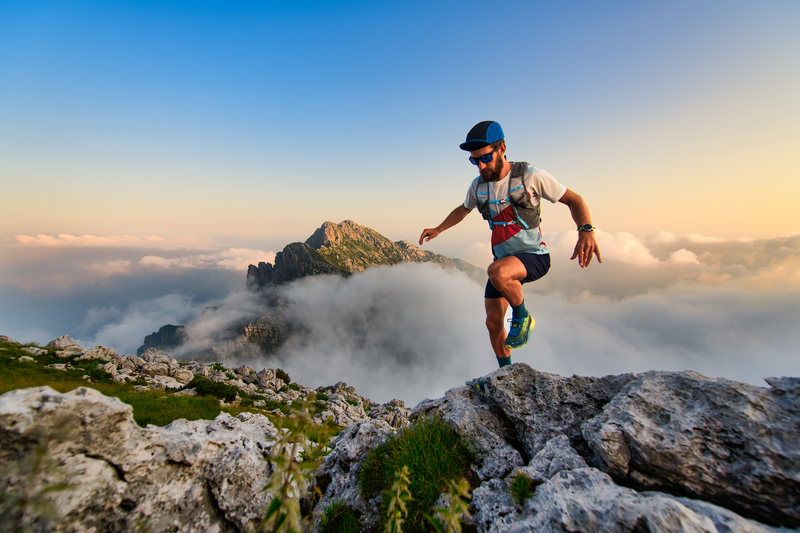
95% of researchers rate our articles as excellent or good
Learn more about the work of our research integrity team to safeguard the quality of each article we publish.
Find out more
MINI REVIEW article
Front. Comput. Sci. , 30 April 2021
Sec. Human-Media Interaction
Volume 3 - 2021 | https://doi.org/10.3389/fcomp.2021.661178
This article is part of the Research Topic Brain-Computer Interfaces for Non-clinical (Home, Sports, Art, Entertainment, Education, Well-being) Applications View all 12 articles
The ability to discriminate and attend one specific sound source in a complex auditory environment is a fundamental skill for efficient communication. Indeed, it allows us to follow a family conversation or discuss with a friend in a bar. This ability is challenged in hearing-impaired individuals and more precisely in those with a cochlear implant (CI). Indeed, due to the limited spectral resolution of the implant, auditory perception remains quite poor in a noisy environment or in presence of simultaneous auditory sources. Recent methodological advances allow now to detect, on the basis of neural signals, which auditory stream within a set of multiple concurrent streams an individual is attending to. This approach, called EEG-based auditory attention detection (AAD), is based on fundamental research findings demonstrating that, in a multi speech scenario, cortical tracking of the envelope of the attended speech is enhanced compared to the unattended speech. Following these findings, other studies showed that it is possible to use EEG/MEG (Electroencephalography/Magnetoencephalography) to explore auditory attention during speech listening in a Cocktail-party-like scenario. Overall, these findings make it possible to conceive next-generation hearing aids combining customary technology and AAD. Importantly, AAD has also a great potential in the context of passive BCI, in the educational context as well as in the context of interactive music performances. In this mini review, we firstly present the different approaches of AAD and the main limitations of the global concept. We then expose its potential applications in the world of non-clinical passive BCI.
The ability to discriminate and attend one specific sound source in a complex auditory environment is of utmost importance in the animal world both in terms of avoiding dangers and finding mates. In humans, this ability goes well-beyond survival and reproduction since it is a fundamental skill for efficient communication. Indeed, it allows us to follow a family conversation or discuss with a friend in a bar. In music, this ability is challenged by the simultaneous layering of several instruments playing together, requiring sound source segregation to fully appreciate the ensemble. This ability is also challenged in hearing-impaired individuals and more precisely in those with a cochlear implant (CI). Indeed, due to the limited spectral resolution of the implant, auditory perception remains quite poor in a noisy environment or in presence of simultaneous auditory sources. Thus, being able to enhance the relevant/attended source would facilitate source separation in individuals with CI. However, monitoring the attended auditory source is not easy, as this changes in time.
Recent methodological advances allow now to detect, on the basis of neural signals, which auditory stream within a set of multiple concurrent streams an individual is attending to. This approach, called EEG-based auditory attention detection (AAD), is based on fundamental research findings demonstrating that, in a multi speech scenario, cortical tracking of the envelope of the attended speech is enhanced compared to the unattended speech (Mesgarani et al., 2009; Ding and Simon, 2012; Mesgarani and Chang, 2012; Pasley et al., 2012; Zion Golumbic et al., 2013). Following these findings, other studies showed that it is possible to use EEG/MEG to explore auditory attention during speech listening in a Cocktail-party-like scenario (Ding and Simon, 2012; O'Sullivan et al., 2015; Akram et al., 2016). This field of research has grown rapidly and several new methods and techniques were developed in the last years to improve the first attempts.
Overall, these findings make it possible to conceive next-generation hearing aids combining customary technology and AAD. Importantly, AAD has also a great potential in the context of passive BCI, in the educational context as well as in the context of interactive music performances.
In this mini review, we firstly present the different approaches of AAD and the main limitations of the global concept. We then expose its potential applications in the world of non-clinical passive BCI.
The main rationale behind this mini-review is to bridge the EEG-based AAD and Passive BCI communities and to provide insights about how the emerging synergy will develop. While previous reviews have been published on technical aspects of AAD, this mini-review attempts to briefly present EEG-based AAD in a broader perspective and to guide the reader to the most relevant sources. The methodology used to find and include papers in the current mini-review was as follows. The search was carried on using both Pubmed and Google Scholar. Keywords included machine learning, decoding, encoding, auditory attention, EEG, and speech. Pubmed gave 88 results and Scholar 8,460 results. These results were then filtered with the following exclusion criteria: articles about engineering techniques that are not directly in relation with EEG-based AAD methodology, articles with methods that were not applied to M/EEG data, articles that were not published in a peer-review journal, articles that were cited <1 time. This reduced the number of included articles to 20 (see Table 1).
There are many different AAD methods based on EEG measures. Identifying the attended speaker using cortical activity measurement is possible because the amplitude envelope of the speech stream (a crucial feature for speech comprehension) is represented in the theta and gamma oscillatory activity in the human auditory cortex (Nourski et al., 2009; Giraud and Poeppel, 2012; Kubanek et al., 2013). Attending a source thus results in greater coupling between the envelope of the source and the envelope of neural activity in these bands.
The vast majority of the studies that explored EEG-based AAD performances used two concurrent spatially separated talkers but some of them have explored the impact of speaker number and their location in auditory scene (Schäfer et al., 2018), background noise (Das et al., 2018), reverberation (Fuglsang et al., 2017), number of EEG electrodes (Mirkovic et al., 2015; Bleichner et al., 2016), or even their location (Fiedler et al., 2017) on the performance of AAD algorithms.
One can distinguish two main categories of approaches to detect auditory attention: linear and non-linear models (see Geirnaert et al., 2020 for a comprehensive review of AAD Algorithms).
In the community of linear models, two main “philosophies” are in competition (see Alickovic et al., 2019 for a complete review on linear models): forward, or encoding (encoding because these models are a description of how the system encodes information), and backward, or decoding, models.
The objective of the forward strategy is to predict the neural response in the neural data (i.e., EEG channels) from the representation of the audio signal via a temporal response function (i.e., an encoder) that describes the linear relationship between a set of neural data and an audio stimulus at certain time points (Crosse et al., 2016). In the simplest case (i.e., one audio signal) a unique representation of the audio signal is created. This representation can be the amplitude envelope (O'Sullivan et al., 2015), the spectrogram of speech signal (O'Sullivan et al., 2017), or the Mel spectrogram for a music signal (Cantisani et al., 2019). Depending on the type of the chosen representation the analysis can be either univariate (an amplitude envelope is a univariate stimulus feature) or multivariate (a spectrogram is a multivariate stimulus feature). Although it is possible to use multivariate TRF with the forward approach, this strategy is, by nature, univariate (Crosse et al., 2016). Afterward, the audio representation is convolved with an unknown channel-specific TRF. To estimate the TRF (i.e., fit the model parameters), an error minimization is performed between the neural response and the one predicted by the convolution (e.g., Mean-Squared Error) using assumptions about noise distribution (Holdgraf et al., 2017). Once the model's parameters have been estimated, the model is validated on new data. These new data could be from the same dataset used to estimate the parameters (leave-n-out procedure) or from data recorded separately. The validation step is crucial because, to be interpretable, the model should be compatible with new data and make accurate predictions (generalization ability). Finally, the rationale of the forward strategy, in auditory research, is to predict neural data on the basis of the sound's features.
Backward models work similarly but by predicting the auditory representation based on neural data (Alickovic et al., 2019). A pre-trained neural linear decoder is applied to the neural data to reconstruct the chosen representation (this is the reason why this type of approach is sometimes called “stimulus reconstruction”). The reconstructed representation is compared to the original representations. A high similarity (correlation) indicates a good performance of the model. Two other approaches can also be mentioned: Canonical Correlation Analysis (CCA) and Bayesian state-space modeling. Canonical Correlation Analysis is a hybrid model that combines a decoding and an encoding model. This approach, developed by de Cheveigné et al. (2018), aims to minimize the irrelevant variance in both neural data and stimulus by a linear transformation. Concerning Bayesian state-space modeling (Miran et al., 2018b), it is composed of three modules: a dynamic encoder/decoder estimation module, an attention marker extraction module, and a real-time state-space estimator module (see Miran et al., 2018a for a complete description of the model) and this approach was developed in the purpose of real-time decoding of auditory attention.
As mentioned before, in the context of AAD, linear models are generally used with two (or more) concurrent speech streams in order to determine which stream the listener is attending to. In this case, a representation of each auditory source is created (e.g., speaker 1 and speaker 2). Once the model has been fitted, no matter which approach was chosen, a two-class classifier is used to decide which of the two streams the participant was focused on. To do so, the classifier compares the correlation coefficients between the model output and the original model input representations (e.g., the correlation between the reconstructed envelope and the original audio signals envelopes in backward strategy) over a certain portion of data (decision time windows). The highest correlation indicates which stream the participant was attending to. The length of the decision time window is a crucial parameter because correlation-based measures need a certain amount of information to perform well. However, short decision time windows (<2 s of data) are of interest in BCI for real-time classification.
Generally, AAD performances are assessed with two accuracy metrics: regression accuracy and classification accuracy (Wong et al., 2018). Regression accuracy evaluates the goodness of fit of the model and it is expressed in terms of correlation coefficient (Pearson's correlation, often ranging 0.1–0.2) between the output of the model and the real value (e.g., speech envelope is correlated with reconstructed envelope for backward models). Classification accuracy, on the other hand, evaluates the ability of the classifier to correctly identify the attended stream for a given decision time window and it is generally expressed in terms of percentage of good classification. Classification accuracy is generally high for long decision time windows (around 85% for 60 s of data) but drops drastically for shorter decision time windows no matter which approach is used.
Recently, Wong et al. (2018) showed that decoding models outperform encoding models in terms of classification accuracies. One of the best classification results obtained so far was 85% with 20-s decision time windows, with the CCA (Geirnaert et al., 2020).
Similarly to linear models, several non-linear model architectures are in competition. But non-linear models are still overlooked because they are more complex to implement and interpret. Nevertheless, they were used by a few studies to explore AAD. Vandecapelle et al. (2020) used two convolutional neural networks to determine the attended speaker in a multi-speaker scene by using the direction of the locus of auditory attention. Their method allows them to decode auditory attention with very short decision time windows and with a good classification accuracy (around 80% for 2 s of data). In another study the authors used a fully-connected neural network to reconstruct the speech envelope and estimate the attended speaker (de Taillez et al., 2017). The classification accuracy obtained with this method appears to be similar to the performance obtained in Vandecapelle et al. (2020) even though the comparison between studies is not straightforward due to differences in experimental and model parameters or accuracy measures (Ciccarelli et al., 2019). However, non-linear models outperform linear models in terms of decision time window/performance ratio. One other potential advantage of this type of model is that it seems more realistic insofar as it may capture the neuronal non-linearity underlying speech perception (O'Sullivan et al., 2015, Mirkovic et al., 2015, de Taillez et al., 2017).
Linear and non-linear models yet suffer from several limitations with respect to AAD. The major problem of linear models lies in the fact that their classification accuracy is strongly influenced by the duration of the decision window. Long windows yield good classification (>80%) while short ones (e.g., 2 s) yield much poorer performance (~60%). This is due to the fact that (1) short decision windows contain less information (Vandecapelle et al., 2020), (2) EEG signals contain a mixture of several physiological and neural processes. Thus, correlations between predicted and actual data are rather weak (between 0.05 and 0.2) and short decision time windows are particularly sensitive to noise (Geirnaert et al., 2020). Moreover, a huge amount of data is needed to fit the model properly. Therefore, these models are difficult to use in real time situations where the selection of the attended speaker must be performed as fast as possible.
For non-linear models, the principal issue is the risk of overfitting, in particular with small datasets (Vandecapelle et al., 2020). Moreover, comparing performances of several non-linear models on different datasets pointed to a low reproducibility of these algorithms (Geirnaert et al., 2020). Besides fitting issues and physiological noise (and non-relevant neural signal), another source of performance variability resides in inter-individual differences at the cognitive level, such as for instance in working memory (WM) (Ciccarelli et al., 2019), attentional control, cognitive inhibition, but also motivation.
Classical active Brain Computer Interfaces (aBCI) exploit the user's voluntary brain activity to control applications or devices. Several years ago, a new category of BCI, named passive Brain Computer Interfaces (pBCI), emerged. Unlike aBCI, pBCI use involuntary brain activity (e.g., cognitive state) to implicitly modify human-machine interactions (Zander and Kothe, 2011; Clerc et al., 2016). passive Brain Computer Interfaces are generally used to monitor attention, fatigue, or workload in real life situations such as driving situations (Haufe et al., 2014) or air traffic control (Aricò et al., 2016) but they can also be used in less operational contexts. For example, pBCI can be used to provide translation of unknown read words (Hyrskykari, 2006) or to display information on the screen when the user needs it (Jacob, 1990). passive Brain Computer Interfaces also have applications in the field of virtual reality and video gaming (Lécuyer et al., 2008; George and Lécuyer, 2010).
Auditory attention detection algorithms could be coupled with passive BCI to extend the usefulness of such methods to more concrete applications. In the next section, we will describe some possible future applications for AAD-pBCI systems.
Since a few years, studies that explore the relationship between children's attention abilities and screen access have shown that precocious screen access may go along with attentional problems (Christakis et al., 2004; Ponti et al., 2017; Tamana et al., 2019, but see Kostyrka-Allchorne et al., 2017 for a systematic review on the relationship between television exposure and children's cognition). AAD-pBCI systems could be used to improve children's attention ability. Such an attempt was made by Cho et al. (2002) who developed an attention enhancement system for ADHD children using EEG biofeedback and a virtual classroom environment. They showed that it is possible to use pBCI to enhance attention in children with ADHD in a school context. An advantage of real-time AAD applications is that they may allow monitoring children's attention. Moreover, they could be of use in serious game applications aiming at enhancing sustained auditory attention (see for instance Figure 1). Importantly, one can hypothesize that, because sustained attention in a complex auditory scene requires segregation and integration abilities but also inhibition and WM, these functions may also benefit from such applications.
Figure 1. Schematic representation of a sustained attention enhancement AAD-pBCI system based on a serious game. While the user is concentrating on a specific auditory source among several others, brain activity is recorded, and preprocessed in real time. Based on this recorded EEG data, the attended auditory source is continuously tracked by the AAD device. The pBCI device collects the AAD performances continuously (i.e., regression accuracy), estimates if a certain threshold has been exceeded, adapts, in real-time, the game parameters (e.g., instructions, auditory scene complexity), and gives feedback to the user.
Such a tool could also benefit musicians who must be able to sustain attention for long periods of time (Bergman Nutley et al., 2014). Interestingly, for musicians, this approach could also enhance the ability to share auditory attention across multiple sources, since this is of great importance in ensemble music making. As for the Sustained Attention Enhancement AAD-pBCI System mentioned above, a Divided Attention Enhancement AAD-pBCI System could also take the form of a musical serious game wherein the player has to learn to switch the focus of attention from one source to another and to share attention across multiple sources.
In the field of art, several attempts have been made to bridge EEG and BCI since the 1970s (Vidal, 1973; Rosenboom, 1977; Williams and Miranda, 2018). More recently, works have been done to develop systems to control an instrument (Arslan et al., 2006) or to generate melodies with brain signals (Wu et al., 2010; Miranda et al., 2011) to name a few. In this sense, there is a place for AAD-pBCI systems to create new kinds of art performances in which brain activity induced by auditory attention could be used to modulate different sound sources (see Figure 2). This could be of particular interest in an immersive listening structure composed of multiple loudspeakers (Pascal, 2020). Such a device would allow the user to select a specific sound source and modify its loudness, spatial location, or motion. In such a setup, the AAD module monitors in real-time the attended source and provides information about the source of interest to the pBCI module. This second module is responsible for analyzing the intentions of the user, translating them into command, and controlling an external device. To do so, the pBCI module classifies among several classes of neural activity induced by different cognitive processes (e.g., imaging a movement of the attended source). Once the user's intention has been detected, the pBCI module translates it into commands that correspond to a particular parameter's modification (e.g., moving the attended source from the upper central loudspeaker to the bottom central one) and sends them to an external device.
Figure 2. Schematic representation of a real-time sound modulation AAD-pBCI system. Based on the real-time EEG data recording, the attended auditory source is continuously tracked by the AAD device. The pBCI device analyses in real-time the user's intentions (e.g., moving the attended source from the upper left loudspeaker to the bottom left one), translates it into commands and sends it to an external device that will modify the loudspeaker's parameters accordingly.
The first reason why AAD has been investigated is to enhance hearing aids and more specifically, CI. Cochlear implant are electronic devices that allow deaf people to partly regain audition by converting audio signals to electrical signals directly stimulating the auditory nerve. While they perform well when the user is facing a unique speaker (or in quiet environment), in presence of multiple speakers performance drops dramatically because all speakers are amplified indistinctly (e.g., Zeng et al., 2008).
The solution to bypass this limitation is to inform hearing aids of the user's attentional focus. In fact, if the hearing aid was able to “know” which audio source the user is attending to, then it should be able to selectively enhance it. Therefore, combining AAD algorithms and hearing aids technologies, should lead to next-generation hearing aids allowing good performances in complex (or noisy) auditory environments (see for example: Das et al., 2016, 2020; Van Eyndhoven et al., 2017; Cantisani et al., 2020; Geirnaert et al., 2020).
One can think about other futuristic applications for AAD, in several distinct domains. For instance, in the entertainment field. It is, for example, possible to develop “auditory games” in which players, equipped with light AAD-pBCI systems, confront each other in musical battles using their auditory attention. In addition to being fun, this kind of game could be interesting to develop cognitive abilities that underlie auditory sustained attention (WM, executive control, etc.) even if it is not its main purpose. Furthermore, such a game could be adapted to a solo or a multiplayer environment.
AAD-pBCI systems could also find applications in the field of domotics. Indeed, a wearable AAD-pBCI system could be useful, in situations where ambient noise is varying constantly (e.g., in a living room), to monitor and adapt in real-time the loudness of the attended sound source (TV, hifi system, home phone, etc.).
Overall, AAD, by providing real-time cues of the auditory attentional state of an individual, opens new avenues to several applications. After a first stage of fundamental research to understand the links between auditory attention and neural signals, we are now in a second stage of applied research optimizing algorithms in terms of both classification performance and speed. In the next few years, when real-time decoding limitations will be overcome and wearable wireless systems will be developed, AAD could find applications in many domains such as education, art, health, or even domotics and online games.
JB and DS conceived the present article. JB wrote the manuscript and designed the figures with the support of DS and MC. All authors contributed to the article and approved the submitted version.
JB is funded by Oticon Medical (research agreement n°14294-CRI04) and Region Provence-Alpes-Côte d'Azur. DS is supported by APA foundation (RD-2016-9), ANR-16-CE28-0012-01 (RALP), ANR-CONV-0002 (ILCB), ANR-11-LABX-0036 (BLRI), and the Excellence Initiative of Aix-Marseille University (A*MIDEX).
The authors declare that the research was conducted in the absence of any commercial or financial relationships that could be construed as a potential conflict of interest.
Akram, S., Presaccoc, A., Simon, J. Z., Shamma, S. A., and Babadi, B. (2016). Robust decoding of selective auditory attention from MEG in a competing-speaker environment via state-space modeling. Neuroimage 124, 906–917. doi: 10.1016/j.neuroimage.2015.09.048
Alickovic, E., Lunner, T., Gustafsson, F., and Ljung, L. (2019). A tutorial on auditory attention identification methods. Front. Neurosci. 13:153. doi: 10.3389/fnins.2019.00153
Aricò, P., Borghini, G., Di Flumeri, G., Colosimo, A., Bonelli, S., Golfetti, A., et al. (2016). Adaptive automation triggered by EEG-based mental workload index: a passive brain-computer interface application in realistic air traffic control environment. Front. Hum. Neurosci. 10:539. doi: 10.3389/fnhum.2016.00539
Arslan, B., Brause, A., Castet, J., Léhembre, R., Simon, C., Filatriau, J. J., et al. (2006). “A real time music synthesis environment driven with biological signals,” in ICASSP, IEEE International Conference on Acoustics, Speech and Signal Processing - Proceedings, Vol. 2 (Toulouse), 1172–1175. doi: 10.1109/icassp.2006.1660557
Bergman Nutley, S., Darki, F., and Klingberg, T. (2014). Music practice is associated with development of working memory during childhood and adolescence. Front. Hum. Neurosci. 7:926. doi: 10.3389/fnhum.2013.00926
Bleichner, M. G., Mirkovic, B., and Debener, S. (2016). Identifying auditory attention with ear-EEG: CEEGrid versus high-density cap-EEG comparison. J. Neural Eng. 13:066004. doi: 10.1088/1741-2560/13/6/066004
Cantisani, G., Essid, S., and Richard, G. (2019). “EEG-based decoding of auditory attention to a target instrument in polyphonic music,” in IEEE Workshop on Applications of Signal Processing to Audio and Acoustics (New Paltz, NY), 80–84. doi: 10.1109/WASPAA.2019.8937219
Cantisani, G., Essid, S., Richard, G., Cantisani, G., Essid, S., Richard, G., et al. (2020). Neuro-steered music source separation with EEG-based auditory attention decoding and contrastive-NMF. Hal Archives-Ouvertes.
Cho, B. H., Lee, J. M., Ku, J. H., Jang, D. P., Kim, J. S., Kim, I. Y., et al. (2002). “Attention enhancement system using virtual reality and EEG biofeedback,” in Proceedings - Virtual Reality Annual International Symposium (Seattle, WA), 156–163. doi: 10.1109/vr.2002.996518
Christakis, D. A., Zimmerman, F. J., DiGiuseppe, D. L., and McCarty, C. A. (2004). Early television exposure and subsequent attentional problems in children. Pediatrics 113, 708–713. doi: 10.1542/peds.113.4.708
Ciccarelli, G., Nolan, M., Perricone, J., Calamia, P. T., Haro, S., O'Sullivan, J., et al. (2019). Comparison of two-talker attention decoding from EEG with nonlinear neural networks and linear methods. Sci. Rep. 9, 1–10. doi: 10.1038/s41598-019-47795-0
Clerc, M., Bougrain, L., and Lotte, F. (2016). Brain–Computer Interfaces 1: Foundations and Methods. New York, NY: Wiley.
Crosse, M. J., Di Liberto, G. M., Bednar, A., and Lalor, E. C. (2016). The multivariate temporal response function (mTRF) toolbox: a MATLAB toolbox for relating neural signals to continuous stimuli. Front. Hum. Neurosci. 10:604. doi: 10.3389/fnhum.2016.00604
Das, N., Bertrand, A., and Francart, T. (2018). EEG-based auditory attention detection: boundary conditions for background noise and speaker positions. BioRxiv 32, 1–18. doi: 10.1101/312827
Das, N., Van Eyndhoven, S., Francart, T., and Bertrand, A. (2016). “Adaptive attention-driven speech enhancement for EEG-informed hearing prostheses,” Proceedings of the Annual International Conference of the IEEE Engineering in Medicine and Biology Society (EMBS) (IEEE), 77–80. doi: 10.1109/EMBC.2016.7590644
Das, N., Zegers, J., Van Hamme, H., Francart, T., and Bertrand, A. (2020). Linear versus deep learning methods for noisy speech separation for EEG-informed attention decoding. J. Neural Eng. 17:046039. doi: 10.1088/1741-2552/aba6f8
de Cheveigné, A., Wong, D. E., Di Liberto, G. M., Hjortkjær, J., Slaney, M., and Lalor, E. (2018). Decoding the auditory brain with canonical component analysis. NeuroImage 172, 206–216. doi: 10.1016/j.neuroimage.2018.01.033
de Taillez, T., Kollmeier, B., and Meyer, B. T. (2017). Machine learning for decoding listeners' attention from EEG evoked by continuous speech. Eur. J. Neurosci. 51, 1234–1241. doi: 10.1111/ijlh.12426
Ding, N., and Simon, J. Z. (2012). Emergence of neural encoding of auditory objects while listening to competing speakers. Proc. Natl. Acad. Sci. U.S.A. 109, 11854–11859. doi: 10.1073/pnas.1205381109
Fiedler, L., Wöstmann, M., Graversen, C., Brandmeyer, A., Lunner, T., and Obleser, J. (2017). Single-channel in-ear-EEG detects the focus of auditory attention to concurrent tone streams and mixed speech. J. Neural Eng. 14:036020. doi: 10.1088/1741-2552/aa66dd
Fuglsang, S. A., Dau, T., and Hjortkjær, J. (2017). Noise-robust cortical tracking of attended speech in real-world acoustic scenes. NeuroImage 156, 435–444. doi: 10.1016/j.neuroimage.2017.04.026
Geirnaert, S., Vandecappelle, S., Alickovic, E., de Cheveigné, A., Lalor, E., Meyer, B. T., et al. (2020). Neuro-steered hearing devices: decoding auditory attention from the brain. ArXiv 802895, 1–20. Available online at: http://arxiv.org/abs/2008.04569
George, L., and Lécuyer, A. (2010). “An overview of research on “passive” brain-computer interfaces for implicit human-computer interaction,” in International Conference on Applied Bionics and Biomechanics ICABB 2010 - Workshop W1 “Brain-Computer Interfacing and Virtual Reality” (Venise). Available online at: http://hal.inria.fr/docs/00/53/72/11/PDF/GeorgeL-LecuyerA.pdf
Giraud, A. L., and Poeppel, D. (2012). Cortical oscillations and speech processing: emerging computational principles and operations. Nat. Neurosci. 15, 511–517. doi: 10.1038/nn.3063
Haufe, S., Kim, J. W., Kim, I. H., Sonnleitner, A., Schrauf, M., Curio, G., et al. (2014). Electrophysiology-based detection of emergency braking intention in real-world driving. J. Neural Eng. 11:056011. doi: 10.1088/1741-2560/11/5/056011
Holdgraf, C. R., Rieger, J. W., Micheli, C., Martin, S., Knight, R. T., and Theunissen, F. E. (2017). Encoding and decoding models in cognitive electrophysiology. Front. Syst. Neurosci. 11:61. doi: 10.3389/fnsys.2017.00061
Hyrskykari, A. (2006). Eyes in Attentive Interfaces: Experiences from Creating iDict, A Gaze-Aware Reading Aid. (Issue January 2006). Available online at: http://acta.uta.fi
Jacob, R. J. K. (1990). “What you look at is what you get: eye movement-based interaction techniques,” in Proceedings of the SIGCHI Conference on Human Factors in Computing Systems (Montréal, QC), 11–18.
Kostyrka-Allchorne, K., Cooper, N. R., and Simpson, A. (2017). The relationship between television exposure and children's cognition and behaviour: a systematic review. Dev. Rev. 44, 19–58. doi: 10.1016/j.dr.2016.12.002
Kubanek, J., Brunner, P., Gunduz, A., Poeppel, D., and Schalk, G. (2013). The tracking of speech envelope in the human cortex. PLoS ONE 8:e53398. doi: 10.1371/journal.pone.0053398
Lécuyer, A., Lotte, F., Reilly, R. B., and College, T. (2008). Brain-computer interfaces, virtual reality, and videogames. Computer 41, 66–72. doi: 10.1109/MC.2008.410
Mesgarani, N., and Chang, E. F. (2012). Selective cortical representation of attended speaker in multi-talker speech perception. Nature 485, 233–236. doi: 10.1038/nature11020
Mesgarani, N., David, S. V., Fritz, J. B., and Shamma, S. A. (2009). Influence of context and behavior on stimulus reconstruction from neural activity in primary auditory cortex. J. Neurophysiol. 102, 3329–3339. doi: 10.1152/jn.91128.2008
Miran, S., Akram, S., Sheikhattar, A., Simon, J. Z., Zhang, T., and Babadi, B. (2018a). Real-time tracking of selective auditory attention from M/EEG: a Bayesian filtering approach. Front. Neurosci. 12:262. doi: 10.3389/fnins.2018.00262
Miran, S., Akram, S., Sheikhattar, A., Simon, J. Z., Zhang, T., and Babadi, B. (2018b). “Real-time decoding of auditory attention from EEG via Bayesian filtering,” in Proceedings of the Annual International Conference of the IEEE Engineering in Medicine and Biology Society, EMBS (New Orleans, LA), 25–28. doi: 10.1109/EMBC.2018.8512210
Miranda, E. R., Magee, W. L., Wilson, J. J., Eaton, J., and Palaniappan, R. (2011). Brain-Computer Music Interfacing (BCMI): from basic research to the real world of special needs. Music Med. 3, 134–140. doi: 10.1177/1943862111399290
Mirkovic, B., Debener, S., Jaeger, M., and De Vos, M. (2015). Decoding the attended speech stream with multi-channel EEG: implications for online, daily-life applications. J. Neural Eng. 12:046007. doi: 10.1088/1741-2560/12/4/046007
Nourski, K. V., Reale, R. A., Oya, H., Kawasaki, H., Kovach, C. K., Chen, H., et al. (2009). Temporal envelope of time-compressed speech represented in the human auditory cortex. J. Neurosci. 29, 15564–15574. doi: 10.1523/JNEUROSCI.3065-09.2009
O'Sullivan, J., Chen, Z., Sheth, S. A., McKhann, G., Mehta, A. D., and Mesgarani, N. (2017). “Neural decoding of attentional selection in multi-speaker environments without access to separated sources,” in Proceedings of the Annual International Conference of the IEEE Engineering in Medicine and Biology Society, EMBS (Jeju), 1644–1647. doi: 10.1109/EMBC.2017.8037155
O'Sullivan, J. A., Power, A. J., Mesgarani, N., Rajaram, S., Foxe, J. J., Shinn-Cunningham, B. G., et al. (2015). Attentional selection in a cocktail party environment can be decoded from single-trial EEG. Cereb. Cortex 25, 1697–1706. doi: 10.1093/cercor/bht355
Pascal, M. (2020). Analyse de la composition de l'espace dans une œuvre acousmatique immersive de Jean Marc Duchenne. Hal Archives-Ouvertes. 2020:hal-02926984.
Pasley, B. N., David, S. V., Mesgarani, N., Flinker, A., Shamma, S. A., Crone, N. E., et al. (2012). Reconstructing speech from human auditory cortex. PLoS Biol. 10:e1001251. doi: 10.1371/journal.pbio.1001251
Ponti, M., Bélanger, S., Grimes, R., Heard, J., Johnson, M., Moreau, E., et al. (2017). Screen time and young children: Promoting health and development in a digital world. Paediatr. Child Health. 22, 461–477. doi: 10.1093/pch/pxx123
Rosenboom, D. (1977). Biofeedback and the arts: results of early experiments. J. Aesthet. Art Critic. 35, 385–386.
Schäfer, P. J., Corona-Strauss, F. I., Hannemann, R., Hillyard, S. A., and Strauss, D. J. (2018). Testing the limits of the stimulus reconstruction approach: auditory attention decoding in a four-speaker free field environment. Trends Hear. 22, 1–12. doi: 10.1177/2331216518816600
Tamana, S. K., Victor, E., Joyce, C., Diana, L. L., Meghan, B. A., et al. (2019). Screen-time is associated with inattention problems in preschoolers: results from the CHILD birth cohort study. PLoS ONE 14:e0213995. doi: 10.1371/journal.pone.0213995
Van Eyndhoven, S., Francart, T., and Bertrand, A. (2017). EEG-informed attended speaker extraction from recorded speech mixtures with application in neuro-steered hearing prostheses. IEEE Trans. Biomed. Eng. 64, 1045–1056. doi: 10.1109/TBME.2016.2587382
Vandecapelle, S., Deckers, L., Das, N., Ansari, A. H., Bertrand, A., and Francart, T. (2020). EEG-based detection of the attended speaker and the locus of auditory attention with convolutional neural networks. BioRxiv [Preprint]. doi: 10.1101/475673
Vidal, J. J. (1973). Toward direct brain-computer communication. Annu. Rev. Biophys. Bioeng. 2, 157–180. doi: 10.1146/annurev.bb.02.060173.001105
Williams, D., and Miranda, E. R. (2018). “BCI for music making: then, now, and next,” in Brain–Computer Interfaces Handbook: Technological and Theoretical Advances, eds C. S. Nam, A. Nijholt, and F.Lotte (Florence, KY: CRC Press), 191–205.
Wong, D. D. E., Fuglsang, S. A., Hjortkjær, J., Ceolini, E., Slaney, M., and de Cheveigné, A. (2018). A comparison of regularization methods in forward and backward models for auditory attention decoding. Front. Neurosci. 12:531. doi: 10.3389/fnins.2018.00531
Wu, D., Li, C., Yin, Y., Zhou, C., and Yao, D. (2010). Music composition from the brain signal: Representing the mental state by music. Comput. Intell. Neurosci. 2010:267671. doi: 10.1155/2010/267671
Zander, T. O., and Kothe, C. (2011). Towards passive brain-computer interfaces: applying brain-computer interface technology to human-machine systems in general. J. Neural Eng. 8:025005. doi: 10.1088/1741-2560/8/2/025005
Zeng, F. G., Rebscher, S., Harrison, W., Sun, X., and Feng, H. (2008). Cochlear implants: system design, integration, and evaluation. IEEE Rev. Biomed. Eng. 1, 115–142. doi: 10.1109/RBME.2008.2008250
Keywords: AAD, EEG, auditory attention, passive BCI, education, art
Citation: Belo J, Clerc M and Schön D (2021) EEG-Based Auditory Attention Detection and Its Possible Future Applications for Passive BCI. Front. Comput. Sci. 3:661178. doi: 10.3389/fcomp.2021.661178
Received: 30 January 2021; Accepted: 06 April 2021;
Published: 30 April 2021.
Edited by:
Camille Jeunet, UMR5287 Institut de Neurosciences Cognitives et Intégratives d'Aquitaine (INCIA), FranceReviewed by:
Lucia Pepa, Marche Polytechnic University, ItalyCopyright © 2021 Belo, Clerc and Schön. This is an open-access article distributed under the terms of the Creative Commons Attribution License (CC BY). The use, distribution or reproduction in other forums is permitted, provided the original author(s) and the copyright owner(s) are credited and that the original publication in this journal is cited, in accordance with accepted academic practice. No use, distribution or reproduction is permitted which does not comply with these terms.
*Correspondence: Joan Belo, am9hbi5iZWxvQGlucmlhLmZy
Disclaimer: All claims expressed in this article are solely those of the authors and do not necessarily represent those of their affiliated organizations, or those of the publisher, the editors and the reviewers. Any product that may be evaluated in this article or claim that may be made by its manufacturer is not guaranteed or endorsed by the publisher.
Research integrity at Frontiers
Learn more about the work of our research integrity team to safeguard the quality of each article we publish.