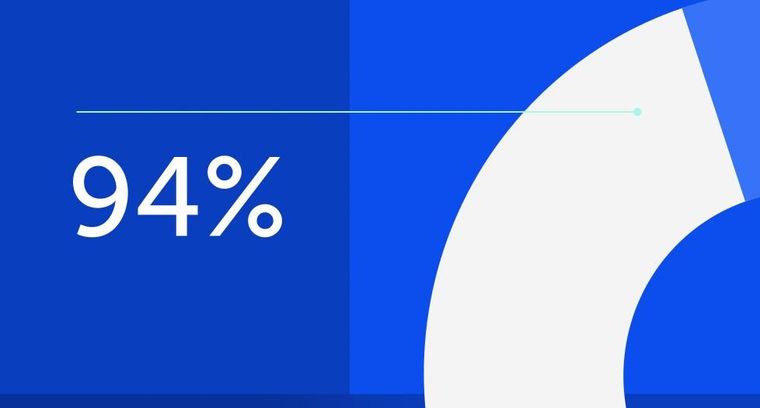
94% of researchers rate our articles as excellent or good
Learn more about the work of our research integrity team to safeguard the quality of each article we publish.
Find out more
EDITORIAL article
Front. Comput. Sci., 24 February 2021
Sec. Digital Public Health
Volume 2 - 2020 | https://doi.org/10.3389/fcomp.2020.636996
This article is part of the Research TopicCreating Evidence from Real World Patient Digital DataView all 14 articles
Editorial on the Research Topic
Creating Evidence from Real World Patient Digital Data
Over the last 5 years there has been a tremendous rise in interest in personalized medicine and patient-centred healthcare. This has resulted in an expanding focus on the value of single-case research methods (also known as N-of-1 or Single-Case Designs (SCDs)). SCDs use repeated measures, frequent data collection and patient-reported outcome measures to draw conclusions about an individual.
We use “SCD” as a broad term that includes all SCD sub-designs, encompassing Single-Case Experimental Designs (SCEDs), N-of-1 randomised controlled trials (N-of-1 trials) and Single-Case Observational Designs (SCODs)—all with specific features (see Box 1).
BOX 1What are Single-Case Designs?
Single-Case Designs (SCDs) gather and interpret repeated measures data from a single participant over time.
What are Single-Case Experimental Designs?
Single-Case Experimental Designs (SCEDs) are experimental designs that test the effect of an intervention on one participant, using repeated measurements, sequential (±randomised) introduction of an intervention and method-specific data analysis, including visual and statistical techniques. Simultaneous or sequential replications are possible with more individuals.
What are N-of-1 trials?
N-of-1 randomised controlled trials (RCTs) provide an opportunity to evaluate individual-person response to interventions, by randomly allocating different time periods within an individual to repeated intervention and control conditions and then comparing responses across these periods.
What are SCODs?
Single-Case Observational Designs (SCODs) involve the repeated measurement of an outcome (e.g., pain) in a person over time, but with no intervention implemented, in order to draw conclusions about naturally occurring patterns and predictors of outcomes over time.
SCDs can complement RCTs in a wide range of clinical research and practice contexts (Gabler et al., 2011; Smith, 2012; Punja et al., 2016; McDonald et al., 2017; Shaffer et al., 2018; Hekler et al., 2019). Since 2015, the number of SCD articles published yearly has rapidly increased. High profile articles have recently been featured in Nature (Schork, 2015) and JAMA (Stunneneberg et al., 2018). Guidelines have been published to improve SCD conduct and reporting quality, including CENT (N-of-1 trials) (Vohra et al., 2016), CENT for TCM (N-of-1 trials for traditional Chinese medicine) (Li et al., 2019), SCRIBE (SCDs in behavioral sciences) (Tate et al., 2017), and SPENT (N-of-1 protocol design) (Porcino et al., 2019).
N-of-1 randomised controlled trials (RCTs) provide an opportunity to evaluate individual-person response to interventions, by randomly allocating different time periods within an individual to repeated intervention and control conditions and then comparing responses across these periods.
N-of-1 observational studies involve the repeated measurement of an outcome (e.g., pain) in a person over time, but with no intervention implemented, in order to draw conclusions about naturally occurring patterns and predictors of outcomes over time.
Both N-of-1 RCTs and observational studies can have a ‘self-study’ design, where an individual conducts the study on themselves, to answer research questions they have generated themselves.
N-of-1 RCTs and observational studies provide individualized findings that can be aggregated to produce results equivalent to those found in traditional group-based RCTs and population-level epidemiological studies, respectively, but may require fewer people for the same statistical power.
Because of their patient-centricity, individualized results, and amenability for use by doctors to tailor therapies to individuals, SCDs are ideally placed to complement, strengthen, and generate advances in precision medicine, patient-centred healthcare, personalized health and digital health (Schork, 2015; Hekler et al., 2020).
Digital health is an exploding field, with over 1,000 relevant studies registered on clinicaltrials.gov in November 2020. Digital health includes digital therapeutics (i.e., actual interventions implemented on a digital device), patient-reported outcomes (e.g., survey responses administered via phone or web app), mobile health tools, such as wearable devices (e.g., worn sensors, implants) that can be used to monitor various aspects of an individual’s health in SCDs, along with telehealth, electronic health record systems, and data analytics tools. Digital health data can potentially be monitored continuously during the SCD, and used to help tailor a treatment to the needs and preferences of each patient. Collecting data digitally can be very convenient for participants, especially if they do not have to actively record data. SCDs can be of longer duration than group-based RCTs, so they are well suited to data collection using digital health devices and related technologies.
SCDs can be ‘self-study’ designs, wherein an individual conducts the study on themselves, to answer research questions important to them. In addition, both use techniques such as meta-analysis to aggregate individual-level findings. This can produce results equivalent to those found in traditional group-based RCTs and population-level epidemiological studies, but may require fewer people to obtain the same statistical power as a larger, group-based study. It is common for a series of SCDs to be conducted, and the results to be pooled to address issues of, e.g., generalisability and population response rate.
To acknowledge the emerging field of digital N-of-1 and SCD research, a team of experts recently completed editing a Frontiers Research Topic entitled ‘Creating Evidence from Real World Patient Digital Data’. This Frontiers Research Topic covered digital health applications, delivery, and analysis of SCDs (including self-studies) in any health discipline. It focused on mobile health (mHealth), smart phone applications (apps), wearable devices, sensors and implants, real-time tracking, data analytics, patient experience of digital health and mobile health, patients as collaborators in personalized medicine, and self-tracking efforts in the “citizen science” community.
The articles covered a selection of original research, methodology pieces, opinion pieces, and study protocols, discussing important themes including the significance of technology, emergence of the “self-scientist”, and the value of using diverse N-of-1 and SCD designs. The 13 articles written by 60 authors have already generated over 37,000 views to January 2021, reflecting the strong interest in these methods globally. The topic has had viewers from all over the world, particularly from the United States, United Kingdom, Germany, France, and China.
A key feature of several articles was use of N-of-1 studies enabled by mobile app technology. For example, Bobe et al. discussed the potential for clinicians and patients to collaboratively use an app-based platform for N-of-1 trials, and reported results of a survey exploring perceptions about implementing an app-based N-of-1 trial platform to support data-driven decisions around insomnia treatment. Kravitz et al. reported on feasibility, acceptability, and influence of mHealth-supported N-of-1 trials for enhanced cognitive and emotional well-being in US volunteers. Bauer et al. described a feasibility study protocol for testing individual-level effects of tamsulosin using the PERSONAL app to track daily urinary symptoms and medication side effects. And Golden et al. detailed a protocol for self-directed, mobile-app-based N-of-1 studies to test the effects of caffeine and l-theanine on cognitive performance.
The use of emerging technology in SCDs is not limited to mobile apps. Chrisinger outlined the opportunities to use GPS technology to create geolocated N-of-1 datasets that could be used to explore relationships between individuals, their environment, and their health, or what has been termed “the quantified self-in-place”. Chrisinger argues that individual-level information in real-world environmental contexts might lead to a better understanding of how treatments and interventions work, for whom, and under which conditions. A number of logistical, methodological, and ethical challenges were identified.
Many articles discussed the concept of the “self-scientist” or “personal science”, which has been enabled by availability of diverse and accessible digital tools to collect personal real-world data. Wolf and de Groot outlined a 5-stage conceptual framework to guide research and education into practice of “personal science”, which they define as using empirical methods to address personal health questions. Important similarities and differences between personal science, citizen science, and single subject (N-of-1/SCD) research were also discussed.
Schwartz et al. discussed the concept of the “digital twin”, where individuals have access to self-generated biobehavioural information derived from data collected from various sensors and devices that may reflect their biological and environmental circumstances, and be used to make predictions about their health. Advances in technology have led to more accurate capture of various biometric, behavioral, emotional, cognitive, and psychological aspects of daily life. Data-driven feedback from their “digital twin” information may inspire users to conduct self-experiments to evaluate their own treatment responses.
Nebeker et al. described the patient perspective of using self-study and peer-to-peer support in conjunction with traditional clinical support guided by external evidence generated from group-based studies. They argue that access to digital health technologies, wearable sensors, affordable lab screenings, etc. may contribute to a paradigm shift wherein “sick” care may become authentic “health” care.
The articles provided in this Research Topic also covered different SCD types. McMillan and Dixon used a series of digital SCODs to characterize self-regulatory processes, motivation to conserve resources, and activity levels in people with chronic pain. They found that motivational and self-regulatory processes during goal pursuit goal may play a key role in an intervention’ success. Similarly, Altman et al. used a series of digital SCODs to characterize processes and mechanisms of change over a course of psychotherapy.
Hendrickson et al. presented findings from statistical simulation studies they conducted to optimize aggregated N-of-1 trial designs for predictive biomarker validation. They described a set of simulation studies comparing the power of four different trial designs to detect relationship between a predictive biomarker (measured at baseline) and subjects' specific responses to the pharmacotherapeutic agent prazosin for Post Traumatic Stress Disorder.
Finally, Munson et al. argued that elicitation of individualized goals and customization of tracking to support those goals are a critical part of conducting N-of-1 studies. Their conclusions serve as an important reminder about the flexibility of these methods and their ability to tailor to preferences and needs of individuals through patient-centred N-of-1 designs.
The great variety of articles illustrates the versatility of this design and the opportunity to use digital methods to collect real world health data. We look forward to seeing the impact of this research on digital health and personalized medicine worldwide.
JN drafted the editorial and revised it after feedback from the other authors. SM, EH, EJD and NJS provided important input and critical review of the manuscript.
JN and SM have a commercial interest in N-of-1 Hub Pty Ltd. consultancy company. Author EJD is employed by the company Evidation Health.
The remaining authors declare that the research was conducted in the absence of any commercial or financial relationships that could be construed as a potential conflict of interest.
A similar article has been published as a blog on the website of The International Collaborative Network (ICN) for N-of-1 Clinical Trials and Single-Case Designs (SCDs). JN and SM are co-chairs of this network.
Gabler, N. B., Duan, N., Vohra, S., and Kravitz, R. L. (2011). N-of-1 trials in the medical literature: a systematic review. Med. Care 49 (8), 761–768. doi:10.1097/MLR.0b013e318215d90d
Hekler, E., Tiro, J. A., Hunter, C. M., and Nebeker, C. (2020). Precision health: the role of the social and behavioral sciences in advancing the vision. Ann. Behav. Med. 54 (Issue 11), 805–826. doi:10.1093/abm/kaaa018
Hekler, E. B., Klasnja, P., Chevance, G., Golaszewski, N. M., Lewis, D., and Sim, I. (2019). Why we need a small data paradigm. BMC Med. 17, 133. doi:10.1186/s12916-019-1366-x
Li, J., Hu, J.-y., Zhai, J.-b., Niu, J.-q., Kwong, J. S. W., Ge, L., et al. CENT for TCM Working Group. (2019). CONSORT extension for reporting N-of-1 trials for traditional Chinese medicine (CENT for TCM): recommendations, explanation and elaboration. Compl. Ther. Med. 46, 180–188. doi:10.1016/j.ctim.2019.08.014
McDonald, S., Quinn, F., Vieira, R., O'Brien, N., White, M., Johnston, D. W., et al. (2017). The state of the art and future opportunities for using longitudinal n-of-1 methods in health behaviour research: a systematic literature overview. Health Psychol. Rev. 11 (4), 307–323. doi:10.1080/17437199.2017.1316672
Porcino, A. J., Shamseer, L., Chan, A. W., Kravitz, R. L., Orkin, A., Punja, S., et al. SPENT group (2020). SPIRIT extension and elaboration for n-of-1 trials: SPENT 2019 checklist. BMJ 368, m122. doi:10.1136/bmj.m122
Punja, S., Bukutu, C., Shamseer, L., Sampson, M., Hartling, L., Urichuk, L., et al. (2016). The design, analysis and meta-analysis of N-of-1 trials: a tapestry of heterogeneity. J. Clin. Epidemiol. 76, 47. doi:10.1016/j.jclinepi.2016.03.023
Schork, N. J. (2015). Personalized medicine: time for one-person trials. Nature 520, 609. doi:10.1038/520609a
Shaffer, J. A., Kronish, I. M., Falzon, L., Cheung, Y. K., and Davidson, K. W. (2018). N-of-1 randomized intervention trials in health psychology: a systematic review and methodology critique. Ann. Behav. Med. 52 (9), 731–742. doi:10.1093/abm/kax026
Smith, J. D. (2012). Single-case experimental designs: a systematic review of published research and current standards. Psychol. Methods 17 (4), 510–550. doi:10.1037/a0029312
Stunnenberg, B. C., Raaphorst, J., Groenewoud, H. M., Statland, J. M., Griggs, R. C., Woertman, W., et al. (2018). Effect of mexiletine on muscle stiffness in patients with nondystrophic myotonia evaluated using aggregated N-of-1 trials. J. Am. Med. Assoc. 320 (22), 2344–2353. doi:10.1001/jama.2018.18020
Tate, R. L., Perdices, M., Rosenkoetter, U., Shadish, W., Vohra, S., Barlow, D. H., et al. (2017). The single-case reporting guideline in BEhavioural interventions (SCRIBE) 2016 statement. Neuropsychol. Rehabil. 27 (1), 1–15. doi:10.1080/09602011.2016.1190533
Keywords: N-of-1 clinical trials, single case experimental designs, digital health, real world data, single case designs
Citation: Nikles J, Daza EJ, McDonald S, Hekler E and Schork NJ (2021) Editorial: Creating Evidence From Real World Patient Digital Data. Front. Comput. Sci. 2:636996. doi: 10.3389/fcomp.2020.636996
Received: 02 December 2020; Accepted: 23 December 2020;
Published: 24 February 2021.
Edited and Reviewed by:
Nilufar Baghaei, Massey University, New ZealandCopyright © 2021 Nikles, Daza, McDonald, Hekler and Schork. This is an open-access article distributed under the terms of the Creative Commons Attribution License (CC BY). The use, distribution or reproduction in other forums is permitted, provided the original author(s) and the copyright owner(s) are credited and that the original publication in this journal is cited, in accordance with accepted academic practice. No use, distribution or reproduction is permitted which does not comply with these terms.
*Correspondence: Jane Nikles, dXFqbmlrbGVAdXEuZWR1LmF1
Disclaimer: All claims expressed in this article are solely those of the authors and do not necessarily represent those of their affiliated organizations, or those of the publisher, the editors and the reviewers. Any product that may be evaluated in this article or claim that may be made by its manufacturer is not guaranteed or endorsed by the publisher.
Research integrity at Frontiers
Learn more about the work of our research integrity team to safeguard the quality of each article we publish.