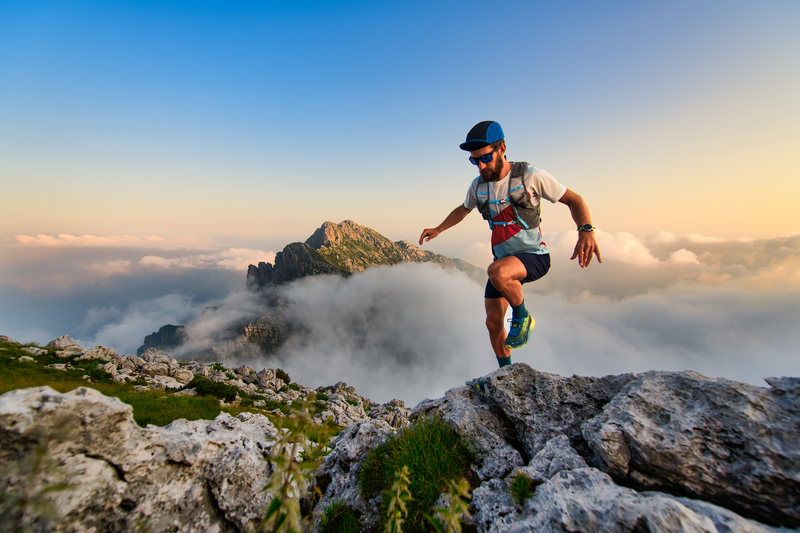
95% of researchers rate our articles as excellent or good
Learn more about the work of our research integrity team to safeguard the quality of each article we publish.
Find out more
ORIGINAL RESEARCH article
Front. Comput. Sci. , 17 January 2022
Sec. Human-Media Interaction
Volume 3 - 2021 | https://doi.org/10.3389/fcomp.2021.652550
This article is part of the Research Topic Brain-Computer Interfaces for Non-clinical (Home, Sports, Art, Entertainment, Education, Well-being) Applications View all 12 articles
To enable virtual reality exposure therapy (VRET) that treats anxiety disorders by gradually exposing the patient to fear using virtual reality (VR), it is important to monitor the patient's fear levels during the exposure. Despite the evidence of a fear circuit in the brain as reflected by functional near-infrared spectroscopy (fNIRS), the measurement of fear response in highly immersive VR using fNIRS is limited, especially in combination with a head-mounted display (HMD). In particular, it is unclear to what extent fNIRS can differentiate users with and without anxiety disorders and detect fear response in a highly ecological setting using an HMD. In this study, we investigated fNIRS signals captured from participants with and without a fear of height response. To examine the extent to which fNIRS signals of both groups differ, we conducted an experiment during which participants with moderate fear of heights and participants without it were exposed to VR scenarios involving heights and no heights. The between-group statistical analysis shows that the fNIRS data of the control group and the experimental group are significantly different only in the channel located close to right frontotemporal lobe, where the grand average oxygenated hemoglobin Δ[HbO] contrast signal of the experimental group exceeds that of the control group. The within-group statistical analysis shows significant differences between the grand average Δ[HbO] contrast values during fear responses and those during no-fear responses, where the Δ[HbO] contrast values of the fear responses were significantly higher than those of the no-fear responses in the channels located towards the frontal part of the prefrontal cortex. Also, the channel located close to frontocentral lobe was found to show significant difference for the grand average deoxygenated hemoglobin contrast signals. Support vector machine-based classifier could detect fear responses at an accuracy up to 70% and 74% in subject-dependent and subject-independent classifications, respectively. The results demonstrate that cortical hemodynamic responses of a control group and an experimental group are different to a considerable extent, exhibiting the feasibility and ecological validity of the combination of VR-HMD and fNIRS to elicit and detect fear responses. This research thus paves a way toward the a brain-computer interface to effectively manipulate and control VRET.
Exposure therapy is a form of therapy that treats anxiety disorders by gradually and repeatedly exposing the client to his/her fear (Brinkman et al., 2009) in the absence of harm. This can activate the fear extinction process and was proven to be an effective intervention (Hofmann, 2008). Recently, virtual reality (VR) has been introduced to exposure therapy by the evidence that realistic virtual circumstances can have a significant influence on a person's mental state (Riva et al., 2007; Martens et al., 2019), which can pave a way to a successful exposure therapy. Among a vast variety of VR hardware, head-mounted display (HMD) has been shown to be effective in improving the sense of presence in a virtual environment (VE), which is the key element of effective application of VR in the mental health domain (Jerdan et al., 2018). Realistic immersive VEs enable researchers to ecologically perform experiments and invent therapy methods, leading to effective and highly ecologically valid virtual reality exposure therapy (VRET) systems (Martens et al., 2019). HMD-based VR enables an immersive VRET that makes the exposure therapy more controlled, safer, and in some cases also less expensive than traditional exposure therapy (Teo et al., 2016; Boeldt et al., 2019; Bălan et al., 2020). Furthermore, the exposure protocol can be completely standardized when using VRET, which increases the therapist's control over the stimuli and the duration of the exposure, as opposed to traditional in vivo exposure (Rizzo et al., 2013). Despite the higher level of control that VRET offers to the therapist, it is still a common practice that the therapist monitors the fear responses of the client (Brinkman et al., 2009). One important reason to do this is to ensure that the gradual exposure to the fear-eliciting stimuli do not overwhelm the client. Excessive exposure to situations that induce fear can, for example, cause panic attacks for the client and might therefore worsen the anxiety instead of treating it (Boeldt et al., 2019).
However, monitoring a person's fear responses while using VR has been a big challenge. The traditional option of tracking facial expressions becomes difficult when the user is wearing a VR-HMD. Subjective ratings suffer from the difficulty in verbalizing current mental state indication (Hill and Bohil, 2016) and memory bias (Rodríguez et al., 2015). Neuroimaging techniques have been recently proposed to objectively and unobtrusively measure fear response during virtual fear exposure but are limited to the use of electroencephalogram (EEG) (Hu et al., 2018; Peterson et al., 2018; Bălan et al., 2020). However, the disadvantages of EEG include susceptibility to motion artifacts and electrical signal interference which can be anticipated when a user interacts with VR technology. On the other hand, functional near-infrared spectroscopy (fNIRS) offers a recording of cortical activity in a natural mobility setting with higher spatial resolution than EEG, less susceptibility to motion artifacts and electrical noises, portability, and lightweight characteristic. These advantages substantiate the great potential for the combination of VR-HMD and fNIRS, which has been recently demonstrated in a bisection task (Seraglia et al., 2011), the assessment of prospective memory (Dong et al., 2017; Dong et al., 2018), the processing of racial stereotypes (Kim et al., 2019), performance monitoring during training (Hudak et al., 2017), and a neurofeedback system to support attention (Aksoy et al., 2019). However, the feasibility and ecological validity of using fNIRS to measure fear response during virtual fear exposure is still unexplored.
The neural mechanisms underpinning the fear circuit have been widely researched. The majority of fNIRS studies on cortical responses to fear-invoking stimuli report an increase in cortical activations in the parietal cortex (Köchel et al., 2013; Zhang et al., 2017) or the prefrontal cortex (PFC) (Glotzbach et al., 2011; Roos et al., 2011; Ma et al., 2013; Landowska, 2018; Rosenbaum et al., 2020) during fearful stimulation. PFC areas in which significant activations were found include the left PFC (Ma et al., 2013), dorsolateral PFC (dlPFC), anterior PFC (Landowska, 2018), left dlPFC, and left ventrolateral PFC (vlPFC) (Rosenbaum et al., 2020). The studies that found activations in the parietal cortex presented subjects to fearful and neutral sounds. Decreased chromophores deoxygenated hemoglobin (HbR) concentration changes (Köchel et al., 2013) and higher oxygenated hemoglobin (HbO) concentration changes (Zhang et al., 2017) were found when subjects were listening to fearful sounds as compared to neutral sounds. The areas with significant activations include the (right) supramarginal gyrus and the right superior temporal gyrus. The studies that found an increased cortical activation in the PFC exposed their subjects to spiders (Rosenbaum et al., 2020), fearful faces (Glotzbach et al., 2011; Roos et al., 2011), or a fear-learning experiment based on shocks (Ma et al., 2013). A recent fNIRS study observed decreased HbO concentration changes in the dlPFC and anterior PFC when participants with moderate acrophobia were exposed to a cave VE that displayed artificial heights (Landowska et al., 2018). The effect was intense during the first exposure session, but the learning process on coping with fear responses affected the following sessions. In general, the majority of fNIRS studies reported increased HbO concentration changes in the PFC when subjects were exposed to the fearful stimuli as compared to the control situations and occasionally reported the complementary decrease in HbR concentration changes (Glotzbach et al., 2011). The increment of HbO concentration changes is also in line with other neuroimaging studies beyond fNIRS that found increased cortical activity in the PFC as fearful responses (Lange et al., 2003; Nomura et al., 2004) of healthy subjects, while the activity in the amygdala is inversely related (Nomura et al., 2004). In contrast, patients with anxiety disorder show decreased activity in the PFC in response to fearful stimuli and increased activity in the amygdala (Etkin and Wager, 2007; Shin and Liberzon, 2010; Price et al., 2011). It is thus evident that the PFC plays an important role in mediating fear responses (Landowska et al., 2018) and is known as a major component of the cognitive control network (Rosenbaum et al., 2020).
Despite evidence of PFC activity due to the fear circuit as reflected by fNIRS signals, little is known about fear responses in highly immersive VR, especially when using HMD. The current study investigates the possibility of inducing and detecting fear responses in VR-HMD using fNIRS. Specifically, we are interested in inducing and detecting a fear of heights response, which is one of the most prevailing types of human fear which can be reproduced in VR, alongside (Garcia-Palacios et al., 2002; Miloff et al., 2019; Lindner et al., 2020), fear of flying (Rothbaum et al., 2000; Maltby et al., 2002; Rothbaum et al., 2006), fear of driving (Wald and Taylor, 2000), and even post-traumatic stress disorders (Rothbaum et al., 2001; Difede and Hoffman, 2002; Gerardi et al., 2008; Rothbaum et al., 2014). Brain studies on fear of heights using fNIRS have been done in VE (Emmelkamp et al., 2001; Donker et al., 2018; Freeman et al., 2018; Gromer et al., 2018), but the previous works recruited participants either with or without fear of heights. The study in VR-HMD remains unexplored and is the main objective in this study, where we aimed to recruit participants both with and without fear of heights to allow a comparison between groups. Our first research question is as follows:
1) To what extent do the fNIRS signals captured from participants with a fear of heights response and participants without it differ?
To answer this question, we invited both participants with fear of heights (experimental group) and participants without fear of heights (control group) to participate in our experiment, during which they were exposed to virtual height and virtual ground conditions. It was hypothesized that the virtual heights will cause a fear response for the experimental group but does not cause a fear response for the control group. Furthermore, it was hypothesized that the ground condition does not cause a fear response for any of the groups.
In addition, we aimed to train simple machine learning classifiers to automatically detect fear responses of the experimental group from fNIRS signals, which has not been done in previous works. Our second research question is as follows:
2) To what extent can a person's fear of heights response to a virtual reality environment be detected by a simple machine learning model using fNIRS data?
To answer this question, we trained and tested linear classifiers in subject-dependent and subject-independent ways on the data of the experimental group and evaluated the performance in distinguishing ground-condition and height-condition data. Our first attempt to achieve a successful classification of different fNIRS responses to fear of heights elicited in VR-HMD would exhibit ecological validity of combining both components, serving as a baseline toward a practical and effective VRET in the future improvement.
Two different groups of participants were recruited and pre-screened by the Acrophobia Questionnaire (AQ), consisting of 20 items that are rated on a seven-point Likert scale, ranging from not anxious at all to extremely anxious (Cohen, 1977; Antony, 2001). Only participants with fear of heights who scored higher than 35 were invited to participate as the experimental group. On the other hand, only participants without fear of heights who scored lower than 20 were invited to participate as the control group (Gromer et al., 2018). Accordingly, 20 participants (nine females, age = 26.10 ± 10.47 years) in the experimental group reported a high AQ score (52.40 ± 11.47), and 21 other participants (nine females, age = 22.95 ± 2.11 years) in the control group reported a low AQ score (9.71 ± 5.89). None of the participants suffered from anxiety disorders.
The study was approved by the institutional ethics committee of University of Twente (reference number: RP 2020-76). All procedures were in accordance with the Helsinki Declaration. After confirming the eligibility of the participants and obtaining written informed consent, the participants were introduced to the Oculus Rift S 1, which is a VR-HMD with six degrees of freedom enabling tracking of head rotations and translations (forward/backward, left/right, up/down). Therefore, the participants were able to look around in the VEs by simply rotating their head and to walk around by moving their body in the physical world. The researcher demonstrated how the VR-HMD should be adjusted to fit the head. Hand-held controllers were given to be held during the experiment to make the tracking of the VR-HMD more reliable, but the participants were not allowed to use the controllers. To familiarize the participants with the VE, the HMD, and holding the controller, a practice round with an example VE similar to the ground condition was included and continued until the participant indicated satisfactory familiarity. Then, the VR-HMD was removed, and the participants were fitted with the fNIRS headset. The fNIRS system was calibrated, and the signal quality was assessed visually by the researcher. Afterwards, the participants were asked to stand in a designated place and to fit the VR-HMD themselves. The straps of the VR-HMD were loosened as much as possible to reduce the risk of optode displacement. Figure 2 shows a participant wearing both the fNIRS headset and the VR-HMD. After preparation, the participants were asked to perform the task. The researcher instructed the participants about the maximum level of movement which they were allowed to perform in order to minimize the motion artifacts in the fNIRS signal. Although the VR-HMD provides the possibility to walk around in the VE, the participants were instructed to refrain. Instead of moving, they were asked to gently look around in the VE, while preventing large head movements. Additionally, they were allowed to bend forward slightly during the height condition but were asked to return to the original position after bending forward.
All participants were tested under the same procedure. There were two conditions of VEs: ground condition and height condition. Each condition was presented alternately for five trials, each of which lasted 30 s and was preceded with a baseline period of 20 s, in which neither visual nor auditory stimuli were presented and the participants were instructed to relax and avoid active thinking. In the ground condition, the participants were virtually standing on a sidewalk or square in the middle of a city in the VE, while in the height condition, the participants were virtually standing by the rooftop of a high building. The VEs were created using the Unity development platform 2. See Figure 1 for examples of the scenes. Both conditions were accompanied by city sounds, to increase the immersiveness of the experience.
FIGURE 1. The experimental design showing the exemplified VE scenes of the ground condition and height condition.
After the experiment, the participants were asked to rate their perceived feelings of distress or fear using the Subjective Units of Distress Scale (SUDS) (Wolpe, 1969) during ground and height conditions on an 11-point Likert scale ranging from 0 (no distress/anxiety) to 100 (worst distress/anxiety that you have ever felt). The SUDS questionnaire is often used to assess exposure settings during cognitive behavioral treatment (Benjamin et al., 2010). Additionally, the participants were asked to fill out 14 items of the IGroup Presence Questionnaire (IPQ), which measures a person's sense of presence in VR (Schubert et al., 2001), to test if the participants felt sufficiently present in the VEs for a fear response to emerge. After that, the participants were asked to fill out the AQ to confirm the group membership; median = 0.82 (Cohen, 1977) indicates adequate test–retest reliability, suggesting that pre- and post-experiment AQ scores should be similar. Finally, a structured interview by the researcher was held to ask the participants if and when they felt fearful or any other emotions during the experiment to gather extra feedback.
Changes in HbO and HbR concentrations were measured using the Artinis Brite 24 3. The Brite is a wireless continuous wave fNIRS device that can measure up to 27 channels. The near-infrared light is emitted at two nominal wavelengths: 760 and 850 nm. Cortical hemodynamic responses were measured at a sampling rate of 10 Hz. The optodes were arranged to cover a large region of the PFC, including the dlPFC, anterior PFC, and part of the vlPFC. Every emitter–detector pair had a maximum distance of 3 cm between the optodes. Figure 2 shows the positioning of the optodes and channels on the scalp, with an overview of the 10–20 system as a reference. In order to prevent near-infrared light being absorbed by hair, the researcher used a narrow, oblong tool to move the participant's hair to the side when it fell between an optode and the participant's scalp. Signal quality was visually validated by confirming the presence of cardiac cycles in the fNIRS signals (Hocke et al., 2018).
FIGURE 2. The experimental setting; (A) a participant wearing the fNIRS headset and the VR-HMD during the experiment; (B) the positioning of the optodes projected on the layout of the 10–20 system, showing the detectors (blue), emitters (yellow), and the channels indicated by a circle with a number in it; (C) the overview of statistical analysis.
The fNIRS data were recorded using the Artinis Oxysoft software4. The raw data were converted to Δ(HbO) and Δ(HbR) signals (Chen, 2016; Pinti et al., 2019) using the modified Beer–Lambert law (Delpy et al., 1988; Scholkmann et al., 2014). After that, the data were exported to Matlab using Oxysoft2Matlab script and visually inspected. Channels with severe motion artifacts (usually with an amplitude of 5 μM) and channels that did not show cardiac cycles (evident by the repetitive alternation of around 0.10 μM of the amplitude) were excluded from further analysis. Motion correction was applied to the remaining channels, using the Temporal Derivative Distribution Repair procedure (Fishburn et al., 2019). After that, the correlation coefficients of every channel's Δ(HbO) and Δ(HbR) signals were calculated. Channels with a positive correlation coefficient were removed, following a previous fNIRS study suggesting that a negative correlation can be expected when the amount of motion artifacts in the signals is low (Cui et al., 2010). Then, a third-order Butterworth band-pass filter (Hocke et al., 2018; Pinti et al., 2019) with low cut-off frequency 0.01 Hz and high cut-off frequency 0.1 Hz was applied to remove physiological noise arising from breath cycles (∼0.2–0.3 Hz), cardiac cycles (∼1 Hz), and Mayer waves (∼0.1 Hz) (Naseer and Hong, 2015; Pinti et al., 2019). The filtered signals were separated into trials and adjusted with a baseline, yielding five ground-condition trials and five height-condition trials. The main duration of trials was set from 0 to 30 s after the stimulus presentation onset, covering the entire task period of each trial. The 5-s period preceding the stimulus presentation was used as a baseline period. For each participant and each channel, the signals were grand averaged across trials in each condition.
A between-group analysis was performed by investigating the significant differences of fNIRS signals between the control group and the experimental group. In order to investigate the effect of height on participants with fear of heights, a within-group analysis was performed on the data of ground-condition trials and height-condition trials of merely the experimental group to investigate significant difference of fNIRS signals in the two conditions. Statistical testing was conducted using Matlab 2020a, and the overview of the statistical analysis performed is illustrated in Figure 2.
To mitigate the inter-subject variability issue, we computed a contrast between the ground-condition and the height-condition grand average Δ[HbO] signals and Δ[HbR] signals for all channels and all participants. The contrast was computed by subtracting the grand average ground condition signal from the grand average height condition signal. For all of these signals, the mean over the window from 3 to 15 s post-stimulus onset was computed, following the evidences that the hemodynamic response only starts to become visible after 3 s [2.8-s lag was found (Lachert et al., 2017)] and that the hemodynamic response is most intense in the first 5–17 s after the stimulus onset (Khan et al., 2020). For every channel, a permutation test with 50,000 permutations was used to test for significant differences between the contrast signal means of the control group and the experimental group, at the significance level α = 0.05. The permutation test was chosen as it is a non-parametric test that can be used on small sample sizes and makes no assumptions about the distribution of the data.
Similar to the between-group analysis, the grand average ground condition Δ(HbO) and Δ(HbR) signals and the grand average height condition Δ(HbO) and Δ(HbR) signals were averaged over the 3–15 s window. For every channel, a permutation test with 50,000 permutations was used to test for significant differences between the ground-condition trial means and the height-condition trial means over the 3–15 s window, at the significance level α = 0.05.
Four statistical analyses were executed on the fNIRS data (between/within group analysis on the Δ[HbO]/Δ[HbR] data) per channel, yielding a total of 4 × 27 = 108 hypothesis tests from all channels. False discovery rate correction, as suggested by Genovese et al. (2002) for neuroimaging data, was executed on the 108 p-values that resulted from the statistical analyses to correct for multiple comparisons. The rate q was set to 0.05.
We used data from all channels that are not corrupted by movement artifacts and hardware malfunctions (as explained in Section 2.4.1) for classification, where the number of available channels differs across participants. Instead of extracting features per channel, we first calculated the averages of Δ[HbO] and Δ[HbR] measurements over the remaining channels, yielding the
Subject-dependent classifiers were trained and tested only for the experimental group due to the clear distinction of fear responses between height-condition trials and ground-condition trials, labeled as fear response and no fear response, respectively. All 1-s windowed data from the first six trials (consisting of three ground-condition trials and three height-condition trials) were used as training data, and the remaining windowed data from four trials were the test data. As data were extracted from 3 to 15 s after stimulus onset, this resulted in 12 × 6 = 72 training data instances and 12 × 4 = 48 test data instances from each participant. In this study, linear discriminant analysis (LDA) and support vector machines (SVM) with linear kernel, implemented in Matlab 2020a, were trained with the standard hyper-parameter settings. Specifically, a linear coefficient threshold of 0 was used with regularized LDA. Sequential minimal optimization was applied to the linear-SVM and without feature scaling. The performance in the modes of 1-, 3-, and 5-s history was measured by the accuracy. Similarly, subject-independent classifiers were trained and tested with the experimental group using leave-one-subject-out cross-validation. It can be useful in real-life VRET settings to classify unseen data from an unknown participant (Bălan et al., 2020). In order to compare the subject-dependent classification with a random classifier (50% accuracy), the 95% confidence interval is calculated for each classifier. The lower (bl) and upper bounds (bu) of the 95% confidence interval are based on the Wilson score interval (Wilson, 1927) and are given by the formula
where
Three participants withdrew from the experiment due to motion sickness caused by the VR-HMD. SUDS threshold at 30 was used to distinguish the feeling of relaxation and fear, where participants should report higher than this threshold when feeling fear during the experimental condition. As a result, two participants were excluded from each group due to the mismatch between the reported SUDs score and the expected range. In addition, the threshold of IPQ was set at 3 as the minimum for the feeling of presence in the VR, leaving two participants, whose scores did not surpass the threshold, out from the experimental group. Besides, the AQ scores were used to reconfirm the group membership after the experiment, resulting in one and two participants removed from the control and experimental groups, respectively. Consequently, a total of 15 participants (nc = 15) remained to be part of the control group and 14 participants (ne = 14) were part of the experimental group. Table 1 shows the mean scores and standard deviations of the questionnaire results for both groups; it suggests a clear distinction between the two groups in terms of AQ scores (pre-experimental as well as post-experimental) and SUDS for the height condition. The two groups scored similarly for SUDS in ground condition and for the experienced presence in the VEs.
TABLE 1. Mean scores and standard deviations of the questionnaire results for the control group and the experimental group.
Figure 3 shows the grand average Δ[HbO] traces of the contrast between the ground condition and the height condition for the two groups for every channel, with the standard error given around every trace. It is apparent that only channel 3 (p = 0.000 8) generated a significant difference between the contrast Δ[HbO] means of both groups using statistical testing with the false discovery rate (FDR) correction. Meanwhile, the results from Δ[HbR] traces, as also shown in Figure 3, suggested that there are some channels (i.e., channels 15, 23, 24, and 27), where the grand average trace of the control group has a different pattern than that of the experimental group. However, none of them are significant after the corrected statistical testing.
FIGURE 3. Grand average Δ(HbO) and Δ(HbR) traces of the contrast between ground condition and height condition for the two groups: control group (black traces) and experimental group (red traces for Δ(HbO) and blue traces for Δ(HbR)), with standard deviation. The gray shaded area (3–15 s post-stimulus) is the window over which the means were taken that were used for the permutation tests. The horizontal axis represents time in seconds, ranging from 0 to 30, and the vertical axis represents concentration change in μM, ranging from −0.4 to 0.6. The plots are corresponding to channel labels, which are arranged in accordance with the optode layout that was used during the experiment, as presented in Figure 2. Channel numbers are labeled in every plot. The plots surrounded by the border shows the channel where a significant difference was found between the means of the control group and the experimental group. The graphs are arranged according to the optode layout that was used during the experiment, as presented in Figure 2.
Figure 4 shows the grand average Δ[HbO] traces of the ground condition and the height condition for the experimental group, with the standard error given around every trace. Apparently, the difference between the two conditions can be clearly observed, especially on the salient increase of Δ[HbO] during 3–15 s after stimulus compared to the rather constant trace in ground condition. FDR-corrected permutation test shows that the distinct patterns are significantly different in channels 1 (p = 0.002 2), 2 (p = 0.002 2), 3 (p = 0.000 01), 4 (p = 0.000 3), 6 (p = 0.002 2), 11 (p = 0.001 6), 12 (p = 0.004 1), 14 (p = 0.001 6), 18 (p = 0.000 9), 20 (p = 0.000 02), 23 (p = 0.000 02), 25 (p = 0.000 1), and 26 (p = 0.002 2). In contrast, Δ[HbR] traces, as also shown in Figure 4, are rather flat for both conditions, whereas only the difference in channel 23 (p = 0.001 7) is significant after the corrected statistical testing.
FIGURE 4. Overview of the grand average Δ(HbO) and Δ(HbR) traces of the ground condition (green traces for Δ(HbO) and blue traces for Δ(HbR)) and height condition (orange traces for Δ(HbO) and purple traces for Δ(HbR)) of the experimental group, with standard deviation. The gray shaded area is the window over which the means were taken that were used for the permutation tests. The horizontal axis represents time in seconds, ranging from 0 to 30, and the vertical axis represents concentration change in μM, ranging from −0.4 to 0.6. The plots are corresponding to channel labels, which are arranged in accordance with the optode layout that was used during the experiment, as presented in Figure 2. The plots surrounded by boarders show the channels where a significant difference was found between the means of the ground condition and the height condition for the experimental group.
Due to motion artifacts and hardware malfunctions, some channels were excluded from the analyses in some participants; i.e., features were extracted from the remaining uncorrupted channels per participant (see Section 2.4.1). In this study, we trained and tested the subject-independent classifiers with the data from only of the uncorrupted Δ[HbO] channels, as many corrupted Δ[HbR] channels were excluded for many participants, which makes it unfeasible to train and test classifiers on these data.
Table 2 shows the accuracies of the subject-dependent classification and the 95% confidence interval, calculated using Eq. 1. The mean accuracy computed across all participants suggests that the SVM on the 3-s history performs best, with a mean accuracy of 69.69% (SD 16.94). However, the mean accuracies of the other classifiers are close to that of the 3-s history SVM, with a maximal difference of roughly 1.4%. Therefore, the amount of history taken into account in the classification seems to have a minimal effect on this metric. LDA and linear SVM achieved similar performance with a maximum of 1.2% in accuracy difference. From Eq. 1 one can easily deduce that if the estimated performance is above 64.5% then the lower bound of the 95% confidence interval is higher than 50%. Recall that a subject-dependent classifier is tested on 48 samples; hence, n = 48. This implies that for most types of classifiers considered, only 7 out of the 14 subject-dependent classifiers perform significantly better than random. In order to show that the mean of the different subject-dependent classification is significantly higher than the mean of a random classifier for each participant, we take a somewhat different approach. Since the mean over all subject-dependent classification is not the outcome of a Bernoulli experiment (it is the mean over different Bernoulli experiments) we cannot apply the Wilson score interval. But the mean performance of 14 subject-dependent random classifiers is equivalent to an estimated performance of a Bernoulli experiment with 14 × 48 trials. Since the success rate of a random classifier is known and equal to 50%, we can estimate the 99% confidence interval (z = 2.576) using Eq. 1 and is given by (45%, 55%). This means that in 99% of the cases, the observed mean of the random classification will be in this interval.
TABLE 2. Accuracies and confidence intervals [lower bound, upper bound] of the subject-dependent classification.
The performance also varies considerably among the different participants; while classification in participants 1, 2, and 9 achieved low accuracy, classification for participants 7 and 10 was almost perfect. In some participants, the accuracy also changed by classification methods and history by an amount of almost 15% (participant 1), while these factors had minimal impact on the accuracy in other participants (e.g., participants 7 and 10). This led us to the analysis of data distribution and its effect on the classification performance.
We investigated the data distribution in feature space of representative participants: participants 2 and 9 with relatively low classification accuracy and participant 7 with high classification performance. Specifically, principal component analysis (PCA) was applied to 1-s history data, and we visualized the distribution of data projected on the first and second principal components (PCs) to investigate if the training and test data are identically distributed as shown in Figure 5. Training and test data are represented in different colors. The decisions made for the test data by the LDA and linear-SVM classifiers are also depicted. It should be noted that data distribution in feature space of 3- and 5-s history data are generally similar to 1-s history data and therefore not shown here. Also, it is worth mentioning that PCA is applied for visualization purposes only and not for feature dimension reduction.
FIGURE 5. Training, test data, and classification decisions from LDA and SVM of the 1-s subject-dependent classification of participants 2 (P2), 7 (P7), and 10 (P10), plotted against the first and second principle components.
In the data of participant 2 there is a high overlap in the test data between the distribution of the fear and non-fear classes, making it difficult to reach decent performance. In participant 9 data, LDA and linear-SVM learned to distinguish classes along the second PC, while the test data are clearly separable along the first PC, thereby yielding performance around chance level. In contrast, data distribution of training and test data are rather similar for participant 7, with a much clearer linear separability in test data. The classification for this participant is therefore high for of the linear classifiers.
Table 3 shows the accuracies of the subject-independent classifiers and the 95% confidence interval, calculated using Eq. 1. For the subject-independent classification, the 95% confidence intervals are smaller, since these are tested on n = 120 samples. This also implies that if the estimated performance is above 59% then the lower bound of the 95% confidence interval is larger than 50%. This implies that for most types of classifiers 12 out of 14 subject-independent classifiers perform significantly better than random. In order to compare the mean performance of the subject-independent classification with the mean of subject-independent random classification, we take the same approach as for the subject-dependent classification. In this case we have 14 × 120 trails, and the 99% confidence interval is given by (47%, 53%). Based on the mean accuracies computed from all participants, it can be inferred that the SVM on the 5-s history performs best, with a mean accuracy of 74.46% (SD 12.34). On the contrary, the SVM on the 1-s history performs the worst on average, with a mean accuracy of 71.67% (SD 11.60). From the calculated 99% confidence interval (47%, 53%) for the mean of random subject-independent classification (see Section 2.6.2), one can easily deduce that the mean of the subject-dependent classification is significantly higher (99.5% confidence) than the mean of random subject-independent classification.
TABLE 3. Accuracies and confidence intervals [lower bound, upper bound] of the subject-independent classification).
Again, the difference between the accuracies of the classifiers that perform best and worst on average is only a few percent, indicating that the amount of history and the classification methods have merely minor influence on the classification performance. Again, the accuracies vary considerably among participants, ranging from 48.33% (participant 8) to 91.67% (participant 10), and the cause of this variation is also investigated by data distribution in PC space.
In the data of participant 8, the training data depicted in Figure 6 (P8) shows that the data labeled as no fear are mostly centered around the negative values of the first PC, while fear data were located around the positive values. However, the test data of Figure 6 (P8) have a different pattern. Instead, the data of the different labels are distributed over the positive and negative values of the second PC and are quite overlapping, indicating the difficulty to separate the test data of the different labels by a linear decision boundary. This might explain why the classifiers, which seemingly learned to separate classes along the first PC, cannot perform well on the test data, yielding low accuracy. In contrast, data distribution in test data from participant 10 [see Figure 6 (P10)] are linearly separable in the first PC. Specifically, data labeled as no fear are centered around the negative values of the first PC, and the test data labeled as fear are centered around the positive values of the first PC. The linear classifiers were therefore successful in generalizing the learned pattern along the first PC to the test data, achieving high classification performance.
FIGURE 6. Training, test data, and classification decisions from LDA and SVM of the 1-s subject-dependent classification of participants 2 (P2) and 10 (P10), plotted against the first and second principle components.
The aim of this study was to measure brain activity of participants with and without fear of heights when exposed to fearful stimuli presented in VR-HMD. Additionally, the study investigated the feasibility to train a simple classifier to recognize a fear response from fNIRS signals recorded from participants with fear of heights. A successful combination of fNIRS measurement and VR-HMD can prove the ecological validity of its use in VRET.
The results from the between-group analysis of the fNIRS signals showed that the grand average contrast Δ[HbO] signals of the control group and the experimental group are significantly different in channel 3. No significant differences were found between the grand average contrast Δ[HbR] signals of the two groups. The evidence that only one out of 27 channels shows a significant difference between the two groups for only one chromophore suggests that the fNIRS signals of participants with fear of heights were not that different from those of participants without fear of heights in general.
However, it is difficult to make a direct comparison of our result with the literature due to the lack of including both experimental and control groups in previous studies on this topic. Despite this, it was discovered that Δ[HbO] measured in (some areas of) the PFC of recruited homogeneous participants increased during fearful conditions (Rosenbaum et al., 2020; Glotzbach et al., 2011; Zhang et al., 2017; Köchel et al., 2013; Roos et al., 2011; Ma et al., 2013; Landowska, 2018), which is in line with our results that the Δ[HbO] signal of the experimental group peaks higher than that of the control group when exposed to fearful stimuli (see Figure 3). In contrast, we found that Δ[HbR] of both groups were quite equal, which is partly in accordance with the evidence that the majority of similar works did not report any change of Δ[HbR] after the exposure to fearful stimuli (Roos et al., 2011; Ma et al., 2013; Zhang et al., 2017; Rosenbaum et al., 2020), but there are some exceptions (Glotzbach et al., 2011; Köchel et al., 2013; Landowska et al., 2018).
Nevertheless, it was also reported in the literature that Δ[HbO] values over the PFC can increase when the participants were experiencing other mental states, such as mental workload, mental stress, affective responses, attention, deception, preference, anticipation, suspicion, and frustration (Suzuki et al., 2008; Ayaz et al., 2012; Kreplin and Fairclough, 2013; Ding et al., 2014; Hirshfield et al., 20142014; Tupak et al., 2014; Arefi Shirvan et al., 2018; Numata et al., 2019). This indicates that increased Δ[HbO] values are not only an indication of fear responses but can also be driven by other psychological factors. This effect is less likely for the experimental group in our study, as they indicated that they were feeling afraid during the height exposure, which makes it improbable that they also experienced other mental states, considering that fear is presumably the most salient feeling they would perceive.
The result of the within-group analysis of the fNIRS signals shows that the grand average Δ[HbO] values are significantly higher during the height condition than during the ground condition. This significant difference was observed in a total of 13 channels, which are all located towards the frontal part of the PFC. These results indicate that during fear responses, the Δ[HbO] values increase significantly as compared to no-fear responses, which is in accordance with the vast majority of previous works on fNIRS measurements taken during fear responses (Glotzbach et al., 2011; Roos et al., 2011; Köchel et al., 2013; Ma et al., 2013; Zhang et al., 2017; Landowska, 2018; Rosenbaum et al., 2020).
Additionally, the results of the within-group analysis show that the grand average Δ[HbR] values of the height condition and the ground condition are significantly different in channel 23. Surprisingly, the grand average Δ[HbR] signal of the height condition is higher than that of the ground condition in this channel. This contradicts some findings from the literature, where decreased Δ[HbR] values are reported for fearful conditions (Glotzbach et al., 2011; Köchel et al., 2013; Landowska et al., 2018). It remains unclear why our results differ from the literature.
The clear distinction of fNIRS signals due to fear exposure in experimental group suggests the possibility to train a classifier to automatically detect fear responses using fNIRS.
The subject-dependent classification results suggest that the amount of history and the choice between the LDA or the linear-SVM algorithm has minimal influence on the subject-dependent classification performance. The linear classifiers do not perform well for some participants due to the difference in data distribution between training and test data. A possible explanation is that the fear responses and accompanying fNIRS measurements of these participants were not stable over time.
Similarly, the choice of classification methods and the amount of history to take into account do not have enormous influence on the performance of subject-independent classifiers. While the overall accuracy is above 71%, classification for participants 8 and 11 achieved poor performance. Our investigation on the participants' AQ, SUDS, and IPQ scores indicated that these participants had a strong fear of heights, felt very anxious during the height trials, felt relaxed during the ground trials, and felt sufficiently present in the VEs. However, we learned from the data distribution analysis that the fNIRS measurements of these participants were not stable over time. This might explain the overlap of fear and no fear fNIRS data trials in the first two PCs of the feature space when taking all data from this participant as a test set (in leave-one-subject-out cross-validation), while the cause of the instability of data over time remains unclear.
It is remarkable that for most participants, the subject-independent classification outperforms the subject-dependent classification. While the training data from the first six trials have a different distribution than the last four trials used as a test set in subject-dependent classification, combining all trials might mitigate the discrepancy between those trials, converging to more common patterns of the other participants. This would explain the relatively good performance of the subject-independent classification. More research is needed to prove this hypothesis.
Overall, the average classification accuracy of the subject-dependent classification is approximately 70%, whereas the subject-independent classification has average accuracies around 74%. These accuracies are statistically significantly higher than random classification. It is noteworthy that the goal of this study is not to find the best classification model but to examine to what extent a simple linear classifier with minimal parameter tuning can discriminate between fear and no-fear responses. Future work on applying sophisticated algorithms could improve the classification performance. It should also be noted that our channel selection used in classification was based solely on signal quality and not influenced by feature correlation.
The accuracies in our study are comparable to those in previous works attempting to classify fear from no-fear responses in VR using physiological signals. Despite achieving slightly higher accuracies from 76% to 89.5% compared to our research, previous work recruited a lower number of participants [seven participants in Handouzi et al. (20132013) using blood volume pulse (BVP) data, eight participants in Bălan et al. (2020) using galvanic skin response (GSR), heart rate, and EEG data]. Among similar studies that recruited a higher number of participants, the study by Šalkevicius et al. (2019) detected public speaking anxiety using BVP, GSR, and skin temperature data from 30 participant and achieved 80.1% accuracy in leave-one-subject-out cross-validation. However, this work did not include brain signals in the study. In contrast, another study (Hu et al., 2018) detecting fear of heights response in VR-HMD from EEG signal achieved 88.77%, but the results were based on 10-fold cross-validation, where the generalizability to classify unseen participant remains unknown.
In general, our classification performance has demonstrated the feasibility to detect a fear of height response from brain signals of a previously unseen participant as a 1-s rate (the size of our sliding window is 1 s). As we encourage other researchers to test other classification paradigms, the physiological data reproducing the results in this study are publicly available.
It is noteworthy that the fNIRS signals comprise multiple components where some of them are potentially confounders that are not task-related. Our method is based on contrasting an experimental condition with a baseline condition, which can subtract out spurious hemodynamic/oxygenation responses from the experimental task (Tachtsidis and Scholkmann, 2016). Thus, it should reduce hemodynamic influences from the extracerebral layer from the fNIRS signals. Alternative approaches can be further incorporated to remove systemic confounders.
Neither the fNIRS headset nor the VR-HMD was originally designed for simultaneous usage of both devices. The incompatibility caused an uncomfortable feeling for many participants. The VR-HMD needed tightening up with a headband around the participant's head. This put an extra pressure on the optodes of the fNIRS headset, which can be unpleasant for some participants, negatively influencing the user experience of the system. Since it is difficult to quantify the effect of the uncomfortable feeling caused by the hardware components, it remains unknown to what extent this affected the participants and the consequent results.
Although the fNIRS technology is less susceptible to motion artifacts and electrical noise, it was often reported in the literature that motion artifacts still occur (Naseer and Hong, 2015; Wilcox and Biondi, 2015; Pinti et al., 2020). Therefore, the participants in our study were instructed to look around very slowly in the VEs and to limit their bodily movements, which might reduce realism of the experience of the VEs for some participants. Still, the motion artifacts were present in our study.
Future research should consider adding more trials per condition and prolonging the duration of each trial. More data are needed to train sophisticated classification models. Prolonged duration opens the possibility to include heart rate variability (HRV) as a feature for the classifier; HRV can be captured from the embedded cardiac cycles in the fNIRS signals and was found to be a useful measure to detect fear (Wiederhold et al., 2002; Peterson et al., 2018). Also, it is worthy to investigate the difference among high-arousal-negative-valence responses, such as mental stress, frustration, and fear; disentanglement of such responses can potentially improve the detection of fear of height.
In this research, we measure distress and the feeling of presence by using established scales to allow the comparison with previous research in fear and VRET. While SUDS has been widely used in the context of fear exposure treatment due to its high comprehensiveness, conciseness, and validity in psychological studies, future works should also consider using recently developed measures that are correlated highly with the SUDS to confirm the validity of the measured fear by the classical SUDS. These include the scale of anxiety (Spielberger, 1972; Masia-Warner et al., 2003), discomfort (Kaplan et al., 1995), disturbance (Harris et al., 2002; Kim et al., 2008), or distress (McCullough, 2002). Similarly, although IPQ was found as the most reliable questionnaire to measure the presence in VR environment (Schwind et al., 2019) among classical measures (Witmer and Singer, 1998; Slater and Steed, 2000; Usoh et al., 2000), the alternative recent questionnaires should also be considered (Grassini and Laumann, 2020).
The statistical analyses of the fNIRS data and the classification performances are merely based on the mean Δ[HbO] and mean Δ[HbR] values. However, it is known from previous fNIRS studies investigating mental states that alternative features such as amplitude, slope, standard deviation, kurtosis, skewness, and signal peaks can provide insights and be used as discriminative features for classifying mental states (Khan and Hong, 2015; Zhang et al., 2016; Aghajani et al., 2017; Parent et al., 2019). In our study, the grand average Δ[HbO] traces revealed that the traces of the experimental group generally rise to a peak value, whereas this pattern is less apparent for the grand average traces of the control group (see Figure 3). A similar observation can be made for the grand average Δ[HbO] traces of the height condition and ground condition of the experimental group (see Figure 4). Based on these observations, it is anticipated that alternative features such as the maximum signal value, the time to peak, and the signal slope have the potential to improve the classification results or enhance the fNIRS difference between groups.
The results answer our first research question by demonstrating that there is significant difference in fNIRS signals between participants with a fear of heights and participants without it when exposed to fear conditions in a VE. Specifically, the contrast between the ground-condition and height-condition fNIRS signals in the experimental group was larger than that in the control group, despite limited statistical significance. The effect of the condition was more salient when focusing only on the experimental group that exhibited significant differences in the grand average Δ[HbO] values during fear responses and during no-fear responses. The effect was dominant in the optode area close to the frontal part of the PFC. To answer our research question regarding to what extent a machine learning model can be successfully trained to recognize fear of heights response using fNIRS, we trained different simple classifiers in a subject-dependent and subject-independent framework and found that subject-dependent classification encountered the issue of subjective variability. Nevertheless, the subject-independent classification results show the potential for usage in online fear of height detection, and the average accuracy in classifying unseen data from a previously unseen participant is above 74.00%. Our study therefore confirmed the ecological validity of combining fNIRS measurement and VR-HMD, which may pave a way toward effective VRET.
The original contributions presented in the study are publicly available. This data can be found here: [https://doi.org/10.4121/17302865].
The studies involving human participants were reviewed and approved by Ethics Committee of the Faculty of Electrical Engineering, Mathematics and Computer Science, University of Twente (reference number: RP 2020-76). The patients/participants provided their written informed consent to participate in this study.
LdW, NT, and MP conceived, planned, designed the experiment. LdW provided a critical review, carried out the experiment, data acquisition, analysis, processing interpretation, and wrote the full research report. NT wrote the manuscript with input from all the authors. MP supervised the research, provided critical feedback, and proofread the manuscript. All authors discussed the results and contributed to the final manuscript.
This work was partially supported by the European Regional Development Fund's operationeel programma oost (OP-OOST EFRO PROJ-00900) and by the Netherlands Organization for Scientific Research (NWA Startimpuls 400.17.602).
The authors declare that the research was conducted in the absence of any commercial or financial relationships that could be construed as a potential conflict of interest.
All claims expressed in this article are solely those of the authors and do not necessarily represent those of their affiliated organizations, or those of the publisher, the editors and the reviewers. Any product that may be evaluated in this article, or claim that may be made by its manufacturer, is not guaranteed or endorsed by the publisher.
The authors gratefully acknowledge Tenzing Dolmans, faculty members, and staff at the Faculty of Behavioural, Management and Social sciences, University of Twente (UT) for providing lab space and materials required for the experiments and facilitating the study. The authors also thank the participants for their time and effort in participating in the experiment. The authors also express gratitude to Dirk Heylen at Human-Media Interaction group, UT, for advice and discussion.
1https://www.oculus.com/rift-s/
3https://www.artinis.com/brite
4https://www.artinis.com/oxysoft
Aghajani, H., Garbey, M., and Omurtag, A. (2017). Measuring Mental Workload with EEG+fNIRS. Front. Hum. Neurosci. 11. doi:10.3389/fnhum.2017.00359
Aksoy, E., Izzetoglu, K., Baysoy, E., Agrali, A., Kitapcioglu, D., and Onaral, B. (2019). Performance Monitoring via Functional Near Infrared Spectroscopy for Virtual Reality Based Basic Life Support Training. Front. Neurosci. 13. doi:10.3389/fnins.2019.01336
Antony, M. M. (2001). Measures for Specific Phobia. Boston, MA: Springer US, 133–158. doi:10.1007/0-306-47628-2_12
Arefi Shirvan, R., Setarehdan, S. K., and Motie Nasrabadi, A. (2018). Classification of Mental Stress Levels by Analyzing fNIRS Signal Using Linear and Non-linear Features. Int. Clin. Neurosci. J. 5, 55–61. doi:10.15171/icnj.2018.11
Ayaz, H., Shewokis, P. A., Bunce, S., Izzetoglu, K., Willems, B., and Onaral, B. (2012). Optical Brain Monitoring for Operator Training and Mental Workload Assessment. NeuroImage 59, 36–47. Neuroergonomics: The human brain in action and at work. doi:10.1016/j.neuroimage.2011.06.023
Bălan, O., Moise, G., Moldoveanu, A., Leordeanu, M., and Moldoveanu, F. (2020). An Investigation of Various Machine and Deep Learning Techniques Applied in Automatic Fear Level Detection and Acrophobia Virtual Therapy. Sensors 20, 496. doi:10.3390/s20020496
Benjamin, C. L., O'Neil, K. A., Crawley, S. A., Beidas, R. S., Coles, M., and Kendall, P. C. (2010). Patterns and Predictors of Subjective Units of Distress in Anxious Youth. Behav. Cogn. Psychother. 38, 497–504. doi:10.1017/S1352465810000287
Boeldt, D., McMahon, E., McFaul, M., and Greenleaf, W. (2019). Using Virtual Reality Exposure Therapy to Enhance Treatment of Anxiety Disorders: Identifying Areas of Clinical Adoption and Potential Obstacles. Front. Psychiatry 10. doi:10.3389/fpsyt.2019.00773
Brinkman, W.-P., Sandino, G., and Mast, C. (2009). “Field Observations of Therapists Conducting Virtual Reality Exposure Treatment for the Fear of Flying,” in VTT Symposium (Valtion Teknillinen Tutkimuskeskus).
Chen, L.-C. (2016). Cortical Plasticity in Cochlear Implant Users. Ph.D. thesis. doi:10.13140/RG.2.2.33251.76324
Cohen, D. C. (1977). Comparison of Self-Report and Overt-Behavioral Procedures for Assessing Acrophobia. Behav. Ther. 8, 17–23. doi:10.1016/S0005-7894(77)80116-0
Cui, X., Bray, S., and Reiss, A. L. (2010). Functional Near Infrared Spectroscopy (NIRS) Signal Improvement Based on Negative Correlation between Oxygenated and Deoxygenated Hemoglobin Dynamics. NeuroImage 49, 3039–3046. doi:10.1016/j.neuroimage.2009.11.050
Delpy, D. T., Cope, M., Zee, P. v. d., Arridge, S., Wray, S., and Wyatt, J. (1988). Estimation of Optical Pathlength through Tissue from Direct Time of Flight Measurement. Phys. Med. Biol. 33, 1433–1442. doi:10.1088/0031-9155/33/12/008
Derosière, G., Dalhoumi, S., Perrey, S., Dray, G., and Ward, T. (2014). Towards a Near Infrared Spectroscopy-Based Estimation of Operator Attentional State. PLOS ONE 9, e92045. doi:10.1371/journal.pone.0092045
Difede, J., and Hoffman, H. G. (2002). Virtual Reality Exposure Therapy for World Trade center post-traumatic Stress Disorder: A Case Report. CyberPsychology Behav. 5, 529–535. doi:10.1089/109493102321018169
Ding, X. P., Sai, L., Fu, G., Liu, J., and Lee, K. (2014). Neural Correlates of Second-Order Verbal Deception: A Functional Near-Infrared Spectroscopy (fNIRS) Study. NeuroImage 87, 505–514. doi:10.1016/j.neuroimage.2013.10.023
Dong, D., Wong, L. K. F., and Luo, Z. (2018). Assess Ba10 Activity in Slide-Based and Immersive Virtual Reality Prospective Memory Task Using Functional Near-Infrared Spectroscopy (fNIRS). Appl. Neuropsychol. Adult 26, 465–471. doi:10.1080/23279095.2018.1443104
Dong, D., Wong, L. K. F., and Luo, Z. (2017). Assessment of Prospective Memory Using fNIRS in Immersive Virtual Reality Environment. Jbbs 07, 247–258. doi:10.4236/jbbs.2017.76018
Donker, T., Van Esveld, S., Fischer, N., and Van Straten, A. (2018). 0Phobia - towards a Virtual Cure for Acrophobia: Study Protocol for a Randomized Controlled Trial. Trials 19. doi:10.1186/s13063-018-2704-6
Emmelkamp, P. M. G., Bruynzeel, M., Drost, L., and van der Mast, C. A. P. G. (2001). Virtual Reality Treatment in Acrophobia: A Comparison with Exposure In Vivo. CyberPsychology Behav. 4, 335–339. doi:10.1089/109493101300210222
Etkin, A., and Wager, T. D. (2007). Functional Neuroimaging of Anxiety: A Meta-Analysis of Emotional Processing in Ptsd, Social Anxiety Disorder, and Specific Phobia. Ajp 164, 1476–1488. doi:10.1176/appi.ajp.2007.07030504
Fishburn, F. A., Ludlum, R. S., Vaidya, C. J., and Medvedev, A. V. (2019). Temporal Derivative Distribution Repair (Tddr): A Motion Correction Method for fNIRS. NeuroImage 184, 171–179. doi:10.1016/j.neuroimage.2018.09.025
Freeman, D., Haselton, P., Freeman, J., Spanlang, B., Kishore, S., Albery, E., et al. (2018). Automated Psychological Therapy Using Immersive Virtual Reality for Treatment of Fear of Heights: a Single-Blind, Parallel-Group, Randomised Controlled Trial. The Lancet Psychiatry 5, 625–632. doi:10.1016/S2215-0366(18)30226-8
Garcia-Palacios, A., Hoffman, H., Carlin, A., Furness, T. A., and Botella, C. (2002). Virtual Reality in the Treatment of Spider Phobia: a Controlled Study. Behav. Res. Ther. 40, 983–993. doi:10.1016/S0005-7967(01)00068-7
Genovese, C. R., Lazar, N. A., and Nichols, T. (2002). Thresholding of Statistical Maps in Functional Neuroimaging Using the False Discovery Rate. NeuroImage 15, 870–878. doi:10.1006/nimg.2001.1037
Gerardi, M., Rothbaum, B. O., Ressler, K., Heekin, M., and Rizzo, A. (2008). Virtual Reality Exposure Therapy Using a Virtual iraq: Case Report. J. Traum. Stress 21, 209–213. doi:10.1002/jts.20331
Glotzbach, E., Mühlberger, A., Gschwendtner, K., Fallgatter, A. J., Pauli, P., and Herrmann, M. J. (2011). Prefrontal Brain Activation during Emotional Processing: A Functional Near Infrared Spectroscopy Study (fNIRS). Open Neuroimag J. 5, 33–39. doi:10.2174/1874440001105010033
Grassini, S., and Laumann, K. (2020). Questionnaire Measures and Physiological Correlates of Presence: A Systematic Review. Front. Psychol. 11, 349. doi:10.3389/fpsyg.2020.00349
Gromer, D., Madeira, O., Gast, P., Nehfischer, M., Jost, M., Müller, M., et al. (2018). Height Simulation in a Virtual Reality Cave System: Validity of Fear Responses and Effects of an Immersion Manipulation. Front. Hum. Neurosci. 12. doi:10.3389/fnhum.2018.00372
Handouzi, W., Maaoui, C., Pruski, A., Moussaoui, A., and Bendiouis, Y. (20132013). Short-term Anxiety Recognition Induced by Virtual Reality Exposure for Phobic People. IEEE Int. Conf. Syst. Man, Cybernetics, 3145–3150. doi:10.1109/smc.2013.536
Harris, S. R., Kemmerling, R. L., and North, M. M. (2002). Brief Virtual Reality Therapy for Public Speaking Anxiety. Cyberpsychol Behav. 5, 543–550. doi:10.1089/109493102321018187
Hill, A. P., and Bohil, C. J. (2016). Applications of Optical Neuroimaging in Usability Research. Ergon. Des. 24, 4–9. doi:10.1177/1064804616629309
Hirshfield, L. M., Bobko, P., Barelka, A., Hirshfield, S. H., Farrington, M. T., Gulbronson, S., et al. (20142014). Using Noninvasive Brain Measurement to Explore the Psychological Effects of Computer Malfunctions on Users during Human-Computer Interactions. Adv. Human-Computer Interaction 2014, 1–13. doi:10.1155/2014/101038
Hocke, L., Oni, I., Duszynski, C., Corrigan, A., FrederickFrederick, B., and Dunn, J. (2018). Automated Processing of fNIRS Data-A Visual Guide to the Pitfalls and Consequences. Algorithms 11, 67–92. doi:10.3390/a11050067
Hofmann, S. (2008). Cognitive Processes during Fear Acquisition and Extinction in Animals and Humans: Implications for Exposure Therapy of Anxiety Disorders. Clin. Psychol. Rev. 28, 199–210. doi:10.1016/j.cpr.2007.04.009
Hu, F., Wang, H., Chen, J., and Gong, J. (2018). “Research on the Characteristics of Acrophobia in Virtual Altitude Environment,” in 2018 IEEE International Conference on Intelligence and Safety for Robotics (ISR), 238–243. doi:10.1109/iisr.2018.8535774
Hu, X.-S., Hong, K.-S., and Ge, S. S. (2012). fNIRS-Based Online Deception Decoding. J. Neural Eng. 9, 026012. doi:10.1088/1741-2560/9/2/026012
Hudak, J., Blume, F., Dresler, T., Haeussinger, F. B., Renner, T. J., Fallgatter, A. J., et al. (2017). Near-infrared Spectroscopy-Based Frontal Lobe Neurofeedback Integrated in Virtual Reality Modulates Brain and Behavior in Highly Impulsive Adults. Front. Hum. Neurosci. 11. doi:10.3389/fnhum.2017.00425
Jerdan, S. W., Grindle, M., van Woerden, H. C., and Kamel Boulos, M. N. (2018). Head-mounted Virtual Reality and Mental Health: Critical Review of Current Research. JMIR Serious Games 6, e14. doi:10.2196/games.9226
Kaplan, D. M., Smith, T., and Coons, J. (1995). A Validity Study of the Subjective Unit of Discomfort (SUD) Score. Measurement and Evaluation in Counseling and Development 27.
Khan, M. J., and Hong, K.-S. (2015). Passive Bci Based on Drowsiness Detection: An fNIRS Study. Biomed. Opt. Express 6, 4063–4078. doi:10.1364/BOE.6.004063
Khan, M. N. A., Bhutta, M. R., and Hong, K.-S. (2020). Task-specific Stimulation Duration for fNIRS Brain-Computer Interface. IEEE Access 8, 89093–89105. doi:10.1109/access.2020.2993620
Kim, D., Bae, H., and Chon Park, Y. (2008). Validity of the Subjective Units of Disturbance Scale in EMDR. J. EMDR Prac Res. 2, 57–62. doi:10.1891/1933-3196.2.1.57
Kim, G., Buntain, N., Hirshfield, L., Costa, M. R., and Chock, T. M. (2019). Processing Racial Stereotypes in Virtual Reality: An Exploratory Study Using Functional Near-Infrared Spectroscopy (fNIRS). Springer Cham, 407–417. doi:10.1007/978-3-030-22419-6_29
Köchel, A., Schöngassner, F., and Schienle, A. (2013). Cortical Activation during Auditory Elicitation of Fear and Disgust: A Near-Infrared Spectroscopy (NIRS) Study. Neurosci. Lett. 549, 197–200. doi:10.1016/j.neulet.2013.06.062
Kreplin, U., and Fairclough, S. H. (2013). Activation of the Rostromedial Prefrontal Cortex during the Experience of Positive Emotion in the Context of Esthetic Experience. An fNIRS Study. Front. Hum. Neurosci. 7. doi:10.3389/fnhum.2013.00879
Lachert, P., Janusek, D., Pulawski, P., Liebert, A., Milej, D., and Blinowska, K. J. (2017). Coupling of Oxy- and Deoxyhemoglobin Concentrations with EEG Rhythms during Motor Task. Sci. Rep. 7, 15414. doi:10.1038/s41598-017-15770-2
Landowska, A. (2018). “Measuring Prefrontal Cortex Response to Virtual Reality Exposure Therapy,”. Ph.D. thesis (Salford, United Kingdom: University of Salford).Freely Moving Participants.
Landowska, A., Roberts, D., Eachus, P., and Barrett, A. (2018). Within- and Between-Session Prefrontal Cortex Response to Virtual Reality Exposure Therapy for Acrophobia. Front. Hum. Neurosci. 12. doi:10.3389/fnhum.2018.00362
Lange, K., Williams, L. M., Young, A. W., Bullmore, E. T., Brammer, M. J., Williams, S. C. R., et al. (2003). Task Instructions Modulate Neural Responses to Fearful Facial Expressions. Biol. Psychiatry 53, 226–232. doi:10.1016/S0006-3223(02)01455-5
Lindner, P., Miloff, A., Bergman, C., Andersson, G., Hamilton, W., and Carlbring, P. (2020). Gamified, Automated Virtual Reality Exposure Therapy for Fear of Spiders: A Single-Subject Trial under Simulated Real-World Conditions. Front. Psychiatry 11, 116. doi:10.3389/fpsyt.2020.00116
Ma, Q., Huang, Y., and Wang, L. (2013). Left Prefrontal Activity Reflects the Ability of Vicarious Fear Learning: A Functional Near-Infrared Spectroscopy Study. Scientific World J. 2013, 1–8. doi:10.1155/2013/652542
Maltby, N., Kirsch, I., Mayers, M., and Allen, G. J. (2002). Virtual Reality Exposure Therapy for the Treatment of Fear of Flying: a Controlled Investigation. J. Consulting Clin. Psychol. 70, 1112–1118. doi:10.1037//0022-006x.70.5.111210.1037/0022-006x.70.5.1112
Martens, M. A., Antley, A., Freeman, D., Slater, M., Harrison, P. J., and Tunbridge, E. M. (2019). It Feels Real: Physiological Responses to a Stressful Virtual Reality Environment and its Impact on Working Memory. J. Psychopharmacol. 33, 1264–1273. doi:10.1177/0269881119860156
Masia-Warner, C., Storch, E. A., Pincus, D. B., Klein, R. G., Heimberg, R. G., and Liebowitz, M. R. (2003). The Liebowitz Social Anxiety Scale for Children and Adolescents: An Initial Psychometric Investigation. J. Am. Acad. Child Adolesc. Psychiatry 42, 1076–1084. doi:10.1097/01.CHI.0000070249.24125.89
McCullough, L. (2002). Exploring Change Mechanisms in EMDR Applied to "Small-T Trauma" in Short-Term Dynamic Psychotherapy: Research Questions and Speculations. J. Clin. Psychol. 58, 1531–1544. doi:10.1002/jclp.10103
Miloff, A., Lindner, P., Dafgård, P., Deak, S., Garke, M., Hamilton, W., et al. (2019). Automated Virtual Reality Exposure Therapy for Spider Phobia vs. In-Vivo One-Session Treatment: A Randomized Non-inferiority Trial. Behav. Res. Ther. 118, 130–140. doi:10.1016/j.brat.2019.04.004
Naseer, N., and Hong, K.-S. (2015). fNIRS-Based Brain-Computer Interfaces: a Review. Front. Hum. Neurosci. 9, 3. doi:10.3389/fnhum.2015.00003
Nomura, M., Ohira, H., Haneda, K., Iidaka, T., Sadato, N., Okada, T., et al. (2004). Functional Association of the Amygdala and Ventral Prefrontal Cortex during Cognitive Evaluation of Facial Expressions Primed by Masked Angry Faces: an Event-Related Fmri Study. NeuroImage 21, 352–363. doi:10.1016/j.neuroimage.2003.09.021
Numata, T., Kiguchi, M., and Sato, H. (2019). Multiple-time-scale Analysis of Attention as Revealed by EEG, NIRS, and Pupil Diameter Signals during a Free Recall Task: A Multimodal Measurement Approach. Front. Neurosci. 13. doi:10.3389/fnins.2019.01307
Parent, M., Peysakhovich, V., Mandrick, K., Tremblay, S., and Causse, M. (2019). The Diagnosticity of Psychophysiological Signatures: Can We Disentangle Mental Workload from Acute Stress with ECG and fNIRS? Int. J. Psychophysiology 146, 139–147. doi:10.1016/j.ijpsycho.2019.09.005
Peterson, S. M., Furuichi, E., and Ferris, D. P. (2018). Effects of Virtual Reality High Heights Exposure during Beam-Walking on Physiological Stress and Cognitive Loading. PLOS ONE 13, e0200306. doi:10.1371/journal.pone.0200306
Pinti, P., Scholkmann, F., Hamilton, A., Burgess, P., and Tachtsidis, I. (2019). Current Status and Issues Regarding Pre-processing of fNIRS Neuroimaging Data: An Investigation of Diverse Signal Filtering Methods within a General Linear Model Framework. Front. Hum. Neurosci. 12, 505. doi:10.3389/fnhum.2018.00505
Pinti, P., Tachtsidis, I., Hamilton, A., Hirsch, J., Aichelburg, C., Gilbert, S., et al. (2020). The Present and Future Use of Functional Near‐infrared Spectroscopy (fNIRS) for Cognitive Neuroscience. Ann. N.Y. Acad. Sci. 1464, 5–29. doi:10.1111/nyas.13948
Price, R. B., Eldreth, D. A., and Mohlman, J. (2011). Deficient Prefrontal Attentional Control in Late-Life Generalized Anxiety Disorder: an Fmri Investigation. Transl Psychiatry 1, e46. doi:10.1038/tp.2011.46
Riva, G., Mantovani, F., Capideville, C. S., Preziosa, A., Morganti, F., Villani, D., et al. (2007). Affective Interactions Using Virtual Reality: The Link between Presence and Emotions. CyberPsychology Behav. 10, 45–56. doi:10.1089/cpb.2006.9993
Rizzo, A., John, B., Newman, B., Williams, J., Hartholt, A., Lethin, C., et al. (2013). Virtual Reality as a Tool for Delivering Ptsd Exposure Therapy and Stress Resilience Training. Mil. Behav. Health 1, 52–58. doi:10.1080/21635781.2012.721064
Rodríguez, A., Rey, B., Clemente, M., Wrzesien, M., and Alcañiz, M. (2015). Assessing Brain Activations Associated with Emotional Regulation during Virtual Reality Mood Induction Procedures. Expert Syst. Appl. 42, 1699–1709. doi:10.1016/j.eswa.2014.10.006
Roos, A., Robertson, F., Lochner, C., Vythilingum, B., and Stein, D. J. (2011). Altered Prefrontal Cortical Function during Processing of Fear-Relevant Stimuli in Pregnancy. Behav. Brain Res. 222, 200–205. doi:10.1016/j.bbr.2011.03.055
Rosenbaum, D., Leehr, E. J., Rubel, J., Maier, M. J., Pagliaro, V., Deutsch, K., et al. (2020). Cortical Oxygenation during Exposure Therapy - In Situ fNIRS Measurements in Arachnophobia. NeuroImage: Clin. 26, 102219. doi:10.1016/j.nicl.2020.102219
Rothbaum, B. O., Anderson, P., Zimand, E., Hodges, L., Lang, D., and Wilson, J. (2006). Virtual Reality Exposure Therapy and Standard (In Vivo) Exposure Therapy in the Treatment of Fear of Flying. Behav. Ther. 37, 80–90. doi:10.1016/j.beth.2005.04.004
Rothbaum, B. O., Hodges, L. F., Ready, D., Graap, K., and Alarcon, R. D. (2001). Virtual Reality Exposure Therapy for Vietnam Veterans with Posttraumatic Stress Disorder. J. Clin. Psychiatry 62, 617–622. doi:10.4088/JCP.v62n0808
Rothbaum, B. O., Hodges, L., Smith, S., Lee, J. H., and Price, L. (2000). A Controlled Study of Virtual Reality Exposure Therapy for the Fear of Flying. J. Consulting Clin. Psychol. 68, 1020–1026. doi:10.1037/0022-006X.68.6.1020
Rothbaum, B. O., Price, M., Jovanovic, T., Norrholm, S. D., Gerardi, M., Dunlop, B., et al. (2014). A Randomized, Double-Blind Evaluation Ofd-Cycloserine or Alprazolam Combined with Virtual Reality Exposure Therapy for Posttraumatic Stress Disorder in Iraq and Afghanistan War Veterans. Ajp 171, 640–648. doi:10.1176/appi.ajp.2014.13121625
Šalkevicius, J., Damaševičius, R., Maskeliunas, R., and Laukienė, I. (2019). Anxiety Level Recognition for Virtual Reality Therapy System Using Physiological Signals. Electronics 8, 1039. doi:10.3390/electronics8091039
Scholkmann, F., Kleiser, S., Metz, A. J., Zimmermann, R., Mata Pavia, J., Wolf, U., et al. (2014). A Review on Continuous Wave Functional Near-Infrared Spectroscopy and Imaging Instrumentation and Methodology. NeuroImage 85, 6–27. doi:10.1016/j.neuroimage.2013.05.004
Schubert, T., Friedmann, F., and Regenbrecht, H. (2001). The Experience of Presence: Factor Analytic Insights. Presence: Teleoperators & Virtual Environments 10, 266–281. doi:10.1162/105474601300343603
Schwind, V., Knierim, P., Haas, N., and Henze, N. (2019). Using Presence Questionnaires in Virtual Reality, 1–12. New York, NY, USA: Association for Computing Machinery.
Seraglia, B., Gamberini, L., Priftis, K., Scatturin, P., Martinelli, M., and Cutini, S. (2011). An Exploratory fNIRS Study with Immersive Virtual Reality: a New Method for Technical Implementation. Front. Hum. Neurosci. 5. doi:10.3389/fnhum.2011.00176
Shin, L. M., and Liberzon, I. (2010). The Neurocircuitry of Fear, Stress, and Anxiety Disorders. Neuropsychopharmacol 35, 169–191. doi:10.1038/npp.2009.83
Slater, M., and Steed, A. (2000). A Virtual Presence Counter. Presence: Teleoperators & Virtual Environments 9, 413–434. doi:10.1162/105474600566925
Suzuki, M., Miyai, I., Ono, T., and Kubota, K. (2008). Activities in the Frontal Cortex and Gait Performance Are Modulated by Preparation. An fNIRS Study. NeuroImage 39, 600–607. doi:10.1016/j.neuroimage.2007.08.044
Tachtsidis, I., and Scholkmann, F. (2016). False Positives and False Negatives in Functional Near-Infrared Spectroscopy: Issues, Challenges, and the Way Forward. Neurophoton 3, 031405. doi:10.1117/1.NPh.3.3.031405
Teo, W.-P., Muthalib, M., Yamin, S., Hendy, A. M., Bramstedt, K., Kotsopoulos, E., et al. (2016). Does a Combination of Virtual Reality, Neuromodulation and Neuroimaging Provide a Comprehensive Platform for Neurorehabilitation? - A Narrative Review of the Literature. Front. Hum. Neurosci. 10. doi:10.3389/fnhum.2016.00284
Tupak, S. V., Dresler, T., Guhn, A., Ehlis, A.-C., Fallgatter, A. J., Pauli, P., et al. (2014). Implicit Emotion Regulation in the Presence of Threat: Neural and Autonomic Correlates. NeuroImage 85, 372–379. doi:10.1016/j.neuroimage.2013.09.066
Usoh, M., Catena, E., Arman, S., and Slater, M. (2000). Using Presence Questionnaires in Reality. Presence: Teleoperators & Virtual Environments 9, 497–503. doi:10.1162/105474600566989
Wald, J., and Taylor, S. (2000). Efficacy of Virtual Reality Exposure Therapy to Treat Driving Phobia: a Case Report. J. Behav. Ther. Exp. Psychiatry 31, 249–257. doi:10.1016/S0005-7916(01)00009-X
Wiederhold, B. K., Jang, D. P., Kim, S. I., and Wiederhold, M. D. (2002). Physiological Monitoring as an Objective Tool in Virtual Reality Therapy. CyberPsychology Behav. 5, 77–82. doi:10.1089/109493102753685908
Wilcox, T., and Biondi, M. (2015). fNIRS in the Developmental Sciences. Wires Cogn. Sci. 6, 263–283. doi:10.1002/wcs.1343
Wilson, E. B. (1927). Probable Inference, the Law of Succession, and Statistical Inference. J. Am. Stat. Assoc. 22, 209–212. doi:10.1080/01621459.1927.10502953
Witmer, B. G., and Singer, M. J. (1998). Measuring Presence in Virtual Environments: A Presence Questionnaire. Presence 7, 225–240. doi:10.1162/105474698565686
Zhang, D., Zhou, Y., Hou, X., Cui, Y., and Zhou, C. (2017). Discrimination of Emotional Prosodies in Human Neonates: A Pilot fNIRS Study. Neurosci. Lett. 658, 62–66. doi:10.1016/j.neulet.2017.08.047
Zhang, Z., Jiao, X., Jiang, J., Pan, J., Cao, Y., Yang, H., et al. (2016). “Passive Bci Based on Sustained Attention Detection: An fNIRS Study,” in Advances in Brain Inspired Cognitive Systems. Editors C.-L. Liu, A. Hussain, B. Luo, K. C. Tan, Y. Zeng, and Z. Zhang (Springer International Publishing), 220–227. doi:10.1007/978-3-319-49685-6_20
Keywords: virtual reality exposure therapy, fNIRS, head-mounted display, fear of heights, classification, brain–computer interface
Citation: de With LA, Thammasan N and Poel M (2022) Detecting Fear of Heights Response to a Virtual Reality Environment Using Functional Near-Infrared Spectroscopy. Front. Comput. Sci. 3:652550. doi: 10.3389/fcomp.2021.652550
Received: 12 January 2021; Accepted: 25 November 2021;
Published: 17 January 2022.
Edited by:
Aleksander Väljamäe, Tallinn University, EstoniaReviewed by:
Felix Putze, University of Bremen, GermanyCopyright © 2022 de With, Thammasan and Poel. This is an open-access article distributed under the terms of the Creative Commons Attribution License (CC BY). The use, distribution or reproduction in other forums is permitted, provided the original author(s) and the copyright owner(s) are credited and that the original publication in this journal is cited, in accordance with accepted academic practice. No use, distribution or reproduction is permitted which does not comply with these terms.
*Correspondence: Nattapong Thammasan, bi50aGFtbWFzYW5AdXR3ZW50ZS5ubA==
Disclaimer: All claims expressed in this article are solely those of the authors and do not necessarily represent those of their affiliated organizations, or those of the publisher, the editors and the reviewers. Any product that may be evaluated in this article or claim that may be made by its manufacturer is not guaranteed or endorsed by the publisher.
Research integrity at Frontiers
Learn more about the work of our research integrity team to safeguard the quality of each article we publish.