- Institute for Hazard Mitigation Planning and Research, University of Washington, Seattle, WA, United States
A major challenge in the communication of spatial hazard information is the existence of substantial variation in people's ability to correctly infer the appropriate conclusions from hazard maps. Examination of the results from a variety of different types of hazard maps has identified patterns of erroneous as well as accurate processing of map information. In response, most hazard map studies have focused on the erroneous inferences and sought to develop displays that would cause hazard map viewers to make more accurate inferences about the hazard. This research has made some significant advances, but it has neglected the development and testing of spatial displays that can help people to make more timely and effective protective action decisions. This article concludes by summarizing the findings from hazard map research and recommending future research that will address these unresolved issues.
Introduction
A hazard map is a common device for providing people with information about the environmental hazards to which they are exposed. Hazard maps differ in the temporal contexts that they address—chronic hazards that will happen sometime in the future vs. acute hazards that are imminent threats. Maps of chronic hazards might be used by specialists such as land use planners, structural engineers, and emergency managers to identify geographic areas that can expect an event of a specified probability (e.g., the area inundated by a 100-year flood) or of a specific magnitude (e.g., the area expected to be affected by a Category 5 hurricane storm surge). They can use these maps to guide community hazard mitigation and emergency preparedness actions that are implemented long before an acute threat arises. In addition, authorities can use these maps to promote household emergency management actions.
Maps of acute hazards can be used by authorities to identify protective action recommendations (PARs) for the risk area population. That is, these maps can be used to guide decisions about who is at risk, what protective action the risk area population should take, and when they should implement those protective actions (Cova et al., 2017). For example, coastal emergency managers use the hazard maps in HURREVAC to guide their decisions about PARs when their jurisdictions are threatened by approaching hurricanes. In turn, authorities can use acute hazard maps to communicate hazard warnings that promote appropriate household protective actions. The objective of these warnings is not only to increase compliance with PARs, but also to decrease shadow evacuation so those who are not at risk do not impede the evacuation of those who are at risk (Yin et al., 2016; Lindell et al., 2019a).
When constructing hazard maps, one might think that hazard analysts only need to draw lines in the right places on a base map and give those maps to risk area populations in order to increase their risk perceptions and, thus, their emergency preparedness for chronic threats and protective actions for acute threats. Unfortunately, that is not the case because studies dating back at least to Handmer (1980) have documented people's misunderstanding of hazard maps. Consequently, risk communicators ranging from warning meteorologists to local emergency managers need to understand the limits of people's comprehension of different types of hazard maps.
At the outset, it is important to understand that hazard maps are one of three means of communicating hazard information—spatial, numeric, and textual. Moreover, the effects of this hazard information can be understood in terms of the Protective Action Decision Model (Lindell and Perry, 2004, 2012; Lindell, 2018), which posits that a hazard map is a form of warning message that is primarily directed toward the warning receiver's perceptions of the threat. Once a hazard map elicits a high threat perception, the receiver is motivated to search for additional information (if the information source is not highly credible, the threat is ambiguous, or the logistics of response are uncertain) and to initiate protective action. Thus, map elements that promote these processes will be more effective in eliciting appropriate protective actions. The following sections will elaborate on these ideas by discussing indicators of map comprehension, cognitive processes in map comprehension, and cognitive abilities affecting map comprehension. The article will then review of research on people's comprehension of, and behavioral response to, different types of hazard maps, and conclude with a summary of research findings and recommendations for future research.
Psychological Processes in Map Utilization
Indicators of Map Comprehension
Map comprehension can be defined as a map viewer's ability to draw the inferences that the map constructor intended. There are only a few studies of map comprehension that have examined the basic elements of map reading skills (Muir, 1985; Milson and Alibrandi, 2008; Aksoy, 2013; Albert et al., 2016). Specifically, (1) symbol recognition involves accurate interpretation of map symbols such as those located in the map legend, (2) direction finding is the determination of geographical directions among landmarks using a map compass, and (3) scale use involves determination of actual geographical distances among landmarks using a map scale. More sophisticated maps, such as topographical maps, require (4) contour interpretation, which is the determination of quantities such as land elevations from the location of points within contours. MacPherson-Krutsky et al. (2020) found differences between basic map skills (compass and scale utilization) and advanced map skills (contour interpretation) even though both require a degree of knowledge of mapping conventions and visuospatial skills. Specifically, these researchers found that experiment participants had significantly higher scores on basic map skills than on advanced map skills and the two scales had only a modest correlation (r = 0.23).
Cognitive Processes in Map Comprehension
Accurate interpretation of a spatial display requires viewers to see the display clearly, pay attention to relevant display features, develop “cognitive maps” (mental representations of the essential elements of a print or electronic map), and make inferences from their cognitive maps to produce judgments, make decisions, and choose actions (Hegarty, 2011). The ability to see visual display elements clearly is affected by aspects of the map display such as the size of those elements and their number (i.e., the degree of display clutter). However, the ability to see visual display elements is also affected by personal factors such as the viewers' visual acuity and color blindness.
Attention is influenced by “bottom-up” processes, in which visually salient features such as bright colors capture viewers' attention. It is also influenced by “top-down” processes in which viewers' expectations direct their attention to specific display elements. These expectations are determined by a viewer's goals, which can be externally imposed (e.g., an experimenter-assigned task to reproduce the map) or self-generated (e.g., viewers' intentions of determining if their homes are in hazard zones). Viewers' expectations about hazard maps are directed by schemas, also known as mental models, which are generic belief structures about entities, their attributes, and the interrelationships among those attributes (Endsley and Jones, 2012). People can have schemas of varying comprehensiveness about maps in general and about the specific map content being displayed in particular. Accordingly, people can range in knowledge from novices (who have rudimentary schemas) to experts (who have comprehensive schemas) in each of these domains (Ooms et al., 2014). Related to the concept of a schema is a heuristic, or simple rule, for processing situations (Tversky and Kahneman, 1974). Although heuristics were originally conceived as suboptimal rules for decision making, subsequent research has found that they are often “fast and frugal” ways to make decisions (Gigerenzer and Goldstein, 1996). Indeed, Keller et al. (2010) identified a number of heuristics that define rules for information search, information search termination, and decision making.
People's cognitive maps are affected by their training and experience (Eley, 1993). However, there appears to be little research on the effects of these topics on map comprehension. Indeed, instruction in map interpretation is inconsistent, even in geography textbooks (Gillen et al., 2010). A more thoroughly studied determinant of map comprehension is the viewer's spatial ability which, following Colom et al. (2002), can be defined as the ability to generate, retain, retrieve, and transform visual images. Unlike research on cognitive processes, which tends to focus on commonalities in the way that all viewers typically process map information, research on cognitive abilities tends to focus on individual differences among map viewers in the ways that they process information.
Multiple studies have found that individuals who have higher levels of spatial ability are better at interpreting and applying map information (Postigo and Pozo, 2004; Hegarty et al., 2010; Aksoy, 2013; Ooms et al., 2015; MacPherson-Krutsky et al., 2020). Moreover, a number of studies report that gender influences map comprehension and spatial ability, but the evidence is mixed. For example, Lindell et al. (2016) and Jon et al. (2019), but not Jon et al. (2018), found that females judged tornado polygons as having significantly higher strike probabilities than males. More generally, many studies of cognitive abilities find significant gender differences, with females tending to have stronger verbal ability and males tending to have stronger spatial ability (Halpern, 1986; Voyer et al., 1995; Lawton and Morrin, 1999; Montello et al., 1999; Halpern and Collaer, 2005). However, other reviews find weaker evidence for gender differences. Hyde and Linn (1988) conclude that gender differences in verbal ability are large on only a few measures; Coluccia and Louse (2004) conclude that gender differences are small in at least one aspect of spatial ability, spatial orientation, especially when using a map. Some recent studies attribute gender differences in spatial performance to corresponding differences in strategy use (Bosco et al., 2004; Picucci et al., 2011) and gender roles (Reilly et al., 2016), both of which can be eliminated by training. Nonetheless, there is no evidence to date that gender differences in visuospatial abilities are decreasing (Halpern and Collaer, 2005).
There is also consistent evidence that performance in a variety of scientific disciplines is correlated with object-scale spatial ability (Hegarty et al., 2010; Hegarty, 2011; Yoon and Mann, 2017; Buckley et al., 2018). In particular, Hegarty et al. (2010) reported significant differences among members of different professions in their self-reported spatial and verbal abilities using the Santa Barbara Sense of Direction Scale (SBSOD, a measure of environmental-scale spatial ability), the Philadelphia Spatial Ability Scale (PSA, a measure of object-scale spatial ability), and the Philadelphia Verbal Ability Scale (PVA, a measure of verbal ability). On the SBSOD, respondents from the physical sciences, biological sciences, geosciences, geography, and engineering scored above the mean; whereas psychology, social sciences, and humanities scored at or below the mean. On the PSA, the pattern was generally the same, although biological sciences scored below the mean. On the PVA, the pattern was the reverse of that for the SBSOD.
Hazard maps
Hazard Map Types
A map is an iconic spatial display, but spatial displays can also be classified relational or hybrid displays (Hegarty, 2011). An iconic display represents spatial objects. A road map is an example of an iconic display that represents a network of roads and the locations of landmarks in a geographical area. A relational display represents non-spatial variables, such as a graph of a hurricane's Saffir-Simpson category plotted over time. A hybrid display combines an iconic display, such a base map, with a relational display to provide a spatial representation of non-spatial categories or quantities, as when 100 and 500-year flood zones are represented by map contours (Allen et al., 2006).
In the literature on risk communication and hazard warnings, there is much more extensive research on verbal elements of warnings than on graphic displays or numeric information (Lindell, 2018). Mileti and Sorensen (1990) summarized research on (primarily verbal) warnings as indicating that the most important message elements are the identity of the hazard, expected time and location of impact, protective action guidance, and identity of the warning source. Hazard maps inherently address the identity of the hazard and the location of impact, and often address the identity of the map source. However, they inherently provide an allocentric perspective of the environment, so viewers must infer their risk by being able to locate their positions on the map. By contrast, verbal warnings provide an allocentric perspective of the environment if broadcast messages only describe the risk area in terms of large-scale features (e.g., which counties are at risk of a tornado). However, they can provide an egocentric perspective of the environment if they personalize the risk (e.g., a public safety officer delivering a face-to-face warning). Unfortunately, face-to-face warnings have substantial resource requirements although more advanced technologies such as Reverse 911 can achieve personalization with lower labor demands (Lindell and Prater, 2010; Lindell et al., 2019a, Chapter 3).
In addition to the type of graphic information that makes a hazard map a hybrid display, hazard maps can contain supplementary information that is numeric (e.g., hurricane forward speed, size, and magnitude/intensity) or textual (e.g., National Hurricane Center's—NHC's—Hurricane Watch and Hurricane Warning). For example, information about tsunamis could include how they are generated, what are their environmental cues, how soon the first wave of a local tsunami could arrive after earthquake shaking stops, how long waves might continue to arrive, and how tsunami wave impact would affect different types of structures (Lindell et al., 2019b). Additional information might include the types of warning sources and channels available in a given community, as well as the identity of sources of additional information and assistance.
One can generate a four-fold typology of hazard maps by drawing two distinctions—whether they have non-interactive or interactive displays and whether they depict a static (chronic/potential) or dynamic (imminent/actual) hazard. Non-interactive maps, whether print or electronic, are defined by a base map and one or more predetermined features that are simultaneously overlaid onto it. By contrast, interactive maps allow the user to select which features are individually or simultaneously overlaid onto the base map. Interactive maps provide additional information while avoiding clutter that is likely to overload the viewer of a static map, although this raises the challenge of users knowing how to navigate the interactivity (Cao et al., 2017; MacPherson-Krutsky et al., 2020).
Static maps depict hazards that are, essentially, constant over time. That is, they provide information about chronic, or potential, hazards. One of the most common examples is a map of a 100-year flood plain, but maps of hurricane risk areas and tsunami evacuation zones are other examples. By contrast, dynamic hazard maps provide a base map but show how an event is changing over time (e.g., Sea Island Software www.hurrevac.com/tutorials.htm; Wu et al., 2015a). Dynamic maps, which are sometimes called animated maps (Cybulski, 2016), can vary in the degree to which the time dimension is discrete (e.g., the 6 h intervals of National Hurricane Center Forecast Advisories) or continuous (e.g., the animation displayed in first-person shooter games).
Static/Non-interactive Maps
Research on static/non-interactive maps, one of the oldest areas of research on hazard maps, has found flood maps to be no more effective than a “100-year flood” label (Bell and Tobin, 2007). This broad finding has been explored further using a variety of dependent variables such as viewer perceptions of risk, risk area accuracy, preferences for map features, misconceptions about visualizations, and effects of user characteristics on performance. One important conclusion is that people need to be able to orient themselves on the map, but studies have differed in their findings about the most effective base map for doing this. Some studies have reported user preference for aerial photographs and 3D maps with clearly labeled landmarks rather than conventional topographical contour maps (Haynes et al., 2007; Dransch et al., 2010; Nave et al., 2010; Cao et al., 2016). However, other studies have shown a preference for topographical contour maps and street maps (Van Kerkvoorde et al., 2018). In addition, isarithmic maps produce the better understanding of uncertainty about hazard exposure than gradational shaded or binned maps (Thompson et al., 2015; Mulder et al., 2017). Moreover, consistent with the broader literature on map comprehension, color coding scheme and probability coding (numerical vs. verbal) also influence participants' judgments (Thompson et al., 2015; Van Kerkvoorde et al., 2018). Furthermore, confusion can occur when aspects of the hazard map are poorly defined by having too many features or arbitrarily defined risk area borders (Zhang et al., 2004; Arlikatti et al., 2006). Hazard maps are easier to interpret if risk area borders are defined by well-recognized features such as rivers, roads, and jurisdictional boundaries than by topographical contours. However, some jurisdictions have defined their hurricane evacuation zones by postal codes because these are even easier to recognize (Wu et al., 2020).
Static/Interactive Maps
In a recent study of a multi-hazard map, MacPherson-Krutsky et al. (2020) found that both basic and advanced map comprehension scores had significant positive correlations with scores on objective test of spatial ability, but not self-report measures. Moreover, self-reported spatial ability had statistically significant, but only moderately strong, correlations with objective spatial ability tests. Interestingly, participants' self-assessments of their performance were significantly correlated with advanced map skill. That is, those who were better at this task were able to assess their performance and conclude that the task was easier. This metacognitive accuracy is the opposite of the Dunning-Kruger effect, in which less competent people are oblivious to their own ignorance (Dunning, 2011). A likely explanation is that map utilization tasks provide the viewer with relatively unambiguous immediate feedback about performance.
Dynamic/Non-interactive Maps
Most of the studies in this category addressed a special case of dynamic maps, which is a single “snapshot” of a dynamic hazard. In addition to varying information (verbal, numeric, or spatial) about the hazard across conditions, most experiments asked participants to judge a snapshot of the hazard (e.g., strike probability) at different locations. Since successive snapshots were presented as independent observations at the same time, they are not dynamic displays in exactly the same sense as the evolution of an event over successive time periods. However, they are addressed here as a special case of dynamic maps because experimenters used these snapshots to map viewers' perceptions of different aspects of a hazard's future behavior.
Wildfire Maps
Cheong et al. (2016) found little difference in viewers' performance among a simple line boundary, spectral color, color shading, black-and-white transparency, and pointillist texture representation when participants had 30 s to judge each wildfire probability map. However, under increasing time pressure, participants performed best when viewing a spectral color map and worst when viewing a text-based or simple line boundary map.
Cao et al. (2016) conducted an online survey of residents in wildfire-prone areas who viewed a hypothetical wildfire described either by text messages or maps, and then tested on their comprehension of the information, their risk perceptions, and their reactions to the mode of presentation. Additionally, the website recorded the time respondents took to interpret the information they viewed. The results showed that maps were more effective than text messages in improving comprehension, increasing risk perceptions, and producing more positive reactions.
Tornado Maps
There has been a significant amount of research on people's responses to tornado warning polygons since Lindell et al. (2013) reviewed the research on tornado warning response. This warning polygon research has yielded seven important findings (Nagele and Trainor, 2012; Sherman-Morris and Brown, 2012; Ash et al., 2014; Mason and Senkbeil, 2014; Casteel and Downing, 2015; Lindell et al., 2016; Jon et al., 2018, 2019). The first finding is the centroid effect—people's tendency to judge the polygon centroid as the location of highest risk (Sherman-Morris and Brown, 2012; Ash et al., 2014; Lindell et al., 2016; Jon et al., 2019). This effect is particularly strong in deterministic polygon-only displays where no contextual information is given about the location of the tornadic storm cell that is the basis for issuing the warning polygon. The second finding is a proximity effect—the degree to which ps judgments decline with increasing distance from the tornadic storm cell rather than distance from the polygon centroid. Providing information about the location of a tornadic storm cell by displaying a radar image leads viewers recognize that there is a great threat at the near edge of the polygon (Jon et al., 2018, 2019). However, this proximity effect reduces, but does not eliminate the centroid effect. That is, viewers fail to recognize that there is a much greater threat at the polygon's near edge than at the centroid.
The third finding is the absence of an edge effect, the degree to which there is a threshold effect in the decrease of strike probability (ps) judgments immediately outside the polygon's boundaries. Contrary to the NWS's intended threshold function, which is a strong edge effect, tornado polygon studies have found only a weak edge effect. That is, ps judgments are indeed lower just outside than just inside the polygon (Ash et al., 2014), but the decrease is only a slightly larger decrease than expected in an otherwise continuous function (Lindell et al., 2016; Miran et al., 2018; Jon et al., 2019). However, it appears that the edge effect might be stronger in the storm cell's direction of travel, that is, along the warning polygon's longitudinal axis, and that lengthening the polygon changes viewers' responses (Klockow-McClain et al., 2020).
The fourth finding is a transect effect, the degree to which ps judgments decline continuously with increasing distance from the polygon's longitudinal axis, even extending outside the warning polygon (Lindell et al., 2016; Jon et al., 2018, 2019). Moreover, Jon et al. (2018) found that a deterministic polygon+radar display and two gradient displays (one with and the other without a radar display) exhibit a transect effect inside the polygon, in which ps judgments decline with distance from the centerline along each transect). This internal transect effect was consistently supported on transect T2 (the transect through the centroid), consistently contradicted the hypothesis on T3 (the transect just inside the polygon's edge farthest from the storm cell), and was inconsistent on T1 (the transect just inside the polygon's edge nearest to the storm cell). Curiously, the results for the two deterministic polygon displays were identical to each other and the results for the two gradient polygon displays were also identical to each other. However, the results for the two types of displays were different from each other. The most likely explanation for this difference is that the lines in the gradient display accentuated the differences in risk between the grid cell on the centerline and the two cells adjacent to it but inside the polygon.
The fifth finding is there are mixed findings about a display effect—whether there are differences among the displays and whether the displays that indicate the location of the tornadic storm cell are superior to the deterministic polygon-only display. Klockow's (2013) comparison of deterministic polygons with probabilistic polygons (which varied in their color schemes) found no significant differences among them. Similarly, Casteel and Downing's (2015) comparison of a text-only alert, text+NWS warning polygon, a text+radar image; and a text+warning polygon+radar image reported that the additional information did not produce statistically significant enhancement of the warning effectiveness. Ash et al. (2014) reported a significant difference between the ps judgments of deterministic polygons and probabilistic polygons (gradient polygons using different colors or different shades of a single color) that produced weaker centroid and edge effects as compared to a deterministic polygon. Miran et al. (2016) reported better accuracy for displays that lacked radar images and that a four-color spectral display (red, orange, yellow, green), a four shade gray gradient display, and a four isopleth contour display were better than a four shade red gradient display—possibly because the polygons were superimposed over the storm cell. Finally, Klockow-McClain et al. (2020) found that, compared to the deterministic polygon, sequential and divergent color displays had significantly lower protective action expectations at low probabilities. Overall, the results from different displays are inconsistent, suggesting display effects are either very small or very complex. If they are small, it would not make a difference what type of polygon the NWS displays in its tornado warnings. If they are very complex, further research will be needed to explain them.
The sixth finding concerns the degree to which ps judgments and emotional reactions to tornado polygon displays are related to expected response actions. Indeed, ps judgments are negatively correlated with expectations of resuming normal activities and positively correlated with expectations of information seeking, sheltering, and evacuating (Ash et al., 2014; Lindell et al., 2016; Jon et al., 2018, 2019). However, Jon et al. (2019) found that viewers had no general tendency to prefer one of the protective actions anywhere inside the polygon, regardless of the type of display they viewed. One possible explanation for this finding is that the participants in this study had generally low levels of experience with tornadoes, so they failed to realize the implication of their ps judgments for the choice of protective action. Of course, behavioral expectations are not necessarily the same as actual behavior, but hurricane evacuation expectations have been found to be significantly correlated with people's actual evacuation behavior 2 years later (Kang et al., 2007). Moreover, a recent statistical meta-analysis found that the results from studies of people's responses to hypothetical hurricane scenarios have been quite similar to those from studies of people's responses to actual hurricanes (Huang et al., 2016). Thus, it is reasonable to continue experimental studies of viewers' responses to hypothetical tornado scenarios.
The seventh finding concerns the degree to which ps judgments and expected response actions are related to experiential and demographic variables. Tornado polygon studies conducted to date have reported that viewers with previous polygon experience are less likely to ignore warnings, as well as that females are less likely to ignore warnings and more likely to take protective actions. However, there is less compelling evidence regarding other experiential and demographic variables. Specifically, none of the correlations of experiential and demographic variables with ps judgments and expected response actions Jon et al. (2018, 2019) or Lindell et al. (2016) reported to be significant in one of these studies were also significant in either of the other two studies and none of the demographic variables were significant in Miran et al. (2020). These findings are consistent with studies concluding that demographic and experiential variables are inconsistent predictors of hurricane evacuation (Baker, 1991; Huang et al., 2016).
In summary, the findings from tornado polygon studies suggest that viewers use a proximity heuristic (Teigen, 2005) that generates a perceived risk gradient in which perceived risk decreases with distance from the hazard source (Lindell and Earle, 1983). When information about the location of a tornadic storm cell outside the polygon is unavailable, the polygon centroid is likely to be mistaken as the location of the hazard source. Thus, the warning polygon is interpreted as a single contour of constant ps, with other contours paralleling the polygon edges—much like a topographical contour that has the centroid as the location of maximum ps. Conversely, information about a tornadic storm cell diminishes the centroid effect by redirecting viewers' attention to the true hazard source at the hook echo in the storm cell. One important implication of these findings is that there is likely to be incomplete compliance with the NWS protective action recommendation (i.e., immediate shelter) in many parts of the tornado polygon. Also contrary to the NWS's recommendations, people are likely to take protective actions in areas beyond the tornado polygon edges. Thus, the findings from tornado polygon research are consistent with those from studies of other hazards that have revealed incomplete compliance and evacuation shadow (Lindell and Perry, 2004, 2012; Lindell, 2018).
Hurricane Maps
Unlike tornado studies, hurricane hazard map studies have not examined a centroid effect. Although the tornado polygon and the hurricane uncertainty cone both show potential future locations of the relevant storm feature—a tornadic hook echo or a hurricane center—a tornado is an ephemeral feature that might not materialize from a hook echo and, thus, whose location on the tracking map is inherently uncertain. By contrast, a hurricane center is a persistent feature whose location on the tracking map is readily identifiable. However, hurricane studies have generally found evidence of a proximity effect. Specifically, reference points that are located closer to the hurricane center generally have higher ratings of likely damage than reference points that are more distant (Boone et al., 2018; Liu L. et al., 2019). Ruginski et al. (2016) also found this result, but only for the track only and ensemble displays.
Hurricane studies have searched for an edge effect since Broad et al. (2007) concluded that many people misinterpret the NHC's uncertainty cone associated with a hurricane's forecast track as indicating that there is zero probability of the track falling outside the uncertainty cone. In fact, the NHC intends the uncertainty cone to provide a 67% confidence interval around the forecast track. However, none of the hurricane studies found evidence of a strong edge effect (judgments of ps = 0 or no damage outside the uncertainty cone) at the aggregate level. Ruginski et al. (2016) looked for evidence of edge effects at the individual level, but even those analyses found no evidence for a strong edge effect. Indeed, Boone et al. (2018) found that only 14% of their experiment participants displayed a strong edge effect outside the boundary but, even among these participants, this was accompanied by a very steep transect effect within the uncertainty cone. Another 12% of the participants displayed a weak edge effect and most of them exhibited a very steep transect effect within the uncertainty cone.
Like the tornado polygon studies, hurricane display studies have consistently found evidence of a transect effect. This effect is found whether the dependent variable is a judgment of ps in eight 45 degree sectors of a 360 circle (Cox et al., 2013; Wu et al., 2014) or likely damage to reference points distributed along a roughly 650 km/400 mi line ahead of the current hurricane center (Ruginski et al., 2016; Liu L. et al., 2017; Boone et al., 2018; Liu D. et al., 2019). Individual level analysis in Boone et al. (2018) showed that 58% of their experiment participants exhibited a simple transect effect.
Also like the tornado studies, hurricane studies have found mixed evidence of display effects. With respect to edge and transect effects, Wu et al. (2014) found no differences among track only, uncertainty cone only, and track+cone displays, whereas Cox et al. (2013) found slight differences between an uncertainty cone only and an ensemble display. Ruginski et al. (2016) found that displays using an uncertainty cone led some viewers to conclude that the storm was intensifying, which was replicated in Padilla et al. (2017) Experiment 1. Padilla et al. (2017) Experiments 2 and 3 found that an ensemble display had the perverse effect of eliciting larger damage estimates for a reference location that was farther away from the hurricane center (i.e., a reverse proximity effect) if that location was on one of the ensemble tracks. Liu L. et al. (2019) studied four ensemble displays that varied in hurricane track density (7, 15, or 63 tracks), with track annotations indicating storm size and intensity either present for the 15 track display in one condition and absent from it in another condition. The 63 track display correctly produced a shallower slope for the 48 h transect than for the 24 h transect, whereas the 15 and 7 track displays incorrectly produced parallel slopes for the two transects. Moreover, the 15 track annotated display produced shallower slopes for the distance-damage relationship than the corresponding unannotated display. Compared to the unannotated display, the annotated display yielded damage judgments that were lower at the point nearest the track distribution centerline and higher at the point farthest from the centerline. In addition, damage judgments increased with annotations indicating increasing size and intensity.
Unlike the tornado studies, none of the hurricane studies have examined the degree to which ps judgments or emotional reactions to hurricane displays are related to expected response actions or the degree to which ps judgments and expected response actions are related to demographic and experiential variables.
Dynamic/Interactive Maps
Wildfire Maps
In research on wildfires, Drews et al. (2015) found that, although Incident Commanders with greater expertise searched for less information before selecting a PAR, this did not affect the quality of those decisions. In addition, participants selected less information in the second phase of the experiment, selected more dynamic information than static information in both phases of the experiment, and were more likely to recommend evacuation—even in cases for which that was not the optimal PAR.
Cao et al. (2017) evaluated an interactive wildfire map comprising 23 elements that displayed information about current and forecast hazard conditions, fire containment status, personal location, and response guidance (PARs, closed roads, and evacuation shelters). Although participants' ratings of different display elements as highly important (M > 4.5 on a 5 point scale) were necessarily associated with high levels of agreement, ratings of different display elements as less important (M < 4.5) were associated with extremely low levels of agreement that they were important (i.e., a uniform distribution of ratings) rather than agreement that they were unimportant.
Hurricane Maps
In research on hurricanes, Meyer et al. (2013) found that experiment participants who viewed a forecast track reported greater evacuation preparation than those who saw an uncertainty cone—even among those located far from the predicted center path. Moreover, participants who had never experienced a hurricane were most likely to express worry about an approaching storm and fastest to undertake preparatory action.
Later, Wu et al. (2015a) conducted a process tracing analysis of participants' dynamic decisions based on hurricane tracking maps. Participants assuming the role of a county emergency manager began by reading a training manual, the Local Official's Guide to Hurricane Evacuation Decision Making, which is an updated version of guidance produced by the Texas Division of Emergency Management. After reading and being tested on the Official's Guide, participants tracked four different hurricanes, each of which had six hurricane forecast advisories. The participants considered a variety of different sources of information—textual, graphic, and numeric—when tracking hurricanes. As revealed by click counts and click durations, participants' information search strategies became more efficient over forecast advisories and with increased experience tracking the four hurricanes. These changes in the search patterns from the first to the fourth hurricane suggest that the presentation of abstract principles in the Official's Guide was not sufficient for them to learn how to track hurricanes efficiently but they were able to significantly improve their search efficiency with a modest amount (roughly an hour) of practice. Overall, these data indicate that information search patterns are complex and deserve greater attention in studies of dynamic decision tasks.
In the same experiment, Wu et al. (2015b) assessed participants' ps judgments and PARs during the four different hurricane scenarios. The results show that participants' ps judgments revealed significant changes in the transect effect over time. There was an increase in ps judgments for target cities (projected landfall locations) and a decrease in ps judgments for adjacent cities and remote cities as the hurricanes approached landfall. In addition, ps judgments were significantly correlated with PARs. Participants also chose more PARs as hurricanes approached landfall, especially for the counties to which the participants were assigned as emergency managers. Nonetheless, they failed to choose as many PARS as appropriate, especially evacuating areas at risk of hurricane impacts. Overall, the results suggest that participants were able to utilize the available hurricane information to make reasonable ps judgments but failed to make the appropriate inferences about the significance of those ps judgments.
In response to the findings of Wu et al. (2015b) and Huang et al. (2017) examined the perceived attributes, behavioral expectations, and expected implementation timing of 11 organizational emergency response actions for a hypothetical Category 4 hurricane. The perceived attributes of the hurricane response actions were characterized by two hazard-related attributes (effectiveness for person protection and property protection) and five resource-related attributes (financial costs, required knowledge/skill, required equipment, required time/effort, and required cooperation). This study's data explain the findings of untimely protective action decision making in Wu et al. (2015b) by revealing distinctly different patterns for the expected implementation of preparatory actions and evacuation recommendations. Participants used the hazard-related and resource-related attributes to differentiate among the response actions and the expected timing of implementation. Moreover, participants' behavioral expectations and expected implementation timing for the response actions were most strongly correlated with those actions' effectiveness for person protection. Finally, participants reported evacuation implementation times that were consistent with a phased evacuation strategy in which risk areas are evacuated in order of their proximity to the coast. However, the late initiation of evacuation in risk areas closest to the coast would have led to very late evacuation of risk areas farther inland.
Sherman-Morris and Del Valle-Martinez (2017) addressed track uncertainty by presenting participants with track+uncertainty cone displays that either maintained a constant bearing or changed bearing in the second day of a 3 day scenario. This scenario addressed meteorologists' concerns about a “windshield wiper” effect in which viewers over-react to a change in hurricane track with lower risk perceptions when the storm resumes its original bearing. Contrary to the hypothesized “windshield wiper” effect, experiment participants' ps judgments appeared to be based solely on the current day's information rather than being influenced by the fluctuations in the storm's heading.
Decision Aids For Dynamic/Interactive Maps
One obvious reason why the participants in the Wu et al. (2015b) study issued tardy PARs is that, even though the hurricane tracking displays provided information about where to initiate PARs, they lacked any graphical guides for deciding when to initiate them. As noted earlier, this issue was addressed by Cova et al. (2017), who suggested that emergency managers establish warning triggers to define the times that PARs should be initiated. Warning triggers address three primary questions: which locations or population segments are at risk, what is the best PAR for each location or population segment, and when should the PARs be initiated? The first question is fundamentally geographic and involves careful identification of population segments that are likely to be adversely affected (Sorensen et al., 1992; Hsu and Peeta, 2014). The second question involves selecting the best available PARs for different target groups (e.g., evacuate or shelter-in-place). The third question requires assessing the amount of time that target groups will need to complete their PARs before hazard impact (Sorensen, 1991; Lindell and Prater, 2007).
The first step, identification of locations at risk is illustrated in Figure 1, which shows a hurricane approaching a coastal jurisdiction. The figure's right-hand side has a small circle depicting the hurricane eye, which is surrounded by a larger circle representing the radius of Tropical Storm force wind. The hurricane track is pointed toward a coastal Risk Area, which is defined by the hurricane center's forecast point of landfall, the distance to either side which is defined by the radius of hurricane force wind that will cause wind and surge damage, and the inland distance that there will be wind and surge damage, which is defined by hurricane intensity. There are Caution Areas adjacent to the Risk Area along the coast because of the uncertainty about the storm track and storm size, as well as inland from the Risk Area because of the uncertainty about the storm intensity.
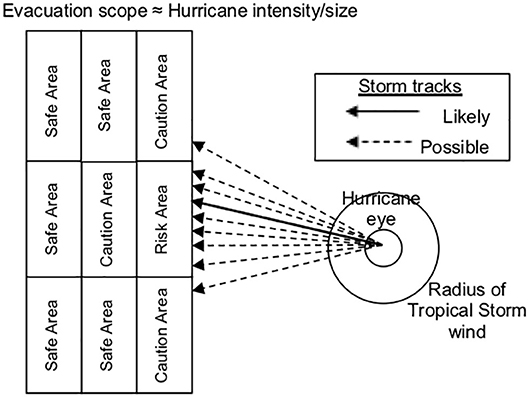
Figure 1. Hurricane protective action zones [adapted from from Lindell et al. (2019a), with permission].
The second step, identifying the appropriate PAR for each location, involves choosing among evacuation, shelter in-place, and allowing people to continue normal activities. Evacuation is expensive and disruptive so it should be recommended only when other actions provide insufficient protection. In turn, the level of protection depends upon three fundamental criteria—time, distance, and shielding. Evacuation addresses the first two criteria by reducing the amount of time that people are exposed to a hazard and it increases distance from the hazard source. By contrast, sheltering in-place provides shielding from a hazard by reducing the infiltration of hazardous materials into a structure (e.g., volcanic ash) or by resisting dangerous temperatures (e.g., wildfires) or pressures (e.g., tornadic wind). Evacuation is common for hurricanes striking the United States because this hazard provides sufficient forewarning to clear a risk area before hazard impact and few single family homes in coastal areas provide adequate protection from wind and surge. However, tornadoes provide insufficient time to evacuate safely, so authorities typically recommend shelter in-place (see Lindell et al., 2019a, Chapter 3, for further discussion).
The third step, determination of when to issue PARs, is based on the principle that protective actions should be completed before the arrival of hazardous conditions. As Figure 2 indicates, hurricane tracking involves an initial detection stage, after which the storm continues to be monitored until it makes landfall. As the storm progresses, meteorologists make projections about landfall location, wind speed, storm surge, and rainfall that, in turn, serve as decision information for emergency managers. These emergency managers use the decision information about expected storm impacts to establish the warning triggers that address which locations or population segments are at risk, what is the best PAR for each location or population segment, and when the PARs should be initiated.
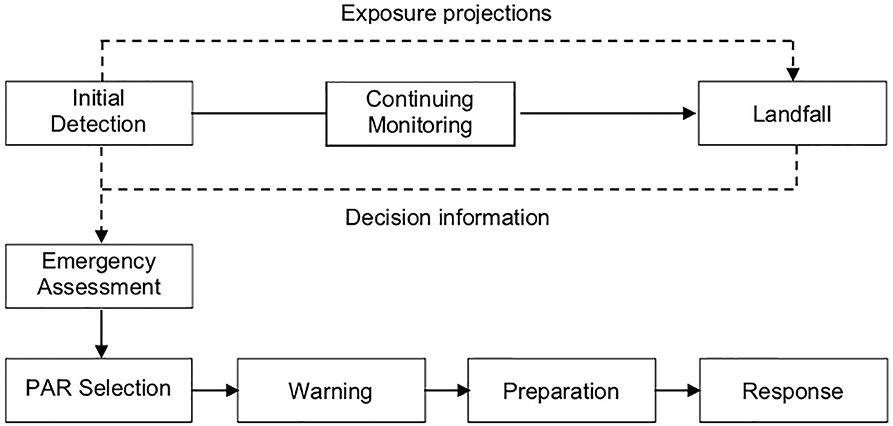
Figure 2. Chains of events for hurricane and community response [adapted from Lindell and Perry (1992), with permission].
A graphical guide for deciding when to initiate PARs can be constructed by recognizing that the time required for a resident or transient household to clear the risk area after incident initiation can be defined as a function of four Evacuation Time Estimate (ETE) components,
where tT is a household's total clearance time, td is the authorities' warning decision time, tw is the household's warning receipt time, tp is the household's evacuation preparation time, and te is the household's evacuation travel time (Lindell et al., 2019a, section 3.2). As Cova et al. (2017) noted, authorities who have a predefined trigger for initiating an evacuation can eliminate td, leaving household warning receipt time, household evacuation preparation time, and household evacuation travel time. Warning receipt time and evacuation preparation time are unique values for each household but can be aggregated into distributions that show the percentage of households over time who have received a warning or completed evacuation preparations. In turn, the ETE can be converted to an evacuation decision arc by recognizing that the critical distance of the hazard from a reference point (e.g., the nearest location of the threatened population) equals rate (the forward speed of the hurricane) multiplied by time (the ETE). The left side of Figure 3 shows the hurricane Risk Area from Figure 1 with three arcs. A conventional evacuation decision arc is defined by the most likely values of the ETE for that Risk Area and the current value of the hurricane's forward speed (the speed at which the hurricane center is moving over the water). However, both the Risk Area's ETE and the hurricane's forward speed are quantities whose uncertainty can be represented by a minimum probable radius, most probable radius, and maximum probable radius for the evacuation decision arc. Using this display, authorities can trigger an evacuation once the hazard reaches the evacuation decision arc (Lindell et al., 2019a, section 3.3.2). In the case of a hurricane, evacuation should begin when the radius of Tropical Storm force wind touches the evacuation decision arc that local authorities have selected. Tropical Storm force wind is a common evacuation trigger because this is the wind speed at which an evacuation could be stalled if high profile vehicles such as buses and recreational vehicles are overturned by high wind striking them from the side.
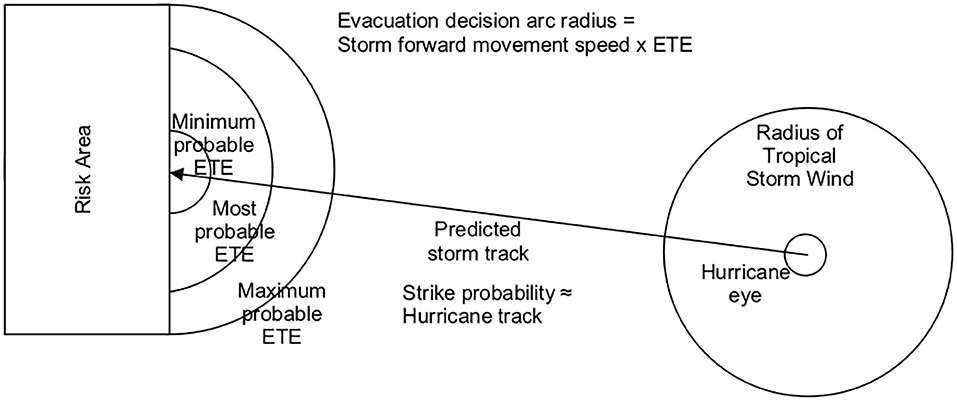
Figure 3. Evacuation decision arcs [reprinted from Lindell et al. (2019a), with permission].
Discussion
There is strong empirical support for some conclusions about people's comprehension of hazard maps. First, people's risk judgments can be characterized by a proximity heuristic (Teigen, 2005), which generates a perceived risk gradient that is a monotone decreasing function of distance from a hazard source (Lindell and Earle, 1983). However, there are differences among hazards regarding the application of this heuristic because there are corresponding differences among hazards regarding the location of the hazard source with respect to contour lines drawn on a hazard map. For wildfires, the hazard source is at the fire perimeter and the principal risk area is downwind of the burn area. For hurricanes, the hazard source is within the radius of hurricane wind at the apex of the uncertainty cone and the principal risk area is along the forecast storm track. Finally, the hazard source for tornadoes is located inside the warning polygon that defines the risk area. Consequently, tornado polygons produce a centroid effect that is not found for wildfires or hurricanes. By contrast, responses to the latter two types of hazard displays exhibit a transect effect, which is a special case of the proximity effect. Instead of proximity to the current location of the hazard source, the transect effect is an outcome of proximity to the future location of the hazard source.
The transect effect found in laboratory experiments is consistent with the results of survey research showing that the rate of sheltering in-place in response to a tornado warning was more strongly correlated with proximity to the forecast track than being in the warning polygon (Nagele and Trainor, 2012). Similarly, Miran et al. (2018) found an effect of proximity to the forecast track on protective action during the May 2013 Oklahoma City tornadoes. Finally, Krocak et al. (2020) found that the probability of a false-positive tornado warning perception as a function of distance from the nearest tornado warning followed an exponential decay function in which the probability declined from approximately p = 1.0 within the warning polygon to approximately p = 0.45 at 100 miles.
The transect effect is so strong that it substantially weakens the edge effect that the NWS intends for tornado polygons (which is different from the hurricane uncertainty cone that is only a 67% confidence interval). The weak edge effect found in tornado and hurricane display experiments is consistent with findings from the many field studies that have reported evacuation shadow—people's evacuation from areas outside the evacuation zones that authorities have officially designated (see Lindell et al., 2019a, Chapter 4). In the case of the tornado polygon, the conflict between the NWS's intended response (there is no need for protective action outside the polygon) and people's typical response is quite likely a result of cascading conservatism that arises from the absence of a clear verbal or numeric definition of the meteorologist's subjective probability of a tornado strike outside the polygon's edges (Sorensen and Mileti, 1987). Since viewers do not know whether the tornado polygon represents a 50, 75, or 99.99% confidence interval, they are more likely to treat it as the former than the latter.
Display effects are difficult to summarize succinctly because different displays produce distinctive types of inferential errors. For example, it is now known that few people are susceptible to error of thinking that a hurricane cannot possibly track outside the uncertainty cone as Broad et al. (2007) warned. However, there does seem to be a significant proportion of viewers who mistake the uncertainty cone's increasing cross-section for an increase in hurricane size (Ruginski et al., 2016; Padilla et al., 2017). This is a potentially important finding, but it is unclear what implications, if any, this has for viewers' protective action decision making. One possibility is that perception of an increasing hurricane size would increase spontaneous evacuation by viewers in areas on either side of a designated evacuation zone (e.g., the Caution Areas on either side of the Risk Area in Figure 1. This possibility should be tested in future research that asks people to report their expected protective actions. Of course, there may be some discrepancies between people's expected protective actions and those that they take in an actual event because, as the PADM indicates, protective actions are not determined by risk perceptions alone; such actions are also affected by perceptions other stakeholders and of the alternative protective actions (Lindell and Perry, 1992, 2004, 2012; Lindell, 2018). Indeed, as Maidl and Buchecker (2015) found, simply distributing static/non-interactive hazard maps to a risk area population has only small impacts on hazard awareness, discussion with others, and expectations of preparedness—let alone actual preparedness. This is undoubtedly a result of the many variables other than hazard map content that influence people's protective actions.
Another issue needing further research is the characterization of map comprehension components. MacPherson-Krutsky et al. (2020) characterized these as basic and advanced skills, but Cao et al. (2016) characterized map effectiveness dimensions as accuracy of understanding, risk perception, response time, and preference and ease of understanding. There is also a need for further research on interactive maps because an increasing number of features, such as the ability to display or suppress different data layers, increases the complexity of hazard map operation. Thus, research will be needed that can identify the most readily understandable procedures for conveying to novice users how to select and deselect different map features (Cao et al., 2017; MacPherson-Krutsky et al., 2020).
There is also a need to examine the degree to which the magnitude of transect effects in both tornado and hurricane studies are influenced by the range of points over which viewers are asked to judge hazard impacts affects the size of the transect effect. Specifically, Wu et al. (2014) obtained a much larger transect effect than Ruginski et al. (2016) almost certainly because Wu and his colleagues asked for ps judgments in a 360 degree circle whereas Ruginski and his colleagues asked for expected damage judgments along a 650 km/400 mi line (see also Boone et al., 2018; Liu L. et al., 2019). This suggests that the range of response options offered to novice map viewers acts as a subtle cue to the range of hazard impacts. A related issue concerns the degree to which the findings from hazard map experiments generalize to risk area residents because most tornado and hurricane displays have obtained ps and damage judgments at experimenter-selected locations on a map. However, many risk area residents cannot identify their locations on a map (Zhang et al., 2004; Arlikatti et al., 2006), so there is a need for hazard map displays to identify the location of the hazard and the viewer (Liu B. F. et al., 2017).
Future research should also examine the possibility that training can correct misconceptions about hazard map displays by identifying commonly held erroneous beliefs and seeking to correct them (e.g., Whitney et al., 2004). In particular, it is important to determine if training can suppress the centroid effect in ps judgments about tornado polygons. For example, researchers might try to see if warning people about the centroid effect during simulated TV broadcasts of tornado polygons would accomplish this aim. However, such warnings might have limited effect because Boone et al. (2018) reported that training had limited success in correcting the misconception that the expanding uncertainty cone indicates increasing hurricane size. Research on hazard map training should also examine viewers' metacognition because the accuracy of people's insight into their map comprehension skills is likely to affect their training motivation (Gully and Chen, 2010).
Another direction of future research is to expand the types of displays that are studied. Hurricane display research conducted to date has emphasized alternatives or supplements to the forecast track, such as the uncertainty cone and the ensemble display. However, there has been no research on the forecast wind swath, which is defined by the locations that could be subjected to hurricane force wind rather the locations through which the hurricane center could pass (which is the definition of the uncertainty cone). Because the wind swath provides a better representation of the locations at risk, it is likely to elicit better compliance with evacuation PARs (Mileti and Sorensen, 1990; Lindell, 2018). Research on hurricane displays might also follow the lead of tornado polygon studies in examining the effect of presenting hurricane radar images, which provide graphic displays of storm size and intensity. This information could have the positive effect of increasing evacuation expectations in risk areas advised to evacuate, but might also have the negative effect of increasing shadow evacuation from inland areas that are not advised to evacuate.
Another suggestion derived from tornado polygon research would be to see if providing impact-based warnings to hurricane risk areas would increase compliance with evacuation PARs in the same way that these warnings increase shelter in-place for tornadoes (Casteel, 2016, 2018). Such studies will need to carefully examine different verbal, numeric, and visual ways of describing impacts because the wording of a Hurricane Ike warning predicting “certain death” for those who failed to evacuation seems to have had no effect on evacuation rates (Wei et al., 2014).
The Cao et al. (2017) display of expected fire front arrival times suggests that displaying Tropical Storm force wind arrival times at different locations might also be useful. This information would be consistent with warning researchers' recommendations to provide information about the expected time of impact so viewers know when to begin evacuation preparations (Mileti and Sorensen, 1990; Lindell, 2018). In addition, hurricane and wildfires displays could be improved by adding evacuation decision arcs that inform viewers when they should leave their homes to ensure that they reach safety before hazardous conditions arrive (Lindell and Prater, 2007; Cova et al., 2017). Such displays could overcome the deficiencies in PAR selection observed in Wu et al. (2015b).
Cao et al. (2017) advocated providing dynamic/interactive wildfire hazard maps viewers with interactive controls so viewers can select the elements that are most important to them. For example, they recommended additional spatial information (e.g., fire location, containment status, current and forecast wind conditions, and fire spread prediction) and textual information (e.g., well-known landmarks, names of jurisdiction subject to PARs, roads that have been closed, and names and addresses of community shelters). However, this calls attention to the need for research into intuitive interface design that relies on viewers' immediate understanding of features such as locating their home on the map, manipulating map layers, accessing non-spatial information, and adjusting the map scale.
In addition, researchers should identify ways to assist risk area residents in focusing their attention on the display elements that the experts judge to be most important. For example, researchers might follow the example of Drews et al. (2014, 2015) in asking expert Incident Commanders to identify the critical variables that should receive the most attention when selecting PARs. One possibility might be to design interfaces so the most important variables are default options. This would allow novice viewers to use the displays with minimal or no training, yet allow more advanced viewers to access additional information. In addition, novice decision makers could be provided with simplified instruction manuals and train on multiple scenarios until they reach proficiency. Unfortunately, this type of training is not feasible for risk area residents and even less feasible for tourists who are visiting the area for only a short duration. Instead, these population segments need well-designed static/non-interactive hazard maps, as well alternatives to maps such as prominent markers at hazard zone boundaries in the community.
Finally, future research on hazard maps should also examine the effects of hazard zone residence, map experience, cognitive variables such as spatial ability, and demographic variables. For example, these studies should examine the extent to which the findings from experiments using convenience samples generalize to risk area residents that have greater levels of hazard knowledge. In addition, researchers should examine people's responses to understudied hazards such as wildfires because most of the articles on wildfire visualization describes specific wildfire hazard maps (e.g., Liu D. et al., 2019) or wildfire decision support systems (e.g., Pence and Zimmerman, 2011). There is also a need to study floods and hazardous materials releases to see if they elicit responses that differ from those of the more extensively studied hazards reviewed here.
Author Contributions
ML reviewed the literature and prepared the manuscript.
Funding
This article is based on work supported by the National Science Foundation under Grants IIS-1212790, IIS-1540469, and CMMI-1826455. Any opinions, findings, and conclusions or recommendations expressed in this material are those of the author and do not necessarily reflect the views of the National Science Foundation.
Conflict of Interest
The author declares that the research was conducted in the absence of any commercial or financial relationships that could be construed as a potential conflict of interest.
References
Aksoy, B. (2013). Investigation of mapping skills of pre-service teachers as regards to various parameters. Educ. Res. Rev. 8, 134–143. doi: 10.5897/ERR12.221
Albert, G., Ilyés, V., Kis, D., Szigeti, C., and Várkonyi, D. (2016). “Testing the map reading skills of university students,” in 6th International Conference on Cartography and GIS (Albena), 188–199.
Allen, G. L., Miller Cowan, C. R., and Power, H. (2006). Acquiring information from simple weather maps: influences of domain specific knowledge and general visual-spatial abilities. Learn. Individ. Differ. 16, 337–349. doi: 10.1016/j.lindif.2007.01.003
Arlikatti, S., Lindell, M. K., Prater, C. S., and Zhang, Y. (2006). Risk area accuracy and hurricane evacuation expectations of coastal residents. Environ. Behav. 38, 226–247. doi: 10.1177/0013916505277603
Ash, K. D., Schumann, I. I. I. R. L, and Bowser, G. C. (2014). Tornado warning trade-offs: evaluating choices for visually communicating risk. Wea. Climate Soc. 6, 104–118. doi: 10.1175/WCAS-D-13-00021.1
Bell, H. M., and Tobin, G. A. (2007). Efficient and effective? The 100-year flood in the communication and perception of flood risk. Environ. Hazards 7, 302–311, doi: 10.1016/j.envhaz.2007.08.004
Boone, A. P., Gunalp, P., and Hegarty, M. (2018). Explicit versus actionable knowledge: the influence of explaining graphical conventions on interpretation of hurricane forecast visualizations. J. Exp. Psychol. Appl. 24, 275–295. doi: 10.1037/xap0000166
Bosco, A., Longoni, A. M., and Vecchi, T. (2004). Gender effects in spatial orientation: cognitive profiles and mental strategies. Appl. Cogn. Psychol. 18, 519–532. doi: 10.1002/acp.1000
Broad, K., Leiserowitz, A., Weinkle, J., and Steketee, M. (2007). Misinterpretations of the “cone of uncertainty” in Florida during the 2004 hurricane season. Bull. Am. Meteorol. Soc. 88, 651–668. doi: 10.1175/BAMS-88-5-651
Buckley, J., Seery, N., and Canty, D. (2018). A heuristic framework of spatial ability: a review and synthesis of spatial factor literature to support its translation into STEM education. Educ. Psychol. Rev. 30, 947–972. doi: 10.1007/s10648-018-9432-z
Cao, Y., Boruff, B. J., and Mcneill, I. M. (2016). Is a picture worth a thousand words? Evaluating the effectiveness of maps for delivering wild fire warning information. Int. J. Disaster Risk Reduct. 19, 179–196, doi: 10.1016/j.ijdrr.2016.08.012
Cao, Y., Boruff, B. J., and McNeill, I. M. (2017). The smoke is rising but where is the fire? Exploring effective online map design for wildfire warnings. Nat. Hazards 88, 1473–1501. doi: 10.1007/s11069-017-2929-9
Casteel, M. A. (2016). Communicating increased risk: an empirical investigation of the National Weather Service's impact-based warnings. Wea. Climate Soc. 8, 219–232. doi: 10.1175/WCAS-D-15-0044.1
Casteel, M. A. (2018). An empirical assessment of impact based tornado warnings on shelter in place decisions. Int. J. Disaster Risk Reduct. 30, 25–33. doi: 10.1016/j.ijdrr.2018.01.036
Casteel, M. A., and Downing, J. R. (2015). Assessing risk following a wireless emergency alert: are 90 characters enough? J. Homeland Secur. Emerg. Manage. 13, 95–112. doi: 10.1515/jhsem-2015-0024
Cheong, L., Bleisch, S., Kealy, A., Tolhurst, K., Wilkening, T., and Duckham, M. (2016). Evaluating the impact of visualization of wildfire hazard upon decision-making under uncertainty. Int. J. Geogr. Inf. Sci. 30, 1377–1404. doi: 10.1080/13658816.2015.1131829
Colom, R., Contreras, M. J., Botella, J., and Santacreu, J. (2002). Vehicles of spatial ability. Pers. Individ. Dif. 32, 903–912. doi: 10.1016/S0191-8869(01)00095-2
Coluccia, E., and Louse, G. (2004). Gender differences in spatial orientation: a review. J. Environ. Psychol. 24, 329–340. doi: 10.1016/j.jenvp.2004.08.006
Cova, T. J., Dennison, P. E., Li, D., Drews, F. A., Siebeneck, L. K., and Lindell, M. K. (2017). Warning triggers in environmental hazards: who should be warned to do what and when? Risk Anal. 37, 601–611. doi: 10.1111/risa.12651
Cox, J., House, D., and Lindell, M. K. (2013). Visualizing uncertainty in predicted hurricane tracks. Int. J. Uncertain. Quantif. 3, 143–156. doi: 10.1615/Int.J.UncertaintyQuantification.2012003966
Cybulski, P. (2016). Design rules and practices for animated maps online. J. Spat. Sci. 61, 461–471. doi: 10.1080/14498596.2016.1147394
Dransch, D., Rotzoll, H., and Poser, K. (2010). The contribution of maps to the challenges of risk communication to the public. Int. J. Digit. Earth 3, 292–311, doi: 10.1080/17538941003774668
Drews, F. A., Musters, A., Siebeneck, L. K., and Cova, T. J. (2014). Environmental factors that influence wildfire protective-action recommendations. Int. J. Emerg. Manage. 10, 153–168. doi: 10.1504/IJEM.2014.066187
Drews, F. A., Siebeneck, L., and Cova, T. (2015). Information search and decision making in computer-based wildfire simulations. J. Cogn. Eng. Decis. Making 9, 229–240. doi: 10.1177/1555343415586478
Dunning, D. (2011). “The Dunning–Kruger effect: on being ignorant of one's own ignorance,” in Advances in Experimental Social Psychology, Vol. 44, eds J.M. Olson, and M.P. Zanna (San Diego, CA: Academic Press), 247–296. doi: 10.1016/B978-0-12-385522-0.00005-6
Eley, M. G. (1993). The differential susceptibility of topographic map interpretation to influence from training. Appl. Cogn. Psychol. 7, 23–42. doi: 10.1002/acp.2350070104
Endsley, M. R., and Jones, D. G. (2012). Designing for Situation Awareness: An Approach to User-Centered Design, 2nd Edn. Boca Raton, FL: CRC Press.
Gigerenzer, G., and Goldstein, D. G. (1996). Reasoning the fast and frugal way: models of bounded rationality. Psychol. Rev. 103, 650–669. doi: 10.1037/0033-295X.103.4.650
Gillen, J., Skryzhevska, L., Henry, M. C., and Green, J. (2010). Map interpretation instruction in introductory textbooks: a preliminary investigation. J. Geogr. 109, 181–189. doi: 10.1080/00221341.2010.501381
Gully, S., and Chen, G. (2010). “Individual differences, attribute-treatment interactions, and training outcomes,” in Learning, Training, And Development in Organizations, eds S.W.J. Kozlowski, and E. Salas (New York, NY: Routledge), 3–64.
Halpern, D. F., and Collaer, M. L. (2005). “Sex differences in visuospatial abilities: more than meets the eye,” in The Cambridge Handbook of Visuospatial Thinking, eds P. Shah, and A. Miyake (New York, NY: Cambridge University Press), 170–212. doi: 10.1017/CBO9780511610448.006
Handmer, J. W. (1980). Flood hazard maps as public information: an assessment within the context of the Canadian flood damage reduction program. Can. Water Resour. J. 5, 82–110. doi: 10.4296/cwrj0504082
Haynes, K., Barclay, J., and Pidgeon, N. (2007). Volcanic hazard communication using maps: an evaluation of their effectiveness. Bull. Volcanol. 70, 123–138. doi: 10.1007/s00445-007-0124-7
Hegarty, M. (2011). The cognitive science of visual-spatial displays: implications for design. Top. Cogn. Sci. 3, 446–474. doi: 10.1111/j.1756-8765.2011.01150.x
Hegarty, M., Crookes, R. D., Dara-Abrams, D., and Shipley, T. F. (2010). Do all science disciplines rely on spatial abilities? Preliminary evidence from self-report questionnaires. Lect. Notes Comput. Sci. 6222, 85–94, doi: 10.1007/978-3-642-14749-4_10
Hsu, Y.-T., and Peeta, S. (2014). Risk-based spatial zone determination problem for stage-based evacuation operations. Transp. Res. C Emerg. Technol. 41, 73–89. doi: 10.1016/j.trc.2014.01.013
Huang, S.-K., Lindell, M. K., and Prater, C. S. (2016). Who leaves and who stays? A review and statistical meta-analysis of hurricane evacuation studies. Environ. Behav. 48, 991–1029. doi: 10.1177/0013916515578485
Huang, S.-K., Lindell, M. K., Wei, H.-L., and Samuelson, C. D. (2017). Perceptions, behavioral expectations, and implementation timing for response actions in a hurricane emergency. Nat. Hazards 88, 533–558. doi: 10.1007/s11069-017-2877-4
Hyde, J. S., and Linn, M. C. (1988). Gender differences in verbal ability: a meta-analysis. Psychol. Bull. 104:53. doi: 10.1037/0033-2909.104.1.53
Jon, I., Huang, S.-K., and Lindell, M. K. (2018). Perceptions and reactions to tornado warning polygons: would a gradient polygon be useful? Int. J. Disaster Risk Reduct. 30, 132–144. doi: 10.1016/j.ijdrr.2018.01.035
Jon, I., Huang, S.-K., and Lindell, M. K. (2019). Perceptions and expected immediate reactions to severe storm displays. Risk Anal. 39, 274–290. doi: 10.1111/risa.12896
Kang, J. E., Lindell, M. K., and Prater, C. S. (2007). Hurricane evacuation expectations and actual behavior in Hurricane Lili. J. Appl. Soc. Psychol. 37, 881–897. doi: 10.1111/j.1559-1816.2007.00191.x
Keller, N., Cokely, E. T., Katsikopoulos, K. V., and Wegwarth, O. (2010). Naturalistic heuristics for decision making. J. Cogn. Eng. Decis. Mak. 4, 256–274. doi: 10.1518/155534310X12844000801168
Klockow, K. E. (2013). Spatializing Tornado Warning Lead-Time: Risk Perception and Response in a Spatio-Temporal Framework. Norman OK: The University of Oklahoma.
Klockow-McClain, K. E., McPherson, R. A., and Thomas, R. P. (2020). Cartographic design for improved decision making: trade-offs in uncertainty visualization for tornado threats. Ann. Am. Assoc. Geogr. 110, 314–333. doi: 10.1080/24694452.2019.1602467
Krocak, M. J., Ernst, S., Allan, J. N., Wehde, W., Ripberger, J. T., Silva, C. L., et al. (2020). Thinking outside the polygon: a study of tornado warning perception outside of warning polygon bounds. Nat. Hazards 102, 1351–1368. doi: 10.1007/s11069-020-03970-5
Lawton, C. A., and Morrin, K. A. (1999). Gender differences in pointing accuracy in computer simulated 3D mazes. Sex Roles 40, 73–92. doi: 10.1023/A:1018830401088
Lindell, M. K. (2018). “Communicating imminent risk,” in Handbook of Disaster Research, eds H. Rodríguez, J. Trainor, and W. Donner (New York, NY: Springer), 449–477. doi: 10.1007/978-3-319-63254-4_22
Lindell, M. K., Bostrom, A., Goltz, J., and Prater, C. S. (2019b). Social Science Evaluation of Tsunami Evacuation Products, Part 1: Assessment of Textual, Graphical, and Numerical Features. Seattle, WA: Environmental Hazards Research Institute.
Lindell, M. K., and Earle, T. C. (1983). How close is close enough: public perceptions of the risks of industrial facilities. Risk Anal. 3, 245–253. doi: 10.1111/j.1539-6924.1983.tb01393.x
Lindell, M. K., Huang, S., Wei, H., and Samuelson, C. D. (2016). Perceptions and expected immediate reactions to tornado warning polygons. Nat. Hazards 80, 683–707. doi: 10.1007/s11069-015-1990-5
Lindell, M. K., Murray-Tuite, P., Wolshon, B., and Baker, E. J. (2019a). Large-Scale Evacuation: The Analysis, Modeling, and Management of Emergency Relocation From Hazardous Areas. New York, NY: Routledge. doi: 10.4324/9781315119045
Lindell, M. K., and Perry, R. W. (1992). Behavioral Foundations of Community Emergency Planning. Washington DC: Hemisphere Press.
Lindell, M. K., and Perry, R. W. (2004). Communicating Environmental Risk in Multiethnic Communities. Thousand Oaks, CA: Sage Publications.
Lindell, M. K., and Perry, R. W. (2012). The protective action decision model: theoretical modifications and additional evidence. Risk Anal. 32, 616–632, doi: 10.1111/j.1539-6924.2011.01647.x
Lindell, M. K., and Prater, C. (2007). A hurricane evacuation management decision support system (EMDSS). Nat. Hazards 40, 627–334. doi: 10.1007/s11069-006-9013-1
Lindell, M. K., and Prater, C. S. (2010). Tsunami preparedness on the Oregon and Washington coast: recommendations for research. Nat. Hazards Rev. 11, 69–81. doi: 10.1061/(ASCE)1527-6988(2010)11:2(69)
Lindell, M. K., Sutter, D. S., and Trainor, J. E. (2013). Individual and household response to tornadoes. Int. J. Mass Emerg. Disasters 31, 373–383.
Liu, B. F., Wood, M. M., Egnoto, M., Bean, H., Sutton, J., Mileti, D., et al. (2017). Is a picture worth a thousand words? The effects of maps and warning messages on how publics respond to disaster information. Public Relations Rev. 43, 493–506. doi: 10.1016/j.pubrev.2017.04.004
Liu, D., Xu, Z., and Fan, C. (2019). Generalized analysis of regional fire risk using data visualization of incidents. Fire Mater. 43, 413–421. doi: 10.1002/fam.2713
Liu, L., Boone, A. P., Ruginski, I. T., Padilla, L., Hegarty, M., Creem-Regehr, S. H., et al. (2017). Uncertainty visualization by representative sampling from prediction ensembles. IEEE Trans. Vis. Comput. Graph 23, 2165–2178. doi: 10.1109/TVCG.2016.2607204
Liu, L., Padilla, L., Creem-Regehr, S. H., and House, D. H. (2019). Visualizing uncertain tropical cyclone predictions using representative samples from ensembles of forecast tracks. IEEE Trans. Vis. Comput. Graph 25, 882–891. doi: 10.1109/TVCG.2018.2865193
MacPherson-Krutsky, C., Brand, B. D., and Lindell, M. K. (2020). Does updating natural hazard maps to reflect best practices increase user comprehension of risk? Int. J. Disaster Risk Reduct. 46:101487. doi: 10.1016/j.ijdrr.2020.101487
Maidl, E., and Buchecker, M. (2015). Raising risk preparedness by flood risk communication. Nat. Hazards Earth Syst. Sci. 15, 1577–1595. doi: 10.5194/nhess-15-1577-2015
Mason, J. B., and Senkbeil, J. C. (2014). Implications of the 2011 Tuscaloosa EF4 tornado for shelter and refuge decisions. Nat. Hazards 74, 1021–1041. doi: 10.1007/s11069-014-1230-4
Meyer, R., Broad, K., Orlove, B., and Petrovic, N. (2013). Dynamic simulation as an approach to understanding hurricane risk response: insights from the Stormview lab. Risk Anal. 33, 1532–1552. doi: 10.1111/j.1539-6924.2012.01935.x
Mileti, D. S., and Sorensen, J. H. (1990). Communication of Emergency Public Warnings: A Social Science Perspective and State-Of-The-Art Assessment (No. ORNL-6609). Oak Ridge, TN: Oak Ridge National Laboratories. doi: 10.2172/6137387
Milson, A. J., and Alibrandi, M. (2008). “Critical map literacy and geographic information systems: the spatial dimension of civic decision making,” in The Electronic Republic? The Impact of Technology on Education for Citizenship, eds P. J. VanFossen, and M. J. Berson (West Lafayette, IN: Purdue University Press), 110–128.
Miran, S. M., Ling, C., and James, J. J. (2020). People's thresholds of decision-making against a tornado threat using dynamic probabilistic hazard information. Int. J. Disaster Risk Reduct. 42:101345. doi: 10.1016/j.ijdrr.2019.101345
Miran, S. M., Ling, C., James, J. J., and Rothfusz, L. (2016). “Comparing effectiveness of four graphical designs for probabilistic hazard information for tornado threat,” in Proceedings of the Human Factors and Ergonomics Society Annual Meeting, Vol. 60 (Los Angeles, CA: SAGE Publications), 2029–2033. doi: 10.1177/1541931213601461
Miran, S. M., Ling, C., and Rothfusz, L. (2018). Factors influencing people's decision-making during three consecutive tornado events. Int. J. Disaster Risk Reduct. 28, 150–157. doi: 10.1016/j.ijdrr.2018.02.034
Montello, D. R., Lovelace, K. L., Golledge, R. G., and Self, C. M. (1999). Sex-related differences and similarities in geographic and environmental spatial abilities. Ann. Assoc. Am. Geogr. 89, 515–534. doi: 10.1111/0004-5608.00160
Muir, S. P. (1985). Understanding and improving students' map reading skills. Elementary School J. 86, 206–216. doi: 10.1086/461444
Mulder, K. J., Lickiss, M., Harvey, N., Black, A., Charlton-Perez, A., Dacre, H., et al. (2017). Visualizing volcanic ash forecasts: scientist and stakeholder decisions using different graphical representations and conflicting forecasts. Wea. Climate Soc. 9, 333–348. doi: 10.1175/WCAS-D-16-0062.1
Nagele, D. E., and Trainor, J. E. (2012). Geographic specificity, tornadoes, and protective action. Wea. Climate Soc. 4, 145–155. doi: 10.1175/WCAS-D-11-00047.1
Nave, R., Isaia, R., Vilardo, G., and Barclay, J. (2010). Re-assessing volcanic hazard maps for improving volcanic risk communication: application to Stromboli Island, Italy. J. Maps 6, 260–269, doi: 10.4113/jom.2010.1061
Ooms, K., De Maeyer, P., and Fack, V. (2014). Study of the attentive behavior of novice and expert map users using eye tracking. Cartogr. Geogr. Inf. Sci. 41, 37–54. doi: 10.1080/15230406.2013.860255
Ooms, K., De Maeyer, P., and Fack, V. (2015). Listen to the map user: cognition, memory, and expertise. Cartogr. J. 52, 3–19, doi: 10.1179/1743277413Y.0000000068
Padilla, L. M., Ruginski, I. T., and Creem-Regehr, S. H. (2017). Effects of ensemble and summary displays on interpretations of geospatial uncertainty data. Cogn. Res. Princ. Impl. 2:40. doi: 10.1186/s41235-017-0076-1
Pence, M., and Zimmerman, T. (2011). The wildland fire decision support system: integrating science, technology, and fire management. Fire Manage. Today 71, 18–22.
Picucci, L., Caffò, A. O., and Bosco, A. (2011). Besides navigation accuracy: gender differences in strategy selection and level of spatial confidence. J. Environ. Psychol. 31, 430–438. doi: 10.1016/j.jenvp.2011.01.005
Postigo, Y., and Pozo, J. I. (2004). On the road to graphicacy: the learning of graphical representation systems. Educ. Psychol. 24, 623–644, doi: 10.1080/0144341042000262944
Reilly, D., Neumann, D. L., and Andrews, G. (2016). Sex and sex-role differences in specific cognitive abilities. Intelligence 54, 147–158. doi: 10.1016/j.intell.2015.12.004
Ruginski, I. T., Boone, A. P., Padilla, L. M., Liu, L., Heydari, N., Kramer, H. S., et al. (2016). Non-expert interpretations of hurricane forecast uncertainty visualizations. Spat. Cogn. Comput. 16, 154–172. doi: 10.1080/13875868.2015.1137577
Sherman-Morris, K., and Brown, M. E. (2012). Experiences of Smithville, Mississippi residents with the 27 April 2011 tornado. Natl. Wea. Digest 36, 93–101.
Sherman-Morris, K., and Del Valle-Martinez, I. (2017). Optimistic bias and the consistency of hurricane track forecasts. Nat. Hazards 88, 1523–1543. doi: 10.1007/s11069-017-2931-2
Sorensen, J. H. (1991). When shall we leave? Factors affecting the timing of evacuation departures. Int. J. Mass Emerg. Disasters 9, 153–165.
Sorensen, J. H., Carnes, S. A., and Rogers, G. O. (1992). An approach for deriving emergency planning zones for chemical munitions emergencies. J. Hazard. Mater. 30, 223–242. doi: 10.1016/0304-3894(92)87001-V
Sorensen, J. H., and Mileti, D. S. (1987). Decision making uncertainties in emergency warning system organizations. Int. J. Mass Emerg. Disasters 5, 33–61.
Teigen, K. H. (2005). The proximity heuristic in judgments of accident probabilities. Br. J. Psychol. 96, 423–440. doi: 10.1348/000712605X47431
Thompson, M. A., Lindsay, J. M., and Gaillard, J. C. (2015). The influence of probabilistic volcanic hazard map properties on hazard communication. Appl. Volcanol. 4, 1–24, doi: 10.1186/s13617-015-0023-0
Tversky, A., and Kahneman, D. (1974). Judgment under uncertainty: heuristics and biases. Science 185, 1124–1131. doi: 10.1126/science.185.4157.1124
Van Kerkvoorde, M., Kellens, W., Verfaillie, E., and Ooms, K. (2018). Evaluation of web maps for the communication of flood risks to the public in Europe. Int. J. Cartogr. 4, 49–64. doi: 10.1080/23729333.2017.1371411
Voyer, D., Voyer, S., and Bryden, M. P. (1995). Magnitude of sex differences in spatial abilities: a meta-analysis and consideration of critical variables. Psychol. Bull. 117, 250–270. doi: 10.1037/0033-2909.117.2.250
Wei, H. L., Lindell, M. K., and Prater, C. S. (2014). “Certain death” from storm surge: a comparative study of household responses to warnings about Hurricanes Rita and Ike. Wea. Climate Soc. 6, 425–433. doi: 10.1175/WCAS-D-13-00074.1
Whitney, D. J., Lindell, M. K., and Nguyen, D. H. (2004). Earthquake beliefs and adoption of seismic hazard adjustments. Risk Anal. 24, 87–102. doi: 10.1111/j.0272-4332.2004.00414.x
Wu, H.-C., Huang, S. K., and Lindell, M. K. (2020). “Chapter 12: Evacuation planning,” in The Routledge Handbook of Urban Disaster Resilience: Integrating Mitigation, Preparedness, and Recovery Planning, ed M.K. Lindell (New York, NY: Routledge), 206–222. doi: 10.4324/9781315714462-12
Wu, H.-C., Lindell, M. K., and Prater, C. S. (2015a). Process tracing analysis of hurricane information displays. Risk Anal. 35, 2202–2220. doi: 10.1111/risa.12423
Wu, H.-C., Lindell, M. K., and Prater, C. S. (2015b). Strike probability judgments and protective action recommendations in a dynamic hurricane tracking task. Nat. Hazards 79, 355–380. doi: 10.1007/s11069-015-1846-z
Wu, H.-C., Lindell, M. K., Prater, C. S., and Samuelson, C. D. (2014). Effects of track and threat information on judgments of hurricane strike probability. Risk Anal. 34, 1025–1039. doi: 10.1111/risa.12128
Yin, W., Murray-Tuite, P., Ukkusuri, S. V., and Gladwin, H. (2016). Modeling shadow evacuation for hurricanes with random-parameter logit model. Transp. Res. Rec. 2599, 43–51. doi: 10.3141/2599-06
Yoon, S. Y., and Mann, E. L. (2017). Exploring the spatial ability of undergraduate students: association with gender, STEM majors, and gifted program membership. Gifted Child Q. 61, 313–327. doi: 10.1177/0016986217722614
Keywords: hazard map, map comprehension, display effects, proximity effect, protective action decision making, evacuation decision arc
Citation: Lindell MK (2020) Improving Hazard Map Comprehension for Protective Action Decision Making. Front. Comput. Sci. 2:27. doi: 10.3389/fcomp.2020.00027
Received: 02 June 2020; Accepted: 23 June 2020;
Published: 31 July 2020.
Edited by:
Roberto Therón, University of Salamanca, SpainReviewed by:
Magdalena Mendez-Lopez, University of Zaragoza, SpainThomas John Cova, The University of Utah, United States
Copyright © 2020 Lindell. This is an open-access article distributed under the terms of the Creative Commons Attribution License (CC BY). The use, distribution or reproduction in other forums is permitted, provided the original author(s) and the copyright owner(s) are credited and that the original publication in this journal is cited, in accordance with accepted academic practice. No use, distribution or reproduction is permitted which does not comply with these terms.
*Correspondence: Michael K. Lindell, bWxpbmRlbGwmI3gwMDA0MDt1dy5lZHU=