- 1US Army Corps of Engineers, Engineer Research and Development Center, Environmental Laboratory, Concord, MA, United States
- 2Department of Engineering Systems and Environment, University of Virginia, Charlottesville, VA, United States
- 3Max Planck Institute for Human Development, Berlin, Germany
- 4Elsevier, New York, NY, United States
- 5Department of Engineering and Public Policy, Carnegie Mellon University, Pittsburgh, PA, United States
Applications of Artificial Intelligence (AI) can be examined from perspectives of different disciplines and research areas ranging from computer science and security, engineering, policymaking, and sociology. The technical scholarship of emerging technologies usually precedes the discussion of their societal implications but can benefit from social science insight in scientific development. Therefore, there is an urgent need for scientists and engineers developing AI algorithms and applications to actively engage with scholars in the social sciences. Without collaborative engagement, developers may encounter resistance to the approval and adoption of their technological advancements. This paper reviews a dataset, collected by Elsevier from the Scopus database, of papers on AI application published between 1997 and 2018, and examines how the co-development of technical and social science communities has grown throughout AI's earliest to latest stages of development. Thus far, more AI research exists that combines social science and technical explorations than AI scholarship of social sciences alone, and both categories are dwarfed by technical research. Moreover, we identify a relative absence of AI research related to its societal implications such as governance, ethics, or moral implications of the technology. The future of AI scholarship will benefit from both technical and social science examinations of the discipline's risk assessment, governance, and public engagement needs, to foster advances in AI that are sustainable, risk-informed, and societally beneficial.
Introduction
Advances in artificial intelligence (AI) have expanded its adoption in computer security of defense and financial systems, economics, education, and many other fields (Wachter et al., 2017; Winfield et al., 2018; Linkov et al., 2020). Emerging technologies like AI will eventually contend with regulatory pressure and public attention that may either hinder emerging technologies or stimulate their development. Academic discourse on the implications of AI, particularly outside the purely technical domains such as its societal risks and trust, is still coalescing. Social science inquiries into AI technology contribute to examining its potential to behave in ways that are harmful. Harms can arise from AI applications that are incorrect, illegal, or inappropriate given an unforeseen context, or that produce results that accelerate negative trends or consequences (Calvert and Martin, 2009), Linkov et al., 2019). For example, potential threats from AI (intentional or not) arise in computer security applications such as classifying cyber attackers as legitimate users or vice-versa, monitoring and predicting activity of individuals in social networks for commercial or surveillance purposes (e.g., social credit systems), with possible implications on privacy and civil rights. Understanding these trends in greater detail can help shape the technological growth, science policy, and public discussion surrounding AI. Artificial intelligence developers must innovate while identifying and mitigating real and perceived risks which may threaten innovation with premature or prohibitive regulation.
The goals of this paper are two-fold. First and primarily, we examine AI scholarship in the last two decades to determine whether research has been dominated by technical development or is accompanied by a discussion of social implications of the technology. We define technical research as expanding the capabilities of AI approaches, methods, and algorithms. The technical domain includes publications where researchers are concerned with developing, testing, and deploying new methods and algorithms. Supervised learning, unsupervised learning, semi-supervised learning, and reinforcement learning are examples of AI topics represented in the technical domain. In contrast, social sciences studies of AI examine the relationship between society and the implications, outcomes, and social responses of AI innovation and commercialization. Topics in the social science domain include economics, ethical, legal and social implications, finance and economics, regulations and governance, and risks and decision making associated with AI applications.
The confluence of technical and social sciences research has been examined in other emerging disciplines such as nanotechnology and synthetic biology (Shapira et al., 2015; Trump et al., 2018a). In the earliest years of a technology's development, scholarly publications reflect the advancements in the technical domain that defines the field. Social science discourse typically emerges later as commentators identify opportunities and concerns that the given technology may present (Bijker et al., 2012). This lag offers a time during a technology's earliest development stages, during which it is able to develop in relative isolation from social commentary. As a technology becomes well-known and demonstrably viable, social scientists learn about and react to the new technology to provide necessary evaluations before the technology fully matures and approaches the marketplace.
However, such a relative delay in the development of social science and the technical domains did not evolve in identical ways for different technologies. Synthetic biology is characterized by social science discourse that emerged in relative step with advancements in technical research (Calvert and Martin, 2009; Torgersen, 2009; Trump et al., 2018b). For synthetic biology, the lag time is 3 or 4 years at most (Trump et al., 2019), indicating that social discourse is being applied to emerging developments in synthetic biology relatively quickly, and before enabling technologies such as gene editing are fully explored. In contrast, for nanotechnology research into technical capabilities emerged first on fullerenes (starting in 1985) or carbon nanotubes (starting in 1991), and was only followed by social science discourse of implications on the technology in the mid-to-late 2000s (Mnyusiwalla et al., 2003; Macnaghten et al., 2005; Shapira et al., 2015; Trump et al., 2018a).
For AI methods and algorithms, social scientists only recently have engaged substantially in discussion related to the field's implications and applications (Elsevier, 2018). For example, in computer security, researchers and developers have long focused in efficient machine learning algorithms to accurately detect cyber-attack or fraud, and only recently discussed issues related to privacy and bias of such AI algorithms (Linkov et al., 2020). Nevertheless, such AI literature in the social science domain has recently appeared (Selbst et al., 2017; Edwards and Veale, 2018), with scholars discussing AI's privacy and security, ethical, legal, economic, and other societal implications and applications (Ananny, 2016; Cath, 2018; Nemitz, 2018). The development of AI's methods and algorithms (technical domain) along with social science inquiries about expected impacts (referred to hereon as “social sciences domain”) may potentially shape the future growth, commodification, and social acceptance of AI technology and its products.
The second goal of this paper is to identify important gaps in the interaction between the technical and social science domains in AI research, and to discuss the implications of these gaps. It is necessary to examine the social science discussion within this field as well as how emerging breakthroughs are framed as beneficial or concerning to policymakers and the general public. This is of particular urgency given the ubiquity with which AI is deployed in the public sector and within personal devices.
Our past work shows the utility of assessing the co-development of a field's technical/physical domain and social sciences domain by reviewing trends in its published literature in nanotechnology and synthetic biology (Trump et al., 2018a, 2019). This paper employs a similar approach to AI scholarship. We quantify the temporal patterns of a bibliographical AI-related dataset retrieved from Elsevier's Scopus database. The approach described in Elsevier (2018) and Siebert et al. (2018) was applied to Scopus to obtain a comprehensive set of AI publications, and then the method described in this paper was employed to classify over 550,000 publications within the technical and social science domains. Our results provide insight on trends of scholarly inquiry between 1997 and 2018.
Methods
Database
An AI-related dataset was retrieved from Elsevier's Scopus database of publications' titles, abstracts, keywords, and other metadata. The AI dataset was obtained using a two-step curation approach employing machine learning and expert assessment as follows. First, a list of keywords was created that is representative of scholarship in the AI discipline. Second, the keyword list was used to query publications from the Scopus database. This curation process is further described in Elsevier (2018), while the technical details are provided in Siebert et al. (2018). The query result is a dataset of about 553,000 items. Each item is a triple where the first value is a unique article identifier, the second is the article's publication year, and the third value is a keyword listed in the article's metadata.
Classification of Articles Into Technical and Social Science Domains
After the dataset described in section Database was obtained, we drafted a list of AI-related keywords to classify the dataset of AI research into the social sciences and technical domains. Our approach assumes that the keywords provided by the authors of an article approximate the research topics advanced by that paper. This approach has been used in other bibliometric databases such as the Microsoft Academic Graph (MAG), which uses keywords to classify research papers into topics and fields (Sinha et al., 2015). For example, Effendy and Yap (2017) used MAG to group papers into general fields of AI and Computer Science based on their keywords. Moreover, the use of keywords as an approximation for topics is also common in industry. An example is Google Trends, which uses frequency of keyword use in web search to estimate trends in the popularity of topics (Google, 2021). Our set of social and technical keywords were separated into two lists of 36 and 38 keywords, respectively, amounting to 74 unique keywords in total. The keywords are listed in Tables 1, 2.
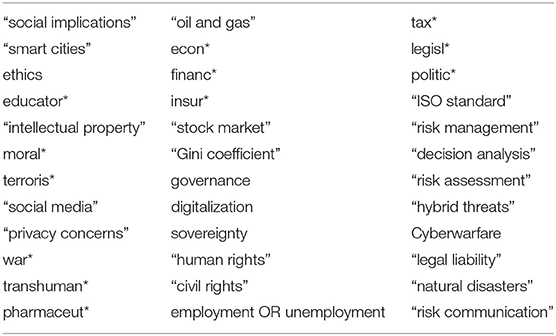
Table 1. Keywords in the Social Domain (quotes indicate keywords formed by multiples words. The asterisk denotes wildcard searches.).
The social keyword list was generated using reviews and perspectives from subject matter experts. The technical keyword list was generated after inspecting the contents, introductions, and, whenever necessary for clarification, textual details of textbooks of AI authorities in the technical field. We grouped the retrieved journal articles, textbooks, and other sources according to domain. The sources of the keywords include:
• Social sciences domain (Horvitz, 2017; Miller, 2019; Montes and Goertzel, 2019; Rahwan et al., 2019).
• Technical domain (Bishop, 2006; Haykin, 2010; Murphy, 2012; Goodfellow et al., 2016; Sutton and Barto, 2018).
For some of the keywords used in the queries, discriminators such as wild cards (*) were employed so as to direct the outcome of the search (Elsevier, 2016). The time span of our queries was 22 years (1997–2018).
Next, we used the keyword lists to associate the dataset of over 550,000 items (i.e., publication-year-keyword) as semantically belonging to the technical and/or social AI domains (Mingers and Leydesdorff, 2015). The two lists enabled us to generate a representative sample of publications corresponding to the two domains, as taken from the curated Scopus dataset.
This approach has been applied in previous work to examine trends and the interaction between the social sciences and technical domains in other fields. In Trump et al. (2019), a set of keywords were used to search the Web of Science database and to classify articles on synthetic biology into the physical/technical and social sciences domains, which enabled a discussion of the evolution of that field. A similar approach was used to discuss the co-evolution of physical/technical and social sciences research in nanotechnology (Trump et al., 2018a).
Trends, Domain Overlaps, and Gaps
Changes in the volume of publications in the two domains over the time of interest are visualized in a time series plot of the number of articles containing keywords in each domain (social sciences and technical) (Figure 1 in the Results section). Any overlap between the domains was also identified and quantified. Overlap is defined as the number of articles that contain keywords from both the social sciences and technical domains, but each article is also individually counted within the social science and technical tallies.
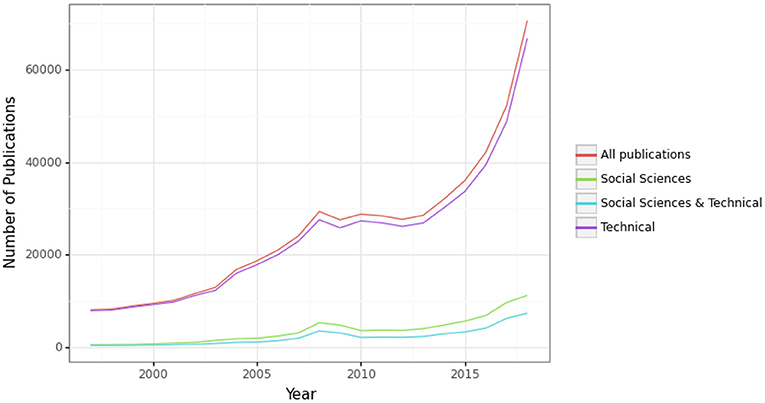
Figure 1. Number of publications per year: all publications, papers with technical keywords only, social sciences only, and papers that have both social and technical keywords.
Terminology Consensus
We also examined the temporal terminology consensus, i.e., how many of the keywords used in 1 year are still in use in subsequent years. We do this by examining the similarity of keywords appearing in every pair of years in the period 1997–2018. To examine the degree of all the keywords' appearance per calendar year as an indicator for terminology consensus, we introduce the Jaccard similarity index (Skiena, 2017), defined as follows:
where A and B are two sets of interest. In our case, A and B are the sets of keywords for any pair of years within the dataset. The Jaccard index varies between 0 (no overlap) and 1 (complete overlap of all keywords in a pair of years), and is defined for at least one of the pair sets A and B as being non-empty. Changes in terminology consensus were identified by visual inspection of the Jaccard similarity matrix, displayed as a heatmap (Figure 7) in the results.
Results
The red curve of Figure 1 shows the number of AI articles in the dataset published per year. The curve shows superlinear growth in the yearly number of articles published during the majority of the 1997–2018 period, except from 2008 to 2013 when AI articles were published at a roughly constant rate. However, since 2013 the total per year has experienced the sharpest growth in the period of analysis.
Trends in the Social Sciences and Technical Domains
The ratio of total number of papers in the technical domain to the number of papers in the social domain is roughly one order of magnitude over the last two decades. Figure 1 shows a time series of AI publications for the technical (green) and social sciences (purple) domains, as well as the articles that contain keywords in both domains (blue). The publication growth rate of the social science literature parallels that of the technical science domain, suggesting a relatively synchronized engagement of the social science community with developments of algorithms and techniques in the technical domain.
Figure 2 shows the ratio of number of articles with social sciences keywords to number of articles with technical keywords (blue curve) and indicates that the number of social science publications is roughly one order of magnitude lower than that in the technical and social domains. Additionally, the majority of social science papers were papers that also mentioned technical keywords. The number of papers examining only social science is the number of papers in “Social Science” minus the number of papers “Social Science and Technical” of Figure 1. Nevertheless, this is a significant social science penetration as compared to other emerging technologies analyzed in the past (Trump et al., 2018a, 2019). It is comparable to the ratio observed in the synthetic biology literature, where concerns related to genetic engineering generated immediate attention to synthetic biology development (Trump et al., 2019). In contrast, nanotechnology shows a ratio of over two orders of magnitude at the onset or the study, with a significant improvement in that gap over the 20 years of that study.
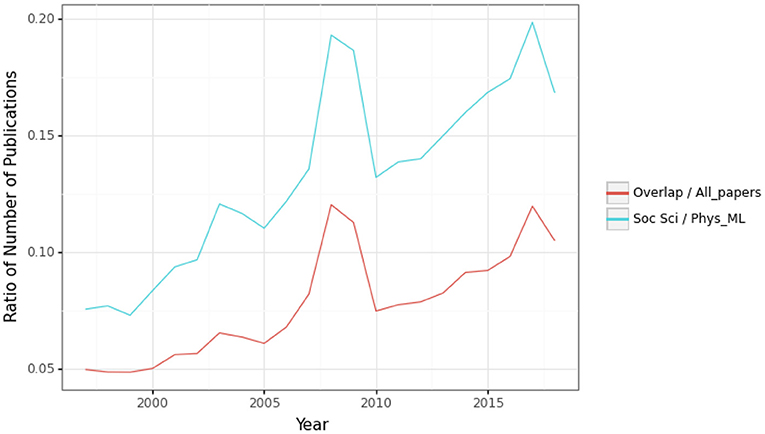
Figure 2. Ratio of number of articles with social sciences keywords to number of articles with technical keywords (blue), and ratio of number of articles with both social sciences, and technical keywords to total number of articles in the dataset (red), per year.
Moreover, the gap in AI research between the two domains has been narrowing over the years, mostly due to a sharper growth in papers that examine social science than the growth in papers in the technical domain. While the ratio of AI articles in social sciences to articles in the technical domain was 0.076 in 1997, the ratio more than doubled in two decades and reached 0.17 in 2018. This suggests that research on the societal implications of AI has been gaining traction as AI methods and algorithms advance.
Interestingly, the red curve of Figure 2 shows that there is a small fraction of articles that include keywords in both the social sciences and technical domains, but that share has been increasing. The ratio of articles with keywords in both domains to that of total number of articles doubles from 0.05 in 1997 to 0.1 in 2018. This finding implies a growing level of interdisciplinary collaboration between researchers in the technical and social sciences domains, and that more recently the majority of AI articles in social sciences are interdisciplinary.
Frequency of Topics in the Technical Domain
Figure 3 shows the number of publications using specific technical domain keywords in 2010 and 2018, indicating that the frequency of all keywords increases in that period. The keyword “networks” is the most frequently used in the technical domain in 2010 and 2018. That keyword alone appears in 66% of all articles in that year, probably because of the longtime interest and evolution of several types of neural networks. Networks is followed by deep learning and feature extraction, the former of which barely appears in 2010 but is the second-most frequently referenced keyword in 2018, indicating new interest in recent years.
Figure 4 shows the nine most frequently-used AI keywords in the technical domain between 1997 and 2018. It shows networks as a dominant topic in all years, although the popularity of deep learning has grown in recent years. Overall, usage of most AI keywords has grown steadily over time, as expected given the general growth in AI scholarship.
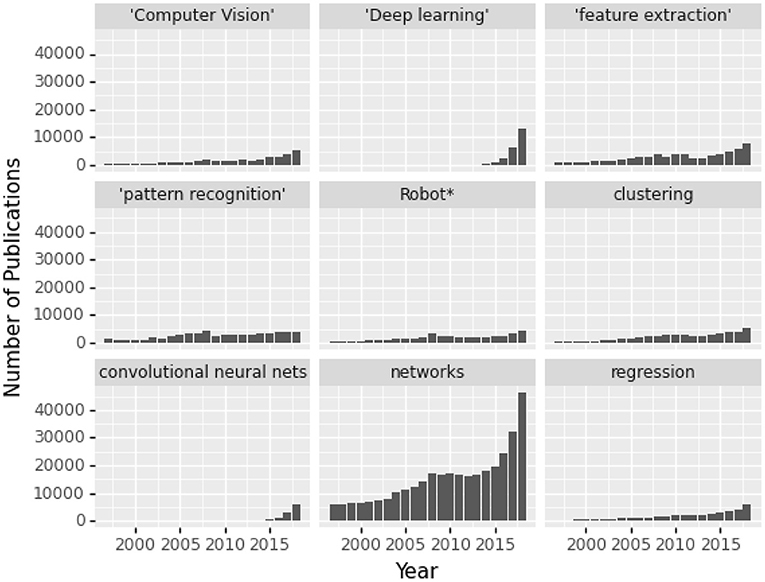
Figure 4. Number of articles for the most frequent keywords in the technical domain, per year (1997–2018).
Frequency of Topics in the Social Sciences Domain
In Figure 5, the keywords most frequent in the social sciences domain both in 2010 and in 2018 are related to economics and education. This may suggest that those fields pioneered a relatively larger body of research on societal implications of AI. It might also suggest that economics and education pioneered research of AI methods applied to those disciplines, which may be a relevant effect given the number of papers in social sciences. Other frequent keywords include social media, which had comparatively few mentions in 2010, and warfare-related topics.
Figure 6 shows the nine most frequently-used keywords in the social sciences domain between 1997 and 2018. It shows economics and education are dominant topics in all years, although the popularity of smart cities and social media have grown between 1997 and 2018. In addition, research using AI related to these keywords has grown steadily over time.
Temporal Trends in Keyword Similarity
The Jaccard similarity matrix displayed in Figure 7 indicates that years close to one another (close to the diagonal) are more likely to use the same terminology, while the greatest dissimilarity in terms used in the abstract-title-keyword format of Scopus (~50%) seems to occur for years far from one another (e.g., 1997 and 2018). This might suggest a shift in the terminology (for instance, NLP—natural language processing—encompassing after 2010 more keywords than it did in the 1990s) or the emergence of keywords not previously used in the AI context (Deep Learning is such an example, which appears in the dataset after 2010).
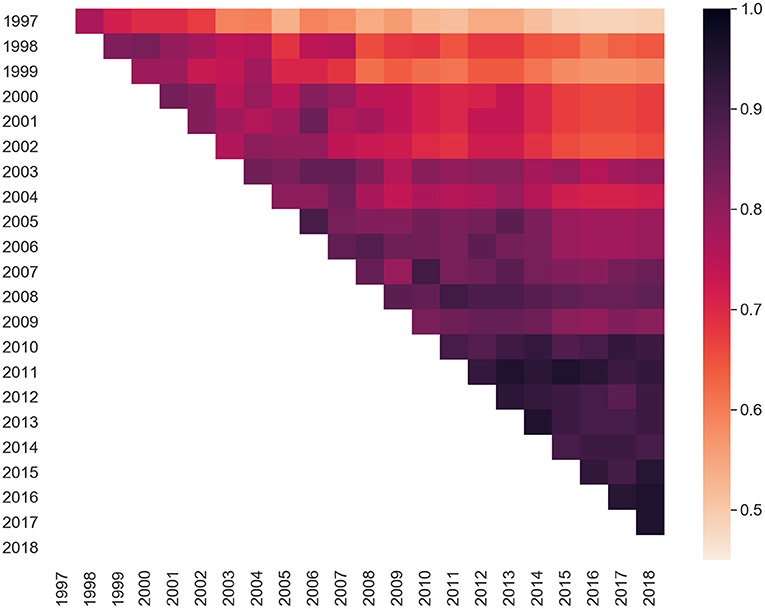
Figure 7. Jaccard similarity heatmap of keywords over pairs of years as an indicator of terminology consolidation in time.
Areas of Overlap Between the Social Science and Technical Domains
Figure 8 sheds light on interdisciplinary topics in the social sciences and technical domains that appear more frequently in research. It shows the number of articles that contain pairs of keywords in both the social sciences and technical domains. Figure 8 suggests that the topics that appear separately in single-domain research (networks, economics, education, etc.) are also the topics where domain-specific AI research or collaboration with domain experts are most frequent in 2010 and 2018. It is plausible that the appearance of keywords from both domains in an article could result from a lax use of those keywords, or commonly used terms (such as “network”) which overlap with our keywords. Nevertheless, keywords from both domains may at least in part result from the cross-disciplinary collaboration.
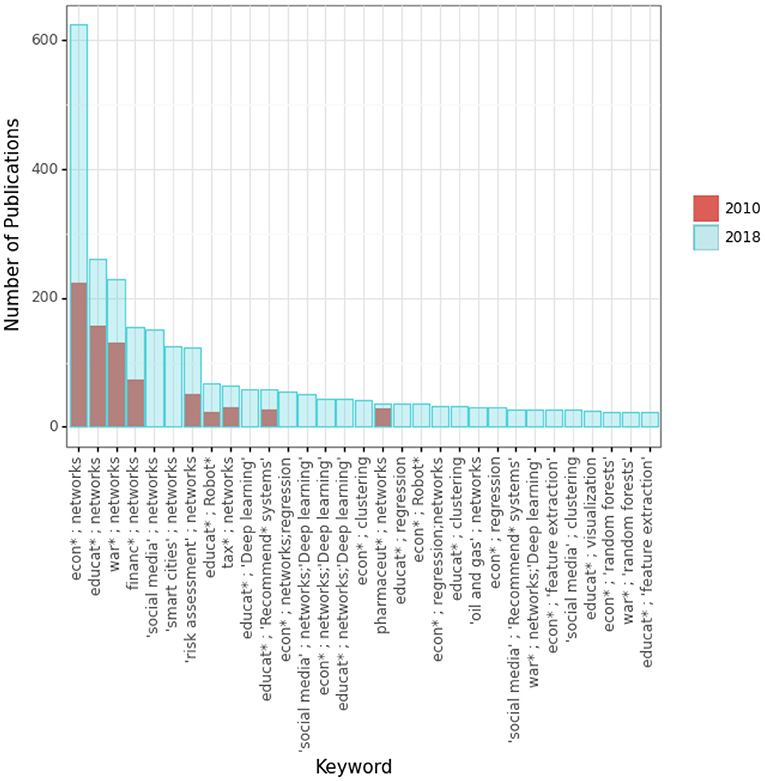
Figure 8. Number of publications with at least one pair of “social”; “technical” keywords in 2010 and 2018.
Figure 9 shows the nine most frequently-used pairs of keywords for both domains from 1997 to 2018 and indicates that collaborations among those topics have been increasing steadily, with a growth rate similar to the increase in the overall number of AI publications (with the exception of the number of articles in economics and networks, which has grown in a faster rate).
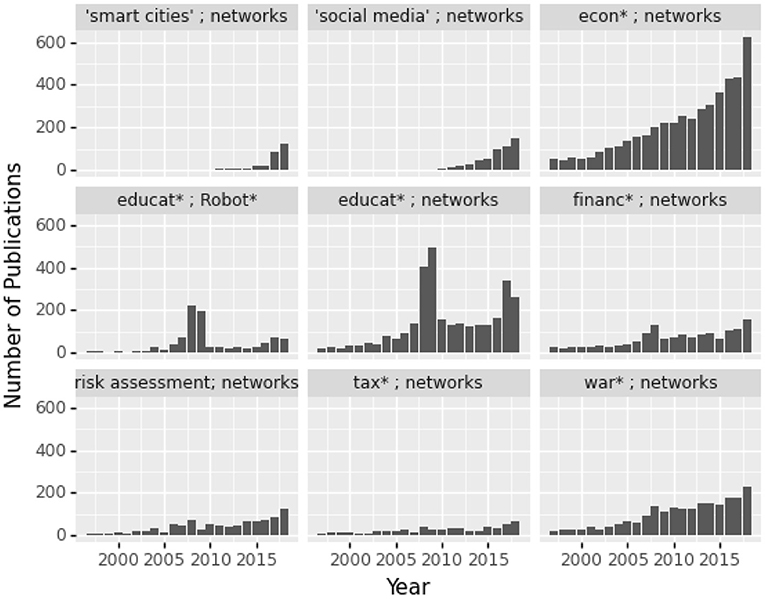
Figure 9. Number of publications for the most frequent “social sciences”; “technical” pairs of keywords (1997–2018).
Governance Gap Between the Social Science and Technical Domains
A noteworthy feature throughout the dataset as illustrated in Figures 8, 9 is the relative low volume of publications containing both AI research and governance-specific terminology. As described in the methodology section, social science keywords captured numerous publications containing keywords such as governance, ethics, legis*, legal liability, civil rights, and others. However, Figures 8, 9 suggest very little interdisciplinary engagement with these topics by AI researchers from both the social science and technical domains (The exception is the combination of risk assessment and networks that appear in the aforementioned graphs.) This “governance gap” in AI research exists despite growing concern by social science researchers about AI governance issues (Wachter et al., 2017; Winfield et al., 2018; Linkov et al., 2020).
This gap also exists despite the interaction between global events and topic-centered research funding. The multi-decade period we considered in this paper covered events ranging from rapid changes in the US national security apparatus following 9/11, the global financial recession in 2008, and other events of international importance to science and technology in general and computer security in particular. Several of these events are referenced in Figure 10. Although there is often some lag, these events are later reflected in academic literature, mediated by the science policy that makes grants available to AI researchers in these areas of emerging concern.
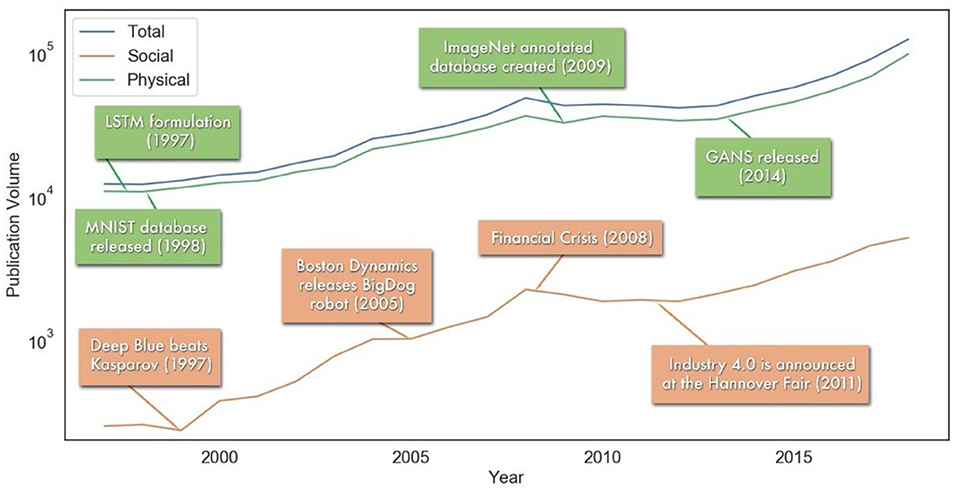
Figure 10. Several selected milestones related to the technical and social domains, correlating the events with the overall annual trends of the domains (1997–2018).
Comprehensive treatment of global events in new AI research would ideally include discussion of governance and ethical issues, but that is not always the case. Intuition tells us that AI research into topics with military and computer security applications, such as image processing and unmanned aerial vehicles, experienced an influx of funding in the decades following 9/11. Important AI keywords for these security and military applications include convolutional neural networks and computer vision, among others. However, our keyword analysis shown in Figures 8, 9 indicates negligible overlap between these technical AI keywords and governance terminology. The governance gap identified by our analysis suggests that the host of ethics and governance issues posed by these technologies have not received deep treatment in the literature.
Discussion
Social science scholarship provides important framing for new technology advancements and implementation. As technology grows in complexity, fewer members of the general population have the technical knowledge to truly understand the technology and evaluate its benefits and potential costs. Social science studies must bridge this gap to ensure that the users of the new technology can understand its implications enough to effectively consent to its use, or, in the converse, to prevent premature refusal of beneficial innovations. For example, users may find it cumbersome to evaluate the security and privacy implications of terms of service for online services. This difficulty may increase with AI-enabled services. Evaluating social implications is not the purview of the technical experts who push the limits of the technology but is equally important in ensuring technology safety for broader society, and ultimately its productive application.
Social science experts are needed to provide social evaluation of technology. They can subsequently bridge uncertainties and communicate the benefits of a technology to the public, especially a skeptical public. A robust social science discourse and evaluation will provide assurances to the public that a technology's application is safe and acceptable according to societal values and may even determine the future trajectory of the field in terms of responding to societal needs.
Social sciences implications of AI have been a concern in teaching and media (Elsevier, 2018), and the need for interdisciplinary research is widely discussed in the computational social sciences community (Kauffman et al., 2017). Nevertheless, our analysis reveals that the body of academic literature in the technical domain is much larger than that in the social science domain. While that gap has narrowed over the approximately 20-year study period, it still shows an output difference in publications between the technical and social domain of one order of magnitude. This finding resembles the results for a similar analysis on synthetic biology (Trump et al., 2018a). In addition to overall trend lines, evidence of the sharp growth of particular AI technical topics is found in the increase in frequency of keywords such as networks and CNNs, deep learning, and feature extraction over the period of study. Therefore, we can conclude from our analysis that academic AI researchers who are conversant with governance issues per se are relatively uncommon. Plausible reasons for this paucity include insufficient AI researcher expertise in governance and ethics, as well as the possibility that governance is commonly considered outside of scope for particular fields or journals (especially the technical ones). However, this analysis also shows that there are overlaps in several important public and commercial sector applications of AI that are current topics of regulatory concern and public conversation.
That gap between technical and social science AI publications is narrowing rather than widening despite the growth and commercial uptake of AI, with a growth in the volume of social science publications mirroring the growth in technical publishing. This suggests intensifying interest on the applications and societal implications of AI by social science researchers. Moreover, the volume of publications addressing topics in both the technical and social science domains suggests a small but increasing volume of interdisciplinary research with possible inter-domain collaboration that has been argued as essential to the development of the AI discipline (Elsevier, 2018). The topics of economics and education appear to be well-represented in literature that encompasses both social science and technical topics. Topics such as smart cities, social media, and risk assessment are relatively less represented in the research addressing both domains, but have increased in frequency over the study period. The point of view of social scientists improves the evaluation of AI research, thus its inclusion in AI studies has bearing on the field at large.
This analysis also shows that the period of analysis (1997–2018) was characterized by relative consensus in terminology, and to some extent avoids effects of emergence, semantic shift, and obsolescence, which could lead to misclassifications of publications across the domains. This suggests that the way the research communities discusses AI has become increasingly consolidated over time in both the technical and the social domains. Remarkably, even between the earliest and latest years of the analysis, we observe that roughly 50% of the keywords used in 1997 were still in use in 2018, suggesting the existence of a stable core of terminology used in the technical and social domains. Unsurprisingly, wider differences in terms are observed between the beginning and end of the study period, and smaller differences occur across shorter periods. We interpret this stability of terminology as beneficial for social science researchers working with technical experts in the AI domain, who now face a narrowed range of disciplinary jargon.
Finally, our analysis suggests a governance gap. There is limited evidence of social science researchers' engagement with important governance topics, as indicated by AI researchers' limited use of the governance keywords such as governance, ethics and moral, human rights, privacy or risk communications. For example, our analysis shows that the volume of technical publications in neural networks grew substantially well before 2005, but governance topics such as risk assessment increased after 2010 or even later. This suggest a period where AI technology may have developed unchecked, with likely benefits for innovative and unrestrained development, but also with the risk of harmful consequences being inadequately considered. An early engagement of social scientists in the development of emerging AI methods may help strike a balance between technical innovation and societal regulation, effectively reducing the governance gap. Explainable AI, for example, may be more regulable.
This gap may be narrowed in the future in a number of ways. First, the social sciences and technical domains can reconcile the concerns of potential drawbacks or challenges posed by social scientists and public stakeholders. Cross-disciplinary research have and may continue to push developers in the lab to foster “safety by design,” while also reducing or eliminating certain research ventures that do not compensate for potentially unacceptable security, ethical, social, or economical outcomes (Cath, 2018; Veale et al., 2018; Winfield et al., 2018). A second way to reduce the governance gap may be in the form of social science research that promotes awareness among the public, for example in the form of research on risk assessment and communications that examine transparency and bias of AI algorithms. This may also enhance the awareness of funding agencies that in turn can promote the engagement of social sciences with AI development. A third way to bridge the governance gap may include research on AI self-governance, such as the use of the technology to enforce ethical principles or to monitor and self-correct bias.
The approach we used to identify trends and the governance gap have been successfully applied to other fields. Nevertheless, our method has limitations that restrict a generalization of our results. Keywords are an imperfect proxy of the topic of research of an article. While some keywords are unequivocally technical (e.g., “gradient descent” or “support vector machines”), others can be ambiguous and characterize research both in the technical and in the social science domains (e.g., smart cities). Moreover, it is possible that the appearance of keywords from both domains in an article could result from a loose use of those keywords, or commonly used terms which overlap with our keywords (For example, the keyword networks may refer to neural networks or to research in 5G cellular communications with no relation to AI). Nevertheless, keywords from both domains may at least in part result from the cross-disciplinary collaboration. In any case, keywords still provide useful (albeit limited) first order approximation of insights about general trends.
In summary, the analytical approach used here has been valuable for its suitability in categorizing and assessing large volumes of publication data. The analysis shows there is a wide but narrowing gap between AI literature published in the technical and social science domains. A potential extension of this work would be to obtain citation information for each publication, which would more clearly assert community structure and causal relationships within the AI dataset. We also note that topics such as AI governance are relatively scarce in the literature, suggesting a need for more research attention. Therefore, in addition to providing an overview for future research, this work has the potential to inform national science and technology research funding strategies which can be leveraged to close those gaps.
Author Contributions
IL and BT developed an idea and proposed methodology. AL and JB conducted analyses, generated results, and interpretation. KR and SG supported literature review and interpretations. BJ and TC queried the keywords of this study on the curated dataset presented in Elsevier (2016, 2018) and returned an abridged version of it, which formed the basis of the study's analysis. All authors contributed to the article and approved the submitted version.
Funding
This study was funded in parts by the US Army Corps of Engineers.
Conflict of Interest
BJ and TC were employed by Elsevier.
The remaining authors declare that the research was conducted in the absence of any commercial or financial relationships that could be construed as a potential conflict of interest.
Acknowledgments
The authors would like to thank Philipp Lorenz-Spreen, Andreas Koher for fruitful discussions and their analytical comments on methodological approaches, as well as Miriam Pollock for their diligent proof-reading of the manuscript and constructive remarks. Further, Maksim Kitsak, Jeff Keisler for their constructive commenting of the manuscript and structural suggestions, as well as Jeff Cegan. The views and opinions expressed in this article are those of the individual authors and not those of the U.S. Army or other sponsor organizations.
References
Ananny, M. (2016). Toward an ethics of algorithms. Sci. Technol. Hum. Values 41, 93–117. doi: 10.1177/0162243915606523
Bijker, W. E., Hughes, T. P., and Pinch, T. (eds.). (2012). The Social Construction of Technological Systems: New Directions in the Sociology and History of Technology. Cambridge, MA: MIT Press.
Calvert, J., and Martin, P. (2009). The role of social scientists in synthetic biology. EMBO Rep. 10, 201–204. doi: 10.1038/embor.2009.15
Cath, C. (2018). Governing artificial intelligence: ethical, legal and technical opportunities and challenges. Philos. Trans. R. Soc. A 376:20180080. doi: 10.1098/rsta.2018.0080
Edwards, L., and Veale, M. (2018). Enslaving the algorithm: from a “right to an explanation” to a “right to better decisions”? IEEE Sec. Priv. 16, 46–54. doi: 10.1109/MSP.2018.2701152
Effendy, S., and Yap, R. H. C. (2017). “Analysing trends in computer science research: a preliminary study using the microsoft academic graph”, in Proceedings of the 26th International Conference on World Wide Web (Perth), 1245–1250. Available online at: http://dblp.uni-trier.de/db/conf/www/www2017c.html#EffendyY17
Elsevier (2016). 6 Simple Search Tips: Lessons Learned from the Scopus Webinar. Available online at: https://blog.scopus.com/posts/6-simple-search-tips-lessons-learned-from-the-scopus-webinar
Elsevier (2018). Artificial Intelligence: How Knowledge is Created, Transferred, and Used. Artificial Intelligence Resource Center. Available online at: https://www.elsevier.com/connect/ai-resource-center
Goodfellow, I., Bengio, Y., and Courville, A. (2016). Deep Learning. MIT Press. Available online at: https://www.deeplearningbook.org
Google (2021). Artificial Intelligence - Explore - Google Trends. Available online at: https://trends.google.com/trends/explore?date=all and geo=US and q=%2Fm%2F0mkz (accessed March 1, 2021).
Kauffman, R. J., Kim, K., and Lee, sang-Y. T. (2017). “Computational social science fusion analytics: combining machine-based methods with explanatory empiricism”, in Proceedings of the 50th Hawaii International Conference on System Sciences (Waikoloa Village, HI), 5048–5057.
Linkov, I., Galaitsi, S., Trump, B. D., Keisler, J. M., and Kott, A. (2020). Cybertrust: from explainable to actionable and interpretable artificial intelligence. Computer 53, 91–96. doi: 10.1109/MC.2020.2993623
Linkov, I., Roslicky, L., and Trump, B. (2019). Resilience and Hybrid Threats: Security and Integrity for the Digital World. Amsterdam: IOS Press.
Macnaghten, P., Kearnes, M. B., and Wynne, B. (2005). Nanotechnology, governance, and public deliberation: what role for the social sciences? Sci. Commun. 27, 268–291. doi: 10.1177/1075547005281531
Miller, T. (2019). Explanation in artificial intelligence: insights from the social sciences. Artif. Intel. 267, 1–38. doi: 10.1016/j.artint.2018.07.007
Mingers, J., and Leydesdorff, L. (2015). A review of theory and practice in scientometrics. Eur. J. Op. Res. 246, 1–19. doi: 10.1016/j.ejor.2015.04.002
Mnyusiwalla, A., Daar, A. S., and Singer, P. A. (2003). ‘Mind the gap': science and ethics in nanotechnology. Nanotechnology 14, R9–R13. doi: 10.1088/0957-4484/14/3/201
Montes, G. A., and Goertzel, B. (2019). Distributed, decentralized, and democratized artificial intelligence. Tech. Forecast. Soc. Change 141, 354–358. doi: 10.1016/j.techfore.2018.11.010
Nemitz, P. (2018). Constitutional democracy and technology in the age of artificial intelligence. Philos. Trans. R. Soc. A 376:20180089. doi: 10.1098/rsta.2018.0089
Rahwan, I., Cebrian, M., Obradovich, N., Bongard, J., Bonnefon, J.-F., Breazeal, C., et al. (2019). Machine behaviour. Nature 568, 477–486. doi: 10.1038/s41586-019-1138-y
Selbst, A. D., Altman, M., Balkin, J., Bambauer, J., Barocas, S., Belt, R., et al. (2017). Disparate impact in big data policing. Georgia Law Rev. 109, 109–196. doi: 10.2139/ssrn.2819182
Shapira, P., Youtie, J., and Li, Y. (2015). Social science contributions compared in synthetic biology and nanotechnology. J. Respons. Innov. 2, 143–148. doi: 10.1080/23299460.2014.1002123
Siebert, M., Kohler, C., Scerri, A., and Tsatsaronis, G. (2018). Technical Background and Methodology for the Elsevier's Artificial Intelligence Report. Elsevier's AI Report.
Sinha, A., Shen, Z., Song, Y., Ma, H., Eide, D., June Hsu, B., et al. (2015). “An overview of microsoft academic service (MAS) and applications”, in Proceedings of the 24th International Conference on World Wide Web (Florence), 243–246. doi: 10.1145/2740908.2742839
Sutton, R. S., and Barto, A. G. (2018). Reinforcement Learning: An Introduction. Cambridge, MA: MIT Press.
Torgersen, H. (2009). Synthetic biology in society: Learning from past experience? Syst. Synth. Biol. 3:9. doi: 10.1007/s11693-009-9030-y
Trump, B. D., Cegan, J., Wells, E., Poinsatte-Jones, K., Rycroft, T., Warner, C., et al. (2019). Co-evolution of physical and social sciences in synthetic biology. Crit. Rev. Biotechnol. 39, 351–365. doi: 10.1080/07388551.2019.1566203
Trump, B. D., Cegan, J. C., Wells, E., Keisler, J., and Linkov, I. (2018a). A critical juncture for synthetic biology. EMBO Rep. 19, 7. doi: 10.15252/embr.201846153
Trump, B. D., Cummings, C., Kuzma, J., and Linkov, I. (2018b). A decision analytic model to guide early-stage government regulatory action: applications for synthetic biology. Regul. Govern. 12, 88–100. doi: 10.1111/rego.12142
Veale, M., Binns, R., and Edwards, L. (2018). Algorithms that remember: model inversion attacks and data protection law. Philos. Trans. A 376:83. doi: 10.1098/rsta.2018.0083
Wachter, S., Mittelstadt, B., and Floridi, L. (2017). Transparent, explainable, and accountable AI for robotics. Sci. Robot. 2:6. doi: 10.1126/scirobotics.aan6080
Keywords: AI, review – systematic, risk, taxonomy, machine learning
Citation: Ligo AK, Rand K, Bassett J, Galaitsi SE, Trump BD, Jayabalasingham B, Collins T and Linkov I (2021) Comparing the Emergence of Technical and Social Sciences Research in Artificial Intelligence. Front. Comput. Sci. 3:653235. doi: 10.3389/fcomp.2021.653235
Received: 14 January 2021; Accepted: 23 March 2021;
Published: 26 April 2021.
Edited by:
Yannick Chevalier, Université Toulouse III Paul Sabatier, FranceReviewed by:
José Antonio Álvarez-Bermejo, University of Almeria, SpainAndrey Chechulin, St. Petersburg Institute for Informatics and Automation (RAS), Russia
Copyright © 2021 Ligo, Rand, Bassett, Galaitsi, Trump, Jayabalasingham, Collins and Linkov. This is an open-access article distributed under the terms of the Creative Commons Attribution License (CC BY). The use, distribution or reproduction in other forums is permitted, provided the original author(s) and the copyright owner(s) are credited and that the original publication in this journal is cited, in accordance with accepted academic practice. No use, distribution or reproduction is permitted which does not comply with these terms.
*Correspondence: Igor Linkov, aWdvci5saW5rb3ZAdXNhY2UuYXJteS5taWw=