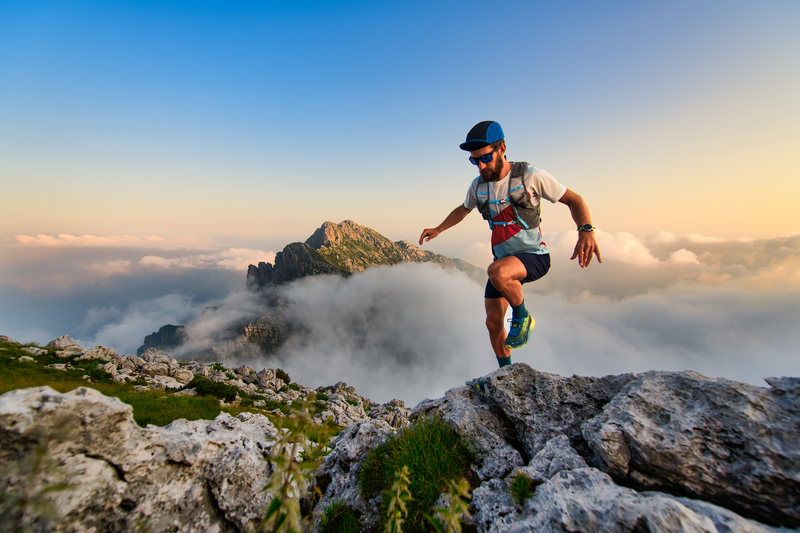
94% of researchers rate our articles as excellent or good
Learn more about the work of our research integrity team to safeguard the quality of each article we publish.
Find out more
REVIEW article
Front. Cell Dev. Biol. , 12 May 2021
Sec. Cellular Biochemistry
Volume 9 - 2021 | https://doi.org/10.3389/fcell.2021.665646
The ubiquitin-proteasome system oversees cellular protein degradation in order to regulate various critical processes, such as cell cycle control and DNA repair. Ubiquitination can serve as a marker for mutation, chemical damage, transcriptional or translational errors, and heat-induced denaturation. However, aberrant ubiquitination and degradation of tumor suppressor proteins may result in the growth and metastasis of cancer. Hence, targeting the ubiquitination cascade reaction has become a potential strategy for treating malignant diseases. Meanwhile, computer-aided methods have become widely accepted as fast and efficient techniques for early stage drug discovery. This review summarizes ubiquitination regulators that have been discovered via virtual screening and their applications for cancer treatment.
Ubiquitin is a 76-residue protein that is highly conserved in most eukaryotes (Finley and Chau, 1991; Ben-Neriah, 2002). The main function of ubiquitin is to mark proteins that need to be broken down and hydrolyze them using the ubiquitin-proteasome system (UPS) (also known as the ubiquitin-proteasome pathway, UPP) (Weissman, 2001). The C-terminus of ubiquitin contains the functional for ligation to acceptor proteins (Rotin et al., 2000; Peng et al., 2003). The ubiquitin-attached proteins are directed to the proteasome for breakdown (Voges et al., 1999). Ubiquitin can also label transmembrane proteins, such as receptors, to remove them from the cell membrane (Foot et al., 2017). The process by which target proteins are labeled with ubiquitin is called ubiquitination, and it is one of the most common forms of post-translational protein modifications (Popovic et al., 2014). Besides ubiquitin itself, ubiquitin-like molecules such as SUMO, NEDD8, or ISG also serve to regular protein homeostasis in cells (Ritchie and Zhang, 2004; Zhong et al., 2012b; Liu and Nussinov, 2013; Gâtel et al., 2020).
The process of ubiquitination includes the sequential actions of three main enzymes (Figure 1): ubiquitin-activating enzymes (E1s), ubiquitin-conjugating enzymes (E2s), and ubiquitin protein ligases (E3s) (Fajerman et al., 2004). E3 enzymes can be further subdivided into RING (really interesting new gene) family and HECT (homologous to E6-APC terminus) family proteins (Uchida and Kitagawa, 2016). In the mechanism of ubiquitination, the C-terminus of ubiquitin is first activated for nucleophilic attack activated by E1s. Then, the activated ubiquitin is transferred onto E2s. Finally, E3s transfer the activated ubiquitin from E2s to the lysine residue of a substrate protein (Rape, 2018). Monoubiquitination is mainly involved in the regulation of endocytosis, meiosis, chromatin remodeling and lysosomal targeting, while polyubiquitination is involved in targeted modification of proteins to achieve proteasome degradation, DNA repair and immune signaling (Sun and Chen, 2004).
Figure 1. Ubiquitination process and potential drug inhibition targets (E1: ubiquitin-activating enzyme; E2: ubiquitin conjugating enzyme; E3: ubiquitin protein ligase; Protein: substrate/target protein; DUB: deubiquitinating enzyme).
Ubiquitination has a central function in protein regulation and mediates various cellular processes, such as DNA repair, cell cycle control, apoptosis, the inflammatory response, and antigen presentation (Pickart, 2001; Zhuo et al., 2015). However, dysregulated ubiquitin-dependent proteolysis can result in pathological events, including cancer (Baarends et al., 1999; Saffari-Chaleshtori et al., 2020). Hence, targeting ubiquitination regulators is an emerging approach for the treatment of cancer. E1s regulate all downstream ubiquitination reactions, thus targeting E1s could potentially affected the ubiquitination status of downstream tumor-related proteins. E2 inhibitors offer higher potential selectivity for anticancer therapy. E3s specifically recognize protein substrates, thus making it a target for a variety of cancer therapies. Besides E1, E2, and E3 inhibitors, proteasome inhibitors have also been developed as anticancer agents. Finally, deubiquitinating enzymes (DUBs) have also been studied as anticancer targets due to their role in mediating the stability of proteins.
Fluorescence resonance energy transfer (FRET), homogeneous time-resolved fluorescence (HTRF), dissociation-enhanced lanthanide fluoroimmunoassay (DELFIA), electrochemiluminescence (ECL)-based assay, scintillation proximity assay (SPA), and laboratory-based in vitro and in vivo ubiquitination assays are widely used assays for screening ubiquitination inhibitors (Sun, 2005). However, each of these methods may have their own distinct disadvantages, such as low-throughput, high-cost, or susceptibility to background interference. Meanwhile, virtual screening has recently emerged as an alternative approach to identify ubiquitination regulators. Virtual screening is usually described as a computational algorithm using cascading sequential filters that can narrow the set of potentially biologically active lead-like hits against predetermined drug targets. Since “testing” is performed in silico, virtual screening does not consume valuable materials (Lavecchia and Di Giovanni, 2013). Subsequently, those companies identified as potential inhibitors by virtual screening can be verified using in vitro experiments (Walters et al., 1998; Shoichet, 2004). With continual advances in computing power, virtual screening is increasingly utilized as a supplement to high-throughput screening to enhance the rapidity and effectiveness of the drug discovery and development program (Good et al., 2000).
Recently, virtual screening has been employed for identifying inhibitors of cancer targets (Zhong et al., 2016; Li et al., 2020). For example, Russo Spena et al. (2019) identified VS10 as a selective PIN1 inhibitor with anti-ovarian cancer activity through virtual screening. Utomo et al. (2012) used virtual screening to identify drug-like compounds that can interrupt the stability of the p53-mortalin complex interaction, an anticancer target. Yousuf et al. (2017) discovered five anti-breast cancer multi-target inhibitors via structure-based virtual screening and molecular docking methods. Pan et al. (2013) based on a combination of ligand-based virtual screening and experimental testing, identified 19 drugs that have significant inhibitory effects on breast cancer resistance protein transport. Virtual screening has also been applied to the study of ubiquitination regulators. Hirayama et al. (2007) identified three compounds with similar basic dihydropyrrole skeletons as DUB (UCH-L3) inhibitors through virtual screening. Pirolli et al. (2019) identified E3 ubiquitin protein ligase NEDD4-1 inhibitors based on a virtual screening method to restore the level of Spry2 in cancer cells.
Compared to the application of virtual screening technologies against other anticancer targets, their use for identifying ubiquitination regulators remains underreported. In this review, we outline different virtual screening methods and highlight the latest developments in the use of virtual screening techniques for discovering ubiquitination regulators (Table 1). The important role of virtual screening technology for the discovery of ubiquitination regulators in the context of anticancer treatments is also described.
The UPS regulates many eukaryotic signaling pathways through controlling the quick and timely degradation of transcription regulators, enzymes and other proteins in the cell (Nandi et al., 2006). The UPS also has an important role in preventing the accumulation of misfolded or harmful proteins (Ciechanover, 1998). Ubiquitin attachment involves the formation of an isopeptide bond between the glycine C-terminus of ubiquitin and the lysine NH2 group of the substrates (Komander and Rape, 2012; Rieser et al., 2013). E1s, E2s, and E3s participate in a series of biochemical reactions in order to covalently bind ubiquitin to the substrate. Protein ubiquitination is also a highly reversible process. In some cases, DUBs can remove ubiquitin from protein substrates, thereby protecting the protein from degradation and releasing free ubiquitin for recycling (Kim et al., 2003). However, in other cases, DUBs also enhance substrate degradation.
Ubiquitination substrates can be monoubiquitinated or polyubiquitinated at one or multiple lysines (Sadowski et al., 2012). The manner of modification can depend on the position of the lysine (K6, K11, K27, K29, K33, K48, K63) or N-terminus methionine of ubiquitin is connected to the end of ubiquitin, resulting in different chain types (Zhou et al., 2014). Critically, the kind of ubiquitin signal governs the physiological consequences of these changes. For instance, K48 and heterotypic K11/K48 chains usually target the 26S proteasome to degrade substrates (Grice et al., 2015; Zheng and Shabek, 2017), whereas chains connected through K6, K27, K33, K63 often have non-proteolytic purposes (Grice and Nathan, 2016). These modifications are read by proteins with ubiquitin-binding domains, which identify chain-specific residues at the ubiquitin ends and the junction region that connects the two ubiquitin molecules. Many proteins dynamically bind to single or multiple ubiquitin molecules or chains, usually determining the half-life, location, or function of the protein (Tenno et al., 2004).
Besides its proteolytic effects, ubiquitination also controls a wide range of non-proteolytic functions, including regulation of DNA repair, enzyme activity, inflammatory signals, autophagy, receptor internalization, protein complex assembly, and intracellular trafficking (Welchman et al., 2005). For example, the control of membrane protein types and abundance is usually regulated by ubiquitination (Hicke and Dunn, 2003). In this process, ubiquitination is a signal to classify, transport, and remove membrane proteins (such as ion channels, transporters, and signal receptors) through endocytosis (MacGurn et al., 2012). Ubiquitination also participates in the shedding of membrane-associated proteins, thus also controlling their potential transport to adjacent cells.
Aberrant ubiquitin signaling is considered to be the molecular cause of certain cancers, neurodegeneration, cardiovascular or immune diseases. Dysregulated ubiquitination may cause abnormal activation or inactivation of pathways resulting in oncogenesis or defects in cell metabolism pathways. Improper or insufficient assembly of protein complexes are associated with inflammation or aberrant DNA repair activity, while the buildup of misfolded proteins within the endoplasmic reticulum or cytoplasm is a hallmark of neurodegenerative diseases. Any of these changes will cause damage to cell function.
Recently, it has been discovered that ubiquitin can also be phosphorylated, acetylated and modified by binding to ubiquitin-like proteins, which indicates that the process and its regulation are much more complicated than originally thought (Swatek and Komander, 2016). Similar to the process of ubiquitination, small ubiquitin-related modifier (SUMO) be attached to the lysine moieties of proteins, thus regulating their localization, stability, and interactions (Johnson, 2004). At present, the most studied ubiquitin modification system that can compete with ubiquitination for the modification of Lys residues is SUMO. The SUMO system is simpler than the ubiquitin system. It only has a single E1 enzyme, a single E2 enzyme and a few E3 enzymes.
The other two common ubiquitin modifiers are NEDD8 (neural precursor cell-expressed developmentally downregulated 8) and ISG15 (interferon-stimulated gene 15) (Xirodimas et al., 2004). NEDD8, a 81-amino acid protein with 60% identity and 80% similarity to ubiquitin, is highly conserved in eukaryotes (Kamitani et al., 1997; Zhong et al., 2015). Neddylation can extensively regulate biological events, such as cell cycle, signal transduction and immune recognition. Enzymes that regulate neddylation include NEDD8-activating enzyme, NEDD8-binding enzyme and NEDD8 ligase. Similar to ubiquitination, neddylation is also a dynamic modification process that a maintains balance between neddylation and deneddylation.
ISG15 can also covalently modify proteins, but does not mediate protein degradation (Morales and Lenschow, 2013). It is currently believed that ISG15 modification is mainly involved in the regulation of the innate immune function through leukocyte chemotaxis and the process of interferon action. ISG15 may also play an important role in stimulating cell proliferation and enhancing cytotoxicity.
Virtual screening is typically performed in hierarchical workflow with sequential steps (each with their own advantages and limitations) that filter and remove unwanted molecules. Molecules that pass all stages of the virtual screening are called the “hit compounds.” One method of sorting the hit compounds is clustering, which is an unsupervised learning technique were input data is fed into the algorithm in order to identify patterns and classify the data into several categories. By using clustering to group hits based on their structure, the biased choice of molecules can be circumvented and representative samples of compounds can be obtained. Hierarchical clustering, HDBSCAN and k-means clustering are different clustering methods for hit selection (Gimeno et al., 2019). Finally, hit compounds must also be verified through experiments to validate their bioactivity.
Recently, virtual screening has become a powerful method to supplement the existing array of high-throughput screening platforms. Using computer-assisted virtual screening, potential hits can be quickly identified in silico to decrease the number of molecules needed to be tested in vitro and in vivo (Ma et al., 2013). Integrating virtual methods into the development of pharmaceutical leads can significantly decrease the economic cost of synthesis or biological experiments (Yang et al., 2016).
Virtual screening protocols can be broadly classed into two categories: structure-based virtual screening (SBVS) and ligand-based virtual screening (LBVS) (Figure 2; Muegge and Oloff, 2006). SBVS, which includes docking, needs a 3D structure of the protein target (Stahura and Bajorath, 2005). In docking, candidate ligands are docked into the target protein, and a scoring function is applied to evaluate the possibility of high-affinity binding between the ligands and proteins. On the other hand, LBVS techniques generally use a library of ligands with known activities (Ripphausen et al., 2011). LBVS uses active compounds as templates, then searches chemical molecular structures that matches the shape or pharmacophore model of the compound. SBVS and LBVS strategies will be discussed individually in more detail in the sections below.
Figure 2. Workflow for ubiquitination regulator discovery by ligand-based virtual screening (LBVS) and structure-based virtual screening (SBVS). Reproduced, with permission, from Reference Raschka and Kaufman (2020).
In SBVS, the three-dimensional structure of the protein is employed. SBVS aims to identify compounds with unknown affinity to the target from their three-dimensional structure (Figure 2; Lionta et al., 2014). The process of SBVS usually starts with the information of the three-dimensional target structure of interest (Kroemer, 2007). This structure can be obtained from experimental data such as X-ray crystallography, nuclear magnetic resonance, or neutron scattering experiments, or can be constructed from homology modeling or molecular dynamics simulations. When performing SBVS, the druggability of the receptor, the selection of the most relevant protein conformation, and the flexibility of the receptor, should also be considered. After the target model is prepared, the compound library also has to be preprocessed in order to assign the proper stereochemistry, tautomeric, and protonation states.
Unlike structure-based methods, LBVS starts with known active ligands as templates (Figure 2). Generally speaking, the LBVS method relies on the use of structural or pharmacophoric descriptors, and analyzes the relationship between active compounds and databases or test compounds (Chen et al., 2007). Many different types of molecular descriptors have been developed, and they have been extensively reviewed elsewhere (Shahlaei, 2013). However, one limitation of ligand-based methods is that they limit the diversity of hits, because they are mainly biased toward the properties of known ligands (Baber et al., 2006). Hence, newer LBVS methods that identify remote similarity connections, that is, compounds that are structurally different from templates but possess similar activities, are being developed. The identification of such compounds is often critical to avoid lead optimization bottlenecks.
There is increasing evidence that the UPS plays a key role in tumorigenesis. Ubiquitin-mediated signals are often altered in cancer cells, including dysregulation of tumor suppressors and oncogenes. For example, the tumor suppressors p53 and p27 can be regulated by ubiquitination (Thibaudeau and Smith, 2019). Hence, the use of proteasome inhibitors could alter balance between pro-apoptotic proteins and anti-apoptotic proteins, and induce cell cycle arrest and apoptosis. Moreover, targeting specific ubiquitin pathway enzymes (E1s, E2s, and E3s) and DUBs could be a method of developing more selective anti-tumor drugs with better toxicity characteristics than proteasome inhibitors such as bortezomib (Mattern et al., 2012).
Meanwhile, virtual screening is an efficient method to screen large libraries of compounds against a pharmacological target in silico. In this section of the review, we highlight examples of ubiquitination regulators that have been discussed by virtual screening.
Inhibitors of E1s are a potentially new class of cancer drug (Yang et al., 2007). Two types of ubiquitin-activating E1 enzymes are known: the main is UBA1, while the newly discovered UBA6 currently has unclear functions. However, due to the lack of specificity of E1s, there are few reports on E1 inhibitors discovered by VS.
Like the ubiquitination pathway, NEDD8 requires activation by the E1-like NEDD8-activating enzyme (NAE). NAE specifically regulates the degradation of substrates of the cullin-ring ubiquitin E3 ligases, and is a potential target for anticancer drugs (Zhong et al., 2014; Wu et al., 2018).
Zhang et al. (2014) adopted a combined strategy of VS to discover diverse covalent inhibitors of NAE, involving docking-enabled pharmacophore model (according to the possible active conformation of the selected covalent inhibitors) and a dynamic structure-based pharmacophore (derived from snapshots of molecular dynamics simulations). The ZINC publicly database containing over 22 million compounds was initially screened with the Align to Selected Substructure module in Discovery Studio 2.5. Conformations of the focused library of 27,996 molecules were then generated using the Build 3D Database utility, which were screened using the Search 3D Database (for ligand-based pharmacophore screening) or Screen Library (for structure-based pharmacophore screening) modules to produce 256 highly ranked hits. Next, covalent docking was performed using Gold 5.0, resulting in eight hits, of which three were confirmed to be active after bioassay evaluation. Compound 1 (LZ3) was determined to be the most effective NAE inhibitor, with IC50 = 1.06 ± 0.18 μM. Moreover, a cell-based assay validated the proposed covalent model for compound 1. MTT assay results showed that compound 1 strongly inhibited the proliferation of Caco-2, Bcl-7402 and MCF-7 cancer cells, with IC50 values of 12.3 to 29.5 μM.
Zhong et al. (2012a) used SBVS of from the ZINC of natural products database of over 90,000 compounds to discover a dipeptide-conjugated deoxyvasicinone compound (2) as an NAE inhibitor. The Internal Coordinate Mechanics was used for docking, where flexible ligands were matched to a grid representation of the protein and scored based on the predicted binding affinity. Nine top-scoring ligands were obtained and tested in a preliminary E1 NAE activity assay, and four of these showed inhibitory effects against Ubc12-NEDD8 conjugation in vitro with the most potent of these being compound 2. Compound 2 was hypothesized to act via reversibly binding to the ATP-binding domain. Compound 2 inhibited NAE activity with micromolar potency in both cell-based and cell-free assays.
SUMO E1 is also a potential therapeutic target for many human diseases including cancer. In order to better identify the SUMO E1 small molecule inhibitors with drug-like properties, Kumar et al. (2013) performed virtual screening against SUMO E1 using the Maybridge small molecule library. Nearly 78,000 molecules in the Maybridge library were docked against SUMO E1 using a two-step docking strategy. First, ligands with non-ideal energetics and geometries were removed using Autodock-Vina. The top-ranked molecules from the initial stage were then re-docked using RosettaLigand, which uses three-dimensional conformations of ligands. Hits were ranked using the RosettaLigand energy function as well as ligand-protein interface scores, followed by prioritization of the highest-scoring docking hits using molecular mechanics. Then, a similarity search against the ZINC database identified a series of new compounds via “scaffold hopping.” Subsequent in vitro testing using revealed that compounds containing a quinazolinyloxy biaryl urea were new inhibitors of SUMO E1, with the most potent compound 3 of this class having an IC50 of 14.4 ± 1.3 μM against SUMO E1. This compound acts by reducing the SUMO E1-SUMO thioester bond formation. By employing virtual screening in conjunction scaffold hopping, they also found that compounds containing pyrazole and thiazolium urea moieties can function as moderate inhibitors of SUMO E1 (Kumar et al., 2016).
As an intermediate between the E1 and E3 proteins, the E2s play an important function in determining the kind of polyubiquitin chain connected (Rodrigo-Brenni and Morgan, 2007). At present, about 40–50 genes encoding ubiquitin-conjugating enzymes have been found in the human genome, which is much greater than the number of E1 enzymes. After E2s bind to the activated ubiquitin, ubiquitin molecules are delivered to ubiquitin ligase E3 (Gundogdu and Walden, 2019). Because each E2 can only associate and cooperate with a specific set of E3 enzymes, targeting the E2–E3 interaction could be a potentially selective anticancer approach. However, E2 enzyme inhibitors are currently only in the developmental and pre-clinical testing stage as they still lack sufficient specificity for E2s.
Cdc34 is an E2 enzymes directly related to oncogenesis. Arrigoni et al. (2014) utilized virtual screening and docking to screen over 735,000 compounds from the ZINC database. In the first stage, coarse molecular selection was performed by shape complementarity using DOCK Blaster. The top 500 ligands from the initial stage were re-docked against Cdc34 using Autodock version 4.2, followed by a filtering step involving spatial criteria and binding free energy to shortlist 20 hit molecules. Common structural features of these 20 molecules could be employed as pharmacophoric elements for future investigations.
The SUMO E2 conjugating enzyme Ubc9 is highly expressed in various human cancer cell lines, suggesting that Ubc9 may be an attractive drug target (Tomasi et al., 2012). Duan et al. (2009) used SBVS to screen small chemical compounds as potential anticancer drugs by determining the structure and potential target sites of Ubc9. They analyzed the interface regions between Ubc9 and its binding partners, in order to identify possible targeting sites on Ubc9 that can be used for virtual screening and ligand design.
The E3s are responsible for the direct ligation of ubiquitin to the protein, thus conferring substrate specificity and selectivity. Mutations or down-regulation of E3 enzymes can often be detected in different tumors (Shafique et al., 2018). As the substrate recognition component of the UPS pathway, there are about 500–1000 ubiquitin ligases in the human body (Ottis et al., 2017). Hence, targeting specific E3 ligases will only affect a particular subset of ubiquitin substrates, without affecting for the entire ubiquitination pathway. As a result, E3 enzymes have great potential for cancer treatment.
The Von Hippel-Lindau (VHL) E3 ubiquitin ligase controls the ubiquitination and subsequent of its substrate, hypoxia inducible factor 1 (HIF-1). VHL is a potential target for a variety of diseases, including anemia, inflammation, neurodegenerative diseases and cancer. Liu Y. et al. (2020) employed a combined ensemble-based and ligand-based virtual screening strategy to finding potential inhibitors against VHL from the Specs database. The use of ensemble-based virtual screening to distinguish the active molecules from inactive molecules increases precision compared to usual single SBVS methods. Ten representative molecules were obtained from the virtual screening, and the predicted binding modes of the first five molecules were analyzed with comparison to the reference ligand. However, further in vitro and in vivo experiments are needed to verify the effectiveness of the ten candidates.
MDM2 protein regulates p53 activity by acting as a ubiquitin E3 ligase (Brooks and Gu, 2006). MDM2 can bind to the transcriptional activation domain of p53, inhibit its transcriptional activity, promote its ubiquitination and degradation, and hence block the functions of p53-regulated cell cycle stagnation and apoptosis induction (Aydin et al., 2020). Nutlin 3a was the first small molecule inhibitor targeting MDM2 that acts through binding to p53 in order to block its interaction with MDM2, thereby increasing the level of p53 in cells.
Atatreh et al. (2018) identified compounds 4 (S01) and 5 (S02) as MDM2 inhibitors through pharmacophore and structure-based in silico screening. First, they constructed a pharmacophore from the p53-binding pocket of the Mdm2 protein (PDB: 3JZK) using three pharmacophore elements: one hydrophobic/aromatic, one hydrophobic, and one aromatic. This pharmacophore was used to preliminarily screen over 580,000 molecules from the TimTec Compound Library. Compounds that matched the pharmacophore were then docked against the targeted protein using GLIDE. The highest-ranked 500 ligands were manually inspected for their binding pose within the p53 binding site of Mdm2, after which 40 were chosen for in vitro Mdm2-p53 inhibition using ELISA. A few hits showed comparable potency to Nutlin-3a at inhibiting Mdm2-p53, including S01 (4) and S02 (5). Compound 4 binds Gly58 at the active site of MDM2, which is a non-canonical interaction observed by MDM2 co-crystallization inhibitors that blocks the interaction of MDM2-p53. These compounds showed potential anticancer activity against breast cancer cell lines of different subtypes.
Skp2 E3 ligase is overexpressed in a number of cancers, and it has functions in cell metastasis, cycle, metabolism, senescence, and cancer progression (Chan et al., 2010). Chan et al. (2013) performed SBVS on 120,000 commercial compounds using HiPCDock. Hits were chosen based on both calculated binding strength as well as other drug-like characteristics including molecular mass and solubility. The top 25 hits were then tested using an in vitro pull-down assay to Skp2 and Skp1 inhibition. From the screening campaign, compound 6 emerged as a potent and specific Skp2 inhibitor. Compound 6 displayed potent antiproliferative activity against prostate cancer cell lines, including PC-3 (IC50 = 5.61 μM) and LNCaP (IC50 = 1.22 μM), but only had slight effects on normal prostate epithelial (PNT1A) cells. Finally, this compound showed powerful in vivo antitumor activity and enhanced sensitivity to chemotherapeutic agents to decrease cancer cell viability.
SCF-Skp2 coordinates with Cks1 to promote the proliferation of cancer cells, via promoting the breakdown of p27, a CDK inhibitor (Tian et al., 2013). Wu et al. (2012) targeted the p27-binding interface within the Skp2-Cks1 complex using in silico screening to discover selective Skp2 inhibitors. ICM-PocketFinder was used to find a site formed by both Skp2 and Cks1 within the Skp2-Cks1-p27 crystal structure. The pocket’s area and volume was estimated to be suitable for small molecule binding. A virtual ligand screening of 315,000 compounds using ICM-VLS generated 202 screening hits with binding energy less than –30 U, and 96 of those ligands were chosen based on Lipinski properties to eliminate non-druglike molecules. These hits were experimentally screened using and four active compounds (7–10) were obtained. These hits selectively reduced p27 degradation regulated by Skp2 through inhibiting p27 binding. In tumor cells, these molecules increased p27 accumulation in a Skp2-dependent manner and promoted cell-type-specific blockages at the G1 or G2/M stages. The compound also raised both p27 protein level and longevity in metastatic melanoma cells, in a manner dependent on Skp2.
Substrates modified by a polyubiquitination chain will be degraded by the 26S proteasome (Marshall and Vierstra, 2019). The 26S proteasome is a multi-subunit protease comprised of the catalytic core 20S proteasome and 19S regulatory subunits. The 20S catalytic core has a barrel structure and consists of four heptameric rings. On the outside are two identical non-catalytic α rings, and in the middle are two identical catalytic β rings. The 19S regulatory subunit has a structure comprised of a lid and a base. The 20S catalytic core of 26S proteasome is an important research target for proteasome inhibitors (Manasanch and Orlowski, 2017).
Di Giovanni et al. (2016) used a hierarchical screening approach for the 65,000 NCI lead-like library, followed by similarity searching over the entire NCI database using the most potent hit, in order to identify the β5/β6-specific tripeptide derivative 11 as a selective inhibitor of chymotrypsin-like proteasome activity. Specifically, three in silico screening modules (FRED, GLIDE, and GOLD) were used. Flexible docking was conducted using default parameters with GLIDE Standard Precision and Extra Precision, generating a shortlist of 500 top-ranked compounds. These molecules were re-docked using GOLD, then scored using the ChemPLP scoring function. For hit selection, two distinct hit lists were produced from the GLIDE and GOLD-ranked molecules. Manual inspection of binding followed by pharmacokinetics and PAINS filtering by FAF-Drugs2 resulted in a top list of 33 molecules, which were tested in an in vitro assay against the 20S proteasome. Finally, scaffold searching using the most active hit identified the most promising compound 11, which showed an antiproliferative IC50 value of 16.2 ± 1.8 μM against multiple myeloma MM.1R cells.
Miller et al. (2015) conducted large-scale SBVS of small molecules at the active sites of the proteasome. The proteasome conformation was docking was prepared by molecular dynamics simulation of homology models with highly potent peptide ligands. By considering binding energy and interactions of known ligands at the binding site, the proteasome conformation at 341 ps was chosen for docking. After docking over 345,000 molecules from the University of Cincinnati database, 288 ligands were shortlisted by consensus scoring using various force field-based energy scoring functions (MM-PBSA and MM-GBSA), as well as visual inspection of binding poses. Of the tested molecules, 19 compounds were active at 5 μM in a CT-L activity assay. Subsequent optimization led to the identification of a non-peptide, reversible proteasome inhibitor compound 12 (G4-1) which showed an IC50 value of 10.5 μM against human pancreatic cancer BxPC-3 cells. The compound also showed excellent metabolic stability and effectively suppressed prostate cancer growth in a mice xenograft model without apparent systemic toxicity.
DUBs can reverse the ubiquitination process through opposing the activity of E3 ligases, then saving the substrate from proteasomal degradation. Hence, DUB activity is critical for regulating physiological events such as cell growth and differentiation, transcription regulation, but its dysfunction can also lead to oncogenesis.
Ubiquitin-specific protease 7 (USP7) is one of the most widely studied and characteristic DUBs, and is a potential cancer treatment target. Through structure-based screening, molecular dynamics simulation and experimental valuation evaluation, Liu S. et al. (2020) discovered compound 13 as a new scaffold structure as an inhibitor of USP7. The Specs database of 250,000 compounds was screened using high-throughput virtual screening, standard-precision, and extra-precision modules in sequence. The top 10% of poses at each stage were shortlisted using Glide Gscore. The root-mean-square deviation and fluctuation metrics, which are related to complex stability, were calculated using the cpptraj module in AmberTools 15. Hydrogen bond occupancy, close contacts, equilibrated trajectories, and binding free energy (ΔGbind) were calculated using MM/PBSA. Finally, the highest-ranking 13 molecules were purchased, from which compound 13 was identified as the most potent hit after biological evaluation. The binding affinity between the USP7 catalytic domain and compound 13 was determined to be Kd = 4.46 ± 0.86 μM. This compound also showed an IC50 value of 15.43 ± 3.49 μM against LNCaP prostate cancer cells.
UCHL-3 (ubiquitin-C-terminal hydrol3) is a DUB involved in the homologous recombination repair mechanism of DNA double-strand breaks (DSBs). A number of studies have shown that UCHL-3 inhibitors can be used in combination therapy for treating cancer. Alakhdar et al. (2020) utilized a combination of virtual screening methods and 3D structures of more than 1.8 million compounds from the ChemBl database for virtual screening. Their strategy involved the combination of Lipinski’s Rule of Five, hierarchical molecular docking, pharmacophore modeling, toxicity and PAINS filter, Veber’s rule, and single-point molecular mechanics Poisson/Boltzmann surface area docking pose rescoring (Ma et al., 2011). This multiple filtering approach resulted in the shortlisting of 21 ligands, which were then analyzed using molecular dynamics simulations to estimate complex stability. Then, MM/PBSA calculations were performed on MD trajectories to calculate the energy per residue contribute to the binding energy. A 3D pharmacophore model was generated for identifying the significant features of reported UCHL3 inhibitors. Finally, three new potential UCHL-3 inhibitors (compounds 14–16) were identified.
Ubiquitination is a key regulatory process in cells, and is also a target for cancer treatment. Meanwhile, virtual screening has emerged as an indispensable part of drug discovery efforts and has become widely employed in pharmaceutical research. In this review, we have highlighted the use of virtual screening strategies to identify ubiquitination regulators, including E2, E3, proteasome and DUB regulators. Most of these studies have used SBVS, while LBVS has not been employed as much for the screening of ubiquitination regulators by comparison.
The main challenge facing virtual screening is still limited accuracy regardless using of the SBVS or LBVS methods. While calculations can be performed quickly relative to biological experiments, the false positive rate is still relatively high. Therefore, it is necessary to continuously improve the screening and scoring methods, or utilize novel techniques, such as predictive algorithms derived from machine and/pr deep learning, in order to improve the hit rate from virtual screening. Toward the future, one aspect that has significant room for enhancement in virtual screening is the prediction of potency. A key advancement would be if more consistent approaches could be developed for identifying more effective and druglike ligands in silico, thus directly benefiting subsequent drug discovery steps.
Y-QS drafted the manuscript of this review article. D-LM and C-HL conceived and supervised the manuscript and approved the final version to be published. CW, K-JW, Q-BH, and X-MM contributed to the conceptualization and provided critical responses to reviewers’ comments. CW and K-JW also collected and organized the information and prepared the table and figures for the revised manuscript. All authors contributed to the article and approved the submitted version.
This work was supported by the Hong Kong Baptist University (FRG2/17–18/003), the Health and Medical Research Fund (HMRF/14150561), the National Natural Science Foundation of China (22077109 and 21775131), the Hong Kong Baptist University Century Club Sponsorship Scheme 2020, the Interdisciplinary Research Matching Scheme (RC–IRMS/16–17/03), Interdisciplinary Research Clusters Matching Scheme (RC–IRCs/17–18/03), Collaborative Research Fund (C5026–16G), SKLEBA and HKBU Strategic Development Fund (SKLP_1920_P02), the Science and Technology Project of Shenzhen (No. JCYJ20160531193812867), the Science and Technology Development Fund, Macau SAR (File No. 0072/2018/A2), SKL-QRCM(UM)-2020-2022, and the University of Macau Research Grant (MYRG2019–00002–ICMS).
The authors declare that the research was conducted in the absence of any commercial or financial relationships that could be construed as a potential conflict of interest.
Alakhdar, A. A., Saleh, A. H., and Arafa, R. K. (2020). Targeting homologous recombination (HR) repair mechanism for cancer treatment: discovery of new potential UCHL-3 inhibitors via virtual screening, molecular dynamics and binding mode analysis. J. Biomol. Struct. Dyn. doi: 10.1080/07391102.2020.1812432 [Epub ahead of print],
Arrigoni, A., Bertini, L., De Gioia, L., and Papaleo, E. (2014). Inhibitors of the Cdc34 acidic loop: a computational investigation integrating molecular dynamics, virtual screening and docking approaches. FEBS Open Bio. 4, 473–484. doi: 10.1016/j.fob.2014.04.011
Atatreh, N., Ghattas, M. A., Bardaweel, S. K., Rawashdeh, S. A., and Sorkhy, M. A. (2018). Identification of new inhibitors of Mdm2-p53 interaction via pharmacophore and structure-based virtual screening. Drug Des. Devel Ther. 12, 3741–3752. doi: 10.2147/DDDT.S182444
Aydin, G., Paksoy, M. N., Orhan, M. D., Avsar, T., Yurtsever, M., and Durdagi, S. (2020). Proposing novel MDM2 inhibitors: combined physics-driven high-throughput virtual screening and in vitro studies. Chem. Biol. Drug Des. 96, 684–700. doi: 10.1111/cbdd.13694
Baarends, W. M., Hoogerbrugge, J. W., Roest, H. P., Ooms, M., Vreeburg, J., Hoeijmakers, J. H. J., et al. (1999). Histone ubiquitination and chromatin remodeling in mouse spermatogenesis. Dev. Biol. 207, 322–333. doi: 10.1006/dbio.1998.9155
Baber, J. C., Shirley, W. A., Gao, Y., and Feher, M. (2006). The use of consensus scoring in ligand-based virtual screening. J. Chem. Inf. Model. 46, 277–288. doi: 10.1021/ci050296y
Ben-Neriah, Y. (2002). Regulatory functions of ubiquitination in the immune system. Nat. Immunol. 3, 20–26. doi: 10.1038/ni0102-20
Brooks, C. L., and Gu, W. (2006). p53 ubiquitination: Mdm2 and beyond. Mol. Cell 21, 307–315. doi: 10.1016/j.molcel.2006.01.020
Chan, C.-H., Lee, S.-W., Wang, J., and Lin, H.-K. (2010). Regulation of Skp2 expression and activity and its role in cancer progression. Sci. World J. 10, 1001–1015. doi: 10.1100/tsw.2010.89
Chan, C.-H., Morrow, J. K., Li, C.-F., Gao, Y., Jin, G., Moten, A., et al. (2013). Pharmacological inactivation of Skp2 SCF ubiquitin ligase restricts cancer stem cell traits and cancer progression. Cell 154, 556–568. doi: 10.1016/j.cell.2013.06.048
Chen, B., Harrison, R. F., Papadatos, G., Willett, P., Wood, D. J., Lewell, X. Q., et al. (2007). Evaluation of machine-learning methods for ligand-based virtual screening. J. Comput. Aided Mol. Des. 21, 53–62. doi: 10.1007/s10822-006-9096-5
Ciechanover, A. (1998). The ubiquitin–proteasome pathway: on protein death and cell life. EMBO J. 17, 7151–7160. doi: 10.1093/emboj/17.24.7151
Di Giovanni, C., Ettari, R., Sarno, S., Rotondo, A., Bitto, A., Squadrito, F., et al. (2016). Identification of noncovalent proteasome inhibitors with high selectivity for chymotrypsin-like activity by a multistep structure-based virtual screening. Eur. J. Med. Chem. 121, 578–591. doi: 10.1016/j.ejmech.2016.05.049
Duan, X., Trent, J. O., and Ye, H. (2009). Targeting the SUMO E2 conjugating enzyme Ubc9 interaction for anti-cancer drug design. Anti Cancer Agents Med. Chem. 9, 51–54. doi: 10.2174/187152009787047716
Fajerman, I., Schwartz, A. L., and Ciechanover, A. (2004). Degradation of the Id2 developmental regulator: targeting via N-terminal ubiquitination. Biochem. Biophys. Res. Commun. 314, 505–512. doi: 10.1016/j.bbrc.2003.12.116
Finley, D., and Chau, V. (1991). Ubiquitination. Annu. Rev. Cell Biol. 7, 25–69. doi: 10.1146/annurev.cb.07.110191.000325
Foot, N., Henshall, T., and Kumar, S. (2017). Ubiquitination and the regulation of membrane proteins. Physiol. Rev. 97, 253–281. doi: 10.1152/physrev.00012.2016
Gâtel, P., Piechaczyk, M., and Bossis, G. (2020). Ubiquitin, SUMO, and Nedd8 as therapeutic targets in cancer. Adv. Exp. Med. Biol. 1233, 29–54. doi: 10.1007/978-3-030-38266-7_2
Gimeno, A., Ojeda-Montes, M. J., Tomás-Hernández, S., Cereto-Massagué, A., Beltrán-Debón, R., Mulero, M., et al. (2019). The light and dark sides of virtual screening: what is there to know? Int. J. Mol. Sci. 20:1375. doi: 10.3390/ijms20061375
Good, A. C., Krystek, S. R., and Mason, J. S. (2000). High-throughput and virtual screening: core lead discovery technologies move towards integration. Drug Discov. Today 5, S61–S69. doi: 10.1016/S1359-6446(00)80056-2
Grice, G. L., Lobb, I. T., Weekes, M. P., Gygi, S. P., Antrobus, R., and Nathan, J. A. (2015). The proteasome distinguishes between heterotypic and homotypic lysine-11-linked polyubiquitin chains. Cell Rep. 12, 545–553. doi: 10.1016/j.celrep.2015.06.061
Grice, G. L., and Nathan, J. A. (2016). The recognition of ubiquitinated proteins by the proteasome. Cell. Mol. Life Sci. 73, 3497–3506. doi: 10.1007/s00018-016-2255-5
Gundogdu, M., and Walden, H. (2019). Structural basis of generic versus specific E2–RING E3 interactions in protein ubiquitination. Protein Sci. 28, 1758–1770. doi: 10.1002/pro.3690
Hicke, L., and Dunn, R. (2003). Regulation of membrane protein transport by ubiquitin and ubiquitin-binding proteins. Annu. Rev. Cell Dev. Biol. 19, 141–172. doi: 10.1146/annurev.cellbio.19.110701.154617
Hirayama, K., Aoki, S., Nishikawa, K., Matsumoto, T., and Wada, K. (2007). Identification of novel chemical inhibitors for ubiquitin C-terminal hydrolase-L3 by virtual screening. Bioorg. Med. Chem. 15, 6810–6818. doi: 10.1016/j.bmc.2007.07.016
Johnson, E. S. (2004). Protein modification by SUMO. Annu. Rev. Biochem. 73, 355–382. doi: 10.1146/annurev.biochem.73.011303.074118
Kamitani, T., Kito, K., Nguyen, H. P., and Yeh, E. (1997). Characterization of NEDD8, a developmentally down-regulated ubiquitin-like protein. J. Biol. Chem. 272, 28557–28562. doi: 10.1074/jbc.272.45.28557
Kim, J. H., Park, K. C., Chung, S. S., Bang, O., and Chung, C. H. (2003). Deubiquitinating enzymes as cellular regulators. J. Biochem. 134, 9–18. doi: 10.1093/jb/mvg107
Komander, D., and Rape, M. (2012). The ubiquitin code. Annu. Rev. Biochem. 81, 203–229. doi: 10.1146/annurev-biochem-060310-170328
Kroemer, R. T. (2007). Structure-based drug design: docking and scoring. Curr. Protein Pept. Sci. 8, 312–328. doi: 10.2174/138920307781369382
Kumar, A., Ito, A., Hirohama, M., Yoshida, M., and Zhang, K. Y. (2013). Identification of quinazolinyloxy biaryl urea as a new class of SUMO activating enzyme 1 inhibitors. Bioorg. Med. Chem. Lett. 23, 5145–5149. doi: 10.1016/j.bmcl.2013.07.022
Kumar, A., Ito, A., Hirohama, M., Yoshida, M., and Zhang, K. Y. J. (2016). Identification of new SUMO activating enzyme 1 inhibitors using virtual screening and scaffold hopping. Bioorg. Med. Chem. Lett. 26, 1218–1223. doi: 10.1016/j.bmcl.2016.01.030
Lavecchia, A., and Di Giovanni, C. (2013). Virtual screening strategies in drug discovery: a critical review. Curr. Med. Chem. 20, 2839–2860. doi: 10.2174/09298673113209990001
Li, G., Henry, S. A., Liu, H., Kang, T.-S., Nao, S.-C., Zhao, Y., et al. (2020). A robust photoluminescence screening assay identifies uracil-DNA glycosylase inhibitors against prostate cancer. Chem. Sci. 11, 1750–1760. doi: 10.1039/C9SC05623H
Lionta, E., Spyrou, G., Vassilatis, D. K., and Cournia, Z. (2014). Structure-based virtual screening for drug discovery: principles, applications and recent advances. Curr. Top. Med. Chem. 14, 1923–1938. doi: 10.2174/1568026614666140929124445
Liu, J., and Nussinov, R. (2013). The role of allostery in the ubiquitin-proteasome system. Crit. Rev. Biochem. Mol. Biol. 48, 89–97. doi: 10.3109/10409238.2012.742856
Liu, S., Zhou, X., Li, M., Zhao, W., Zhou, S., Cheng, K., et al. (2020). Discovery of ubiquitin-specific protease 7 (USP7) Inhibitors with novel scaffold structures by virtual screening, molecular dynamics simulation, and biological evaluation. J. Chem. Inf. Model. 60, 3255–3264. doi: 10.1021/acs.jcim.0c00154
Liu, Y., Lei, Y., Guo, S., and Zuo, Z. (2020). Ensemble-based virtual screening in discovering potent inhibitors targeting von hippel-lindau (VHL) E3 ubiquitin ligase. Life Sci. 262:118495. doi: 10.1016/j.lfs.2020.118495
Ma, D. L., Chan, D. S. H., and Leung, C. H. (2011). Molecular docking for virtual screening of natural product databases. Chem. Sci. 2, 1656–1665. doi: 10.1039/C1SC00152C
Ma, D.-L., Chan, D. S.-H., and Leung, C.-H. (2013). Drug repositioning by structure-based virtual screening. Chem. Soc. Rev. 42, 2130–2141. doi: 10.1039/c2cs35357a
MacGurn, J. A., Hsu, P.-C., and Emr, S. D. (2012). Ubiquitin and membrane protein turnover: from cradle to grave. Annu. Rev. Biochem. 81, 231–259. doi: 10.1146/annurev-biochem-060210-093619
Manasanch, E. E., and Orlowski, R. Z. (2017). Proteasome inhibitors in cancer therapy. Nat. Rev. Clin. Oncol. 14, 417–433. doi: 10.1038/nrclinonc.2016.206
Marshall, R. S., and Vierstra, R. D. (2019). Dynamic regulation of the 26S proteasome: from synthesis to degradation. Front. Mol. Biosci. 6:40. doi: 10.3389/fmolb.2019.00040
Mattern, M. R., Wu, J., and Nicholson, B. (2012). Ubiquitin-based anticancer therapy: carpet bombing with proteasome inhibitors vs surgical strikes with E1, E2, E3, or DUB inhibitors. Biochim. Biophys. Acta 1823, 2014–2021. doi: 10.1016/j.bbamcr.2012.05.005
Miller, Z., Kim, K.-S., Lee, D.-M., Kasam, V., Baek, S. E., Lee, K. H., et al. (2015). Proteasome inhibitors with pyrazole scaffolds from structure-based virtual screening. J. Med. Chem. 58, 2036–2041. doi: 10.1021/jm501344n
Morales, D. J., and Lenschow, D. J. (2013). The antiviral activities of ISG15. J. Mol. Biol. 425, 4995–5008. doi: 10.1016/j.jmb.2013.09.041
Muegge, I., and Oloff, S. (2006). Advances in virtual screening. Drug Discov. Today Technol. 3, 405–411. doi: 10.1016/j.ddtec.2006.12.002
Nandi, D., Tahiliani, P., Kumar, A., and Chandu, D. (2006). The ubiquitin-proteasome system. J. Biosci. 31, 137–155. doi: 10.1007/BF02705243
Ottis, P., Toure, M., Cromm, P. M., Ko, E., Gustafson, J. L., and Crews, C. M. (2017). Assessing different E3 ligases for small molecule induced protein ubiquitination and degradation. ACS Chem. Biol. 12, 2570–2578. doi: 10.1021/acschembio.7b00485
Pan, Y., Chothe, P. P., and Swaan, P. W. (2013). Identification of novel breast cancer resistance protein (BCRP) inhibitors by virtual screening. Mol. Pharm. 10, 1236–1248. doi: 10.1021/mp300547h
Peng, J., Schwartz, D., Elias, J. E., Thoreen, C. C., Cheng, D., Marsischky, G., et al. (2003). A proteomics approach to understanding protein ubiquitination. Nat. Biotechnol. 21, 921–926. doi: 10.1038/nbt849
Pickart, C. M. (2001). Mechanisms underlying ubiquitination. Annu. Rev. Biochem. 70, 503–533. doi: 10.1146/annurev.biochem.70.1.503
Pirolli, D., Righino, B., Tropea, B., Gurrieri, F., Sangiorgi, E., and Rosa, M. C. D. (2019). In silico design of E3 ubiquitin-protein ligase NEDD4-1 inhibitors: an alternative approach for targeting the MAPK pathway in cancer therapy. Proceedings 22:10. doi: 10.3390/proceedings2019022010
Popovic, D., Vucic, D., and Dikic, I. (2014). Ubiquitination in disease pathogenesis and treatment. Nat. Med. 20, 1242–1253. doi: 10.1038/nm.3739
Rape, M. (2018). Ubiquitylation at the crossroads of development and disease. Nat. Rev. Mol. Cell Biol. 19, 59–70. doi: 10.1038/nrm.2017.83
Raschka, S., and Kaufman, B. (2020). Machine learning and AI-based approaches for bioactive ligand discovery and GPCR-ligand recognition. Methods 180, 89–110. doi: 10.1016/j.ymeth.2020.06.016
Rieser, E., Cordier, S. M., and Walczak, H. (2013). Linear ubiquitination: a newly discovered regulator of cell signalling. Trends Biochem. Sci. 38, 94–102. doi: 10.1016/j.tibs.2012.11.007
Ripphausen, P., Nisius, B., and Bajorath, J. (2011). State-of-the-art in ligand-based virtual screening. Drug Discov. Today. 16, 372–376. doi: 10.1016/j.drudis.2011.02.011
Ritchie, K. J., and Zhang, D. E. (2004). ISG15: the immunological kin of ubiquitin. Semin. Cell Dev. Biol. 15, 237–246. doi: 10.1016/j.semcdb.2003.12.005
Rodrigo-Brenni, M. C., and Morgan, D. O. (2007). Sequential E2s drive polyubiquitin chain assembly on APC targets. Cell 130, 127–139. doi: 10.1016/j.cell.2007.05.027
Rotin, D., Staub, O., and Haguenauer-Tsapis, R. (2000). Ubiquitination and endocytosis of plasma membrane proteins: role of Nedd4/Rsp5p family of ubiquitin-protein ligases. J. Membr. Biol. 176, 1–17. doi: 10.1007/s00232001079
Russo Spena, C., De Stefano, L., Poli, G., Granchi, C., El Boustani, M., Ecca, F., et al. (2019). Virtual screening identifies a PIN1 inhibitor with possible antiovarian cancer effects. J. Cell. Physiol. 234, 15708–15716. doi: 10.1002/jcp.28224
Sadowski, M., Suryadinata, R., Tan, A. R., Roesley, S. N. A., and Sarcevic, B. (2012). Protein monoubiquitination and polyubiquitination generate structural diversity to control distinct biological processes. IUBMB Life 64, 136–142. doi: 10.1002/iub.589
Saffari-Chaleshtori, J., Asadi-Samani, M., Rasouli, M., and Shafiee, S. M. (2020). Autophagy and ubiquitination as two major players in colorectal cancer: a review on recent patents. Recent Pat. Anticancer Drug Discov. 15, 143–153. doi: 10.2174/1574892815666200630103626
Shafique, S., Ali, W., Kanwal, S., and Rashid, S. (2018). Structural basis for Cullins and RING component inhibition: targeting E3 ubiquitin pathway conductors for cancer therapeutics. Int. J. Biol. Macromol. 106, 532–543. doi: 10.1016/j.ijbiomac.2017.08.047
Shahlaei, M. (2013). Descriptor selection methods in quantitative structure–activity relationship studies: a review study. Chem. Rev. 113, 8093–8103. doi: 10.1021/cr3004339
Shoichet, B. K. (2004). Virtual screening of chemical libraries. Nature 432, 862–865. doi: 10.1038/nature03197
Stahura, F. L., and Bajorath, J. (2005). New methodologies for ligand-based virtual screening. Curr. Pharm. Des. 11, 1189–1202. doi: 10.2174/1381612053507549
Sun, L., and Chen, Z. J. (2004). The novel functions of ubiquitination in signaling. Curr. Opin. Cell Biol. 16, 119–126. doi: 10.1016/j.ceb.2004.02.005
Sun, Y. (2005). Overview of approaches for screening for ubiquitin ligase inhibitors. Methods Enzymol. 399, 654–663. doi: 10.1016/S0076-6879(05)99043-5
Swatek, K. N., and Komander, D. (2016). Ubiquitin modifications. Cell Res. 26, 399–422. doi: 10.1038/cr.2016.39
Tenno, T., Fujiwara, K., Tochio, H., Iwai, K., Morita, E. H., Hayashi, H., et al. (2004). Structural basis for distinct roles of Lys63- and Lys48-linked polyubiquitin chains. Genes Cells 9, 865–875. doi: 10.1111/j.1365-2443.2004.00780.x
Thibaudeau, T. A., and Smith, D. M. (2019). A practical review of proteasome pharmacology. Pharmacol. Rev. 71, 170–197. doi: 10.1124/pr.117.015370
Tian, Y.-F., Chen, T.-J., Lin, C.-Y., Chen, L.-T., Lin, L.-C., Hsing, C.-H., et al. (2013). SKP2 overexpression is associated with a poor prognosis of rectal cancer treated with chemoradiotherapy and represents a therapeutic target with high potential. Tumour Biol. 34, 1107–1117. doi: 10.1007/s13277-013-0652-z
Tomasi, M. L., Tomasi, I., Ramani, K., Pascale, R. M., Xu, J., Giordano, P., et al. (2012). S-adenosyl methionine regulates ubiquitin-conjugating enzyme 9 protein expression and sumoylation in murine liver and human cancers. Hepatology 56, 982–993. doi: 10.1002/hep.25701
Uchida, C., and Kitagawa, M. (2016). RING-, HECT-, and RBR-type E3 ubiquitin ligases: involvement in human cancer. Curr. Cancer Drug Targets 16, 157–174. doi: 10.2174/1568009616666151112122801
Utomo, D. H., Widodo, N., and Rifa’i, M. (2012). Identifications small molecules inhibitor of p53-mortalin complex for cancer drug using virtual screening. Bioinformation 8, 426–429. doi: 10.6026/97320630008426
Voges, D., Zwickl, P., and Baumeister, W. (1999). The 26S proteasome: a molecular machine designed for controlled proteolysis. Annu. Rev. Biochem. 68, 1015–1068. doi: 10.1146/annurev.biochem.68.1.1015
Walters, W. P., Stahl, M. T., and Murcko, M. A. (1998). Virtual screening—an overview. Drug Discov. Today 3, 160–178. doi: 10.1016/S1359-6446(97)01163-X
Weissman, A. M. (2001). Themes and variations on ubiquitylation. Nat. Rev. Mol. Cell Biol. 2, 169–178. doi: 10.1038/35056563
Welchman, R. L., Gordon, C., and Mayer, R. J. (2005). Ubiquitin and ubiquitin-like proteins as multifunctional signals. Nat. Rev. Mol. Cell Biol. 6, 599–609. doi: 10.1038/nrm1700
Wu, K.-J., Zhong, H.-J., Li, G., Liu, C., Wang, H.-M. D., Ma, D.-L., et al. (2018). Structure-based identification of a NEDD8-activating enzyme inhibitor via drug repurposing. Eur. J. Med. Chem. 143, 1021–1027. doi: 10.1016/j.ejmech.2017.11.101
Wu, L., Grigoryan, A. V., Li, Y., Hao, B., Pagano, M., and Cardozo, T. J. (2012). Specific small molecule inhibitors of Skp2-mediated p27 degradation. Chem. Biol. 19, 1515–1524. doi: 10.1016/j.chembiol.2012.09.015
Xirodimas, D. P., Saville, M. K., Bourdon, J.-C., Hay, R. T., and Lane, D. P. (2004). Mdm2-mediated NEDD8 conjugation of p53 inhibits its transcriptional activity. Cell 118, 83–97. doi: 10.1016/j.cell.2004.06.016
Yang, C., Wang, W., Chen, L., Liang, J., Lin, S., Lee, M. Y., et al. (2016). Discovery of a VHL and HIF1α interaction inhibitor with in vivo angiogenic activity via structure-based virtual screening. Chem. Commun. 52, 12837–12840. doi: 10.1039/c6cc04938a
Yang, Y., Kitagaki, J., Dai, R.-M., Tsai, Y. C., Lorick, K. L., Ludwig, R. L., et al. (2007). Inhibitors of ubiquitin-activating enzyme (E1), a new class of potential cancer therapeutics. Cancer Res. 67:9472. doi: 10.1158/0008-5472.CAN-07-0568
Yousuf, Z., Iman, K., Iftikhar, N., and Mirza, M. U. (2017). Structure-based virtual screening and molecular docking for the identification of potential multi-targeted inhibitors against breast cancer. Breast Cancer (Dove Med. Press). 9, 447–459. doi: 10.2147/BCTT.S132074
Zhang, S., Tan, J., Lai, Z., Li, Y., Pang, J., Xiao, J., et al. (2014). Effective virtual screening strategy toward covalent ligands: identification of novel NEDD8-activating enzyme inhibitors. J. Chem. Inf. Model. 54, 1785–1797. doi: 10.1021/ci5002058
Zheng, N., and Shabek, N. (2017). Ubiquitin ligases: structure, function, and regulation. Annu. Rev. Biochem. 86, 129–157. doi: 10.1146/annurev-biochem-060815-014922
Zhong, H. J., Lee, B. R., Boyle, J. W., Wang, W., Ma, D. L., Hong Chan, P. W., et al. (2016). Structure-based screening and optimization of cytisine derivatives as inhibitors of the menin-MLL interaction. Chem. Commun. 52, 5788–5791. doi: 10.1039/c6cc01079b
Zhong, H. J., Leung, K. H., Lin, S., Chan, D. S. H., Han, Q. B., Chan, S. L. F., Ma, D. L. et al. (2015). Discovery of deoxyvasicinone derivatives as inhibitors of NEDD8-activating enzyme. Methods 71:71. doi: 10.1016/j.ymeth.2014.08.014
Zhong, H.-J., Liu, L.-J., Chan, D. S.-H., Wang, H.-M., Chan, P. W. H., Ma, D.-L., et al. (2014). Structure-based repurposing of FDA-approved drugs as inhibitors of NEDD8-activating enzyme. Biochimie 102, 211–215. doi: 10.1016/j.biochi.2014.03.005
Zhong, H.-J., Ma, V. P., Cheng, Z., Shiu-Hin Chan, D., He, H.-Z., Leung, K.-H., et al. (2012). Discovery of a natural product inhibitor targeting protein neddylation by structure-based virtual screening. Biochimie 94, 2457–2460. doi: 10.1016/j.biochi.2012.06.004
Zhong, H. J., Yang, H., Chan, D. S. H., Leung, C. H., Wang, H. M., and Ma, D. L. (2012). A Metal-based inhibitor of NEDD8-activating enzyme. PLoS One 7:e49574. doi: 10.1371/journal.pone.0049574
Zhou, M. J., Chen, F. Z., and Chen, H. C. (2014). Ubiquitination involved enzymes and cancer. Med. Oncol. 31:93. doi: 10.1007/s12032-014-0093-6
Keywords: ubiquitination regulator, virtual screening, drug discovery, cancer, treatment
Citation: Song Y-Q, Wu C, Wu K-J, Han Q-B, Miao X-M, Ma D-L and Leung C-H (2021) Ubiquitination Regulators Discovered by Virtual Screening for the Treatment of Cancer. Front. Cell Dev. Biol. 9:665646. doi: 10.3389/fcell.2021.665646
Received: 08 February 2021; Accepted: 15 March 2021;
Published: 12 May 2021.
Edited by:
Masashi Maekawa, Ehime University, JapanReviewed by:
Zhe-Sheng Chen, St. John’s University, United StatesCopyright © 2021 Song, Wu, Wu, Han, Miao, Ma and Leung. This is an open-access article distributed under the terms of the Creative Commons Attribution License (CC BY). The use, distribution or reproduction in other forums is permitted, provided the original author(s) and the copyright owner(s) are credited and that the original publication in this journal is cited, in accordance with accepted academic practice. No use, distribution or reproduction is permitted which does not comply with these terms.
*Correspondence: Chung-Hang Leung, ZHVuY2FubGV1bmdAdW0uZWR1Lm1v; Dik-Lung Ma, ZWRtb25kbWFAaGtidS5lZHUuaGs=
Disclaimer: All claims expressed in this article are solely those of the authors and do not necessarily represent those of their affiliated organizations, or those of the publisher, the editors and the reviewers. Any product that may be evaluated in this article or claim that may be made by its manufacturer is not guaranteed or endorsed by the publisher.
Research integrity at Frontiers
Learn more about the work of our research integrity team to safeguard the quality of each article we publish.