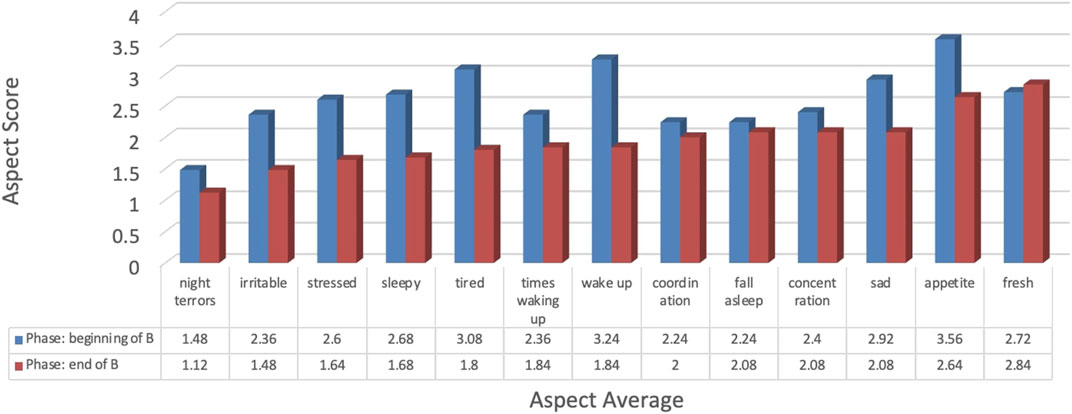
FIGURE 4. Score differences across all participants between the beginning of Phase B and end-of-B on perceived state questions.

TABLE 3. Statistical computations between the beginning of Phase B and end-of-B on perceived state questions.
Looking within these measures, we see significant positive changes in sleep quality attributes: nightmares/night terrors were reduced. Mood categories of perceived tiredness, sleepiness, stress and irritability improved, as did appetite (cravings are reduced). The other categories: number of times waking up, ease of falling asleep, concentration and coordination changed positively but not significantly. The only slight negative change, though not significant, was feeling fresh when waking up (Table 3), which may be due to this being one of the first times people were focusing on this factor regularly.
Phase C Effect
Of the 25 people who completed the study, 7 months later, 16 agreed to complete a follow-up survey of their experience with the SleepBetter XB, and how this experience may have continued to affect their sleep practices. This questionnaire was entirely self-report, and so suffers from the known issues of overly optimistic assessment of performance. Even given that, we were surprised by the degree of at least reported persistence of practice. Comparing their sleep quality between before the study and after, 75% rated it now as “good” and 19% at “very good”, well up from their Phase A baselines.
In one question we relisted Table 2 experiments and their “tips”, like “getting at least 7 h of sleep a night”. All 16 respondents reported remembering all of these tips with 15 continuing to use them at least some of the time. 81% of the participants reported continuing to use the tested protocols at least three times a week, with 43.8% reporting use of protocols every day. When asked if they had continued to use the App post-study, only four reported yes, with one of them using it for a month “to try other experiments,” two used it for a few days, and one for an additional week, suggesting the main and lasting effect was created during the Phase B of the study. There is also evidence, however, that people explored practices from the XB experiments after the study. For example, the top three most chosen experiments from Phase B were, from Table 2: L1—increasing bright light exposure during the day (19), S2—sleeping no less than 7 h per night (17) and C1—not drinking caffeine within 6 h of going to sleep (12). Of the Phase C respondents, while 12 reported getting more than 7 h of sleep/night since the study (Figure 5), only seven followed that protocol (S2) during Phase B. More broadly, 75% of respondents agreed that they were sleeping better. Significantly, all but one of the Phase C respondents attributed these changes to their engagement with the XB SleepBetter study.
Qualitative Reflections on Experiment in a Box Experience
Tw of key questions for us were: 1) Are these small doses of how to use these protocols to feel better in the XB sufficiently strong to have a perceived immediate benefit; and then 2) Are they sufficiently strongly felt to maintain these practices over time and without the app? The Phase C data suggests yes, at least among those who responded to our 7-months request (64% of our sample): XBs seem to support experience, effect, practice over time, and that once learned, practices are accessible enough not to be forgotten. Our post Phase B interviews and Phase C open questions, reported below, were designed to explore which attributes of the XB interaction were experienced as supporting these.
In an effort to be as unbiased as possible in the interviews, we asked about initial expectations of the app, what interested people in participating, and also asked about the experience with each section of the app: aspects that worked; what could be improved; length of an experiment; reasons for switching experiments or not; and what participants feel they learned about themselves during the study. The following uses ID-codes for participants.
Experience in a Box
The inspiration of our work has been to make a connection between the lived experience of feeling better as a result of testing a health protocol. After 7 months, 50% of our Phase C respondents said the most important aspect of what XB facilitated was the opportunity to “try a sleep practice, rather than being told it’s something you can do.” When asked what aspects of the app let them learn about sleep, which they would not otherwise have learned, they pointed to their well-being results from 1) experiencing how even very small changes can make a difference; 2) how important sleep is to performance, and 3) what doesn’t make a difference: A9—“I also tried other experiments, but they didn’t make any big impact so I didn’t really stick to them.”
Pre-Fabricated Experiments to Build Experience Access
Overall, the opportunity to choose from a set of protocols to test was received positively. As A9 put it: “It was nice that I could try different experiments and see which is working better for me.” In other cases, participants mapped to experiments they thought were most related to their sleep issue—A13: “I chose the experiment based on what I thought my problem was.” A16 said, “I chose the ones that would be related to my everyday needs and activities.” One feature—the opportunity to select experiments in comparison to be “told what to do” was seen as particularly valuable—A4: “I think it is a good idea to let people choose instead of forcing something onto them because different things work for different people, letting them discover what works for them and then giving them the chance to stick with it of they found something good, or switch to something else if it didn’t work.” A2 framed this as empowering: “Because I was the one choosing the experiment made me really more motivated so it’s not like someone was telling me what to do.” All ten experiments could have a similar effect/benefit, but enabling participants to assess which might be easier for them to test or adopt was also a plus—A12: “making me choose which (experiment) I was more comfortable with would help me see which one affects my sleep the most, by going through each one at a time.” The length of time for the experiments also seemed appreciated. As A1 put it: “it gave participants enough time to get used to the experiment and feel a difference and be able to decide which one suits them better.” Implicit within these comments is also an appreciation that they are not being told what works but are testing effects for themselves.
Future Experiment Options
Some participants suggested that they had such a benefit from an experiment that they wanted to keep using it even while trying out another experiment in the following round. A12 stated that “after seeing that one experiment worked, I tried to keep it even though I switched to another one.” Indeed, after the first 5 days, 73.1% of the participants switched to another experiment. However, on the second experiment change, 69.6% remained with their current experiment for the last 5 days. Many of these said they would have liked to stack experiments together.
Some participants recommended that the app narrow down the set of experiments offered to make the selection even less of a choice, based on captured personal information. A13 suggests to “add a feature that would make the app choose the experiment for the user, based on their answers in the surveys and evolution.” Others, however, wanted an even wider set to find experiments even more in keeping with their daily lives. As A2 observes: “one thing to change about the app is the number of experiments available and the number of suggestions that the user has because for me personally half of the experiments were not applicable so I had to choose between only two or three options.”
Connecting Practice With Experience
A goal in the XB design was to create mechanisms to assist, and potentially accelerate connecting the experience of a health practice into the value of adopting that practice. A critical component of building this experience was what we called guided self-reflection. We offered several mechanisms for this support: 1) the daily questionnaires, 2) the experiment-completion overview questionnaire that occurred on the fifth day of each experiment, 3) the notification reminders to complete the questionnaires and check the FAQ, and 4) the data visualisations.
We were surprised by the number of people who explicitly mentioned the once-a-day guided self-reflection practice as particularly helpful. Talking about the daily questionnaire, B5 and A9 reflected the general view: “the best part of the application was that at each end of the day you had one questionnaire to check your progress”; B5: “I guess the Questionnaire was the part I used the most” (also stated by A9). Several design factors seemed to contribute to the experience. The brevity of the questions was seen as a plus. As A17 said: “the questionnaire was not too long and it did not take too much time.” A2 appreciated the portability the app offered for the study: “you can actually take the experiment home with you so you didn’t have to go anywhere or talk to anyone.” Intriguingly, in Phase C, when asked what features of the XB people valued the most, only 12.5% reported that it was the questionnaires.
For others, the data visualization of the questionnaire responses over time was their main way to reflect on their experience. A17 reflects this general sentiment: “It helped me a lot because I was able to visualize my progress and see what I still have to work on.”
With respect to the Goal Diary, 14 people of the 25 used it. These entries would describe most often how participants felt about their progress, or something out of the ordinary affecting their progress, like the succinct “Mosquito woke me up” of A4. Some of the users, like A1 have used the diary to record their actions (by adding how much they slept each day—“Slept 7 h”). Others used it for their own recommendations based on their experience: A15 has logged in “Should go to sleep earlier.” and A10 has set their own goal “I will wake up early every day.”
The Calendar was also used to check engagement: A1 stated “the calendar was most helpful because it shows a recap of the day.” The ability to hover over a date to get more information about the experiment on that day proved useful as well: “I was able to check my status at any point in time” (A12).
Making Felt Connections
A key part of our work was to understand how feeling better affected perceptions of experience. When asked about their takeaways from the experience with the app, a sense of visceral experience was common. A1 said “I noticed that when my goal was reached I could concentrate more and feel less sleepy throughout the day.” Similarly, B5 said, “I learned that I could have a better sleep, and I can feel more rested and relaxed. And I saw that I can have a certain routine and respect it.”
There were also specific insights as well in terms of self-knowledge to inform future practice choices. A17 notes, “(the light experiment) helped me realize that my concentration really depends on the time I spend outside in the sunlight because it made me more focused and more positive.” Likewise, A10 learned about coffee timing’s effect on sleep, reporting “Even after finishing the experiment, I have given up my afternoon coffee, and kept that up.”
Design Suggestions
We also received a number of suggestions about how to improve the app, in particular to bring in more personal informatics, on demand. B5 suggested using a graph to display physical activity, heart rate or nutrition, whereas A4 requested to be able to track external factors affecting a protocol. Several participants wanted to track their hours slept more specifically throughout the experiment; others also wanted to integrate other health practices like water or exercise.
In the Phase C responses, only three of the 16 respondents said they would have appreciated a longer intervention to have support to translate a practice into a habit. This request explicitly ties into our research question: do these experiences help build bridges/motivation toward adopting these healthier practices? The answer seems to be a qualified yes: for the majority of those who responded, they report ongoing practice without additional digital support. For these few others, they want to sustain the practice with more assistance. There is a role potentially here for building synergies from XB toward apps that then specifically support a tested and desirable practice.
Study Two Summary
It’s clear from a comparison between Phases A and B, that, in this participant sample, the experience of using an XB was effective in having a perceived benefit on participants’ sleep. In Phase C, it also seems clear that the period of the intervention—15 days—was sufficient to establish knowledge, skills, and practice that continued to be used, without further aid of technology, for at least half a year post-intervention.
Study Three: Offering Knowledge and Generic Practice vs. Knowledge and Practice Plus Self-Study Skills
A key question stemming from the perceived utility of XBs in the previous studies is it the features of the XB creating this effect, or is it simply giving people what may be new information and encouragement to try out these health skills? The XB Model provides a structure to support self-regulatory skills of self-study plus knowledge and practice encapsulated in generic health heuristics. Hence, we had the following hypothesis:
The XB Model would be more effective at promoting improved sleep quality compared to providing knowledge, health behavioral skills (arguably current standard of care) while not being taught the self-study skills encapsulated in an XB.
Specifically, the common approach within health communication is to offer knowledge based on prior science and evidence-based recommendations and then offer concrete suggested activities and skills that one could enact as a regular practice. For example: Caffeine can interrupt sleep (Knowledge). Based on this, avoid caffeine 6 h prior to bedtime (Practice). The key distinction between this and the XB model is two-fold: 1) how health heuristics are presented; and 2) helping people build the skills of self-study. The XB does not differ much in terms of the knowledge it is grounded in. It does make adjustments though in how to offer a health heuristic: specifically, we see it as 1) critical to frame health heuristics that inspire curiosity, 2) actionable, 3) can result in meaningful effects relatively quickly, and 4) the health heuristic is offered in a way that inspires a person to not enact it as a habit (which is often the implied target for generic messaging), but instead to experiment with the heuristic. Further, in terms of skills, the XB model provides people a structure to translate a generic health heuristic into an actionable personal health heuristic.
Procedure
To explore this feature, we designed a between-subjects study in which participants were randomized into either the control condition, in line with standard of care, or into our XB model. The study ran again for 1 week set up, with a baseline questionnaire about sleep quality, a 15-days execution with the invitation to run one to three experiments per 5 days/experiment. We deployed two versions of our SleepBetterXB app: the same app used in study two (now both Android and iOS) vs. an app that provided them knowledge about sleep and offered them basic sleep heuristics, with a suggestion to try it for 5 days. During the 5 days, the person would be provided with the health heuristic and be informed on how many days were left trying it out. After 5 days, people were suggested to either keep doing the same health heuristic or try a new one. In both groups, this was a 15-days study, with three experiments. Like study two, participants were recruited via social media, but went outside the university zone, deliberately also recruiting for gender balance across conditions.
Findings
In the XB condition, there were 16 participants: eight men and eight women; in the Control condition there were 15: 7 men, seven women and one Prefer Not to Say. No one was paid to participate or compensated for their participation. Our two-target outcomes were completion and sleep quality. Completion was defined as engagement in all aspects of the experience, including using the app daily and completing the sleep quality assessments, particularly at baseline and after the 15-days study. Based on this definition, four out of 15 (26.6%) participants completed the control condition compared to 15 out of 16 (93.4%) in the XB group. Central to this, most participants in the control stopped engaging with the app within the first 5 days.
Given the significant lack of completion in the control group, we did not run any statistical analysis related to sleep quality in that group. The fact that the control abandonment was so high and happened within the first 5 days of the study launch indicated to us that there is a perceived difference in experience, thus establishing a strong justification to not use statistics as the between-group differences are clear and strong without inferential statistics. In line with study two, a paired t-test of pre/post intervention with the XB groups showed a significant effect (PreMean = 2.9 ± 0.63, PostMean 2.42 ± 0.60, t = 2.186 with df = 14, and p = 0.046).
Overall, these results indicate a strong difference in completion between the control and the XB apps. Further, results replicate the study two findings, suggesting that the XB app—in particular its features supporting engagement and reflection—facilitated improvements in sleep quality.
Summary of Findings Across Three Studies—A Case for Consilience
As a reminder, the goals of our three studies were to advance the key goals of phase I and phase IIa of the ORBIT model. Specifically, this means that our goal was to define and refine the XB approach and then to test if we were getting a meaningful signal. If a clear signal is observed, this would be robust initial evidence that would justify more rigorous clinical trials in later phase II and phase III trials within ORBIT. Overall, the studies enabled us to iteratively define and refine the XB approach, to enable it to be more formalized and repeatable across three studies, with relatively minimal changes between study two and three. Further, our series of proof-of-concept studies suggest that, indeed, there is a signal of benefit for our approach and illustrate a general pattern of benefit of the XB approach across three different groups and, between study one vs. two and three, different XBs. Further, results from study three, particularly with the strong drop-off, which is consistent with standard use of most mobile health apps [e.g. (Bot et al., 2016)]. Taken together, these results highlight the value of supporting people explore health practices, and to enable people to test these practices for personal efficacy in terms of the simple heuristic: how do you feel: better or worse or the same today than yesterday? And then to have experience of different skills they might bring to bear to feel better.
In particular, the concept of XB as an experiment, a time-limited exploration of health heuristics is effective at making it safe to explore these practices via its “try it first before committing” approach (Study One). We see also see that XB’s translation into digital interventions provide a sufficient foundation for the practices tested to endure initial exposure to the app, and then, as we had hoped would be the case, to be able to continue these practices without the app (Study Two). We also have strong indication that the XB attributes that foster active practice and deliberate reflection are key to people engaging, exploring and building these personal heuristics (Study Three). Taken together, we conclude that there is sufficient evidence to warrant further exploration and, subsequently, more rigorous experimental (in the sense of the word used in health sciences) evaluation of the approach.
The Proposed Experiment in a Box Framework
From the results of this work, we propose seven components from the XB design components of generalized XB framework as the foundation for future work:
1. Experiment as Conceptual Framing. The framing of the interaction as an experiment has multiple traits that show up as contributing to the overall utility of the approach. Aligned with self-determination theory (Stevens et al., 2016), an experiment supports autonomy, competency and relatedness. It supports autonomy by enabling the person to choose and run their own experiment, and to respect their own determination about it. It supports competency by first asserting that their determination about the intervention’s utility is valid, and it helps them build new KSP about healthful choices that work for them. The experiment as a frame also emphasizes the approach being tested, not the person. Participants sharing their experiences/data from the experiments, as we did in Study One, also helps build relatedness. This approach we are seeing is allowing us to frame difficult questions related to sense of healthy selves, too. We are collaborating with an area college to engage students’ interest in their own personal sleep practice experiments’ data for multiple curriculum purposes, but grounded in a provocation these students asked to explore: “am I normal?”—what does that mean, and how explore it. Thus, there is motivation to explore statistical to social science curricula 1) to makes sense of one’s own findings relative to their cohorts’ and other national groups 2) to problematize with these live examples how statistical “norms” intersect with and can conflict with associated cultural practice norms 3) to explore how these results and concepts can be constructed as healthy or not 4) to explore complexities of policy making relative to the uses of such qualitative and quantitative data.
2. Multiple, Pre-Fabricated Heuristic Experiments: There are two aspects to this design factor. One, we offer multiple paths to the same experience: there is, for example, more than one way to get a better night’s sleep. That multiplicity in itself is a signal that there is a lot of choice around how to achieve a health win. Indeed, as we saw, a few of our participants wanted that choice space, not to go away, but in fact to be narrowed. That request may be an opportunity for machine learning eventually to pick up more personal parameters to reduce the set of candidates. Second, the experiment here is being used to enable what is frequently a new experience. We are using that experience as a bridge into new practices. To this end, we use already-known science to inform health heuristics, rather than creating new science. This focus is in contrast to most current self-experimentation work that seems to be used to create new answers to solve a perceived problem, whether via large scale N-of-1 studies (Pandey et al., 2017), or within a single-case study (Karkar et al., 2015). This is hugely valuable and important work. In our case, our focus is simply different, though we think strongly complementary. Though there are opportunities for these informal XB experiments to contribute to science, that is not our innovation focus here. We want to help people find, connect-with and test existing solutions-as-heuristics to build resilient health practices. We translate established protocols into experiments so that a person can sample for themselves what may be an entirely novel experience, and to assess it in terms of simplicity—but perhaps profoundly—how they feel—as opposed to any formal effects measured in blood chemistry. In this way, we are helping them create not only inbodied signal sensitivity but providing and emphasizing that they have options on what to do about the signal.
3. Control and Choice The simple opportunity to choose for oneself from a range of strategies to feel better was roundly applauded. Having the opportunity to try several or to stick with one method over 15 days was another choice factor that was a win. The approach also somewhat mirrors/reverses SleepCoacher (Daskalova et al., 2016), where external sources are vetting sleep data and suggesting protocol refinements. These related strategies suggest there may be times and conditions under which one approach is a better starting point than another. We suspect that there is value, as the MAHI work showed (Mamykina et al., 2008), in enabling a sense of empowerment in exploring health paths.
4. Guided Self-Reflection: Self-reflection is a key aspect of health self-study (Li et al., 2011). In our case, we built in what we foreground as guided self-reflection to help steer participants to focus on key parts of their experience with a given protocol relative to its perceived effect on their overall personal state and their desired state. We saw quite strongly that offering multiple types of guided reflections on the practice was effective. Participants appreciated the daily notifications and pauses to reflect on 1) how the protocol they were testing was being experienced, and 2) for a number of our participants, on what this seemed to tell them, beyond the experiment, about their lifestyles.
5. Time: Experiments run for a set (and we think, critically, short) period to test an effect of a specific protocol. As we saw with our participants, it is not only far easier for someone to commit to do some protocol for a minimal period like 5 days than 12 weeks; it is also possible to feel the effect of an intervention and make a judgment about it in that time. Indeed, a key design marker for experiments is: what is the evidence that an experience can be felt within this window? If there is such support, it is a good candidate for an XB. A related aspect around self-tracking may be that: more people may be willing to use something when the tracking is seen to be for only a short time, toward a very clear purpose like assessment of a practice. This could have an added benefit of creating new data resources for further research benefit.
6. Try It: All Data Are Good Data: The opportunity to test out practices was the most valued part of the XB. This “try before you buy” is very distinct from the dominant prescriptive approach in health to do a specific thing—usually for the rest of one’s life, if time is specified at all. This exploratory approach switches the dynamic to say that the person is in control of this process, not the other way around: the person can accept or reject a protocol based on a very simple heuristic: “Do you feel better or worse after doing it?” The XB approach signaled strongly also that the person was assessing these practices: if they were not effective, it was not the person’s fault. That lack of blame also seems empowering in these designs. This is echoed by A12 who in the Phase C survey said “It (dong the XB study) made me curious of more sleeping practices that I can try. Since I bought a google assistant, I have also been listening to the sound of rain while sleeping. This has significantly improved my sleep and I don’t think I would have been willing to explore this If I hadn’t been given the chance to try new sleep practices.” We plan to explore designs to more deliberately support developing just this kind of confidence.
7. You Are Your Own Sensor. A guiding principle for the XB is to create personal health heuristics that can outlast a technology. To that end, the heuristics we are building are ones that will support health without requiring technology beyond one’s senses. When we ask “How do you feel?” as the main question, we aim to connect the sensory-oriented heuristic with the visceral practice. With a range of health heuristics, they will also have the start of building fundamentals, as we have seen from our participants to debug their experiences when they feel better or worse.
Future Work
The work presented here showed XBs were successful in helping the people who used them build resilient health heuristics across three exploratory studies. With this, future work is needed to do more rigorous evaluation of the work. In addition, greater study of the proposed XB Framework elements would be valuable: are all seven of the proposed XB framework components equally necessary to create this effect as a gestalt? For example, while SDT requires “relatedness” as part of best support for engagement, Study Two did not have that feature, and yet participants engaged and built lasting skills. Future work should examine how study participation itself may have played a role in enacting a sense of relatedness, as implied by self-determination theory (Stevens et al., 2016). Future work may tease apart these attributes to better understand when and where any of these attributes may be more critical. Various participants have requested being able to “stack” experiments. We see this as valuable, as participants could blend the in five with their own variables. For instance, a participant may wish to put movement and sleep together with consideration of light and air quality: does movement indoors vs. outdoors affect sleep quality or daytime alertness? We are also keen to support group use for XBs to explore how collective health practices may inform health and wellbeing cultures in groups, and where the experimental outputs may be used as evidence/support to underpin organizational decision making as co-design rather than performance monitoring. Fundamentally, we are most keen to see how others might use the XB framework to create new experiment boxes as we anticipate that the framework can generalize to other life-skill learning, beyond health, as well.
Conclusion
Our three studies produced both a clear definition of the XB framework plus also, across three proof-of-concept studies, a clear signal of effect. Together, this work establishes the XB framework and provides justification for further research and investment in it. In particular, we propose a newly proposed continuum across two dimensions: outsourcing to insourcing and habit to heuristic to guide and interpret development and testing of interactive health technologies. The XB lets us ask, as a design question, where the ownership of a health practice is being developed: trust in the device/prescription in outsourcing, or knowledge, skills, and practice-building in the individual? We suggest this framing may help create new kinds of health interactions in particular that may be attractive to the known groups of users for whom health tracking is a non-starter or has been abandoned, but for whom engagement in health practices would be a benefit. This work also proposed health heuristics as a complement to the more familiar concept health habits. The key to this distinction is that a personal health heuristic enables a person to adapt their practice to different contexts. This contextual resilience is essential as it aligns well with the evolving definition of health (Nobile, 2014) as a process of adapting, of dynamic resilience, rather than state.
Key contributions of this work include the XB framework to instantiate health “Experiments in a Box” to help individuals explore, evaluate and develop personal health heuristics. We offer several formative studies that demonstrate how the XB model can be instantiated both broadly across health topics and more deeply within one health topic. The XB is framed as an “experiment,” as the word is used colloquially, to foreground the opportunity to evaluate the utility of a heuristic and that it may or may not work for an individual as a way to help normalize this evaluation as part of building a health practice. We frame this experimental engagement as translating a general health heuristic to a personal health heuristic. We show that with even short engagement with the XB process, participants develop health practices from the interventions that are still in use long after the intervention is finished. Overall, the work offers a new scaffolding by which to frame time-limited interactive technology interventions to support building the knowledge skills and practice that can thrive in that person, significantly both post-interventions, and independent of that technology.
Data Availability Statement
The raw data supporting the conclusion of this article can be made available by the authors, upon request, without undue reservation.
Ethics Statement
The studies involving human participants were reviewed and approved by the ERGO Ethics and Research Governance Office (43589.A1), University of Southampton. The patients/participants provided their written informed consent to participate in this study. ERGO Ethics and Research Governance Office, University of Southampton (43589.A1).
Author Contributions
MS developed the XB approach and study one; GM co-designed and lead study two; EH co-designed study three and contributed to analysis and organization of results.
Funding
UKRI EPSRC Health Resilience Interactive Technology, ReFresh and GetAMoveOn (No. EP/T007656/1, EP/K021907/1, and EP/N027299/1) are various government funded projects, the work from which has enabled the exploration of the ideas in this paper.
Conflict of Interest
The authors declare that the research was conducted in the absence of any commercial or financial relationships that could be construed as a potential conflict of interest.
Acknowledgments
We acknowledge support from the UKRI EPSRC, in particular, the Health Resilience Interactive Technology Established Career Fellowship, the ReFresh Project and the GetAMoveOn Health Network+ (EP/T007656/1, EP/K021907/1, EP/N027299/1). We also acknowledge support from the office of the Pro Vice Chancellor, Interdisciplinary Research, University of Southampton, United Kingdom. Finally, we are grateful to the reviewers whose excellent feedback only enhanced this work.
Footnotes
1https://www.apple.com/uk/watchos/watchos-7/
2In just the past ten years, 6481 papers in the ACM Digital Library include “behavior change” in the title, most in HCI (sigCHI) associated venues.
References
Agapie, E., Colusso, L., Munson, S. A., and Hsieh, G. (2016). “PlanSourcing,” in Proceedings of the 19th ACM Conference on Computer-Supported Cooperative Work & Social Computing (CSCW ’16. New York, NY, USA: ACM), 119–133. doi:10.1145/2818048.2819943
Ajana, B. (2017). Digital Health and the Biopolitics of the Quantified Self. Digital Health 3, 205520761668950. doi:10.1177/2055207616689509
Allen, Hunter., Coggan, Andrew. R., and McGregor, Stephen. (2019). Training and Racing with a Power Meter. Boulder, CO, USA: VeloPress.
Ameen, Mohamed. S., Cheung, Lok., Hauser, Theresa., Hahn, Michael. A., and Schabus, Manuel. (2019). About the Accuracy and Problems of Consumer Devices in the Assessment of Sleep. Sensors 19 (19), 4160. doi:10.3390/s19194160
Barcena, Mario. Ballano., Wueest, Candid., and Lau, Hon. How Safe Is Your Quantified Self? SECURITY RESPONSE 38.
Baron, K. G., Abbott, S., Jao, N., Manalo, N., and Mullen, R. (2017). Orthosomnia: Are Some Patients Taking the Quantified Self Too Far? J. Clin. Sleep Med. 13 (2), 351–354. doi:10.5664/jcsm.6472
Barry, V. W., Baruth, M., Beets, M. W., Durstine, J. L., Liu, J., and Blair, S. N. (2014). Fitness vs. Fatness on All-Cause Mortality: A Meta-Analysis. Prog. Cardiovasc. Dis. 56 (4), 382–390. doi:10.1016/j.pcad.2013.09.002
Bauer, J., Consolvo, S., Benjamin, G., Schooler, J., Wu, E., Watson, N. F., et al. (2012). “ShutEye,” in Proceedings of the SIGCHI Conference on Human Factors in Computing Systems (CHI ’12. New York, NY, USA: ACM), 1401–1410. doi:10.1145/2207676.2208600
Bechara, A., Damasio, H., and DamasioDamasio, A. R. (2003). Role of the Amygdala in Decision-Making. Ann. N Y Acad. Sci. 985, 356–369. doi:10.1111/j.1749-6632.2003.tb07094.x
Bentley, F., Tollmar, K., Stephenson, P., Levy, L., Jones, B., Robertson, S., et al. (2013). Health Mashups. ACM Trans. Comput.-Hum. Interact. 20 (5), 1–27. doi:10.1145/2503823
Destro, B., Paolo Anagnostou, G., Capocasa, M., Bencivelli, S., Cerron, A., Contreras, J., Enke, N., et al. (2014). Perspectives on Open Science and Scientific Data Sharing: An Interdisciplinary Workshop (Rochester, NY: Social Science Research Network). SSRN Scholarly Paper ID 2456712. https://papers.ssrn.com/abstract=2456712
Blandford, A. (2019). HCI for Health and Wellbeing: Challenges and Opportunities. Int. J. Human-Computer Stud. 131, 41–51. doi:10.1016/j.ijhcs.2019.06.007
Bot, B. M., Suver, C., Neto, E. C., Kellen, M., Klein, A., Bare, C., et al. (2016). The MPower Study, Parkinson Disease Mobile Data Collected Using ResearchKit. Sci. Data 3 (1), 1–9. doi:10.1038/sdata.2016.11
Brooks, Richard (2020). ‘The Failure of Test and Trace Shows the Folly of Handing Huge Contracts to Private Giants | Richard Brooks’. London, United Kingdom: The Guardian. http://www.theguardian.com/commentisfree/2020/oct/13/failure-test-trace-folly-huge-contracts-private-giants-uk.
Buman, M. P., Giacobbi, P. R., Dzierzewski, J. M., Morgan, A. A., McCrae, C. S., Roberts, B. L., et al. (2011). Peer Volunteers Improve Long-Term Maintenance of Physical Activity with Older Adults: A Randomized Controlled Trial. J. Phys. Activity Health 8 (s2), S257–S266. doi:10.1123/jpah.8.s2.s257
Chan, R., Shum, D., Toulopoulou, T., and Chen, E. (2008). Assessment of Executive Functions: Review of Instruments and Identification of Critical Issues. Arch. Clin. Neuropsychol. 23 (2), 201–216. doi:10.1016/j.acn.2007.08.010
Chevance, G., Perski, O., and Hekler, E. B. (2020). Innovative Methods for Observing and Changing Complex Health Behaviors: Four Propositions. Translational Behav. Med. 11 (2), 676–685. doi:10.1093/tbm/ibaa026
Choe, E. K., Lee, B., Kay, M., Pratt, W., and Kientz, J. A. (2015). “SleepTight,” in Proceedings of the 2015 ACM International Joint Conference on Pervasive and Ubiquitous Computing (UbiComp ’15. New York, NY, USA:: ACM), 121–132. doi:10.1145/2750858.2804266
Choe, E. K., Lee, B., and schraefel, m. c. (2015a). Characterizing Visualization Insights from Quantified Selfers' Personal Data Presentations. IEEE Comput. Grap. Appl. 35 (4), 28–37. doi:10.1109/MCG.2015.51
Churchill, E. F., and schraefel, m. c. (2015). MHealth + Proactive Well-Being = Wellth Creation. Interactions 22 (1), 60–63. doi:10.1145/2690853
Clawson, J., Pater, J. A., Miller, A. D., Mynatt, E. D., and Mamykina, L. (2015). “No Longer Wearing,” in Proceedings of the 2015 ACM International Joint Conference on Pervasive and Ubiquitous Computing (UbiComp ’15. New York, NY, USA: ACM), 647–658. doi:10.1145/2750858.2807554
Consolvo, S., Klasnja, P., McDonald, D. W., Avrahami, D., Froehlich, J., LeGrand, L., et al. (2008). “Flowers or a Robot Army?,” in Proceedings of the 10th International Conference on Ubiquitous Computing (UbiComp ’08. New York, NY, USA:: ACM), 54–63. doi:10.1145/1409635.1409644
Czajkowski, S. M., Powell, L. H. Nancy. Adler., Adler, N., Naar-King, S., Reynolds, K. D., Hunter, C. M., et al. (2015). From Ideas to Efficacy: The ORBIT Model for Developing Behavioral Treatments for Chronic Diseases. Health Psychol. 34 (10), 971–982. doi:10.1037/hea0000161
Daskalova, N., Desingh, K., Papoutsaki, A., Schulze, D., Sha, H., and Huang, J. (2017). Lessons Learned from Two Cohorts of Personal Informatics Self-Experiments. Proc. ACM Interact. Mob. Wearable Ubiquitous Technol. 1 (3), 1–22. doi:10.1145/3130911
Daskalova, N., Metaxa-Kakavouli, D., Tran, A., Nugent, N., Boergers, J., McGeary, J., et al. (2016). “SleepCoacher,” in Proceedings of the 29th Annual Symposium on User Interface Software and Technology (UIST ’16. New York, NY, USA:: ACM), 347–358. doi:10.1145/2984511.2984534
Dinis, J., and Bragança, M. (2018). Quality of Sleep and Depression in College Students: A Systematic Review. Sleep Sci. 11 (4), 290–301. doi:10.5935/1984-0063.20180045
Duan, N., Kravitz, R. L., and Schmid, C. H. (2013). Single-Patient (N-of-1) Trials: A Pragmatic Clinical Decision Methodology for Patient-Centered Comparative Effectiveness Research. J. Clin. Epidemiol. 66 (8), S21–S28. doi:10.1016/j.jclinepi.2013.04.006
Dulaud, P., Di Loreto, I., and Mottet, D. (2020). Self-Quantification Systems to Support Physical Activity: From Theory to Implementation Principles. Ijerph 17 (24), 9350. doi:10.3390/ijerph17249350
Epstein, D. A., Caraway, M., Johnston, C., Ping, A., Fogarty, J., and Munson, S. A. (2016). “Beyond Abandonment to Next Steps,” in Proceedings of the 2016 CHI Conference on Human Factors in Computing Systems (CHI ’16. New York, NY, USA: ACM), 1109–1113. doi:10.1145/2858036.2858045
Erbas, Y., Ceulemans, E., Kalokerinos, E. K., Houben, M., Koval, P., Pe, M. L., et al. (2018). Why I Don't Always Know what I'm Feeling: The Role of Stress in Within-Person Fluctuations in Emotion Differentiation. J. Personal. Soc. Psychol. 115 (2), 179–191. doi:10.1037/pspa0000126
Espie, C. A., Kyle, S. D., Hames, P., Gardani, M., Fleming, L., and Cape, J. (2014). The Sleep Condition Indicator: a Clinical Screening Tool to Evaluate Insomnia Disorder:. BMJ Open 4 (3), e004183. doi:10.1136/bmjopen-2013-004183
Feldman, R. (2020). What Is Resilience: An Affiliative Neuroscience Approach. World Psychiatry 19 (2), 132–150. doi:10.1002/wps.20729
Ferriss, Timothy. (2009). The 4-Hour Workweek: Escape 9-5, Live Anywhere, and Join the New Rich. Expanded, Updated Ed. Edition. New York: Harmony.
Freedland, Kenneth. E. (2020a). Pilot Trials in Health-Related Behavioral Intervention Research: Problems, Solutions, and Recommendations. Health Psychol. 39 (10), 851–862. doi:10.1037/hea0000946
Freedland, Kenneth. E. (2020b). Purpose-Guided Trial Design in Health-Related Behavioral Intervention Research. Health Psychol. 39 (6), 539–548. doi:10.1037/hea0000867
Griffith, E. (2020). ‘Peloton: The Latest Virus Panic Buy’. Chicagotribune.Com. New York, NY, USA. 7 May 2020. https://www.chicagotribune.com/coronavirus/sns-nyt-peloton-boom-workout-exercise-equipment-coronavirus-20200509-vu47jlvg2fdkxapxxtt6zx7lqe-story.html.
Haidt, Jonathan. (2012). The Righteous Mind: Why Good People Are Divided by Politics and Religion. New York, NY, USA: Vintage.
Haraway, Donna. Jeanne. (1991). Simians, Cyborgs, and Women: The Reinvention of Nature. New York: Routledge. http://archive.org/details/simianscyborgswo0000hara.
Hashemzadeh, M., Rahimi, A., Zare-Farashbandi, F., Alavi-Naeini, A. M., and Daei, A.i (2019). Transtheoretical Model of Health Behavioral Change: A Systematic Review. Iran J. Nurs. Midwifery Res. 24 (2), 83–90. doi:10.4103/ijnmr.IJNMR_94_17
Heffernan, Margaret. (2013). What Happened after the Foxconn Suicides. New York, NY: CBSNews. CBS Interactive.
Hekler, E. B., Buman, M. P., Rivera, Poothakandiyil. N., Dzierzewski, Joseph. M., Morgan, Adrienne. Aiken., Rivera, D. E., et al. (2013). Exploring Behavioral Markers of Long-Term Physical Activity Maintenance. Health Educ. Behav. 40 (1_Suppl. l), 51S–62S. doi:10.1177/1090198113496787
Hekler, E. B., Rivera, D. E., Martin, Cesar. A., Phatak, Sayali. S., Freigoun, Mohammad. T., Korinek, Elizabeth., et al. (2018). Tutorial for Using Control Systems Engineering to Optimize Adaptive Mobile Health Interventions. J. Med. Internet Res. 20 (6), e214. doi:10.2196/jmir.8622
Hekler, Eric. B., Klasnja, Predrag., Chevance, Guillaume., Golaszewski, Natalie. M., Lewis, Dana., and Sim, Ida. (2019). Why We Need a Small Data Paradigm. BMC Med. 17 (1), 1–9. doi:10.1186/s12916-019-1366-x
Hekler, Eric. B. (2013). “Winslow Burleson, and Jisoo LeeA DIY Self-Experimentation Toolkit for Behavior Change,” in ACM Conference on Human Factors in Computing Systems. doi:10.1145/2470654.2466452
Hekler, E., Tiro, J. A., Hunter, C. M., and Nebeker, C. (2020). Precision Health: The Role of the Social and Behavioral Sciences in Advancing the Vision. Ann. Behav. Med. 54 (11), 805–826. doi:10.1093/abm/kaaa018
Heyen, N. B. (2020). From Self-Tracking to Self-Expertise: The Production of Self-Related Knowledge by Doing Personal Science. Public Underst Sci. 29 (2), 124–138. doi:10.1177/0963662519888757
Ibáñez, V., Silva, J., and Cauli, O. (2018). A Survey on Sleep Questionnaires and Diaries. Sleep Med. 42, 90–96. doi:10.1016/j.sleep.2017.08.026
Ji, S., Cherry, C. R., Han, L. D., and Jordan, D. A. (2014). Electric Bike Sharing: Simulation of User Demand and System Availability. J. Clean. Prod. 85, 250–257. doi:10.1016/j.jclepro.2013.09.024
Karkar, R., Fogarty, J., Kientz, J. A., Munson, S. A., Vilardaga, R., and Zia, J. (2015). “Opportunities and Challenges for Self-Experimentation in Self-Tracking,” in Proceedings of the 2015 ACM International Joint Conference on Pervasive and Ubiquitous Computing and Proceedings of the 2015 ACM International Symposium on Wearable Computers - UbiComp ’15 (Osaka, Japan: ACM Press), 991–996. doi:10.1145/2800835.2800949
Karkar, R., Schroeder, J., Epstein, D. A., Pina, L. R., Scofield, J., Fogarty, J., et al. (2017). TummyTrials. CHI Conf. 2017, 6850–6863. doi:10.1145/3025453.3025480
Karkar, R., Zia, J., Vilardaga, R., Mishra, S. R., Fogarty, J., Munson, S. A., et al. (2016). A Framework for Self-Experimentation in Personalized Health. J. Am. Med. Inform. Assoc. JAMIA 23 (3), 440–448. doi:10.1093/jamia/ocv150
Kay, M., Morris, D., schraefel, m., and Kientz, J. A. (2013). “There's No Such Thing as Gaining a Pound,” in Proceedings of the 2013 ACM International Joint Conference on Pervasive and Ubiquitous Computing (UbiComp ’13. New York, NY, USA:: ACM), 401–410. doi:10.1145/2493432.2493456
Kearney, Lauren. (2018). 20 Tactics Bear Grylls Uses to Survive the Wilderness (That Actually Work). Quebec, Canada: TheTravel17 August 2018. https://www.thetravel.com/20-tactics-bear-grylls-uses-to-survive-the-wilderness-that-actually-work/
Kleckner, Ian. R., Zhang, Jiahe., Touroutoglou, Alexandra., Chanes, Lorena., Xia, Chenjie., Simmons, W. Kyle., et al. (2017). Evidence for a Large-Scale Brain System Supporting Allostasis and Interoception in Humans. Nat. Hum. Behav. 1 (5), 1–14. doi:10.1038/s41562-017-0069
Kravitz, Richard. L., Aguilera, Adrian., Chen, Elaine. J., Choi, Yong. K., Hekler, Eric., Karr, Chris., et al. (2020). Feasibility, Acceptability, and Influence of MHealth-Supported N-Of-1 Trials for Enhanced Cognitive and Emotional Well-Being in US Volunteers. Front. Public Health 8, 260. doi:10.3389/fpubh.2020.00260
Kwasnicka, D., Dombrowski, S. U., White, M., and Sniehotta, F. (2016). Theoretical Explanations for Maintenance of Behaviour Change: A Systematic Review of Behaviour Theories. Health Psychol. Rev. 10 (3), 277–296. doi:10.1080/17437199.2016.1151372
Lee, J., Walker, E., Burleson, W., Kay, M., Buman, M., Hekler, E. B., et al. (2017). “Self-Experimentation for Behavior Change: Design and Formative Evaluation of Two Approaches,” in Proceedings of the 2017 CHI Conference on Human Factors in Computing Systems (New York, NY, USA: Association for Computing Machinery), 6837–6849. doi:10.1145/3025453.3026038
Lerman, Rachel. (2020). Feeling Stressed? Meditation Apps See Surge in Group Relaxation. Washington, DC: Washington Post. https://www.washingtonpost.com/technology/2020/04/21/meditation-up-during-coronavirus/.
Li, I., Dey, A. K., and Forlizzi, J. (2011). “Understanding My Data, Myself,” in Proceedings of the 13th International Conference on Ubiquitous Computing (UbiComp ’11. New York, NY, USA:: ACM), 405–414. doi:10.1145/2030112.2030166
Lindquist, K. A., Wager, T. D., Kober, H., Bliss-Moreau, E., and Barrett, L. F. (2012). The Brain Basis of Emotion: A Meta-Analytic Review. Behav. Brain Sci. 35 (3), 121–143. doi:10.1017/s0140525x11000446
Lockton, D., Zea-Wolfson, T., Chou, J., Song, Y., Ryan, E., and Walsh, C. (2020). “Sleep Ecologies,” in Proceedings of the 2020 ACM Designing Interactive Systems Conference (New York, NY, USA: Association for Computing Machinery), 1579–1591. doi:10.1145/3357236.3395482
Loke, L., and Schiphorst, T. (2018). The Somatic Turn in Human-Computer Interaction. Interactions 25 (5), 54–5863. doi:10.1145/3236675
Louzon, P., Jessica, L. A., Torres, X., Pyles, E., Ali, M., Du, Y., et al. (2020). Characterisation of ICU Sleep by a Commercially Available Activity Tracker and its Agreement with Patient-Perceived Sleep Quality. BMJ Open Resp Res. 7 (1), e000572. doi:10.1136/bmjresp-2020-000572
Luft, A. R., and Buitrago, M. M. (2005). Stages of Motor Skill Learning. Mn 32 (3), 205–216. doi:10.1385/mn:32:3:205
Lunde, P., Nilsson, B. B., Bergland, A., Kværner, K. J., and Bye, A. (2018). The Effectiveness of Smartphone Apps for Lifestyle Improvement in Noncommunicable Diseases: Systematic Review and Meta-Analyses. J. Med. Internet Res. 20 (5), e162. doi:10.2196/jmir.9751
Mamykina, L., Mynatt, E., Davidson, P., and Greenblatt, D. (2008). “Mahi,” in Proceedings of the SIGCHI Conference on Human Factors in Computing Systems (CHI ’08. New York, NY, USA:: ACM), 477–486. doi:10.1145/1357054.1357131
Mander, B. A., Winer, J. R., and Walker, M. P. (2017). Sleep and Human Aging. Neuron 94 (1), 19–36. doi:10.1016/j.neuron.2017.02.004
Manzar, M. D., BaHammam, A. S., SpenceHameed, D. W., Pandi-Perumal, S. R., Moscovitch, A., and Streiner, D. L. (2018). Dimensionality of the Pittsburgh Sleep Quality Index: A Systematic Review. Health Qual. Life Outcomes 16 (1), 89. doi:10.1186/s12955-018-0915-x
Mary, A., Schreiner, S., and Peigneux, P. (2013). Accelerated Long-Term Forgetting in Aging and Intra-sleep Awakenings. Front. Psychol. 4, 750. doi:10.3389/fpsyg.2013.00750
McKay, F. H., Cheng, C., Wright, A., Shill, J., Stephens, H., and Uccellini, M. (2018). Evaluating Mobile Phone Applications for Health Behaviour Change: A Systematic Review. J. Telemed. Telecare 24 (1), 22–30. doi:10.1177/1357633X16673538
Milojevich, H. M., and Lukowski, A. F. (2016). Sleep and Mental Health in Undergraduate Students with Generally Healthy Sleep Habits. PLOS ONE 11 (6), e0156372. doi:10.1371/journal.pone.0156372
Murphy, J., Catmur, C., and Bird, G. (2019). Classifying Individual Differences in Interoception: Implications for the Measurement of Interoceptive Awareness. Psychon. Bull. Rev. 26 (5), 1467–1471. doi:10.3758/s13423-019-01632-7
Nebeker, Camille., Weisberg, Bethany., Hekler, Eric., and Kurisu, Michael. (2020). Using Self-Study and Peer-To-Peer Support to Change “Sick” Care to “Health” Care: The Patient Perspective, Front. Digit. Health, doi:10.3389/fdgth.2020.00002
Nielsen, J., and Molich, R. (1990). “Heuristic Evaluation of User Interfaces,” in Proceedings of the SIGCHI Conference on Human Factors in Computing Systes (CHI ’90. New York, NY, USA: Association for Computing Machinery), 249–256. doi:10.1145/97243.97281
Ogilvy Consulting UK. (2014). 5 Ogilvy Behavioural Science Wellbeing Experiment. https://www.youtube.com/watch?v=UOS7ykGgzeA&ab_channel=OgilvyConsultingUK. doi:10.1093/obo/9780199830060-0106
Olszewska, Alicja. M., Gaca, Maciej., Herman, Aleksandra. M., Jednoróg, Katarzyna., and Marchewka, Artur. (2021). How Musical Training Shapes the Adult Brain: Predispositions and Neuroplasticity. Front. Neurosci. 15, 630829. doi:10.3389/fnins.2021.630829
Oulasvirta, A., Rattenbury, T., Ma, L., and Raita, E. (2012). Habits Make Smartphone Use More Pervasive. Pers Ubiquit Comput. 16 (1), 105–114. doi:10.1007/s00779-011-0412-2
Pandey, V., Amir, A., Debelius, J., Hyde, E. R., Kosciolek, T., Knight, R., et al. (2017). “Gut Instinct,” in Proceedings of the 2017 CHI Conference on Human Factors in Computing Systems - CHI ’17 (Denver, Colorado, USA: ACM Press), 6825–6836. doi:10.1145/3025453.3025769
Phatak, Sayali., Chen, Elaine., Stephen, Schueller, Kravitz, Richard., Sim, Ida., Schmid, Christopher., et al. (2018). “Design of a Large-Scale Self-Experimentation Tool for Scientific Self-Explorations,” in 12th EAI International Conference on Pervasive Computing Technologies for Healthcare – Demos (New York: Posters, Doctoral Colloquium). doi:10.4108/eai.20-4-2018.2276355
Pierce, J., Schiano, D. J., and Paulos, E. (2010). “Home, Habits, and Energy,” in Proceedings Of the SIGCHI Conference On Human Factors In Computing Systems, 1985–94 (New York, NY, USA: Association for Computing Machinery). doi:10.1145/1753326.1753627
Pinder, C., Vermeulen, J., Cowan, B. R., and Beale, R. (2018). Digital Behaviour Change Interventions to Break and Form Habits. ACM Trans. Comput.-Hum. Interact. 25 (3), 1–66. doi:10.1145/3196830
Prince, J. D. (2014). The Quantified Self: Operationalizing the Quotidien. J. Electron. Resour. Med. Libraries 11 (2), 91–99. doi:10.1080/15424065.2014.909145
Prochaska, J. O., and Velicer, W. F. (1997). The Transtheoretical Model of Health Behavior Change. Am. J. Health Promot. 12 (1), 38–48. doi:10.4278/0890-1171-12.1.38
Rahman, T., Czerwinski, M., Gilad-Bachrach, R., and Johns, P. (2016). “Predicting "About-To-Eat" Moments for Just-In-Time Eating Intervention,” in Proceedings of the 6th International Conference on Digital Health Conference (DH ’16. New York, NY, USA: Association for Computing Machinery), 141–150. doi:10.1145/2896338.2896359
Riley, W. T., Rivera, D. E., Atienza, A. A., Nilsen, W., Allison, S. M., and Mermelstein, R. (2011). Health Behavior Models in the Age of Mobile Interventions: Are Our Theories up to the Task? Behav. Med. Pract. Pol. Res. 1 (1), 53–71. doi:10.1007/s13142-011-0021-7
Sabrina, Siddiqui., and Sadie, Gurman. (2021). Biden Signs Executive Order to Phase Out Federal Use of Private Prisons. Wall Street J. 26. January 2021, sec. Politics. https://www.wsj.com/articles/biden-to-sign-executive-orders-to-phase-out-federal-use-of-private-prisons-11611682272
Sanches, P., Janson, A., Karpashevich, P., Nadal, C., Qu, C., Roquet, Claudia. Daudén., et al. (2019). “HCI and Affective Health,” in Proceedings of the 2019 CHI Conference on Human Factors in Computing Systems (CHI ’19. New York, NY, USA: Association for Computing Machinery), 1–17. doi:10.1145/3290605.3300475
Schlarb, Angelika., Bihlmaier, Isabel., Hautzinger, Martin., Gulewitsch, Marco. D., and Schwerdtle, Barbara. (2015). Nightmares and Associations with Sleep Quality and Self-Efficacy Among University Students. J. Sleep Disord. Manag. 11–5. https://pub.uni-bielefeld.de/publication/2910766.
schraefel, m. c., Andres, Josh., Liu, Abby. Wanyu., Jones, Mike., Murname, Elizabeth., Tabor, Aaron., et al. (2021). Body as Starting Point 4: Inbodied Interaction Design for Health Ownership. New York, NY: Extended Abstracts of the 2021 CHI Conference on Human Factors in Computing Systems. https://wellthlab.ac.uk/inbodied4.
schraefel, m. c. (2014). “Burn the Chair, We’Re Wired to Move: Exploring the Brain/Body Connexion for HCI Creativity & Cognition,” in Proceedings Of HCI Korea, 153–61. HCIK ’15 (South Korea: Hanbit Media, Inc). http://dl.acm.org/citation.cfm?id=2729485.2729509.
schraefel, m. c. (2019). “in5,” in Extended Abstracts of the 2019 CHI Conference on Human Factors in Computing Systems (CHI EA ’19. New York, NY, USA: Association for Computing Machinery), 1–6. doi:10.1145/3290607.3312977
schraefel, m. c. (2020). Inbodied Interaction: Introduction. Interactions 27 (2), 32–37. doi:10.1145/3380811
schraefel, m. c., Tabor, A., and Andres., J. (2020). Toward Insourcing-Measurement in Inbodied Interaction Design. Interactions 27 (2), 56–60. doi:10.1145/3381340
Sherwin, E., Rea, K., Dinan, T. G., and Cryan, J. F. (2016). A Gut (Microbiome) Feeling about the Brain. Curr. Opin. Gastroenterol. 32 (2), 96–102. doi:10.1097/MOG.0000000000000244
Spence, S. (1995). Descartes' Error: Emotion, Reason and the Human Brain. BMJ 310 (6988), 1213. doi:10.1136/bmj.310.6988.1213
Stawarz, K., Cox, A. L., and Blandford, A. (2015). “Beyond Self-Tracking and Reminders,” in Proceedings of the 33rd Annual ACM Conference on Human Factors in Computing Systems (CHI ’15. New York, NY, USA: ACM), 2653–2662. doi:10.1145/2702123.2702230
Stevens, S. L., Wood, S., Koshiaris, C., Law, K., Glasziou, P., Stevens, R. J., et al. (2016). Blood Pressure Variability and Cardiovascular Disease: Systematic Review and Meta-Analysis. Bmj 354, i4098. doi:10.1136/bmj.i4098
Sullivan, Alice., and Brown, Matt. (2013). Overweight and Obesity in Mid-life: Evidence from the 1970 Birth Cohort Study at Age 42. London, UK: Centre for Longitudinal Studies, Institute of Education, University of London. http://discovery.ucl.ac.uk/10018085/.
The Sufferfest, ‘The Sufferfest: Complete Training App for Cyclists and Triathletes’. n.d. Atlanta, GA: The SufferfestAccessed 29 January 2021. https://thesufferfest.com/.
Thys, E., Sabbe, B., and De Hert, M. (2014). The Assessment of Creativity in Creativity/psychopathology Research - a Systematic Review. Cogn. Neuropsychiatry 19 (4), 359–377. doi:10.1080/13546805.2013.877384
TrainingPeaks, ‘TrainingPeaks | New Year. New Focus.’ n.d. New York, NY: TrainingPeaksAccessed 29 January 2021. https://www.trainingpeaks.com/.
Troacă, Victor-Adrian., and Bodislav, Dumitru-Alexandru. (2012). Outsourcing. The Concept. Theor. Appl. Econ. 6 (6), 51.
Tsakiris, M., and Critchley, H. (2016). Interoception beyond Homeostasis: Affect, Cognition and Mental Health. Phil. Trans. R. Soc. B 371, 20160002. doi:10.1098/rstb.2016.0002
Tuominen, J., Peltola, K., Saaresranta, T., and Valli, K. (2019). Sleep Parameter Assessment Accuracy of a Consumer Home Sleep Monitoring Ballistocardiograph Beddit Sleep Tracker: A Validation Study. J. Clin. Sleep Med. 15 (3), 483–487. doi:10.5664/jcsm.7682
Vandercammen, L., Hofmans, J., and Theuns, P. (2014). Relating Specific Emotions to Intrinsic Motivation: On the Moderating Role of Positive and Negative Emotion Differentiation. PLoS One 9 (12), e115396. doi:10.1371/journal.pone.0115396
Verkhoshansky, , Y., Vitalievitch, and Cunningham Siff, Mel. (2009). Supertraining 6th Ed. - Expanded Ed. Rome, Italy: [Michigan: Verkhoshansky ; Distributed by Ultimate Athlete Concepts.
West, J. H., Hall, P. C., Hanson, C. L., Barnes, M. D., Giraud-Carrier, C., and Barrett, J. (2012). There's an App for that: Content Analysis of Paid Health and Fitness Apps‘There’s an App for that: Content Analysis of Paid Health and Fitness Apps’. J. Med. Internet Res. 14 (3), e72. doi:10.2196/jmir.1977
Wolf, Gary. Isaac., and De Groot, Martijn. (2021). A Conceptual Framework for Personal Science. Frontiers in Computer Science 2. https://doi.org/10.3389/fcomp.2020.0002.
Wood, W., and Rünger, D. (2016). Psychology of Habit. Annu. Rev. Psychol. 67 (1), 289–314. doi:10.1146/annurev-psych-122414-033417
Zucker, D. R., Schmid, C. H., McIntosh, M. W., D'Agostino, R. B., Selker, H. P., and Lau, J. (1997). Combining Single Patient (N-Of-1) Trials to Estimate Population Treatment Effects and to Evaluate Individual Patient Responses to Treatment. J. Clin. Epidemiol. 50 (4), 401–410. doi:10.1016/S0895-4356(96)00429-5
Keywords: inbodied interaction, insourcing, outsourcing, continuua, inbodied, knowledge skills and practice, ksp, heuristics
Citation: schraefel mc, Muresan GC and Hekler E (2021) Experiment in a Box (XB): An Interactive Technology Framework for Sustainable Health Practices. Front. Comput. Sci. 3:661890. doi: 10.3389/fcomp.2021.661890
Received: 31 January 2021; Accepted: 06 May 2021;
Published: 26 May 2021.
Edited by:
Gennaro Cordasco, University of Campania Luigi Vanvitelli, ItalyReviewed by:
Miriam Sturdee, Lancaster University, United KingdomMike Fraser, University of Bath, United Kingdom
Copyright © 2021 schraefel, Muresan and Hekler. This is an open-access article distributed under the terms of the Creative Commons Attribution License (CC BY). The use, distribution or reproduction in other forums is permitted, provided the original author(s) and the copyright owner(s) are credited and that the original publication in this journal is cited, in accordance with accepted academic practice. No use, distribution or reproduction is permitted which does not comply with these terms.
*Correspondence: m. c. schraefel, mc+w@ecs.soton.ac.uk