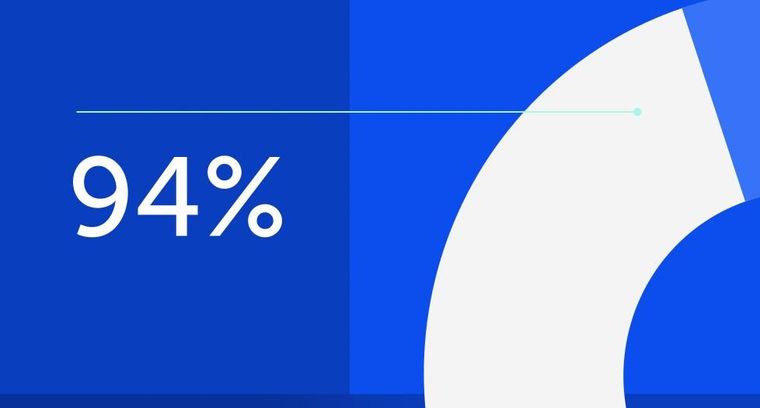
94% of researchers rate our articles as excellent or good
Learn more about the work of our research integrity team to safeguard the quality of each article we publish.
Find out more
ORIGINAL RESEARCH article
Front. Commun., 28 April 2023
Sec. Psychology of Language
Volume 8 - 2023 | https://doi.org/10.3389/fcomm.2023.1148240
This article is part of the Research TopicScience, Technology, and Art in the Spoken Expression of MeaningView all 12 articles
Visual facial information, particularly hyperarticulated lip movements in clear speech, has been shown to benefit segmental speech perception. Little research has focused on prosody, such as lexical tone, presumably because production of prosody primarily involves laryngeal activities not necessarily distinguishable through visible articulatory movements. However, there is evidence that head, eyebrow, and lip movements correlate with production of pitch-related variations. One subsequent question is whether such visual cues are linguistically meaningful. In this study, we compare movements of the head, eyebrows and lips associated with plain (conversational) vs. clear speech styles of Mandarin tone articulation to examine the extent to which clear-speech modifications involve signal-based overall exaggerated facial movements or code-based enhancement of linguistically relevant articulatory movements. Applying computer-vision techniques to recorded speech, visible movements of the frontal face were tracked and measured for 20 native Mandarin speakers speaking in two speech styles: plain and clear. Thirty-three head, eyebrow and lip movement features based on distance, time, and kinematics were extracted from each individual tone word. A random forest classifier was used to identify the important features that differentiate the two styles across tones and for each tone. Mixed-effects models were then performed to determine the features that were significantly different between the two styles. Overall, for all the four Mandarin tones, we found longer duration and greater movements of the head, eyebrows, and lips in clear speech than in plain speech. Additionally, across tones, the maximum movement happened relatively earlier in clear than plain speech. Although limited evidence of tone-specific modifications was also observed, the cues involved overlap with signal-based changes. These findings suggest that visual facial tonal modifications for clear speech primarily adopt signal-based general emphatic cues that strengthen signal saliency.
It is well known that having both audio and video information in a noisy environment, or when talking with non-native speakers or cochlear implant users can help with speech perception and intelligibility (e.g., Sumby and Pollack, 1954; Desai et al., 2008; Wang et al., 2008). In such challenging listening contexts, speakers tend to use a clear, hyperarticulated speech style (relative to plain, conversational style)1 with exaggerated acoustic features such as increased voice intensity, fundamental frequency (F0), duration, and hyper-articulation with more extreme spectral features to help speech intelligibility (Ferguson and Kewley-Port, 2002; Cooke and Lu, 2010; 2007; Krause and Braida, 2004; Smiljanić and Bradlow, 2005; Lu and Cooke, 2008; Hazan and Baker, 2011; Kim and Davis, 2014; Smiljanić, 2021). In addition to enhanced audio features, visual articulatory cues provided by speakers' mouth movements have been found to improve speech intelligibility (Perkell et al., 2002; Traunmüller and Öhrström, 2007; Kim and Davis, 2014), and perception of such visual cues can be further enhanced in clear speech (Gagné et al., 1994, 2002; Helfer, 1997; Lander and Capek, 2013; Van Engen et al., 2014).
While most clear-speech studies focus on speech segments, little research has examined clear-speech effects on prosody (including lexical tone), especially in the visual domain, presumably because prosodic production does not rely on vocal tract configurations and may less likely provide reliable visual speech cues. However, there is evidence that head, jaw, neck, eyebrow, and lip movements may convey visual information in prosodic tonal production and perception (Burnham et al., 2001; Yehia et al., 2002; Munhall et al., 2004; Chen and Massaro, 2008; Attina et al., 2010; Cvejic et al., 2010; Swerts and Krahmer, 2010; Kim et al., 2014). Furthermore, research by our team suggests such movements provide linguistically meaningful cues to signal tonal category distinctions (Garg et al., 2019).
These findings present an interesting case with respect to how these cues are utilized in clear-speech tone modification. On the basis of acoustic characteristics, clear speech has been claimed to involve two levels of modifications (Bradlow and Bent, 2002; Zhao and Jurafsky, 2009; Redmon et al., 2020), namely, signal-based and code-based. Signal-based clear-speech modifications involve changes across the entire speech signal independent of specific sound features, resulting in enhancement of overall signal saliency rather than distinctions of specific speech sounds; e.g., longer duration across vowels (Leung et al., 2016) or higher intensity across lexical tones (Tupper et al., 2021). In contrast, code-based clear-speech changes involve sound-specific modifications resulting in enhancement of phonemic contrasts; e.g., increased F2 for front vowels and decreased F2 for back vowels (Leung et al., 2016) or steeper downward F0 slope for the falling tone (Tupper et al., 2021). Likewise, clear-speech modifications of visual articulatory features may also involve signal-based changes (e.g., greater mouth opening across vowels) vs. code-based changes (e.g., greater horizontal lip stretching for /i/ and greater lip rounding for /u/, Tang et al., 2015). Effective clear-speech modifications must involve coordination of signal- and code-based strategies to enhance as well as preserve phonemic category distinctions (Moon and Lindblom, 1994; Ohala, 1995; Smiljanić and Bradlow, 2009; Tupper et al., 2018; Smiljanić, 2021). Such coordination may be challenging in cases where cues are less definitive in serving code-based functions, as in the case of visual articulatory correlates to lexical tone. As such, lexical tone provides a unique platform for testing these clear-speech principles with respect to the extent to which signal- and code-based visual cues are adopted in visual articulatory clear-speech modifications.
In the present study, we examine how the visual tonal cues identified in Garg et al. (2019) are enhanced in clear speech in the production of Mandarin Chinese tones, using state-of-the-art computer vision, image processing, and machine learning techniques.
Kinematic studies focusing on segmental articulatory features of speech production show that speakers articulate in a more exaggerated manner in adverse listening conditions, presumably to be more intelligible to perceivers (e.g., Tasko and Greilick, 2010; Kim et al., 2011; Kim and Davis, 2014; Garnier et al., 2018). For example, studies using an Optotrak system examined articulatory movements of clear speech produced in noise and in quiet by tracking the motion of face markers as speakers produce English sentences (Kim et al., 2011; Kim and Davis, 2014). The results of these studies revealed increased movements of the jaw and mouth in speech produced in noise (clear speech) compared to that produced in quiet (plain speech). Similarly, using electromagnetic articulography (EMA), Garnier et al. (2018) examined articulatory movements in the production of French CVC words in clear speech produced in noisy environments. They found patterns of hyperarticulation in lip movements in clear (relative to plain) speech, with greater contrasts in lip aperture between low and high vowels, and in lip spreading and protrusion between spread and rounded vowels. In another EMA study, Šimko et al. (2016) examined the production of Slovak syllables containing long and short vowels in noise, allowing the comparison of clear-speech effects on segmental and suprasegmental (durational) features. They found that overall, hyperarticulated speech produced in noise was associated with expansion of movement of the jaw, the lips and the tongue as well as increased utterance duration. Furthermore, suprasegmental-level (durational) modifications associated with jaw opening appeared to be separate from segmental-level modifications associated with lip movements. Studies have also examined tongue movements in clear vs. plain speech production using a midsagittal X-ray microbeam system to track tongue fleshpoints (Tasko and Greilick, 2010). Results revealed that, in clear relative to plain productions of the word-internal diphthong /aI/, the tongue began in a lower position at the onset of diphthong transition (i.e., lowered tongue for /a/) and ended in a higher position at transition offset (i.e., higher tongue position for /I/), indicating that clear speech resulted in significantly larger and longer movements of the tongue toward the target of the vowel components.
Recent research conducted by our team has developed an approach using computerized facial detection and image processing techniques to measure articulatory movements (Tang et al., 2015; Garg et al., 2019). For example, in Tang et al. (2015), we examined front- and side-view videos of speakers' faces while they articulated English words in clear vs. plain speech containing vowels differing in visible articulatory features. The results revealed significant plain-to-clear speech modifications with greater mouth opening across vowels, as well as vowel-specific modifications corresponding to the vowel-inherent articulatory features, with greater horizontal lip stretch for front unrounded vowels (e.g., /i, I/) and greater degree of lip rounding and protrusion for rounded vowels (e.g., /u, ℧/).
Taken together, both kinematic and video-based articulatory studies consistently show hyper-articulation in clear speech, with modifications being both signal-based and generic (e.g., increased mouth opening) and code-based and segment-specific (e.g., greater lip protrusion for rounded vowels).
As discussed previously, although F0 information cannot be directly triggered by vocal tract configurations, movements of the head, jaw, neck, eyebrows, as well as lips have been found to be associated with changes in prosody, including lexical tone (Burnham et al., 2001, 2022; Yehia et al., 2002; Munhall et al., 2004; Chen and Massaro, 2008; Attina et al., 2010; Swerts and Krahmer, 2010; Kim et al., 2014). Further research has revealed that facial movements (e.g., head, eyebrow, lip) in terms of spatial and temporal changes in distance, direction, speed, and timing can be aligned with acoustic features of tonal changes in height, contour, and duration (Attina et al., 2010; Garg et al., 2019).
For prosody in general, movements of the head have been shown to be correlated with F0 changes. Specifically, greater head movements are found in sentences with strong focus (Swerts and Krahmer, 2010; Kim et al., 2014), in stressed syllables (Scarborough et al., 2009), and in interrogative intonation (Srinivasan and Massaro, 2003), suggesting that the magnitude of head motion can be aligned with the amount of F0 variation. In addition to the head, eyebrow movements are also claimed to be associated with prosodic articulation (Yehia et al., 2002; Munhall et al., 2004; Swerts and Krahmer, 2010; Kim and Davis, 2014). For example, focused, accented, and stressed words in a sentence have been found to involve larger vertical eyebrow displacement and higher peak velocity of eyebrow movements (Scarborough et al., 2009; Flecha-García, 2010; Swerts and Krahmer, 2010; Kim et al., 2014), indicating that eyebrow movements may be coordinated with F0 for prosodic contrasts. However, it has been pointed out that the specific connection to F0 changes in terms of height and direction is not straightforward or invariably evident (Ishi et al., 2007; Reid et al., 2015). Moreover, although mouth configurations typically signal segmental rather than prosodic contrasts, there has been evidence that lip movements such as lip opening and lowering may be spatially and temporally aligned with prosodic variations (Dohen and Loevenbruck, 2005; Dohen et al., 2006; Scarborough et al., 2009). For example, using a facial-motion tracking system with retro-reflectors attached to the face (Qualisys), Scarborough et al. (2009) found lip movements to be larger for stressed than unstressed syllables.
Attempts have also been made to identify visible facial cues associated with lexical tone production. In particular, computer-vision research from our team has found that spatial and temporal changes in distance, direction, speed, and timing are related to acoustic features of Mandarin tonal changes in height, contour, and duration (Garg et al., 2019). From tracking head movements, Garg et al. (2019) has revealed that Mandarin high-level tone (Tone 1), which involves minimal F0 variation compared to the other contour tones, exhibits minimal head movements and low movement velocity. These patterns are consistent with previous kinematic sensor-based results showing that head movements (e.g., nodding, tilting, rotation toward the back) are correlated with F0 changes in Cantonese tones (Burnham et al., 2006, 2022). Similar to head movements, the spatial and temporal changes in eyebrow motion also follow the trajectories of F0 height and contour in Mandarin tones. Garg et al. (2019) shows that the magnitude of eyebrow displacement along with its movement velocity is smaller for the level tone as compared to the contour tones, for which the eyebrow movements are aligned with the direction and timing of the rising (Tone 2), dipping (Tone 3), and falling (Tone 4) trajectories. The spatial and temporal events in tone production may also coordinate to mouth movements. For example, compared to the other tones, Tone 4 exhibits the longest time to reach the maximum velocity of lip closing, accompanied by the longest time for the head and the eyebrows to reach maximum lowering, suggesting later lowering movement corresponding to the falling F0 trajectory of this tone (Garg et al., 2019). Findings from sensor-based studies also corroborate a general correlation between lip movements and F0, with lip raising movements corresponding to the high F0 nature of Tone 1 and lip protrusion relating to the rising contour of Tone 2 (Attina et al., 2010).
Together, the findings based on the analyses of head, eyebrow and lip movements reveal linguistically meaningful facial cues in tone articulation. One subsequent question yet to be addressed is whether speakers make use of such cues to modify their speech, such as in clear speech in adverse listening contexts with the intention of enhancing intelligibility. Han et al. (2019) analyzed videos of Mandarin tone production teaching (clear) style by four Mandarin instructors. They found a greater total amount of facial movements and longer durations in clear relative to natural (plain) speech. There were also tone-specific differences, with greater horizontal movements for the high-level tone and greater vertical movements for the rising and falling tones in clear than plain speech. However, the measures were limited to the three general facial movement measures (total amount, horizontal, vertical) and were not associated with particular facial regions (e.g., eyebrows, lips) as revealed by other research (Attina et al., 2010; Garg et al., 2019). It is thus unclear whether the exaggerated facial movements observed in clear speech are associated with code-based linguistically meaningful tonal cues identified previously.
In this study, we compare movements of the head, eyebrows and lips associated with clear vs. plain speech styles of Mandarin tone articulation. The comparisons are based on a comprehensive set of static (distance- and time-based) cues as well as dynamic (kinematic-based) cues identified to characterize different Mandarin tone categories in our previous research (Garg et al., 2019).
Mandarin tone provides a unique case in examining clear-speech characteristics in articulation. As mentioned previously, the articulation of tone primarily involves laryngeal activities not necessarily distinguishable through visible articulatory movements. It is thus unclear if clear-speech modifications involve exaggerated signal-based facial movements in general or enhancement of code-based linguistically relevant articulatory movements. The current study aims at disentangling which articulatory features are used in clear-speech modifications across tones (signal-based) and which features are unique for individual tones, and furthermore, if such tone-specific adjustments are aligned with the (code-based) category-defining features for each tone identified in Garg et al. (2019). Such findings will have implications for unraveling which visual cues may enhance tone perception and intelligibility.
Twelve female and eight male native Mandarin speakers aged between 18 and 28 years (mean: 22.6 years) were recruited. The speakers were born and have spent at least the first 18 years of their lives in either Northern China or Taiwan. They had resided in Canada for less than five years at the time of recording.
The stimuli were monosyllabic Mandarin words, each containing the vowel /ɤ/ with one of the four Mandarin tones, carrying the meaning of “graceful” (/ɤ1/; Tone 1, high-level tone), “goose” (/ɤ2/; Tone 2, mid-high-rising tone), “nauseous” (/ɤ3/; Tone 3, low-dipping tone), or “hungry” (/ɤ4/; Tone 4, high-falling tone), respectively.
The elicitation of plain and clear tones followed the procedures developed previously (Maniwa et al., 2009; Tang et al., 2015). A simulated interactive computer speech recognition system was developed using MATLAB (The Mathworks, R2013, Natick, MA, USA), where the program seemingly attempted to recognize a target stimulus produced by a speaker. The speaker was first instructed to read each of the stimuli that was shown on the screen naturally (to elicit plain style productions, e.g., /ɤ4/). Then the program would show its “guess” of the produced token. The software would systematically make wrong guesses due to “recognition” tonal errors (e.g., Did you say /ɤ3/?). The speaker was then requested to repeat the token more clearly (to elicit clear style productions, e.g., /ɤ4/). A total of 96 pronunciations of tone quadruplet words in two speaking styles (plain, clear) were videotaped from each speaker over three recording sessions (4 tones x 2 styles x 12 repetitions). The average duration of the target stimuli was 580 ms (SD = 193 ms) across styles, tones and speakers. In addition to the /ɤ/ word we also recorded /i/ and /u/ words as fillers.
The data was collected in a sound-attenuated booth in the Language and Brain Lab at Simon Fraser University. The speaker sat approximately three feet from a 15-inch LCD monitor on which the stimulus word was presented. The monitor was positioned at eye-level to facilitate the placement of a front-view video camera, which was placed below the monitor on a desktop tripod. A high-definition Canon Vixia HF30 camera was used to record the front-face of the speaker. The frame rate of the camera is 29 fps. Each speaker was made to sit with their back against a monochromatic green backdrop and was recorded separately. For interaction with the computer display, speakers were instructed in the usage of a video game wireless controller, which offered a comfortable and quiet way to interact with the display with minimal movement required from the speaker and introduced minimal interference with the video and audio recordings.
The analysis followed the tone articulation analysis approach previously developed by our team (Garg et al., 2019). It first involved extraction of articulatory features. Two analyses were subsequently conducted across tones and for each tone. First, discriminative analysis of the extracted motion features in clear and plain styles was conducted via random forest classification (Paul and Dupont, 2015). Random forest tests the features using multivariate analysis to identify which features significantly differentiate plain and clear styles and rank the importance of these features in contributing to the plain-clear differentiation. Second, for each of the features identified by random forest, the extent of movements (e.g., head movement distance) in plain vs. clear speech were compared using mixed-effects modeling to determine which of the features involved a significant difference between the two styles.
A total of 33 facial articulatory features which were previously identified as tone characterizing features (Garg et al., 2019) were included in this study to examine the plain-clear style differences.
Feature extraction involved the following steps using computer-vision and image processing techniques. First, regions of interest (ROI) on the face such as eyes, nose and lips of the speaker were identified on the first frame of the video and were subsequently tracked in the rest of the video. Briefly, the bounding box on the regions of interest are identified using LBP (Local Binary Pattern) cascade filters and then landmark-outlines are identified using active contour models. Specific keypoints on the landmark outlines such as nose tip, inner corner of the left eyebrow,2 and cupid's bow on lips are identified for tracking purposes. The Kanade-Lucas-Tomasi (KLT) feature-tracking algorithm was then used to track the aforementioned keypoints after they were found on the first frame of each video token. Next, the 33 features were computed on the motion trajectories of four keypoints identified on the nose tip (proxy for head movement), the left eyebrow, and the midpoints of the upper and lower lips. Then, each set of features was normalized to account for between-speaker differences, by dividing the feature values by a normalization factor computed as the shortest distance between the line joining the two eyes and the nose tip for subsequent analyses.
The absolute mean value was computed for each feature and each style to compare if the magnitude of the movements is different in clear speech than plain speech and when these movements occur during the tone production.
The 33 features can be generally classified into three categories: (1) distance-based, characterizing the minimum or maximum total displacement of a keypoint from its initial resting position to a target position; (2) time-based, characterizing the time it takes the displacement of a keypoint to reach maximum or minimum distance; and (3) kinematic, characterizing the velocity and acceleration of a keypoint at a specific time instance marked by a target event (e.g., instance when velocity reaches a maximum).
Table 1 contains a list of all the features and their descriptions. The distances are measured in pixels and the relative times are measured by the number of video frames divided by the total number of frames. Each feature is normalized to remove the variations due to head size among different speakers. Normalization was done by dividing the feature values by a normalization factor computed as the shortest distance between the line joining the two eyes and nose tip in that particular token. Since the features are normalized, the reported feature magnitudes are unitless. Figure 1 illustrates an example video frame showing the keypoints which are tracked for head, eyebrow and lip movements, and movement trajectories for a sample token in plain and clear speech styles. The distance-based features were calculated as the minimum and maximum distances that each of the tracked keypoints moved from its initial resting state. The positive measurements from the resting state signify an action of rising or opening, whereas negative measurements represent an action of lowering or closing. Velocities were then calculated as rate of change of the curve (i.e., slope). Finally, the acceleration is computed by the rate of change in the velocity curve.
Table 1. The set of 33 features used to represent tone articulation in each video token (cf. Garg et al., 2019).
Figure 1. The left-side image shows the landmark points (keypoints) at which the trajectory of the vertical movement is measured. A total of four keypoints are selected corresponding to the nose tip (proxy for the head, blue), left eyebrow (red), and lips (white). The right-side plots show the head, eyebrow, and lip movement trajectories for a sample token comparing the clear and plain speech style. Normalization factor used in the analysis is the length of the line segment AN. D, distance; t, time; v, velocity; max, raising/opening; min, lowering/closing (The face is generated by AI.).
We assessed the physical head size of two randomly selected speakers—one male and one female—in order to relate the derived measures from pixels to physical units (i.e., mm). For distance-based features, each pixel measured to 0.33 mm for male and 0.36 mm for female. For time-based and kinematic features, the videos were recorded at 29 fps and can be used to convert the per frame unit to per seconds. For examples, (1) the average head displacement for the male speaker during head-raising is 1.58 mm (4.75 pixels) and 5.88 mm (16.14 pixels) for the female speaker, and (2) the maximum head velocity during head-raising is 0.32 mm/s (0.98 pixels/s) for the male speaker and 0. 67 mm/s (1.83 pixels/s) for the female speaker. Further information for each feature can be found in Supplementary Table A1 of Garg et al. (2019).
We adopted the improved random forest approach developed by Paul and Dupont (2015), which was especially appropriate for this study since it enabled us to assess both the significant features and the ranking of these features that differentiate the two styles.
Random forest classification works by training an ensemble of basic decision trees, each of which predicts (or outputs) a class label given an input pool of features, with the final class label being determined by computing the average of the class label predictions from each tree. By using a random subset of samples and a random subset of features to train each tree, randomness is introduced into the system. In order to ensure reproducibility of the experiments, a random seed is set so that in every run the same random numbers are generated.
In our experiments, 1,500 trees were used for random forest classifiers. The style discriminative analysis involved a binary classification of clear vs. plain speech style from the recorded video tokens described above.
Our main goal was to examine which of the tone-defining features reported in Garg et al. (2019) could significantly differentiate the two speech styles. To this end, we conducted two types of comparisons: style differences across tones, and style differences within each tone.
The random forest analysis first provided us with features that differentiate the two styles across tones. All the features from different tones were pooled together and used as a training set for the random forest classifier. Then each feature importance was computed by permuting the samples in that feature and measuring the change in the prediction power. For each feature, if the measured changes were deemed statistically significant, then that feature would be considered important in differentiating the two styles across tones. We then employed the feature importance weights (Paul and Dupont, 2015) to rank the features in decreasing order of importance using leave-one-out cross-validation. The larger the weight, the more important the feature is for style discrimination. The features that were found to be important were further analyzed using linear mixed-effects modeling with style as the independent variable and the value of each important feature as the dependent variable using the MATLAB fitlme. The random intercept and slope of style on speaker were included in the models with the following syntax:
The final set of features that involve a significant style difference as determined by the mixed-effects modeling are considered generic (non-tone-specific) style features.
To examine which features were different between the two styles within each tone, we performed similar random forest and mixed-effects modeling analyses as described in Section 2.3.1. for each tone separately. After identifying a set of features that involve a significant difference in style for each tone, we compared these style-characterizing features to those obtained in Garg et al. (2019) that define each tone. We hypothesized that, for a particular tone, any style-characterizing features that overlap with tone-defining features are considered involving tone-specific clear-speech modifications. In contrast, any that overlap with the cross-tone features identified in 2.3.1 should be style-specific only.
First, we present the discriminant analysis results on the 33 features using random forest (RF). Using the procedure described in Section 3, thirteen features were found to be significant using RF classifier in differentiating the two speech styles, as shown in Figure 2. The features are arranged in descending order of their importance as determined by the random forest classifier. For each feature, the weight is the increase in prediction error if the values of that feature are permuted across the out-of-bag observations. This measure is computed for every decision tree in RF, then averaged over the entire ensemble and divided by the standard deviation over the entire ensemble. A larger error means that the feature is more important in classifying the style. Among the thirteen features, eight were found to be distance-based and five were related to time. The distance-based features primarily involve changes in the vertical distance of the head, lips and eyebrows, and the time-based features primarily involve changes in the time when the vertical head, lip and eyebrow movements reach maximum velocity. The feature importance ranking further revealed that the “Relative time at which the lip velocity during lip-opening was maximum” was the most differentiating feature to distinguish the two styles followed by the “Maximum displacement of the head while head-lowering from its starting position”, whereas the “Maximum lips velocity during lip-closing” was the least significant factor.
Figure 2. Important features identified by the random forest analysis in differentiating the two speech styles (clear and plain). The y-axis shows the feature importance measured by the random forest. The feature importance measures the increase in classification error if the values of the feature are permuted. The greater the error the higher the importance of the feature is.
To further determine the significance of the differences of the speech style for each of the thirteen features identified by the random forest classifier, the mean values of the normalized feature in clear and plain speech were compared using linear mixed-effects analysis as described in 2.3.1. The results, as summarized in Table 2, show that twelve out of thirteen features involve a significant clear-plain difference. Figure 3 displays the clear and plain style comparisons for each feature.
Table 2. Summary of the mixed-effects linear regression model for each of the features that involves a significant clear-plain difference across tones.
Figure 3. Comparisons of the group means of each of the thirteen important features (determined by random forest analyses) in clear and plain style irrespective of the tones. The y-axis shows the average normalized feature value for that particular style group. Twelve features were found to exhibit statistically significant differences between the two speech styles by mixed-effects modeling. The error bar on each of the bar plots shows the standard error. A * above a bar represents a p-value smaller than 0.05.
Specifically, the eight features where the magnitude of change is larger in clear than plain style include:
1. Maximum displacement of the head while head-raising from its starting position.
2. Maximum displacement of the head while head-lowering from its starting position.
3. Maximum lip-opening distance.
4. Maximum displacement of the left eyebrow while eyebrow-raising from its starting position.
5. Average distance left eyebrow moved during utterance.
6. Total distance traveled by left eyebrow during the utterance.
7. Maximum head velocity during head-raising.
8. Relative time at which the amount of lip-closing reached maximum.
The four features where the magnitude of change is smaller in clear than plain style are:
1. Relative time at which the head velocity was maximum during head-raising.
2. Relative time at which the lip velocity during lip-opening was maximum.
3. Relative time at which the left eyebrow velocity was maximum during eyebrow-raising.
4. Relative time at which the amount of lip-opening reached maximum.
The above list and Figure 3 shows that eight significant features had a larger movement magnitude in clear speech than in plain speech. These eight features are either distance or time related, including greater maximum distance of head raising or lowering, eyebrow raising and movement, and lip opening from their starting positions in clear than plain style, as well as longer time at which the amount of lip closing reached maximum in clear than plain style. These patterns suggest larger head, eyebrow and lip movements and faster arrival at the movement peak in clear relative to plain tone production. In contrast, four time-related features involved smaller magnitude of change in clear than plain speech, including shorter time taken for head raising, lip opening and eyebrow raising to reach maximum velocity. These features indicate that movement maxima happened earlier in clear than plain style, suggesting faster arrival at the movement peak in clear relative to plain tone production.
Overall, these patterns consistently reveal larger maximum displacement of head, eyebrow and lips, and faster arrival at these positions in clear than plain tone production, demonstrating exaggerated articulation in clear speech.
Next, we analyze each tone separately to identify tone-specific features that can differentiate the two styles. These features are then compared with the set of features characterizing each tone as reported in Garg et al. (2019) to determine the extent to which clear speech modifications adopt tone-intrinsic features.
First, the random forest analysis showed six important features differentiating the two speaking styles in Tone 1, listed in Figure 4 in decreasing order of their feature importance values. Four out of six features were time related, with the “Relative time at which the lip velocity during lip-opening was maximum” having the largest feature importance whereas “Relative time at which the displacement of the eyebrow while eyebrow-raising was maximum” having the smallest weight. Apart from these time-related features, “Maximum displacement of the head while head-lowering from its starting position” and “Maximum eyebrow velocity during eyebrow-raising” were also found to be important.
Figure 4. Important features identified by the random forest analysis in differentiating the two speech styles (clear and plain) in Tone 1. The y-axis shows the feature importance measured by the random forest. The feature importance measures the increase in classification error if the values of the feature are permuted. The greater the error the higher the importance of the feature is.
The difference in the magnitude of the mean values was then evaluated between the two styles using mixed-effects modeling. As displayed in Figure 5, for Tone 1, two features were found significant in differentiating the two styles, with 'maximum displacement of the head while head-lowering from its starting position' being larger in clear than plain speech (β = 0.039, standard error (SE) = 0.015, t (406) = 2.30, p < 0.05) and ‘the relative time at which the displacement of the head while head-raising was maximum' being smaller in clear than in plain speech (β = −0.045, SE = 0.023, t (406)= −1.979, p < 0.05). The first feature regarding head lowering distance has been identified not only as a tone-generic feature (3.1) but also as one of the defining features for Tone 1 in Garg et al. (2019), where head-lowering distance is the smallest in value among all the tones, reflecting that articulation of Tone 1 involves minimal head movement compared to the other tones.
Figure 5. Comparisons of the group means of each of the six important features (determined by random forest analyses) in clear and plain style in Tone 1. The y-axis shows the average normalized feature value for that particular style group. Two features were found to exhibit a statistically significant difference between the two speech styles by mixed-effects modeling. The error bar on each of the bar plots shows the standard error. A * above a bar represents a p-value smaller than 0.05.
The random forest analysis identified five out of 33 features as important features in style discrimination for Tone 2, in descending importance ranking (see Figure 6):
1. Relative time at which the lip velocity during lip-opening was maximum.
2. Relative time at which the lip velocity during lip-closing was maximum.
3. Relative time at which the amount of lip-closing reached maximum.
4. Total distance traveled by head during the utterance.
5. Total distance traveled by left eyebrow during the utterance.
Figure 6. Important features identified by random forest in differentiating the two speech styles (clear and plain) in Tone 2. The y-axis shows the feature importance measured by the random forest. The feature importance measures the increase in classification error if the values of the feature are permuted. The greater the error the higher the importance of the feature is.
Mixed-effects modeling revealed that the two styles were significantly different for all the five features (Figure 7). In both distance-related features clear speech had larger magnitude of movement than plain speech, indicating that the total distance traveled by the head (β = 1.686, SE = 0.631, t (454) = 2.669, p < 0.01) and eyebrow (β = 1.338, SE = 0.549, t (454) = 2.437, p < 0.05) are longer in clear than plain speech. For time-related features, clear relative to plain speech took shorter time for the lip-opening (β = −0.042, SE = 0.012, t (454) = −3.443, p < 0.001) and lip-closing (β = −0.043, SE = 0.014, t (454) = −3.004, p < 0.05) velocity to reach maximum, while the lips took more time to close (β = 0.107, SE = 0.040, t (454) = 2.659, p < 0.05). Thus, although clear speech may involve larger movement and may take longer to complete than plain speech, it tends to reach movement maxima sooner. These patterns are aligned with the overall clear speech features across tones reported above.
Figure 7. Comparisons of the group means of each of the five important features (determined by random forest analyses) in clear and plain style in Tone 2. The y-axis shows the average normalized feature value for that particular style group. All features were found to exhibit a statistically significant difference between the two speech styles by mixed-effects modeling. The error bar on each of the bar plots shows the standard error. A * above a bar represents a p-value smaller than 0.05.
Garg et al. (2019) reported that the feature distinguishing Tone 2 from the rest of the tones was that 'relative time at which the displacement of the head while head-raising was maximum' was longer for Tone 2 than for the other tones. The current results show that this feature was not used in the clear-plain speech distinction. Hence, for Tone 2, all the identified features characterizing the clear-plain differences involve style-specific modifications.
For Tone 3, nine features were found to be important based on random forest discriminative analysis (Figure 8). Six out of these nine features are time related and the other three are distance based. The most important feature was “Maximum displacement of the head while head-raising from its starting position” whereas the least weighted feature was “Relative time at which the displacement of the eyebrow while eyebrow-raising was maximum.”
Figure 8. Important features identified by random forest in differentiating the two speech styles (clear and plain) in Tone 3. The y-axis shows the feature importance measured by the random forest. The feature importance measures the increase in classification error if the values of the feature are permuted. The greater the error the higher the importance of the feature.
Follow-up mixed-effects modeling reveals three features to be significantly different in their magnitude between the two speech styles (Figure 9). Two features are time-related. Specifically, “relative time taken for lip-opening velocity to reach maximum” (β = −0.041, SE = 0.016, t (519) = −2.625, p < 0.05) and “relative time taken for head-raising velocity to reach maximum” (β = −0.039, SE = 0.017, t (519) = −2.330, p < 0.05) are shorter in clear than in plain speech, indicating a faster approach to target gesture in clear speech. The third feature is distance-related, where “maximum displacement of the head while head-raising from its starting position” (β = 0.035, SE = 0.012, t (519) = 2.863, p < 0.05) is larger in clear than in plain speech, indicating larger movements in clear speech style.
Figure 9. Comparisons of the group means of each of the nine important features (determined by random forest analyses) in clear and plain style in Tone 3. The y-axis shows the average normalized feature value for that particular style group. Three features were found to exhibit a statistically significant difference between the two speech styles by mixed-effects modeling. The error bar on each of the bar plots shows the standard error. A * above a bar represents a p-value smaller than 0.05.
Among the three significant style-distinguishing features, the feature involving the “relative time at which the head velocity was maximum during head-raising” was identified as one of the Tone 3-specific features previously (Garg et al., 2019), where it was shorter for Tone 3 relative to the other tones. However, this change is also a universal clear-speech pattern across tones.
For Tone 4, random forest analysis revealed eight features to be important in style distinctions (Figure 10), among which seven are time-based and one is related to distance. The most important feature is the “Relative time at which the displacement of the head while head-lowering was maximum” and the least important feature is the “Relative time at which the amount of lip-closing reached maximum.”
Figure 10. Important features identified by random forest in differentiating the two speech styles (clear and plain) in Tone 4. The y-axis shows the feature importance measured by the random forest. The feature importance measures the increase in classification error if the values of the feature are permuted. The greater the error the higher the importance of the feature is.
Three out of these eight features are shown to involve significant differences between plain and clear speech, as determined by further mixed-effects analysis (Figure 11). Specifically, the “relative time at which the head velocity was maximum during head-raising” was found to be shorter in clear than in plain speech (β = −0.043, SE = 0.016, t (424) = −2.735, p < 0.05). The second feature involves “relative time at which the amount of lip-closing reached maximum” (β = 0.092, SE = 0.036, t (424) = 2.581, p < 0.05), which occurred later in clear than in plain style, suggesting longer duration in clear-speech production. The third feature is the “total distance traveled by head during the utterance “(β = 1.948, SE = 0.703, t (424) = 2.770, p < 0.05), which appears to be larger in clear than plain speech, as expected.
Figure 11. Comparisons of the group means of each of the eight important features (determined by random forest analyses) in clear and plain style in Tone 4. The y-axis shows the average normalized feature value for that particular style group. Three features were found to exhibit a statistically significant difference between the two speech styles by mixed-effects modeling. The error bar on each of the bar plots shows the standard error. A * above a bar represents a p-value smaller than 0.05.
One of these significant features was a Tone 4-specific feature (Garg et al., 2019); that is, the “relative time at which the head velocity was maximum during head-raising” was shorter for Tone 4 relative to most of the other tones, indicating faster return of the head to the resting position after the head lowering gesture for the falling Tone 4. Clear speech, with an even faster head-raising velocity, apparently enhanced this feature.
In summary, across tones, clear speech demonstrated exaggerated articulation compared to plain speech, with larger maximum displacement of head, eyebrows and lips, and faster arrival at these positions. The analysis of individual tones showed that these general clear-speech enhancement patterns primarily hold for each tone, while certain tone-specific features were also strengthened.
For Tone 1, two features showed a significant difference between plain and clear speech, including one tone-specific feature, namely head lowering distance. However, the direction of this clear-speech modification was in conflict with the Tone 1 intrinsic feature. That is, while Tone 1, as a level tone, was characterized as having smaller head lowering compared to the other tones, the movement was not further restrained in clear speech. Instead, clear speech demonstrated larger head lowering than plain speech, consistent with the tone-general pattern of larger movements in clear relative to plain speech. Similarly, the second significant feature showing a plain-to-clear difference, which involved shorter time taken for head-raising to reach maximum in clear speech, was also in line with the across-tone patterns of faster arrival at the movement peak in clear than plain tone production.
For Tone 2, what significantly distinguished clear and plain styles involved no Tone 2-specific features. Instead, plain-to-clear speech modifications of Tone 2 involved larger head and eyebrow movements and longer (lip-closing) time to complete the production, as well as quicker lip movements to reach target gesture, which were primarily aligned with the overall clear speech features across tones.
Tone 3 clear speech modifications involved one unique Tone 3 feature. The quicker attainment of head-raising velocity maximum in clear relative to plain speech was aligned with the patterns characterizing this tone, where the time taken to achieve maximum head-raising velocity was shorter for Tone 3 than for the other tones. However, this feature is also identified as a tone-universal clear-speech modification (cf. Figure 3). Moreover, the clear-speech modifications of larger head raising and faster lip opening velocity did not involve Tone 3-specific features. Thus, Tone 3 clear-speech modifications essentially adopt universal features characterizing clear-speech tone.
Tone 4 clear-plain differences made use of one Tone 4-specific feature. That is, the time taken for head-raising velocity to reach maximum, which was shorter for Tone 4 than for most of the other tones, was even shorter in clear than in plain speech, suggesting faster return of the head to the resting position after the head lowering gesture for the falling Tone 4. However, this feature, along with the shorter time taken for eyebrow-raising velocity maximum, was also consistent with the patterns across tones. Additionally, “the time taken for the distance of lip-closing to reach maximum”, occurred later in clear than in plain style, suggesting longer duration in clear-speech production.
In this study, we examined how visual tonal cues are enhanced in clear speech in the production of Mandarin Chinese tones. As tone production lacks a direct association with vocal tract configurations, it is believed to be less distinguishable through visible articulatory movements. The question thus raised in this study was, in the production of clear-speech tones, whether any modifications of the visual articulatory features strengthen overall visual saliency (signal-based) or augment tone-specific distinctions (code-based). To this end, we compared which visual cues were adopted in clear speech across tones (as evidence of signal-based modifications) and which ones were aligned with the category-defining features for each tone as identified in Garg et al. (2019) (as evidence of code-based modifications).
Through computer vision analyses, this study tracked and quantified 33 facial features associated with head, eyebrow, and lip movements to determine the distance, duration, and kinematic characteristics between each of the keypoints in clear vs. plain tone productions. A 2-step discriminant analysis based on random forest and subsequent mixed-effects modeling was performed, first across tones and then for each tone, to identify the visual features differentiating clear and plain tone productions and rank the importance of these features, and then compare the values of each feature in clear and plain speech to assess if they are significantly different.
The results show differences in visual features between the two speech styles from both cross-tone and within-tone comparisons. Overall, the difference between the two styles lies both in spatial and temporal features as indicated by changes in distance, duration, velocity and acceleration of lip, eyebrow and head movements associated with clear vs. plain tone productions. The common trend exhibited through these features indicates that signal-based plain-to-clear tone modifications are more dominant than code-based modifications, and are evidenced by both across tone and individual tone results.
Across tones, clear (compared to plain) productions show longer overall duration, larger maximum displacement of the head, eyebrows and lips and faster arrival at these movement peaks. First, the larger displacement maxima and longer duration indicate that clear-speech production of all the tones involves more extended articulatory trajectories, and consequently, takes longer to complete. Such patterns are consistent with previous studies revealing exaggerated articulation in clear speech segments. For example, studies on vowel articulation have consistently revealed longer duration along with greater articulatory movements (involving larger lip and jaw displacement across vowels) for clear relative to plain speech (Kim and Davis, 2014; Tang et al., 2015). Similar exaggerated articulatory activities have also been identified at the suprasegmental level such as long and short vowels (Šimko et al., 2016) as well as lexical tones (Han et al., 2019). Moreover, aside from these spatial features, the current results additionally reveal that, despite the longer distance, clearly produced tones generally reach movement peak positions faster. Such a combination of motion may consequently make the visual cues more prominent, thus enhancing the saliency of tones in clear speech. These findings consistently demonstrate signal-based modifications in clear-speech production across tones through both distance-based and time-based changes with overall enhancement of visual saliency.
Results of individual tone analyses corroborate the patterns across tones, revealing signal-based modifications predominantly. In addition to these general patterns, the current individual-tone findings are particularly noteworthy in that they strengthen the signal-based nature of clear-speech tone modifications in three ways. First, certain tone-general modifications are found to be incompatible with the inherent characteristics of individual tones. For example, the Tone 1 plain-to-clear modification followed the tone-general pattern of larger head lowering. However, as a level tone, Tone 1 inherently involves minimal head and eyebrow movements, presumably attributable to its small variation in pitch (Yehia et al., 2002; Munhall et al., 2004; Kim et al., 2014; Garg et al., 2019). Thus, it appears that signal-based information was adopted in clear Tone 1 modification even when it is in conflict with the intrinsic characteristics of this tone. Second, although some modifications involve tone-characterizing features, they are also aligned with universal clear-speech patterns. For example, for Tone 3, the quicker attainment of head-raising velocity maximum in clear relative to plain speech is aligned with the tone-general patterns as well as being an intrinsic property of this tone. Consequently, such tone-specific adjustments cannot be regarded as code-based alone. Third, significant tone-specific features fail to exhibit changes in clear speech. Notably, Tone 2 and Tone 3, as dynamic tones, have been identified as having multiple category-defining features (Garg et al., 2019). However, most of the crucial features of these tones, such as the low tone nature of Tone 3 (associated with head lowering, Garg et al., 2019) or its dynamicity (associated with lip closing and raising, Attina et al., 2010), did not exhibit corresponding modifications in clear speech. Taken together, consistent with the cross-tone results, these individual tone patterns suggest that signal-based cues outweigh code-based ones in clear-speech modification.
Therefore, unlike the patterns found at the segmental level for consonants and vowels showing signal- and code-based clear-speech modification working in tandem (Smiljanić, 2021), the current results suggest that visual clear-speech tone modifications primarily do not rely on code-based, tone-specific cues. Although previous findings on tone articulation indeed suggest alignments of facial movements with spatial and temporal pitch movement trajectories of individual tones (Attina et al., 2010; Garg et al., 2019; Han et al., 2019), most of these cues were not adopted in making tone-specific adjustments in clear speech. One possibility could be that the visual tonal cues, which are shown to be based on spatial and temporal correspondence to acoustic (F0) information rather than a direct association with vocal tract configuration (as is the case for segmental production), are not adequately distinctive (Hannah et al., 2017; Burnham et al., 2022). This is especially true for lip movements, which have been found to be less reliable in differentiating tones (Attina et al., 2010; Garg et al., 2019). Previous segmental studies suggest a trade-off in clear speech production between cue enhancements and maintenance of sound category distinctions (Lindblom, 1990; Ohala, 1995; Smiljanić, 2021). Speakers have been found to refrain from making clear-speech adjustments which would blur category distinctions (Leung et al., 2016; Smiljanić, 2021). In the case of the current study, the speakers may have more readily adopted the universal features that strengthen overall visual saliency since enhancing tone-specific features cannot reliably distinguish different tone categories.
Finally, it is worth noting that the acoustic analysis of the same data set by our research team (Tupper et al., 2021) has also revealed that the speakers primarily utilize signal-based acoustic changes (longer duration, higher intensity) in clear-speech tone modifications rather than code-based F0 changes that enhance the contrast between tones. This may also explain the lack of code-based articulatory modifications in the current study, given the presumed audio-spatial correspondence between pitch and visual articulatory movements (Connell et al., 2013; Garg et al., 2019). These findings lead to the subsequent question as to whether these articulatory and acoustic adjustments in clear speech benefit tone intelligibility and whether these universal saliency enhancing cues affect the perception of individual tones differently. The latter could in turn help disentangle the signal- vs. code-based nature of clear tone production.
The raw data supporting the conclusions of this article will be made available by the authors, without undue reservation.
The studies involving human participants were reviewed and approved by Office of Research Ethics, Simon Fraser University. The patients/participants provided their written informed consent to participate in this study.
SG wrote the MATLAB code and analysis and helped in preparing the draft. GH provided feedback and suggestions in the analysis and reviewed the analysis and provided computing resources to perform the analysis. JS and AJ helped with the problem question, reviewed the analysis, and provided feedback with the writing. YW helped with the problem question, reviewed the analysis, and wrote the introduction and discussion and helped with the other writing. All authors contributed to the article and approved the submitted version.
This project has been supported by research grants from the Natural Sciences and Engineering Research Council of Canada (NSERC Discovery Grant No. 2017-05978) and the Social Sciences and Humanities Research Council of Canada (SSHRC Insight Grant No. 435-2019-1065).
We thank Lisa Tang for helping with the code and experiments as well as the write-up. We also thank Keith Leung, Jane Jian, Charles Turo, and Dahai Zhang from the SFU Language and Brain Lab for their assistance, as well as WestGrid and Compute Canada for their IT support.
The authors declare that the research was conducted in the absence of any commercial or financial relationships that could be construed as a potential conflict of interest.
All claims expressed in this article are solely those of the authors and do not necessarily represent those of their affiliated organizations, or those of the publisher, the editors and the reviewers. Any product that may be evaluated in this article, or claim that may be made by its manufacturer, is not guaranteed or endorsed by the publisher.
1. ^The use of the terms “clear (hyperarticulated) style” and “plain (conversational) style” follows the convention in previous clear-speech studies (e.g., Ferguson and Kewley-Port, 2002; Maniwa et al., 2008; Tang et al., 2015; Smiljanić, 2021; Tupper et al., 2021). These two terms refer to the more enunciated vs. normal speech styles, respectively, resulting from elicitation procedures to instruct talkers to speak an utterance “normally” first in the manner used in a plain, natural conversation, and then repeat it “clearly” with the goal of improving intelligibility.
2. ^Left eyebrow was chosen based on the previous findings that the left relative to right eyebrow is more prominent in prosodic production and is more strongly correlated with prosodic patterns (Cavé et al., 1996; Swerts and Krahmer, 2008). Future research could compare measures of left vs. right eyebrows to further examine how left and right eyebrows contribute to plain-to-clear modifications of Mandarin tones.
Attina, V., Gibert, G., Vatikiotis-Bateson, E., and Burnham, D. (2010). “Production of Mandarin lexical tones: Auditory and visual components,” in Proceedings of International Conference on Auditory-visual Speech Processing (AVSP) 2010, Hakone.
Bradlow, A. R., and Bent, T. (2002). The clear speech effect for non-native listeners. J. Acoust. Soc. Am. 112, 272–284. doi: 10.1121/1.1487837
Burnham, D., Ciocca, V., and Stokes, S. (2001). Auditory-visual perception of lexical tone. In, P. Dalsgaard, B. Lindberg, H. Benner, and Z. H. Tan, (eds.), Proceedings of the 7th Conference on Speech Communication and Technology, EUROSPEECH 2001, Scandinavia, pp. 395–398. doi: 10.21437/Eurospeech.2001-63
Burnham, D., Reynolds, J., Vatikiotis-Bateson, E., Yehia, H., Ciocca, V., Morris, R., et al. (2006). “The perception and production of phones and tones: The role of rigid and non-rigid face and head motion,” in Proceedings of the International Seminar on Speech Production 2006, Ubatuba.
Burnham, D., Vatikiotis-Bateson, E., Barbosa, A. V., Menezes, J. V., Yehia, H. C., Morris, R. H., et al. (2022). Seeing lexical tone: head and face motion in production and perception of Cantonese lexical tones. Speech Commun. 141, 40–55. doi: 10.1016/j.specom.2022.03.011
Cavé, C., Guaïtella, I., Bertrand, R., Santi, S., Harlay, F., Espesser, R., et al. (1996). About the relationship between eyebrow movements and F0 variations. Proceedings of the ICSLP (pp. 2175–2179), Philadelphia. doi: 10.21437/ICSLP.1996-551
Chen, T. H., and Massaro, D. W. (2008). Seeing pitch: Visual information for lexical tones of Mandarin-Chinese. J. Acoust. Soc. Am. 123, 2356–2366. doi: 10.1121/1.2839004
Connell, L., Cai, Z. G., and Holler, J. (2013). Do you see what i'm singing? visuospatial movement biases pitch perception. Brain and Cognition 81, 124–130. doi: 10.1016/j.bandc.2012.09.005
Cooke, M., and Lu, Y. (2010). Spectral and temporal changes to speech produced in the presence of energetic and informational maskers. J. Acoust. Soc. Am. 128, 2059–2069. doi: 10.1121/1.3478775
Cvejic, E., Kim, J., and Davis, C. (2010). Prosody off the top of the head: Prosodic contrasts can be discriminated by head motion. Speech Commun. 52, 555–564. doi: 10.1016/j.specom.2010.02.006
Desai, S., Stickney, G., and Zeng, F. G. (2008). Auditory-visual speech perception in normal-hearing and cochlear-implant listeners. J. Acoust. Soc. Am. 123, 428–440. doi: 10.1121/1.2816573
Dohen, M., and Loevenbruck, H. (2005). “Audiovisual Production and Perception of Contrastive Focus in French: a multispeaker study,” in Interspeech/Eurospeech 2005 (pp. p-2413). doi: 10.21437/Interspeech.2005-49
Dohen, M., Loevenbruck, H., and Hill, H. C. (2006). “Visual correlates of prosodic contrastive focus in French: Description and inter-speaker variability,” in Speech Prosody, edsR. Hoffmann and H. Mixdorff, 221–224.
Ferguson, S. H., and Kewley-Port, D. (2002). Vowel intelligibility in clear and conversational speech for normal-hearing and hearing-impaired listeners. J. Acoust. Soc. Am. 112, 259–271. doi: 10.1121/1.1482078
Ferguson, S. H., and Kewley-Port, D. (2007). Talker differences in clear and conversational speech: Acoustic characteristics of vowels. Journal of Speech, Language, and Hearing Research 50, 1241–1255. doi: 10.1044/1092-4388(2007/087)
Flecha-García, M. L. (2010). Eyebrow raises in dialogue and their relation to discourse structure, utterance function and pitch accents in English. Speech Commun. 52, 542–554. doi: 10.1016/j.specom.2009.12.003
Gagné, J. P., Masterson, V., Munhall, K. G., Bilida, N., and Querengesser, C. (1994). Across talker variability in auditory, visual, and audiovisual speech intelligibility for conversational and clear speech. J. Academy Rehabil. Audiol. 27, 135–158.
Gagné, J. P., Rochette, A. J., and Charest, M. (2002). Auditory, visual and audiovisual clear speech. Speech Commun. 37, 213–230. doi: 10.1016/S0167-6393(01)00012-7
Garg, S., Hamarneh, G., Jongman, A., Sereno, J. A., and Wang, Y. (2019). Computer-vision analysis reveals facial movements made during Mandarin tone production align with pitch trajectories. Speech Commun. 113, 47–62. doi: 10.1016/j.specom.2019.08.003
Garnier, M., Ménard, L., and Alexandre, B. (2018). Hyper-articulation in Lombard speech: an active communicative strategy to enhance visible speech cues?. J. Acoust. Soc. Am. 144, 1059–1074. doi: 10.1121/1.5051321
Han, Y., Goudbeek, M., Mos, M., and Swerts, M. (2019). Effects of modality and speaking style on Mandarin tone identification by non-native listeners. Phonetica 76, 263–286. doi: 10.1159/000489174
Hannah, B., Wang, Y., Jongman, A., Sereno, J. A., Cao, J., Nie, Y., et al. (2017). Cross-modal association between auditory and visuospatial information in Mandarin tone perception in noise by native and non-native perceivers. Front. Psychol. 8, 2051. doi: 10.3389/fpsyg.2017.02051
Hazan, V., and Baker, R. (2011). Acoustic-phonetic characteristics of speech produced with communicative intent to counter adverse listening conditions. J. Acoust. Soc. Am. 130, 2139–2152. doi: 10.1121/1.3623753
Helfer, K. S. (1997). Auditory and auditory-visual perception of clear and conversational speech. J. Speech Lang. Hearing Res. 40, 432–443. doi: 10.1044/jslhr.4002.432
Ishi, C. T., Ishiguro, H., and Hagita, N. (2007). Analysis of head motions and speech in spoken dialogue. INTERSPEECH 2007 8th. Annual Conference of the International Speech Communication Association 2, 670–673. doi: 10.21437/Interspeech.2007-286
Kim, J., Cvejic, E., and Davis, C. (2014). Tracking eyebrows and head gestures associated with spoken prosody. Speech Commun. 57, 317–330. doi: 10.1016/j.specom.2013.06.003
Kim, J., and Davis, C. (2014). Comparing the consistency and distinctiveness of speech produced in quiet and in noise. Comp. Speech Lang. 28, 598–606. doi: 10.1016/j.csl.2013.02.002
Kim, J., Sironic, A., and Davis, C. (2011). Hearing speech in noise: Seeing a loud talker is better. Perception 40, 853–862. doi: 10.1068/p6941
Krause, J. C., and Braida, L. D. (2004). Acoustic properties of naturally produced clear speech at normal speaking rates. J. Acoust. Soc. Am. 115, 362–378. doi: 10.1121/1.1635842
Lander, K., and Capek, C. (2013). Investigating the impact of lip visibility and talking style on speechreading performance. Speech Commun. 55, 600–605. doi: 10.1016/j.specom.2013.01.003
Leung, K. K., Jongman, A., Wang, Y., and Sereno, J. A. (2016). Acoustic characteristics of clearly spoken English tense and lax vowels. J. Acoust. Soc. Am. 140, 45–58. doi: 10.1121/1.4954737
Lindblom, B. (1990). Explaining phonetic variation: A sketch of the HandH theory. In W. Hardcastle and A. Marchal (Eds.), Speech Production and Speech Modelling (pp. 403–439). Dordrecht: Springer. doi: 10.1007/978-94-009-2037-8_16
Lu, Y., and Cooke, M. (2008). Speech production modifications produced by competing talkers, babble, and stationary noise. J. Acoust. Soc. Am. 124, 3261–3275. doi: 10.1121/1.2990705
Maniwa, K., Jongman, A., and Wade, T. (2008). Perception of clear fricatives by normal-hearing and simulated hearing-impaired listeners. J. Acoust. Soc. Am. 123, 1114–1125. doi: 10.1121/1.2821966
Maniwa, K., Jongman, A., and Wade, T. (2009). Acoustic characteristics of clearly spoken English fricatives. J. Acoust. Soc. Am. 125, 3962–3973. doi: 10.1121/1.2990715
Moon, S. J., and Lindblom, B. (1994). Interaction between duration, context, and speaking style in English stressed vowels. J. Acoust. Soc. Am. 96, 40–55. doi: 10.1121/1.410492
Munhall, K. G., Jones, J. A., Callan, D. E., Kuratate, T., and Vatikiotis-Bateson, E. (2004). Visual prosody and speech intelligibility: Head movement improves auditory speech perception. Psychol. Sci. 15, 133–137. doi: 10.1111/j.0963-7214.2004.01502010.x
Ohala, J. (1995). Clear speech does not exaggerate phonemic contrast. In Proceedings of the 4th European Conference on Speech Communication and Technology (pp. 1323–1325). doi: 10.21437/Eurospeech.1995-344
Paul, J., and Dupont, P. (2015). Inferring statistically significant features from random forests. Neurocomputing 150, 471–480. doi: 10.1016/j.neucom.2014.07.067
Perkell, J. S., Zandipour, M., Matthies, M. L., and Lane, H. (2002). Economy of effort in different speaking conditions. I. A preliminary study of intersubject differences and modeling issues. J. Acoust. Soc. Am. 112, 1627–1641. doi: 10.1121/1.1506369
Redmon, C., Leung, K., Wang, Y., McMurray, B., Jongman, A., Sereno, J. A., et al. (2020). Cross-linguistic perception of clearly spoken English tense and lax vowels based on auditory, visual, and auditory-visual information. J. Phon. 81, 100980. doi: 10.1016/j.wocn.2020.100980
Reid, A., Burnham, D., Kasisopa, B., Reilly, R., Attina, V., Rattanasone, N. X., et al. (2015). Perceptual assimilation of lexical tone: the roles of language experience and visual information. Attent. Percep. Psychophysics 77, 571–591. doi: 10.3758/s13414-014-0791-3
Scarborough, R., Keating, P., Mattys, S. L., Cho, T., and Alwan, A. (2009). Optical phonetics and visual perception of lexical and phrasal stress in English. Lang. Speech 52, 135–175. doi: 10.1177/0023830909103165
Šimko, J., Benuš, Š., and Vainio, M. (2016). Hyperarticulation in Lombard speech: Global coordination of the jaw, lips and the tongue. J. Acoust. Soc. Am. 139, 151–162. doi: 10.1121/1.4939495
Smiljanić, R. (2021). “Clear speech perception: Linguistic and Cognitive benefits,” in The Handbook of Speech Perception, eds Pardo, J.S., Nygaard, L.C., Remez, R.E., and Pisoni, D.B., 2nd Edition. Wiley. pp. 177-205. doi: 10.1002/9781119184096.ch7
Smiljanić, R., and Bradlow, A. R. (2005). Production and perception of clear speech in Croatian and English. J. Acoust. Soc. Am. 118, 1677–1688. doi: 10.1121/1.2000788
Smiljanić, R., and Bradlow, A. R. (2009). Speaking and hearing clearly: Talker and listener factors in speaking style changes. Lang. Linguist. Compass 3, 236–264. doi: 10.1111/j.1749-818X.2008.00112.x
Srinivasan, R. J., and Massaro, D. W. (2003). Perceiving prosody from the face and voice: Distinguishing statements from echoic questions in English. Lang. Speech 46, 1–22. doi: 10.1177/00238309030460010201
Sumby, W. H., and Pollack, I. (1954). Visual contribution to speech intelligibility in noise. J. Acoust. Soc. Am. 26, 212–215. doi: 10.1121/1.1907309
Swerts, M., and Krahmer, E. (2008). Facial expression and prosodic prominence: Effects of modality and facial area. J. Phon. 36, 219–238. doi: 10.1016/j.wocn.2007.05.001
Swerts, M., and Krahmer, E. (2010). Visual prosody of newsreaders: Effects of information structure, emotional content and intended audience on facial expressions. J. Phon. 38, 197–206. doi: 10.1016/j.wocn.2009.10.002
Tang, L. Y., Hannah, B., Jongman, A., Sereno, J., Wang, Y., Hamarneh, G., et al. (2015). Examining visible articulatory features in clear and plain speech. Speech Commun. 75, 1–13. doi: 10.1016/j.specom.2015.09.008
Tasko, S. M., and Greilick, K. (2010). Acoustic and articulatory features of diphthong production: a speech clarity study. J. Speech Lang. Hear. Research, 53, 84–99. doi: 10.1044/1092-4388(2009/08-0124)
Traunmüller, H., and Öhrström, N. (2007). Audiovisual perception of openness and lip rounding in front vowels. J. Phon. 35, 244–258. doi: 10.1016/j.wocn.2006.03.002
Tupper, P., Leung, K. K., Wang, Y., Jongman, A., and Sereno, J. A. (2018). Identifying the distinctive acoustic cues of Mandarin tones. J. Acoust. Soc. Am. 144, 1725–1725. doi: 10.1121/1.5067655
Tupper, P., Leung, K. W., Wang, Y., Jongman, A., and Sereno, J. A. (2021). The contrast between clear and plain speaking style for Mandarin tones. J. Acoust. Soc. Am. 150, 4464–4473. doi: 10.1121/10.0009142
Van Engen, K. J., Phelps, J. E., Smiljanic, R., and Chandrasekaran, B. (2014). Enhancing speech intelligibility: Interactions among context, modality, speech style, and masker. J. Speech Lang. Hearing Res. 57, 1908–1918. doi: 10.1044/JSLHR-H-13-0076
Wang, Y., Behne, D. M., and Jiang, H. (2008). Linguistic experience and audio-visual perception of non-native fricatives. J. Acoust. Soc. Am. 124, 1716–1726. doi: 10.1121/1.2956483
Yehia, H. C., Kuratate, T., and Vatikiotis-Bateson, E. (2002). Linking facial animation, head motion and speech acoustics. J. Phon. 30, 555–568. doi: 10.1006/jpho.2002.0165
Keywords: speech style, Mandarin, facial cues, computer vision, video processing, Mandarin tones
Citation: Garg S, Hamarneh G, Sereno J, Jongman A and Wang Y (2023) Different facial cues for different speech styles in Mandarin tone articulation. Front. Commun. 8:1148240. doi: 10.3389/fcomm.2023.1148240
Received: 19 January 2023; Accepted: 06 April 2023;
Published: 28 April 2023.
Edited by:
Plinio Almeida Barbosa, State University of Campinas, BrazilReviewed by:
Marzena Zygis, Leibniz Center for General Linguistics (ZAS), GermanyCopyright © 2023 Garg, Hamarneh, Sereno, Jongman and Wang. This is an open-access article distributed under the terms of the Creative Commons Attribution License (CC BY). The use, distribution or reproduction in other forums is permitted, provided the original author(s) and the copyright owner(s) are credited and that the original publication in this journal is cited, in accordance with accepted academic practice. No use, distribution or reproduction is permitted which does not comply with these terms.
*Correspondence: Saurabh Garg, c3JiaC5nYXJnQGdtYWlsLmNvbQ==; Yue Wang, eXVld0BzZnUuY2E=
Disclaimer: All claims expressed in this article are solely those of the authors and do not necessarily represent those of their affiliated organizations, or those of the publisher, the editors and the reviewers. Any product that may be evaluated in this article or claim that may be made by its manufacturer is not guaranteed or endorsed by the publisher.
Research integrity at Frontiers
Learn more about the work of our research integrity team to safeguard the quality of each article we publish.