- 1The First Clinical Medical College of Zhejiang Chinese Medical University, Hangzhou, Zhejiang, China
- 2Department of Oncology, Affiliated Hangzhou First People’s Hospital, School of Medicine, Westlake University, Hangzhou, China
β-secretase 1, one of the most important proteins, is an aspartate protease. This membrane-associated protein is used for treating Alzheimer’s disease (AD). Several inhibitors have been pursued against β-secretase 1, but they still have not resulted effectively. Virtual screening based on pharmacophores has been shown to be useful for lead optimization and hit identification in the preliminary phase of developing a new drug. Here, we screen the commercially available databases to find the hits against β-secretase 1 for drug discovery against AD. Virtual screening for 200,000 compounds was done using the database from the Vitas-M Laboratory. The phase screen score was utilized to assess the screened hits. Molecular docking was performed on compounds with phase scores >1.9. According to the study, the 66H ligand of the crystal structure has the maximum performance against β-secretase 1. The redocking of the co-crystal ligand showed that the docked ligand was seamlessly united with the crystal structure. The reference complex had three hydrogen bonds with Asp93, Asp289, and Gly291; one van der Waals interaction with Gly74; and three hydrophobic interactions. After equilibration, the RMSD of the reference compound sustained a value of ∼1.5 Å until 30 ns and then boosted to 2.5 Å. On comparison, the RMSD of the S1 complex steadily increased to ∼2.5 Å at 15 ns, displayed slight aberrations at approximately ∼2.5–3 Å until 80 ns, and then achieved steadiness toward the end of the simulation. The arrangements of proteins stayed condensed during the mockup when bonded to these complexes as stable Rg values showed. Furthermore, the MM/GBSA technique was employed to analyze both compounds’ total binding free energies (ΔGtotal). Our research study provides a new understanding of using 66H as anti-β-secretase 1 for drug development against AD.
1 Introduction
Neurodegenerative disorders (NDDs) are characterized by the gradual deterioration and loss of specific groups of neurons, primarily those affiliated with the central nervous system (Behl et al., 2021). Alzheimer’s disease (AD) causes neurodegenerative changes characterized by an advanced, irreversible, and subtle weakening of cognitive function, including memory loss and various cognitive impairments (Yusufzai et al., 2018). AD is a widespread and frequently encountered type of dementia (Bogdanovic et al., 2020), associated with decreased memory and cognition (Marelli et al., 2020; Wilson et al., 2012). A sixth leading cause of death is in the geriatric population (Gaugler et al., 2019). The progression of AD comprises three primary aspects. First, a lack of cholinergic transmission results from losing cholinergic neurons. Second, the buildup of extracellular residues of β-amyloid protein occurs, owing to the catalytic action of β-secretase 1 (BACE1). Last, neurofibrillary bundles comprise a tau protein in the phosphorylated form (Falco et al., 2016; Selkoe and Hardy, 2016; Gaugler et al., 2019). The development of extracellular residues of the β-amyloid peptide and the buildup of unsolvable plaques in neurons are central to the amyloid hypothesis, which connects AD to this pathophysiological process. This process starts the transmembrane protein (amyloid precursor protein-APP) breakdown by the enzyme BACE1. Another enzyme, γ-secretase, terminates this cleavage process and generates the β-amyloid peptide (Aβ), aggregating into oligomers. These oligomers form plaques that accumulate in many regions of the brain, mainly in neurons located in the cortex entorhinal, hippocampus, basal nucleus, and associative cortex (Sabbah and Zhong, 2016). A recently FDA-approved drug called Aduhelm© (aducanumab) is among the few medications utilized to address the amyloid hypothesis (FDA Grants Accelerated Approval for Alzheimer’s Drug | FDA). This drug functions as a monoclonal antibody specifically designed to target combined forms of amyloid beta agglomerates, thereby reducing the accumulation of extracellular deposits of the β-amyloid peptide. BACE1 is one of the most essential membrane-associated aspartate protease proteins focused on treating AD (Ghosh et al., 2012; Kandalepas and Vassar, 2012; Yan and Vassar, 2014). Beta-amyloid peptide (Aβ) development in AD can be terminated by inhibiting BACE1 (Boutajangout et al., 2011; Kwak et al., 2011; Yan and Vassar, 2014). The formation of BACE1 inhibitors, which is followed for many years, has still not been established as an effective treatment method. However, constant improvement in this sphere has led to the formation of inhibitors that display widespread activity, from nano to micromolar. Consequently, evolving inhibitors for BACE1 have been an effective curative approach for AD drug discovery. A pharmacophore is an organization of structural elements and molecular features related to biological activity (Wermuth, 2006). Lately, this phrase has become one of the most well-known icons in the discovery of drugs. As an esteemed tool for drug planning, virtual pharmacophore screening has been established as valuable for lead optimization and hit identification in the preliminary phase of the novel drug development (Gautam et al., 2023). The benefit of this method is that virtually, most compounds can be screened for hit identification. Points in 3D space usually signify pharmacophore features. A feature of pharmacophores could be composed of functional groups, such as the hydrogen bond acceptor (HBA), hydrogen bond donor (HBD), anions, cations, hydrophobic area (Hyp), and aromatics (Dror et al., 2004; Hou et al., 2006). Cautiousness should be employed while controlling the structural flexibility for generating pharmacophores where the active conformation of particles is hypothesized.
The study aimed to address the urgent need for effective treatments for AD, characterized by the accumulation of amyloid-beta plaques in the brain. BACE1, being a crucial protein involved in the production of amyloid-beta, represents a promising therapeutic target for AD. However, despite extensive efforts, existing BACE1 inhibitors have not been sufficiently effective in clinical trials.
The method of receptor or ligand-based pharmacophore virtual screening includes a diversity of chronological computational steps: target identification, preparation of database, pharmacophore model creation, 3D screening, and arrangement of complexes for the final confirmation of biological activity (Köppen, 2009; Liu et al., 2023). Virtual screening provides a profitable, time-saving method in the novel lead compound search (Muhammed and Esin, 2021). Screening virtually is an obligatory part of the drug discovery pipeline and a vital procedure for finding hits or chemical probes (Kumar and Zhang, 2015; Leung and Ma, 2015). In this study, we have targeted the commercially available databases to discover the pharmacophore-based virtual screening against BACE1 for drug discovery against AD.
The study offers medicinal chemists, biochemists, and pharmacologists a promising avenue for advancing AD therapeutics through the identification and characterization of a novel compound, 66H, targeting BACE1. Through virtual screening and molecular docking, 66H emerged as a lead candidate, with subsequent molecular dynamics simulations confirming its stable binding to BACE1. The study also explored the key molecular interactions and assessed the compound’s binding affinity using MM/GBSA analysis, providing crucial insights for further medicinal chemistry optimization and biochemical validation.
2 Methodology
2.1 Pharmacophore hypothesis development
Protein Data Bank (https://www.rcsb.org/) was used for retrieving the crystal structures of the ΒΑCE1 protein. One of the studies suggested the co-crystal ligands’ activity against ΒΑCE1 was taken into consideration (Stamford and Strickland, 2013; Egbertson et al., 2015; Gupta et al., 2020). The pharmacophore model, which was receptor–ligand-based, was developed according to the inhibitor with the highest activity against BACE1. The Schrödinger phase tool acquired the pharmacophore hypothesis (Dixon et al., 2006). The protein-binding pocket and ligand sites were targeted for building the hypothesis. Moreover, the receptor was prepared by following the steps in Section 2.4 before the hypothesis was developed.
2.2 Preparation and virtual screening of database
The VITAS-M Laboratory database comprised 1.4 million compounds, from which 0.2 million complexes were selected. (Dixon et al., 2006), transferred, and arranged through phases. Ten conformers were produced for each ligand to expand the search for chemical space. Epik generated diverse likely states at pH 7, while the tautomeric states (Shelley et al., 2007), having high-energy, were eliminated from the database. Then, virtual screening was initiated from the prepared database, agreeing to the developed hypothesis. The phase screen score was used to assess the screened hits according to the mixture of volume score, RMSD, and site matching. Phase scores >1.9 were identified for the molecular docking studies.
2.3 ADMET and drug-likeness
The selective commercial complexes were subjected to absorption, metabolism, distribution, excretion, and toxicity (ADMET) in the QikProp module of Maestro, and ADME (http://www.swissadme.ch) and ADMETlab 2.0 (https://admetmesh.scbdd.com/) to evaluate ADMET and drug-likeness parameters. Compounds which passed the Lipinski’s rule of five and toxicity parameters were considered for further analysis. Different software tools, such as QikProp, ADME, and ADMET, have been employed to comprehensively assess the pharmacokinetic and pharmacodynamics properties (ADMET) of the identified compound. QikProp is known for its ability to predict a wide range of physicochemical properties. ADME specializes in offering insights into the compound’s bioavailability and metabolic stability. ADMET mainly focuses on predicting potential adverse effects and toxicity profiles.
2.4 Molecular docking
The β-secretase 1 (PDB ID: 5HU0) crystal structure was prepared in Maestro (Madhavi Sastry et al., 2013). The receptor was preprocessed by introducing hydrogens and charges, eliminating water and setting the residue side-chain atoms. The redundant sequences were eliminated, while the tautomeric states at pH 7 were produced (Sadeer et al., 2019), employing PropKa. The protein receptor was further organized and minimized by OPLS_2005 force field (Shivakumar et al., 2012). The framework was created by choosing the site-specific crystal ligand to complete docking. To unstiffen the activity of non-polarized receptor slices, the radii of the receptor atom, i.e., the van der Waals, were graduated to 1.0, with the partial charge limit set to 0.25. The coordinates X, Y, and Z results were 23.55, 10.39, and 21.58, respectively. After grid creation, the ligands were primed by the LigPrep tool of Maestro before docking (Matsuoka et al., 2017). Diverse ionization shapes were produced at pH 7 by employing Epik (Shelley et al., 2007). The isomers of complexes with definite chirality are produced using the OPLS_2005 force field. A glide docking tool was used to stop ligands’ arrangement to the set protein receptor, and the binding positions were evaluated according to the glide score.
2.5 Molecular dynamics (MD) simulation
The best binding poses of the particular hits and reference complex with protein were subjected to 100 ns using NAMD (Phillips et al., 2020) and VMD (Humphrey et al., 1996) to discover their strength. As an initial phase, the preliminary records necessary to execute the simulation were arranged through the elements (Case et al., 2021) of AmberTools 21. The components of an antechamber created the parameters of the conjugate solution, while the LEAP program (Case et al., 2005) added the lost hydrogen atoms in the protein arrangements. TIP3P water particles were introduced to the structures (Jorgensen and Chandrasekhar, 1983) in a periodic box after the parameterization of 10 Å and then defused by adding sodium cations. The energy conflicts were eliminated by minimizing the technique using the ff14SB force field for GAFF ligands and protein (Duan et al., 2003). After depreciation, solvation was equilibrated for 10,000 steps, which was trailed by temperature balancing at 200, 250, and 300 K. The concluding equilibrated procedures were then exposed to a 100-ns production run, and the trajectories were saved at every 2 ps for the evaluation. The Bio3D package of R was used to calculate the MD trajectories (Grant et al., 2021).
3 Results
3.1 Alignment of protein structures
Protein Data Bank was used to retrieve the crystal structures of the ΒΑCE1 protein. The literature was searched for the IC50 cut-offs of the co-crystal ligands. As evident, the ligand of co-crystal 66H has shown maximum performance against the protease protein between the selected ligands; thus, it was chosen to study further. The IDs of PDB crystals and the IC50 cut-offs are presented in Table 1.
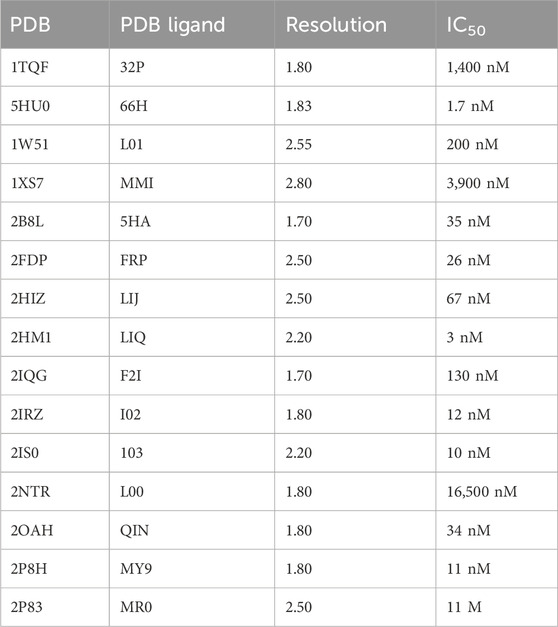
Table 1. IDs of PDB, ligands, and the chemical mechanism of a co-crystal ligand against the BACE1 protein.
3.2 Generation of the receptor-based pharmacophore
A five-feature pharmacophore model was created by choosing ligand sites and pocket residues with specific binding. The pharmacophore hypothesis comprised features such as R9, R10, R11, D9, and D7, along with their coordinates in the structure of protein (Table 2; Figure 1A). The descriptions of the binding pocket’s cavity are witnessed in Figure 2B.
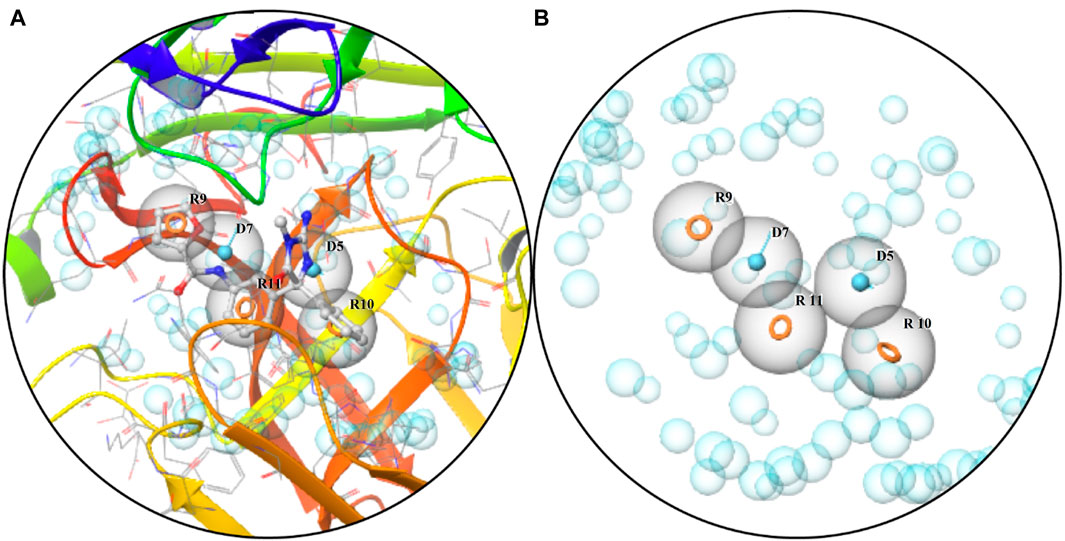
Figure 1. Pharmacophore: (A) characteristics of the binding pocket and (B) pharmacophore hypothesis and the binding pocket cavity.
3.3 Virtual screening
The database of Vitas-M Laboratory library was utilized to screen the hypothesis of pharmacophores virtually. At least four features have been selected to identify a complex as a hit. The final ranking of hits from the screening, contributing to the phase fitness score, was determined by comprising vector arrangements, volume scores, and matching RMSD site. The range of vector scores is from −1 to 1, where greater scores indicate improved alignment. On the other hand, the volume scores ranging from 0 to 1 indicate a higher overlap among the reference and aligned ligand levels with higher scores. It is determined as the overlaying of both the volumes of the ligand divided by the total volume of both ligands. The score is zero if no reference ligand is present. A cutoff score of 1.9 during the phase screen was chosen to identify the potential hits above this threshold (Table 3). The structures of 84 hits have been provided in Supplementary Figures S1–S84.
3.4 β-secretase 1 structure and sequence analysis
The sequence of the BACE1 precursor (P56817) was acquired from UniProt.
Physiochemical features: The physiochemical features of the sequences of the BACE1 precursor were determined by ProtParam. The amino acid profile of the BACE1 precursor showed 9.8% residues of leucine along with 8.6% and 7.4% residues of glycine and valine, respectively. The molecular weight was approximately 55,763.79. There were 42 positively charged arginine and lysine residues along with the 55 negatively charged aspartate and glutamic acid residues. The isoelectric point (PI) value of 5.31 specifies that the protein is slightly acidic, whereas the instability index of 44.23 shows that it is somewhat unstable. This instability was predicted due to the existence of certain dipeptides that are lacking from steady proteins. A greater aliphatic index (88.14) revealed that the protein was mildly thermostable, while a lesser GRAVY score (−0.064) implied that the protein may interact well with water. The extinction coefficient is 85,425 M-1cm-1 at 280 nm, similar to cysteine, tryptophan, and tyrosine concentrations. To calculate protein–ligand and protein–protein interactions in the solution, this coefficient is valuable.
3.5 ADMET
The computational tools QikProp, SwissADME, and ADMETlab 2.0 were used to make predictions for a variety of physiochemical (Supplementary Table S1), medicinal chemistry (Supplementary Table S2), absorption, distribution (Supplementary Table S3), metabolism, excretion (Supplementary Table S4), and toxicity parameters (Supplementary Table S5) for a total of 84 distinct chemicals. As a result, ligands with significant pharmacokinetic features fall within acceptable ranges when using ADMET analysis. The ADMET properties show that all compounds were found to have good pharmacokinetic characteristics and no notable side effects. It was also thought that the potential for medical use was positive.
3.6 Molecular docking
The BACE1 receptor was docked by the hits employing the typical precise procedure of the glide tool. Before the docking of screened hits with protein, the effectiveness of this procedure was measured by the redocking of the co-crystal ligand. The redocking of the co-crystal ligand showed that the docked ligand is aligned with the crystal structure (Figure 3A). The docked hits were compared with the reference ligand, and two hits were selected for further analysis. The selected hits with the glide scores are specified in Table 4. The connections of the molecules for the chosen hits were analyzed and compared with the reference compound. The reference complex made three hydrogen bonds with Gly291, Asp93, and Asp289; one van der Waals interaction with Gly74; and three hydrophobic interactions (Figure 3B).
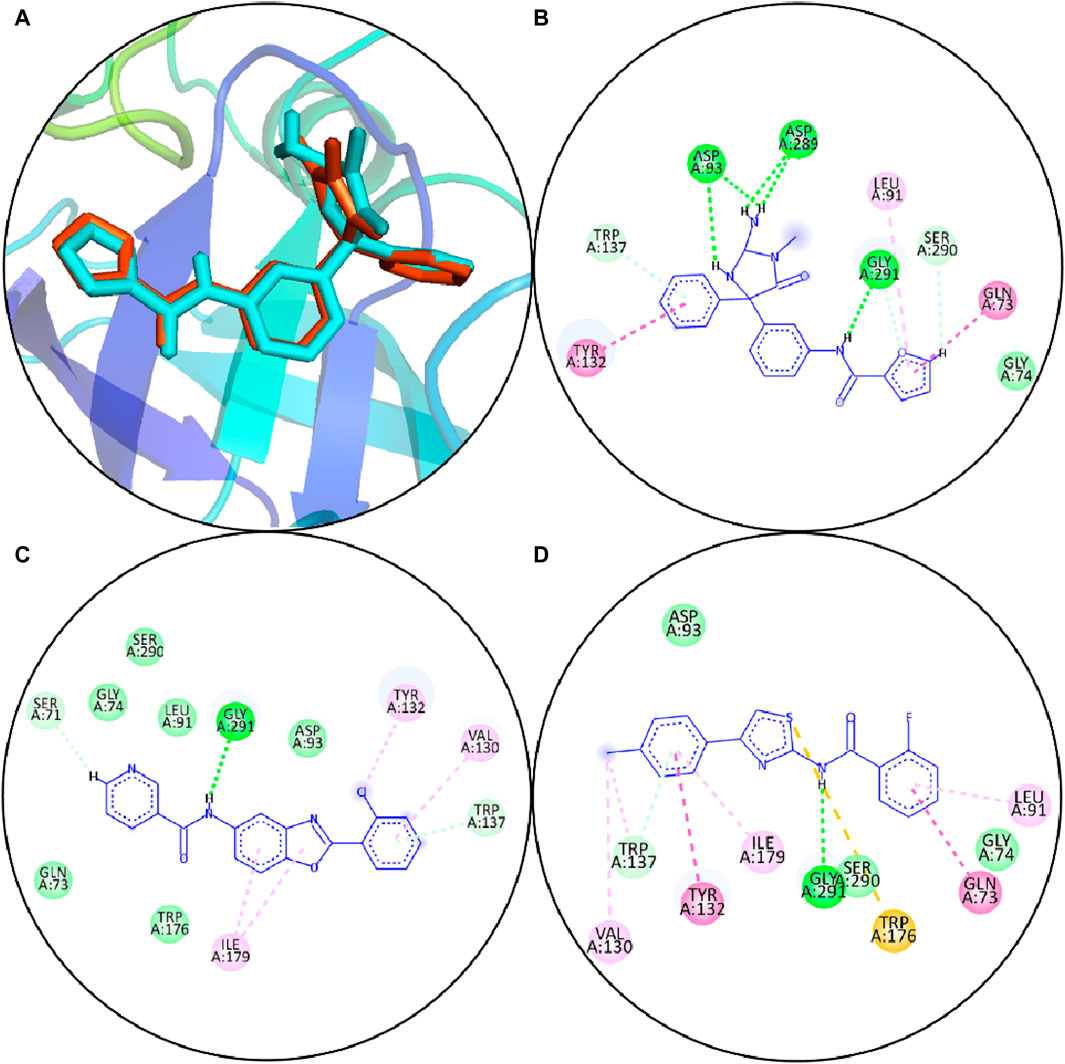
Figure 3. (A) Redocking of the reference compound. Orange sticks show the reference pose, and cyan sticks show the docked pose. (B) Molecular interactions of reference compounds. (C) Molecular interfaces of S1. (D) Molecular interactions of S2. Green lines display the hydrogen bonds; light green shows the van der Waals interactions; magenta lines show hydrophobic interactions; purple lines show pi–sigma bonds, and pi–sulfur interactions are shown by orange lines.
In comparison, S1 made one hydrogen bond with Gly291 and five van der Waals interactions with Gln73, Gly74, Leu91, Trp176, and Ser290. Moreover, the reference complex had three hydrophobic interfaces (Figure 3C). S2 also made one hydrogen bond with Gly291; three van der Waals interactions with Gly74, Asp93, and Ser290; and five hydrophobic interactions (Figure 3D). The plausible binding modes of selected docked hits were also analyzed (Figures 4A–C).
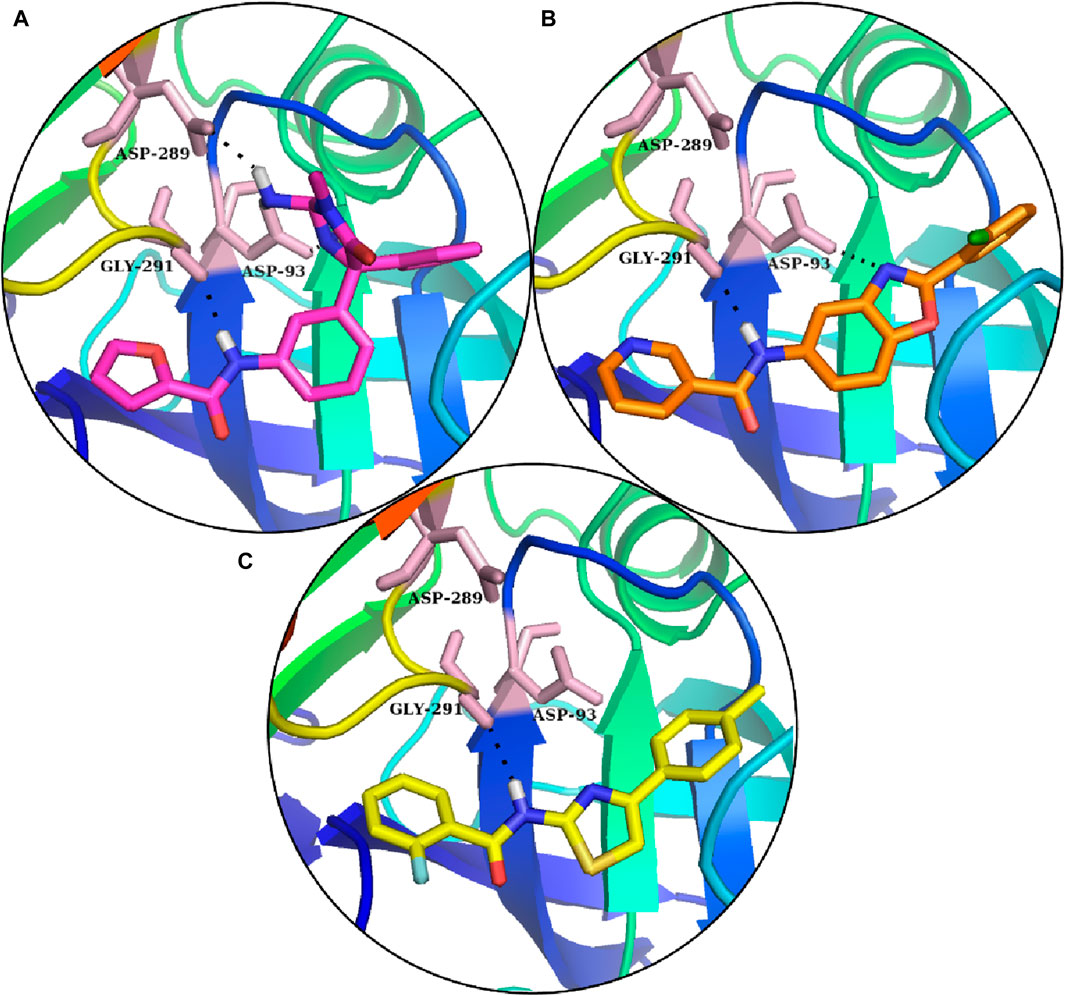
Figure 4. Reference’s plausible binding modes and selected compounds are represented with the sticks in the binding pocket of BACE1. (A) Reference compounds: (B) S1 hit. (C) S2 hit.
3.7 MD simulation
The docked poses of the selected hit ligands were superposed on the co-crystal ligand, as shown in Figures 5A, B, and then subjected to MD simulation for the protein–ligand stability analysis.
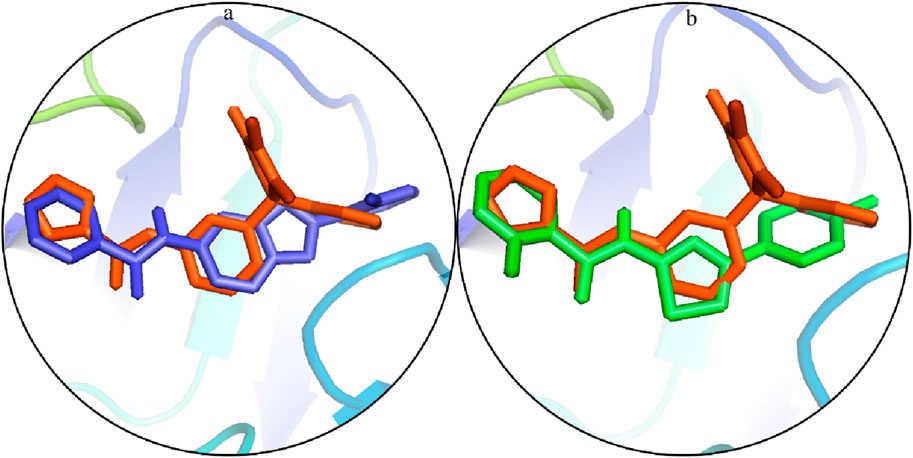
Figure 5. Potential binding configurations of the ligand with the crystal structure: (A) S1 is illustrated using blue sticks. (B) S2 is depicted using green sticks.
The significant molecules of the protein structure complexed with the reference compound, and hit root mean square deviation (RMSD) values were calculated from the trajectories to assess the steadiness of the protein–ligand compound (Sargsyan et al., 2017). It was witnessed that at 5 ns, all compounds were balanced (Figure 6A). After balancing, the deviation of the reference compound upheld a value of ∼1.5 Å near 30 ns and then enhanced to 2.5 Å. Passing the 30-ns milestone, the RMSD stayed at approximately∼ 1.75–2 Å until the end. On comparison, the RMSD of the S1 complex slowly amplified to ∼2.5 Å near 15 ns, showed slight deviations at approximately ∼2.5–3 Å near 80 ns, and then achieved steadiness toward the end of the simulation. The RMSD values of the S2 complex were approximately ∼1.25–1.5 Å during the simulation. The protein structure’s physical density, when bound to hit and reference complexes, was evaluated by analyzing the radius of gyration (Lobanov et al.). Lesser values of Rg indicated structural solidity, while greater Rg values meant structural deformities throughout the simulation. The Rg profiles for the compounds indicated that Rg values were kept within the range of approximately 20.75–21.5 Å after a 5-ns equilibration period. The Rg value for the S1 complex consistently remained near 21.5 Å during the simulation, whereas the Rg values for the S2 complex remained at approximately 20.75 Å. These stable Rg scores imply that the arrangements of proteins stayed compressed throughout the simulation in the presence of these compounds (refer to Figure 6B). When bound to these ligands, the dynamic behavior of protein restudies was calculated by the root mean square fluctuations (RMSFs) (Martínez, 2015). The RMSF scores of protein deposits fluctuate less than 1 Å throughout the simulation period, excluding the loop regions (Figure 6C). The RMSF figure indicated that the protein residues were rigid and did not show major fluctuations during simulation, suggesting the steadiness of the protein–ligand complex. The RMSF value of the loop residues extended to the highest value of ∼5 Å in the reference compound–complex.
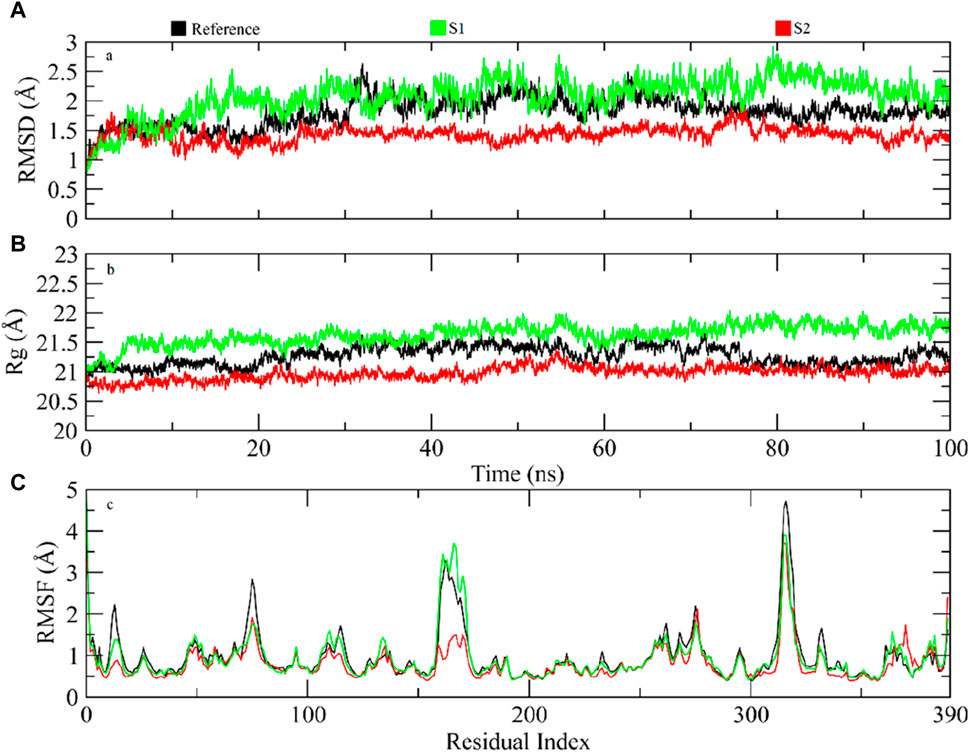
Figure 6. (A) RMSD profiles for protein and ligand simulation over 100 ns. (B) Analysis of Rg for the density of the protein structure. (C) Evaluating residual variations in the protein structure during simulations by RMSF values.
The RMSF graph reveals some regions with high fluctuations within the protein structure, indicating areas of increased flexibility. Specifically, residues around index 100, between indices 200–210, and near index 300 exhibit significant peaks, with the peak around residue 300 being the most pronounced. Additionally, there is also a noticeable flexibility near residue 390. These fluctuations suggest that these particular residues are more dynamic, potentially due to specific interactions in the protein’s structure.
3.8 Molecular mechanics/generalized born surface area
The molecular mechanics/generalized born surface area (MM/GBSA) system helped analyze both complexes’ total binding free energy (ΔGtotal). The result is typically used to evaluate the strength of the ligand–protein compound (Du et al., 2011). The lower ΔGtotal values specify that the compound is steadier and conversed. It was calculated as a sum of the ligand–protein compound and the difference of protein and its ligands’ free energies. The total binding free energy assessed utilizing the MM/GBSA method is the result of the input of several protein–ligand interfaces such as electrostatic energy (ΔEele), van der Waals energy (ΔEvdW), and electrostatic contribution to solvation-free energy by generalized born (ΔGGB). The total binding free energies are presented in Table 5. The ΔEvdW role of the S1 compound was more than that of the reference and S2 complexes. At the same time, the electrostatic contribution was more in the reference complex. The GB contribution showed that the reference has a higher GB value than the hits. Both hits’ total binding free energies were more than those of the reference compound, as shown in the table. The total binding free energy and its influence on each energy module are shown in Figure 7.
The structural assessment of STK346841 and STK122203 shows that both have feature benzene rings as foundational elements, characteristic of many aromatic compounds (Figure 8). Each structure also incorporates a heterocyclic ring; specifically, a pyridine ring is present in both, indicating a common structural motif where a nitrogen atom is integrated into a six-membered aromatic ring. This shared feature suggests a similarity in some aspects of their chemical reactivity and possible applications.
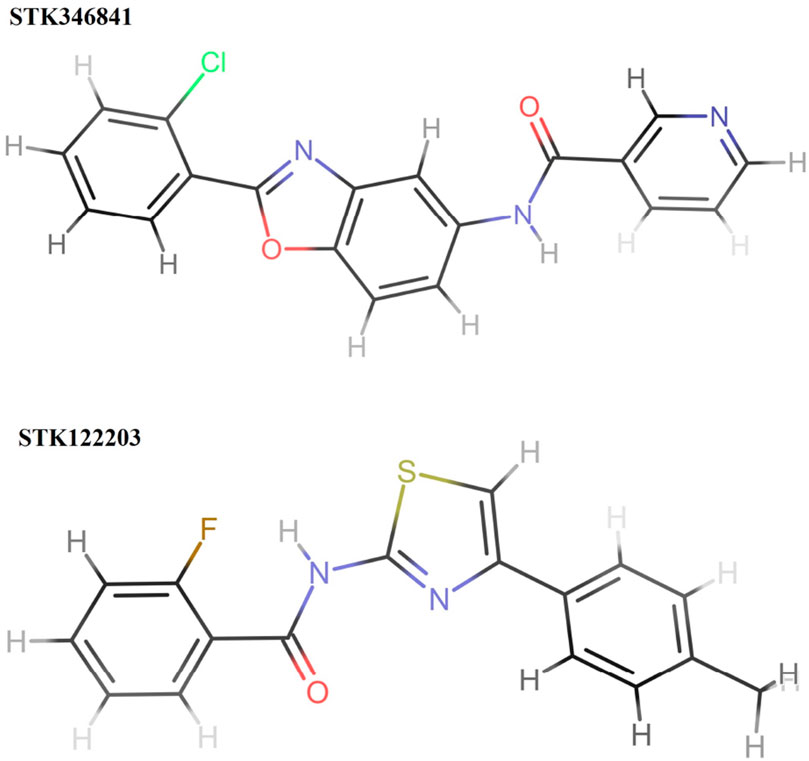
Figure 8. Structures of two hits (STK346841 and STK122203). STK346841 (IUPAC: N-[2-(2-chlorophenyl)-1,3-benzoxazol-5-yl]pyridine-3-carboxamide) has a chlorine (Cl) atom and an imidazole ring as substituents. STK122203 (IUPAC: 2-fluoro-N-[4-(4-methylphenyl)-1,3-thiazol-2-yl]benzamide) features a fluorine (F) atom and a thiazole ring (a ring containing both sulfur and nitrogen).
The post-simulation interaction analysis was performed at 0 ns and 100 ns (Figure 9). There was no significant difference at 0-ns and 100-ns snap shots. All three ligands (references: BACE1, S1-BACE1, and S2-BACE1) show a pi–pi stacked interaction with Tyr75, indicating a critical role of this residue in stabilizing the ligand through aromatic interactions. Similarly, both references BAC1 and S1-BAC1 exhibit pi–alkyl interactions with Leu34, suggesting that hydrophobic interactions with this residue are also important. Moreover, the reference BACE1 has conventional hydrogen bonds with Asp36 and Aap232, which are not present in S2-BACE1. S1-BACE1 retains the hydrogen bond with Asp36 and exhibited one more with Trp80.
4 Discussion
The prevalence of neurodegenerative diseases has significantly increased in the medical field over recent years, posing a significant health concern. Various molecular targets are implicated in the pathogenesis of these diseases. These clusters of diseases, including AD and other related disorders such as spinal muscular atrophy (SMA), Parkinson’s disease (PD), Huntington’s disease (HD), spinocerebellar ataxia (SCA), prion disease, and motor neuron diseases (MND), have been reported to affect millions of people worldwide (Dugger and Dickson, 2017). BACE1 is an aspartate protease. This membrane-associated protein treats AD (Ghosh et al., 2012; Kandalepas and Vassar, 2012). The development of beta-amyloid peptide (Aβ) in AD can be terminated by inhibiting BACE1 (Boutajangout et al., 2011; Kwak et al., 2011; Yan and Vassar, 2014). The formation of BACE1 inhibitors, which is followed for many years, has still not been established as an effective treatment. However, constant improvement in this sphere has led to the formation of inhibitors that display widespread activity, from nano to micromolar. Consequently, evolving inhibitors for BACE1 have been an effective curative approach for AD drug discovery.
In this study, out of 1.4-M compounds in the database of Vitas-M Laboratory, 0.2-M complexes were selected, transferred, and arranged through phase (Dixon et al., 2006). Several groups of researchers have performed similar studies, such as for the discoveries of vaccines (Stokes et al., 2020), for in silico drug repositioning for AD (Galeana-Ascencio et al., 2023), and some other neurodegenerative diseases (Ishola et al., 2021). Others have performed similar approaches for de novo drug design (Wang et al., 2022). The Protein Data Bank (https://www.rcsb.org/) was used for retrieving the crystal structures of the BACE1 protein. One of the studies suggested the co-crystal ligands’ activity against BACE1, which was taken into consideration (Stamford and Strickland, 2013; Egbertson et al., 2015; Gupta et al., 2020). The pharmacophore model, which was receptor–ligand-based, was developed according to the inhibitor with the highest activity against BACE1. The Schrödinger phase tool acquired the pharmacophore hypothesis (Dixon et al., 2006).
Some previously reported BACE1 inhibitors, verubecestat (MK-8931) and its analog umibecestat (CNP-520), reached phase II/III clinical trials (Neumann et al., 2018; Thaisrivongs et al., 2018). However, these inhibitors were discontinued in February 2018 (Merck 2018.) and July 2019 (NIA, 2019), respectively, because they were associated with a decline in cognitive functions in participants.
In the current study, the two compounds S1 and S2 exhibited many hydrophobic and hydrogen bonding including hydrophobic interactions of S1; Gln>73, Gly>74, Leu>91, Asp>93, Trp>137, Trp>176, and Ser>290; hydrogen binding of Val>130, Tyr>132, Ile>179, and Gly>291; S2 hydrophobic interactions including Gly>74, Leu>91, and Asp>93; and hydrogen bonding of Gln>73, Val>130, Tyr>132, Trp>137, Trp>176, Ile>179, Ser>290, and Gly>291 (Figure 3). The previous study indicated that the AM-6494 inhibitor demonstrated active binding with BACE1, primarily involving interactions with specific amino acid residues including Lys>9, Gly>11, Gly>13, Tyr>14, Leu>30, Gly>34, Tyr>71, Thr>72, Phe>108, Trp>115, Ile>118, Val>170, Gly>230, Thr>231, Thr>232, Arg>235, Arg>307, and Ala>335. It is noteworthy that these interactions occur alongside the protonated Asp>32 and Asp>228 catalytic dyad (Ugbaja et al., 2021). The study also reported that 19 amino acid residues demonstrated interactions with the CNP-520 ligand, in which eight amino acids (Ser>35, Thr>72, Ile>110, Trp>115, Thr>231, Thr>232, Arg>235, and Ala>335) formed van der Waals (vdW) interactions (Ugbaja et al., 2021).
BACE1 harbors two aspartate amino acids (aa) within its extracellular protein domain (aa 93–96 and 289–292), both crucial for its protease function (Hussain et al., 1999). These residues are strategically located to facilitate the cleavage of APP at the β-site. In our study, both ligands S1 and S2 are responsible for hydrogen bonding with residues Asp>93, Gly>291, and Ser>290.
The protein-binding pocket and ligand sites were targeted for building the hypothesis. Virtual screening of pharmacophores has shown beneficial for hit identification and lead optimization in the initial phase of new drug development programs (Gautam et al., 2023). The main advantage of this approach is that virtually, millions of compounds can be screened for hit identification. Recently, virtual screening has been an obligatory part of drug research and development pipeline and an essential technique for discovering hits or chemical probes (Kumar and Zhang, 2015; Leung and Ma, 2015). Database screening from Vitas-M Laboratory library was executed virtually. At least four features must be matched to identify a complex as a hit. The final ranking of hits from the screening, contributing to the phase fitness score, was determined by vector arrangements, volume scores, and matching RMSD sites. The structures of the BACE1 protein were recovered from the Protein Data Bank. The literature was searched for the IC50 cut-offs of the co-crystal ligands. As evident, the ligand of crystal 66H showed maximum performance against the protease protein between the considered ligands. The identification of PDB, the arrangements, and the complementary IC50 values of other ligand studies are shown in Table 1. The compound STK081237 (chemical name: 6-hydrazinyl-N'-(naphthalen-1-yl)-N,N-diphenyl-1,3,5-triazine-2,4-diamine and empirical formula: C25H21N7) showed the align score of 0.31. Three compounds STK280616 (chemical name: ethyl4-phenyl-2-[(phenylcarbonyl) amino]thiophene-3-carboxylate and empirical formula: C20H17NO3S), compound STK057995 (chemical name: ethyl4-(3,4-dimethoxyphenyl)-2-[(pyridin-4-ylcarbonyl)amino]thiophene-3-carboxylate and empirical formula: C21H20N2O5S), and compound STK408850 (chemical name: ethyl4-(4-fluorophenyl)-2-[(3-methoxyphenyl)carbonyl]amino thiophene-3-carboxylate and empirical formula: C21H18FNO4S) obtained similar results of 0.453 align scores (Table 3). Recently, pharmacophore-based virtual screening and molecular docking studies of cyclin-dependent kinase inhibitors (CDKIs) have been reported (Shawky et al., 2021). Others studied applying pharmacophore modeling techniques to protease inhibitor development (Pautasso et al., 2014). A group of researchers recently reviewed the general aspects of AI and ML from the perspective of drug discovery in the CNS (Gautam et al., 2023). It is found that the co-crystal ligand 66H has the highest activity against BACE1 and can be potentially considered an inhibitor in drug development.
5 Conclusion
BACE1 is one of the most critical membrane-associated aspartate proteases that targets AD. Several inhibitors of ΒΑCE1 have been introduced, but effective therapies are still unavailable. Here, we attempted to find the most effective inhibitors against ΒΑCE1 for drug development against AD. We downloaded and prepared 200,000 compounds from the Vitas-M Laboratory database for virtual screening. We generated 10 conformers for each ligand to enhance the search in chemical space. It was found that among the studied ligands, the 66H crystal ligand exhibited the maximum performance against the protein. Our study provides a new perception of using 66H as anti ΒΑCE1 for drug development against AD.
Data availability statement
Publicly available datasets were analyzed in this study. These data can be found in the manuscript and are also available here: https://vitasmlab.biz.
Author contributions
XH: conceptualization, data curation, formal analysis, investigation, methodology, software, validation, visualization, and writing–original draft. KG: conceptualization, investigation, methodology, project administration, supervision, and writing–review and editing.
Funding
The author(s) declare that no financial support was received for the research, authorship, and/or publication of this article.
Conflict of interest
The authors declare that the research was conducted in the absence of any commercial or financial relationships that could be construed as a potential conflict of interest.
Publisher’s note
All claims expressed in this article are solely those of the authors and do not necessarily represent those of their affiliated organizations, or those of the publisher, the editors, and the reviewers. Any product that may be evaluated in this article, or claim that may be made by its manufacturer, is not guaranteed or endorsed by the publisher.
Supplementary material
The Supplementary Material for this article can be found online at: https://www.frontiersin.org/articles/10.3389/fchem.2024.1412349/full#supplementary-material
References
APECS (2024). Merck announces discontinuation of APECS study evaluating verubecestat (MK-8931) for the treatment of people with prodromal Alzheimer’s disease—merck.com. Available at: https://www.merck.com/news/merck-announces-discontinuation-of-apecs-study-evaluating-verubecestat-mk-8931-for-the-treatment-of-people-with-prodromal-alzheimers-disease/.
BACE (2019). Statement on discontinuation of BACE 1 inhibitor CNP520 in the Alzheimer’s prevention initiative generation study 1. NIA Office of Communications & Public Liaison. Available at: https://www.nia.nih.gov/news/statement-discontinuation-bace-1-inhibitor-cnp520-alzheimers-prevention-initiative-generation.
Behl, T., Makkar, R., Sehgal, A., Singh, S., Sharma, N., Zengin, G., et al. (2021). Current trends in neurodegeneration: cross talks between oxidative stress, cell death, and inflammation. Int. J. Mol. Sci. 22 (14), 7432. doi:10.3390/ijms22147432
Bogdanovic, N., Hansson, O., Zetterberg, H., Basun, H., Ingelsson, M., Lannfelt, L., et al. (2020). Alzheimer's disease-the most common cause of dementia. Lakartidningen 117.
Boutajangout, A., M Sigurdsson, E., and K Krishnamurthy, P. (2011). Tau as a therapeutic target for Alzheimer's disease. Curr. Alzheimer Res. 8 (6), 666–677. doi:10.2174/156720511796717195
Case, D. A., Aktulga, H. M., Belfon, K., Ben-Shalom, I., Brozell, S. R., Cerutti, D., et al. (2021). Amber 2021: reference manual.
Case, D. A., Cheatham III, T. E., Darden, T., Gohlke, H., Luo, R., Merz Jr, K. M., et al. (2005). The Amber biomolecular simulation programs. J. Comput. Chem. 26 (16), 1668–1688. doi:10.1002/jcc.20290
Dixon, S. L., Smondyrev, A. M., Knoll, E. H., Rao, S. N., Shaw, D. E., and Friesner, R. A. J. J. o. c.-a. m. d. (2006). PHASE: a new engine for pharmacophore perception, 3D QSAR model development, and 3D database screening: 1. Methodology and preliminary results. J. Comput. Aided. Mol. Des. 20, 647–671. doi:10.1007/s10822-006-9087-6
Dror, O., Shulman-Peleg, A., Nussinov, R., and Wolfson, H. J. (2004). Predicting molecular interactions in silico: I. A guide to pharmacophore identification and its applications to drug design. Curr. Med. Chem. 11 (1), 71–90. doi:10.2174/0929867043456287
Du, J., Sun, H., Xi, L., Li, J., Yang, Y., Liu, H., et al. (2011). Molecular modeling study of checkpoint kinase 1 inhibitors by multiple docking strategies and prime/MM–GBSA calculation. J. Comput. Chem. 32 (13), 2800–2809. doi:10.1002/jcc.21859
Duan, Y., Wu, C., Chowdhury, S., Lee, M. C., Xiong, G., Zhang, W., et al. (2003). A point-charge force field for molecular mechanics simulations of proteins based on condensed-phase quantum mechanical calculations. J. Comput. Chem. 24 (16), 1999–2012. doi:10.1002/jcc.10349
Dugger, B. N., and Dickson, D. W. (2017). Pathology of neurodegenerative diseases. Cold Spring Harb. Perspect. Biol. 9 (7), a028035. doi:10.1101/cshperspect.a028035
Egbertson, M., McGaughey, G. B., Pitzenberger, S. M., Stauffer, S. R., Coburn, C. A., Stachel, S. J., et al. (2015). Methyl-substitution of an iminohydantoin spiropiperidine β-secretase (BACE-1) inhibitor has a profound effect on its potency. Bioorg. Med. Chem. Lett., 25(21), 4812–4819. doi:10.1016/j.bmcl.2015.06.082
Falco, A. D., Cukierman, D. S., Hauser-Davis, R. A., and Rey, N. A. (2016). Doença de Alzheimer: hipóteses etiológicas e perspectivas de tratamento. Quím. Nova 39, 63–80.
Galeana-Ascencio, R. A., Mendieta, L., Limon, D. I., Gnecco, D., Terán, J. L., Orea, M. L., et al. (2023). β-Secretase-1: in silico drug reposition for Alzheimer’s disease. Int. J. Mol. Sci. 24 (9), 8164. doi:10.3390/ijms24098164
Gaugler, J., James, B., Johnson, T., Marin, A., and Weuve, J. (2019). 2019 Alzheimer's disease facts and figures. Alzheimers dementia 15 (3), 321–387. doi:10.1016/j.jalz.2019.01.010
Gautam, V., Gaurav, A., Masand, N., Lee, V. S., and Patil, V. M. (2023). Artificial intelligence and machine-learning approaches in structure and ligand-based discovery of drugs affecting central nervous system. Mol. Divers 27 (2), 959–985. doi:10.1007/s11030-022-10489-3
Ghosh, A. K., Brindisi, M., and Tang, J. (2012). Developing β-secretase inhibitors for treatment of Alzheimer’s disease. J. Neurochem. 120, 71–83. doi:10.1111/j.1471-4159.2011.07476.x
Grant, B. J., Skjærven, L., and Yao, X. Q. J. P. S. (2021). The Bio3D packages for structural bioinformatics. , 30(1), 20–30. doi:10.1002/pro.3923
Gupta, S., Parihar, D., Shah, M., Yadav, S., Managori, H., Bhowmick, S., et al. (2020). Computational screening of promising beta-secretase 1 inhibitors through multi-step molecular docking and molecular dynamics simulations - pharmacoinformatics approach. J. Mol. Struct. 1205, 127660. doi:10.1016/j.molstruc.2019.127660
Hou, T., Wang, J., Zhang, W., Wang, W., and Xu, X. (2006). Recent advances in computational prediction of drug absorption and permeability in drug discovery. Curr. Med. Chem. 13 (22), 2653–2667. doi:10.2174/092986706778201558
Humphrey, W., Dalke, A., and Schulten, K. J. J. o. m. g. (1996). VMD: visual molecular dynamics. J. Mol. Graph. 14 (1), 33–38. doi:10.1016/0263-7855(96)00018-5
Hussain, I., Powell, D., Howlett, D. R., Tew, D. G., Meek, T. D., Chapman, C., et al. (1999). Identification of a novel aspartic protease (Asp 2) as β-secretase. Mol. Cell. Neurosci. 14 (6), 419–427. doi:10.1006/mcne.1999.0811
Ishola, A. A., Oyinloye, B. E., Ajiboye, B. O., and Kappo, A. P. (2021). Molecular docking studies of flavonoids from Andrographis paniculata as potential acetylcholinesterase, butyrylcholinesterase and monoamine oxidase inhibitors towards the treatment of neurodegenerative diseases. Biointerface Res. Appl. Chem. 11, 9871–9879.
Jorgensen, W., and Chandrasekhar, J. J. J. C. P. (1983). Comparison of simple potential functions for simulating liquid water. RW Impey, ML Klein 79, 926–935. doi:10.1063/1.445869
Kandalepas, P. C., and Vassar, R. (2012). Identification and biology of β-secretase. J. Neurochem. 120, 55–61. doi:10.1111/j.1471-4159.2011.07512.x
Köppen, H. (2009). Virtual screening-what does it give us? Curr. Opin. drug Discov. Dev. 12 (3), 397–407.
Kumar, A., and Zhang, K. Y. (2015). Hierarchical virtual screening approaches in small molecule drug discovery. Methods 71, 26–37. doi:10.1016/j.ymeth.2014.07.007
Kwak, Y.-D., Wang, R., Li, J. J., Zhang, Y.-W., Xu, H., and Liao, F.-F. (2011). Differential regulation of BACE1 expression by oxidative and nitrosative signals. Mol. Neurodegener. 6 (1), 17–10. doi:10.1186/1750-1326-6-17
Leung, C. H., and Ma, D. L. (2015). Recent advances in virtual screening for drug discovery. Methods 71, 1–3. doi:10.1016/j.ymeth.2014.12.012
Liu, H., Wang, C., Liu, P., Liu, C., Wang, Z., and Wei, Z. (2023). Efficient large-scale virtual screening based on heterogeneous many-core supercomputing system. IEEE J. Biomed. Health Inf. 27, 3579–3588. doi:10.1109/jbhi.2023.3272563
Madhavi Sastry, G., Adzhigirey, M., Day, T., Annabhimoju, R., and Sherman, W. J. J. o. c.-a. m. d. (2013). Protein and ligand preparation: parameters, protocols, and influence on virtual screening enrichments. J. Comput. Aided. Mol. Des. 27 (3), 221–234. doi:10.1007/s10822-013-9644-8
Marelli, C., Hourregue, C., Gutierrez, L.-A., Paquet, C., Menjot de Champfleur, N., De Verbizier, D., et al. (2020). Cerebrospinal fluid and plasma biomarkers do not differ in the presenile and late-onset behavioral variants of frontotemporal dementia. J. Alzheimer's Dis. 74 (3), 903–911. doi:10.3233/jad-190378
Martínez, L. J. P. o. (2015). Automatic identification of mobile and rigid substructures in molecular dynamics simulations and fractional structural fluctuation analysis. PLoS ONE 10 (3), e0119264. doi:10.1371/journal.pone.0119264
Matsuoka, M., Kumar, A., Muddassar, M., Matsuyama, A., Yoshida, M., and Zhang, K. Y. J.Modeling (2017). Discovery of fungal denitrification inhibitors by targeting copper nitrite reductase from Fusarium oxysporum. J. Chem. Inf. Model. 57 (2), 203–213. doi:10.1021/acs.jcim.6b00649
Muhammed, M. T., and Esin, A.-Y. (2021). Pharmacophore modeling in drug discovery: methodology and current status. J. Turkish Chem. Soc. Sect. A Chem. 8 (3), 749–762. doi:10.18596/jotcsa.927426
Neumann, U., Ufer, M., Jacobson, L. H., Rouzade-Dominguez, M., Huledal, G., Kolly, C., et al. (2018). The BACE-1 inhibitor CNP520 for prevention trials in Alzheimer’s disease. EMBO Mol. Med. 10 (11), e9316. doi:10.15252/emmm.201809316
Pautasso, C., Troia, R., Genuardi, M., and Palumbo, A. (2014). Pharmacophore modeling technique applied for the discovery of proteasome inhibitors. Expert Opin. Drug Discov. 9 (8), 931–943. doi:10.1517/17460441.2014.923838
Phillips, J. C., Hardy, D. J., Maia, J. D., Stone, J. E., Ribeiro, J. V., Bernardi, R. C., et al. (2020). Scalable molecular dynamics on CPU and GPU architectures with NAMD. J. Chem. Phys. 153 (4), 044130. doi:10.1063/5.0014475
Sabbah, D. A., and Zhong, H. A. (2016). Modeling the protonation states of β-secretase binding pocket by molecular dynamics simulations and docking studies. J. Mol. Graph. Model. 68, 206–215. doi:10.1016/j.jmgm.2016.07.005
Sadeer, N. B., Llorent-Martínez, E. J., Bene, K., Mahomoodally, M. F., Mollica, A., Sinan, K. I., et al. (2019) Chemical profiling, antioxidant, enzyme inhibitory and molecular modelling studies on the leaves and stem bark extracts of three African medicinal plants, 174, 19–33.
Sargsyan, K., Grauffel, C., and Lim, C. J. J. o. c. t.computation (2017). How molecular size impacts RMSD applications in molecular dynamics simulations. J. Chem. Theory Comput. 13 (4), 1518–1524. doi:10.1021/acs.jctc.7b00028
Selkoe, D. J., and Hardy, J. (2016). The amyloid hypothesis of Alzheimer's disease at 25 years. EMBO Mol. Med. 8 (6), 595–608. doi:10.15252/emmm.201606210
Shawky, A. M., Ibrahim, N. A., Abourehab, M. A. S., Abdalla, A. N., and Gouda, A. M. (2021). Pharmacophore-based virtual screening, synthesis, biological evaluation, and molecular docking study of novel pyrrolizines bearing urea/thiourea moieties with potential cytotoxicity and CDK inhibitory activities. J. enzyme inhibition Med. Chem. 36 (1), 15–33. doi:10.1080/14756366.2020.1837124
Shelley, J. C., Cholleti, A., Frye, L. L., Greenwood, J. R., Timlin, M. R., and Uchimaya, M. J. (2007). Epik: a software program for pK(a) prediction and protonation state generation for drug-like molecules, J. Comput. Aided. Mol. Des. 21, 681–691. doi:10.1007/s10822-007-9133-z
Shivakumar, D., Harder, E., Damm, W., Friesner, R. A., and Sherman, W. J. J. o. c. t.computation (2012). Improving the prediction of absolute solvation free energies using the next generation OPLS force field. J. Chem. Theory Comput. 8 (8), 2553–2558. doi:10.1021/ct300203w
Stamford, A., and Strickland, C. (2013). Inhibitors of BACE for treating Alzheimer's disease: a fragment-based drug discovery story. Curr. Opin. Chem. Biol., 17(3), 320–328. doi:10.1016/j.cbpa.2013.04.016
Stokes, J. M., Yang, K., Swanson, K., Jin, W., Cubillos-Ruiz, A., Donghia, N. M., et al. (2020). A deep learning approach to antibiotic discovery. Cell., 180(4), 688–702.e13. doi:10.1016/j.cell.2020.01.021
Thaisrivongs, D. A., Morris, W. J., Tan, L., Song, Z. J., Lyons, T. W., Waldman, J. H., et al. (2018). A next generation synthesis of BACE1 inhibitor verubecestat (MK-8931). Org. Lett. 20 (6), 1568–1571. doi:10.1021/acs.orglett.8b00259
Ugbaja, S. C., Sanusi, Z. K., Appiah-Kubi, P., Lawal, M. M., and Kumalo, H. M. (2021). Computational modelling of potent β-secretase (BACE1) inhibitors towards Alzheimer's disease treatment. Biophys. Chem. 270, 106536. doi:10.1016/j.bpc.2020.106536
Wang, M., Wang, Z., Sun, H., Wang, J., Shen, C., Weng, G., et al. (2022). Deep learning approaches for de novo drug design: an overview. Curr. Opin. Struct. Biol., 72, 135–144. doi:10.1016/j.sbi.2021.10.001
Wermuth, C. G. (2006). Pharmacophores: historical perspective and viewpoint from a medicinal chemist. Pharmacophores pharmacophore searches 32, 1–13. doi:10.1002/3527609164.ch1
Wilson, R. S., Segawa, E., Boyle, P. A., Anagnos, S. E., Hizel, L. P., and Bennett, D. A. (2012). The natural history of cognitive decline in Alzheimer's disease. Psychol. aging 27 (4), 1008–1017. doi:10.1037/a0029857
Yan, R., and Vassar, R. (2014). Targeting the β secretase BACE1 for Alzheimer's disease therapy. Lancet Neurology 13 (3), 319–329. doi:10.1016/s1474-4422(13)70276-x
Yusufzai, S. K., Khan, M. S., Sulaiman, O., Osman, H., and Lamjin, D. N. (2018). Molecular docking studies of coumarin hybrids as potential acetylcholinesterase, butyrylcholinesterase, monoamine oxidase A/B and β-amyloid inhibitors for Alzheimer’s disease. Chem. Cent. J. 12 (1), 128. doi:10.1186/s13065-018-0497-z
Keywords: pharmacophore-based virtual screening, β-secretase 1, Alzheimer’s disease, databases, crystal structure
Citation: Han X and Guo K (2024) Pharmacophore-based virtual screening of commercial databases against β-secretase 1 for drug development against Alzheimer’s disease. Front. Chem. 12:1412349. doi: 10.3389/fchem.2024.1412349
Received: 04 April 2024; Accepted: 11 June 2024;
Published: 09 July 2024.
Edited by:
Ahmed A. Al-Karmalawy, Ahram Canadian University, EgyptReviewed by:
Shikha Kumari, Virginia Tech, United StatesAyman Abo Elmaaty, Port Said University, Egypt
Copyright © 2024 Han and Guo. This is an open-access article distributed under the terms of the Creative Commons Attribution License (CC BY). The use, distribution or reproduction in other forums is permitted, provided the original author(s) and the copyright owner(s) are credited and that the original publication in this journal is cited, in accordance with accepted academic practice. No use, distribution or reproduction is permitted which does not comply with these terms.
*Correspondence: Kaibo Guo, a2Fib2d1b0BvdXRsb29rLmNvbQ==