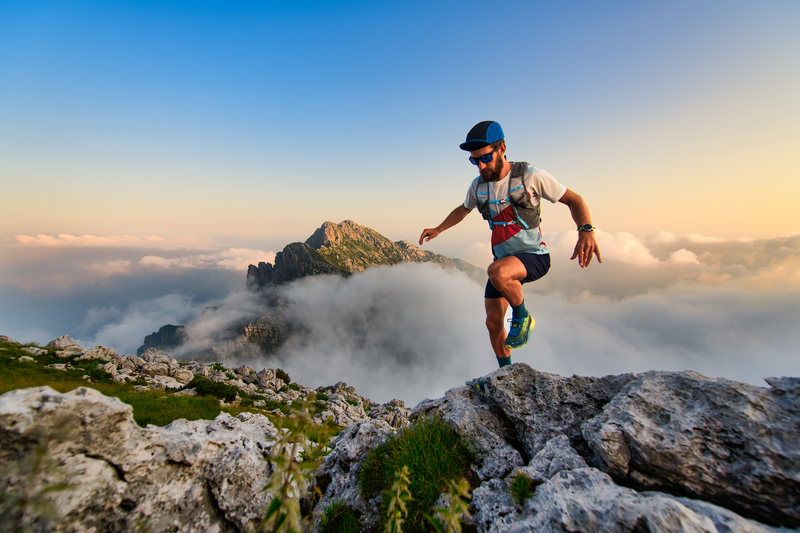
95% of researchers rate our articles as excellent or good
Learn more about the work of our research integrity team to safeguard the quality of each article we publish.
Find out more
PERSPECTIVE article
Front. Clim. , 16 September 2022
Sec. Predictions and Projections
Volume 4 - 2022 | https://doi.org/10.3389/fclim.2022.955414
This article is part of the Research Topic Insights in Predictions and Projections: 2022 View all 7 articles
Multi-annual to decadal changes in climate are accompanied by changes in extreme events that cause major impacts on society and severe challenges for adaptation. Early warnings of such changes are now potentially possible through operational decadal predictions. However, improved understanding of the causes of regional changes in climate on these timescales is needed both to attribute recent events and to gain further confidence in forecasts. Here we document the Large Ensemble Single Forcing Model Intercomparison Project that will address this need through coordinated model experiments enabling the impacts of different external drivers to be isolated. We highlight the need to account for model errors and propose an attribution approach that exploits differences between models to diagnose the real-world situation and overcomes potential errors in atmospheric circulation changes. The experiments and analysis proposed here will provide substantial improvements to our ability to understand near-term changes in climate and will support the World Climate Research Program Lighthouse Activity on Explaining and Predicting Earth System Change.
Climate variability and change cause major impacts on human society and natural ecosystems. Of particular concern are large-scale changes in atmospheric circulation, since they can alter rainfall patterns, threatening food and water security, and increase the chances of extreme events. Prominent examples on decadal timescales include the United States “Dust Bowl” during the 1930s (Schubert et al., 2004), the Sahel droughts during the 1970s (Kandji et al., 2006), the mid-1990s increase in Atlantic hurricane activity (Goldenberg et al., 2001), the early twentieth century warming (Hegerl et al., 2018) and the early twenty-first century slowdown in global surface warming (Medhaug et al., 2017). Recent multi-decadal trends in atmospheric circulation also likely contributed to several extreme seasonal events including heatwaves in Europe and Russia, floods in Pakistan, and wildfires across the west coast of the United States (Teng et al., 2022) and Australia (Canadell et al., 2021), and even daily extremes such as rainfall and storms are strongly modulated by multidecadal fluctuations in large scale patterns such as the North Atlantic Oscillation (NAO; Scaife et al., 2008; Dawkins et al., 2016). Some recent extremes are also far outside the range of previously observed variability, causing severe impacts and creating serious challenges for climate adaptation (Fischer et al., 2021). Hence there is an urgent need to understand the causes of regional multi-annual to decadal changes in climate.
Decadal climate predictions are now issued operationally by the World Meteorological Organisation (WMO) and offer the potential to provide early warnings of changes in climate (Kushnir et al., 2019). By comparing retrospective forecasts (hindcasts) with observations, it has been established that decadal predictions can potentially forecast many aspects of climate over the coming years, including Atlantic Multidecadal Variability (AMV, Doblas-Reyes et al., 2013; Hermanson et al., 2014; Yeager and Robson, 2017; Borchert et al., 2021), Atlantic hurricane activity (Smith et al., 2010; Caron et al., 2018), Sahel rainfall (Sheen et al., 2017; Yeager et al., 2018), droughts and wildfires in southwestern United States (Chikamoto et al., 2017), carbon fluxes (Li et al., 2019; Lovenduski et al., 2019a,b), European precipitation and temperature in summer (Müller et al., 2012; Yeager et al., 2018) and winter (Simpson et al., 2019), summer temperatures in north-east Asia (Monerie et al., 2018), the occurrence of warm summer temperature extremes (Borchert et al., 2019), Tibetan Plateau summer rainfall (Hu and Zhou, 2021), and winter atmospheric circulation in the North Atlantic (Athanasiadis et al., 2020; Smith et al., 2020). Each year the WMO issues a Global Annual to Decadal Climate Update (GADCU, Hermanson et al., 2022) that synthesizes the forecasts from several international centers. These forecasts show clear signals for atmospheric circulation and precipitation, as well as for near surface temperature (Hermanson et al., 2022), but greater confidence is needed for them to become “actionable”. In particular, skill in hindcasts does not guarantee skill in forecasts since the drivers may be different. Similarly, absence of skill in hindcasts does not necessarily mean absence of skill in forecasts since the drivers that produce predictable signals may only just be emerging. Understanding the drivers of multi-annual changes in climate is therefore essential for gaining further confidence in forecasts.
Despite its importance, our ability to understand and attribute multi-annual to decadal changes in climate is immature, as evidenced by the recent debate over the slowdown in global surface warming (Medhaug et al., 2017). To address this, the World Climate Research Programme (WCRP) has set up a Lighthouse Activity on Explaining and Predicting Earth System Change (LHA-EPESC) with three major themes (Findell et al., 2022): (i) monitoring and modeling Earth system change; (ii) integrated attribution, prediction and projection; and (iii) assessment of current and future hazards. A key component of this especially for theme (ii) will be the Large Ensemble Single Forcing Model Intercomparison Project (LESFMIP) experiments proposed here.
On multi-annual to decadal timescales climate is potentially influenced by several factors: changes in greenhouse gas concentrations, anthropogenic aerosols, volcanic aerosols, solar irradiance, ozone, land use, biomass burning, dust, and internal variability especially involving the ocean (some of which may be predictable). In most regions a long-term warming trend from greenhouse gases has been partially offset by the cooling effects of anthropogenic aerosols. However, aerosol effects are much more heterogeneous both in time and space, due to strong regional patterns of emission changes and indirect effects through interactions with clouds. Many studies have shown potential anthropogenic aerosol impacts on decadal climate including AMV (Booth et al., 2012; Bellucci et al., 2017; Murphy et al., 2017; Bellomo et al., 2018; Watanabe and Tatebe, 2019), Atlantic Meridional Overturning Circulation (AMOC, Menary et al., 2020; Hassan et al., 2021), the Aleutian Low and Pacific Decadal Variability (PDV, Allen et al., 2014; Smith et al., 2016; Takahashi and Watanabe, 2016; Oudar et al., 2018; Wilcox et al., 2019; Dittus et al., 2021; Dow et al., 2021), mid-latitude atmospheric jets (Wang Y. et al., 2020; Dong et al., 2022), southern hemisphere atmospheric circulation (Gillett et al., 2013; Rotstayn et al., 2013; Wang H. et al., 2020), Atlantic hurricanes (Mann and Emanuel, 2006; Dunstone et al., 2013), Sahel rainfall (Ackerley et al., 2011; Marvel et al., 2020; Hirasawa et al., 2022), and monsoon rainfall (Bollasina et al., 2011; Polson et al., 2014; Ma et al., 2017; Zhou et al., 2020), though aerosol indirect effects are particularly uncertain and these links are still debated (Oudar et al., 2018; Zhang R. et al., 2019; Baek et al., 2022).
Major volcanic eruptions inject aerosols into the stratosphere, warming the equatorial stratosphere and cooling global mean surface temperature for several years. Warming of the equatorial stratosphere strengthens the polar vortex in both hemispheres with subsequent surface impacts (Robock, 2000; Shindell et al., 2004), though the size of the response (Azoulay et al., 2021; DallaSanta and Polvani, 2022) and whether it is underestimated by climate models (Stenchikov et al., 2006; Driscoll et al., 2012; Swingedouw et al., 2017; Hermanson et al., 2020) is debated. Although not predictable in advance, volcanic eruptions are important for understanding climate variability and provide predictability for several years after they occur (Timmreck et al., 2016; Ménégoz et al., 2018). Multi-annual to decadal impacts of volcanic eruptions include AMV (Otterå et al., 2010; Knudsen et al., 2014; Swingedouw et al., 2017; Wang J. et al., 2017; Birkel et al., 2018; Mann et al., 2021), AMOC (Stenchikov et al., 2009; Mignot et al., 2011; Iwi et al., 2012; Zanchettin et al., 2013; Swingedouw et al., 2015; Hermanson et al., 2020), El Niño (Maher et al., 2015; Khodri et al., 2017; Zuo et al., 2018; Hermanson et al., 2020), PDV (Wang T. et al., 2012; Gregory et al., 2019), tropical cyclones (Evan, 2012; Pausata and Camargo, 2019), and global precipitation patterns (Iles and Hegerl, 2014; Tejedor et al., 2021) including Sahel rainfall (Haywood et al., 2013), monsoons (Fasullo et al., 2019; Zuo et al., 2019a) and rainfall in global arid regions (Zuo et al., 2019b).
Aerosols can also change through biomass burning and natural variations in dust. Biomass burning has been implicated in decadal changes in temperature and the hydrological cycle (Fasullo et al., 2022; Heyblom et al., 2022), and dust variations have been linked to AMV, Sahel rainfall and tropical cyclones (Evan et al., 2011; Wang C. et al., 2012; Strong et al., 2018).
Although changes in solar irradiance are small, they can have significant impacts on climate (Gray et al., 2010). “Top-down” influences occur through increases in ultra-violet radiation and associated ozone feedbacks which warm the tropical stratosphere, strengthening the polar vortex (Kodera and Kuroda, 2002) and promoting a positive NAO. This is seen both in the response to the 11-year solar cycle (Ineson et al., 2011; Gray et al., 2013; Andrews et al., 2015; Thiéblemont et al., 2015; Ma et al., 2018; Kuroda et al., 2021) and on multi-decadal timescales (Shindell et al., 2001; Ineson et al., 2015; Maycock et al., 2015; Chiodo et al., 2016; Spiegl and Langematz, 2020), potentially influencing the Atlantic Ocean and the AMOC (Scaife et al., 2013; Menary and Scaife, 2014). “Bottom-up” influences occur mainly through changes in visible radiation which particularly affect the tropical Pacific, though there is uncertainty whether increased solar irradiance causes a La Niña response (Meehl et al., 2009) or a reduction in the Walker Circulation more typical of El Niño (Misios et al., 2019), and whether the response depends on the timescale of the forcing (Meehl et al., 2013). Furthermore, changes in the tropical Pacific may generate Rossby waves that could impact the NAO (Swingedouw et al., 2011; Chiodo et al., 2016). Solar influences on tropical tropospheric temperatures have also been suggested to influence multi-decadal variations of the Southern Annular Mode (Wright et al., 2022).
Since stratospheric ozone warms the stratosphere by absorbing solar radiation, depletion of ozone over the Antarctic caused local cooling that increased the meridional temperature gradient and promoted a positive SAM in austral summer (Thompson and Solomon, 2002; Son et al., 2010; McLandress et al., 2011; Polvani et al., 2011; Thompson et al., 2011). Conversely, ozone recovery tends to shift the SAM toward the negative phase, opposing the positive SAM signal driven by greenhouse gases (Perlwitz et al., 2008; Banerjee et al., 2020). Stratospheric ozone-driven changes in the SAM are accompanied by changes in the Southern Hemisphere Hadley cell and associated sub-tropical jet (Jebri et al., 2020) and precipitation (Kang et al., 2011; Delworth and Zeng, 2014). Tropospheric ozone may also affect tropical expansion (Allen et al., 2012) and Southern Ocean warming (Liu et al., 2022).
Land use changes affect surface albedo, evapotranspiration and surface roughness, and hence the exchange of heat, moisture, and momentum between the land surface and the atmosphere (Findell et al., 2007; Lawrence et al., 2016; Pongratz et al., 2021). This can result in local changes in temperature, rainfall, and associated extremes (Mueller et al., 2016; Findell et al., 2017), and remote impacts are possible through atmospheric teleconnections (Snyder, 2010; Teng et al., 2019, 2022; Wang et al., 2019).
Multi-annual to decadal changes in climate may also occur in the absence of external drivers through natural internal variability (Cassou et al., 2018), especially in the Atlantic (Knight et al., 2005; Delworth et al., 2007), Pacific (Power et al., 2021), and Southern oceans (Zhang L. et al., 2019), but also the atmosphere (Dimdore-Miles et al., 2022). Moreover, many multi-annual to decadal changes in climate may occur through a combination of external forcing and internal variability (e.g., Huang et al., 2020; Bonnet et al., 2021; Wu et al., 2021) complicating the separation of forcing's from the natural variability in observed changes.
Observations alone are insufficient for robust attribution because it is difficult to separate multiple external drivers and internal variability, causality cannot be assessed unequivocally, and they are not yet available to assess forecast signals. Hence, we propose numerical model experiments that are designed to isolate the impacts of individual external drivers (Table 1). To the extent that the representation of the relevant physical processes in a model can be trusted, these model experiments can indicate the causal influence of external forcing factors on historical trends. However, analysis of these experiments must consider potential model errors (see below), non-additivity of drivers, potential errors in forcings (Wang et al., 2021; Fasullo et al., 2022) and potential impacts of missing processes including glacial melt (Rye et al., 2020; Devilliers et al., 2021). Any changes that cannot be explained by external factors after accounting for all of these factors would be assumed to be internal variability.
Experiment set 1 will enable the modeled response to individual forcings to be determined over the historical period. Experiments 1.1–1.4 are identical to those proposed by the Detection and Attribution Model Intercomparison Project (DAMIP, Gillett et al., 2016) but with increased ensemble size. Experiment 1.5 (hist-totalO3) has the same ozone prescribed as that used in the historical and SSP2-4.5 simulations. In models with interactive ozone chemistry, the simulated ozone from the historical and SSP2-4.5 simulations should be prescribed, though we encourage groups that are able to assess sensitivities to this (Ivanciu et al., 2021). These simulations are complementary to the hist-stratO3 experiments in DAMIP and are included here to complete the set of individual forcings and allow their additivity to be assessed by comparing with All-forcing simulations (experiment 3.1). We encourage groups to perform hist-stratO3 with at least 10 ensemble members if they are able to allow the effects of tropospheric and stratospheric ozone changes to be separately identified. Experiment 1.6 is an important addition to assess the impact of land use and land cover changes in a consistent manner, providing additional information to the experiments proposed in the Land Use Model Intercomparison Project (Lawrence et al., 2016).
Experiment set 2 extends set 1 by using updated estimates and projections of forcings. This will be especially important in the event of the next future major volcanic eruption, but will also allow deviations in aerosol and GHG emissions and other forcings from the scenario used in experiment set 1 to be assessed, potentially allowing the effects of mitigation measures to be simulated, and possibly detected in observations (e.g., Tebaldi and Friedlingstein, 2013). A focus of LHA-EPESC will be to create updated forcing datasets for experiment set 2 in a timely manner, ensuring their compatibility with the CMIP6 forcings used in experiment set 1. Experiment set 2 extends up to 10 years into the future to provide attribution of forecast signals as well as improved attribution of recent changes.
Experiment set 3 enables the additivity of multiple forcings to be assessed (Shiogama et al., 2013) by comparing with the sum of individual forcings from experiment set 1. Note that solar and volcanic influences on ozone that were applied in the single forcing DAMIP protocol will need to be accounted for.
Experiment sets 4 and 5 are similar to 1 and 2 except that each individual forcing is held constant with all others specified according to the All-forcing simulations (Deser et al., 2020). Experiments 3.1 and 3.3 are therefore also required to make use of experiments 4 and 5. By comparing with experiment sets 1 and 2 experiment sets 4 and 5 will allow the influence of changes in the background climate on attribution to be assessed (Meehl et al., 2004; Ming and Ramaswamy, 2009; Deng et al., 2020), and the assumption of linear additivity of the response to forcings to be tested (e.g., Bindoff et al., 2013).
Large ensembles (ideally 50 members, but a minimum of 10) are requested in order to quantify forced signals on regional scales in the presence of internal variability. This requirement exists regardless of model errors, but is amplified by specific challenges in simulating changes in atmospheric circulation which are discussed below. Although fully coupled climate models are needed to simulate the full responses, we also encourage the use of a hierarchy of models, including slab oceans, to assess the roles of ocean-atmosphere coupling and dynamic ocean variability (e.g., Chemke et al., 2022).
A key challenge in analyzing these experiments will be accounting for model errors (Bellprat et al., 2019). For example, although some models simulate AMV in response to external forcings that closely matches observations (Booth et al., 2012; Murphy et al., 2017; Bellomo et al., 2018) whether they do so for the right reasons is debated (Zhang R. et al., 2019). In particular, AMV is strongly related to the NAO integrated over previous decades in observations, but this relationship is much weaker in models (Peings et al., 2016; O'Reilly et al., 2019; Lai et al., 2022). Furthermore, models underestimate multi-decadal variability, including for temperatures (Cheung et al., 2017; Kravtsov, 2017; Qasmi et al., 2017), especially for the North Atlantic (Wang X. et al., 2017; Kim et al., 2018), the North Atlantic atmospheric jet (Bracegirdle et al., 2018; Simpson et al., 2018), and trends in the NAO (Eade et al., 2022).
A potentially very important model error is the underestimation of predictable or forced atmospheric circulation signals. This has been termed the “signal-to-noise paradox” (SNP, Scaife and Smith, 2018) since a model can unexpectedly predict the real world better than itself despite being a perfect representation of itself. First diagnosed in seasonal forecasts of the NAO (Eade et al., 2014; Scaife et al., 2014) the SNP has now been found in sub-seasonal (Domeisen et al., 2020), seasonal (Baker et al., 2018; Dunstone et al., 2018), interannual (Dunstone et al., 2016, 2020), multi-annual (Sheen et al., 2017; Yeager et al., 2018; Hu and Zhou, 2021) and decadal (Smith et al., 2019a, 2020; Athanasiadis et al., 2020) forecasts. On seasonal timescales the SNP occurs mainly in the extratropics, especially the North Atlantic where the NAO signal is underestimated by a factor of 2 to 3 (Eade et al., 2014; Scaife et al., 2014; Dunstone et al., 2016; Baker et al., 2018). However, on decadal timescales the SNP appears to be stronger, with the NAO underestimated by an order of magnitude (Smith et al., 2020), and more widespread, affecting the tropics as well as the extratropics (Eade et al., 2014; Smith et al., 2019a). Although the SNP may vary over time or be model dependent, it appears to be present whenever the NAO skill is high (Weisheimer et al., 2019). Where atmospheric signals are underestimated, taking models at face value (Deser et al., 2017) will give misleading conclusions about the role of irreducible internal variability. Importantly, the SNP is also found in uninitialized historical simulations (Sévellec and Drijfhout, 2019; Zhang and Kirtman, 2019; Klavans et al., 2021; Zhang et al., 2021). It is therefore not simply an artifact of initialization, and models underestimate the response to at least some external forcings. This is consistent with other evidence that modeled responses to volcanoes (Stenchikov et al., 2006; Driscoll et al., 2012; Swingedouw et al., 2017; Hermanson et al., 2020) and solar variability (Stott et al., 2003; Matthes et al., 2004; Gray et al., 2013; Scaife et al., 2013) may be too weak, and may explain the lack of signals noted in some studies (Schurer et al., 2014; Chiodo et al., 2019; Azoulay et al., 2021; DallaSanta and Polvani, 2022).
The cause of the SNP is currently unknown, though underestimated eddy feedback (Scaife et al., 2019; Hardiman et al., 2022) and errors in ocean-atmosphere interactions (Ossó et al., 2020; Zhang et al., 2021) could be important. For atmospheric circulation patterns such as the NAO, the error can potentially be overcome by taking the mean of a very large ensemble to diagnose the modeled signal and then inflating its variance to match the observed predictable component (Eade et al., 2014; Smith et al., 2020). The ensemble size required scales as the square of the signal deficit: an order of magnitude underestimation of the decadal NAO signal requires 100 times more ensemble members than would a perfect model (Smith et al., 2020). Hence, LESFMIP requests large ensembles (Table 1). However, scaling will not overcome SNP-related errors in variables such as temperature and rainfall, which are impacted by atmospheric circulation errors (Smith et al., 2020). To illustrate why, we partition a climate signal (T) into dynamically (Tdyn) and thermodynamically (Ttherm) driven components:
where ε is the residual. In some regions, Tdyn≫Ttherm in reality, but Tdyn≪Ttherm in the model ensemble mean if the atmospheric circulation response to forcings is severely underestimated. Scaling the ensemble mean will retain the incorrect ratio and fail to attribute dynamical signals.
To overcome this error new approaches are needed to analyse model output. We illustrate this for the extreme positive NAO period (1986–1997) for which observations show a clear pattern of warm anomalies over northern Europe and south-east USA and cold anomalies over northern Africa and eastern Canada (Figure 1A). The raw ensemble mean of decadal predictions shows very little signal (Figure 1B) and scaling to match the observed variance shows little improvement (Figure 1C) because the dynamical signals that produced the observed extremes are underestimated. A potential solution is to select ensemble members that, by chance, have the correct magnitude of dynamical signals. The correct magnitude is diagnosed by scaling the ensemble mean NAO to match the observed predictable component, and can be obtained in forecasts and projections since it only requires the scaling factor to be diagnosed from past cases. Note that if the predictable or forced signals are underestimated then no single ensemble member would be expected to reproduce the observed timeseries, and the selected ensemble members will likely be different at each time. This approach, referred to as “NAO-matching” (Smith et al., 2020), reveals much more realistic temperature patterns (Figure 1D) and similar improvements for northern European rainfall (Figures 1E,F). It also improves AMV (Figures 1G,H) suggesting that NAO variations cannot be solely attributed to AMV and revealing an important dynamical influence on AMV that would affect attribution analysis. Note that other approaches such as using observed regression, analogs from models or observations (Deser et al., 2016; Sippel et al., 2019), or ensemble sub-selection based on drivers (Dobrynin et al., 2018), are also possible and warrant further investigation.
Figure 1. The need for new approaches to assess model output. Near surface temperature anomalies for the extreme NAO period (1986–1997) in (A) observations, and decadal predictions shown as (B) raw ensemble mean, (C) scaled ensemble mean to match the observed variance at each grid point, (D) NAO-matched ensemble mean. Middle and right columns show observed 8-year mean time-series (black) and decadal predictions (years 2–9, ensemble mean in red with 5–95% confidence interval shaded) as raw ensemble means (middle) and NAO-matched ensemble means (right) for (E,F) northern European rainfall (10°W−25°E, 55–70°N) and (G,H) AMV index (Trenberth and Shea, 2006). Decadal predictions start each year from 1960 to 2005 and consist of a total of 169 ensemble members from 13 different models. (A–D) Show standardized values, obtained by dividing by the standard deviation of rolling 8-year means at each grid point. (A,B) show signals standardized by the observed variability, (C,D) show signals standardized by the ensemble mean variability. NAO-matching is achieved by selecting the 20 ensemble members at each time that are closest to the ensemble mean after scaling this to account for its underestimation of the magnitude of the predictable signal. All panels show boreal winter (December-March) anomalies relative the average over all year 2–9 predictions. Adapted from Smith et al. (2020), where further details are available.
An important aspect of the analysis of LESFMIP experiments will be to diagnose the real-world response to individual forcings over the historical period. We propose to develop “emergent constraints” (Hall et al., 2019) that exploit differences between models and relate them to observed quantities. For example, the atmospheric response to future Arctic sea ice loss depends on atmospheric eddy feedback which is underestimated by models, constraining the real world toward the upper end of the model simulations (Smith et al., 2022). Multi-model LESFMIP simulations are therefore essential, as is the need to develop constraints directly tied to the underlying physical processes (Smith et al., 2022).
The optimum analysis procedure will require further research and will be developed as the experimental results become available. Based on the discussion above, the key ingredients must allow for potentially underestimated atmospheric circulation signals and should therefore not treat model ensemble members as alternative realizations of the observations. As a start we suggest the following:
1. For a given event or forecast signal, identify the relevant patterns of atmospheric circulation.
2. For each model ensemble mean, perform “detection and attribution” analysis (multiple linear regression) on the atmospheric circulation patterns to obtain scaling factors for each driver.
3. Develop emergent constraints to exploit differences in scaling factors between models to diagnose the influence of each driver in the real-world.
4. Sub-select ensemble members, or obtain model or observed analogs, which match the real-world influence of each driver diagnosed in step 3.
5. For the variable of interest compute the contribution of each driver using the sub-selected or analogs ensembles from step 4.
Step 3 in particular is non-trivial and will require further research to understand the physical reasons for model differences. Even if constraints cannot be found, the experiments will still be extremely valuable for assessing model uncertainties in the potential roles of individual drivers and internal variability, and for highlighting specific areas where model improvements are needed. Step 2 assumes linear additivity of forcings which should be assessed using experiment set 3 or other methods. The LESFMIP experiments will also allow the investigation of pattern recognition to single out the influence of external forcing in ensemble simulations (Wills et al., 2020). Step 4 requires further research to extend NAO-matching or similar techniques to other modes of atmospheric variability. Further developments will also be required to account for uncertainties in forcing and observations, and to assess potential non-linearities and dependencies on the background state. The analysis plan proposed here could potentially be applied to extreme events as well as multi-year to decadal signals, enabling model errors and differences to be taken into account in extreme event attribution studies (e.g., van Oldenborgh et al., 2022), though further testing will be required to assess this application.
The requested diagnostics are the same for all LESFMIP experiments. Given the large ensemble sizes the data request is substantially reduced and is the same as for the Polar Amplification Model Intercomparison Project (PAMIP, Smith et al., 2019b): Appendix D in Boer et al., 2016 with the addition of wave activity diagnostics [Table 3 in Smith et al. (2019b), see Gerber and Manzini (2016), for details on how to compute these variables]. In addition, we request monthly mean ambient aerosol optical thickness at 550 nm (od550aer) to assess aerosol forcing. We stress that the data request is not intended to exclude other variables and participants are encouraged to retain variables requested by other MIPs if possible. The model output from LESFMIP will be distributed through the Earth System Grid Federation (ESGF).
There is an urgent need to better understand the drivers of multi-annual to decadal changes in climate both to attribute recent events, including extremes, and to gain further confidence in forecasts that are issued each year by the WMO. Multi-annual to decadal changes in climate are influenced by multiple factors including greenhouse gases, aerosols, ozone, land use, volcanic eruptions, solar variations, and internal variability. The Large Ensemble Single Forcing Model Intercomparison Project (LESFMIP) documented here proposes a set of coordinated model experiments to isolate and assess the impacts of these different factors. Additional experiments are included to take advantage of updated estimates of forcings, and to assess potential non-linearities and dependencies on the background state. A key part of the analysis will be to account for model errors. For this, multi-model simulations are needed to diagnose the real-world situation by exploiting model differences with constraints based on the key physical processes, though research will be required to identify these. Large ensembles are also needed to extract the forced signals which may be too weak in models, especially for changes in atmospheric circulation. In this case it will also be necessary to adopt new approaches to analyse the model output, possibly by selecting those ensemble members with the required magnitude of atmospheric circulation signals. The simulations proposed here could lead to a step change in our ability to understand regional climate variability, change and predictability, and will be an important contribution to the WCRP Lighthouse Activity on Explaining and Predicting Earth System Change goal to develop an operational capability to attribute multi-annual to decadal changes in climate.
The original contributions presented in the study are included in the article/supplementary material, further inquiries can be directed to the corresponding author/s.
DS led the design and writing. NG, IS, PA, JB, IB, TB, RB, OB, KF, GG, SG, LH, LL, JM, WM, SO, OO, GP, AS, GS, HS, RS, DS, SY, TZh, and TZi commented on the design and manuscript. All authors contributed to the article and approved the submitted version.
DS, LH, and AS were supported by the Met Office Hadley Centre Climate Programme funded by BEIS and Defra. DS, RB, and OB were also supported by the European Commission Horizon 2020 CONSTRAIN project (GA 820829). Climate modeling at GISS is supported by the NASA Modeling, Analysis and Prediction program. LL was supported by U.S. Department of Energy Office of Science Biological and Environmental Research through the Global and Regional Model Analysis program area. PNNL is operated for DOE by the Battelle Memorial Institute under contract DE-AC05-76RL01830. TZi received funding from the Australian Government under the National Environmental Science Program. RS was supported by the UK National Centre for Atmospheric Science. HS was supported by the Advanced Studies of Climate Change Projection (SENTAN, JPMXD0722680395) of the Ministry of Education, Culture, Sports, Science and Technology of Japan. SY was supported by the Danish National Centre for Climate Research (NCKF). IS was supported by the National Center for Atmospheric Research, which is a major facility sponsored by the National Science Foundation under the Cooperative Agreement 1852977. JM and GG were also supported by the JPI climate/JPI ocean ROADMAP ANR-19-JPOC-003 and ANR-MOPGA ARCHANGE projects. TZh was supported by the National Natural Science Foundation of China under Grant No. 41988101.
Thanks to Hiro Murakami for providing valuable comments on a draft of the manuscript and to Dave Plummer for input on ozone datasets.
The authors declare that the research was conducted in the absence of any commercial or financial relationships that could be construed as a potential conflict of interest.
All claims expressed in this article are solely those of the authors and do not necessarily represent those of their affiliated organizations, or those of the publisher, the editors and the reviewers. Any product that may be evaluated in this article, or claim that may be made by its manufacturer, is not guaranteed or endorsed by the publisher.
Ackerley, D., Booth, B. B., Knight, S. H., Highwood, E. J., Frame, D. J., Allen, M. R., et al. (2011). Sensitivity of 20th-century Sahel rainfall to sulfate aerosol and CO2 forcing. J. Clim. 24 4999–5014 doi: 10.1175/JCLI-D-11-00019.1
Allen, R. J., Norris, J. R., and Kovilakam, M. (2014). (2014). Influence of anthropogenic aerosols and the pacific decadal oscillation on tropical belt width. Nat. Geosci. 7, 270–274. doi: 10.1038/ngeo2091
Allen, R. J., Sherwood, S. C., Norris, J. R., and Zender, C. S. (2012). Recent North Hemisphere tropical expansion primarily driven by black carbon and tropospheric ozone. Nature 485, 350–354. doi: 10.1038/nature11097
Andrews, M. B., Knight, J. R., and Gray, L. J. (2015). A simulated lagged response of the North Atlantic Oscillation to the solar cycle over the period 1960–2009. Environ. Res. Lett. 10, 1–10. doi: 10.1088/1748-9326/10/5/054022
Athanasiadis, P. J., Yeager, S., Kwon, Y. O., Bellucci, A., Smith, D. W., Tibaldi, S., et al. (2020). Decadal predictability of North Atlantic blocking and the NAO. NPJ Clim. Atmos. Sci. 3, 20. doi: 10.1038/s41612-020-0120-6
Azoulay, A., Schmidt, H., and Timmreck, C. (2021). The arctic polar vortex response to volcanic forcing of different strengths. J. Geophys. Res. Atmos. 126, 1–18. doi: 10.1029/2020JD034450
Baek, S. H., Kushnir, Y., Ting, M., Smerdon, J. E., and Lora, J. M. (2022). Regional signatures of forced north atlantic sst variability: a limited role for aerosols and greenhouse gases. Geophys. Res. Lett. 49, e2022GL097794. doi: 10.1029/2022GL097794
Baker, L. H., Shaffrey, L. C., Sutton, R. T., Weisheimer, A., and Scaife, A. A. (2018). An intercomparison of skill and over/underconfidence of the wintertime North Atlantic Oscillation in multi-model seasonal forecasts. Geophys. Res. Lett. 45, 7808–7817. doi: 10.1029/2018GL078838
Banerjee, A., Fyfe, J. C., Polvani, L. M., Waugh, D., and Chang, K. A. (2020). Pause in Southern Hemisphere circulation trends due to the Montreal Protocol. Nature 579, 544–548. doi: 10.1038/s41586-020-2120-4
Bellomo, K., Murphy, L. N., Cane, M. A., Clement, A. C., and Polvani, L. M. (2018). Historical forcings as main drivers of the Atlantic multidecadal variability in the CESM large ensemble. Clim. Dyn. 50, 3687–3698. doi: 10.1007/s00382-017-3834-3
Bellprat, O., Guemas, V., Doblas-Reyes, F., and Donat, M. (2019). Towards reliable extreme weather and climate event attribution. Nat. Commun. 10, 1732. doi: 10.1038/s41467-019-09729-2
Bellucci, A., Mariotti, A., and Gualdi, S. (2017). The role of forcings in the twentieth-century North Atlantic multidecadal variability: the 1940–75 North Atlantic cooling case study. J. Clim. 30, 7317–7337. doi: 10.1175/JCLI-D-16-0301.1
Bindoff, N. L., Stott, P. A. K. M., AchutaRao, M. R., Allen, N., Gillett, D., Gutzler, K., et al. (2013). “Detection and attribution of climate change: from global to regional,” in Climate Change 2013: The Physical Science Basis,” in Contribution of Working Group I to the Fifth Assessment Report of the Intergovernmental Panel on Climate Change, eds T.F. Stocker, D. Qin, G.-K. Plattner, M. Tignor, S.K. Allen, J. Boschung, A. et al. (Cambridge: Cambridge University Press).
Birkel, S. D., Mayewski, P. A., Maasch, K. A., Kurbatov, A. V., and Lyon, B. (2018). Evidence for a volcanic underpinning of the Atlantic multidecadal oscillation. NPJ Clim. Atmos. Sci. 1, 24. doi: 10.1038/s41612-018-0036-6
Boer, G. J., Smith, D. M., Cassou, C., Doblas-Reyes, F., Danabasoglu, G., Kirtman, B., et al. (2016). The decadal climate prediction project (DCPP) contribution to CMIP6. Geosci. Model Dev. 9, 3751–3777. doi: 10.5194/gmd-9-3751-2016
Bollasina, M. A., Ming, Y., and Ramaswamy, V. (2011). Anthropogenic aerosols and the weakening of the South Asian summer monsoon. Science 334, 502–505. doi: 10.1126/science.1204994
Bonnet, R., Swingedouw, D., Gastineau, G., Boucher, O., Deshayes, J., Hourdin, F., et al. (2021). Increased risk of near term global warming due to a recent AMOC weakening. Na.t Commun. 12, 6108. doi: 10.1038/s41467-021-26370-0
Booth, B. B. B., Dunstone, N. J., Halloran, P. R., Andrews, T., and Bellouin, N. (2012). Aerosols implicated as a prime driver of twentieth-century North Atlantic climate variability. Nature 484, 228–232. doi: 10.1038/nature10946
Borchert, L. F., Menary, M. B., Swingedouw, D., Sgubin, G., Hermanson, L., and Mignot, J. (2021). Improved decadal predictions of North Atlantic subpolar gyre SST in CMIP6. Geophys. Res. Lett. 48, e2020GL091307 doi: 10.1029/2020GL091307
Borchert, L. F., Pohlmann, H., Baehr, J., Neddermann, N.-C., Suarez-Gutierrez, L., Müller, W. A., et al. (2019). Decadal predictions of the probability of occurrence for warm summer temperature extremes. Geophys. Res. Lett. 46, 14042–14051. doi: 10.1029/2019GL085385
Bracegirdle, T., Lu, H., Eade, R., and Woollings, T. (2018). Do CMIP5 models reproduce observed low-frequency north Atlantic jet variability? Geophys. Res. Lett. 45, 7204–7212. doi: 10.1029/2018GL078965
Canadell, J. G., Meyer, C. P., Cook, G. D., Dowdy, A., Briggs, P. R., Knauer, J., et al. (2021). Multi-decadal increase of forest burned area in Australia is linked to climate change. Nat. Commun. 12, 1–11. doi: 10.1038/s41467-021-27225-4
Caron L-. P. Hermonson L. Dobbin A. Imbers J. Lledó L. Vecchi G. A. (2018)How skillful are the multiannual forecasts of Atlantic hurricane activity? Bull. Amer. Meteor. Soc. 99, 403–413. doi: 10.1175/BAMS-D-17-0025.1
Cassou, C., Kushnir, Y., Hawkins, E., Pirani, A., Kucharski, F., Kang, I., et al. (2018). Decadal climate variability and predictability: challenges and opportunities. Bull. Am. Meteorol. Soc. 99, 479–490. doi: 10.1175/BAMS-D-16-0286.1
Chemke, R., Zanna, L., Orbe, C., Sentman, L. T., and Polvani, L. M. (2022). The future intensification of North Atlantic winter storms: The key role of dynamic ocean coupling. J. Clim. 35, 2407–2421. doi: 10.1175/JCLI-D-21-0407.1
Cheung, A. H., Mann, M. E., Steinman, B. A., Frankcombe, L. M., England, M. H., and Miller, S.K. (2017). Comparison of low-frequency internal climate variability in CMIP5 models and observations. J. Clim. 30, 4763–4776. doi: 10.1175/JCLI-D-16-0712.1
Chikamoto, Y., Timmermann, A., Widlansky, M. J., Balmaseda, M. A., and Stott, L. (2017). Multi-year predictability of climate, drought, and wildfire in southwestern North America. Sci. Rep. 7, 6568. doi: 10.1038/s41598-017-06869-7
Chiodo, G., García-Herrera, R., Calvo, N., Vaquero, J. M., Añel, J. A., Barriopedro, D., et al. (2016). The impact of a future solar minimum on climate change projections in the Northern Hemisphere. Environ. Res. Lett. 11, 034015. doi: 10.1088/1748-9326/11/3/034015
Chiodo, G., Oehrlein, J., Polvani, L. M., Fyfe, J. C., and Smith, A. K. (2019). Insignificant influence of the 11-year solar cycle on the North Atlantic Oscillation. Nat. Geosci. 12, 94–99. doi: 10.1038/s41561-018-0293-3
DallaSanta, K., and Polvani, L. M. (2022). Volcanic stratospheric injections up to 160 Tg(S) yield a Eurasian winter warming indistinguishable from internal variability. Atmos. Chem. Phys. Discuss. 22, 8843–8862. doi: 10.5194/acp-2022-58
Dawkins, L. C., Stephenson, D. B., Lockwood, J. F., and Maisey, P. E. (2016). The 21st century decline in damaging European windstorms. Nat. Hazards Earth Syst. Sci. 16, 1999–2007. doi: 10.5194/nhess-16-1999-2016
Delworth, T., and Zeng, F. (2014). Regional rainfall decline in Australia attributed to anthropogenic greenhouse gases and ozone levels. Nat. Geosci. 7, 583–587. doi: 10.1038/ngeo2201
Delworth, T. L., Zhang, R., and Mann, M. E. (2007). Decadal to centennial variability of the Atlantic from observations and models. Geophys. Monograph Am. Geophys. Union 173, 131. doi: 10.1029/173GM10
Deng, J., Dai, A., and Xu, H. (2020). Nonlinear climate responses to increasing CO2 and anthropogenic aerosols simulated by, C. E. S. M. J. Clim. 33, 281–301. doi: 10.1175/JCLI-D-19-0195.1
Deser, C., Hurrell, J. W., and Phillips, A. S. (2017). The role of the North Atlantic Oscillation in European climate projections. Clim. Dyn. 49, 3141–3157. doi: 10.1007/s00382-016-3502-z
Deser, C., Phillips, A. S., Simpson, I. R., Rosenbloom, N., Coleman, D., Lehner, F., et al. (2020). Isolating the evolving contributions of anthropogenic aerosols and greenhouse gases: a new CESM1 large ensemble community resource. J. Clim. 33, 7835–7858. doi: 10.1175/JCLI-D-20-0123.1
Deser, C., Terray, L., and Phillips, A. S. (2016). Forced and internal components of winter air temperature trends over North America during the past 50 years: mechanisms and implications. J. Clim. 6, 2237–2258. doi: 10.1175/JCLI-D-15-0304.1
Devilliers, M., Swingedouw, D., Mignot, J., Deshayes, J., Garric, G., and Ayache, M. (2021). A realistic Greenland ice sheet and surrounding glaciers and ice caps melting in a coupled climate model. Clim. Dynamics 57, 2467–2489. doi: 10.1007/s00382-021-05816-7
Dimdore-Miles, O., Gray, L., Osprey, S., Robson, J., Sutton, R., and Sinha, B. (2022). Interactions between the stratospheric polar vortex and Atlantic circulation on seasonal to multi-decadal timescales. Atmos. Chem. Phys. 22, 4867–4893. doi: 10.5194/acp-22-4867-2022
Dittus, A. J., Hawkins, E., Robson, J. I., Smith, D. M., and Wilcox, L. J. (2021). Drivers of recent North Pacific Decadal Variability: The role of aerosol forcing. Earth's Future 9, e2021EF002249. doi: 10.1029/2021EF002249
Doblas-Reyes, F. J., Andreu-Burillo, I., Chikamoto, Y., Garcia-Serrano, J., Guemas, V., Kimoto, M., et al. (2013). Initialized near-term regional climate change prediction. Nat. Commun. 4, 1715. doi: 10.1038/ncomms2704
Dobrynin, M., Domeisen, D. I., Müller, W. A., Bell, L., Brune, S., Bunzel, F., and Baehr, J. (2018). Improved teleconnection-based dynamical seasonal predictions of boreal winter. Geophys. Res. Lett. 45, 3605–3614. doi: 10.1002/2018GL077209
Domeisen, D., Butler, A. H., Charlton-Perez, A. J., Ayarzaguena, B., Baldwin, M. P., Dunn-Sigouin, E., et al. (2020). The role of the stratosphere in subseasonal to seasonal prediction. Part I: predictability of the stratosphere. J. Geophys. Res. Atmos. 124, 531. doi: 10.1029/2019JD030923
Dong, B., Sutton, R. T., Shaffrey, L., et al. (2022). Recent decadal weakening of the summer Eurasian westerly jet attributable to anthropogenic aerosol emissions. Nat. Commun. 13, 1148. doi: 10.1038/s41467-022-28816-5
Dow, W. J., Maycock, A. C., Lofverstrom, M., and Smith, C. J. (2021). The effect of anthropogenic aerosols on the Aleutian low. J. Clim. 34, 1725–1741. doi: 10.1175/JCLI-D-20-0423.1
Driscoll, S., Bozzo, A., Gray, L. J., Robock, A., and Stenchikov, G. (2012). Coupled Model Intercomparison Project 5 (CMIP5) simulations of climate following volcanic eruptions. J. Geophys. Res. 117, D17105. doi: 10.1029/2012JD017607
Dunstone, N., Smith, D., Yeager, S., Danabasoglu, G., Monerie, P.-A., Hermanson, L., et al. (2020). Skilful interannual climate prediction from two large initialised model ensembles. Environ. Res. Lett. 15, 094083. doi: 10.1088/1748-9326/ab9f7d
Dunstone, N. J., Smith, D., Scaife, A., Hermanson, L., Eade, R., Robinson, N., et al. (2016). Skilful predictions of the winter North Atlantic Oscillation one year ahead. Nat. Geosci. 9, 809–814. doi: 10.1038/ngeo2824
Dunstone, N. J., Smith, D., Scaife, A., Hermanson, L., Fereday, D., O'Reilly, C., et al. (2018). Skilful seasonal predictions of Summer European rainfall. Geophys. Res. Letts. 45, 3246–3254.doi: 10.1002/2017GL076337
Dunstone, N. J., Smith, D. M., Booth, B. B. B., Hermanson, L., and Eade, R. (2013). Anthropogenic aerosol forcing of Atlantic tropical storms. Nat. Geosci. 6, 534–539. doi: 10.1038/ngeo1854
Eade, R., Smith, D., Scaife, A., Wallace, E., Dunstone, N., Hermanson, L., et al. (2014). Do seasonal-to-decadal climate predictions underestimate the predictability of the real world? Geophys. Res. Lett. 41, 5620–5628. doi: 10.1002/2014GL061146
Eade, R., Stephenson, D. B., Scaife, A. A., and Smith, D. M. (2022). Quantifying the rarity of extreme multi-decadal trends: how unusual was the late twentieth century trend in the North Atlantic Oscillation? Clim. Dyn. 58, 1555–1568. doi: 10.1007/s00382-021-05978-4
Evan, A., Foltz, G., Zhang, D., and Vimont, D. J. (2011). Influence of African dust on ocean–atmosphere variability in the tropical Atlantic. Nat. Geosci. 4, 762–765. doi: 10.1038/ngeo1276
Evan, A. T. (2012). Atlantic hurricane activity following two major volcanic eruptions. J. Geophys. Res. 117, D06101. doi: 10.1029/2011JD016716
Eyring, V., Bony, S., Meehl, G. A., Senior, C. A., Stevens, B., Stouffer, R. J., et al. (2016). Overview of the coupled model intercomparison project phase 6 (CMIP6) experimental design and organisation. Geosci. Model Dev. 8, 10539–10583. doi: 10.5194/gmdd-8-10539-2015
Fasullo, J. T., Lamarque, J.-F., Hannay, C., Rosenbloom, N., Tilmes, S., DeRepentigny, P., et al. (2022). Spurious late historical-era warming in CESM2 driven by prescribed biomass burning emissions. Geophys. Res. Lett. 49, e2021GL.097420. doi: 10.1029/2021GL097420
Fasullo, J. T., Otto-Bliesner, B. L., and Stevenson, S. (2019). The influence of volcanic aerosol meridional structure on monsoon responses over the last millennium. Geophys. Res. Lett. 46, 12350–12359. doi: 10.1029/2019GL084377
Findell, K. L., Berg, A., Gentine, P., Krasting, J. P., Lintner, B. R., Malyshev, S., et al. (2017). The impact of anthropogenic land use and land cover change on regional climate extremes. Nat. Commun. 8, 989. doi: 10.1038/s41467-017-01038-w
Findell, K. L., Shevliakova, E., Milly, P. C. D., and Stouffer, R. J. (2007). Modeled impact of anthropogenic land cover change on climate. J. Clim. 20, 3621–3634. doi: 10.1175/JCLI4185.1
Findell, K. L., Sutton, R. T., Caltabiano, N., Brookshaw, A., Heimbach, P., Kimotot, M., et al. (2022). Explaining and predicting earth system change: A world climate research programme call to action. Bull. Amer. Met. Soc. [Un published].
Fischer, E. M., Sippel, S., and Knutti, R. (2021). Increasing probability of record-shattering climate extremes. Nat. Clim. Chang. 11, 689–695. doi: 10.1038/s41558-021-01092-9
Fyfe, J. C., Kharin, V. V., Santer, B. D., Cole, J. N. S., and Gillett, N. P. (2021). Significant impact of forcing uncertainty in a large ensemble of climate model simulations. Proc. National Acad. Sci. 118, e2016549. doi: 10.1073/pnas.2016549118
Gerber, E. P., and Manzini, E. (2016). The Dynamics and Variability Model Intercomparison Project (DynVarMIP) for CMIP6: assessing the stratosphere–troposphere system. Geosci. Model Dev. 9, 3413–3425. doi: 10.5194/gmd-9-3413-2016
Gillett, N. P., Fyfe, J. C., and Parker, D. E. (2013). Attribution of observed sea level pressure trends to greenhouse gas, aerosol, and ozone changes. Geophys. Res. Lett. 40, 2302–2306. doi: 10.1002/grl.50500
Gillett, N. P., Shiogama, H., Funke, B., Hegerl, G., Knutti, R., Matthes, K., et al. (2016). The Detection and Attribution Model Intercomparison Project (DAMIP v1.0) contribution to CMIP6. Geosci. Model Dev. 9, 3685–3697. doi: 10.5194/gmd-9-3685-2016
Goldenberg, S. B., Landsea, C. W., Mestas-Nunez, A. M., and Gray, W. M. (2001). The recent increase in Atlantic hurricane activity: Causes and implications. Science 293, 474–479. doi: 10.1126/science.1060040
Gray, L. J., Beer, J., Geller, M., Haigh, J. D., Lockwood, M., Matthes, K., et al. (2010). Solar influence on climate. Rev. Geophys. 48, RG4001. doi: 10.1029/2009RG000282
Gray, L. J., Scaife, A. A., Mitchell, D. M., Osprey, S., Ineson, S., Hardiman, S., et al. (2013). A lagged response to the 11-year solar cycle in observed winter Atlantic/European weather patterns. J. Geophys. Res. Atmos. 118, 405–420. doi: 10.1002/2013JD020062
Gregory, J. M., Andrews, T., Ceppi, P., Mauritsen, T., and Webb, M. J. (2019). How accurately can the climate sensitivity to CO2 be estimated from historical climate change? Clim. Dyn. 54, 129–157. doi: 10.1007/s00382-019-04991-y
Hall, A., Cox, P., Huntingford, C., and Klein, S. (2019). Progressing emergent constraints on future climate change. Nat. Clim. Change 9, 269–278. doi: 10.1038/s41558-019-0436-6
Hardiman S. C. Dunstone N. J. Scaife A. A. Smith D. M. Comer R. and Ren, H.-L. (2022). Missing eddy feedback may explain weak signal to noise ratios in climate predictions, NPJ Clim. Atmos. Sci. 5, 57. doi: 10.1038/s41612-022-00280-4
Hassan, T., Allen, R. J., Liu, W., and Randles, C. A. (2021). Anthropogenic aerosol forcing of the Atlantic meridional overturning circulation and the associated mechanisms in CMIP6 models. Atmos. Chem. Phys. 21, 5821–5846. doi: 10.5194/acp-21-5821-2021
Haywood, J., Jones, A., Bellouin, N., and Stevenson, D. (2013). Asymmetric forcing from stratospheric aerosols impacts Sahelian rainfall. Nat. Clim. Change 3, 660–665. doi: 10.1038/nclimate1857
Hegerl, G. C., Brönnimann, S., Schurer, A., and Cowan, T. (2018). The early 20th century warming: anomalies, causes, and consequences. WIREs Clim. Chang. 9, e522. doi: 10.1002/wcc.522
Hermanson, L., Bilbao, R., Dunstone, N., Menegoz, M., Ortega, P., Pohlmann, H., et al. (2020). Multi-year climate impacts of volcanic eruptions. J. Geophys. Res. Atmos. 125, e2019JD031739. doi: 10.1029./2019JD031739
Hermanson, L., Eade, R., Robinson, N. H., Dunstone, N. J., Andrews, M. B., Knight, J. R., et al. (2014). Forecast cooling of the Atlantic subpolar gyre and associated impacts. Geophys. Res. Letts. 41, 5167–5174. doi: 10.1002/2014GL060420
Hermanson, L., Smith, D., Seabrook, M., Bilbao, R., Doblas-Reyes, F., Tourigny, E., et al. (2022). WMO global annual to decadal climate update: a prediction for 2021–2025, Bull. Amer. Met. Soc. 103, E1117–E1129. doi: 10.1175/BAMS-D-20-0311.1
Heyblom, K. B., Singh, H. A., Rasch, P. J., and DeRepentigny, P. (2022). Increased variability of biomass burning emissions in CMIP6 amplifies hydrologic cycle in the CESM2 large ensemble. Geophys. Res. Lett. 49, e2021GL.096868. doi: 10.1029/2021GL096868
Hirasawa, H., Kushner, P. J., Sigmond, M., Fyfe, J., and Deser, C. (2022). Evolving sahel rainfall response to anthropogenic aerosols driven by shifting regional, oceanic, and emission influences. J. Clim. 35, 3181–3193. doi: 10.1175/JCLI-D-21-0795.1
Hu, S., and Zhou, T. (2021). Skillful prediction of summer rainfall in the Tibetan Plateau on multiyear time scales. Sci. Adv. 7, eabf9395. doi: 10.1126/sciadv.abf9395
Huang, X., Zhou, T., Turner, A., Dai, A., Chen, X., Clark, R., et al. (2020). The recent decline and recovery of indian summer monsoon rainfall: relative roles of external forcing and internal variability. J. Clim. 33, 5035–5060. doi: 10.1175/JCLI-D-19-0833.1
Iles, C. E., and Hegerl, G. C. (2014). The global precipitation response to volcanic eruptions in the CMIP5 models. Environ. Res. Lett. 9, 104012. doi: 10.1088/1748-9326/9/10/104012
Ineson, S., Maycock, A. C., Gray, L. J., Scaife, A. A., Dunstone, N. J., Harder, J. W., et al. (2015). Regional climate impacts of a possible future grand solar minimum. Nat. Commun. 6, 7535. doi: 10.1038/ncomms8535
Ineson, S., Scaife, A. A., Knight, J. R., Manners, J. C., Dunstone, N. J., Gray, L. J., et al. (2011). Solar forcing of winter climate variability in the Northern Hemisphere. Nat. Geosci. 4, 753–757. doi: 10.1038/ngeo1282
Ivanciu, I., Matthes, K., Wahl, S., Harla,ß, J., and Biastoch, A. (2021). Effects of prescribed CMIP6 ozone on simulating the Southern Hemisphere atmospheric circulation response to ozone depletion. Atmos. Chem. Phys. 21, 5777–5806. doi: 10.5194/acp-21-5777-2021
Iwi, A., Hermanson, L., Haines, K., and Sutton, R. (2012). Mechanisms linking volcanic aerosols to the Atlantic meridional overturning circulation. J. Clim. 25, 3039–3051. doi: 10.1175/2011JCLI4067.1
Jebri, B., Khodri, M., Echevin, V., Gastineau, G., Thiria, S., Vialard, J., et al. (2020). Contributions of internal variability and external forcing to the recent trends in the southeastern pacific and peru–chile upwelling system. J. Climate 33, 10555–10578. doi: 10.1175/JCLI-D-19-0304.1
Kandji, S. T., Verchot, L., and Mackensen, J. (2006). Climate Change and Variability in the Sahel Region: Impacts and Adaptation Strategies in the Agricultural Sector. Nairobi: World Agroforestry Centre (ICRAF) and United Nations Environment Programme (UNEP).
Kang, S. M., Polvani, L. M., Fyfe, J. C., and Sigmond, M. (2011). Impact of polar ozone depletion on subtropical precipitation. Science 332, 951–954. doi: 10.1126/science.1202131
Khodri, M., Izumo, T., Vialard, J., Janicot, S., Cassou, C., Lengaigne, M., et al. (2017). Tropical explosive volcanic eruptions can trigger El Niño by cooling tropical Africa. Nat. Commun. 8, 778. doi: 10.1038/s41467-017-00755-6
Kim, W. M., Yeager, S. G., and Danabasoglu, G. (2018). Key role of internal ocean dynamics in Atlantic multidecadal variability during the last half century. Geophys. Res. Lett. 45, 13449–13457. doi: 10.1029/2018GL080474
Klavans, J. M., Cane, M. A., Clement, A. C., and Murphy, L. N. N. A. O. (2021). Predictability from external forcing in the late 20th century. NPJ Clim. Atmos. Sci. 4, 22. doi: 10.1038/s41612-021-00177-8
Knight, J. R., Allan, R. J., Folland, C. K., Vellinga, M., and Mann, M. E. A. (2005). Signature of persistent natural thermohaline circulation cycles in observed climate. Geophys. Res. Lett. 32, 1–4. doi: 10.1029/2005GL024233
Knudsen, M., Jacobsen, B., Seidenkrantz, M. S., and Olsen, J. (2014). Evidence for external forcing of the Atlantic Multidecadal Oscillation since termination of the Little Ice Age. Nat. Commun. 5, 3323. doi: 10.1038/ncomms4323
Kodera, K., and Kuroda, Y. (2002). Dynamical response to the solar cycle. J. Geophys. Res. 107, D4749. doi: 10.1029/2002JD002224
Kravtsov, S. (2017). Pronounced differences between observed and CMIP5-simulated multidecadal climate variability in the twentieth century. Geophys. Res. Lett. 44, 5749–5757. doi: 10.1002/2017GL074016
Kuroda, Y., Kodera, K., Yoshida, K., Yukimoto, S., and Gray, L. (2021). Influence of the solar cycle on the North Atlantic Oscillation., J. Geophys. Res. Atmos. 127, e2021JD035519. doi: 10.1002/essoar.10507546.1
Kushnir, Y., Scaife, A. A., Arritt, R., Balsamo, G., Boer, G., Doblas-Reyes, F., and Wu, B. (2019). Towards operational predictions of the near-term climate. Nat. Clim. Change 9, 94–101. doi: 10.1038/s41558-018-0359-7
Lai, W. K. M., Robson, J. I., Wilcox, L. J., and Dunstone, N. (2022). Mechanisms of Internal Atlantic Multidecadal Variability in HadGEM3-GC3. 1 at Two Different Resolutions. J. Clim. 35, 1365–1383. doi: 10.1175/JCLI-D-21-0281.1
Lawrence, D. M., Hurtt, G. C., and Arneth, A. (2016). The land use model intercomparison project (LUMIP) contribution to CMIP6: rationale and experimental design. Geosci. Model Dev. 9, 2973–2998. doi: 10.5194/gmd-9-2973-2016
Li, H., Ilyina, T., Müller, W. A., and Landschützer, P. (2019). Predicting the variable ocean carbon sink. Sci. Adv. 5, eaav6471. doi: 10.1126/sciadv.aav6471
Liu, W., Hegglin, M. I., Checa-Garcia, R., Shouwei, L., Gillett, N. P., Lyu, K., et al. (2022). Stratospheric ozone depletion and tropospheric ozone increases drive Southern Ocean interior warming. Nat. Clim. Chang. 12, 365–372. doi: 10.1038/s41558-022-01320-w
Lovenduski, N., Bonan, G. B., Yeager, S. G., Lindsay, K., and Lombardozzi, D. L. (2019b). High predictability of terrestrial carbon fluxes from an initialized decadal prediction system. Environ. Res. Lett. 14, 124074. doi: 10.1088/1748-9326/ab5c55
Lovenduski, N., Yeager, S. G., Lindsay, K., and Long, M. C. (2019a). Predicting nearterm variability in ocean carbon uptake. Earth Syst. Dyn. 10, 45–57. doi: 10.5194/esd-10-45-2019
Ma, H., Chen, H., Gray, L., Zhou, L., Li, X., Wang, R., et al. (2018). Changing response of the North Atlantic/European winter climate to the 11 year solar cycle. Environ. Res. Lett. 13, 034007. doi: 10.1088/1748-9326/aa9e94
Ma, S., Zhou, T., Stone, D. A., Polson, D., Dai, A., Stott, P. A., et al. (2017). Detectable anthropogenic shift toward heavy precipitation over eastern China. J. Clim. 30, 1381–1396. doi: 10.1175/JCLI-D-16-0311.1
Maher, N., McGregor, S., England, M. H., and Gupta, A. S. (2015). Effects of volcanism on tropical variability. Geophys. Res. Lett. 42, 6024–6033. doi: 10.1002/2015GL064751
Mann, M. E., and Emanuel, K. A. (2006). Atlantic hurricane trends linked to climate change. Eos Trans. Am. Geophys. Union 87, 233–241. doi: 10.1029/2006EO240001
Mann, M. E., Steinman, B. A., Brouillette, D. J., and Miller, S. K. (2021). Multidecadal climate oscillations during the past millennium driven by volcanic forcing. Science 371, 1014–1019. doi: 10.1126/science.abc5810
Marvel, K., Biasutti, M., and Bonfils, C. (2020). Fingerprints of external forcing agents on Sahel rainfall: aerosols, greenhouse gases, and model-observation discrepancies. Environ. Res. Lett. 15, 084023. doi: 10.1002/essoar.10502593.1
Matthes, K., Langematz, U., Gray, L. L., Kodera, K., and Labitzke, K. (2004). Improved 11-year solar signal in the Freie Universität Berlin climate middle atmosphere model (FUB-CMAM). J. Geophys. Res. 109, D06101. doi: 10.1029/2003JD004012
Maycock, A. C., Ineson, S., Gray, L. J., Scaife, A. A., Anstey, J. A., Lockwood, M., et al. (2015). Possible impacts of a future grand solar minimum on climate: stratospheric and global circulation changes. J. Geophys. Res. Atmos. 120, 9043–9058. doi: 10.1002/2014JD022022
McLandress, C., Shepherd, T. G., Scinocca, J. F., Plummer, D. A., Sigmond, M., Jonsson, A. I., et al. (2011). Separating the dynamical effects of climate change and ozone depletion. Part II: southern hemisphere troposphere. J. Clim. 24, 1850–1868. doi: 10.1175/2010JCLI3958.1
Medhaug, I., Stolpe, M., Fischer, E., and Knutti, R. (2017). Reconciling controversies about the ‘global warming hiatus, '. Nature 545, 41–47. doi: 10.1038/nature22315
Meehl, G. A., Arblaster, J. M., and Marsh, D. R. (2013). Could a future grand solar minimum like the maunder minimum stop global warming? Geophys. Res. Lett. 40, 1789–1793 doi: 10.1002/grl.50361
Meehl, G. A., Arblaster, J. M., Matthes, K., Sassi, F., and van Loon, H. (2009). Amplifying the Pacific climate system response to a small 11-year solar cycle forcing. Science 325, 1114–1118. doi: 10.1126/science.1172872
Meehl, G. A., Washington, W. M., Ammann, C. M., Arblaster, J. M., Wigley, T. M. L., and Tebaldi, C. (2004). Combinations of natural and anthropogenic forcings in twentieth-century climate. J. Clim. 17, 3721–3727. doi: 10.1175/1520-0442(2004)017%3C3721:CONAAF%3E2.0.CO;2
Menary, M. B., Robson, J., Allan, R. P., Booth, B. B. B., Cassou, C., Gastineau, G., et al. (2020). Aerosol-forced AMOC changes in CMIP6 historical simulations. Geophys. Res. Lett. 47, e2020GL.088166. doi: 10.1029/2020GL088166
Menary, M. B., and Scaife, A. A. (2014). Naturally forced multidecadal variability of the Atlantic meridional overturning circulation. Clim. Dyn. 42, 1347–1362. doi: 10.1007/s00382-013-2028-x
Ménégoz, M., Bilbao, R., Bellprat, O., Guemas, V., and Doblas-Reyes, F. J. (2018). Forecasting the climate response to volcanic eruptions: Prediction skill related to stratospheric aerosol forcing. Environ. Res. Lett. 13, 64022. doi: 10.1088/1748-9326/aac4db
Mignot, J., Khodri, M., Frankignoul, C., and Servonnat, J. (2011). Volcanic impact on the Atlantic Ocean over the last millennium. Clim. Past 7, 1439–1455. doi: 10.5194/cp-7-1439-2011
Ming, Y., and Ramaswamy, V. (2009). Nonlinear climate and hydrological responses to aerosol effects. J. Clim. 22, 1329–1339. doi: 10.1175/2008JCLI2362.1
Misios, S., Gray, L. J., Knudsen, M. F., Karoff, C., Schmidt, H., Haigh, J. D., et al. (2019). Slowdown of the Walker circulation at solar cycle maximum. Proc. Natl. Acad. Sci. U. S. A 116, 7186–7191. doi: 10.1073/pnas.1815060116
Monerie, P. A., Robson, J., Dong, B., and Dunstone, D. A. (2018). Role of the Atlantic Ocean in predicting summer surface air temperature over North East Asia? Cli.m Dyn. 51, 473–491. doi: 10.1007/s00382-017-3935-z
Mueller, N., Butler, E., McKinnon, K., Rhines, A., Tingley, M., Holbrook, N. M., et al. (2016). Cooling of US Midwest summer temperature extremes from cropland intensification. Nature Clim. Change 6, 317–322. doi: 10.1038/nclimate2825
Müller, W. A., Baehr, J., Haak, H., Jungclaus, J. H., Kröger, J., Matei, D., et al. (2012). Forecast skill of multi-year seasonal means in the decadal prediction system of the Max Planck Institute for Meteorology. Geophys. Res. Lett. 39, L22707. doi: 10.1029/2012GL053326
Murphy, L. N., Bellomo, K., Cane, M., and Clement, A. (2017). The role of historical forcings in simulating the observed Atlantic multidecadal oscillation. Geophys. Res. Lett. 44, 2472–2480. doi: 10.1002/2016GL071337
O'Reilly, C. H., Zanna, L., and Woollings, T. (2019). Assessing external and internal sources of Atlantic multidecadal variability using models, proxy data, and early instrumental indices. J. Clim. 32, 7727–7745. doi: 10.1175/JCLI-D-19-0177.1
Ossó, A., Sutton, R., Shaffrey, L., and Dong, B. (2020). Development, amplification, and decay of Atlantic/European summer weather patterns linked to spring North Atlantic sea surface temperatures. J. Clim. 33, 5939–5951. doi: 10.1175/JCLI-D-19-0613.1
Otterå, O. H., Bentsen, M., Drange, H., and Suo, L. (2010). External forcing as a metronome for Atlantic multidecadal variability. Nat. Geosci. 3, 688–694. doi: 10.1038/ngeo955
Oudar, T., Kushner, P. J., Fyfe, J. C., and Sigmond, M. (2018). No impact of anthropogenic aerosols on early 21st century global temperature trends in a large initial-condition ensemble. Geophys. Res. Lett. 45, 9245–9252. doi: 10.1029/2018GL078841
Pausata, F. S., and Camargo, S. J. (2019). Tropical cyclone activity affected by volcanically induced ITCZ shifts. Proc. Natl. Acad. Sci. U. S. A. 116, 7732–7737. doi: 10.1073/pnas.1900777116
Peings, Y., Simpkins, G., and & Magnusdottir, G. (2016). Multidecadal fluctuations of the North Atlantic Ocean and feedback on the winter climate in CMIP5 control simulations. J. Geophys. Res. Atmos. 121, 2571–2592. doi: 10.1002/2015JD024107
Perlwitz, J., Pawson, S., Fogt, R. L., Nielsen, J. E., and Neff, W. D. (2008). Impact of stratospheric ozone hole recovery on Antarctic climate. Geophys. Res. Lett. 35, L08714. doi: 10.1029/2008GL033317
Polson, D., Bollasina, M., Hegerl, G. C., and Wilcox, L. J. (2014). Decreased monsoon precipitation in the Northern Hemisphere due to anthropogenic aerosols. Geophys. Res. Lett. 41, 6023–6029. doi: 10.1002/2014GL060811
Polvani, L. M., Waugh, D. W., Correa, G. J. P., and Son, S.-W. (2011). Stratospheric ozone depletion: the main driver of twentieth-century atmospheric circulation changes in the Southern Hemisphere. J. Clim. 24, 795–812. doi: 10.1175/2010JCLI3772.1
Pongratz, J., Schwingshackl, C., Bultan, S., Obermeier, W., Havermann, F., and Guo, S. (2021). Land use effects on climate: current state, recent Progress, and emerging topics. Curr. Clim. Change Rep. 7, 99–120. doi: 10.1007/s40641-021-00178-y
Power, S., Lengaigne, M., Capotondi, A., Khodri, M., Vialard, J., Jebri, B., et al. (2021). Decadal climate variability in the tropical Pacific: characteristics, causes, predictability, and prospects. Science 374, eaay9165. doi: 10.1126/science.aay9165
Qasmi, S., Cassou, C., and Boé, J. (2017). Teleconnection between Atlantic multidecadal variability and European temperature: Diversity and evaluation of the coupled model intercomparison project phase 5 models. Geophys. Res. Lett. 44, 11–140. doi: 10.1002/2017GL074886
Robock, A. (2000). Volcanic eruptions and climate. Rev. Geophys. 38, 191–219. doi: 10.1029/1998RG000054
Rotstayn, L. D., Collier, M. A., Jeffrey, S. J., Kidston, J., Syktus, J. I., Wong, K. K., et al. (2013). Anthropogenic effects on the subtropical jet in the Southern Hemisphere: aerosols versus long-lived greenhouse gases. Environ. Res. Lett. 8, 014030. doi: 10.1088/1748-9326/8/1/014030
Rye, C. D., Marhsall, J., Kelley, M., Russell, G., Nazarenko, L. S., Kostov, Y., et al. (2020). Antarctic glacial melt as a driver of recent Southern Ocean climate trends. Geophys.Res. Lett. 47, e2019GL.086892. doi: 10.1029/2019GL086892
Scaife, A. A., Arribas, A., Blockley, E., Brookshaw, A., Clark, R. T., Dunstone, N., et al. (2014). Skillful long-range prediction of European and north American winters. Geophys. Res. Lett. 41, 2514–2519. doi: 10.1002/2014GL059637
Scaife, A. A., Camp, J., Comer, R., Davis, P., Dunstone, N., Gordon, M., et al. (2019). Does increased atmospheric resolution improve seasonal climate predictions? Atmos. Sci. Lett. 20, e922. doi: 10.1002/asl.922
Scaife, A. A., Folland, C. K., Alexander, L., Moberg, A., and Knight, J. R. (2008). European climate extremes and the North Atlantic Oscillation. J. Clim. 21, 72–83. doi: 10.1175/2007JCLI1631.1
Scaife A. A. Ineson S. Knight J. R. Gray L. Kodera K. and D. M. Smith A. (2013). Mechanism for lagged North Atlantic climate response to solar variability. Geophys. Res. Lett. 40, 434–439. doi: 10.1002/grl.50099
Scaife, A. A., and Smith, D. (2018). A signal-to-noise paradox in climate science. NPJ Clim. Atmos. Sci. 1, 28. doi: 10.1038/s41612-018-0038-4
Schubert, S. D., Suarez, M., Pegion, P. J., Koster, R. D., and Bacmeister, J. T. (2004). On the cause of the 1930s dustbowl. Science 33, 1855–1859. doi: 10.1126/science.1095048
Schurer, A. P., Tett, S. F., and Hegerl, G. C. (2014). Small influence of solar variability on climate over the past millennium. Nat. Geosci. 7, 104–108. doi: 10.1038/ngeo2040
Sévellec, F., and Drijfhout, S. S. (2019). The signal-to-noise paradox for interannual surface atmospheric temperature predictions. Geophys. Res. Lett. 46, 9031–9041. doi: 10.1029/2019GL083855
Sheen, K. L., Smith, D. M., Dunstone, N. J., Eade, R., Rowell, D. P., and Vellinga, M. (2017). Skilful prediction of Sahel summer rainfall on inter-annual and multi-year timescales. Nat. Comms. 8, 14966. doi: 10.1038/ncomms14966
Shindell, D. T., Schmidt, G. A., Mann, M. E., and Faluvegi, G. (2004). Dynamic winter climate response to large tropical volcanic eruptions since 1600. J. Geophys. Res. 109, D05104. doi: 10.1029/2003JD004151
Shindell, D. T., Schmidt, G. A., Mann, M. E., Rind, D., and Waple, A. (2001). Solar forcing of regional climate change during the maunder minimum. Science 294, 2149–2152. doi: 10.1126/science.1064363
Shiogama, H., Stone, D. A., Nagashima, T., Nozawa, T., and Emori, S. (2013). On the linear additivity of climate forcing-response relationships at global and continental scales. Int. J. Climatol. 33, 2542–2550. doi: 10.1002/joc.3607
Simpson, I. R., Deser, C., McKinnon, K. A., and Barnes, E. A. (2018). Modeled and observed multidecadal variability in the North Atlantic jet stream and its connection to sea surface temperatures. J. Clim. 7, 703–708.doi: 10.1175/JCLI-D-18-0168.1
Simpson, I. R., Yeager, S. G., McKinnon, K. A., and Deser, C. (2019). Decadal predictability of late winter precipitation in western Europe through an ocean–jet stream connection. Nat. Geosci. 12, 613–619. doi: 10.1038/s41561-019-0391-x
Sippel, S., Meinshausen, N., Merrifield, A., Lehner, F., Pendergrass, A. G., Fischer, E., et al. (2019). Uncovering the forced climate response from a single ensemble member using statistical learning. J. Clim. 32, 5677–5699. doi: 10.1175/JCLI-D-18-0882.1
Smith, D. M., Booth, B. B. B., Dunstone, N. J., Eade, R., Hermanson, L., Jones, G. S., et al. (2016). Role of volcanic and anthropogenic aerosols in recent slowdown in global surface warming. Nat. Clim. Change 6, 936–940. doi: 10.1038/nclimate3058
Smith, D. M., Eade, R., Andrews, M. B., Ayres, H., Clark, A., Chripko, S., et al. (2022). Robust but weak winter atmospheric circulation response to future Arctic sea ice loss. Nat. Commun. 13, 727. doi: 10.1038/s41467-022-28283-y
Smith, D. M., Eade, R., Dunstone, N. J., Fereday, D., Murphy, J. M., Pohlmann, H., et al. (2010). Skilful multi-year predictions of Atlantic hurricane frequency. Nat. Geosci. 3, 846–849. doi: 10.1038/ngeo1004
Smith, D. M., Eade, R., Scaife, A. A., Caron, L. P., Danabasoglu, G. T. M., DelSole, T., et al. (2019a). Robust skill of decadal climate predictions. NPJ Clim. Atmos. Sci. 2, 13. doi: 10.1038/s41612-019-0071-y
Smith, D. M., Scaife, A. A., Eade, R., Athanasiadis, P., Bellucci, A., Bethke, I., et al. (2020). North Atlantic climate far more predictable than models imply. Nature 583, 796–800. doi: 10.1038/s41586-020-2525-0
Smith, D. M., Screen, J. A., Deser, C., Cohen, J., Fyfe, J. C., García-Serrano, J., et al. (2019b). The Polar Amplification Model Intercomparison Project (PAMIP) contribution to CMIP6: investigating the causes and consequences of polar amplification. Geosci. Model Dev. Discuss. 12, 1139–1164. doi: 10.5194/gmd-12-1139-2019
Snyder, P. K. (2010). The influence of tropical deforestation on the Northern Hemisphere climate by atmospheric teleconnections. Earth Int. 14, 1–34. doi: 10.1175/2010EI280.1
Son, S.-W., Gerber, E. P., Perlwitz, J., Polvani, L. M., Gillett, N. P., Seo, K.-H., et al. (2010). Impact of stratospheric ozone on Southern Hemisphere circulation change: a multimodel assessment. J. Geophys. Res. 115, D00M.07. doi: 10.1029/2010JD014271
Spiegl, T., and Langematz, U. (2020). Twenty-first-century climate hot spots in the light of a weakening sun. J. Clim. 33, 3431–3447. doi: 10.1175/JCLI-D-19-0059.1
Stenchikov, G., Delworth, T. L., Ramaswamy, V., Stouffer, R. J., Wittenberg, A., Zeng, F., et al. (2009). Volcanic signals in oceans. J. Geophys. Res. 114, D16104. doi: 10.1029/2008JD011673
Stenchikov, G., Hamilton, K., Stouffer, R. J., Robock, A., Ramaswamy, V., Santer, B., et al. (2006). Arctic Oscillation response to volcanic eruptions in the IPCC AR4 climate models. J. Geophys. Res. 111, D07107. doi: 10.1029/2005JD006286
Stott, P. A., Jones, G. S., and Mitchell, J. F. (2003). Do models underestimate the solar contribution to recent climate change? J. Clim. 16, 4079–4093. doi: 10.1175/1520-0442(2003)016%3C4079:DMUTSC%3E2.0.CO;2
Strong, J. D., Vecchi, G. A., and Ginoux, P. (2018). The climatological effect of Saharan dust on global tropical cyclones in a fully coupled GCM. J. Geophys. Res. Atmos. 123, 5538–5559. doi: 10.1029/2017JD027808
Swingedouw, D., Mignot, J., Ortega, P., Khodri, M., Menegoz, M., Cassou, C., et al. (2017). Impact of explosive volcanic eruptions on the main climate variability modes. Global Planet. Change 150, 24–45. doi: 10.1016/j.gloplacha.2017.01.006
Swingedouw, D., Ortega, P., Mignot, J., Guilyardi, E., Masson-Delmotte, V., Butler, P. G., et al. (2015). Bidecadal North Atlantic ocean circulation variability controlled by timing of volcanic eruptions. Nat. Commun. 6, 6545. doi: 10.1038/ncomms7545
Swingedouw, D., Terray, L., Cassou, C., Voldoire, A., Salas-Melia, D., and Servonnat, J. (2011). Natural forcing of climate during the last millennium: fingerprint of solar variability. Clim. Dyn. 36, 1349–1364. doi: 10.1007/s00382-010-0803-5
Takahashi, C., and Watanabe, M. (2016). Pacific trade winds accelerated by aerosol forcing over the past two decades. Nat. Clim. Change 6, 768–772. doi: 10.1038/nclimate2996
Tebaldi, C., and Friedlingstein, P. (2013). Delayed detection of climate mitigation benefits due to climate inertia and variability. Proc. Natl. Acad. Sci. 110, 17229–17234. doi: 10.1073/pnas.1300005110
Tejedor, E., Steiger, N. J., Smerdon, J. E., Serrano-Notivoli, R., and & Vuille, M. (2021). Global hydroclimatic response to tropical volcanic eruptions over the last millennium. Natl. Acad. Sci. U. S. A. 118, e2019145118. doi: 10.1073/pnas.2019145118
Teng, H., Branstator, G., Tawfik, A. B., and Callaghan, P. (2019). Circumglobal response to prescribed soil moisture over North America. J. Clim. 32, 4525–4545. doi: 10.1175/JCLI-D-18-0823.1
Teng, H., Leung, R., Branstator, G., Lu, J., and Ding, Q. (2022). Warming pattern over the northern hemisphere midlatitudes in boreal summer. J. Clim. 35, 3479–3494. doi: 10.1175/JCLI-D-21-0437.1
Thiéblemont, R., Matthes, K., Omrani, N. E., Kodera, K., and Hansen, F. (2015). Solar forcing synchronizes decadal North Atlantic climate variability. Nat. Commun. 6, 8268. doi: 10.1038/ncomms9268
Thompson, D. W. J., and Solomon, S. (2002). Interpretation of recent Southern Hemisphere climate change. Science 296, 895–899. doi: 10.1126/science.1069270
Thompson, D. W. J., Solomon, S., Kushner, P. J., England, M. H., Grise, K. M., and Karoly, D. J. (2011). Signatures of the Antarctic ozone hole in Southern Hemisphere surface climate change. Nat. Geosci. 4, 741–749. doi: 10.1038/ngeo1296
Timmreck, C., Pohlmann, H., Illing, S., and Kadow, C. (2016). The impact of stratospheric volcanic aerosol on decadal-scale climate predictions. Geophys. Res. Lett. 43, 834–842. doi: 10.1002/2015GL067431
Trenberth, K. E., and Shea, D. J. (2006). Atlantic hurricanes and natural variability in 2005. Geophys. Res. Lett. 33, L12704. doi: 10.1029/2006GL026894
van Oldenborgh, G. J., Wehner, M. F., Vautard, R., Otto, F. E. L., Seneviratne, S. I., Stott, P. A., et al. (2022). Attributing and projecting heatwaves is hard: we can do better. Earth Future. 10, e2021EF002271. doi: 10.1029/2021EF002271
Wang, C., Dong, S., Evan, A. T., Foltz, G. R., and Lee, S.-K. (2012). Multidecadal covariability of North Atlantic sea surface temperature, African dust, Sahel rainfall, and Atlantic hurricanes. J. Clim. 25, 5404–5415. doi: 10.1175/JCLI-D-11-00413.1
Wang, H., Schubert, S., Koster, R., and Chang, Y. (2019). Phase-locking of the boreal summer atmospheric response to dry land surface anomalies in the Northern Hemisphere. J. Clim. 32, 1081–1099. doi: 10.1175/JCLI-D-18-0240.1
Wang, H., Xie, S., Zheng, X., Kosaka, Y., Xu, Y., Geng, Y., et al. (2020). Dynamics of Southern Hemisphere atmospheric circulation response to anthropogenic aerosol forcing. Geophys. Res. Lett. 47, e2020GL.089919. doi: 10.1029/2020GL089919
Wang, J., Yang, B., Ljungqvist, F. C., Luterbacher, J., Osborn, T. J., Briffa, K. R., et al. (2017). Internal and external forcing of multidecadal Atlanticclimate variability over the past 1,200 years. Nat. Geosci. 10, 512–517. doi: 10.1038/ngeo2962
Wang, T., Otterå, O. H., Gao, Y., and Wang, H. (2012). The response of the North Pacific Decadal Variability to strong tropical volcanic eruptions. Clim. Dyn. 39, 2917–2936. doi: 10.1007/s00382-012-1373-5
Wang, X., Li, J., Sun, C., and Liu, T. (2017). NAO and its relationsheip with the Northern Hemisphere mean surface temperature in CMIP5 simulations. J. Geophys. Res. Atmos. 122, 4202–4227. doi: 10.1002/2016JD025979
Wang, Y., Le, T., Chen, G., Yung, Y. L., Su, H., Seinfeld, J. H., et al. (2020). Reduced European aerosol emissions suppress winter extremes over northern Eurasia. Nat. Clim. Chang. 10, 225–230. doi: 10.1038/s41558-020-0693-4
Wang, Z., Lin, L., Xu, Y., Che, H., Zhang, X., Zhang, H., et al. (2021). Incorrect Asian aerosols affecting the attribution and projection of regional climate change in CMIP6 models. NPJ Clim. Atmos. Sci. 4, 2. doi: 10.1038/s41612-020-00159-2
Watanabe, M., and Tatebe, H. (2019). Reconciling roles of sulphate aerosol forcing and internal variability in Atlantic multidecadal climate changes. Clim. Dyn. 53, 4651–4665. doi: 10.1007/s00382-019-04811-3
Weisheimer, A., Decremer, D., MacLeod, D., O'Reilly, C., Stockdale, T. N., Johnson, S., et al. (2019). How confident are predictability estimates of the winter North Atlantic Oscillation? Q. J. Royal Meteorol. Soc. 145, 140–159. doi: 10.1002/qj.3446
Wilcox, L. J., Dunstone, N., Lewinschal, A., Bollasina, M., Ekman, A. M. L., Highwood, E. J., et al. (2019). Mechanisms for a remote response to Asian anthropogenic aerosol in boreal winter. Atmos. Chem. Phys. 19, 9081–9095. doi: 10.5194/acp-19-9081-2019
Wills, R. C. J., Battisti, D. S., Armour, K. C., Schneider, T., and Deser, C. (2020). Pattern recognition methods to separate forced responses from internal variability in climate model ensembles and observations. J. Clim. 33, 8693–8719. doi: 10.1175/JCLI-D-19-0855.1
Wright, N. M., Krause, C. E., Phipps, S. J., Boschat, G., and Abram, N. J. (2022). Influence of long-term changes in solar irradiance forcing on the Southern Annular Mode. Clim. Past 18, 1509–1528.doi: 10.5194/cp-2021-156
Wu, M., Zhou, T., Li, C., Li, H., Chen, X., Wu, B., et al. (2021). A very likely weakening of Pacific Walker Circulation in constrained near-future projections. Nat. Commun. 12, 1–8. doi: 10.1038/s41467-021-26693-y
Yeager, S. G., Danabasoglu, G., Rosenblum, N. A., Strand, W., Bates, S. C., Meehl, G. A., et al. (2018). Predicting near-term changes in the earth system: a large ensemble of initialized decadal prediction simulations using the community earth system model. Bull. Am. Meteorol. Soc. 99, 1867–1886. doi: 10.1175/BAMS-D-17-0098.1
Yeager S. G. and Robson, J. I. . (2017). Recent progress in understanding and predicting Atlantic decadal climate variability. Curr. Clim. Change Rep. 3, 112–127. doi: 10.1007/s40641-017-0064-z
Zanchettin, D., Bothe, O., Graf, H. F., Lorenz, S. J., Luterbacher, J., Timmreck, C., et al. (2013). Background conditions influence the decadal climate response to strong volcanic eruptions. J. Geophys. Res. 118, 4090–4106. doi: 10.1002/jgrd.50229
Zhang, L., Delworth, T. L., Cooke, W., and Yang, X. (2019). Natural variability of Southern Ocean convection as a driver of observed climate trends. Nat. Clim. Change 9, 59–65. doi: 10.1038/s41558-018-0350-3
Zhang, R., Sutton, R., Danabasoglu, G., Kwon, Y., Marsh, R., Yeager, S. G., et al. (2019). A review of the role of the atlantic meridional overturning circulation in atlantic multidecadal variability and associated climate impacts. Rev. Geophys. 57, 316–375. doi: 10.1029/2019RG000644
Zhang W. and Kirtman, B.. (2019). Understanding the signal-to-noise paradox with a simple Markov model. Geophys. Res. Lett. 46, 13308–13317. doi: 10.1029/2019GL085159
Zhang, W., Kirtman, B., Siqueira, L., Clement, A., and Xia, J. (2021). Understanding the signal-to-noise paradox in decadal climate predictability from CMIP5 and an eddying global coupled model. Clim. Dyn. 56, 2895–2913. doi: 10.1007/s00382-020-05621-8
Zhou, T., Zhang, W., Zhang, L., Zhang, X., Qian, Y., Peng, D., et al. (2020). The dynamic and thermodynamic processes dominating the reduction of global land monsoon precipitation driven by anthropogenic aerosols emission. Sci. China Earth Sci. 63, 919–933. doi: 10.1007/s11430-019-9613-9
Zuo, M., Man, W. M., Zhou, T. J., and Guo, Z. (2018). Different impacts of northern, tropical, and southern volcanic eruptions on the tropical pacific SST in the last millennium. J. Clim. 31, 6729–6744. doi: 10.1175/JCLI-D-17-0571.1
Zuo, M., Zhou, T., and Man, W. (2019a). Hydroclimate responses over global monsoon regions following volcanic eruptions at different latitudes. J. Clim. 32, 4367–4385. doi: 10.1175/JCLI-D-18-0707.1
Keywords: decadal climate, attribution, external forcings, large ensembles, model intercomparison, Lighthouse Activity
Citation: Smith DM, Gillett NP, Simpson IR, Athanasiadis PJ, Baehr J, Bethke I, Bilge TA, Bonnet R, Boucher O, Findell KL, Gastineau G, Gualdi S, Hermanson L, Leung LR, Mignot J, Müller WA, Osprey S, Otterå OH, Persad GG, Scaife AA, Schmidt GA, Shiogama H, Sutton RT, Swingedouw D, Yang S, Zhou T and Ziehn T (2022) Attribution of multi-annual to decadal changes in the climate system: The Large Ensemble Single Forcing Model Intercomparison Project (LESFMIP). Front. Clim. 4:955414. doi: 10.3389/fclim.2022.955414
Received: 28 May 2022; Accepted: 26 July 2022;
Published: 16 September 2022.
Edited by:
Gaby Sophie Langendijk, Climate Service Center Germany (GERICS), GermanyReviewed by:
Kevin Grise, University of Virginia, United StatesCopyright © 2022 Smith, Gillett, Simpson, Athanasiadis, Baehr, Bethke, Bilge, Bonnet, Boucher, Findell, Gastineau, Gualdi, Hermanson, Leung, Mignot, Müller, Osprey, Otterå, Persad, Scaife, Schmidt, Shiogama, Sutton, Swingedouw, Yang, Zhou and Ziehn. This is an open-access article distributed under the terms of the Creative Commons Attribution License (CC BY). The use, distribution or reproduction in other forums is permitted, provided the original author(s) and the copyright owner(s) are credited and that the original publication in this journal is cited, in accordance with accepted academic practice. No use, distribution or reproduction is permitted which does not comply with these terms.
*Correspondence: Doug M. Smith, ZG91Zy5zbWl0aEBtZXRvZmZpY2UuZ292LnVr
Disclaimer: All claims expressed in this article are solely those of the authors and do not necessarily represent those of their affiliated organizations, or those of the publisher, the editors and the reviewers. Any product that may be evaluated in this article or claim that may be made by its manufacturer is not guaranteed or endorsed by the publisher.
Research integrity at Frontiers
Learn more about the work of our research integrity team to safeguard the quality of each article we publish.