- 1Department of Environmental Science and Engineering, School of Energy and Environment, Southeast University, Nanjing, China
- 2State Environmental Protection Key Laboratory of Soil Environmental Management and Pollution Control, Nanjing Institute of Environmental Sciences, Ministry of Ecology and Environment, Nanjing, China
- 3Geo-engineering Investigation Institute of Jiangsu Province, Nanjing, China
- 4Nanjing Research Institute of Environmental Protection, Nanjing Environmental Protection Bureau, Nanjing, China
Due to the complicated transport and reactive behavior of organic contamination in groundwater, the development of mathematical models to aid field remediation planning and implementation attracts increasing attentions. In this study, the approach coupling response surface methodology (RSM), artificial neural networks (ANN), and kinetic models was implemented to model the degradation effects of nano-zero-valent iron (nZVI) activated persulfate (PS) systems on benzene, a common organic pollutant in groundwater. The proposed model was applied to optimize the process parameters in order to help predict the effects of multiple factors on benzene degradation rate. Meanwhile, the chemical oxidation kinetics was developed based on batch experiments under the optimized reaction conditions to predict the temporal degradation of benzene. The results indicated that benzene (0.25 mmol) would be theoretically completely oxidized in 1.45 mM PS with the PS/nZVI molar ratio of 4:1 at pH 3.9°C and 21.9 C. The RSM model predicted well the effects of the four factors on benzene degradation rate (R2 = 0.948), and the ANN with a hidden layer structure of [8-8] performed better compared to the RSM (R2 = 0.980). In addition, the involved benzene degradation systems fit well with the Type-2 and Type-3 pseudo-second order (PSO) kinetic models with R2 > 0.999. It suggested that the proposed statistical and kinetic-based modeling approach is promising support for predicting the chemical oxidation performance of organic contaminants in groundwater under the influence of multiple factors.
1 Introduction
The widespread use of organics in industrial production and the lack of management of organic wastewater leakage and discharge have led to an increasing threat of organic contaminants to the public safety of subsurface ecosystems (Padhi and Gokhale, 2017). Benzene, as an important aromatic compound existing in pesticide intermediates and petroleum products, is widely used as an organic solvent in the industry (Liu et al., 2010; Zhao et al., 2020). Benzene has the potential to readily infiltrate the subsurface milieu during various stages of its lifecycle, encompassing production, storage, and transportation. Its inclusion in the U.S. EPA’s National Priorities List is attributed to its well-documented carcinogenic, teratogenic, and mutagenic properties, which are observed in both its liquid and gaseous states (Xu Q. et al., 2021; Agency, 2023). Subsurface environmental exposure to benzene has become a global environmental problem (Singh and Fulekar, 2010; Padhi and Gokhale, 2014). Therefore, the rapid and precise remediation of benzene-contaminated groundwater to reduce public risk has become a widespread concern.
Among the many remediation strategies for organic compound contaminated groundwater, in situ chemical oxidation (ISCO) has attracted much attention for its advantages of economy and high efficiency (Matzek and Carter, 2016). At present, the sulfate radical-based advanced oxidation processes (
The traditional one-factor-at-a-time (OFAT) approach for process parameter optimization necessitates an excessive amount of time and runs to ensure precision in effect estimation, and its ability to determine the interaction between input variables and the optimal levels of various factors is limited (Sachaniya et al., 2020). The process-centered, statistics-based response surface methodology (RSM) overcomes these disadvantages. RSM is a statistical modeling method that uses multiple quadratic regression equations to fit the global functional relationship between the factors and the response values through reasonable experimental design (Amiri et al., 2019; Bahrami et al., 2019). By analyzing the response surface contour plot, the interactions among the process parameters and contaminant degradation rate in the PS/nZVI oxidation system of organic contamination in groundwater would be determined, and the optimal factor value could be predicted by regression equation (Kasiri et al., 2008). The commonly used RSM design methods include Box-Benkhen design (BBD) and Center Composite Design (CCD). It is generally believed that CCD was suitable for situations with multi-factor and continuous variables, and when the number of variables is small (three to seven variables), and BBD could reveal the interaction of multiple factors affecting the remediation effect by using fewer experimental groups than CCD (Ray et al., 2009).
However, the quadratic regression modeling, frequently employed in RSM, may prove inadequate for capturing the intricate relationships between these factors and the responses. Integrating Artificial neural network (ANN) as a modeling tool alongside RSM may further enrich our understanding of the intricate connections between inputs (characteristic factors) and outputs (degradation rate). ANN has garnered extensive utilization in diverse scientific and engineering domains for simulation and prediction purposes (Aycan DÜMencİ et al., 2021). Its ability to adapt, learn, identify, verify, and reproduce associations enables ANN to effectively interpret the interaction of highly complex factors in the remediation process of contaminated groundwater, and to simulate and predict degradation data through the analysis of characteristic parameters (Zafar et al., 2012). Currently, ANN has demonstrated its efficacy in tackling numerous challenging issues within the realm of environmental remediation, such as the prediction of the elimination of hazardous compounds from industrial wastewater and the precise management of diverse degradation processes associated with organic contaminants (Lenzi et al., 2016; Srivastava et al., 2021a).
Chemical degradation kinetic models play a vital role in providing insight into transient degradation rates and comprehending the chemical oxidation process, thereby furnishing crucial information for simulating site-specific contaminant degradation on a larger scale (Lominchar et al., 2018). At present, the pseudo-first-order kinetic model (PFO), pseudo-second-order kinetic model (PSO), and intraparticle diffusion kinetic model (IPD, W-M equation) have been demonstrated as the simplified mathematical models for simulating advanced oxidation process (Zulfiqar et al., 2019). Therefore, combining the chemical degradation kinetic model with the RSM-ANN approach may provide a deeper insight into the transient degradation processes within the system, thus furnishing a more realistic framework to guide practical applications. Currently, the RSM-ANN-kinetic approach has been successfully applied in the areas such as optimizing wastewater treatment processes (Nayak and Pal, 2020; Igwegbe et al., 2023) and evaluating catalyst performance (Fattahi et al., 2014; Kassahun et al., 2021).
Many studies have been carried out in modeling and optimizing the degradation of organic contaminants through activated PS oxidation techniques using kinetic models based on OFAT (Cabrera-Reina et al., 2020; Heidari et al., 2022; Conte et al., 2023). Other studies focused on modeling and optimizing the pollutant degradation process via activated PS using the combined approach of RSM and ANN (Zhang et al., 2018; Asgari et al., 2020; Qiu et al., 2021). However, few studies have systematically explored the effectiveness of the coupled RSM-ANN-kinetic approach in predicting the oxidation of organic contaminants in groundwater by activated PS.
In this study, the modeling approach coupling two optimization systems namely, RSM-BDD and ANN with kinetic model was innovatively implemented to optimize the benzene degradation process parameters and predict the effect of PS/nZVI oxidation system with benzene. The RSM models of four independent parameters, including PS dosage, nZVI dosage, pH, and temperature were established based on batch experiments. The simulation accuracy of the RSM models was optimized using ANN to enhance the prediction accuracy of the advanced oxidation degradation models based on statistical data. In addition, the kinetic models suitable for predicting the degradation process of benzene-contaminated groundwater by the PS/nZVI oxidation system were developed.
2 Materials and methods
2.1 Field sample
The soil and groundwater samples used in this study were collected from an abandoned pesticide factory in Jiangsu Province, China. Benzene, toluene, ethylbenzene, and xylene (BTEX) was found to contaminate the core area of the site. The soils used in this work were taken from the non-polluted area at the edge of the site, and the uncontaminated groundwater was obtained from the upstream of the contaminated site. The soil samples were dried (for a week) in a greenhouse before being screened, and collected with a particle size of less than 2 mm for later use.
2.2 Microcosm experiment setup
Benzene was added to the collected uncontaminated groundwater to a final concentration of 0.25 mM (equivalent to 20 mg/L). The groundwater was then aliquoted to the standard 40 mL threaded vials filled with the pre-prepared soil and capped with a Teflon/silicone gasket to prevent the volatilization of contaminants. PS (purity >98%, Sinopharm Chemical Reagent Co., Ltd., China) and nZVI (Sinopharm Chemical Reagent Co., Ltd., China) were added to the above vials according to experimentally designed concentrations of RSM-BBD and sodium hydroxide solution (0.1 M) and hydrochloric acid solution (0.1 M) were used for pH adjustment. All the microcosmic vials were kept for 12 h at the design temperature after uniform oscillation. Then the sample were filtered through a 0.45 μm membranes before analysis. The residual benzene concentration analysis was conducted using a gas chromatography-mass spectrometrometry system (GC-MS, 7890A/5975C, Agilent, United States) equipped with a capillary column (J&W Scientific DB-624 60 m × 0.25 mm × 1.4 μm, Agilent) after a purge and trap concentrator (Eclipse 4,552and4,660, OI Analytical, United States). Three parallel experiments were set in each group to eliminate experimental errors and determine the reproducibility of the results, and one blank control group was set without degradant to eliminate interference caused by adsorption. The removal rate of benzene was calculated by the following formula:
2.3 Experimental designs with RSM method
Design Expert software (DES, Version 11.0) was used to analyze the influence of different environmental parameters on the degradation rate of benzene (response) in contaminated groundwater. A four-variable with three-level BBD experiments with three central points were designed to investigate the degradation trend of benzene in contaminated groundwater in the PS/nZVI oxidation system. Four independent variables were selected, including 1) oxidant (PS) dose, 2) activator (nZVI) dose, 3) pH, and 4) temperature, with three levels designed for each independent variable (coded values + 1, 0, and −1, see Table 1). The BBD-RSM method was used to design 29 sets of experiments under different process conditions (Table 2). These 4 independent factors were presented as
In the formula, Y is the response factor predicting the degradation efficiency of benzene,
2.4 ANN design
The learning network used in this research was a hierarchical feedforward neural network with a back-propagation method, also known as an improved BP learning algorithm. Levenberg-Marquardt (LM) algorithm was selected for supervised learning functions to train the network because of its advantages of fast convergence and high computational accuracy (Srivastava et al., 2021a). The four variables determined by the response surface method were used as input layer neurons (04), and output layer neuron (01) was set as the response of benzene degradation rate. This research adopted the double-layer structure network with better generalization ability than the single-hidden layer structure which optimized the network structure of the hidden layer (Figure 1). Estimation of the number of hidden layers and the number of neurons in each layer was the main difficulties in optimizing the structure of the neural network, this problem was solved by applying the thumb rule (Gazzaz et al., 2012). In all sample datasets, 70% were used for training the network learning, 15% were used for forming the verification set, and the other 15% were used for forming the network test set (Taqvi et al., 2017), to cross-validate the ANN model for benzene degradation by the PS/nZVI oxidation system. The specific model parameters in the ANN were shown in Table 3. The scenario settings used to optimize the hidden layer structure of the ANN model were shown in Table 4. The development of the ANN model was completed by MATLAB 9.8.0.1323502 (R2020A).
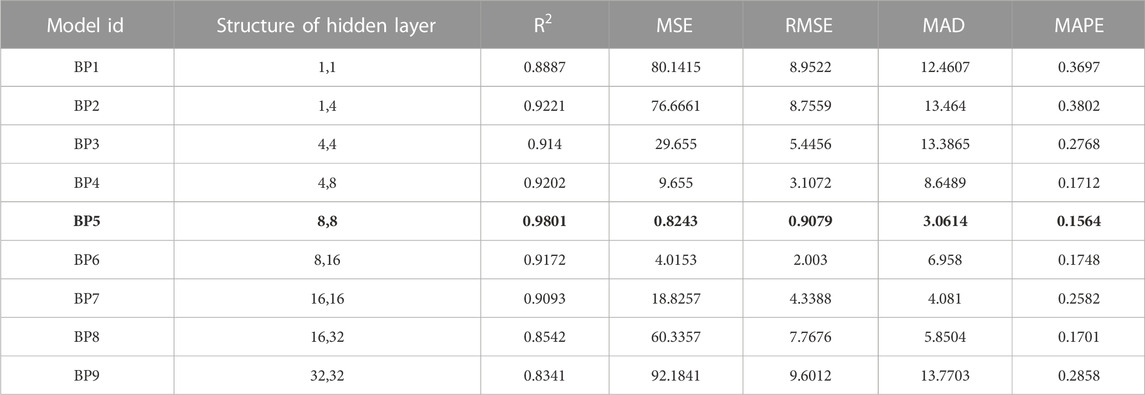
TABLE 4. Performance of ANN models with different structure of hidden layer (Bold values represent the optimal hidden layer structure).
The prediction performance of the models was evaluated via error functions that include the coefficient of determination (
Where
2.5 Kinetic model study
Under the optimum conditions given by RSM and ANN, the degradation kinetics of benzene with different concentrations (0.0625–1.25 mM) by the PS/nZVI oxidation system was studied. Degradation experiments were carried out on benzene solutions of specified concentrations based on optimized process parameters, and samples were collected at 20-min intervals to measure the residual benzene concentration in the system. The degradation results of benzene in the optimized experiment were modeled using the Pseudo-first order (PFO) and Pseudo-second order (PSO) kinetic models, which were widely used in the analysis of the degradation mechanism (Chowdhury et al., 2016). The diffusion mechanism of the degradation system was studied by the intraparticle diffusion (IPD) kinetic model (Zulfiqar et al., 2019).
i. PFO kinetic model
PFO kinetic model is an extensively adopted model to simulate the degradation process, which could be represented as follows:
In the formula,
ii. PSO kinetic model
Five different forms of PSO kinetic models were used to fit the degradation rate results of benzene, namely,: Type-1 PSO, Type-2 PSO, Type-3 PSO, Type-4 PSO and Type-5 PSO. These Lagergren equations are extensively used for the degradation of liquid-solid phase based on solid capacity, and these PSO kinetic formulas could be expressed as following equations (Zulfiqar et al., 2018):
Where
iii. IPD kinetic model
Another kinetic theory considered the controlling factor of the reaction rate of the PS/nZVI oxidation system from the perspective of solid-liquid mass transfer. The degradation process of the benzene molecules in the system could be summarized into three steps: 1) the mass transfer of the pollutant through the outer boundary layer at the solid-liquid interface; 2) the diffusion of the liquid through the solid-phase particle channels; and 3) the oxidative degradation of the
Where
3 Results and discussion
3.1 Statistical analysis for BBD-RSM
The BBD-RSM successfully simulated and predicted the PS/nZVI oxidation of benzene in synthetic contaminated groundwater at each design level of the four factors. The benzene degradation rate was between 53.52% and 98.29% in the batch experiments (Table 2). The ANOVA of BBD-RSM for benzene degradation with the PS/nZVI technology showed that the predicted degradation rate of the quadratic model was highly consistent (p < 0.0001) with the actual degradation rate (Supplementary Table S1).
The value of determination coefficient R2 (0.948) indicated that the suggested quadratic equation was useful for predicting the benzene degradation rate in the PS/nZVI oxidation system within the range of experimental conditions. The R2-adj value (0.895) of the RSM model was close enough to the R2 value (0.948), indicating that the precision of the suggested response model was only slightly affected by the insignificant model terms. In general, R2 values would increase by adding an item which has insignificant effects (p-value>0.05) to the proposed model, but the adjusted R2 (R2-adj) representing significant effects would not increase (Salarian et al., 2016). In addition, the predicted R2 (0.792) also has favorable anastomose with the R2-adj (0.895) (a difference of less than 0.2 was considered acceptable), further indicating that the constructed BBD-RSM model had acceptable reproducibility.
According to the screening principle of the significance of RSM model variables (p-value <0.05 and F value >5), independent variables
The prediction accuracy of the proposed model for the degradation of benzene by the PS/nZVI oxidation system was verified using four different evaluation methods. The measured value and predicted value of the degradation rate of benzene showed a high degree of consistency (Supplementary Figure S1A), which provided the most direct evidence for the great performance of the prediction model. The externally studentized residual analysis showed that the error values were normally distributed along a mathematical expectation value μ) close to zero and a constant variance (
The factor interaction analysis of the response surface methodology showed that the interaction between the PS and nZVI dosages exerted the dominant effects on the benzene degradation in synthetic contaminated groundwater (Figure 2A). In the response surface of the interaction between PS and nZVI dosages, the degradation rate of benzene showed a drastic increase with the dosage of PS increasing from 0.25 mM to 1.25 mM (53.52%–98.29%), while the effect of the activator on the reaction result was more moderate. However, when the amount of activator alone was controlled to increase from a 2:1 M ratio to 6:1, a 15% increase in benzene degradation rate was also observed (Figure 2A). This may owe to the
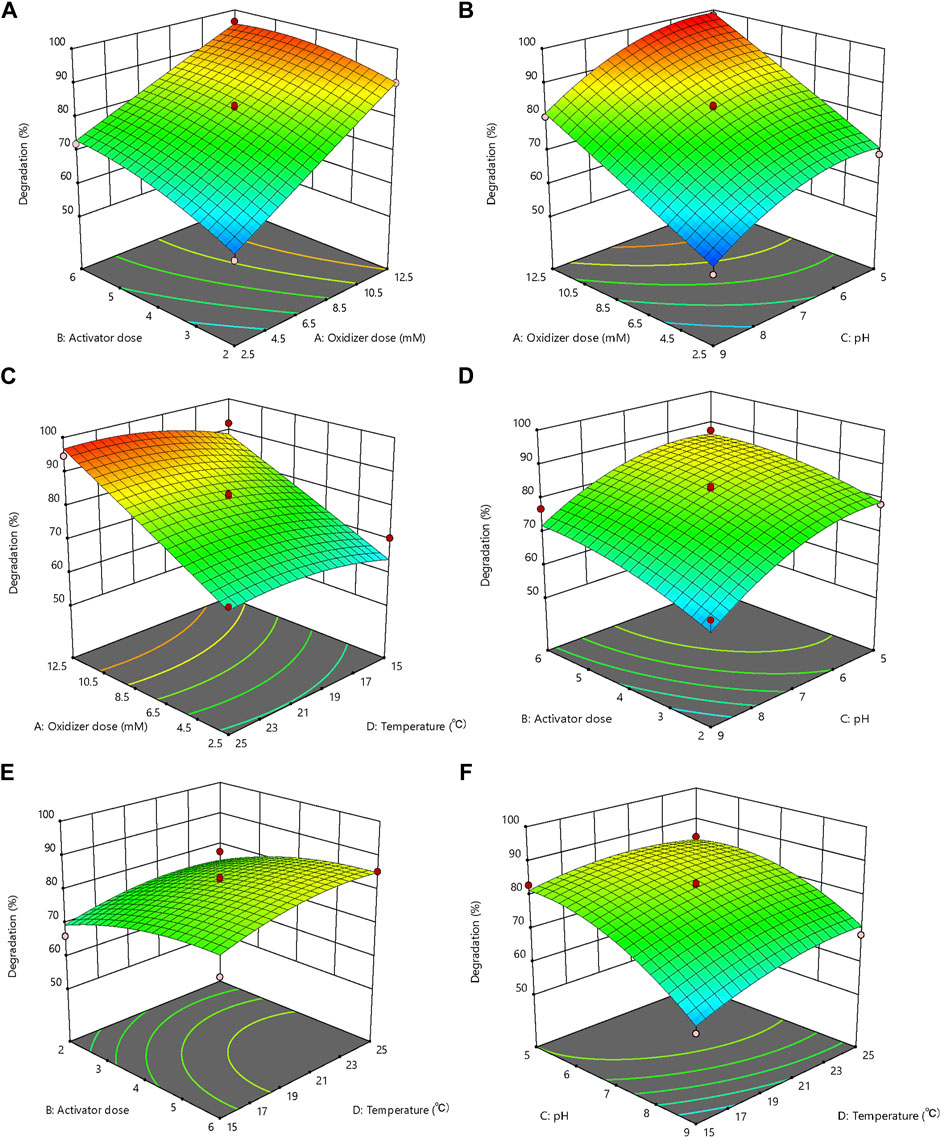
FIGURE 2. The response surface plot of benzene degradation as the function of (A) oxidizer and activator dosage, (B) pH and oxidizer dosage, (C) oxidizer dosage and temperature, (D) activator dosage and pH, (E) activator dosage and temperature, and (F) temperature and pH.
The response surface of the interaction between the PS dosage and pH showed that the degradation rate of benzene decreased with the transition from the acidic to the alkaline solution at a constant PS dosage (Figure 2B). Although both
3.2 Development of ANN model
The optimization results of the topological structure of the ANN model showed that when both hidden layers contain eight neurons, the evaluation function MSE, RMSE, MAD, and MAPE have the smallest values (Table 4, Scenario BP5), which represents a perfect match between the predicted value and the actual value. However, if the number of neurons in any hidden layer exceeds 8, the error of the ANN model increased instead. It was speculated that too many neurons in the hidden layer may lead to overfitting, increase the error of the test set and further increase the overall error function (Mutasa et al., 2020). Therefore, the optimal topology structure of the ANN model for the PS/nZVI oxidation of benzene was [4-8-8-1], in which case the lowest performance evaluation functions value and the highest
The simulation of benzene degradation rates using the ANN model structured in scenario BP5 showed high goodness of fit for training, validation, and test subset (Figures 3A–C), with the
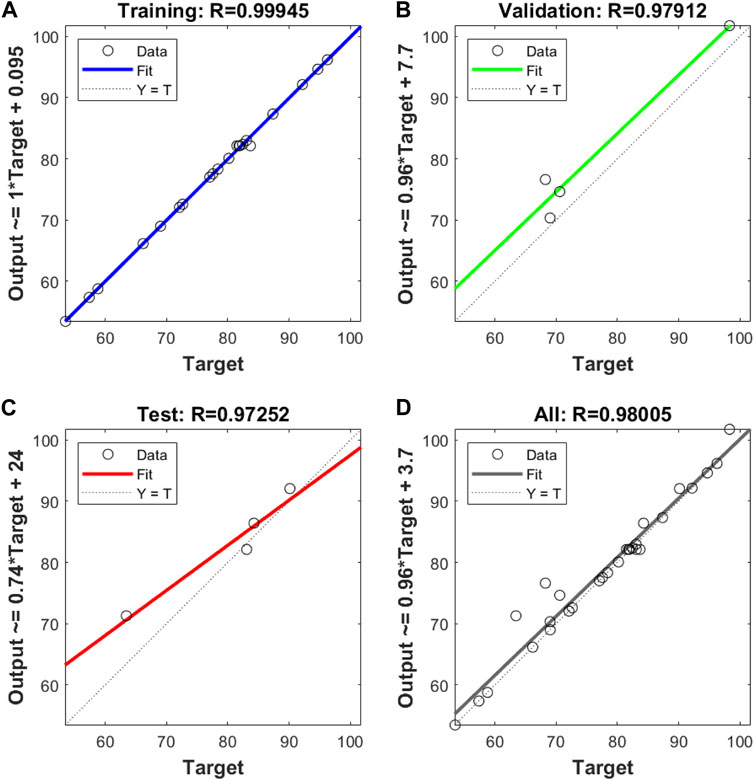
FIGURE 3.
3.3 Comprehensive evaluation comparison between RSM and ANN models
The empirical modeling tool ANN displayed better prediction ability than RSM in the simulation and prediction of the PS/nZVI oxidation process of benzene in contaminated groundwater (Figure 4). We compared the observed values with the predicted values of the quadratic model obtained by RSM and the trained ANN model, respectively. Their predicted performance parameters were analyzed and the deviation of the calculated values from the actual values of the two models was plotted. Compared with the RSM, the predicted value distribution of ANN was closer to the actual value. The evaluation function values for ANN were lower, and the values of the
3.4 External validation of the model
Three groups of process parameters recommended by the proposed models that should completely degrade benzene in synthetic contaminated groundwater were selected and the predictive ability of the proposed models was externally validated by replicated experiments (Supplementary Table S3). The results showed that under the condition of complete degradation of benzene predicted by the RSM model, the measured degradation rates of the three experimental groups were 100%, 99.87%, and 98.79%, respectively, and the predicted benzene degradation rates by the trained ANN (BP5, which with the best predictive performance) were 99.86%, 99.98%, and 99.10%, respectively. The agreement between the experimental and predicted values indicated that it was feasible to use the proposed model to simulate the actual degradation situation. The MSE between the predicted and measured values of the two models were 0.4937 (RSM) and 0.0426 (ANN), respectively, which indicated that the simulation errors of both models were acceptable in the external validation phase, but ANN still outperformed RSM.
3.5 Reaction kinetic studies
The kinetic parameters (
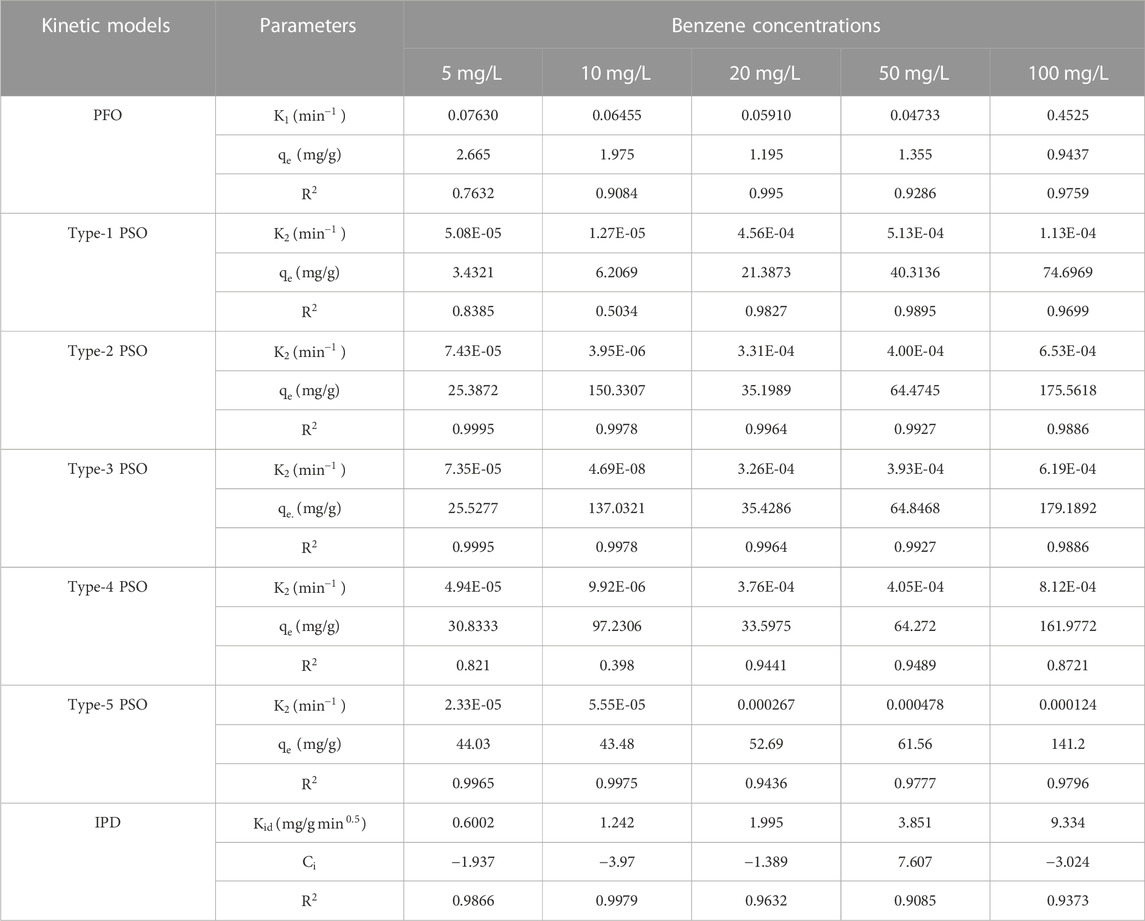
TABLE 5. PFO, Type-1 PSO, Type-2 PSO, Type-3 PSO, Type-4 PSO, Type-5 PSO, and IPD kinetic parameters for benzene in groundwater degradation onto PS/nZVI process.
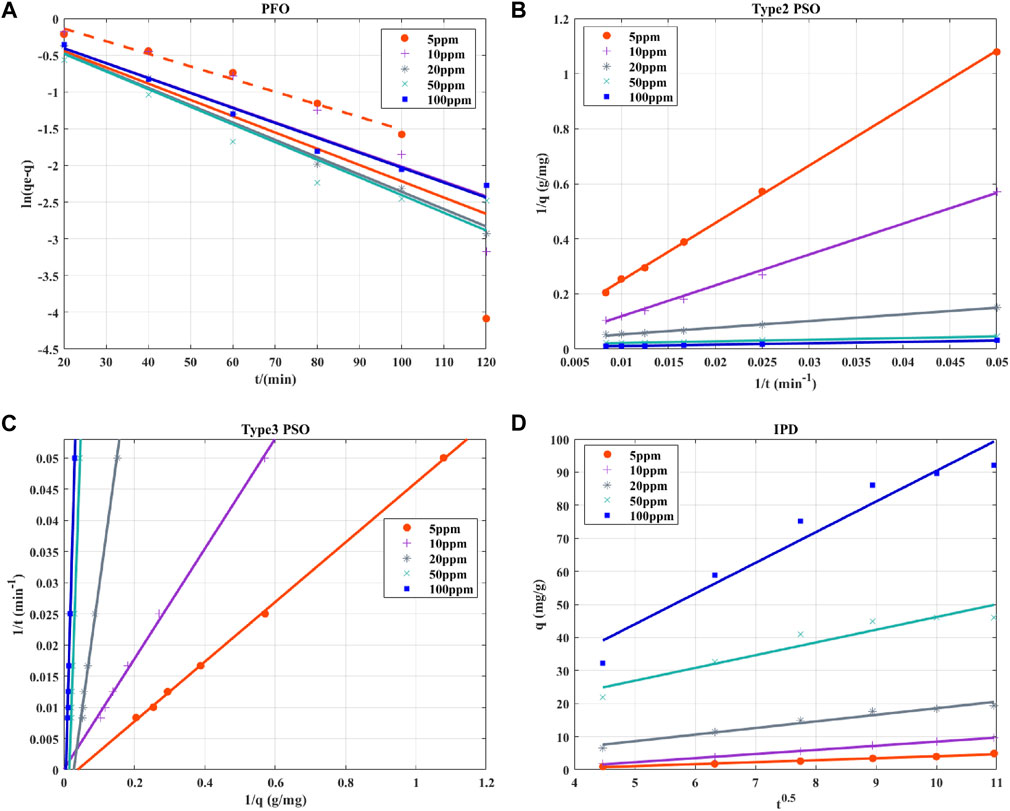
FIGURE 5. (A) PFO, (B) Type-2 PSO, (C) Type-3 PSO and (4) IPD kinetic models for benzene removal at optimized conditions given by suggested model.
In the IPD kinetic model, as the initial benzene concentration increased from 0.0625 mM, the value of diffusivity constant
4 Conclusion
In this work, the modeling approach coupling BBD-RSM and modified ANN with kinetic model was implemented to optimize the benzene degradation process parameters and predict the effect of PS/nZVI oxidation system. The results indicated that the modeled optimum levels of variables were 1.45 mM PS with a Na+/nZVI molar ratio of 4:1 at pH 3.9°C and 21.9°C, under which 0.25 mM of benzene would theoretically be completely removed. The ANN model had better prediction performance compared with RSM, due to its strong nonlinear fitting ability. The structure of the hidden layer and the number of neurons contained in each layer significantly affected the predictive performance of the ANN. By employing the thumb rule during the test, we found that when the structure of hidden layers was [8-8], the evaluation indices of the ANN reached the optimal level. Furthermore, the developed degradation reaction kinetics showed that Type-2 and Type-3 PSO kinetic models were more suitable to explain the benzene degradation process by the PS/nZVI oxidation system. Our study is expected to provide a new approach for modeling and optimization of chemical oxidative remediation of organic contamination in groundwater.
Data availability statement
The original contributions presented in the study are included in the article/Supplementary Material, further inquiries can be directed to the corresponding authors.
Author contributions
ML: Conceptualization, Methodology, Resources, Writing–original draft. XZ: Methodology, Writing–review and editing. TL: Funding acquisition, Writing–review and editing. SC: Methodology, Writing–review and editing. MZ: Validation, Writing–review and editing. XZ: Conceptualization, Funding acquisition, Methodology, Writing–review and editing. RY: Funding acquisition, Writing–review and editing.
Funding
The author(s) declare financial support was received for the research, authorship, and/or publication of this article. This work was supported by the National Key R&D Program of China (grant number 2018YFC1803100), the National Natural Science Foundation of China (grant number 52270119), and Foundation Program of Jiangsu Geological Engineering Survey Institute [grant number KY-202205]. Program of Nanjing Institute of Environmental Sciences, Ministry of Ecology and Environment: Integration of Ecological and Environmental Protection in Yangtze River Delta [grant number ZX2022QT047].
Conflict of interest
The authors declare that the research was conducted in the absence of any commercial or financial relationships that could be construed as a potential conflict of interest.
Publisher’s note
All claims expressed in this article are solely those of the authors and do not necessarily represent those of their affiliated organizations, or those of the publisher, the editors and the reviewers. Any product that may be evaluated in this article, or claim that may be made by its manufacturer, is not guaranteed or endorsed by the publisher.
Supplementary material
The Supplementary Material for this article can be found online at: https://www.frontiersin.org/articles/10.3389/fchem.2023.1270730/full#supplementary-material
References
Abdelwahab, O., and Amin, N. K. (2013). Adsorption of phenol from aqueous solutions by Luffa cylindrica fibers: kinetics, isotherm and thermodynamic studies. Egypt. J. Aquatic Res. 39 (4), 215–223. doi:10.1016/j.ejar.2013.12.011
Amiri, M. J., Bahrami, M., and Dehkhodaie, F. (2019). Optimization of Hg(II) adsorption on bio-apatite based materials using CCD-RSM design: characterization and mechanism studies. J. Water Health 17 (4), 556–567. doi:10.2166/wh.2019.039
Asgari, G., Shabanloo, A., Salari, M., and Eslami, F. (2020). Sonophotocatalytic treatment of AB113 dye and real textile wastewater using ZnO/persulfate: modeling by response surface methodology and artificial neural network. Environ. Res. 184, 109367. doi:10.1016/j.envres.2020.109367
Aycan Dümencİ, N., Cagcag Yolcu, O., Aydin Temel, F., and Turan, N. G. (2021). Identifying the maturity of co-compost of olive mill waste and natural mineral materials: modelling via ANN and multi-objective optimization. Bioresour. Technol. 338, 125516. doi:10.1016/j.biortech.2021.125516
Bahrami, M., Amiri, M. J., and Bagheri, F. (2019). Optimization of the lead removal from aqueous solution using two starch based adsorbents: design of experiments using response surface methodology (RSM). J. Environ. Chem. Eng. 7 (1), 102793. doi:10.1016/j.jece.2018.11.038
Cabrera-Reina, A., Miralles-Cuevas, S., Oller, I., Sánchez-Pérez, J. A., and Malato, S. (2020). Modeling persulfate activation by iron and heat for the removal of contaminants of emerging concern using carbamazepine as model pollutant. Chem. Eng. J. 389, 124445. doi:10.1016/j.cej.2020.124445
Cheung, W. H., Szeto, Y. S., and McKay, G. (2007). Intraparticle diffusion processes during acid dye adsorption onto chitosan. Bioresour. Technol. 98 (15), 2897–2904. doi:10.1016/j.biortech.2006.09.045
Chowdhury, S., Mandal, P. C., Zulfiqar, M., and Subbarao, D. (2016). “Development of ionothermal synthesis of titania nanomaterial for waste-water treatment,” in Advanced materials research (Transactions Technical Publication), 537–541.
Conte, L. O., Legnettino, G., Lorenzo, D., Cotillas, S., Prisciandaro, M., and Santos, A. (2023). Degradation of Lindane by persulfate/ferrioxalate/solar light process: influential operating parameters, kinetic model and by-products. Appl. Catal. B-Environmental 324, 122288. doi:10.1016/j.apcatb.2022.122288
Fattahi, M., Kazemeini, M., Khorasheh, F., and Rashidi, A. (2014). Kinetic modeling of oxidative dehydrogenation of propane (ODHP) over a vanadium-graphene catalyst: application of the DOE and ANN methodologies. J. Industrial Eng. Chem. 20 (4), 2236–2247. doi:10.1016/j.jiec.2013.09.056
Gazzaz, N. M., Yusoff, M. K., Aris, A. Z., Juahir, H., and Ramli, M. F. (2012). Artificial neural network modeling of the water quality index for Kinta River (Malaysia) using water quality variables as predictors. Mar. Pollut. Bull. 64 (11), 2409–2420. doi:10.1016/j.marpolbul.2012.08.005
Heidari, M., Sadeghi, M., Arbabi, M., Mardani, G., Sedehi, M., and Emadi, Z. (2022). Combination of nonionic surfactants with thermal-activated persulfate for simultaneous removal of phenanthrene and pyrene from alluvial soil: a kinetic study. Soil and Sediment Contam. 31 (4), 468–482. doi:10.1080/15320383.2021.1963668
Igwegbe, C. A., Obi, C. C., Ohale, P. E., Ahmadi, S., Onukwuli, O. D., Nwabanne, J. T., et al. (2023). Modelling and optimisation of electrocoagulation/flocculation recovery of effluent from land-based aquaculture by artificial intelligence (AI) approaches. Environ. Sci. Pollut. Res. 30 (27), 70897–70917. doi:10.1007/s11356-023-27387-2
Jiang, N., Zhao, Y., Shang, K., Lu, N., Li, J., and Wu, Y. (2020). Degradation of toluene by pulse-modulated multistage DBD plasma: Key parameters optimization through response surface methodology (RSM) and degradation pathway analysis. J. Hazard. Mater. 393, 122365. doi:10.1016/j.jhazmat.2020.122365
Kasiri, M. B., Aleboyeh, H., and Aleboyeh, A. (2008). Modeling and optimization of heterogeneous photo-fenton process with response surface methodology and artificial neural networks. Environ. Sci. Technol. 42 (21), 7970–7975. doi:10.1021/es801372q
Kassahun, S. K., Kiflie, Z., Kim, H., and Baye, A. F. (2021). Process optimization and kinetics analysis for photocatalytic degradation of emerging contaminant using N-doped TiO2-SiO2 nanoparticle: artificial Neural Network and Surface Response Methodology approach. Environ. Technol. Innovation 23, 101761. doi:10.1016/j.eti.2021.101761
Lenzi, G. G., Evangelista, R. F., Duarte, E. R., Colpini, L. M. S., Fornari, A. C., Menechini Neto, R., et al. (2016). Photocatalytic degradation of textile reactive dye using artificial neural network modeling approach. Desalination Water Treat. 57 (30), 14132–14144. doi:10.1080/19443994.2015.1064035
Liang, C., Huang, C. F., and Chen, Y. J. (2008). Potential for activated persulfate degradation of BTEX contamination. Water Res. 42 (15), 4091–4100. doi:10.1016/j.watres.2008.06.022
Liu, J.-H., Maity, J. P., Jean, J.-S., Chen, C.-Y., Chen, C.-C., and Ho, S.-Y. (2010). Biodegradation of benzene by pure and mixed cultures of Bacillus spp. World J. Microbiol. Biotechnol. 26 (9), 1557–1567. doi:10.1007/s11274-010-0331-9
Lominchar, M. A., Rodriguez, S., Lorenzo, D., Santos, N., Romero, A., and Santos, A. (2018). Phenol abatement using persulfate activated by nZVI, H2O2 and NaOH and development of a kinetic model for alkaline activation. Environ. Technol. 39 (1), 35–43. doi:10.1080/09593330.2017.1294203
Lopez, M. E., Rene, E. R., Boger, Z., Veiga, M. C., and Kennes, C. (2017). Modelling the removal of volatile pollutants under transient conditions in a two-stage bioreactor using artificial neural networks. J. Hazard Mater 324, 100–109. doi:10.1016/j.jhazmat.2016.03.018
Matzek, L. W., and Carter, K. E. (2016). Activated persulfate for organic chemical degradation: a review. Chemosphere 151, 178–188. doi:10.1016/j.chemosphere.2016.02.055
Mutasa, S., Sun, S., and Ha, R. (2020). Understanding artificial intelligence based radiology studies: what is overfitting? Clin. Imaging 65, 96–99. doi:10.1016/j.clinimag.2020.04.025
Nayak, A. K., and Pal, A. (2020). Statistical modeling and performance evaluation of biosorptive removal of Nile blue A by lignocellulosic agricultural waste under the application of high-strength dye concentrations. J. Environ. Chem. Eng. 8 (2), 103677. doi:10.1016/j.jece.2020.103677
Ocampo-Perez, R., Leyva-Ramos, R., Mendoza-Barron, J., and Guerrero-Coronado, R. M. (2011). Adsorption rate of phenol from aqueous solution onto organobentonite: surface diffusion and kinetic models. J. Colloid Interface Sci. 364 (1), 195–204. doi:10.1016/j.jcis.2011.08.032
Padhi, S. K., and Gokhale, S. (2017). Benzene biodegradation by indigenous mixed microbial culture: kinetic modeling and process optimization. Int. Biodeterior. Biodegrad. 119, 511–519. doi:10.1016/j.ibiod.2016.10.011
Padhi, S. K., and Gokhale, S. (2014). Biological oxidation of gaseous VOCs – rotating biological contactor a promising and eco-friendly technique. J. Environ. Chem. Eng. 2 (4), 2085–2102. doi:10.1016/j.jece.2014.09.005
Qiu, Y., Zhang, Q., Wang, Z. H., Gao, B., Fan, Z. X., Li, M., et al. (2021). Degradation of anthraquinone dye reactive blue 19 using persulfate activated with Fe/Mn modified biochar: radical/non-radical mechanisms and fixed-bed reactor study. Sci. Total Environ. 758, 143584. doi:10.1016/j.scitotenv.2020.143584
Ray, S., Lalman, J. A., and Biswas, N. (2009). Using the Box-Benkhen technique to statistically model phenol photocatalytic degradation by titanium dioxide nanoparticles. Chem. Eng. J. 150 (1), 15–24. doi:10.1016/j.cej.2008.11.039
Rodriguez, S., Vasquez, L., Costa, D., Romero, A., and Santos, A. (2014). Oxidation of Orange G by persulfate activated by Fe(II), Fe(III) and zero valent iron (ZVI). Chemosphere 101, 86–92. doi:10.1016/j.chemosphere.2013.12.037
Sachaniya, B. K., Gosai, H. B., Panseriya, H. Z., and Dave, B. P. (2020). Bioengineering for multiple PAHs degradation for contaminated sediments: response surface methodology (RSM) and artificial neural network (ANN). Chemom. Intelligent Laboratory Syst. 202, 104033. doi:10.1016/j.chemolab.2020.104033
Salarian, A.-A., Hami, Z., Mirzaei, N., Mohseni, S. M., Asadi, A., Bahrami, H., et al. (2016). N-doped TiO2 nanosheets for photocatalytic degradation and mineralization of diazinon under simulated solar irradiation: optimization and modeling using a response surface methodology. J. Mol. Liq. 220, 183–191. doi:10.1016/j.molliq.2016.04.060
Shuchi, S. B., Suhan, M. B. K., Humayun, S. B., Haque, M. E., and Islam, M. S. (2021). Heat-activated potassium persulfate treatment of Sudan Black B dye: degradation kinetic and thermodynamic studies. J. Water Process Eng. 39, 101690. doi:10.1016/j.jwpe.2020.101690
Singh, D., and Fulekar, M. H. (2010). Benzene bioremediation using cow dung microflora in two phase partitioning bioreactor. J. Hazard. Mater. 175 (1), 336–343. doi:10.1016/j.jhazmat.2009.10.008
Song, Y., Fang, G., Zhu, C., Zhu, F., Wu, S., Chen, N., et al. (2019). Zero-valent iron activated persulfate remediation of polycyclic aromatic hydrocarbon-contaminated soils: an in situ pilot-scale study. Chem. Eng. J. 355, 65–75. doi:10.1016/j.cej.2018.08.126
Srivastava, A., Aghilesh, K., Nair, A., Ram, S., Agarwal, S., Ali, J., et al. (2021a). Response surface methodology and artificial neural network modelling for the performance evaluation of pilot-scale hybrid nanofiltration (NF) and reverse osmosis (RO) membrane system for the treatment of brackish ground water. J. Environ. Manag. 278, 111497. doi:10.1016/j.jenvman.2020.111497
Srivastava, A., Aghilesh, K., Nair, A., Ram, S., Agarwal, S., Ali, J., et al. (2021b). Response surface methodology and artificial neural network modelling for the performance evaluation of pilot-scale hybrid nanofiltration (NF) and reverse osmosis (RO) membrane system for the treatment of brackish ground water. J. Environ. Manag. 278, 111497. doi:10.1016/j.jenvman.2020.111497
Taqvi, S. A., Tufa, L. D., Zabiri, H., Mahadzir, S., Shah Maulud, A., and Uddin, F. (2017). “Artificial neural network for anomalies detection in distillation column,” in Modeling, design and simulation of systems. Editors M. S. Mohamed Ali, H. Wahid, N. A. Mohd Subha, S. Sahlan, M. A.Md. Yunus, and A. R. Wahap (Singapore: Springer), 302–311.
Xu, Q., Shi, F., You, H., and Wang, S. (2021a). Integrated remediation for organic-contaminated site by forcing running-water to modify alkali-heat/persulfate via oxidation process transfer. Chemosphere 262, 128352. doi:10.1016/j.chemosphere.2020.128352
Xu, Z., Huang, J., Fu, R., Zhou, Z., Ali, M., Shan, A., et al. (2021b). Enhanced trichloroethylene degradation in the presence of surfactant: pivotal role of Fe(II)/nZVI catalytic synergy in persulfate system. Sep. Purif. Technol. 272, 118885. doi:10.1016/j.seppur.2021.118885
Zafar, M., Kumar, S., Kumar, S., and Dhiman, A. K. (2012). Optimization of polyhydroxybutyrate (PHB) production by Azohydromonas lata MTCC 2311 by using genetic algorithm based on artificial neural network and response surface methodology. Biocatal. Agric. Biotechnol. 1 (1), 70–79. doi:10.1016/j.bcab.2011.08.012
Zhang, L. L., Ding, W., Qiu, J. T., Jin, H., Ma, H. K., Li, Z. F., et al. (2018). Modeling and optimization study on sulfamethoxazole degradation by electrochemically activated persulfate process. J. Clean. Prod. 197, 297–305. doi:10.1016/j.jclepro.2018.05.267
Zhao, B., Huang, F., Zhang, C., Huang, G., Xue, Q., and Liu, F. (2020). Pollution characteristics of aromatic hydrocarbons in the groundwater of China. J. Contam. Hydrology 233, 103676. doi:10.1016/j.jconhyd.2020.103676
Zhu, C., Fang, G., Dionysiou, D. D., Liu, C., Gao, J., Qin, W., et al. (2016). Efficient transformation of DDTs with persulfate activation by zero-valent iron nanoparticles: a mechanistic study. J. Hazard. Mater. 316, 232–241. doi:10.1016/j.jhazmat.2016.05.040
Zulfiqar, M., Chowdhury, S., Sufian, S., and Omar, A. A. (2018). Enhanced photocatalytic activity of Orange II in aqueous solution using solvent-based TiO2 nanotubes: kinetic, equilibrium and thermodynamic studies. J. Clean. Prod. 203, 848–859. doi:10.1016/j.jclepro.2018.08.324
Zulfiqar, M., Samsudin, M. F. R., and Sufian, S. (2019). Modelling and optimization of photocatalytic degradation of phenol via TiO2 nanoparticles: an insight into response surface methodology and artificial neural network. J. Photochem. Photobiol. A Chem. 384, 112039. doi:10.1016/j.jphotochem.2019.112039
Keywords: benzene, activated persulfate, response surface methodology, artificial neural network, kinetics
Citation: Luo M, Zhang X, Long T, Chen S, Zhan M, Zhu X and Yu R (2023) Modeling and optimization study on degradation of organic contaminants using nZVI activated persulfate based on response surface methodology and artificial neural network: a case study of benzene as the model pollutant. Front. Chem. 11:1270730. doi: 10.3389/fchem.2023.1270730
Received: 01 August 2023; Accepted: 09 October 2023;
Published: 19 October 2023.
Edited by:
Tamer S. Saleh, National Research Centre, EgyptReviewed by:
Mehdi Bahrami, Fasa University, IranAmin Mohammadpour, Shiraz University of Medical Sciences, Iran
Khalid Z. Elwakeel, Jeddah University, Saudi Arabia
Copyright © 2023 Luo, Zhang, Long, Chen, Zhan, Zhu and Yu. This is an open-access article distributed under the terms of the Creative Commons Attribution License (CC BY). The use, distribution or reproduction in other forums is permitted, provided the original author(s) and the copyright owner(s) are credited and that the original publication in this journal is cited, in accordance with accepted academic practice. No use, distribution or reproduction is permitted which does not comply with these terms.
*Correspondence: Ran Yu, eXVyYW5Ac2V1LmVkdS5jbg==; Xin Zhu, emh1eGluQG5pZXMub3Jn