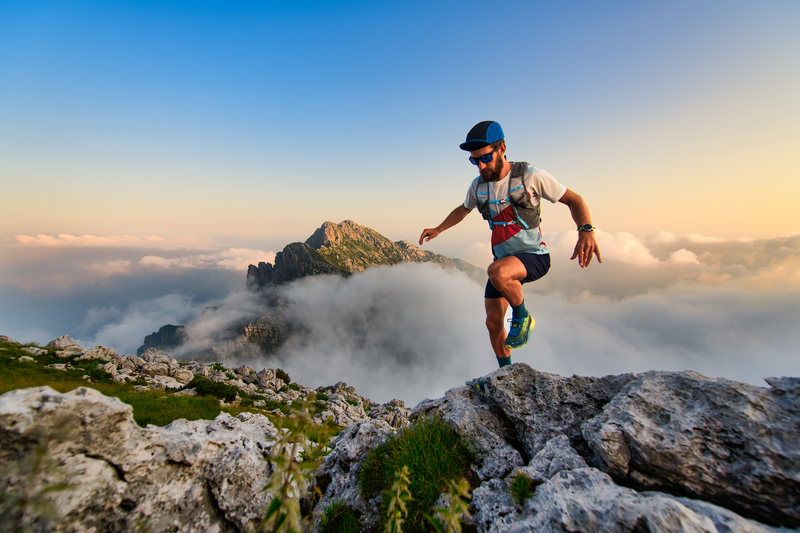
95% of researchers rate our articles as excellent or good
Learn more about the work of our research integrity team to safeguard the quality of each article we publish.
Find out more
ORIGINAL RESEARCH article
Front. Chem. , 31 October 2024
Sec. Theoretical and Computational Chemistry
Volume 12 - 2024 | https://doi.org/10.3389/fchem.2024.1480468
As ionic liquids (ILs) continue to be prepared, there is a growing need to develop theoretical methods for predicting the properties of ILs, such as gas solubility. In this work, different strategies were employed to obtain the solubility of CO2 and N2, where a conductor-like screening model for real solvents (COSMO-RS) was used as the basis. First, experimental data on the solubility of CO2 and N2 in ILs were collected. Then, the solubility of CO2 and N2 in ILs was predicted using COSMO-RS based on the structures of cations, anions, and gases. To further improve the performance of COSMO-RS, two options were used, i.e., the polynomial expression to correct the COSMO-RS results and the combination of COSMO-RS and machine learning algorithms (eXtreme Gradient Boosting, XGBoost) to develop a hybrid model. The results show that the COSMO-RS with correction can significantly improve the prediction of CO2 solubility, and the corresponding average absolute relative deviation (AARD) is decreased from 43.4% to 11.9%. In contrast, such an option cannot improve that of the N2 dataset. Instead, the results obtained from coupling machine learning algorithms with the COSMO-RS model agree well with the experimental results, with an AARD of 0.94% for the solubility of CO2 and an average absolute deviation (AAD) of 0.15% for the solubility of N2.
Since the dawn of the industrial revolution, the rising consumption of fossil fuels has caused a significant increase in atmospheric carbon dioxide (CO2) levels. The worldwide atmospheric CO2 levels have increased from an average of 280 parts per million (ppm) in the late 18th century to 414 ppm by the year 2021 (Cheng et al., 2022). As a consequence, this rise has triggered numerous environmental challenges, such as global warming and the acidification of oceans. Mitigating CO2 emissions is thus crucial. Meanwhile, CO2 serves as an inexpensive, non-toxic, and abundant C1-feedstock, and it can be converted into alcohols, ethers, acids, and various other value-added chemicals. Therefore, CO2 capture and utilization via conversion is one of the effective strategies to mitigate CO2 emission and produce carbon-based chemicals.
Among different CO2 conversion methods, the electrochemical CO2 reduction reaction (eCO2RR) stands out as an appealing strategy to convert renewable electricity, together with CO2, into fuels and feedstocks in the form of chemical bonds (Vasileff et al., 2018). Notably, the electrochemical synthesis of compounds with C-N bonds, such as urea, amide, and amino acids, from CO2 and N2 as well as their derivatives is gaining recognition as a viable and sustainable approach (Chen et al., 2020a; Jouny et al., 2019). Additionally, nitrogen (N2), comprising 78% of the atmospheric air, is a highly appealing source of nitrogen. Consequently, the electrocatalytic N2 reduction reaction (NRR) for ammonia production has attracted substantial attention for its advantages in energy conservation and environmental sustainability. Despite considerable progress, the low solubility of CO2 and N2 in water and conventional electrolyte solutions leads to low efficiency of the aforementioned reactions, thus hindering its development and application (Chen et al., 2021; Ren et al., 2021). Hence, for both eCO2RR with C-N coupling and NRR mentioned above, enhancing the solubility of CO2 and N2 is a vital prerequisite for the subsequent conversion reaction.
For eCO2RR and NRR, the gas solubility can be adjusted by developing novel electrolytes. Ionic liquids (ILs) are a type of organic salt that remains liquid at or near room temperature and consists of cations and anions. As a kind of green medium, they possess many outstanding characteristics, such as flexible tunability, high ionic conductivity, and wide electrochemical window. ILs have been extensively studied and shown great potential in many fields, such as electrocatalytic conversion, over the past decade. For example, the work by Chen et al. demonstrated that the faradaic efficiency (FE) and current density in 0.5 M [Bmim] PF6/MeCN for CO2 electrochemical reduction to CO are much higher than those in 0.5 M KHCO3, and the high CO2 solubility of [Bmim][PF6]/MeCN is one of the reasons (Chen et al., 2020b). Zhou et al. studied the electrochemical ammonia synthesis at ambient conditions and achieved a FE of NRR higher than 60%. A key factor in this high efficiency was the relatively elevated N2 solubility in the IL electrolyte (Zhou et al., 2017). Therefore, using IL as electrolytes can be an effective strategy to enhance the gas solubility and thus improve the performance of eCO2RR and NRR.
ILs can be theoretically composed of any combination of cations and anions, making ILs highly desirable but also time-consuming and expensive to measure their properties experimentally. Therefore, a fast and reliable predictive method is needed to screen out the suitable ILs for specific tasks, such as finding ILs with high gas solubility for electrocatalytic conversion of CO2 and N2. Several models have been developed and applied to predict the solubility of gases in the systems containing ILs. Molecular dynamics (MD) simulations, frequently combined with density functional theory (DFT), provide valuable microscopic insights and serve as a robust complement to experimental results (Zhao et al., 2024). While these computational techniques have significantly enhanced our understanding of ILs properties, their limitations such as complex model architectures and extended computational times have constrained the efficiency and broader application of these methods in IL research. In addition, the activity coefficient models, such as UNIFAC (Chen et al., 2020c), UNIQUAC, (Kamgar and Rahimpour, 2016) etc., usually show good capabilities in predicting the solubility of gases in ILs. However, these models require parameters of each functional group and the binary interaction coefficients among them, and their application is limited to a certain extent. The methods based on quantum chemistry (QM) overcome the limitations of the aforementioned techniques by obtaining missing molecular properties through ab initio calculations, being independent of experimental data. Furthermore, some QM-based methods have already been applied to Computer-Aided Molecular Design (CAMD) methods, such as those based on the Conductor-like Screening Model (COSMO), including the Conductor-like Screening Model for Realistic Solvents (COMSO-RS) proposed by Klamt (1995) and the COSMO segment activity coefficient (COMSO-SAC). Ali et al. employed COSMO-RS to predict the solubility of CO2 in eight different ILs. The predictions were then compared to experimental data, showing similar trends and a moderate level of agreement, with deviations ranging from 8% to 62% (Hadj-Kali et al., 2020). The CO2 absorption capacity of 1,2,4-triazolium-based ILs and the imidazolium-based ILs with different anions was predicted with COSMO-RS, and the triazolium-based ILs exhibited higher values (Mohammed et al., 2023). It was also found that the HOMO energy level of the anion plays a more prominent role in solubility compared to the LUMO energy level of the cation, which can be explained by the greater tendency of CO2 to accept electrons more rather than donate them. Manan et al. verified the predictive accuracy of COSMO-RS by investigating the solubility of 15 gases, including CO2 and N2, in 27 different ILs. The study demonstrated that, while COSMO-RS can qualitatively predict solubility, its accuracy needs to be further improved for reliable quantitative predictions. For example, the absolute relative deviations (ARD) of the CO2 solubility in [Bmim][BF4] is as high as 32.4% and that for N2 is 57.8% (Manan et al., 2009). A common method to improve the prediction performance of COSMO-RS is to employ experimental data to correct the model predictions. For instance, Zhao et al. (2017), Liu et al. (2021), Wang et al. (2021), and Farahipour et al. (2016) used a linear expression to correct the Henry’s law constants obtained from COSMO-RS. However, no work has been done so far to study a wide range of ILs, and the work is on the CO2 solubility but not on the N2 solubility.
In recent years, benefiting from the rapid development of machine learning algorithms, quantitative structure-property relationship (QSPR) models have been extensively applied to predict the properties of ILs, such as density, viscosity, activity coefficient, gas solubility, and so on. Song et al. (2020) employed the artificial neural network (ANN) and support vector machine (SVM) algorithms to construct predictive models based on group contribution (GC) methods, effectively predicting the solubility of CO2 in various ILs using 10,116 datasets across different temperatures and pressures. The ANN-GC model has an estimated mean absolute error (MAE) of 0.0202 and a coefficient of determination (R2) of 0.9836, while the SVM-GC model shows a MAE of 0.0240 and a R2 of 0.9783. Tian et al. integrated ANN and SVM with the ionic fragments contribution (IFC) to predict the solubility of CO2 and N2 in ILs. In their work, 13,055 datasets of CO2 solubility and 415 datasets of N2 solubility were collected for model training and validation. As a result, the R2 values obtained for the CO2 solubility predictions are 0.9855 for IFC-SVM and 0.9732 for IFC-ANN in the training sets. Similarly, the R2 values for the N2 solubility predictions are 0.9966 and 0.9909 for IFC-SVM and IFC-ANN, respectively (Tian et al., 2023). Recently, Tian et al. established two models based on both the random forest (RF) and gradient boosting regressor (GBR) to predict the N2 solubility in ILs. The input features of the model include temperature, pressure, and COSMO-derived descriptors. After training the model with four of five folders, R2 and AARD were obtained with values of 0.9986% and 14.24% for RF-IFC and 0.9999% and 5.28% for GBR-IFC, respectively (Tian et al., 2024). Ali and co-workers developed two deep learning models, namely, ANN and long short-term memory (LSTM), to predict CO2 solubility in ILs using a dataset of 10,116 data points across 164 kinds of ILs under various temperature and pressure conditions. Both models demonstrated strong predictive performance, with R2 values of 0.986 and 0.985 for ANN and LSTM, respectively. Moreover, the results showed that while both models provided excellent accuracy in predicting CO2 solubility, the ANN model achieved reliable accuracy with significantly lower computational time compared to the LSTM model (Ali et al., 2024). The above results confirm that the prediction models originated from the GC methods combined with the ML algorithms can be used to predict the solubility of CO2 and N2 effectively.
However, to the best of our knowledge, it was found that there are only a few research using COSMO-RS to predict the solubility of N2 in ILs, and its prediction capacity of COSMO-RS is uncertain. In addition, there is a lack of robust models to predict the gas solubilities based on COSMO-RS that already qualitatively represent the gas solubility. Hence, in this work, the solubility of CO2 and N2 in various ILs over wide ranges of temperature and pressure was extensively studied based on COSMO-RS. Firstly, a comprehensive collection of the literature data on the solubility of CO2 and N2 in ILs was conducted. Subsequently, COSMO-RS was utilized to predict the solubility of CO2 and N2 in ILs, accompanied by discussion and analysis. To further improve the performance of COSMO-RS, the modification was carried out, including two options: a correction method and a hybrid model based on the ML algorithm and GC method.
All COSMO-RS calculations were performed using the COSMOtherm software (version 19.0.4, with the BP_TZVP_19.ctd parameterization, COSMOlogic, Leverkusen, Germany). To begin with, the quantum chemical Gaussian09 package was employed to optimize the structures of the studied compounds, which include CO2, N2, and components of IL, at the B3LYP/6-31++G (d, p) level. Frequency calculations were conducted to confirm that the optimized structures correspond to true minima. Second, the resulting COSMO files of the optimized structures were subsequently imported into the COSMOtherm program to compute the solubility of CO2 and N2 in the studied ILs. For the solubility calculations of gases in ILs, the cation and anion components are treated as separate molecules with equal molar fractions (ncation = nanion = nIL), furthermore, the input variables (T, P) were set to be consistent with the experimental conditions reported in the literature.
At present, multiple ML algorithms have been used to estimate the physical and thermodynamic properties of ILs and IL-involved systems. Among them, the XGBoost algorithm proposed by Chen and Guestrin (2016) is a powerful and efficient algorithm owing to its high training efficiency, good prediction effect, multi-controllable parameters, and user-friendly features. XGBoost can be regarded as a variant of Gradient Boosting Decision Tree (GBDT). Unlike GBDT, XGBoost introduces regular terms to limit the model complexity to reduce the probability of over-fitting, and the second-order derivative information is used for optimization, which accelerates the convergence process of the model and improves the training efficiency. By assuming a dataset contains n examples and m features, the mathematic expressions (Equation 1) and objective function (Equation 2) of the XGBoost algorithm are outlined as follows:
Here, fk is the kth independent tree, and F represents the space of regression trees.
where l is a differentiable convex loss function that measures the difference between the prediction
Since the selection and number of features (i.e., the functional groups) significantly affect the accuracy and generalization ability of the ML model, the division of the functional groups followed the JR method in this work (Nannoolal et al., 2007), with the detailed information provided in Supplementary Table S1. Also, for the studied ILs, the same functional group may be contained in both cations and anions, and to better describe the impact of functional groups in anions and cations on solubility, a “-” sign was added after the functional groups from anions. Consequently, the studied ILs were divided into 41 groups for CO2 solubility modeling and 38 groups for N2 solubility modeling.
Before model development, the data used were normalized and standardized to eliminate the effects of data magnitude. First, the CO2 and N2 solubility datasets were divided into the training set and the test set, with a division ratio of 8:2. The input features for the XGBoost-GC model include temperature (T), pressure (P), and groups on cations and anions (41 for CO2 dataset, and 38 for N2 dataset). The target variable for the CO2 dataset was set to be the relative deviation (
The optimal parameters were obtained through the Bayesian optimization algorithm. Since the XGBoost algorithm is a decision tree-based model, the number of trees should be proper, and too few trees will result in poor prediction, while too many trees may lead to over-learning and over-fitting. The same goes for the maximum depth of the tree. Therefore, simultaneous optimization was performed on these parameters, where the number of trees ranged from 1 to 100 with corresponding maximum depth from 1 to 10. The ranges for the learning rate and subsample ratio were set to 0.01–0.3 and 0–1, respectively. The number of iterations was 200, and the specific parameters are listed in Supplementary Table S2.
Given that the work of Lei et al. (2014) systematically collected CO2 solubility data in ILs published before 2013 and used it as a database, the CO2 solubility data used in this work mainly come from literature reported in the past decade (Nonthanasin et al., 2014; Tagiuri et al., 2014; Gonzalez-Miquel et al., 2014; Makino et al., 2014a; Bahadur et al., 2015; Carvalho et al., 2014; Makino et al., 2014b; Zhou et al., 2014; Zeng et al., 2015; Almantariotis et al., 2017; Liu et al., 2016; Zhou et al., 2016; Zoubeik et al., 2016; Nematpour et al., 2016; Watanabe et al., 2016; Zubeir et al., 2016a; Zubeir et al., 2016b; Dai et al., 2017; Jalili et al., 2017; Bai et al., 2017; Mirzaei et al., 2018; Zhao et al., 2018; Jalili et al., 2019; Nath and Henni, 2020; Safarov et al., 2019; Wang et al., 2022; Henni et al., 2023; Kodama et al., 2023; Mirzaei et al., 2023; Suzuki et al., 2024a; Suzuki et al., 2024b), and the experimental data with zero or negative solubility are not considered. Finally, 3,036 sets of CO2 solubility (mole fraction: 0.00116–0.713) in 72 different ILs were selected at temperatures of 273.15–413.15 K and pressures of 9.7–6,532.8 kPa.
However, for N2, the relevant experimental data are much less abundant than for CO2. Here, we collected and screened N2 solubility data in the previous literature (Zhou et al., 2014; Almantariotis et al., 2017; Liu et al., 2016; Zhou et al., 2016; Jacquemin et al., 2006a; Jacquemin et al., 2006b; Zhou et al., 2013; Almantariotis et al., 2012; Stevanovic and Gomes, 2013; Zhao et al., 2011; Anderson et al., 2007; Yuan et al., 2006; Afzal et al., 2015; Zhang et al., 2017; Bentley et al., 2023). Similarly, the datasets with zero or negative solubility were discarded. A total of 457 N2 solubility data points in 31 types of ILs were collected, with values ranging from 0.000171 to 0.6187 mol fraction at 283.20–353.20 K and 4.69–14982 kPa. Supplementary Tables S3, S4 provided the detailed experimental ranges of temperature, pressure, and solubility for various CO2-IL and N2-IL systems.
Supplementary Figures S1, S2 show the temperature, pressure, and solubility distributions. It could be seen that the temperature data distribution of the two datasets is relatively uniform, while the pressure data were mainly concentrated in 0–1,000 kPa. The CO2 solubility data is relatively evenly distributed, while the N2 solubility data is mainly concentrated below 0.05.
The chemical structures of the cations and anions investigated in this work are illustrated in Supplementary Table S5. The cations include imidazolium, pyridinium, pyrrolidinium, ammonium, and phosphonium, and the anions contain acetate, sulfate, sulfonate, tetrafluoroborate [BF4], hexafluorophosphate [PF6], Bis [(trifluoromethyl)sulfonyl]azanide [NTf2], etc.
Appropriate model evaluation metrics are crucial for evaluating the accuracy of the model. To provide a reasonable evaluation, the average absolute relative deviation (AARD, Equation 3) and coefficient of determination (R2, Equation 4) were used to quantify the discrepancies between the experimental and predicted CO2 solubilities, where the former is a bias-centric metric while the latter is a variance-oriented one. However, for the N2 dataset, considering the low accuracy of experimental measurements linked to the low solubility of N2 in the solvents, the verage absolute deviation (AAD, Equation 5) and R2 were used.
where N is the total number of samples, the experimental and predicted values of gas solubility in ILs are denoted as
As described in Section 2.1, the solubility of CO2 and N2 in the identified ILs under the same conditions (T, P, ILs) as reported in the literature was predicted using COSMOthermX (version 19.0.4) and compared with the experimental values (see Supplementary Tables S6, S7). Figures 1A, B present the comparison of the experimentally determined and COSMO-RS predicted gas solubility of CO2 and N2, respectively. In Figure 1A, it is evident that the COSMO-RS model tends to underpredict the solubility of CO2 in ILs, with an AARD of 43.4% and a R2 of 0.599. For N2, as depicted in Figure 1B, the solubility data are spread on either side of the diagonal, with an AAD of 4.95% and a R2 of 0.242.
Figure 1. Comparison of experimental and COSMO-RS predicted solubility for (A) CO2 and (B) N2 in various ILs, (C) Comparison of experimentally determined and COSMO-RS predicted N2 solubility in [HMIM][eFAP] and [MDEA][Cl], (D) AAD of COSMO-RS model predictions at different temperatures.
It should be emphasized that the overall trend of the solubilities predicted by COSMO-RS is consistent with the experimental data at various temperatures and pressures (Supplementary Figures S3, S4), confirming that the qualitative prediction of COSMO-RS can be used to reliably screen ILs based on their gas solubilities. To further analyze the model predictions, the N2 solubility in two ILs was taken as an example to discuss the effects of temperature and pressure. As depicted in Figure 1C, the model prediction performance for [HMIM][eFAP] depends on the studied temperature. As the temperature increase, the points on the consistency diagram get closer to the diagonal line, i.e., the model prediction gets close to the experimental results, and thus the corresponding AAD gradually decreases. This indicates that the prediction of COSMO-RS is more accurate at relatively high temperatures. The same trend was observed for [MDEA][Cl] (Figures 1C, D). Additionally, when the temperature remains constant (e.g., T = 303.4 K), as the pressure increases, both the experimentally measured and theoretically predicted solubilities of N2 in [HMIM][eFAP] show the same increasing trend and the accuracy of COSMO-RS is gradually decreasing (Supplementary Figure S5). The results of this study demonstrate that, within a certain temperature and pressure range, COSMO-RS can accurately capture the effects of input variables (T, P) on the solubility of N2 in different cation and anion combinations.
Furthermore, the results of the COSMO-RS model developed in this study were compared with other predictive models reported in the literature. For example, Kamgar and Rahimpour (2016) used UNIQUAC and quantum models to predict the solubility of CO2 in seven ILs. The study found that UNIQUAC showed good prediction ability for the ILs studied, the ARD in most cases lower than 5%, and the maximum ARD is 9.17%. The predictions of the UNIQUAC model in the literature perform better. Additionally, the COSMO-RS model was also used to predict the CO2 solubility for the same system, showing an ARD ranging from 6.1% to 62.4%, especially, when the pressure increases, the error becomes larger. Recently, Chen and co-workers used a hierarchical extension strategy to develop a UNIFAC-IL-Gas model for gas solubility prediction. The results showed that for 13 types of gases, including CO2 and N2, its prediction performance exceeded the COSMO-RS model (Chen et al., 2020c). The above results further confirm that compared to models that require parameters obtained from the fitting of experimental data, the COSMO-RS model without requirements of any experimental information predicts results qualitatively.
As mentioned before, many studies have demonstrated that higher accuracy can be achieved by performing linear regression on the predicted values obtained by COSMO-RS. These corrected models typically use the experimental values as the target variables. However, in this work, it is evidenced that there is no simple linear relationship between T, P,
For CO2, the relative deviation (
For N2, the absolute deviation (
Here, k1-k5 are the adjustable parameters.
Based on the collected data point, the adjustable parameters were obtained, as listed in Supplementary Table S8. Figure 2 shows the comparison between the experimental gas solubilities and those predicted by the two models. It can be evident from Figure 2A that the CO2 solubility predictions from the modified model align more closely with the experimental values than those from the original COSMO-RS model. After the model modification, its AARD was decreased to 11.9% with a R2 of 0.970. For comparison, the AARD for the original COSMO-RS is 43.4%. For N2, the modified model shows only a very slight decrease in AAD, and there is no noticeable improvement in R2 compared with that before the modification. These results demonstrate that the corrected model improves the accuracy of the COSMO-RS model for predicting CO2 solubility. However, such a correction does not work for the solubility of N2 in ILs. The reasons for the above phenomenon are summarized as follows: 1) The CO2 dataset and the N2 dataset may have different quality levels. The data in the CO2 dataset is more accurate and complete and thus can be corrected for better accuracy. 2) The model assumptions themselves and the selection of features are less applicable to the N2 dataset than to the CO2 dataset. 3) The insufficient number of samples in the N2 dataset prevents the model from effectively learning the relationship between the initial predicted value and the experimental value.
Figure 2. Comparison of experimental and model-predicted solubility of (A) CO2 and (B) N2 in various ILs.
The COSMO-RS model can be used for qualitative prediction, which is sufficient for IL screening. The correction with a polynomial expression on COSMO-RS can improve the prediction capability in the solubility for certain gases (CO2, etc.) but not for all (e.g., N2). In this section, an alternative option was used to develop a hybrid model, where XGBoost-GC was coupled with COSMO-RS to achieve reliable predictions of CO2 and N2 solubility in ILs.
The comparison between experimentally determined and XGBoost-GC model-predicted CO2 solubility for both the training and test sets is depicted in Figure 3A, with the detailed data listed in Supplementary Table S9. Unlike the corrected COSMO-RS model (as seen in Figure 2A), the XGBoost-GC model demonstrates a significantly better alignment with the diagonal, indicating an improved prediction accuracy. The AARD for the entire dataset is as low as 0.94%, with a R2 of 0.9996. In comparison, the XGBoost-GC-D model, which directly uses experimental values as target variables, also shows good prediction capabilities, achieving an AARD of 3.74% and an R2 of 0.9985. This performance may be due to the meticulous division of IL groups and the optimization of the model Hyperparameter.
Figure 3. Comparison of experimental CO2 solubility in ILs with predictions from (A) the XGBoost-GC and (C) the XGBoost-GC-D models, (The inset shows the prediction errors for CO2 solubility by the XGBoost-GC model and XGBoost-GC-D model). Distribution of prediction errors for CO2 solubility as predicted by (B) the XGBoost-GC model and (D) the XGBoost-GC-D model.
For a thorough evaluation of the model predictions, the discrepancies between experimental and model-predicted CO2 solubilities are plotted against the experimental values (refer to the inset in Figure 3A). The error distribution is also displayed in Figure 3B. It is clear that the majority of the errors are closely clustered around zero, signifying a high degree of accuracy for the XGBoost-GC model, with only a small fraction of errors exceeding ± 0.03. These larger errors tend to occur when the solubility of CO2 exceeds 0.3, with the maximum absolute error being approximately −0.034. On the other hand, the error distribution for the XGBoost-GC-D model (Figure 3D) exhibits a more disordered pattern, with errors distributed across a wider range, and the maximum error is −0.049. This suggests that the XGBoost-GC-D model is less accurate compared to XGBoost-GC. Therefore, it can be concluded that the XGBoost-GC model provides more accurate predictions, making it the more reliable hybrid model for predicting CO2 solubility.
We further compared the performance of the established model with those reported in the literature. The detailed statistical results are shown in Table 1. To predict the CO2 solubility, regardless of whether the input features are group information or other descriptors, the hybrid model XGBoost-GC achieved higher prediction accuracy with less data, reflecting the superior performance of the XGBoost-GC model.
Table 1. Comparison of the models established in this work and reported in the literature for CO2 solubility prediction.
The experimentally determined and ML model-predicted N2 solubility for the both training and test sets are illustrated in Figures 4A, C, detailed data are provided in Supplementary Table S10. It can be clearly observed from Figure 4A that the majority of data points, for both the training and test sets, are closely aligned along the y = x line, indicting high accuracy in the predictions of the XGBoost-GC model. The model achieved an R2 of 0.9981 and an AAD of 0.15% across the entire dataset, demonstrating significant improvement in the predictions of the hybrid model over the original COSMO-RS model. Similarly, the XGBoost-GC-D model also exhibits good predictive performance, though slightly less accurate than the XGBoost-GC model. As shown in Figures 4A, B, the majority of the errors for the XGBoost-GC model fall within the range of ± 0.02, with the maximum absolute error being around −0.062. In contrast, Figure 4D illustrates that most of the errors for the XGBoost-GC-D model are close to zero, although a few errors exceed ± 0.03, with the maximum reaching approximately 0.036. This discrepancy could potentially be due to the limited amount of available data, highlighting the importance of conducting more experimental measurements to improve the robustness of the model.
Figure 4. Comparison of experimental N2 solubility in ILs with predictions from (A) the XGBoost-GC and (C) the XGBoost-GC-D models, (The inset shows the prediction errors for N2 solubility by the XGBoost-GC model and XGBoost-GC-D model). Distribution of prediction errors for N2 solubility as predicted by (B) the XGBoost-GC model and (D) the XGBoost-GC-D model.
Table 2 summarizes a comparison of different models, mainly including IFC-SVM, IFC-ANN, RF-IFC and GBR-IFC. The table shows that when the amount of data and the number of ILs are both similar, the R2 and AAD of the XGBoost-GC model are better than those of the SVM-IFC, ANN-GC, and RF-IFC models proposed by Tian et al., but not as good as the GBR-IFC model. This may be attributed to the fact that they introduced COSMO-derived descriptors as input variables, which contain more molecular information such as electronic distribution, molecular size, etc., making the input parameter information more comprehensive and thus achieving higher prediction accuracy.
Table 2. Comparison of the models established in this work and reported in the literature for N2 solubility prediction.
Machine learning has demonstrated significant potential in predicting various properties of ILs, particularly in fields such as green chemistry and electrochemical processes. ILs possess a variety of tunable properties, which are often time-consuming and costly to determine experimentally. ML models, trained on experimental data or theoretical predictions, offer a rapid and efficient means of predicting key properties such as viscosity, density, conductivity, and solubility. However, the performance of ML models is highly dependent on the quality and comprehensiveness of the datasets used for training, and thus the availability of high-quality data remains a critical challenge.
In addition, various thermodynamic models have shown high prediction accuracy for IL-containing systems due to their solid thermodynamic foundations. Effectively combining ML algorithms with these models to improve prediction accuracy without relying on large amounts of experimental data is crucial yet highly challenging.
The accuracy of ML models is highly depended on the selection of meaningful features, such as temperature, pressure, and structural information. The selection of features that better represent the geometric and electronic structures of ILs, along with the application of data-cleaning techniques, can further improve prediction accuracy. Additionally, future advancements may involve the implementation of more sophisticated algorithms, such as deep neural networks, which have the potential to capture complex, non-linear relationships between the structures of ILs and their corresponding properties.
Ionic liquids (ILs) are an emerging category of chemicals that have shown promise as electrolytes or co-catalysts for CO2 and N2 electrocatalytic conversion. The combination of cations and anions makes it highly designable but also presents a significant challenge in screening out suitable ILs for specific tasks. In this work, we developed different strategies based on the COSMO-RS model to accurately predict the CO2 and N2 solubility, thus aiding in the screening of the optimal ILs for the electrocatalytic conversion of CO2 and N2. We first established a database containing 3,036 solubility data for CO2 and 457 solubility data for N2 in ILs at various temperatures and pressures. The COSMO-RS model was employed to predict the solubility of CO2 and N2. The AARD between the experimental and COSMO-RS predicted solubilities of the CO2 was relatively high, i.e., 43.4% and the R2 for the CO2 and N2 datasets are 0.599 and 0.242, respectively. Polynomial regression was employed to correct the COSMO-RS predicted solubilities, resulting in a significant decrease in AARD for CO2 and a slight decrease in AAD for N2. Further performance improvements were achieved through a hybrid model that combined COSMO-RS with machine learning and group information methods. The developed hybrid model demonstrated better prediction performance, with high R2 and low AARD for the CO2 dataset and low AAD for the N2 dataset.
The datasets presented in this study can be found in online repositories. The names of the repository/repositories and accession number(s) can be found in the article/Supplementary Material.
HQ: Conceptualization, Formal Analysis, Investigation, Methodology, Writing–original draft. KW: Writing–review and editing. XM: Software, Validation, Writing–review and editing. FL: Writing–review and editing, Software. YL: Conceptualization, Resources, Supervision, Writing–review and editing. XJ: Conceptualization, Resources, Supervision, Writing–review and editing.
The author(s) declare that financial support was received for the research, authorship, and/or publication of this article. YL thanks to the support by the National Key Research and Development Program of China (No. 2022YFA1505300), the CAS Project for Young Scientists in Basic Research (No. YSBR-050), the National Natural Science Foundation of China (No. 22278402), the research fund of State Key Laboratory of Mesoscience and Engineering (MESO-23-A08). XJ thanks the financial support from Horizon-EIC, Pathfinder challenges, Grant Number: 101070976. KW thanks to the Natural Science Foundation of Henan Province (242300421141), the National Natural Science Foundation of China (No. 22208348).
The authors declare that the research was conducted in the absence of any commercial or financial relationships that could be construed as a potential conflict of interest.
All claims expressed in this article are solely those of the authors and do not necessarily represent those of their affiliated organizations, or those of the publisher, the editors and the reviewers. Any product that may be evaluated in this article, or claim that may be made by its manufacturer, is not guaranteed or endorsed by the publisher.
The Supplementary Material for this article can be found online at: https://www.frontiersin.org/articles/10.3389/fchem.2024.1480468/full#supplementary-material
Afzal, W., Liu, X., and Prausnitz, J. M. (2015). Physical data for a process to separate krypton from air by selective absorption in an ionic liquid. Fluid Phase Equilibria 404, 124–130. doi:10.1016/j.fluid.2015.06.037
Ali, M., Sarwar, T., Mubarak, N. M., Karri, R. R., Ghalib, L., Bibi, A., et al. (2024). Prediction of CO2 solubility in Ionic liquids for CO2 capture using deep learning models. Sci. Rep. 14 (1), 14730. doi:10.1038/s41598-024-65499-y
Almantariotis, D., Pensado, A., Gunaratne, H. N., Hardacre, C., Pádua, A., Coxam, J.-Y., et al. (2017). Influence of fluorination on the solubilities of carbon dioxide, ethane, and nitrogen in 1-n-Fluoro-alkyl-3-methylimidazolium bis (n-fluoroalkylsulfonyl) amide ionic liquids. J. Phys. Chem. B 121 (2), 426–436. doi:10.1021/acs.jpcb.6b10301
Almantariotis, D., Stevanovic, S., Fandino, O., Pensado, A., Padua, A. A., Coxam, J.-Y., et al. (2012). Absorption of carbon dioxide, nitrous oxide, ethane and nitrogen by 1-Alkyl-3-methylimidazolium (cnmim, n = 2,4,6) tris(pentafluoroethyl)trifluorophosphate ionic liquids (eFAP). J. Phys. Chem. B 116 (26), 7728–7738. doi:10.1021/jp304501p
Anderson, J. L., Dixon, J. K., and Brennecke, J. F. (2007). Solubility of CO2, CH4, C2H6, C2H4, O2, and N2 in 1-Hexyl-3-methylpyridinium bis (trifluoromethylsulfonyl) imide: comparison to other ionic liquids. Accounts Chem. Res. 40 (11), 1208–1216. doi:10.1021/ar7001649
Bahadur, I., Osman, K., Coquelet, C., Naidoo, P., and Ramjugernath, D. (2015). Solubilities of carbon dioxide and oxygen in the ionic liquids methyl trioctyl ammonium bis (trifluoromethylsulfonyl) imide, 1-butyl-3-methyl imidazolium bis (trifluoromethylsulfonyl) imide, and 1-butyl-3-methyl imidazolium methyl sulfate. J. Phys. Chem. B 119 (4), 1503–1514. doi:10.1021/jp5061057
Bai, L., Shang, D., Li, M., Dai, Z., Deng, L., and Zhang, X. (2017). CO2 absorption with ionic liquids at elevated temperatures. J. energy Chem. 26 (5), 1001–1006. doi:10.1016/j.jechem.2017.07.009
Bentley, C. L., Song, T., Morales-Collazo, O., and Brennecke, J. F. (2023). Solubility of nitrogen in ionic liquids at 295–353 K and pressures to 140 bar. J. Chem. and Eng. Data 68 (8), 2003–2013. doi:10.1021/acs.jced.3c00159
Carvalho, P. J., Regueira, T., Fernández, J., Lugo, L., Safarov, J., Hassel, E., et al. (2014). High pressure density and solubility for the CO2+ 1-ethyl-3-methylimidazolium ethylsulfate system. J. Supercrit. Fluids 88, 46–55. doi:10.1016/j.supflu.2014.01.011
Chen, C., He, N., and Wang, S. (2021). Electrocatalytic C–N coupling for urea synthesis. Small Sci. 1 (11), 2100070. doi:10.1002/smsc.202100070
Chen, C., Sun, X., Yan, X., Wu, Y., Liu, H., Zhu, Q., et al. (2020b). Boosting CO2 electroreduction on N, P-co-doped carbon aerogels. Angew. Chem. 132 (27), 11216–11222. doi:10.1002/ange.202004226
Chen, C., Zhu, X., Wen, X., Zhou, Y., Zhou, L., Li, H., et al. (2020a). Coupling N2 and CO2 in H2O to synthesize urea under ambient conditions. Nat. Chem. 12 (8), 717–724. doi:10.1038/s41557-020-0481-9
Chen, T., and Guestrin, C. (2016). XGBoost. Proc. 22nd ACM SIGKDD Int. Conf. Knowl. Discov. Data Min. 11, 785–794. doi:10.1145/2939672.2939785
Chen, Y., Liu, X., Woodley, J. M., and Kontogeorgis, G. M. (2020c). Gas solubility in ionic liquids: UNIFAC-IL model extension. Industrial and Eng. Chem. Res. 59 (38), 16805–16821. doi:10.1021/acs.iecr.0c02769
Cheng, W., Dan, L., Deng, X., Feng, J., Wang, Y., Peng, J., et al. (2022). Global monthly gridded atmospheric carbon dioxide concentrations under the historical and future scenarios. Sci. Data 9 (1), 83. doi:10.1038/s41597-022-01196-7
Dai, C., Lei, Z., and Chen, B. (2017). Gas solubility in long-chain imidazolium-based ionic liquids. AIChE J. 63 (6), 1792–1798. doi:10.1002/aic.15711
Farahipour, R., Mehrkesh, A., and Karunanithi, A. T. (2016). A systematic screening methodology towards exploration of ionic liquids for CO2 capture processes. Chem. Eng. Sci. 145, 126–132. doi:10.1016/j.ces.2015.12.015
Gonzalez-Miquel, M., Bedia, J., Palomar, J., and Rodriguez, F. (2014). Solubility and Diffusivity of CO2 in [hxmim] [NTf2],[omim] [NTf2], and [dcmim] [NTf2] at T=(298.15, 308.15, and 323.15) K and Pressures up to 20 bar. J. Chem. and Eng. Data 59 (2), 212–217. doi:10.1021/je4001944
Hadj-Kali, M. K., Althuluth, M., Mokraoui, S., Wazeer, I., Ali, E., and Richon, D. (2020). Screening of ionic liquids for gas separation using COSMO-RS and comparison between performances of ionic liquids and aqueous alkanolamine solutions. Chem. Eng. Commun. 207 (9), 1264–1277. doi:10.1080/00986445.2019.1680363
Henni, N., Henni, A., and Ibrahim, H. (2023). Solubility of carbon dioxide in promising methylimidazolium-based ionic liquids. Fluid Phase Equilibria 565, 113619. doi:10.1016/j.fluid.2022.113619
Jacquemin, J., Gomes, M. F. C., Husson, P., and Majer, V. (2006b). Solubility of carbon dioxide, ethane, methane, oxygen, nitrogen, hydrogen, argon, and carbon monoxide in 1-butyl-3-methylimidazolium tetrafluoroborate between temperatures 283 K and 343 K and at pressures close to atmospheric. J. Chem. Thermodyn. 38 (4), 490–502. doi:10.1016/j.jct.2005.07.002
Jacquemin, J., Husson, P., Majer, V., and Gomes, M. F. C. (2006a). Low-pressure solubilities and thermodynamics of solvation of eight gases in 1-butyl-3-methylimidazolium hexafluorophosphate. Fluid Phase Equilibria 240 (1), 87–95. doi:10.1016/j.fluid.2005.12.003
Jalili, A. H., Mehrabi, M., Zoghi, A. T., Shokouhi, M., and Taheri, S. A. (2017). Solubility of carbon dioxide and hydrogen sulfide in the ionic liquid 1-butyl-3-methylimidazolium trifluoromethanesulfonate. Fluid Phase Equilibria 453, 1–12. doi:10.1016/j.fluid.2017.09.003
Jalili, A. H., Shokouhi, M., Maurer, G., Zoghi, A. T., Ahari, J. S., and Forsat, K. (2019). Measuring and modelling the absorption and volumetric properties of CO2 and H2S in the ionic liquid 1-ethyl-3-methylimidazolium tetrafluoroborate. J. Chem. Thermodyn. 131, 544–556. doi:10.1016/j.jct.2018.12.005
Jouny, M., Lv, J.-J., Cheng, T., Ko, B. H., Zhu, J.-J., Goddard III, W. A., et al. (2019). Formation of carbon–nitrogen bonds in carbon monoxide electrolysis. Nat. Chem. 11 (9), 846–851. doi:10.1038/s41557-019-0312-z
Kamgar, A., and Rahimpour, M. R. (2016). Prediction of CO2 solubility in ionic liquids with QM and UNIQUAC models. J. Mol. Liq. 222, 195–200. doi:10.1016/j.molliq.2016.06.107
Klamt, A. (1995). Conductor-like screening model for real solvents: a new approach to the quantitative calculation of solvation phenomena. J. Phys. Chem. 99 (7), 2224–2235. doi:10.1021/j100007a062
Kodama, D., Suzuki, Y., Makino, T., Kanakubo, M., Kodama, S., Watanabe, T., et al. (2023). Density, viscosity, and CO2 solubility in phosphonium-based bis (trifluoromethanesulfonyl) amides and bis (pentafluoroethanesulfonyl) amides. Fluid Phase Equilibria 574, 113886. doi:10.1016/j.fluid.2023.113886
Lei, Z., Dai, C., and Chen, B. (2014). Gas solubility in ionic liquids. Chem. Rev. 114 (2), 1289–1326. doi:10.1021/cr300497a
Liu, T., Fan, D., Chen, Y., Dai, Y., Jiao, Y., Cui, P., et al. (2023). Prediction of 2 solubility in ionic liquids via convolutional autoencoder based on molecular structure encoding. AIChE J. 69 (10), e18182. doi:10.1002/aic.18182
Liu, X., He, M., Lv, N., Xu, H., and Bai, L. (2016). Selective absorption of CO2 from H2, O2 and N2 by 1-hexyl-3-methylimidazolium tris (pentafluoroethyl) trifluorophosphate. J. Chem. Thermodyn. 97, 48–54. doi:10.1016/j.jct.2016.01.013
Liu, Y., Dai, Z., Zhang, Z., Zeng, S., Li, F., Zhang, X., et al. (2021). Ionic liquids/deep eutectic solvents for CO2 capture: reviewing and evaluating. Green Energy and Environ. 6 (3), 314–328. doi:10.1016/j.gee.2020.11.024
Makino, T., Kanakubo, M., Masuda, Y., Umecky, T., and Suzuki, A. (2014a). CO2 absorption properties, densities, viscosities, and electrical conductivities of ethylimidazolium and 1-ethyl-3-methylimidazolium ionic liquids. Fluid Phase Equilibria 362, 300–306. doi:10.1016/j.fluid.2013.10.031
Makino, T., Kanakubo, M., and Umecky, T. (2014b). CO2 solubilities in ammonium bis (trifluoromethanesulfonyl) amide ionic liquids: effects of ester and ether groups. J. Chem. and Eng. Data 59 (5), 1435–1440. doi:10.1021/je400971q
Manan, N. A., Hardacre, C., Jacquemin, J., Rooney, D. W., and Youngs, T. G. (2009). Evaluation of gas solubility prediction in ionic liquids using COSMOthermX. J. Chem. and Eng. Data 54 (7), 2005–2022. doi:10.1021/je800857x
Mirzaei, M., Mokhtarani, B., Badiei, A., and Sharifi, A. (2018). Solubility of carbon dioxide and methane in 1-hexyl-3-methylimidazolium nitrate ionic liquid, experimental and thermodynamic modeling. J. Chem. Thermodyn. 122, 31–37. doi:10.1016/j.jct.2018.03.003
Mirzaei, M., Sharifi, A., and Abaee, M. S. (2023). Experimental study on solubility of CO2 and CH4 in the ionic liquid 1-benzyl-3-methylimidazolium nitrate. J. Supercrit. Fluids 199, 105963. doi:10.1016/j.supflu.2023.105963
Mohammed, S. A. S., Yahya, W. Z. N., Bustam, M. A., and Kibria, M. G. (2023). Experimental and computational evaluation of 1, 2, 4-triazolium-based ionic liquids for carbon dioxide capture. Separations 10 (3), 192. doi:10.3390/separations10030192
Nannoolal, Y., Rarey, J., and Ramjugernath, D. (2007). Estimation of pure component properties. Fluid Phase Equilibria 252 (1-2), 1–27. doi:10.1016/j.fluid.2006.11.014
Nath, D., and Henni, A. (2020). Solubility of carbon dioxide (CO2) in four bis (trifluoromethyl-sulfonyl) imide ([Tf2N]) based ionic liquids. Fluid Phase Equilibria 524, 112757. doi:10.1016/j.fluid.2020.112757
Nematpour, M., Jalili, A. H., Ghotbi, C., and Rashtchian, D. (2016). Solubility of CO2 and H2S in the ionic liquid 1-ethyl-3-methylimidazolium trifluoromethanesulfonate. J. Nat. Gas Sci. Eng. 30, 583–591. doi:10.1016/j.jngse.2016.02.006
Nonthanasin, T., Henni, A., and Saiwan, C. (2014). Densities and low pressure solubilities of carbon dioxide in five promising ionic liquids. RSC Adv. 4 (15), 7566–7578. doi:10.1039/c3ra46339g
Ren, Y., Yu, C., Tan, X., Huang, H., Wei, Q., and Qiu, J. (2021). Strategies to suppress hydrogen evolution for highly selective electrocatalytic nitrogen reduction: challenges and perspectives. Energy and Environ. Sci. 14 (3), 1176–1193. doi:10.1039/d0ee03596c
Safarov, J., Guluzade, A., and Hassel, E. (2019). Carbon dioxide solubility in 1-ethyl-3-methylimidazolium trifluoromethanesulfonate or 1-butyl-3-methylimidazolium trifluoromethanesulfonate ionic liquids. J. Chem. and Eng. Data 65 (3), 1060–1067. doi:10.1021/acs.jced.9b00463
Sedghamiz, M. A., Rasoolzadeh, A., and Rahimpour, M. R. (2015). The ability of artificial neural network in prediction of the acid gases solubility in different ionic liquids. J. CO2 Util. 9, 39–47. doi:10.1016/j.jcou.2014.12.003
Song, Z., Shi, H., Zhang, X., and Zhou, T. (2020). Prediction of CO2 solubility in ionic liquids using machine learning methods. Chem. Eng. Sci. 223, 115752. doi:10.1016/j.ces.2020.115752
Stevanovic, S., and Gomes, M. C. (2013). Solubility of carbon dioxide, nitrous oxide, ethane, and nitrogen in 1-butyl-1-methylpyrrolidinium and trihexyl (tetradecyl) phosphonium tris (pentafluoroethyl) trifluorophosphate (eFAP) ionic liquids. J. Chem. Thermodyn. 59, 65–71. doi:10.1016/j.jct.2012.11.010
Suzuki, Y., Takahashi, K., Watanabe, M., Kodama, D., Makino, T., Kanakubo, M., et al. (2024a). Density, viscosity, and CO2 solubility in ether-functionalized phosphonium-based bis (trifluoromethanesulfonyl) amide ionic liquids. J. Chem. Thermodyn. 192, 107248. doi:10.1016/j.jct.2024.107248
Suzuki, Y., Watanabe, M., Kodama, D., Makino, T., Kanakubo, M., and Sasaki, M. (2024b). Density, viscosity, CO2 and CH4 solubility, and CO2/CH4 selectivity of protic and aprotic imidazolium and ammonium bis (trifluoromethanesulfonyl) amide and tetrafluoroborate ionic liquids. J. Chem. and Eng. Data 69, 1013–1025. doi:10.1021/acs.jced.3c00574
Tagiuri, A., Sumon, K. Z., and Henni, A. (2014). Solubility of carbon dioxide in three [Tf2N] ionic liquids. Fluid Phase Equilibria 380, 39–47. doi:10.1016/j.fluid.2014.07.015
Tian, Y., Wang, X., Liu, Y., and Hu, W. (2023). Prediction of CO2 and N2 solubility in ionic liquids using a combination of ionic fragments contribution and machine learning methods. J. Mol. Liq. 383, 122066. doi:10.1016/j.molliq.2023.122066
Tian, Y., Wang, X., Liu, Y., and Hu, W. (2024). Prediction of nitrogen solubility in ionic liquids by machine learning methods based on COSMO-derived descriptors. Chem. Eng. Sci. 284, 119482. doi:10.1016/j.ces.2023.119482
Vasileff, A., Xu, C., Jiao, Y., Zheng, Y., and Qiao, S.-Z. (2018). Surface and interface engineering in copper-based bimetallic materials for selective CO2 electroreduction. Chem 4 (8), 1809–1831. doi:10.1016/j.chempr.2018.05.001
Wang, K., Xu, H., Yang, C., and Qiu, T. (2021). Machine learning-based ionic liquids design and process simulation for CO2 separation from flue gas. Green Energy and Environ. 6 (3), 432–443. doi:10.1016/j.gee.2020.12.019
Wang, N., Cheng, H., Wang, Y., Yang, Y., Teng, Y., Li, C., et al. (2022). Measuring and modeling the solubility of carbon dioxide in protic ionic liquids. J. Chem. Thermodyn. 173, 106838. doi:10.1016/j.jct.2022.106838
Watanabe, M., Kodama, D., Makino, T., and Kanakubo, M. (2016). CO2 absorption properties of imidazolium based ionic liquids using a magnetic suspension balance. Fluid Phase Equilibria 420, 44–49. doi:10.1016/j.fluid.2015.12.055
Yuan, X., Zhang, S., Chen, Y., Lu, X., Dai, W., and Mori, R. (2006). Solubilities of gases in 1, 1, 3, 3-tetramethylguanidium lactate at elevated pressures. J. Chem. and Eng. Data 51 (2), 645–647. doi:10.1021/je050437s
Zeng, S., Wang, J., Bai, L., Wang, B., Gao, H., Shang, D., et al. (2015). Highly selective capture of CO2 by ether-functionalized pyridinium ionic liquids with low viscosity. Energy and Fuels 29 (9), 6039–6048. doi:10.1021/acs.energyfuels.5b01274
Zhang, Y., Zhao, X., Yang, Q., Zhang, Z., Ren, Q., and Xing, H. (2017). Long-chain carboxylate ionic liquids combining high solubility and low viscosity for light hydrocarbon separations. Industrial and Eng. Chem. Res. 56 (25), 7336–7344. doi:10.1021/acs.iecr.7b00660
Zhao, Y., Gani, R., Afzal, R. M., Zhang, X., and Zhang, S. (2017). Ionic liquids for absorption and separation of gases: an extensive database and a systematic screening method. AIChE J. 63 (4), 1353–1367. doi:10.1002/aic.15618
Zhao, Y., Wang, X., Li, Z., Wang, H., Zhao, Y., and Qiu, J. (2024). Understanding the positive role of ionic liquids in CO2 capture by poly (ethylenimine). J. Phys. Chem. B 128 (4), 1079–1090. doi:10.1021/acs.jpcb.3c06510
Zhao, Y., Zhang, X., Dong, H., Zhen, Y., Li, G., Zeng, S., et al. (2011). Solubilities of gases in novel alcamines ionic liquid 2-[2-hydroxyethyl (methyl) amino] ethanol chloride. Fluid phase equilibria 302 (1-2), 60–64. doi:10.1016/j.fluid.2010.08.017
Zhao, Z., Xing, X., Tang, Z., Zheng, Y., Fei, W., Liang, X., et al. (2018). Experiment and simulation study of CO2 solubility in dimethyl carbonate, 1-octyl-3-methylimidazolium tetrafluoroborate and their mixtures. Energy 143, 35–42. doi:10.1016/j.energy.2017.10.116
Zhou, F., Azofra, L. M., Ali, M., Kar, M., Simonov, A. N., McDonnell-Worth, C., et al. (2017). Electro-synthesis of ammonia from nitrogen at ambient temperature and pressure in ionic liquids. Energy and Environ. Sci. 10 (12), 2516–2520. doi:10.1039/c7ee02716h
Zhou, L., Fan, J., and Shang, X. (2014). CO2 capture and separation properties in the ionic liquid 1-n-butyl-3-methylimidazolium nonafluorobutylsulfonate. Materials 7 (5), 3867–3880. doi:10.3390/ma7053867
Zhou, L., Fan, J., Shang, X., and Wang, J. (2013). Solubilities of CO2, H2, N2 and O2 in ionic liquid 1-n-butyl-3-methylimidazolium heptafluorobutyrate. J. Chem. Thermodyn. 59, 28–34. doi:10.1016/j.jct.2012.11.030
Zhou, L., Shang, X., Fan, J., and Wang, J. (2016). Solubility and selectivity of CO2 in ether-functionalized imidazolium ionic liquids. J. Chem. Thermodyn. 103, 292–298. doi:10.1016/j.jct.2016.08.028
Zoubeik, M., Mohamedali, M., and Henni, A. (2016). Experimental solubility and thermodynamic modeling of CO2 in four new imidazolium and pyridinium-based ionic liquids. Fluid Phase Equilibria 419, 67–74. doi:10.1016/j.fluid.2016.03.009
Zubeir, L. F., Nijssen, T. M., Spyriouni, T., Meuldijk, J., Hill, J. r.-R. d., and Kroon, M. C. (2016a). Carbon dioxide solubilities and diffusivities in 1-alkyl-3-methylimidazolium tricyanomethanide ionic liquids: an experimental and modeling study. J. Chem. and Eng. Data 61 (12), 4281–4295. doi:10.1021/acs.jced.6b00657
Zubeir, L. F., Spyriouni, T., Roest, D., Hill, J. r.-R. d., and Kroon, M. C. (2016b). Effect of oxygenation on carbon dioxide absorption and thermophysical properties of ionic liquids: experiments and modeling using electrolyte PC-SAFT. Industrial and Eng. Chem. Res. 55 (32), 8869–8882. doi:10.1021/acs.iecr.6b01984
Keywords: ionic liquid, CO2 solubility, N2 solubility, COSMO-RS, machine learning
Citation: Qin H, Wang K, Ma X, Li F, Liu Y and Ji X (2024) Predicting the solubility of CO2 and N2 in ionic liquids based on COSMO-RS and machine learning. Front. Chem. 12:1480468. doi: 10.3389/fchem.2024.1480468
Received: 16 August 2024; Accepted: 14 October 2024;
Published: 31 October 2024.
Edited by:
Ziqi Tian, Ningbo Institute of Materials Technology and Engineering, Chinese Academy of Sciences (CAS), ChinaReviewed by:
Jikuan Qiu, Henan Normal University, ChinaCopyright © 2024 Qin, Wang, Ma, Li, Liu and Ji. This is an open-access article distributed under the terms of the Creative Commons Attribution License (CC BY). The use, distribution or reproduction in other forums is permitted, provided the original author(s) and the copyright owner(s) are credited and that the original publication in this journal is cited, in accordance with accepted academic practice. No use, distribution or reproduction is permitted which does not comply with these terms.
*Correspondence: Yanrong Liu, eXJsaXVAaXBlLmFjLmNu; Xiaoyan Ji, eGlhb3lhbi5qaUBsdHUuc2U=
Disclaimer: All claims expressed in this article are solely those of the authors and do not necessarily represent those of their affiliated organizations, or those of the publisher, the editors and the reviewers. Any product that may be evaluated in this article or claim that may be made by its manufacturer is not guaranteed or endorsed by the publisher.
Research integrity at Frontiers
Learn more about the work of our research integrity team to safeguard the quality of each article we publish.