- 1Department of Communication Studies, University of Georgia, Athens, GA, United States
- 2School of Communication, The Ohio State University, Columbus, OH, United States
- 3Department of Communication, Cornell University, Ithaca, NY, United States
Although uncertainty is inherent in science, public audiences vary in their openness to information about preliminary discoveries and the caveats and limitations of research. These preferences shape responses to science communication, and science communicators often adapt messaging based on assumed preferences. However, there has not been a validated instrument for examining these preferences. Here, we present an instrument to capture preferences for information about uncertainty in science, validated with a large U.S. adult sample. Factor analysis results show that preferring certain scientific information and preferring uncertain scientific information are orthogonal constructs requiring separate measures. The final Preference for Information about Uncertain Science (or “PIUS-11”) scale comprises two dimensions: preferring complete information (i.e., caveats, limitations, and hedging included) and being open to learning about preliminary science. The final Preference for Certain Science Information (or “PCSI-9”) scale comprises two dimensions: preferring streamlined information (i.e., caveats, limitations, and hedging removed) and preferring to learn only about established science. We present psychometric properties of each scale and report observed relationships between each set of preferences and an individual's scientific understanding, trust in science, need for cognitive closure, and sociodemographic factors.
Introduction
Scientific discoveries disseminated to the public are often related to health and medicine, and how public audiences receive this information can have bearing on individual and public health (National Academies of Sciences, Engineering, and Medicine, 2017). Nonexperts view the scientific process differently from experts (Bromme and Goldman, 2014; National Science Board, 2020), posing challenges for conveying the inherent complexities of scientific evidence to the public (Einsiedel and Thorn, 1999). Adding to these challenges, “the public” is not a single monolithic entity but is instead comprised of multiple publics with a diverse mix of backgrounds, dispositional characteristics, and preferences (Scheufele, 2018). Studies demonstrate mixed effects of communicating scientific caveats and limitations to public audiences (see reviews by van der Bles et al., 2019; Gustafson and Rice, 2020; Ratcliff et al., 2022), underscoring the likelihood that these reactions depend, at least in part, on individual differences.
A growing body of research finds support for the influence of audience characteristics on reactions to uncertain science communication. These characteristics include level of education (Jensen et al., 2017; Adams et al., 2023), dispositional uncertainty tolerance (Ratcliff et al., 2021), and beliefs about the nature of the scientific process (Rabinovich and Morton, 2012; Kimmerle et al., 2015; Ratcliff et al., 2023). While insightful, these characteristics only sometimes moderate the effects of communicating uncertainty,1 suggesting that additional factors warrant investigation. Gustafson and Rice (2019, p. 699) recommend that “research should study more carefully how individual characteristics moderate these effects—especially characteristics that relate to attitudes toward, or preferences for, uncertainty.” Emerging evidence suggests that public audiences do indeed have diverse preferences with regard to learning about uncertainty in scientific discoveries (Maier et al., 2016; Wegwarth et al., 2020; Post et al., 2021; Ratcliff and Wicke, 2023). Yet the relationship between these preferences and other individual characteristics is still unclear, and the literature lacks a comprehensive and validated tool for measuring such preferences.
Being able to directly capture preferences for (un)certain science information is an important step toward better public science communication. It paves the way for research that uncovers the factors shaping these preferences and, ultimately, for identifying ways to effectively disseminate uncertain scientific evidence to different publics, which remains a topmost goal in health, risk, and science communication (Jensen et al., 2013; National Academies of Sciences, Engineering, and Medicine, 2017; Sopory et al., 2019; Paek and Hove, 2020; Simonovic et al., 2023). To this end, we use the current project to develop and validate a comprehensive measure of information preferences related to uncertain science. We build on an existing measure (the 7-item Preference for Information about Uncertain Science, or “PIUS-7,” scale; Ratcliff and Wicke, 2023), which captures the extent to which people want to hear about preliminary scientific discoveries and the specific caveats and limitations of research. Initial tests of that scale in two health contexts—genetic depression risk and COVID-19 vaccines—demonstrated that people's preferences and expectations regarding science communication help to explain their reactions to (un)certainty messages. Specifically, we found that communicating uncertainties in COVID-19 vaccine research had a negative effect on perceived source credibility only for people with low PIUS scores (Ratcliff et al., 2023). Similarly, we found that communicating uncertainties in research on genetic depression risk enhanced perceived source credibility for those with high PIUS scores (Ratcliff and Wicke, 2023). Although these tests of the initial PIUS scale were insightful, critical next steps are to (1) explore additional facets of preference not captured by that measure, (2) examine the psychometric properties of the measure, and (3) identify which individual characteristics are associated with these preferences. In the current project, we develop and test a large battery of items capturing preferences for both certainty and uncertainty communication. We test the factor structure, reliability, and validity of an expanded instrument and examine individual difference correlates in order to better understand which factors influence or relate to (un)certainty information preferences.
Characterizing (un)certain science information preferences
Scientific uncertainty takes many forms and comes from many different sources (Han et al., 2011; van der Bles et al., 2019; Ratcliff, 2021). Preliminary or initial scientific discoveries are inherently uncertain (Dumas-Mallet et al., 2018). Yet nearly all scientific claims—even well-established ones—are characterized by some degree of uncertainty, owing to methodological limitations, uncertainty associated with probabilistic models, or the sheer complexity of some phenomena (Han et al., 2011; Gustafson and Rice, 2020). Journalists and other science communicators choose whether to present preliminary science or to only report established findings with a strong evidence base and scientific consensus (Friedman et al., 1999). They also choose whether to convey complete information about uncertainties, or to instead streamline research reports—that is, to “remove caveats, limitations, and hedging so science appears simple and more certain” (Jensen et al., 2013, p. 1).
Science communicators' choices about whether to communicate uncertainty or to streamline science, and whether or not to share preliminary evidence, are often based on beliefs about audience preferences (Friedman et al., 1999; Guenther and Ruhrmann, 2016; Maier et al., 2016). Most often, these beliefs result in the systematic removal of uncertainty from science (Jensen et al., 2013; Dumas-Mallet et al., 2018; Ratcliff, 2021). Yet there have been few empirical attempts to actually understand public expectations and preferences for (un)certain science information. Are general audiences really so averse to communication of scientific uncertainty? Importantly, desiring communication of uncertainty does not indicate a preference for being in a state of uncertainty, but for learning about the uncertainty of others (e.g., scientific experts). A preference for communication of certainty, in contrast, captures a desire for streamlined and definitive summaries of complex and potentially uncertain science.
Why would some individuals choose to receive complete information about scientific findings, while others want a streamlined (even if inaccurate) account? Factors driving a preference for learning about research caveats and limitations include associating these with transparent (i.e., complete, honest, and accurate) information (Maier et al., 2016) and wanting full information about the research in order to make one's own judgement (Post et al., 2021). An interest in learning about preliminary science can arise from an acceptance of uncertainty as a normal part of science, with an awareness that conclusions may change in the future (Frewer et al., 2002; Maier et al., 2016). It can also stem from associating preliminary science with scientific progress and cause for optimism (Biesecker et al., 2014; Maier et al., 2016) or from viewing it as sometimes the best available evidence (Frewer et al., 2002). In contrast, a preference for streamlined science information can be shaped by a belief that one doesn't have the knowledge to make sense of uncertainty (Maier et al., 2016) and a desire to instead rely on experts' interpretations (Einsiedel and Thorn, 1999). It can also be shaped by a belief that uncertain language is unnecessarily complex and confusing (Greiner Safi et al., 2023). Another common factor underlying preference for both established science and streamlined claims is a belief that information about certain science is more reliable and useful, while communicating uncertainty signals that the research is unreliable, unfinished, or poor quality (Frewer et al., 2002; Biesecker et al., 2014; Maier et al., 2016; Post et al., 2021).
A notable gap in the literature, however, is evidence about what shapes these appraisals and their associated preferences. Below, we outline three individual factors—a person's view of how science works, level of trust in science, and ability to manage ambiguity in general—that may underpin preferences for certain or uncertain science information. Additionally, we explore potential relationships between preferences and sociodemographic factors.
Understanding of science
Appraisal of uncertainty can be shaped by one's familiarity or experience with the issue (Mishel, 1988). Therefore, a person's scientific understanding is likely to play a central role in the formation of preferences for (un)certain science information. According to Miller (1983), scientific literacy is comprised of three dimensions: comprehension of specific scientific concepts (i.e., factual knowledge), understanding of the scientific process (i.e., understanding the process of conducting a scientific study), and awareness of contemporary scientific issues. The first two dimensions are likely to correlate with whether a person views uncertainty as a normal part of science; that is, whether they have “sophisticated epistemic beliefs” and view science as an ongoing process of discovery or have “simple epistemic beliefs” and view scientific knowledge as stable, concrete, and absolute (Kimmerle et al., 2015; Retzbach et al., 2016). Research has found that people with greater understanding of the concept of a scientific study react more favorably when scientific information is framed as uncertain rather than certain (Ratcliff et al., 2021), as do those with sophisticated epistemic beliefs (Ratcliff et al., 2023) and those who view science as “a debate between alternative positions” rather than as “a quest for absolute truths” (Rabinovich and Morton, 2012, p. 993). Further, Post et al. (2021) observed a connection between believing science is certain and preferring journalists to report definitive information during the COVID-19 pandemic. Given this, we investigate whether preferring information about uncertain science is related to greater scientific understanding; specifically, greater factual scientific knowledge, understanding of the concept of a scientific study, and sophisticated beliefs about the nature of scientific knowledge.
Trust in science
A person's trust in scientists and deference to science could influence their comfort with uncertain science information. In the absence of expertise, members of the public often rely on experts to inform their attitudes toward scientific issues (Hendriks et al., 2016). Potentially, being generally inclined to trust scientists—that is, to believe in their integrity and benevolence (Hendriks et al., 2016)—could predispose someone to interpret uncertainty and preliminary science in a favorable light. Conversely, people with low trust in scientists' integrity and goodwill might use motivated reasoning to interpret information about uncertainty as further evidence of untrustworthiness (Gustafson and Rice, 2020). Deference to science represents the extent to which an individual is likely to accept, rather than challenge, information from scientific entities and to view scientists as an authority on scientific-related matters (Binder et al., 2016). It also taps a belief that science need not be subject to the same level of regulation as other institutions, such as corporations and government entities (Brossard and Nisbet, 2007). It is possible that those with greater deference to scientific authority are more comfortable with information about uncertainty, perhaps because they believe that uncertain science is still the best information available, or they feel confident scientists will be able to resolve this uncertainty. An alternative possibility is that deference and trust lead individuals to believe science can provide definitive answers, or to prefer that scientists streamline their findings, choosing what information to present and how it should be interpreted. To date, studies have not found clear linkages between deference to science and reactions to messages about scientific uncertainty (e.g., Clarke et al., 2015; Binder et al., 2016; Dunwoody and Kohl, 2017). Thus, it remains in question whether trust in scientists and deference to science cause an openness to uncertain information and preliminary science, or whether these inspire a preference to receive certain scientific information.
Need for cognitive closure
Individuals are thought to have a general orientation toward uncertainty, which shapes their tolerance for ambiguity and other sources of uncertainty across a range of life contexts (Han et al., 2011; Hillen et al., 2017). As it pertains to information preferences, those less tolerant of uncertainty tend to have a high need for cognitive closure, defined as a desire for “an answer on a given topic, any answer, … compared to confusion and ambiguity” (Kruglanski, 1990, p. 337). Potentially, need for cognitive closure—or simply “need for closure”—influences a person's scientific information preferences: those with low need for closure may be much more open to learning about uncertain science and specific sources of uncertainty surrounding a discovery, while those with high need for closure may prefer to learn only about established science and may prefer certain or concrete (even if less accurate) information. An important question is whether scientific uncertainty information preferences are related to but distinct from a general need for closure.
Sociodemographic factors
Groups that differ in their response to (un)certain science communication may be distinguished by sociodemographic factors. Receptiveness to information about emerging or controversial science, such as new medical technologies or climate change, tends to be associated with factors such as race, political affiliation, political ideology, and religiosity (Drummond and Fischhoff, 2017; National Academies of Sciences, Engineering, and Medicine, 2017; Rutjens et al., 2022). However, aside from education level, which has been shown to moderate the effects of communicating scientific uncertainty (Jensen et al., 2017; Adams et al., 2023), relationships between sociodemographic variables and responses to uncertain communication are largely unexplored. Therefore, we investigate age, gender, education, race, ethnicity, religiosity, political affiliation, and political ideology as potential correlates of uncertainty preferences.
Research questions
Based on the conceptual and theoretical frameworks and evidence described above, we developed a measure of preferences for (un)certain science information with the goal of addressing the following research questions:
RQ1: What is the nature of (un)certain science information preferences, in terms of valid indicators and the factor structure of the construct(s)?
RQ2: Do preferences for (un)certain science information relate to scientific understanding (i.e., epistemic beliefs, factual science literacy, and understanding the concept of a scientific study), trust in science (i.e., trust in scientists and deference to science), or a need for cognitive closure?
RQ3: Do preferences for (un)certain science information relate to sociodemographic factors?
Item development
To develop a pool of items for testing, we built on the aforementioned PIUS-7 scale (Ratcliff and Wicke, 2023) with a focus on achieving broader coverage of the construct(s) and using language that resonates with nonexperts. In line with the original PIUS measure, we sought to capture preferences for both complete information (i.e., communication of research caveats, limitations, and unknowns) and information about preliminary science (i.e., wanting to learn about emerging science, not just established science). These dimensions share common attributes as both involve communicating about uncertain science. Yet, as previously discussed, they differ insofar as all scientific evidence is characterized by some degree of uncertainty, and therefore information about uncertainty can be communicated about most science (even relatively established evidence), whereas preliminary science is by nature highly uncertain. Moreover, the former preference emphasizes transparency about research details, while the latter emphasizes an interest in learning about novel discoveries even if they may be tentative.
A key limitation of the original PIUS scale was its focus on uncertainty preferences. Although it is possible that certainty-focused and uncertainty-focused preferences represent opposite sides of a spectrum—where low preference for uncertainty is the same as high preference for certainty—it is also possible that these are not mutually exclusive and instead represent distinct constructs. For example, although the original scale contained three items focused on preference for certainty, these were removed because they did not load inversely on the uncertainty preference factor (Ratcliff and Wicke, 2023). This suggests the possibility that preferences for certain and uncertain information can coexist and should therefore be captured with separate measures. Therefore, we created two distinct sets of items: one representing uncertainty preferences (with subsets for complete information and preliminary science) and the other representing certainty preferences (with subsets for streamlined information and established science). Within this conceptual framework of four possible preference dimensions, we developed a bank of items for further testing.
Because nonexperts view the scientific process differently from experts (Bromme and Goldman, 2014; National Science Board, 2020), we consulted qualitative studies (e.g., Maier et al., 2016; Greiner Safi et al., 2023) and existing scales (e.g., Frewer et al., 2002; Post et al., 2021) to get a sense of the language nonexperts use to describe preferences for learning about uncertainty. To enhance the informativeness of the scale, we sought to include a mix of straightforward item statements that simply capture information preferences, along with statements that capture specific reasons for these preferences, guided by the literature described in the previous section. Integrating these observations, we retained the seven uncertainty-focused items from the original PIUS scale without modification (items 1–7 in Box 1). We revised two certainty-focused items from the original scale2 and developed an additional 20 items, rendering a battery of 29 items for testing. This initial item bank is presented in Box 1, with items labeled according to their intended conceptual dimensions.
Scale testing
Next, we conducted a survey study to test the factor structure and psychometric properties of the instrument, to remove irrelevant or uninformative items, and to examine individual difference correlates of the final measure(s).
Methods
Protocol
We conducted an online study using a sample obtained through Qualtrics Panels. Protocols were approved by the University of Georgia IRB (Project 00003819). In the absence of prior information to guide expectations about the levels of communality and number of factors present in a given battery of items, it is recommended that researchers obtain as large a sample as possible for factor analysis (MacCallum et al., 1999). Therefore, we set a target sample size of 2,000 participants in order to have subsamples of at least 1,000 for exploratory factor analysis (EFA) and confirmatory factor analysis (CFA).
Given the observed relationship between education level and scientific understanding (Miller, 1983), we set quotas to achieve a sample with a diverse range of education levels. In light of limited evidence linking scientific understanding to other demographic factors, as previously discussed, we did not set quotas for other demographic characteristics. Consistent with prior research (Jensen et al., 2017), we dichotomized level of education into two groups: less education (a high school education or less) and more education (completed some college or more). We set quotas so that roughly half of participants would belong to each group. Characteristics of the final sample are reported in Supplementary material 1.
Data screening
Four criteria were used to eliminate responses from participants who were not answering thoughtfully. Cases were removed if participants (a) completed the full survey in under half the median time (i.e., 309 s), (b) completed the 29-item preference instrument in under half the median time (i.e., 54 s), (c) failed the attention check questions, or (d) did not complete the survey. Out of 2,738 cases collected, the final sample contained 2,008 participants.
Measures
Participants first answered the battery of 29 scale items (Box 1). We retained the response set from the original scale (i.e., strongly disagree to strongly agree on a 5-point Likert scale) because it appeared to capture sufficient variance in earlier tests (Ratcliff and Wicke, 2023; Ratcliff et al., 2023). The scale prompt was: “For this set of questions, we're interested in your own preferences, as opposed to what you think the general public wants to know. In general, how much do you agree or disagree with the following statements?” Given the length of the statements and large number of items, we encouraged participants to “Please answer carefully and take your time.”
The measures used to investigate RQ2 and RQ3 are shown in Table 1.
Results
Bivariate correlations between all study variables are presented in Supplementary material 2. Descriptives for all 29 items are reported in Table 2.
Factor analysis
We conducted EFA using one half of the sample (N = 1,004) and CFA using the other half of the sample (N = 1,004). To retain the education quotas within each subsample, we sorted the full sample into two groups (high school education or less vs. some college or more). Within each education level group, we randomly sorted the data and then assigned half the cases to the EFA subsample and half to the CFA subsample.
Bartlett's test of sphericity and the Kaiser–Meyer–Olkin (KMO) measure of sampling adequacy are indicators of whether the data violate statistical assumptions. Data are considered to have acceptable properties if Bartlett's test is statistically significant and the KMO value is above 0.80 (Howard, 2016). We checked Bartlett's test of sphericity and KMO for each subsample. The data did not violate statistical assumptions, and KMO values were similar between the two subsamples, suggesting each had a similar amount of common variance (Lorenzo-Seva, 2022). Statistics for the EFA subsample were X2(406) = 14,746.59, p < 0.001, KMO = 0.92, and for the CFA subsample, X2(406) = 14,759.38, p < 0.001, KMO = 0.94. Therefore, we proceeded with factor analysis using these subsamples.
EFA and item selection
EFA was conducted using SPSS v29. We used the principal axis factoring (PAF) factor analytic method, which provides adequate results in most situations compared to other factor analytic methods and is robust to data non-normality (Howard, 2016). We used oblique (direct oblimin) rotation to allow factors to correlate, as we assumed processes underlying the factors would be correlated. Factor retention decisions were based on the Kaiser criterion (i.e., eigenvalues greater than 1) and a visual examination of the scree plot. Retention of items was guided by the “40-30-20” rule (Howard, 2016); that is, a cutoff factor loading of 0.40 should be used, and the item should not load onto multiple factors >0.30 unless the loading on the primary factor is at least 0.20 higher.
In the initial solution, five factors were extracted. Factor loadings are presented in Supplementary material 3. Three items did not meet retention criteria as they did not load onto any factor ≥0.40 (items 13, 21, and 29). The mean score for item 13 was relatively low compared to related items. This statement may have represented something akin to “guessing” for participants and thus been viewed as more universally undesirable. In contrast, the mean scores for items 21 and 29 were relatively high. These items captured an expectation that scientists should present information in a “simplified” way, and it is possible this was not perceived to be about oversimplification or streamlining but merely simplicity, which is a generally desirable attribute of science communication. Therefore, we removed these items and ran the EFA again.
In this solution, only four factors remained. However, additional items did not meet retention criteria due to problematic cross-loadings: items 3, 6, 8, and 11 had near-equivalent loadings on factors 1 and 3. Notably, items 3, 6, and 8 all contained the phrase “I like to learn about new scientific discoveries”—which could apply to people who prefer information about uncertainty and preliminary science—while the latter portion of each statement was focused specifically on preliminary science, explaining the potential conceptual overlap. Additionally, item 20 had near-equivalent loadings on factors 2 and 4. This item captured negative reactions to “presenting findings as uncertain or preliminary,” which could explain the overlap (however, item 19 included similar phrasing and did not exhibit problematic cross-loading).3 One item (item 17) exhibited very low communality (0.17) relative to the other items but otherwise met retention criteria, so we retained it at this stage.
After removing items 3, 6, 8, 11, and 20, a four-factor solution was extracted with all remaining items meeting criteria for retention. As shown in Box 2, these factors were conceptually aligned with the four dimensions of preference we previously articulated: preferring communication of uncertainty (factor 1, henceforth “Complete”), preferring to learn about preliminary science (factor 3, henceforth “Preliminary”), preferring communication of certainty (factor 4, henceforth “Streamlined”), and preferring to learn about established science (factor 2, henceforth “Established”). Factors were represented by 4–7 items.
As shown in Table 3, factor loadings were within acceptable ranges: Complete (0.69–0.80), Established (0.45–0.83), Preliminary (0.50–0.75), and Streamlined (0.47–0.63). Eigenvalues are reported in Supplementary material 4. The final four-factor instrument explained 62.20% of variance cumulatively, with the Complete and Established factors explaining the bulk of the variance.
As expected, the Complete and Preliminary factors were highly correlated (r = 0.58), and the Streamlined and Established factors were highly correlated (r = 0.51). However, there was no evidence of an inverse relationship between certainty-focused and uncertainty-focused preferences. Certainty preference items did not exhibit negative loadings on the factors representing uncertainty preferences (Table 3). Moreover, the factor correlation matrix for the final solution revealed small positive correlations between the uncertainty- and certainty-focused factors (r = 0.12–0.21; see Table 4). Conceptually, this suggests that certainty-focused and uncertainty-focused preferences are not opposite sides of a spectrum but instead represent discrete dimensions of information preferences.
Construct reliability and validity
We examined additional indicators of construct reliability and validity using the EFA sample and the factor structure suggested by the final EFA (see Box 2 for items included at this stage). Providing evidence of construct reliability for each of the four factors, the retained items within each factor demonstrated good internal consistency (Complete: Cronbach's α = 0.91, Preliminary: α = 0.81, Streamlined: α = 0.76, and Established: α = 0.85). With one exception, reliability for each subscale was not improved by removing any item. But for the Streamlined factor, reliability was slightly improved without item 17 (α = 0.77). Combined items from the Complete and Preliminary factors were internally consistent (α = 0.90), as were combined items from the Streamlined and Established factors (α = 0.86).
The average variance extracted (AVE) can be a useful statistic for assessing divergent and convergent validity. Given the significant correlations between the Complete and Preliminary factors, and between the Streamlined and Established factors, we examined discriminant validity for each pair of factors. Discriminant validity was assessed using a statistical formula proposed by Fornell and Larcker (1981). There is evidence of discriminant validity if the AVE for each construct is greater than the square of the correlation between the factors. In this case, the AVEs for the Complete factor (0.55) and the Preliminary factor (0.45) were greater than the square of the correlation between these factors (R2 = 0.34; see factor correlations in Table 4). The AVEs for the Established factor (0.49) and the Streamlined factor (0.27 with item 17 or 0.28 without it) were greater than the square of the correlation between these factors (R2 = 0.26). This indicates that the two factors within each pair represent distinct dimensions.
Strong evidence of convergent validity within a factor is indicated by an AVE of 0.50 or higher. As shown in the previous paragraph, AVE values were close to 0.50 for the Complete, Preliminary, and Established factors but much lower for the Streamlined factor. Yet Fornell and Larcker (1981) argue the 0.50 criterion is a conservative benchmark, and other indicators, such as composite reliability, are important to consider. Therefore, we used AVE to calculate composite reliability (or construct reliability), which is an alternative measure of internal consistency of scale items similar to Cronbach's alpha. Composite reliability was excellent for the Complete factor (0.90) and good for the Established (0.82) and Preliminary (0.76) factors but suboptimal for the Streamlined factor (0.64 with item 17, 0.60 without it).
Taken together, we interpret these results as initial evidence that each factor is a distinct dimension and that the subscales representing the Complete, Preliminary, and Established factors are valid and reliable indicators of their respective dimensions. The subscale representing the Streamlined factor appears acceptable at this exploratory stage but would benefit from further development (see Discussion).
CFA
To confirm the results of the EFA, we conducted CFA using the other half of the sample (N = 1,004). We tested the factor structure suggested by the EFA and included only the 21 retained items (see Box 2). Structural equal modeling (SEM) was performed in Mplus v8.6 using the maximum likelihood method of estimation. Following recommendations, we used three model fit statistics for analyses: root mean square error of approximation (RMSEA), confirmatory fit index (CFI), and standardized root mean squared residual (SRMR) (Hu and Bentler, 1999; Holbert and Grill, 2015). Conventional standards suggest that cutoffs for strong model fit are RMSEA of 0.06 or lower, CFI of 0.95 or higher, and SRMR of 0.09 or lower, whereas models with RMSEA above 0.09 or CFI below 0.90 are considered a poor fit and should not be evaluated (Holbert and Grill, 2015). The chi-square distribution test can be overly sensitive when a sample size is large, but it remains a useful index for model comparison, with lower values being a comparative indicator of better fit (Holbert and Grill, 2015). Therefore, we report and assess the chi-square distribution test for each model.
We initially tested a single-order, four-factor model, which treats each factor as a separate unidimensional construct. We specified the 21 items to load onto their respective factors (see Box 2) and specified correlations between the two uncertainty factors, and between the two certainty factors. The model fit the data relatively well: X2(183) = 874.22, p < 0.001, RMSEA = 0.06 (90% CI: 0.057, 0.065), CFI = 0.93, SRMR = 0.06, X2/df ratio = 4.78. Modification indices suggested fit would be improved if the error terms for items 27 and 28 were allowed to correlate. Additionally, item 17 exhibited a small loading (0.33) compared to all other items. This item had low communality in the EFA and, as previously noted, removing it slightly improved internal consistency for the Streamlined factor. Similar to other removed items, item 17 may capture a preference for simple (as opposed to streamlined) science communication. Therefore, we removed it from the model.
Removing item 17 and allowing error terms of items 27 and 28 to correlate improved model fit: X2(163) = 658.30, p < 0.001, RMSEA = 0.06 (90% CI: 0.051, 0.059), CFI = 0.95, SRMR = 0.05. The X2/df ratio was 4.04. This model yielded a good fit to the data, which we interpret as evidence confirming the factor structure suggested by the EFA. The model diagram is depicted in Figure 1.
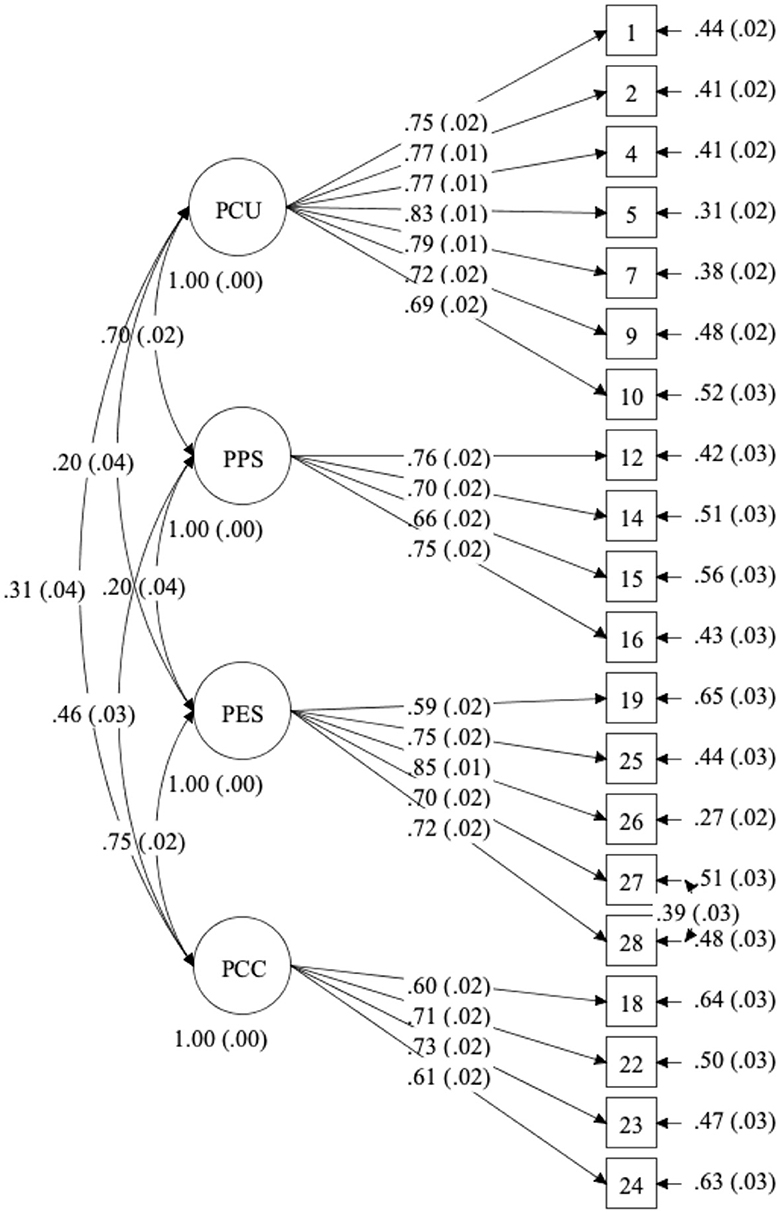
Figure 1. Single-order four-factor model. PCU, prefer communication of uncertainty (i.e., complete); PPS, prefer preliminary science; PES, prefer established science; PCC, prefer communication of certainty (i.e., streamlined).
We also tested alternative models, all without item 17. Given correlations between the two uncertainty factors, and between the two certainty factors, we tested a single-order model with all items from the Complete and Preliminary factors loading onto a single latent construct, as well as a single-order model with all items from the Streamlined and Established factors loading onto a single latent construct. Fit statistics for the unidimensional uncertainty model were: X2(44) = 730.16, p < 0.001, RMSEA = 0.13 (90% CI: 0.117, 0.133), CFI = 0.88, SRMR = 0.07, X2/df ratio = 16.59. Fit statistics for the unidimensional certainty model were: X2(27) = 490.26, p < 0.001, RMSEA = 0.13 (90% CI: 0.121, 0.141), CFI = 0.87, SRMR = 0.06, X2/df ratio = 18.16. In both cases, model fit was comparatively worse than previous models.
A second-order model with all four factors loading onto a single latent variable was also a poor fit to the data, X2(166) = 1136.74, p < 0.001, RMSEA = 0.08 (90% CI: 0.072, 0.081), CFI = 0.90, SRMR = 0.10, X2/df ratio = 6.85. Given the low correlations between the certainty- and uncertainty-focused factors observed in the EFA, this outcome was not surprising.
However, two second-order models—one with the Complete and Preliminary factors loading onto a second-order latent variable, and one with the Streamlined and Established factors loading onto a second-order latent variable—demonstrated excellent fit. To allow for an identified two-factor model, the loading of both first-order factors on the second-order factor was fixed to 1. Model fit statistics for the uncertainty preference second-order model were X2(43) = 218.56, p < 0.001, RMSEA = 0.06 (90% CI: 0.056, 0.072), CFI = 0.97, SRMR = 0.04, X2/df ratio = 5.08. Fit statistics for the certainty preference second-order model (with correlated error terms for items 27 and 28) were X2(25) = 92.10, p < 0.001, RMSEA = 0.05 (90% CI: 0.041, 0.063), CFI = 0.98, SRMR = 0.03, X2/df ratio = 3.68. In all, these models were comparable to the final single-order, four-factor model in terms of fit, but these second-order models appeared to be a slightly stronger fit to the data. Model diagrams are depicted in Figures 2A, B.
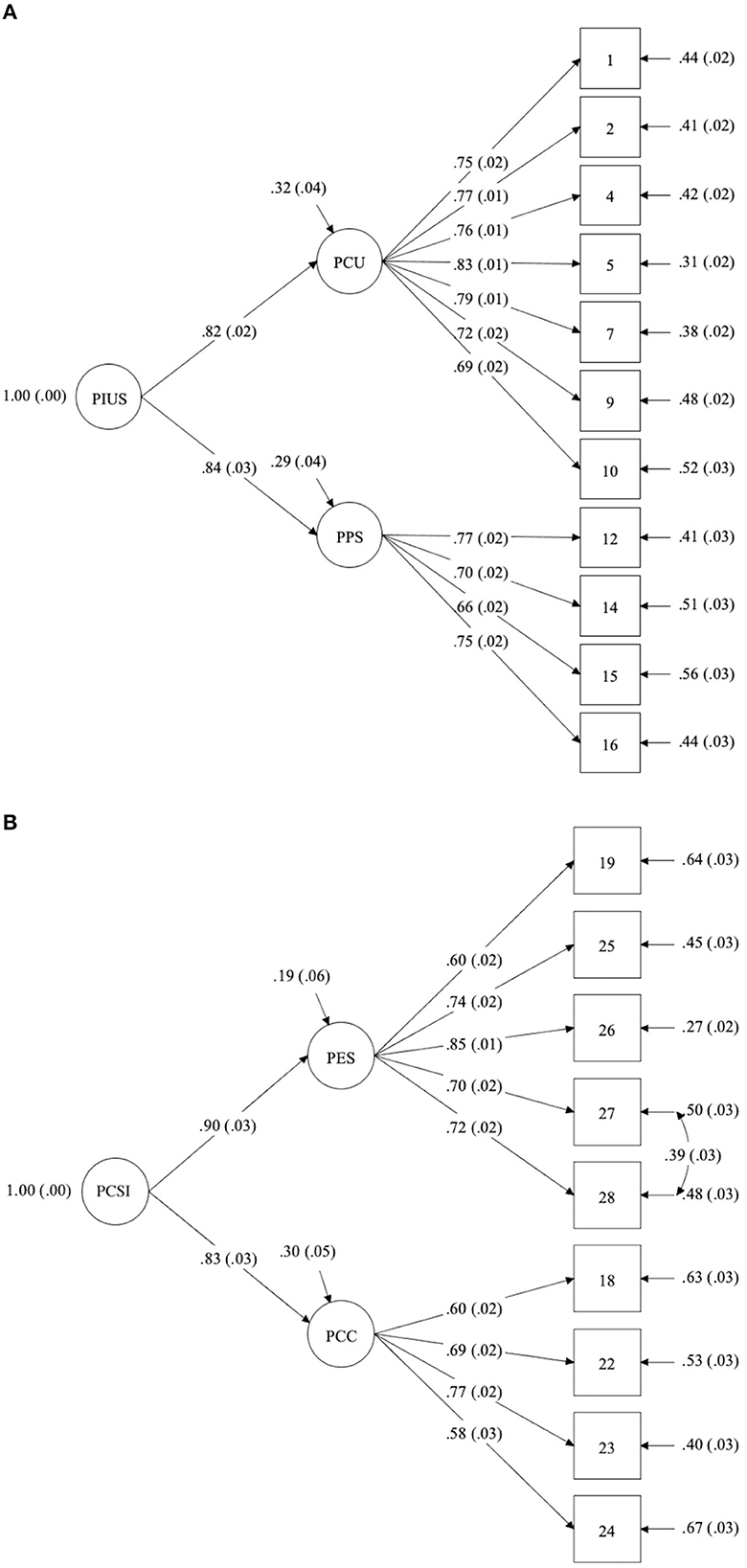
Figure 2. (A) Second-order factor structure of PIUS scale. (B) Second-order factor structure of PCSI scale. PCU, prefer communication of uncertainty (i.e., complete); PPS, prefer preliminary science; PES, prefer established science; PCC, prefer communication of certainty (i.e., streamlined).
Answering RQ1 by taking into account the results of CFA, EFA, and additional tests of construct reliability and validity, we find strong evidence for two second-order latent constructs, each represented by two discrete dimensions. To this end, we believe it makes the most sense to divide the final measure into two separate scales: 11 items capturing Preference for Information about Uncertain Science (henceforth PIUS-11, comprising the Complete and Preliminary subdimensions) and 9 items capturing Preference for Certain Science Information (henceforth PCSI-9, comprising the Streamlined and Established subdimensions). Descriptives for the final subscales, based on the full study sample, are presented in Table 5.
An important question is whether the subscales comprising PIUS, or comprising PCSI, can be combined and treated as unidimensional for analyses. The aforementioned results suggest that the Complete and Preliminary subscales can be analyzed as separate variables or combined, depending on the researcher's interests. The same can be said for the Streamlined and Established subscales. Alternatively, PIUS-11 or PCSI-9 could be modeled as a second-order latent construct with first-order factors allowed to correlate.
Supplemental item response theory (IRT) analysis
IRT is a family of latent variable measurement models that can provide useful insights for scale construction above and beyond what can be gleaned from factor analysis (Edwards, 2009). IRT can help researchers determine which items are a good fit and which provide more or less information—that is, which are uniquely informative (vs. redundant) and which appear to best capture the construct. Given this, we conducted a supplemental IRT analysis using the full sample of 2,008 cases. Using the Winsteps software package, we fitted a Rasch model (specifically, a partial credit model) to the data. Because this model assumes unidimensionality and the EFA and CFA results showed that the certainty and uncertainty items formed distinct dimensions of preference, we fitted two separate models: one for the uncertainty data (items 1–16) and one for the certainty data (items 17–29). Overall, results aligned with the factor analyses, providing evidence of good fit for all retained items and showing that removed items were, indeed, performing poorly. We provide these results in Supplementary material 5.
In summary, results of the IRT analysis corroborated the EFA and CFA results, leading us to keep the 11-item PIUS scale and 9-item PCSI scale as shown in Box 2.
Correlates of preferences
RQ2 and RQ3 explore audience characteristics that relate to and may influence science information preferences. To answer RQ2, we examined relationships between each of the four preference dimensions and scientific understanding (i.e., epistemic beliefs, factual science literacy, and understanding a scientific study), trust in science (i.e., trust in scientists and deference to science), and need for cognitive closure. To answer RQ3, we examine relationships between each of the four preference dimensions and sociodemographic factors (i.e., age, gender, education, race, ethnicity, religiosity, political party affiliation, and political ideology). Pearson's correlations are reported in Table 6 and a full correlation matrix is presented in Supplementary material 2. We summarize key patterns below. Given the large sample size, we focus our interpretation on correlations with p < 0.001.
Answering RQ2, scientific understanding aligned with preferences in expected and unexpected ways. Factual science literacy correlated positively with uncertainty preferences (Complete and Preliminary) and negatively with certainty preferences (Streamlined and Established). Along the same lines, having sophisticated epistemic beliefs was positively correlated with both types of uncertainty preferences, and having simple epistemic beliefs was positively correlated with both types of certainty preferences. Overall, these patterns are in expected directions. However, having simple epistemic beliefs was not inversely correlated with preferring uncertainty, and having sophisticated epistemic beliefs was not inversely correlated with preferring certainty, as one might expect. Self-reported understanding of a scientific study correlated positively with both uncertainty preferences (Complete and Preliminary) but also Streamlined, while it was unrelated to Established preference.
All four dimensions correlated positively with deference to science and trust in scientists. In other words, trust in experts and the scientific enterprise seems to associate with stronger preferences for, and openness to, scientific information of any kind (whether certain or uncertain). Similarly, all four dimensions correlated positively with having a higher need for cognitive closure, suggesting that need for closure, too, drives a greater desire for scientific information of any kind (whether certain or uncertain).
Answering RQ3, education correlated positively with both uncertainty preference dimensions (Complete and Preliminary)—resembling aforementioned results for scientific understanding—but bore no relationship to certainty preferences (Streamlined and Established). Being older, Republican, and politically conservative correlated with lower interest in preliminary science, while being Hispanic related to higher interest. Identifying as Republican also correlated with lower preference for streamlined communication. Interestingly, there was a small positive correlation between identifying as religious and all four preference dimensions. There were minimal relationships between preferences and a person's gender or race.
Synthesizing these results, we present an initial typology of each preference dimension and factors that shape these preferences (Box 3), based on the final set of items that represent each dimension and the individual difference variables that correlate with each dimension.
Discussion
As Scheufele (2018, p. 1123) argued, “Understanding different publics for science communication is more important than ever before.” It is tempting to ascribe universal truths to the public—to say that public audiences expect uncertainty as a normal part of science communication, or that public audiences are unable to understand this inherent uncertainty and expect concrete communication of scientific conclusions. Yet our research presents evidence that public audiences expect both, to varying degrees and depending on a range of individual characteristics. This builds on prior observations, in which nonexperts expressed a range of expectations and preferences for certain or uncertain scientific information (e.g., Frewer et al., 2002; Maier et al., 2016; Post et al., 2021; Ratcliff and Wicke, 2023), underscoring that instead of asking whether the public wants information about scientific uncertainty, it is more useful to ask “who prefers communication of uncertainty, who does not, and why?”
To begin answering these questions, the current project put forward a set of measures for capturing individual preferences for certain and uncertain science information. Previous measures have consisted of only a few items (Post et al., 2021) or were specific to one scientific issue, such as food risks (Frewer et al., 2002), cancer (Carcioppolo et al., 2016), or medical decision making (Han et al., 2009). Building on these measures, our purpose was to examine the validity and utility of a more comprehensive, general measure. We sought to explore whether individuals have general information preferences when it comes to scientific uncertainty, just as a person can have a general disposition toward uncertainty (Hillen et al., 2017) even though their reactions to uncertainty may also vary to some degree depending on the context (Brashers, 2001). Further, we sought to determine whether preference for certain science information is distinguishable from dispositional intolerance of uncertainty; more specifically, a need for cognitive closure (Kruglanski, 1990).
Results of this study provide evidence of construct validity for measures of four distinct dimensions of information preferences. Two of these dimensions (preferring complete information and openness to preliminary science) mapped onto a latent construct representing a preference for receiving information about uncertain science, and these together formed the final PIUS 11-item scale. The other two dimensions (preferring streamlined information and desiring only established science) mapped onto a latent construct representing a preference for receiving certain science information, and these together formed the final PCSI 9-item scale. As we discuss below, results of this study go far in illuminating the nature of preferences for certain and uncertain science information, explicating each as a multifaceted construct that is likely influenced by a range of individual factors.
The nature of (un)certainty information preferences
As previously noted, desiring communication of uncertainty does not indicate a preference for being in a state of uncertainty, but for learning about scientific uncertainty (or the uncertainty of others). In contrast, a preference for communication of certainty captures a desire for streamlined and definitive information. Overall, our results show that some individuals prefer streamlined science information while others want the “full picture,” corroborating results of other studies (e.g., qualitative research by Maier et al., 2016). As reported in Table 5, participants were slightly more inclined to prefer communication of uncertainty and preliminary science than certainty and established science.
Somewhat surprisingly, our study found that preferences for certain and uncertain information formed orthogonal constructs, rather than representing opposite sides of a spectrum. The preference dimensions comprising PIUS and PCSI were not inversely correlated, but rather slightly positively correlated (see Table 4). This suggests that individuals may simultaneously hold conflicting desires for communication of certainty and uncertainty about scientific evidence. We observed a similar pattern in individuals' epistemic beliefs: whereas simple and sophisticated epistemic beliefs are thought to capture opposite expectations of science (i.e., as stable or evolving), these exhibited a small positive correlation (r = 0.09, p < 0.001; see Supplementary material 2). This further illustrates the possibility that some individuals hold seemingly contradictory expectations of science, which could drive conflicting expectations of science communication—a possibility that warrants further investigation.
In the meantime, we take these results as an indication that preferences for certain and uncertain science information should be assessed using separate measures, rather than reversing certainty-focused items to represent uncertainty preferences or vice versa. Patterns that emerged in the relationships between preferences and individual differences further support this perspective, as we describe below.
Correlates of (un)certainty information preferences
Information preferences were clearly related to scientific understanding. Having sophisticated epistemic beliefs, higher factual science literacy, and a stronger understanding of the concept of a scientific study all correlated positively with preferring uncertainty (that is, wanting to receive complete information and to learn about preliminary science). In a similar vein, higher factual science literacy was negatively associated with preferring streamlined communication and information about established science, while having simple epistemic beliefs was positively associated with these preferences. Yet self-reported understanding of the concept of a scientific study also correlated with preferring streamlined communication. One possible interpretation is that subjective understanding really captures interest in science, while objective understanding (insofar as this is represented by the factual science literacy measure) better reflects a person's scientific understanding. More scientifically literate individuals may have a better ability to make sense of uncertainty (thus being more open to it) and an expectation of it as being inherent to science (thus preferring it to be communicated). Individuals who are interested in science—regardless of their beliefs about its nature as stable or evolving—may respond favorably to any type of science communication. In other words, having strong information preferences (that is, high scores on any of the four dimensions) might be driven by an interest in science.
Deference to science and trust in scientists were also positively correlated with all four preference dimensions, suggesting that faith in science and scientists may predispose someone to respond favorably to any type of science communication. As another possibility, faith in science may lead some individuals to be more tolerant of uncertainty but lead others to expect absolute truths and want scientists to distill complex science information into concrete and actionable insights. Fascinatingly, deference to science and trust in scientists were strongly correlated with having simple epistemic beliefs (r = 0.54 and 0.42, respectively; see Supplementary material 2).
All four preference dimensions also correlated positively with having a higher need for cognitive closure, suggesting that need for closure, too, drives a desire for information of any kind. Alternatively, it could be that need for closure drives some people to want certain information and others to want uncertain information. Need for cognitive closure is defined as a desire for “an answer on a given topic, any answer, … compared to confusion and ambiguity” (Kruglanski, 1990, p. 337). Perhaps for some high-need for closure individuals, having “all the information” (even information about uncertainty) reduces ambiguity about the state of the evidence. Other high-need for closure individuals may prefer simple and definitive scientific conclusions (even if prematurely) as a means of reducing ambiguity. Other factors, such as those related to scientific understanding, may influence whether a need for closure drives someone to want certainty or uncertainty communicated. Although need for closure captures one form of ambiguity aversion (Webster and Kruglanski, 1994), other measures of uncertainty (in)tolerance may prove useful to examine in further unpacking this relationship, as they may exhibit different relationships with PIUS and PCSI.
For exploratory purposes, we also examined relationships between preferences and sociodemographic variables. Preferences were largely unrelated to demographic factors such as age, race, ethnicity, and gender. However, education was positively correlated with preferring Complete and Preliminary Science information. This aligns with findings from prior research, in which individuals with a high school education or less reacted unfavorably to communication of scientific uncertainty while those with more than a high school education did not (Jensen et al., 2017; Adams et al., 2023). This may simply reflect a link between education and scientific understanding, which was also observed in our data (see correlations in Supplementary material 2). Interestingly, however, education was not related to certainty preferences.
Republicans had less interest in preliminary science, yet also expressed lower preference for streamlined information. Potentially, this is explained by a lower deference to science among Republicans than Democrats (Blank and Shaw, 2015), which can be seen in our data (see Supplementary material 2). Interestingly, there was a small positive correlation between identifying as religious and all four dimensions. Our measure assessed belonging to an “organized religion, faith or spiritual community” (see Table 6), making this finding surprising, since Rutjens et al. (2022) found spirituality to be a strong predictor of science skepticism. It could prove insightful to further examine relationships among science skepticism, political ideology/party affiliation, religiosity/spirituality, and preferences for certain or uncertain scientific information.
Synthesizing these observations, the results suggest two things: first, that preferences for (un)certain scientific information are distinct constructs from need for closure, scientific understanding, trust in science, and education (given small to medium correlations), and second, that each dimension of preference is shaped by a unique combination of these (and perhaps other) factors. In all, this highlights the complexity of individual uncertainty management strategies. More work to understand the complex interplay of motivations underlying each type of preference, perhaps through qualitative interviews, could help to further contextualize these results. In the interim, we present an initial typology in Box 3.
Practical and theoretical implications
The key to effective science communication is knowing your audience (van der Bles et al., 2019). Understanding (un)certain science information preferences, as well as the individual characteristics that correlate with these preferences, can help health and science communicators design messages that resonate with different audience groups.
Although our results show that information preferences vary, it is generally advisable to communicate uncertainty when it exists, because transparency earns public trust (Jensen et al., 2013; Blastland et al., 2020). Communicating unwarranted certainty can backfire and harm the credibility of institutions, as we saw during the COVID-19 pandemic (Caulfield et al., 2020; Ratcliff et al., 2022). Yet it may be possible to develop uncertainty messages that are received favorably by those who prefer certainty. The PCSI and PIUS scales presented here can be useful for investigating this possibility. One promising avenue will be to examine whether certain types of “normalizing” frames—which depict uncertainty (Han et al., 2021; Simonovic and Taber, 2022), conflicting or evolving evidence (Nagler et al., 2023), and even “failure” (Ophir and Jamieson, 2021) as a normal and healthy part of science—lead to more favorable reception of uncertainty by those who prefer certain science information. Ideally, such frames could highlight the value in knowing about scientific uncertainty from a citizen's perspective, or equip nonscientists with ways to manage or make sense of scientific uncertainty.
The PCSI and PIUS measures could also facilitate the development of much needed predictive theories of uncertainty communication effects. Despite ongoing calls for theory about the effects of communicated uncertainty (e.g., Hurley et al., 2011; Paek and Hove, 2020), we still lack theory to explain how people respond to information about scientific uncertainty in mass mediated contexts (Sopory et al., 2019; Ratcliff et al., 2022; Simonovic et al., 2023). Potentially, the PIUS and PCSI scales can help researchers to gain a better understanding of what types of communication approaches work effectively for individuals with different preference types, and why.
Limitations and future directions for scale development
Although the PIUS-11 and PCSI-9 scales exhibited good psychometric properties overall, there are several potential areas for improvement of the instruments. First, long statements may be a limitation of some scale items. In order to create items that captured the complex concepts of interest with as much precision as possible, many of the item statements used compound sentences. Using similar language and parallel stems, where possible, may have helped to reduce cognitive load. But it could be useful to test shorter versions of the statements, ideally developed in consultation with a science or health literacy expert.
Second, the final subscales for Preliminary and Streamlined preferences contained just four items, and these each explained only a small amount of variance. Although having between 4-7 items is considered ideal for a scale (Lozano et al., 2008), MacCallum et al. (1999) note that 6-7 is generally better than 3–4, and this may be especially true for complex constructs such as those in question here. Adding additional items to the four-item subscales may further improve reliability and strengthen validity. Results of the EFA and CFA suggested that the factor representing Streamlined preferences, in particular, may be underdetermined. Many of the items intended to capture Preliminary and Streamlined preferences were excluded due to problematic cross-loadings (e.g., items 3, 6, and 8, intended to capture Preliminary preferences) or statements that were likely worded too vaguely, such that they specified more universally desirable or undesirable attributes than we had intended (e.g., items 17, 21, 29, intended to capture Streamlined preferences). These could be revised and reintroduced into the scale for further testing. Additionally, items 19 and 20 may have tapped both the Streamlined and Established dimensions. These items could be revised to represent aversion to portrayal of science as either “uncertain” or “preliminary” and included in the respective subscale.
Whereas the PIUS and PCSI scales can be useful for getting a general sense of a person's information preferences, we also recognize that preferences for (un)certain information will naturally depend, to some extent, on the specific context. Prior research suggests that people's information preferences regarding uncertain science are also influenced by their prior issue beliefs (Gustafson and Rice, 2020; Kelp et al., 2022). Preferences for (un)certain information may also be influenced by the severity of the issue and its relevance to the individual (see discussion in Ratcliff et al., 2023). Just as scales exist to examine individual preferences for information about uncertainty in the contexts of medical decision-making (Han et al., 2009), cancer (Carcioppolo et al., 2016), and food safety (Frewer et al., 2002), the PIUS and PCSI scales could be adapted to investigate information preferences for a specific scientific domain (e.g., COVID-19 or climate science) or to compare preferences between domains (e.g., high vs. low stakes issues or controversial vs. non-controversial issues). Future research could also include open-ended questions to ask participants whether specific cases or contexts, such as medical science, came to mind when they completed the measures. It may also be insightful to examine relationships between PIUS and PCSI and health-specific scales such as medical ambiguity aversion (Han et al., 2009).
Research has shown that public audiences tend to be least tolerant of information about conflicting evidence (i.e., “consensus uncertainty”; Gustafson and Rice, 2020; Nagler et al., 2020; Iles et al., 2022). With the exception of item 25, our proposed scales do not directly capture preferences related to information about consensus uncertainty. Identifying whether preferences differ for different types of conflicting scientific information (Iles et al., 2022) compared to other types of scientific uncertainty (e.g., technical, deficient, or epistemic uncertainty; Gustafson and Rice, 2020; Ratcliff, 2021) is an important next step. Researchers could develop additional items (perhaps as part of the Established subscale of PCSI) or create a separate scale altogether.
Lastly, the simultaneous existence of conflicting preferences—as demonstrated in the orthogonality of the PIUS and PCSI constructs and the contradictory correlates of preferences—suggests untapped complexity. Individuals' uncertainty management strategies are often layered and nuanced (Brashers, 2001), and preferences expressed in abstraction are unlikely to fully capture this complexity. We believe the proposed PIUS and PCSI scales represent a promising start toward understanding diverse preferences for (un)certain scientific information among public audiences, yet there is much more complexity to tease out moving forward.
Data availability statement
The dataset presented in the study is publicly available. This data can be found at: https://osf.io/5rynm/?view_only=3bd1d1d1c81447e8be2295e467903524.
Ethics statement
The study involving humans was approved by University of Georgia Institutional Review Board. The study was conducted in accordance with the local legislation and institutional requirements. The participants provided their written informed consent to participate in this study.
Author contributions
CR, BH, and RW conceptualized and designed the study and wrote the manuscript. CR supervised the data collection and performed the data analyses. All authors contributed to the article and approved the submitted version.
Funding
Data collection was supported by the University of Georgia Owens Institute for Behavioral Research.
Acknowledgments
We are grateful to Ye Yuan for assisting with data analysis and to Alice Fleerackers and the reviewers for helpful comments on the paper.
Conflict of interest
The authors declare that the research was conducted in the absence of any commercial or financial relationships that could be construed as a potential conflict of interest.
Publisher's note
All claims expressed in this article are solely those of the authors and do not necessarily represent those of their affiliated organizations, or those of the publisher, the editors and the reviewers. Any product that may be evaluated in this article, or claim that may be made by its manufacturer, is not guaranteed or endorsed by the publisher.
Supplementary material
The Supplementary Material for this article can be found online at: https://www.frontiersin.org/articles/10.3389/fcomm.2023.1245786/full#supplementary-material
Footnotes
1. ^For example, uncertainty tolerance had no moderating effect in Simonovic and Taber (2022) and education was not a significant moderator in Ratcliff et al. (2023).
2. ^The original items from Ratcliff and Wicke (2023) used the word “guess,” which may have the potential for unintended negative interpretation (e.g., signaling carelessness or a complete lack of evidence). Therefore, we revised these items by replacing “guess” with the phrases “educated judgements” and “experts' best interpretation” (items 22 and 24 in Box 1).
3. ^In light of the results observed here, it may be helpful to reword these statements to focus on either the “uncertain” or the “preliminary” aspect when using these items in the future.
References
Adams, D. R., Ratcliff, C. L., Pokharel, M., Jensen, J. D., and Liao, Y. (2023). Communicating scientific uncertainty in the early stages of the COVID-19 pandemic: a message experiment. Risk Anal. doi: 10.1111/risa.14256. [Epub ahead of print].
Biesecker, B. B., Klein, W., Lewis, K. L., Fisher, T. C., Wright, M. F., Biesecker, L. G., et al. (2014). How do research participants perceive “uncertainty” in genome sequencing? Genet. Med. 16, 977–980. doi: 10.1038/gim.2014.57
Binder, A. R., Hillback, E. D., and Brossard, D. (2016). Conflict or caveats? Effects of media portrayals of scientific uncertainty on audience perceptions of new technologies. Risk Anal. 36, 831–846. doi: 10.1111/risa.12462
Blank, J. M., and Shaw, D. (2015). Does partisanship shape attitudes toward science and public policy? The case for ideology and religion. Ann. Am. Acad. Pol. Soc. Sci. 658, 18–35. doi: 10.1177/0002716214554756
Blastland, M., Freeman, A. L. J., van der Linden, S., Marteau, T. M., and Spiegelhalter, D. (2020). Five rules for evidence communication. Nature 587, 362–364. doi: 10.1038/d41586-020-03189-1
Brashers, D. E. (2001). Communication and uncertainty management. J. Commun. 51, 477–497. doi: 10.1111/j.1460-2466.2001.tb02892.x
Bromme, R., and Goldman, S. R. (2014). The public's bounded understanding of science. Educ. Psychol. 49, 59–69. doi: 10.1080/00461520.2014.921572
Brossard, D., and Nisbet, M. C. (2007). Deference to scientific authority among a low information public: understanding U.S. opinion on agricultural biotechnology. Int. J. Public Opin. Res. 19, 24–52. doi: 10.1093/ijpor/edl003
Carcioppolo, N., Yang, F., and Yang, Q. (2016). Reducing, maintaining, or escalating uncertainty? The development and validation of four uncertainty preference scales related to cancer information seeking and avoidance. J. Health Commun. 21, 979–988. doi: 10.1080/10810730.2016.1184357
Caulfield, T., Bubela, T., Kimmelman, J., and Ravitsky, V. (2020). Let's do better: public representations of COVID-19 science. FACETS 6, 403–423. doi: 10.1139/facets-2021-0018
Clarke, C. E., Dixon, G., Holton, A., and McKeever, B. W. (2015). Including “evidentiary balance” in news media coverage of vaccine risk. Health Commun. 30, 461–472. doi: 10.1080/10410236.2013.867006
Drummond, C., and Fischhoff, B. (2017). Individuals with greater science literacy and education have more polarized beliefs on controversial science topics. Proc. Nat. Acad. Sci. 114, 9587–9592. doi: 10.1073/pnas.1704882114
Dumas-Mallet, E., Smith, A., Boraud, T., and Gonon, F. (2018). Scientific uncertainty in the press: how newspapers describe initial biomedical findings. Sci. Commun. 40, 124–141. doi: 10.1177/1075547017752166
Dunwoody, S., and Kohl, P. A. (2017). Using weight-of-experts messaging to communicate accurately about contested science. Sci. Commun. 39, 338–357. doi: 10.1177/1075547017707765
Edwards, M. C. (2009). An introduction to item response theory using the need for cognition scale. Soc. Personal. Psychol. Compass 3, 507–529. doi: 10.1111/j.1751-9004.2009.00194.x
Einsiedel, E. F., and Thorn, B. (1999). “Public responses to uncertainty,” in Communicating Uncertainty: Media Coverage of New and Controversial Science, eds S. M. Friedman, S. Dunwoody, and C. L. Rogers (Mahwah, NJ: Lawrence Erlbaum Associates), 43–57.
Fornell, C., and Larcker, D. F. (1981). Evaluating structural equation models with unobservable variables and measurement error. J. Mark. Res., 18, 39. doi: 10.1177/002224378101800313
Frewer, L. J., Miles, S., Brennan, M., Kuznesof, S., Ness, M., and Ritson, C. (2002). Public preferences for informed choice under conditions of risk uncertainty. Public Underst. Sci. 11, 363–372. doi: 10.1088/0963-6625/11/4/304
Friedman, S. M., Dunwoody, S., and Rogers, C. L, . (eds) (1999). Communicating Uncertainty: Media Coverage of New and Controversial Science. Mahwah, NJ: Lawrence Erlbaum Associates.
Greiner Safi, A., Kalaji, M., Avery, R., Niederdeppe, J., Mathios, A., Dorf, M., et al. (2023). Examining perceptions of uncertain language in potential e-cigarette warning labels: Results from 16 focus groups with adult tobacco users and youth. Health Commun. doi: 10.1080/10410236.2023.2170092. [Epub ahead of print].
Guenther, L., and Ruhrmann, G. (2016). Scientific evidence and mass media: investigating the journalistic intention to represent scientific uncertainty. Public Underst. Sci. 25, 927–943. doi: 10.1177/0963662515625479
Gustafson, A., and Rice, R. E. (2019). The effects of uncertainty frames in three science communication topics. Sci. Commun. 41, 679–706. doi: 10.1177/1075547019870811
Gustafson, A., and Rice, R. E. (2020). A review of the effects of uncertainty in public science communication. Public Underst. Sci. 29, 614–633. doi: 10.1177/0963662520942122
Han, P. K. J., Klein, W. M. P., and Arora, N. K. (2011). Varieties of uncertainty in health care: a conceptual taxonomy. Med. Decis. Making 31, 828–838. doi: 10.1177/0272989X10393976
Han, P. K. J., Reeve, B. B., Moser, R. P., and Klein, W. M. P. (2009). Aversion to ambiguity regarding medical tests and treatments: measurement, prevalence, and relationship to sociodemographic factors. J. Health Commun. 14, 556–572. doi: 10.1080/10810730903089630
Han, P. K. J., Scharnetzki, E., Scherer, A. M., Thorpe, A., Lary, C., Waterston, L. B., et al. (2021). Communicating scientific uncertainty about the COVID-19 pandemic: online experimental study of an uncertainty-normalizing strategy. J. Med. Internet Res. 23, e27832. doi: 10.2196/27832
Hendriks, F., Kienhues, D., and Bromme, R. (2016). Disclose your flaws! Admission positively affects the perceived trustworthiness of an expert science blogger. Stud. Commun. Sci. 16, 124–131. doi: 10.1016/j.scoms.2016.10.003
Hillen, M. A., Gutheil, C. M., Strout, T. D., Smets, E. M. A., and Han, P. K. J. (2017). Tolerance of uncertainty: conceptual analysis, integrative model, and implications for healthcare. Soc. Sci. Med. 180, 62–75. doi: 10.1016/j.socscimed.2017.03.024
Holbert, R. L., and Grill, C. (2015). Clarifying and expanding the use of confirmatory factor analysis in journalism and mass communication research. Journal. Mass Commun. Q. 92, 292–319. doi: 10.1177/1077699015583718
Howard, M. C. (2016). A review of exploratory factor analysis decisions and overview of current practices: what we are doing and how can we improve? Int. J. Hum. Comput. Interact. 32, 51–62. doi: 10.1080/10447318.2015.1087664
Hu, L., and Bentler, P. M. (1999). Cutoff criteria for fit indexes in covariance structure analysis: conventional criteria versus new alternatives. Struct. Equ. Model. 6, 1–55. doi: 10.1080/10705519909540118
Hurley, R. J., Kosenko, K. A., and Brashers, D. (2011). Uncertain terms: message features of online cancer news. Commun. Monogr. 78, 370–390. doi: 10.1080/03637751.2011.565061
Iles, I. A., Gillman, A. S., O'Connor, L. E., Ferrer, R. A., and Klein, W. M. (2022). Understanding responses to different types of conflicting information about cancer prevention. Soc. Sci. Med. 311, 115292. doi: 10.1016/j.socscimed.2022.115292
Jensen, J. D., Krakow, M., John, K. K., and Liu, M. (2013). Against conventional wisdom: when the public, the media, and medical practice collide. BMC Med. Inform. Decis. Mak. 13, 1–7. doi: 10.1186/1472-6947-13-S3-S4
Jensen, J. D., Pokharel, M., Scherr, C. L., King, A. J., Brown, N., and Jones, C. (2017). Communicating uncertain science to the public: how amount and source of uncertainty impact fatalism, backlash, and overload. Risk Anal. 37, 40–51. doi: 10.1111/risa.12600
Kelp, N. C., Witt, J. K., and Sivakumar, G. (2022). To vaccinate or not? The role played by uncertainty communication on public understanding and behavior regarding COVID-19. Sci. Commun. 44, 223–239. doi: 10.1177/10755470211063628
Kienhues, D., and Bromme, R. (2012). Exploring laypeople's epistemic beliefs about medicine – a factor-analytic survey study. BMC Public Health 12, 759. doi: 10.1186/1471-2458-12-759
Kimmerle, J., Flemming, D., Feinkohl, I., and Cress, U. (2015). How laypeople understand the tentativeness of medical research news in the media: an experimental study on the perception of information about deep brain stimulation. Sci. Commun. 37, 173–189. doi: 10.1177/1075547014556541
Kruglanski, A. W. (1990). “Motivations for judging and knowing: implications for causal attribution,” in The Handbook of Motivation and Cognition: Foundation of Social Behavior, eds E. T. Higgins, and R. M. Sorrentino, Vol. 2 (New York, NY: Guilford Press), 333–368.
Lorenzo-Seva, U. (2022). SOLOMON: A method for splitting a sample into equivalent subsamples in factor analysis. Behav. Res. Methods 54, 2665–2677. doi: 10.3758/s13428-021-01750-y
Lozano, L. M., García-Cueto, E., and Muñiz, J. (2008). Effect of the number of response categories on the reliability and validity of rating scales. Methodology 4, 73–79. doi: 10.1027/1614-2241.4.2.73
MacCallum, R. C., Widaman, K. F., Zhang, S., and Hong, S. (1999). Sample size in factor analysis. Psychol. Methods 4, 84–99. doi: 10.1037/1082-989X.4.1.84
Maier, M., Milde, J., Post, S., Günther, L., Ruhrmann, G., and Barkela, B. (2016). Communicating scientific evidence: Scientists', journalists' and audiences' expectations and evaluations regarding the representation of scientific uncertainty. Communications 41, 239–264. doi: 10.1515/commun-2016-0010
Mishel, M. H. (1988). Uncertainty in illness. Image J. Nurs. Scholarsh. 20, 225–232. doi: 10.1111/j.1547-5069.1988.tb00082.x
Nagler, R. H., Gollust, S. E., Yzer, M. C., Vogel, R. I., and Rothman, A. J. (2023). Sustaining positive perceptions of science in the face of conflicting health information: an experimental test of messages about the process of scientific discovery. Soc. Sci. Med. 334, 116194. doi: 10.1016/j.socscimed.2023.116194
Nagler, R. H., Vogel, R. I., Gollust, S. E., Rothman, A. J., Fowler, E. F., and Yzer, M. C. (2020). Public perceptions of conflicting information surrounding COVID-19: results from a nationally representative survey of U.S. adults. PLOS ONE 15, e0240776. doi: 10.1371/journal.pone.0240776
National Academies of Sciences Engineering, and Medicine. (2017). Communicating Science Effectively: A Research Agenda. Washington, DC: The National Academies Press.
National Science Board (2020). Science and Technology: Public Attitudes, Knowledge, and Interest. Science and Engineering Indicators 2020. NSB-2020-7. Alexandria, VA. Available online at: https://ncses.nsf.gov/pubs/nsb20207/ (accessed September 1, 2023).
Ophir, Y., and Jamieson, K. H. (2021). The effects of media narratives about failures and discoveries in science on beliefs about and support for science. Public Underst. Sci. 30, 1008–1023. doi: 10.1177/09636625211012630
Paek, H.-J., and Hove, T. (2020). Communicating uncertainties during the COVID-19 outbreak. Health Commun. 35, 1729–1731. doi: 10.1080/10410236.2020.1838092
Post, S., Bienzeisler, N., and Lohöfener, M. (2021). A desire for authoritative science? How citizens' informational needs and epistemic beliefs shaped their views of science, news, and policymaking in the COVID-19 pandemic. Public Underst. Sci. 30, 496–514. doi: 10.1177/09636625211005334
Rabinovich, A., and Morton, T. A. (2012). Unquestioned answers or unanswered questions: beliefs about science guide responses to uncertainty in climate change risk communication: model of science and response to uncertainty. Risk Anal. 32, 992–1002. doi: 10.1111/j.1539-6924.2012.01771.x
Ratcliff, C. L. (2021). Communicating scientific uncertainty across the dissemination trajectory: a precision medicine case study. Sci. Commun. 43, 597–623. doi: 10.1177/10755470211038335
Ratcliff, C. L., Fleerackers, A., Wicke, R., Harvill, B., King, A. J., and Jensen, J. D. (2023). Framing COVID-19 preprint research as uncertain: a mixed-method study of public reactions. Health Commun. doi: 10.1080/10410236.2023.2164954. [Epub ahead of print].
Ratcliff, C. L., and Wicke, R. (2023). How the public evaluates media representations of uncertain science: an integrated explanatory framework. Public Underst. Sci. 32, 410–427. doi: 10.1177/09636625221122960
Ratcliff, C. L., Wicke, R., and Harvill, B. (2022). Communicating uncertainty to the public during the COVID-19 pandemic: a scoping review of the literature. Ann. Int. Commun. Assoc. 46, 260–289. doi: 10.1080/23808985.2022.2085136
Ratcliff, C. L., Wong, B., Jensen, J. D., and Kaphingst, K. A. (2021). The impact of communicating uncertainty on public responses to precision medicine research. Ann. Behav. Med. 55, 1048–1061. doi: 10.1093/abm/kaab050
Retzbach, J., Otto, L., and Maier, M. (2016). Measuring the perceived uncertainty of scientific evidence and its relationship to engagement with science. Public Underst. Sci. 25, 638–655. doi: 10.1177/0963662515575253
Roets, A., and Van Hiel, A. (2011). Item selection and validation of a brief, 15-item version of the Need for Closure Scale. Pers. Individ. Dif. 50, 90–94. doi: 10.1016/j.paid.2010.09.004
Rutjens, B. T., Sengupta, N., Der Lee, R. V., van Koningsbruggen, G. M., Martens, J. P., Rabelo, A., et al. (2022). Science skepticism across 24 countries. Soc. Psychol. Personal. Sci. 13, 102–117. doi: 10.1177/19485506211001329
Scheufele, D. A. (2018). Beyond the choir? The need to understand multiple publics for science. Environ. Commun. 12, 1123–1126. doi: 10.1080/17524032.2018.1521543
Simonovic, N., and Taber, J. M. (2022). Psychological impact of ambiguous health messages about COVID-19. J. Behav. Med. 45, 159–171. doi: 10.1007/s10865-021-00266-2
Simonovic, N., Taber, J. M., Scherr, C. L., Dean, M., Hua, J., Howell, J. L., et al. (2023). Uncertainty in healthcare and health decision making: five methodological and conceptual research recommendations from an interdisciplinary team. J. Behav. Med. 46, 541–555. doi: 10.1007/s10865-022-00384-5
Sopory, P., Day, A. M., Novak, J. M., Eckert, K., Wilkins, L., Padgett, D. R., et al. (2019). Communicating uncertainty during public health emergency events: a systematic review. Rev. Commun. Res. 7, 67–108. doi: 10.12840/ISSN.2255-4165.019
van der Bles, A. M., van der Linden, S., Freeman, A. L., Mitchell, J., Galvao, A. B., Zaval, L., et al. (2019). Communicating uncertainty about facts, numbers and science. R. Soc. Open Sci. 6, 181870. doi: 10.1098/rsos.181870
Webster, D. M., and Kruglanski, A. W. (1994). Individual differences in need for cognitive closure. J. Pers. Soc. Psychol. 67, 1049–1062. doi: 10.1037/0022-3514.67.6.1049
Keywords: science communication, health communication, information preferences, uncertain science, scientific uncertainty, uncertainty tolerance, science literacy, public understanding of science
Citation: Ratcliff CL, Harvill B and Wicke R (2023) Understanding public preferences for learning about uncertain science: measurement and individual difference correlates. Front. Commun. 8:1245786. doi: 10.3389/fcomm.2023.1245786
Received: 23 June 2023; Accepted: 07 November 2023;
Published: 08 December 2023.
Edited by:
Nicole Kelp, Colorado State University, United StatesReviewed by:
Douglas Ashwell, Massey University Business School, New ZealandPaul K. J. Han, National Institutes of Health (NIH), United States
Copyright © 2023 Ratcliff, Harvill and Wicke. This is an open-access article distributed under the terms of the Creative Commons Attribution License (CC BY). The use, distribution or reproduction in other forums is permitted, provided the original author(s) and the copyright owner(s) are credited and that the original publication in this journal is cited, in accordance with accepted academic practice. No use, distribution or reproduction is permitted which does not comply with these terms.
*Correspondence: Chelsea L. Ratcliff, Y2hlbHNlYS5yYXRjbGlmZiYjeDAwMDQwO3VnYS5lZHU=