- 1Division of Molecular and Structural Preclinical Imaging, Department of Biomedical Imaging and Image-Guided Therapy, Medical University of Vienna, Vienna, Austria
- 2Medical Imaging Cluster, Medical University of Vienna, Vienna, Austria
- 3Core Facility Proteomics, Medical University of Vienna, Vienna, Austria
- 4Department of Experimental Pathology and Laboratory Animal Pathology, Medical University of Vienna, Vienna, Austria
- 5Christian Doppler Laboratory for Applied Metabolomics, Medical University of Vienna, Vienna, Austria
- 6Computational Imaging Research Lab, Department of Biomedical Imaging and Image-Guided Therapy, Medical University of Vienna, Vienna, Austria
- 7Division of Molecular and Gender Imaging, Department of Biomedical Imaging and Image-Guided Therapy, Medical University of Vienna, Vienna, Austria
- 8Division of Nuclear Medicine, Department of Biomedical Imaging and Image-Guided Therapy, Medical University of Vienna, Vienna, Austria
- 9Christian Doppler Laboratory for Molecular Stress Research in Peritoneal Dialysis, Department of Pediatrics and Adolescent Medicine, Medical University of Vienna, Vienna, Austria
- 10Division of Pediatric Nephrology and Gastroenterology, Department of Pediatrics and Adolescent Medicine, Comprehensive Center for Pediatrics, Medical University of Vienna, Vienna, Austria
- 11Unit of Laboratory Animal Pathology, University of Veterinary Medicine, Vienna, Austria
- 12CBmed GmbH-Center for Biomarker Research in Medicine, Graz, Austria
- 13Breast Imaging Service, Department of Radiology, Memorial Sloan Kettering Cancer Center, New York, United States
- 14Division of Breast Imaging, Department of Radiology, Columbia University Irving Medical Center (CUIMC), New York, United States
Preclinical high-field magnetic resonance imaging (MRI) systems offer a diverse array of MRI techniques, providing rich multiparametric MRI (mpMRI) platforms for studying numerous biological parameters. mpMRI platforms prove particularly indispensable when investigating tumors that exhibit profound intratumoral heterogeneity, such as breast cancer. A thoughtful comprehension of the origins of intratumoral heterogeneity is imperative for the judicious assessment of new targeted therapies and treatment interventions. Furthermore, when data from mpMRI are complemented with data from other in vivo imaging modalities, such as positron emission tomography (PET), and correlated with data from ex vivo modalities, such as matrix-assisted laser desorption imaging mass spectrometry (MALDI IMS), the in vivo parameters can be further elucidated at a molecular level and microscopic scale. Nevertheless, extracting meaningful scientific insights from such complex datasets necessitates the utilization of machine learning (ML) approaches to discern region-specific radiomic features. The development of correlative, multimodal imaging (CMI) workflows, such as one incorporating MRI, PET and MALDI IMS, is inherently challenging, given the many technological and methodological challenges related to multimodal data acquisition as well as the physiological limitations of the laboratory mice of the investigation. Standardization efforts in image acquisition and processing are required to increase the reproducibility and translatability of CMI data. To address the challenges of developing standardized CMI workflows and stimulate dialog regarding this area of need, we present a practical workflow to investigate tumor heterogeneity in breast cancer xenografts across various spatial scales. Our workflow entails simultaneous functional MRI and PET acquisitions in living mice, followed by correlation with post-imaging MALDI IMS and histologic data. Additionally, we propose data preprocessing steps for potential ML applications. We illustrate the feasibility of this workflow through two examples, showcasing its effectiveness in comparing in vivo and ex vivo images to evaluate tumor metabolism and hypoxia in mice with breast cancer xenografts.
1 Introduction
Breast cancer (BC) is the second most prevalent cancer in the world and is a leading cause of mortality in the female population. It is characterized by vast inter- and intratumoral heterogeneity which impedes the assignment of an exact histologic type to a given tumor or to differentiate the many genetic and molecular subpopulations within it. Moreover, hypoxia promotes the development of aggressive and treatment-resistant subpopulations of tumor cells within the tumor itself and in the surrounding tumoral microenvironment. Consequently, selecting the optimal treatment for the personalized treatment of BC remains challenging (Schmitz et al., 2016). In vivo imaging techniques such as positron emission tomography (PET), computed tomography (CT), and magnetic resonance imaging (MRI) can provide non-invasive whole-tumor information. However, none of these alone provides sufficient information on tumor heterogeneity. Therefore, hybrid imaging applications (e.g., combining PET and MRI) for imaging BC are constantly evolving (Romeo et al., 2023). In preclinical research, hybrid imaging can be even further expanded in the form of correlative multimodal imaging (CMI), taking advantage of easy access to whole-tumor and tissue samples and the ex vivo assessment of such samples by histological (e.g., multiplexed immunofluorescence) and molecular/spectrometric (e.g., matrix-assisted laser desorption/ionization imaging mass spectrometry—MALDI IMS) techniques. Since CMI allows the cross-validation of in vivo imaging techniques with histology at different spatial scales, it also allows the establishment of relationships between imaging biomarkers and underlying biological changes (Svirkova et al., 2018; Walter et al., 2020; Walter et al., 2021; Tuck et al., 2022).
Regarding in vivo imaging techniques, a number of MRI techniques are currently available on both preclinical and clinical high-field MRI systems. Such techniques provide physiological information that is especially useful for assessing tumor hallmarks within the tumor and the tumoral microenvironment. For example, chemical exchange saturation transfer (CEST) MRI allows indirect in vivo quantification of metabolites with exchangeable protons (e.g., protons present in hydroxyl groups). When applying several saturation pulses around the proton resonance frequency, the exchange rate between the exchangeable protons of an administered metabolite and tissue water can be measured (van Zijl and Yadav, 2011; Zaiss and Bachert, 2013). One such endogenous metabolite is the glucose derivative 2-deoxy-D-glucose, which enables the quantification of aerobic glycolysis within the tumor and within the tumoral microenvironment (Zaiss et al., 2019; Capozza et al., 2022). Blood oxygenation level-dependent (BOLD) MRI provides data on tissue oxygenation and tumor hypoxia. The BOLD signal originates from the fraction of deoxygenated hemoglobin in red blood cells, which creates local variations in magnetic susceptibility that increase the relaxation rate (R2* = 1/T2*). Conversely, oxygenated hemoglobin leads to an increase in the T2*-weighted signal. The ratio of oxygenated and deoxygenated hemoglobin creates a diagnostic contrast which can be exploited in BOLD-MRI examinations (Greve, 2011). Diffusion-weighted imaging (DWI) measures the random movement of water molecules (Brownian diffusion). If this movement is hindered by the presence of cellular membranes, the directional changes are reflected by a lower diffusion coefficient (D). D serves as a marker of extravascular microstructural diffusion within the tumor and within the tumoral microenvironment (Guadilla et al., 2018). Moreover, by using a specialized DWI approach called “intravoxel incoherent motion (IVIM) MRI”, the intravascular blood microcirculation of tissues can be visualized, allowing blood flow in capillaries to be monitored without the need for external contrast agents (Le Bihan, 2019). IVIM-MRI involves multiple MRI acquisitions at low diffusion weightings (b-values; b < 200 s/mm2) and with a segmented biexponential fit to quantify the diffusion signal. The resulting derived IVIM fraction (fIVIM) and perfusion coefficient (D*) display the fraction of microperfusion within a given (tumor) tissue as well as the amount of diffusion within tumor microvessels and therefore provide information on tumor angiogenesis (Iima, 2021; Bartsch et al., 2024). Finally, dynamic contrast-enhanced (DCE)-MRI assesses tissue vascularity via the administration of gadolinium-based, T1-shortening contrast agents. A series of fast T1-weighted image acquisitions are used to measure the uptake kinetics of the contrast agent. The volume fraction of plasma in the tissue (vp), the extravascular, extracellular volume fraction (ve), and the transfer constant (ktrans) of the contrast agent are derived from DCE-MRI using a compartmental modeling approach (Sourbron and Buckley, 2013; Barnes et al., 2015). The combined analysis of these three parameter maps is currently considered the gold standard for the assessment of tumor vascularization.
Combining in vivo MRI techniques with additional complementary in vivo techniques, such as PET, is appealing for obtaining holistic information about a tumor (Marino et al., 2018). Moreover, in preclinical research, CMI can be achieved by performing spatial mapping of imaging parameters from simultaneous PET and MRI acquisitions with pathological features obtained from ex vivo techniques like MALDI IMS. This provides spatial visualization of differentially expressed proteins, allowing the characterization of tissue composition and pixel-wise co-registration and correlation of proteomic and radiomic information. The proteomic ex vivo protocol includes washing steps, in situ trypsin digestion, matrix application, and mass spectrometry signal acquisition. During MALDI IMS acquisition, singly charged ions are accelerated in an electric field and transferred to a time-of-flight (TOF) mass analyzer. Ions of the same charge acquire the same kinetic energy, such that lighter ions reach the detector faster than heavier ones (Glish and Vachet, 2003). MALDI IMS datasets hold vast histoproteomic data, necessitating computational techniques for data condensation, image formation, and statistical scrutiny.
Machine learning (ML) is invaluable for bridging in vivo and ex vivo techniques due to its ability to unveil patterns beyond human perception (Erickson et al., 2017). For the assessment of medical images, machine learning can be combined with radiomics, a method that involves calculating diverse image features within a region of interest (ROI) to predict cancer subtypes or treatment response after rigorous feature selection (Conti et al., 2021). Although deep learning, a subset of ML, obviates the need for predefined regions or feature selection, data preparation is crucial. This entails aligning, co-registering, and sampling images to uniform pixel dimensions, as well as segmenting regions of interest for radiomics. Tools like advanced normalization tools (ANTs) (Avants et al., 2009) aid in co-registration and are often implemented in Python alongside various Python libraries like “scikit-image” (van der Walt et al., 2014), SimpleITK (Lowekamp et al., 2013), and “torchvision” (www.pytorch.org) for image processing.
Despite the technological advancements and opportunities presented by CMI and ML pipelines for analyzing CMI data, establishing preclinical CMI workflows is inherently difficult due to technical, methodological, and biological challenges arising from the acquisition of data across various methods, modalities, and spatial scales. Ensuring data reproducibility and translatability necessitates the identification of influential factors in the CMI workflow and implementing measures to mitigate their variability. This entails the standardization of preclinical image data acquisition procedures and postprocessing workflows, coupled with the transparent reporting of these parameters. Recent multicenter studies in preclinical PET (Mannheim et al., 2019) and functional MRI (Grandjean et al., 2020) demonstrate the importance of standardized workflows in light of their impact on study results and data interpretation.
To showcase some of the challenges of developing standardized CMI workflows and to stimulate dialog regarding this area of need, we describe in detail a practical workflow involving (a) the performance of simultaneous in vivo PET/MRI in tumor-bearing mice, followed by (b) the correlation of in vivo PET/MRI images with ex vivo MALDI IMS and histologic data, and finally (c) the preprocessing of multimodal data for ML applications (Figure 1). To demonstrate the feasibility of the workflow, we applied it to preclinical research in BC xenograft-bearing mice for (1) assessing changes in glucose metabolism in tumors using simultaneous glucoCEST MRI and [18F]fluorodeoxyglucose ([18F]FDG)-PET and (2) regional mapping of hypoxia and hypoxia-induced tumor angiogenesis using simultaneous mpMRI and [18F]fluoromisonidazole ([18F]FMISO)-PET.
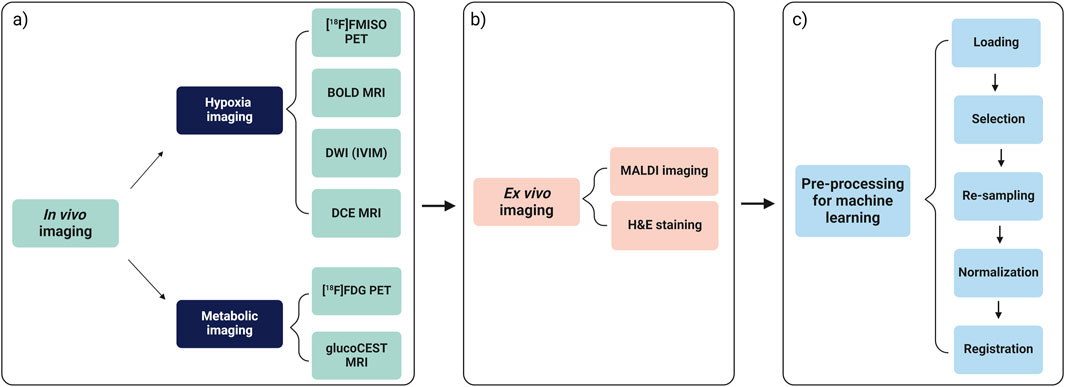
Figure 1. Overview of the proposed workflow consisting of (A) steps to acquire simultaneous PET/MRI for metabolic imaging and hypoxia imaging in breast cancer xenografted mice, followed by (B) steps to perform ex vivo MALDI IMS and (C) data preprocessing steps of multimodal data for potential ML applications.
2 Material & methods
2.1 General
2.1.1 Chemicals
All cell culture media, phosphate buffered saline (PBS), and fetal bovine serum (FBS) were purchased from Sigma-Aldrich Handels GmbH (Vienna, Austria) or Gibco (Fisher Scientific GmbH, Schwerte, Germany) and were used without further purification. Estrogen pellets were obtained from Innovative Research of America (Sarasota, FL, United States). We purchased 2-deoxy-D-glucose as a CEST contrast agent from Sigma-Aldrich (Merck KGaA, Darmstadt, Germany). Isoflurane was obtained from Zoetis Österreich GmbH (Vienna, Austria). [18F]FDG was obtained from the in-house radiopharmacy unit of the Medical University of Vienna and diluted for administration into animals with 0.9% (w/v) aqueous saline solution. For this study, [18F]FMISO was synthetized via cassette-based radiosynthesis (FASTlab PET-FMISO Cassette, GE Healthcare Handels GmbH, Vienna, Austria) on a commercial synthesis module (GE Healthcare, Chicago, IL, United States) with a radiochemical purity of >96% following established procedures (Kniess et al., 2023).
Gelatin from bovine skin, ammonium bicarbonate, trifluoroacetic acid (TFA), potassium sulfate (K2SO4), acetonitrile (ACN), red phosphorus, and α-Cyano-4-hydroxycinnamic acid (HCCA) were purchased from Sigma-Aldrich Handels GmbH (Merck Life Science, Darmstadt, Germany). Carnoy’s solution consisted of absolute ethanol (EtOH)–chloroform –acetic acid at a 6:3:1 ratio; all chemicals for this solution were acquired from Sigma-Aldrich Handels GmbH. Trypsin/Lys-C Mix, Mass Spec Grade was sourced from Promega GmbH (Madison, WI, United States). Hematoxylin according to Mayer and 1% eosin (aqueous solution) were procured from Morphisto GmbH (Frankfurt, Germany).
2.1.2 Generation of BC xenografts
BC cells of luminal A (MCF-7), HER2+ (SKBR-3), and triple negative (MDA-MB-231) subtypes were purchased from the American Type Culture Collection (Manassas, VA, United States) and kept under standardized conditions in a humidified incubator at 37°C in an atmosphere of 5% CO2. MCF-7 cells were cultivated in RPMI medium, while SKBR-3 and MDA-MB-231 cells were cultivated in Dulbecco’s Modified Eagle Medium (DMEM), both supplemented with 10% FBS. To generate BC xenografts, female athymic BALB/c-derived nude mice were purchased at age 4–6 weeks from Charles River Laboratories (Wilmington, MA, United States). Mice were housed in groups of 7–10 individuals in a temperature-controlled facility on a 12-h light/dark cycle with water and a standard laboratory diet provided ad libitum. An acclimatization period of ≥2 weeks was allowed before mice were used in experiments. One week prior to the inoculation of MCF-7 cells, estrogen pellets (0.36 mg/day, 60-day release, Innovative Research of America, Sarasota, FL, United States) were implanted subcutaneously into the neck region of isoflurane-anesthetized mice via a 10G precision trocar to support luminal A tumor growth. Thereafter, the mice were inoculated under anesthesia with 1 × 107 MCF-7 cells, suspended in FBS-free RPMI medium, in their right flank. Alternatively, for the generation of HER2+ and triple negative BC xenografts, the mice were inoculated under anesthesia in their right flank with 3 × 106 SKBR-3 or 3 × 106 MDA-MB-231 cells, suspended in PBS. Tumors were grown for 14–21 days until reaching a size of 650–1,000 mm3—suitable for imaging studies. All studies involving laboratory research animals were approved by the Austrian Federal Ministry of Education, Science and Research [66.009/0284-WF/V/3b/2017; 2020–0.363.124; 2022–0.726.820] and the Intramural Committee for Animal Experimentation of the Medical University of Vienna. Study procedures were in accordance with the European Community’s Council Directive of 22 September 2010 (2010/63/EU), and all data reported in the study adhered to ARRIVE (Animal Research: Reporting of In Vivo Experiments) guidelines.
2.1.3 PET/MRI scanner hardware
All PET/MRI scans were performed using a 9.4 T Bruker Biospin 30/94 USR preclinical MRI system equipped with a PET insert (Bruker, Ettlingen, Germany) combined with a BGA 20 gradient system and a PET-optimized 35-mm 1H volume coil. To perform PET and MRI simultaneously, all scans were acquired with the PET insert installed. The PET insert Si 198 (Bruker, Ettlingen, Germany) consisted of three rings of detector blocks. Each ring was arranged with eight monolithic LYSO crystals (50 mm × 50 mm × 10 mm³), coupled to an array of 12 × 12 silicon photomultipliers (SiPMs), resulting in a total of 144 units used for projection readout. The field of view (FOV) was fixed at 90 mm × 90 mm × 150 mm. The system was operated using the ParaVision v360.3 software suite (Bruker, Ettlingen, Germany).
2.2 Metabolic imaging using simultaneous [18F]FDG-PET and glucoCEST MRI
2.2.1 General [18F]FDG-PET/glucoCEST MRI protocol
The proposed protocol of simultaneous glucoCEST MRI and [18F]FDG-PET for the assessment of changes in glucose metabolism is shown in Figure 2. Animals were anesthetized, canulated, intraperitoneally administered with [18F]FDG, and positioned into the MRI gantry. According to the proposed protocol, scanning began with the acquisition of T2-weighted images to serve as anatomical references, and then glucoCEST MRI acquisitions began with the initial acquisition of a T1-weighted map followed by repeated cycles of a modified glucoCEST sequence (Section 2.2.2). Simultaneously with glucoCEST acquisition and 50 min after [18F]FDG administration, a 60-min dynamic [18F]FDG-PET acquisition was initiated, during which 150 µL of 2-deoxy-D-glucose at a dose of 0.5 g/kg as a glucoCEST MRI contrast agent in 0.9% (w/v) aqueous saline solution was injected. Taking the animal´s body weight into account during the preparation of the 2-deoxy-D-glucose solution, the concentration of 2-deoxy-D-glucose was 0.130 g/mL for mice up to a body weight of 25 g and 0.150 g/mL for mice up to a body weight of 30 g. We remotely infused 2-deoxy-D-glucose in three steps: 0.04 mL at an initial rate of 0.03 mL/min to displace residual saline in the intravenous catheter, followed by 0.06 mL at 0.015 mL/min and 0.05 mL at a rate of 0.03 mL/min. Splitting the infusion of 2-deoxy-D-glucose into two stages enhanced the tolerability of the animals while still enabling rapid signal accumulation for effective glucoCEST MRI measurements. The total anesthesia time for the animals for the whole imaging protocol was 120 min (10 min preparation and 110 min scan activities).
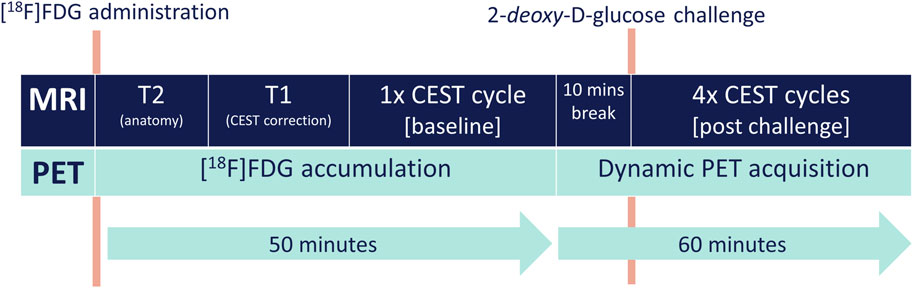
Figure 2. General overview of metabolic imaging using glucoCEST MRI and [18F]FDG-PET in breast cancer xenografted mice.
2.2.2 Animal preparation and [18F]FDG-PET/glucoCEST MRI parameters
Animals were positioned in an induction box, and anesthesia was initiated using 3% isoflurane mixed into medical air as a carrier gas. Then, under continued anesthesia, animals were repositioned on a heated pad (37°C), and a 10-cm catheter equipped with a 30G needle and filled with heparinized 0.9% (w/v) aqueous saline solution was inserted into their lateral tail vein. Including the needle connectors, the volume of tail vein catheters was approximately 50 µL. Finally, the animals were repositioned on the MRI cradle, and a respiratory pad was placed below them to monitor their breathing rate throughout [18F]FDG PET/MRI (SA Instruments Inc., Stony Brook, NY, United States). Additionally, the tail vein catheter was connected to a 1-mL syringe mounted on a remote-controlled infusion pump (PHD 2000, Harvard Apparatus, Holliston, MA, United States) via a 2-m long administration tubing line with an inner diameter of 0.25 mm (Tycon® Flexible Plastic Tubing, Compagnie de Saint-Gobain, Courbevoie, France) and prefilled with a solution of 2-deoxy-D-glucose. Before commencing scanning, 100–150 µL of [18F]FDG solution containing 10.54 ± 3.10 MBq of activity was injected intraperitoneally using an insulin syringe. During scanning, the isoflurane concentration levels were adjusted (range: 0.5%–1.5% (v/v)) to maintain a respiratory rate of 60–80 breaths per min.
Following scout image acquisition, T2-weighted anatomical images were acquired using a RARE sequence (repetition time [TR]: 2,000 ms; echo time [TE]: 35.0 ms; spatial resolution: 0.102 mm × 0.107 mm; matrix size: 320 × 320; number of slices: 10; slice thickness: 1 mm; acquisition time [TA]: 1 min 20 s). Then, also using a RARE sequence, a T1-weighted map was acquired to correct for T1 effects in image postprocessing in the axial slice with maximal tumor diameter but minimal necrosis based on visual inspection of T2-weighted anatomical images (variable TR [VTR]: 867–6,000 ms; TE: 28 ms; spatial resolution: 0.234 mm × 0.234 mm; matrix size: 128 × 128; FOV: 30 mm × 30 mm; slice thickness: 1 mm; TA: 4 min 27 s). Subsequently, glucoCEST MRI acquisitions were performed based on the sequence described in Villano et al. (2021) but modified to operate using the scanner console software ParaVision v360.3. A single image slice Z-spectrum (CEST-spectrum) in the same position as the T1 map was acquired, including 120 frequency offsets between 6 and −6 ppm from water protons (TR: 3.000 ms; TE: 4.3 ms; spatial resolution: 0.234 mm × 0.234 mm; FOV 30 mm × 30 mm; matrix size: 128 × 128; saturation pulse strength: 3 μT; interval: 0.1 ppm; slice thickness: 1 mm; TA: 7 min). To correct for shifts in water resonance frequency (due to B0 drift), water saturation shift referencing (WASSR) correction was used (Lim et al., 2014). WASSR correction measurements with 30 images of frequency offsets between 1.5 ppm and −1.5 ppm were repeated prior to each measurement of the Z-spectrum (TR: 3,313 ms; TE: 4.3 ms; spatial resolution: 0.234 mm × 0.234 mm; matrix size: 128 × 128; FOV: 30 mm × 30 mm; saturation pulse strength: 0.2 µT; interval: 0.1 ppm; slice thickness: 1 mm; TA: 2 min). Meanwhile, following baseline glucoCEST MRI, a 60-min dynamic [18F]FDG-PET acquisition with an energy window of 358–664 keV and a timing window of 7 ns was initiated. Prior to the onset of the 2-deoxy-D-glucose challenge, 10 min of baseline [18F]FDG-PET data were acquired. After the final step in 2-deoxy-D-glucose infusion, four additional repeated cycles of the glucoCEST MRI sequence were performed to monitor changes in glucoCEST MRI-derived parameters over time.
2.2.3 Image postprocessing and analysis
For glucoCEST image postprocessing and analysis, T2-weighted anatomical images were exported as DICOM files from ParaVision v360.3, while glucoCEST MRI images and corresponding T1 parameter maps were exported as raw imaging files (*.2Dseq). GlucoCEST MRI images were analyzed in MATLAB (version R2018a) using a code published previously in Zaiss and Bachert (2013). After WASSR correction involving fitting the WASSR Z-spectrum with spline functions on a pixel-wise basis and aligning the measured Z-spectra to the water resonance frequency at 0 ppm frequency offset, magnetization transfer ratio asymmetries (MTRasym) were quantified using the formula:
where
For [18F]FDG-PET image postprocessing and analysis, [18F]FDG-PET images were reconstructed using the maximum likelihood expectation maximization (MLEM) algorithm (isotropic resolution: 0.5 mm; 18 iterations; corrections: scatter, randoms decay; image size: 180 × 180 × 300; FOV: 90 mm × 90 mm × 150 mm) in ParaVision v360.3. [18F]FDG-PET images were split into 20 timeframes of 3 min to generate a dynamic [18F]FDG-PET dataset. [18F]FDG activity concentrations given in kBq/cc were transformed into standardized uptake values (SUVs) by taking the injected activity and body weight of the animals into account, yielding SUVbw. [18F]FDG-PET images were then exported as DICOM files from ParaVision v360.3. The exported [18F]FDG-PET images were analyzed using the “Fusion” module in pmod (version 4.3, PMOD Technologies LLC, Switzerland). [18F]FDG-PET images were co-registered with T2-weighted anatomical reference images using rigid matching. Three-dimensional volumes of interest (VOIs) covering the whole tumor were defined on T2-weighted anatomical images and loaded onto [18F]FDG-PET images to derive time–radioactivity concentration curves expressed in units of SUVbw. To correlate findings from [18F]FDG-PET with findings from the single-slice glucoCEST MRI, a separate two-dimensional ROI from the corresponding glucoCEST-MRI section was transferred to the respective plane of the PET image.
2.3 Hypoxia imaging using simultaneous [18F]FMISO-PET and mpMRI
2.3.1 General [18F]FMISO-PET/mpMRI protocol
The proposed protocol of simultaneous mpMRI and [18F]FMISO-PET for quantifying hypoxia and hypoxia-induced angiogenesis is shown in Figure 3. According to the proposed protocol, BOLD-MRI and [18F]FMISO-PET acquisitions were conducted under normoxic and hyperoxic conditions by elevating the isoflurane carrier gas levels from normal air (21% oxygen) to 100% oxygen, respectively, with the aid of an air–oxygen blender between the individual scans. Additionally, IVIM-MRI and DCE-MRI were performed. Scanning began 1 h after the animals were intravenously administered with [18F]FMISO. Following [18F]FMISO administration, they were anesthetized, canulated, positioned into the MRI gantry, and T2 anatomical MRI images were acquired. Subsequently, a BOLD T2*map was acquired under normoxic conditions followed by a simultaneous IVIM-MRI and the first 10-min static PET scan. Thence, the oxygen level was increased to 100% followed by a 10-min equilibration phase after which simultaneous BOLD and PET acquisitions were performed. Finally, DCE-MRI scans were conducted, during which 30 µL of 0.2 mM/kg gadopentetate-dimeglumin (Magnevist®, Bayer Vital GmbH, Leverkusen, Germany) were intravenously administered. The overall protocol required two intravenous administrations for which both lateral tail veins of the animals (one for [18F]FMISO administration; the contralateral one for contrast agent administration) were used. Total anesthesia time for the animals for the whole imaging protocol was 120 min (10 min preparation time and 110 min scan activities).
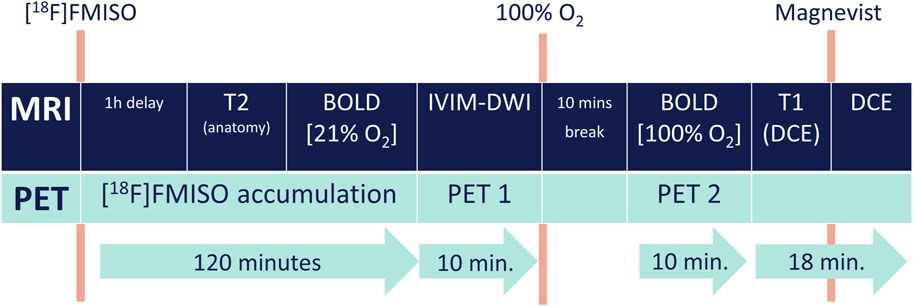
Figure 3. General overview of metabolic imaging using mpMRI and [18F]FMISO-PET in breast cancer xenografted mice.
2.3.2 Animal preparation
On the day of imaging, the animals were placed in an induction box and anesthesia was initiated using 3% isoflurane mixed into medical air as a carrier gas. Then, under continued anesthesia, they were repositioned on a heated pad (37°C), and a catheter equipped with a 30G needle was inserted into their lateral tail vein. Subsequently, 100 µL [18F]FMISO solution containing 15.5 ± 3.09 MBq of initial activity was administered via the tail vein over approximately 30 s. The catheter was flushed using 50 µL saline solution to minimize residual activity. Following tracer administration, the animals were placed back into their cage with water and standard laboratory nutrition provided ad libitum. Approximately 50 min after radiotracer injection, they were anesthetized again and prepared for scanning. Another tail vein catheter was placed into the contralateral tail vein for the administration of contrast agent for DCE-MRI. Afterwards, the animals were repositioned on the MRI cradle, with the tail vein catheter connected to a 1 mL syringe mounted on a remote-controlled infusion pump prefilled with 0.9% (w/v) saline solution.
2.3.3 BOLD-MRI
Following scout and T2-weighted anatomical image acquisition (TR: 2000 ms; TE: 35.0 ms; spatial resolution: 0.234 mm × 0.234 mm; matrix size: 128 × 128; FOV: 30 mm × 30 mm; number of slices: 10; slice thickness: 1 mm; TA: 1 min 20 s), baseline BOLD measurements at 21% oxygen as an anesthetic carrier gas were conducted. For this, a T2* map was acquired using a multi-gradient-echo sequence (TE: 14 echoes between 2.8 and 34.4 ms; TR: 500 ms; repetitions: 3; flip angle [FA]: 15⁰; spatial resolution: 0.234 mm × 0.234 mm; matrix size 128 × 128; FOV: 30 mm × 30 mm; number of slices: 10; slice thickness: 1 mm; TA: 2 min 24 s). The fraction of oxygen delivered to the animal was modulated by an air–oxygen blender (Sensor Medics Corporation, Yorba Lina, CA, United States). “Challenged” BOLD measurements were conducted using identical sequence parameters 10 min after switching to 100% oxygen.
2.3.4 IVIM-MRI
Following baseline BOLD acquisition, IVIM-MRI using 14 diffusion weightings, i.e., b-values, was performed to assess tumor microvascularity. Echo-planar imaging (EPI) sequences were acquired using the following parameters: TE: 24.08 ms; TR: 1,500 ms; spatial resolution: 0.313 mm × 0.313 mm; matrix size: 96 × 96; FOV: 30 mm × 30 mm; readout segments: 24; bandwidth: 87,719 Hz; number of slices: 10; slice thickness: 1 mm; b-values: 20, 40, 60, 80, 100, 120, 140, 160, 180, 200, 500, 800, 1,000 s/mm2 [max. gradient duration 5 ms and gradient separation 11.12 ms]; TA: 8 min 24 s.
2.3.5 DCE-MRI
DCE-MRI was performed to assess the microvascular parameters vp, ve, and ktrans. To account for tissue T1 independent of the administration of the contrast agent, a single-slice T1 mapping in the slice with maximal tumor diameter and minimal necrosis based on visual inspection of T2-weighted anatomical images was performed before the administration of the contrast agent (TE: 28 ms; VTR: 200–6,000 ms in 12 steps; spatial resolution: 0.234 mm × 0.234 mm; matrix size: 128 × 128; FOV 35 mm × 35 mm; slice thickness: 1 mm; TA: 6 min 50 s). Magnevist served as the contrast agent, where a bolus injection of 0.2 mM/kg Magnevist was administered via the tail vein catheter at a flow rate of 600 μL/min and an injection volume of 150 µL (including 30 µL 0.2 mM/kg Magnevist and 120 µL physiological saline solution to flush the catheter) using a remote-controlled syringe pump. In DCE-MRI, a fast FLASH sequence (TE: 83 ms; TR: 10 ms; number of repetitions: 600; FA: 15⁰; acquisition time: 10 min) with a time resolution of approximately 1 s per image and the same position as the T1 mapping was used, with the contrast agent being administered 1 min after baseline acquisition.
2.3.6 [18F]FMISO-PET
Two hours following the intravenous administration of [18F]FMISO, 10-min static PET acquisition was initiated with an energy window of 358–664 keV and a timing window of 7 ns. By the end of a 10-min break, during which the amount of oxygen in the anesthetic gas was increased to 100% for BOLD-MRI challenged measurements, a second PET acquisition using identical parameters was initiated.
2.3.7 Image postprocessing and analysis
Figure 4 provides an overview of [18F]FMISO-PET/mpMRI image postprocessing. T2-weighted anatomical images were exported as DICOM files from ParaVision v360.3.
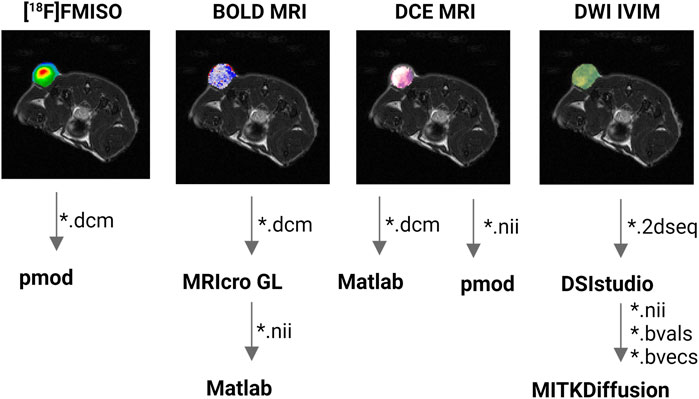
Figure 4. General overview of the postprocessing of PET/MRI data. Segmented tumors on PET images and MRI parameter maps are masked onto T2-weighted anatomical reference images. File formats in which image data are imported into the respective image analysis software application are specified next to the arrows in the figure (*.dcm, DICOM image format; *.nii, NIfTI image format; *.2dseq, Bruker raw data format; *.bvals, b-value file in FSL datasets; *.bvec, b-vector file in FSL datasets).
For BOLD-MRI, T2* maps were calculated using the image sequence analysis tool built into the ParaVision v360.3 software suite. For further processing, the T2* maps were exported as DICOM files from ParaVision v360.3 and converted to NIfTI files using the conversion tool in MRIcro-GL (version 1.2.20210317). Subsequently, the T2* maps were analyzed using in-house MATLAB code (MATLAB version R2018a) as follows. First, T2* maps, which were originally calculated in milliseconds, were converted into seconds. Then, the transverse relaxation rate
where
For IVIM-MRI, Bruker raw data (*.2dseq) were loaded into DSI studio software (version 10/2020) and converted into an FMRIB Software Library (FSL) dataset, consisting of a *.nii file with the image data, a *.bval file containing a list of b-values, and a *.bvec file containing the exact diffusion vectors used for IVIM-MRI acquisition. These files were further processed using the IVIM module built into the MITK diffusion software package (version 4.13.2, Deutsches Krebsforschungszentrum, Heidelberg, Germany). A segmented fitting model was used to calculate parameter maps for the IVIM-related perfusion coefficient (D*) and IVIM fraction (fIVIM), as well as the diffusion coefficient (D). The calculations of D* and fIVIM were based on diffusion-weighted images acquired at b-values below 500 s/mm2.
For DCE-MRI, the Kinetic module of the pmod software package (version 4.303) was used. Each pixel was treated as a separate region in the PKIN module to allow pixel-wise model fitting. For quantitative analysis, a population-based arterial input function (AIF), quantified from a separate cohort of four animals using the same bolus administration protocol, was used in all specimen as a standardized reference of Magnevist uptake. A one-tissue, two-compartment model was calculated, and the corresponding parameters vp, ktrans, and ve for each pixel of the imaging slice location were extracted as a *.csv file with each row representing a single pixel and each column representing corresponding DCE parameter values. To generate DCE parameter maps, *.csv files were loaded into a MATLAB routine developed in-house. The script transformed each column into a matrix of 128 × 128 cells and saved them as a *.nii file for further analyses.
For [18F]FMISO-PET, images were reconstructed as one-frame static images using the MLEM algorithm (isotropic resolution: 0.5 mm; 18 iterations; corrections: scatter, randoms, decay; image size 180 × 180 × 300; FOV 90 mm × 90 mm × 150 mm) in ParaVision v360.3. The reconstructed [18F]FMISO PET images were exported as DICOM files from ParaVision v360.3 and analyzed using the Fusion module in pmod (version 4.303). PET images were aligned to T2-weighted anatomical images by rigid matching to define tumor VOIs. In addition to a three-dimensional VOI, a second ROI was drawn in the same slice position as in the single-slice acquisition of DCE-MRI. Summary statistics on SUVbw values were extracted for further analysis.
2.4 Ex vivo imaging of BC specimens
2.4.1 Resection of BC xenografts and preparation for ex vivo imaging
Figure 5 shows a detailed depiction of the tumor resection protocol in preparation for ex vivo imaging. Following in vivo PET/MRI, animals were sacrificed by intravenous injection of 300 mg/kg pentobarbital (Release®, WDT, Garbsen, Germany). To retain the tumor orientation for subsequent co-registration, we applied the following protocol to all specimens. The skin surrounding the tumor was removed and the tumor orientation in situ was labeled on the right and dorsal side with different colored tissue dyes (Thermo Fisher Scientific, Waltham, MA, United States). Subsequently, the tumor was resected, and its left side was marked with another color of tissue dye. To prepare the tumor for MALDI IMS and subsequent hematoxylin and eosin (H&E) staining, tumor specimens were cut in half. A T2-weighted anatomical image was used as a reference for the cutting position so that the cutting plane most likely reflects the slice of the corresponding single-slice glucoCEST-MRI or DCE-MRI images (Supplementary Figure S1). The rostral half of the tumor was embedded in 10% (v/v) gelatin and stored at −80°C. The caudal half was fixed in 4% formalin and stored in 70% (v/v) ethanol at 4°C for long-term storage before paraffin-embedding.

Figure 5. Preparation of breast cancer xenograft tissue probes for ex vivo analysis. Following the cultivation of MCF-7 (luminal A), SKBR-3 (HER2+), and MDA-MB-231 (triple negative) cells, female athymic BALB/c nude mice were inoculated, and tumors were allowed to grow for 14–21 days. Mice were sacrificed after MRI/PET examinations, and tumors were resected. Resected tumors were labeled using three different colors of tissue dye on the left, right, and dorsal sides, and then cut along the same axial slice as the slice measured in the corresponding glucoCEST MRI or DCE-MRI images. The anterior half was embedded in gelatine and stored at −80°C, and the posterior half was embedded for 24 h in formaldehyde and then transferred into 70% ethanol for storage prior paraffin embedding.
2.4.2 MALDI IMS
The proposed MALDI IMS protocol is depicted in Figure 6. According to this protocol, one section of each tumor was cut at 10-μm thickness using a cryostat (CM3050S, Leica Microsystems, Wetzlar, Germany) and thaw-mounted onto indium-tin-oxide-coated conductive slides (Bruker Daltonics, Bremen, Germany). Thence, Carnoy´s washing procedure was immediately used, which included the following steps: 70% EtOH, 100% EtOH, Carnoy´s solution, 0.2% TFA, and 100% EtOH. After washing, the slides were dried in a desiccator for 30 min and then scanned at a resolution of 3,200 dpi (Epson Scan; Image Scanner III) prior to MALDI IMS to enable accurate co-registration. Trypsin was dissolved in 20 mM ammonium bicarbonate/0.01% glycerol to a final concentration of 0.025 μg/μL and applied in 20 layers using a HTX M5 sprayer (HTX Technologies, Chapel Hill, United States). After enzyme deposition, the tissue was incubated in saturated K2SO4 solution for 16 h. Finally, the tissue was coated with six layers of α-cyano-4-hydroxycinnamic acid (5 mg/mL in 50% ACN/0.3% TFA).
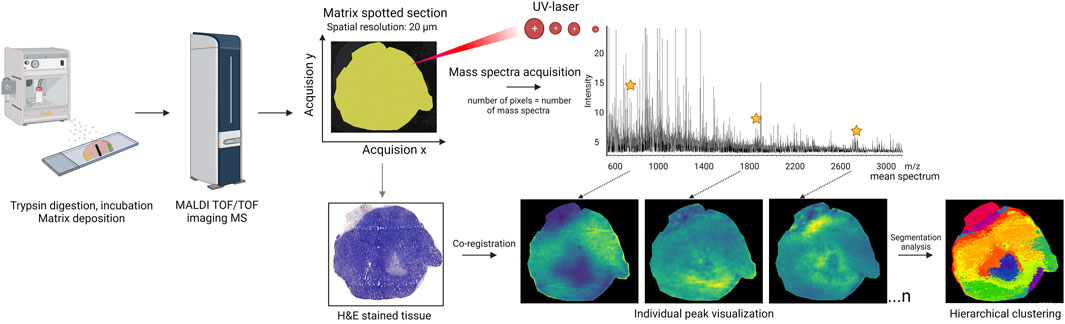
Figure 6. MALDI-IMS ex vivo analysis. MALDI-IMS sample processing includes sectioning and mounting the tissue section onto slides. Both trypsin and matrix solutions were homogenously sprayed over the tissue. Using FlexImaging software (Bruker Daltonics), ROIs on the tissue sections were created, and the number of pixels within the ROIs was calculated. A laser systematically irradiated the specified ROI in a raster pattern, producing a unique mass spectrum for every measurement spot. For each ROI, an aggregate spectrum was compiled. Detected mass signals, characterized by mass-to-charge ratio (m/z), are illustrated as color intensity maps, or ion images, which correlate molecular patterns with specific cell types. Finally, the collected mass spectra underwent hierarchical clustering analysis, offering a comprehensive and intuitive view of the multidimensional data.
MALDI IMS TOF/TOF measurements were obtained using a mass spectrometer (RapifleX™ MALDI Tissuetyper™, Bruker Daltonics, Bremen, Germany) at spatial resolutions of 20 μm, 200 Hz, and 200 accumulated laser shots per pixel. Red phosphorus was used to calibrate the instrument prior to all measurements. Tissue sections were measured in the positive ion mode with a mass range of 700–3,000 m/z. Ions were detected with a digitization rate of 0.63 GS/s in reflectron mode. After measurements were obtained, slides were stored in a desiccator at 4°C until staining with H&E.
Mass spectrometer measurements were imported and analyzed with SCiLS Lab software (version 2023c, Bruker Daltonics) using the Data Import wizard and selecting a preference for the reduced spectra format (.dat). For baseline removal, the convolution algorithm and Savitzky–Golay smoothing (width 0.005 m/z, one cycle) in SCiLS Lab were applied. Total ion count (TIC) normalization was selected to normalize the datasets with ±0.1 as an interval width. To identify relevant peaks, an orthogonal matching persuit algorithm for peak detection in individual spectra was used. The “Move Peaks to Local Max Tool” function was utilized to create m/z intervals where each peak center was individually marked by its maximum intensity. Matrix-related signals were identified and removed by correlation analysis to the matrix peaks (peaks with a Pearson correlation coefficient of ≥0.5 were considered matrix-related). This provides background and noise removal, and therefore enormous data reduction, as the original files from Rapiflex are tens of gigabytes, whereas these pre-processing steps more than halve the file size.
Subsequently, segmentation based on hierarchical clustering was used to distinguish between different regions. This approach grouped the most similar spectra (Pearson correlation as a distance metric, combined with an average linkage algorithm), thus identifying heterogeneous regions within morphologically homogeneous sections of BC xenograft tissue without needing a predefined number of clusters. MALDI IMS datasets were exported to the vendor-neutral imzML file format which contained a large number of mass spectra for producing ion images for the whole slide as well as for extracting individual spectra for any coordinate. We selected 30 of the most intense ions belonging to the tumor region (after TIC normalization) as exemplary peaks and exported them as a peak list.
After MALDI IMS analysis, the remaining matrix was removed with 70% ethanol, and slides were stained according to a standard H&E protocol and scanned using a Vectra Polaris imaging system (Akoya Biosciences, MA, United States) at a resolution of 0.25 μm/pixel. The histopathological evaluation of the tissue was performed by an expert pathologist, as our aim was to target heterogeneity within the tumor and exclude mouse-specific tissues, such as muscle cut out with the tumor. Furthermore, the Rapiflex settings did not allow for precise ROI definition. Tissue annotation was performed in QuPath and then imported into SCiLS Lab for co-registration, ensuring the elimination of irrelevant spectra around the tissue (see Supplementary Figures S2, S3 for a detailed explanation).
Therefore, tissue region margins were annotated using QuPath (version 0.3.2.) and ROIs were imported to SCiLS Lab. The co-registration process of the annotated H&E scan to the MALDI imaging images defines the precise location and orientation of the imported image within the global coordinate system of the data set. This involves defining the image orientation relative to the reference image, followed by accurate image co-registration.
H&E-stained bright-field images of the tissue sections were overlaid with MALDI IMS data in SCiLS Lab for visualization and analysis. ROIs annotated in QuPath (Supplementary Figure S5) were then used to extract region-specific proteomic signatures from the MALDI IMS data.
2.5 Data preprocessing of multimodal imaging data for ML applications
2.5.1 General data preprocessing steps
Figure 7 provides a general overview of the proposed data preprocessing steps that precede ML applications. In addition to these steps, the images were normalized. In general, data preprocessing for ML applications was performed with Python as a programming language using open-source frameworks and the library for deep learning models PyTorch (Paszke et al., 2019).

Figure 7. Preprocessing of multimodal data for potential ML applications. (A) Data selection: example of an image (BOLD-MRI in this case) with artifacts in the ROI of the tumor that would be excluded due to the data selection process; (B) resampling: example of an image with a different x- and y-resolution than the target resolution being resampled to uniform resolution; (C) cropping: example showing the tumor ROI being cropped out; (D) co-registration: example of co-registration of cropped images using affine and elastic deformations.
2.5.2 Data selection
Datasets were assessed for possible image artifacts such as 1) geometrical distortions (especially affecting EPI-based sequences), 2) B0 inhomogeneity (affecting, e.g., CEST measurements), 3) movement artifacts, and 4) flow artifacts. Subsequently, data selection was performed to exclude images where artifacts completely overlapped with ROIs using the following criterion:
Consequently, a subset of the image data I of all samples Dall was selected within image data IROI as the final dataset D which had sufficient image quality and which was free of artifacts that completely overlapped the ROIs. In samples that contained artifacts within the overlapping ROIs, the respective areas were masked to exclude the affected region from further data analysis.
2.5.3 Resampling
The scikit-image library (van der Walt et al., 2014) was used for image resampling to a target resolution of 128 × 128 pixels. The “skimage.transform.resize” transform was used to up- or downsize the images to one equal image shape. Scans with different matrices—[18F]FDG PET (320 × 320 px) and the diffusion maps D, D*, and fIVIM (96 × 96 px) —were resampled accordingly. The order of the spline interpolation was set to 0, while the antialiasing was turned off. This was done to minimize interpolation during image resampling.
2.5.4 Data normalization
Data normalization was performed using NumPy (Harris et al., 2020) to normalize multimodal imaging data to the same range of image signals. A typically used normalization is the range [0,1]. The normalized Image Ĩ was calculated based on the image data I as
2.5.5 Cropping
Cropping was performed using NumPy (Harris et al., 2020) using a square bounding box of side length s = 2d, where d is the distance from the ROI center of mass to the edge of the bounding box, so that it was large enough to include the lesion as well as surrounding tissue (Figure 7C). For known image dataset I (i.e., lesion annotation), the cropped image Ic was extracted as
with the position of the center of mass of the ROI data (cx,cy).
2.5.6 Co-registration of multimodal imaging data
For image co-registration, the commonly available algorithm ANTs that is available for Python in the library ANTsPy (Avants et al., 2009), using mutual information (MI) and cross correlation (CC) approaches, was used (Avants et al., 2011).
The respective image co-registration(s) were formulated as an optimization problem to find the transformation ϕ(x,p), with parameters p and spatial position x that minimize a similarity metric M between images I and J (Avants et al., 2014):
ANTs image co-registration was performed based on affine and non-affine transformations. Affine transformations include translation, rotation, and scaling. Non-affine transformations, such as deformable or elastic transformations, include more complex operations. Except for the images derived from EPI sequences, the images were not deformed and so it was optimal to use affine transformations like “Affine,” “AffineFast”, or “TRSAA” from the ANTs library. For images derived from EPI sequences, it was necessary to apply a non-affine transformation. In this case, non-affine transformations like SyN or SynCC from the ANTs library using MI and CC as the similarity metric, respectively, were used.
In addition to requiring the type of transformation to be defined, ANTs image co-registration also requires the fixed and moving image to be defined. The fixed image is the target and the moving image is the deformed image that needs to be co-registered to the target image by applying the transformation. For images with large differences in intensity distribution, initial co-registration was poor. This specifically applied to the DCE maps as well as to the parameter maps for D*. In these cases, co-registration was based on manually drawn ROI (tumor lesion) masks that were annotated on both images by an biological expert to subsequently calculate the respective image transformation. Once the transformation was calculated, it was applied to the image.
For multiple images resulting from the same scan, it was possible to calculate the transformation once and apply it to all other images, ensuring the exact same co-alignment of the images after the transformation. This way the transformation was calculated for the diffusion map of D and also used for D* and fIVIM. The same was done for the DCE maps: calculating the transformation for ktrans and applying it to “ve” and “vp”.
The quality of the co-registration was validated thus: 1) the value distributions (histograms) of the pre- and post-registration images were compared to ensure values were not altered by the transformation; 2) the quality was analyzed by visual inspection of the pre- and post-registration images.
3 Results
3.1 Metabolic imaging using simultaneous [18F]FDG-PET and glucoCEST MRI
Using the proposed simultaneous [18F]FDG-PET and glucoCEST MRI imaging workflow, a total of 58 female athymic nude mice which grew xenograft tumors of three differently aggressive BC cell lines (luminal A: n = 16; HER2+: n = 17; triple negative: n = 25) were examined. The imaging protocol was tolerated by the animals, with marked reactions (hypoventilation) following 2-deoxy-D-glucose infusion. One mouse inoculated with luminal A BC cells one died during the experiment, probably due to a misplaced intraperitoneal tracer injection. For the purpose of this study, example images derived from in vivo simultaneous [18F]FDG-PET/glucoCEST MRI of a HER2+ (SKBR-3) BC xenograft, compared to images derived from ex vivo MALDI IMS, are shown in Figure 8. Figure 8A shows the T2-weighted anatomical image which served as the reference for [18F]FDG-PET and glucoCEST MRI co-registration. Figure 8B illustrates the parameter map of the tumor´s MTRasym at 1.2 ppm frequency offset, 16 min following 2-deoxy-D-glucose administration (MTRasym: mean ± SD = 0.0816 ± 0.102), superimposed onto the T2-weighted anatomical image. More pronounced MTRasym corresponds to a higher exchange of hydrogen between sugar-related hydroxyl groups and the surrounding water pool. The extent of MTRasym is therefore higher in more active and well-perfused tumor regions. Due to artifacts, the upper left section of the tumor was not analyzed and is not shown on the MTRasym map. Intratumoral heterogeneity of tumor metabolism (Figure 8B) is also confirmed by the superimposed [18F]FDG PET image in Figure 8C, which clearly shows heterogeneous tracer uptake 60 min after tracer administration (SUVbw: mean ± SD = 2.79 ± 0.42). The pattern of [18F]FDG uptake is slightly different from the change in MTRasym at 1.2 ppm frequency offset shown in Figure 8B, which corroborates the notion of complementary, rather than redundant, information captured by [18F]FDG and glucoCEST MRI images. Figure 8D depicts an example MALDI IMS ion image at 1,282.901 m/z, demonstrating heterogeneous signal distribution within the tissue. Figure 8E shows a data-driven tissue section segmentation map that was built based on region-specific peptide fingerprints, and different tissue regions with similar peptides signatures were grouped together and given a specific color. Different tissue regions in breast cancer sections exhibit obvious color diversity, suggesting that there are differences in the spatial expression of peptides. The H&E-stained tissue in Figure 8F offers a view of the tissue’s cellular architecture, facilitating the identification of tumor boundaries and the overall shape of the tumor. The H&E scan reveals that the tumor region consists solely of the tumor mass (Supplementary Figure S5).
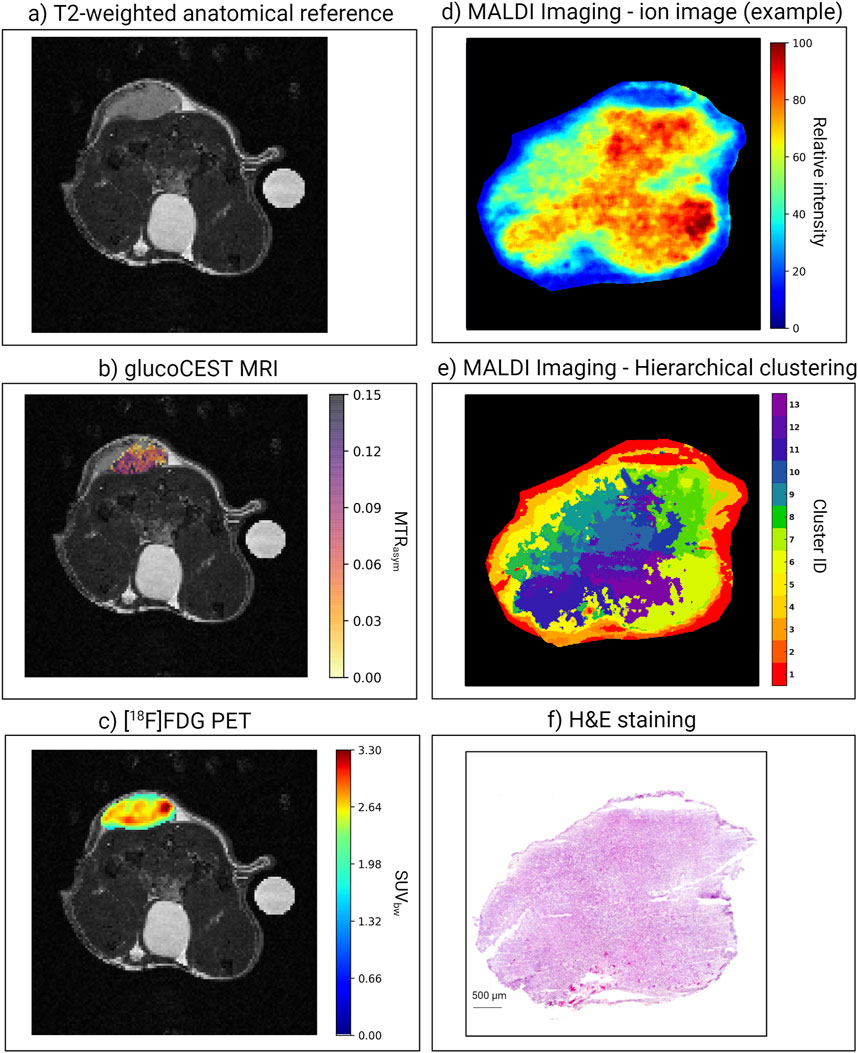
Figure 8. Example images of SKBR-3 breast cancer xenograft derived from the proposed [18F]FDG-PET/glucoCEST protocol for metabolic imaging. (A) T2-weighted image serving as an anatomical reference. (B) GlucoCEST MRI parameter map showing MTRasym values at 1.2ppm frequency offset (high values correspond to high asymmetry, i.e., much glucose present in this area of the tumor). (C) [18F]FDG-PET image of the tumor, showing high tracer uptake on the ventral side of the tumor. (D) MALDI IMS ion images: the first image provides a visualization of the tumor at 1,282.901 m/z. (E) Subsequent image employs hierarchical clustering to define variations among pixels. Hierarchical clustering in MALDI IMS groups pixels based on the similarity of their spectral profiles, thus highlighting areas with distinct molecular compositions. (F) H&E staining.
3.2 Hypoxia imaging using simultaneous [18F]FMISO-PET and mpMRI
A total of 32 female athymic nude mice underwent imaging using the proposed simultaneous multiparametric [18F]FMISO-PET and mpMRI imaging protocol (luminal A: n = 11; HER2+: n = 10; triple negative: n = 11). Among the mice inoculated with luminal A BCs, two died during PET/MRI imaging, presumably due to renal insufficiency as a result of estradiol pellet implantation. For this study, exemplary images derived from in vivo simultaneous [18F]FMISO PET/mpMRI of a triple negative (MDA-MB-231) BC xenograft, compared to images derived from ex vivo MALDI IMS, are shown in Figure 9. Figure 9A shows the T2-weighted anatomical image which served as the reference for [18F]FMISO-PET and mpMRI co-registration. The [18F]FMISO-PET image in Figure 9B reveals a relatively high degree of hypoxia in the tumor core (SUVbw: mean ± SD = 0.84 ± 0.25). The small change in
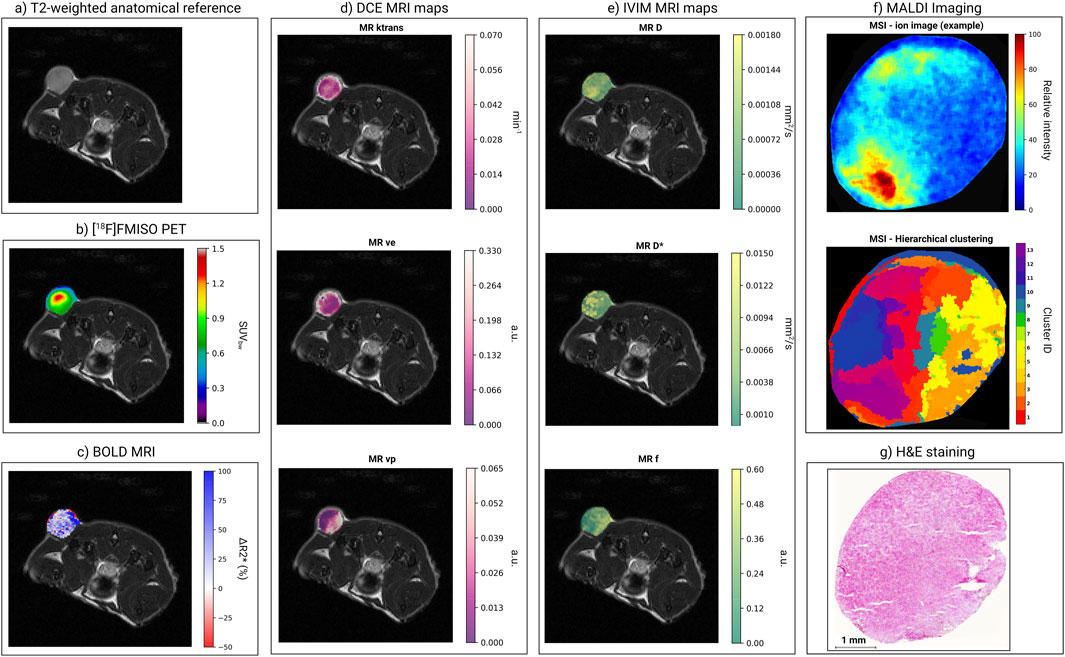
Figure 9. Example images of an MDA-MB-231 breast cancer xenograft derived from the proposed [18F]FMISO-PET/mpMRI protocol for hypoxia imaging. (A) T2-weighted image serving as an anatomical reference. (B) [18F]FMISO PET image of tumor, showing high tracer uptake in its hypoxic center. (C) BOLD-related parameter map of ΔR2*, in which large absolute values correspond to areas where the fraction of oxygenized hemoglobin increased, following the hyperoxic gas challenge. (D) DCE-related parameter maps of the transfer constant ktrans (with high values in areas of increased blood vessel leakage), extravascular volume fraction ve, and plasma volume fraction vp (with high values in areas with increased blood supply). (E) IVIM-DWI parameter maps of diffusion coefficient D (with low values in areas of increased tissue density), perfusion coefficient D* (with high values in areas of increased blood flow), and IVIM-fraction fIVIM (with high values in areas of high blood vessel density). (F) MALDI IMS data. Images generated using SCiLs Lab software. Figures reconstructed from pixel information, including coordinates (x and y) and mass-to-charge (m/z) values detected at each pixel, including their intensities. MALDI IMS ion images: the first image provides a visualization of the tumor at 2,483.473 m/z. The subsequent image employs hierarchical clustering to define variations among pixels. Hierarchical clustering in MALDI IMS groups pixels based on the similarity of their spectral profiles, thus highlighting areas with distinct molecular compositions. (G) H&E staining.
3.3 Ex vivo assessment using MALDI IMS and H&E staining
The use of a MALDI TOF/TOF mass spectrometer enabled the collection of high-resolution spectral data across a mass range of 700–3,000 m/z with a spatial resolution of 20 µm. Figure 8D, e) and Figure 9F present the processed MALDI IMS data of the tumor xenografts. Visualization was reconstructed from pixel information, including spatial coordinates (x and y) and mass-to-charge (m/z) values, along with their intensities. The figures show that hierarchical clustering in MALDI IMS successfully reveals distinct regions within morphologically homogeneous sections of the xenograft tissue (Supplementary Figure S4). Hierarchical clustering, which does not rely on a predefined number of clusters, allows for the data-driven identification of unique proteomic signatures.
Furthermore, MALDI IMS data overlaid with H&E-stained bright-field images (see Supplementary Figure S3 for detailed explanation) provided a composite analysis of proteomic and histological landscapes. ROIs annotated in QuPath were instrumental in extracting region-specific proteomic signatures, which were subsequently correlated with visual histopathological evaluation. H&E staining merely allows for the delineation of the boundaries of neoplastic areas. Given the correlation of MALDI IMS and histopathological data, MALDI IMS is thus essential for identifying distinct cellular populations in tumor tissue.
4 Discussion
Herein, we describe in detail a proposed preclinical workflow for simultaneous in vivo PET/MRI, followed by ex vivo MALDI IMS tissue analysis, showcasing their potential for assessing tumor metabolism and the hypoxic microenvironment of BC xenografts in vivo. The workflow also addresses the intrinsic challenges in data post-processing to co-register multi-scaled images obtained using different imaging methods. Furthermore, the proposed workflow describes the data preprocessing process to obtain datasets suitable for potential ML applications.
Technological advances in preclinical instrumentation have enabled holistic approaches to image tumors and their microenvironments (Ramamonjisoa and Ackerstaff, 2017; Matsumoto et al., 2021). In MRI, functional and spectroscopic protocols added to anatomical imaging allows the interrogation of the profound heterogeneity within the tumor and in the surrounding tissue. Combining MRI with PET simultaneously adds even more information, enabling the detection of malignant tumor phenotypes, the differentiation of tumor and healthy tissues, and the classification of the tumor microenvironment. However, while preclinical PET/MRI presents great promise, the degrees of freedom for preclinical PET/MRI protocols are ultimately limited by the physiology of the investigated animal—laboratory mice in most cases. The total blood volume of mice is approximately 1.8 mL, effectively limiting the maximum amount of injectable volume via the intravenous route to ∼0.125 mL when administered as a single bolus with administration times below 1 min (Diehl et al., 2001). Multiple intravenous injections, even when administered at slow rates (<0.025 mL/min), may lead to significant changes in blood hemodynamics, which must be considered in quantitative image analysis, and more importantly, are not well-tolerated in mice. Another challenge for PET/MRI protocols is the prolonged period of anesthesia required for the immobilization of mice in the respective imaging device during image acquisition. In most cases, inhalant anesthetics such as isoflurane or sevoflurane are used. However, anesthetic concentration, duration, and the depth of consciousness of the investigated animal, as well as the carrier gas delivering anesthetic to the animal itself, strongly affect imaging outcomes and must be adjusted and standardized for each respective application (Navarro et al., 2021).
Regarding our proposed metabolic imaging protocol, we initially addressed the challenge of sequentially administering the PET tracer [18F]FDG and the glucoCEST MRI contrast agent 2-deoxy-D-Glucose in a single protocol. Fortunately, it has been shown for [18F]FDG that uptake kinetics and biodistribution at 50 min after administration does not differ between intravenous or intraperitoneal administration. Studies have demonstrated that tissue uptake in major organs and subcutaneous tumor models is comparable at that timepoint and that intraperitoneal injections are a suitable alternative to intravenous injections for PET studies with [18F]FDG (Fueger et al., 2006; Wong et al., 2011). Therefore, intraperitoneal injections of [18F]FDG were performed to avoid intravenous infusion volumes that would not be tolerated by mice and also due to the necessity of injecting 2-deoxy-D-glucose intravenously for glucoCEST-MRI. This procedure also kept the overall anesthesia time as short as possible and omitted the need to place two catheters into both lateral tail veins to avoid administration problems due to incompatible solvents in the administered solutions. Notably, the total mass of injected [18F]FDG in mice was within the nanogram range. Therefore, interference by [18F]FDG with baseline glucoCEST assessments is highly unlikely given the intrinsic low sensitivity of glucoCEST imaging and the requirement to administer pharmacologically active amounts of 2-deoxy-D-glucose to actually measure significant changes in MTRasym (Capozza et al., 2022). To increase blood glucose levels enough to induce diagnostic changes in MTRasym, 0.5 g/kg 2-deoxy-D-glucose had to be administered. To avoid acute hyperglycemic shocks, we split the infusion into three steps over approximately 8 min. Glucose administrations and the resulting changes in blood glucose levels significantly alter [18F]FDG uptake kinetics and tissue/tumor distribution. We therefore performed the first 10 min of the dynamic [18F]FDG-PET scan before 2-deoxy-D-glucose administration. To obtain further data on glucoCEST dynamics, we then continued with simultaneous PET acquisitions and repeated cycles of glucoCEST scans after administration. Currently, the exact origin of the glucoCEST signal is still being investigated, but it most probably originates from changes in glucose concentration between the intracellular and extracellular matrix. Therefore, data on the temporal and spatial distribution and kinetics of glucoCEST signal are needed. However, the temporal dynamics of glucose concentration are difficult to obtain with glucoCEST MRI, and comparative studies of glucoCEST MRI and [18F]FDG PET in BC are of high interest (Kim et al., 2022). The protocol presented herein provides the basis for further studies on the post-challenge glucoCEST kinetics in BC xenografts and facilitates research on the complementary value of [18F]FDG PET to glucoCEST MRI.
Our proposed hypoxia imaging protocol addressed several challenges. Two intravenous administrations were required: one for the hypoxia PET radiotracer [18F]FMISO and a second for the gadolinium-based contrast agent for DCE-MRI performed at the end of the protocol. Additionally, we needed to account for the fact that the uptake kinetics of hypoxia radiotracers are highly dependent on the anesthetic agent, the inhalant anesthesia carrier gas, and the timepoint after administration used for imaging. We also needed to account for the fact that the uptake of [18F]FMISO in target (hypoxic) tissues and clearance from non-target tissues is generally slow due the lack of a specific uptake mechanism and dependency on passive, diffusion-driven intracellular uptake mechanisms. For hypoxia imaging in tumors by simultaneous BOLD-MRI, two data acquisitions—at normoxic conditions (room air) followed by a second scan at hyperoxic (100% O2) conditions—are needed (Bartsch et al., 2023). This requires a change of the oxygen concentration in the isoflurane carrier gas during the imaging protocol, which in turn influences or even compromises [18F]FMISO uptake in hypoxic tumor areas. Moreover, changes in the isoflurane anesthetic conditions directly affect blood pressure and tissue oxygenation, and the resulting changes in blood microcirculation perfusion do interfere with IVIM-MRI. Hence, the intravenous administrations, the long [18F]FMISO uptake phase, the anesthesia management, timing of oxygen challenge, and ultimately the order of mpMRI sequences were the key methodological challenges in this protocol requiring standardization.
Initial experiments with intraperitoneal administered [18F]FMISO yielded insignificant systemic uptake from the peritoneum and, hence, proved unsuitable for tumor imaging (data not shown). However, intravenous tracer administrations into awake animals were technically challenging and potentially increased the radiation exposure of the personnel involved. As a compromise between radiation protection measures and animal welfare considerations, we performed the first intravenous administration at low anesthetic depth conditions (immediately after the loss of the frightening reflex) and initiated recovery as soon as the intravenous administration was finished. The overall procedure was accomplished within several minutes, and therefore a standardized 60-min uptake interval under awake conditions in the animals’ breathing room air, followed by another 60 min of uptake time during the initial MRI scans, was easily manageable. The optimal time window for [18F]FMISO PET imaging has been found to be in the range of 2–3 h after intravenous administration (Dos Santos et al., 2023). Exactly 120 min after [18F]FMISO administration, the first static PET acquisition was initiated under normoxic conditions, followed by a change of the anesthetic carrier gas from air to 100% oxygen for hyperoxic BOLD-MRI. Recent studies using oxygen-sensitive electrodes in tissues have confirmed that both tumoral and muscle oxygenation rapidly increase within 10 min when the isoflurane carrier gas is switched from air to 100% oxygen (Mahling et al., 2015). We therefore selected a 10-min equilibration interval in our workflow as a compromise between the overall anesthesia time for the animal and sufficient oxygen distribution time within the animal before continuing with hyperoxic BOLD-MRI and the second simultaneous [18F]FMISO PET acquisition. DCE-MRI was included as the last sequence in our workflow, as the infusion of the gadolinium-based contrast agent would interfere with the detection of small changes in the spin–spin relaxation rate during hyperoxic BOLD-MRI and to maximize the time interval between the two intravenous administrations.
Great attention has been given to the resection of tumors and the labeling of tumor orientation, which was required during tumor embedding to allow in vivo and ex vivo image co-registration. Although studies such as Baldi et al. (2019) have suggested the fabrication of 3D-printed tumor molds, we found that these approaches were not manageable as a standard resection protocol for large-scale animal studies. Instead, we developed a protocol which included marking the tumor on three sides using differently colored tissue dye prior to its resection from the mouse. To ensure the same cutting plane as the slice in single-slice MRI acquisitions, the T2-weighted anatomical image was used as a guide when cutting the tumors in halves for embedding.
The subsequent ex vivo analysis of resected tissue was focused on MALDI IMS, a powerful tool for mapping the molecular complexity of BC tissues due to its ability to directly visualize the distribution of a high number of proteins within tissue sections. A notable advantage of MALDI IMS, as demonstrated in our study, is its ability to reveal the subtle nuances of tumor heterogeneity. By applying hierarchical clustering to the MALDI IMS data, we identified distinct molecular patterns that define heterogeneity within BC xenografts. This analytical approach facilitated the distinction of proteomic profiles that morphological assessments alone could not reveal. The segmentation of peptides to reveal tissue heterogeneity is crucial for identifying potential therapeutic targets and understanding the mechanisms that drive tumor progression and resistance.
An additional advantage of MALDI IMS is its ability to perform H&E staining on the same tissue subsequent to MALDI IMS measurement, facilitating the straightforward co-registration of molecular data with histopathological features. The utility of MALDI IMS in revealing the proteomic landscape of BC is further enhanced when compared to in vivo imaging modalities. In our study, the co-registration of MALDI IMS data with in vivo imaging data from [18F]FDG PET/glucoCEST MRI and [18F]FMISO PET/mpMRI could offer a more comprehensive interpretation of the tumor. This multimodal approach effectively captures the spatial heterogeneity of tumors, thereby offering a composite view that integrates the dynamic metabolic and structural information from PET and MRI with the molecular snapshot provided by MALDI IMS. Co-registration allows for multiscale analysis that combines macroscopic imaging findings with microscopic molecular details. Such an approach is instrumental in enhancing our understanding of the pathophysiological processes, potentially leading to the identification of novel biomarkers and therapeutic targets.
Despite the findings provided by MALDI IMS of BC xenografts, several challenges persist. The technique’s sensitivity to sample preparation, matrix application, and ionization efficiency can lead to variability in the data, highlighting the need to standardize the experimental protocol. The vast quantity of data generated requires sophisticated computational methods for effective data reduction and image reconstruction—a bottleneck for researchers without access to advanced bioinformatics tools.
Concerning ML analyses of CMI data, data preprocessing and preparation for subsequent ML applications are, in many aspects, similar to clinical data. However, there are substantial differences in sample size and the underlying biological rationale. The typical tumor ROIs of a mouse are significantly smaller than that of human tumors (i.e., BC in this case). Thus, the first step we presented, dataset selection, is most important. Small-scale motion and susceptibility artifacts are more pronounced in preclinical high-field PET/MRI systems than in clinical MRI hardware. They have a profound effect on small-animal imaging data and can render the whole image unusable. The next steps we presented, resampling, normalization, and cropping, are very similar to the established clinical preprocessing pipelines and are equally critical for the application of ML to radioproteomic datasets and preclinical image data. Furthermore, these next steps are important, as ML algorithms depend on equidistant pixels and uniform value ranges. The last step, co-registration, addresses a non-trivial problem that is an area of active research. We applied an approach using ANTs, which has become a standard approach in the field (Avants et al., 2009). The validation and quality control of co-registration remain challenging as there is usually no ground-truth (i.e., non-deformed, perfectly aligned) scan available.
Lastly, it must be mentioned that all proposed imaging workflows are non-recovery investigations, with subsequent ex vivo MALDI IMS investigations requiring the euthanasia of the animals at the end of the imaging workflows. For longitudinal assessments (e.g., for predictive assessments of therapy response in distinct BC subtypes), new workflows will need to be determined which consider aspects such as peri-anesthetic management after each respective imaging session, an optimized recovery phase after anesthesia employing oxygen support and body temperature maintenance, fluid management by subcutaneous administrations of (warmed) supportive isotonic solutions, and the minimum time interval for full recovery before the next imaging session. Moreover, considerable volume and shape changes of tumors during progression and in response to therapeutic interventions pose additional challenges in image co-registration and -analysis.
In summary, we present here in detail a practical simultaneous multiparametric PET/MRI preclinical workflow for in vivo metabolic and hypoxia imaging, maximizing the number of assessed parameters within a reasonable anesthesia time that was well-tolerated by the investigated mice. The workflow provides further guidance on the subsequent collection of tumor samples in a comparable anatomical orientation for ex vivo analysis and showcases the correlative value of MALDI IMS to PET/MRI for assessing tumor heterogeneity. For instance, the identification of hypoxic and normoxic tumor regions using multiparametric PET/MRI imaging could be of great use for the longitudinal assessment of differential response following novel tumor microenvironment-targeting therapy. For a multifaceted, holistic view, these imaging biomarkers can only be validated by ex vivo molecular data from CMI datasets. Finally, the workflow describes data preprocessing steps for the co-registration of image data from different in vivo sources at different scale levels, with the clear perspective of facilitating ML radioproteomic applications.
Data availability statement
The raw data supporting the conclusions of this article will be made available by the corresponding author upon reasonable request.
Ethics statement
The animal study was approved by Austrian Federal Ministry of Education, Science and Research [66.009/0284-WF/V/3b/2017; 2020-0.363.124; 2022-0.726.820] and the Intramural Committee for Animal Experimentation of the Medical University of Vienna. The study was conducted in accordance with local legislation and institutional requirements.
Author contributions
SB: writing–original draft, writing–review and editing, investigation, data curation, formal analysis, visualization, and validation. KB: formal analysis, investigation, visualization, writing–original draft, writing–review and editing, and methodology. CF: formal analysis, investigation, methodology, writing–original draft, and writing–review and editing. JF: investigation and writing–review and editing. DL: investigation and writing–review and editing. TH: writing–review and editing, conceptualization, funding acquisition, resources, and supervision. MH: writing–review and editing and resources. CK: writing–review and editing and investigation. KK: writing–review and editing, supervision, and resources. LK: resources, supervision, writing–review and editing, conceptualization, and funding acquisition. GL: conceptualization, supervision, writing–review and editing, funding acquisition, and resources. KP: funding acquisition, resources, supervision, writing–review and editing, and conceptualization. TW: conceptualization, investigation, methodology, project administration, supervision, writing–original draft, and writing–review and editing.
Funding
The authors declare that financial support was received for the research, authorship, and/or publication of this article. This research was funded by the WWTF (Vienna Science and Technology Fund) grant number LS19-018.
Acknowledgments
Financial support by the Austrian Federal Ministry of Science, Research and Economy is gratefully acknowledged. The authors further thank their colleagues from the Medical Imaging Cluster, the Core Facility laboratory animal breeding and husbandry (CFL), and the radiopharmacy department from the Division of Nuclear Medicine for their continuous support. The authors thank Joanne Chin for proofreading the manuscript and Barbara Dekan for her support with cell cultivation. The authors are grateful for the support of Bruker, Ettlingen, Germany, in the course of a collaboration agreement. Figures were created with BioRender.com.
Conflict of interest
Author LK was employed by the CBmed GmbH-Center for Biomarker Research in Medicine.
The remaining authors declare that the research was conducted in the absence of any commercial or financial relationships that could be construed as a potential conflict of interest.
The author(s) declared that they were an editorial board member of Frontiers, at the time of submission. This had no impact on the peer review process and the final decision.
Publisher’s note
All claims expressed in this article are solely those of the authors and do not necessarily represent those of their affiliated organizations, or those of the publisher, the editors, and the reviewers. Any product that may be evaluated in this article, or claim that may be made by its manufacturer, is not guaranteed or endorsed by the publisher.
Supplementary Material
The Supplementary Material for this article can be found online at: https://www.frontiersin.org/articles/10.3389/fbiom.2024.1420114/full#supplementary-material
References
Avants, B. B., Tustison, N., and Song, G. (2009). Advanced normalization tools (ANTS). Insight J. 2 (365), 1–35. doi:10.54294/uvnhin
Avants, B. B., Tustison, N. J., Song, G., Cook, P. A., Klein, A., and Gee, J. C. (2011). A reproducible evaluation of ANTs similarity metric performance in brain image registration. Neuroimage 54, 2033–2044. doi:10.1016/j.neuroimage.2010.09.025
Avants, B. B., Tustison, N. J., Stauffer, M., Song, G., Wu, B., and Gee, J. C. (2014). The Insight ToolKit image registration framework. Front. Neuroinformatics 8, 44. doi:10.3389/fninf.2014.00044
Baldi, D., Aiello, M., Duggento, A., Salvatore, M., and Cavaliere, C. (2019). MR imaging-histology correlation by tailored 3D-printed slicer in oncological assessment. Contrast Media Mol. Imaging 2019, 1–9. doi:10.1155/2019/1071453
Barnes, S. L., Sorace, A. G., Loveless, M. E., Whisenant, J. G., and Yankeelov, T. E. (2015). Correlation of tumor characteristics derived from DCE-MRI and DW-MRI with histology in murine models of breast cancer. NMR Biomed. 28, 1345–1356. doi:10.1002/nbm.3377
Bartsch, S. J., Brozova, K., Ehret, V., Friske, J., Furbock, C., Kenner, L., et al. (2024). Non-contrast-enhanced multiparametric MRI of the hypoxic tumor microenvironment allows molecular subtyping of breast cancer: a pilot study. Cancers (Basel) 16 (2), 375. doi:10.3390/cancers16020375
Bartsch, S. J., Ehret, V., Friske, J., Frohlich, V., Laimer-Gruber, D., Helbich, T. H., et al. (2023). Hyperoxic BOLD-MRI-based characterization of breast cancer molecular subtypes is independent of the supplied amount of oxygen: a preclinical study. Diagn. (Basel) 13 (18), 2946. doi:10.3390/diagnostics13182946
Capozza, M., Anemone, A., Dhakan, C., Della Peruta, M., Bracesco, M., Zullino, S., et al. (2022). GlucoCEST MRI for the evaluation response to chemotherapeutic and metabolic treatments in a murine triple-negative breast cancer: a comparison with[(18)F]F-FDG-PET. Mol. Imaging Biol. 24, 126–134. doi:10.1007/s11307-021-01637-6
Conti, A., Duggento, A., Indovina, I., Guerrisi, M., and Toschi, N. (2021). Radiomics in breast cancer classification and prediction. Semin. Cancer Biol. 72, 238–250. doi:10.1016/j.semcancer.2020.04.002
Diehl, K. H., Hull, R., Morton, D., Pfister, R., Rabemampianina, Y., Smith, D., et al. (2001). A good practice guide to the administration of substances and removal of blood, including routes and volumes. J. Appl. Toxicol. 21 (1), 15–23. doi:10.1002/jat.727
Dos Santos, S. N., Wuest, M., Jans, H. S., Woodfield, J., Nario, A. P., Krys, D., et al. (2023). Comparison of three (18)F-labeled 2-nitroimidazoles for imaging hypoxia in breast cancer xenografts: [(18)F]FBNA, [(18)F]FAZA and [(18)F]FMISO. Nucl. Med. Biol. 124-125, 108383. doi:10.1016/j.nucmedbio.2023.108383
Erickson, B. J., Korfiatis, P., Akkus, Z., and Kline, T. L. (2017). Machine learning for medical imaging. radiographics 37 (2), 505–515. doi:10.1148/rg.2017160130
Fueger, B. J., Czernin, J., Hildebrandt, I., Tran, C., Halpern, B. S., Stout, D., et al. (2006). Impact of animal handling on the results of 18F-FDG PET studies in mice. J. Nucl. Med. 47 (6), 999–1006.
Glish, G. L., and Vachet, R. W. (2003). The basics of mass spectrometry in the twenty-first century. Nat. Rev. Drug Discov. 2, 140–150. doi:10.1038/nrd1011
Grandjean, J., Canella, C., Anckaerts, C., Ayranci, G., Bougacha, S., Bienert, T., et al. (2020). Common functional networks in the mouse brain revealed by multi-centre resting-state fMRI analysis. Neuroimage 205, 116278. doi:10.1016/j.neuroimage.2019.116278
Greve, J. M. (2011). “The BOLD effect,” in In vivo NMR imaging: methods and protocols. Editors L. Schröder, and C. Faber (Totowa, NJ: Humana Press), 153–169.
Guadilla, I., Calle, D., and Lopez-Larrubia, P. (2018). Diffusion-weighted magnetic resonance imaging. Methods Mol. Biol. 1718, 89–101. doi:10.1007/978-1-4939-7531-0_6
Harris, C. R., Millman, K. J., van der Walt, S. J., Gommers, R., Virtanen, P., Cournapeau, D., et al. (2020). Array programming with NumPy. Nature 585 (7825), 357–362. doi:10.1038/s41586-020-2649-2
Iima, M. (2021). Perfusion-driven intravoxel incoherent motion (IVIM) MRI in oncology: applications, challenges, and future trends. Magn. Reson Med. Sci. 20, 125–138. doi:10.2463/mrms.rev.2019-0124
Kim, M., Eleftheriou, A., Ravotto, L., Weber, B., Rivlin, M., Navon, G., et al. (2022). What do we know about dynamic glucose-enhanced (DGE) MRI and how close is it to the clinics? Horizon 2020 GLINT consortium report. Magn. Reson Mat. Phys. Bio Med. 35 (1), 87–104. doi:10.1007/s10334-021-00994-1
Kniess, T., Zessin, J., Mading, P., Kuchar, M., Kiss, O., and Kopka, K. (2023). Synthesis of [(18)F]FMISO, a hypoxia-specific imaging probe for PET, an overview from a radiochemist's perspective. EJNMMI Radiopharm. Chem. 8 (1), 5. doi:10.1186/s41181-023-00190-7
Knutsson, L., Xu, X., van Zijl, P. C. M., and Chan, K. W. Y. (2023). Imaging of sugar-based contrast agents using their hydroxyl proton exchange properties. NMR in Biomedicine 36 (6), e4784. doi:10.1002/nbm.4784
Le Bihan, D. (2019). What can we see with IVIM MRI? Neuroimage 187, 56–67. doi:10.1016/j.neuroimage.2017.12.062
Lim, I. A., Li, X., Jones, C. K., Farrell, J. A., Vikram, D. S., and Van Zijl, P. C. (2014). Quantitative magnetic susceptibility mapping without phase unwrapping using WASSR. Neuroimage 86, 265–279. doi:10.1016/j.neuroimage.2013.09.072
Lowekamp, B. C., Chen, D. T., Ibanez, L., and Blezek, D. (2013). The design of SimpleITK. Front. Neuroinform 7, 45. doi:10.3389/fninf.2013.00045
Mahling, M., Fuchs, K., Thaiss, W. M., Maier, F. C., Feger, M., Bukala, D., et al. (2015). A comparative pO2 probe and [18F]-Fluoro-Azomycinarabino-Furanoside ([18F]FAZA) PET study reveals anesthesia-induced impairment of oxygenation and perfusion in tumor and muscle. PLoS One 10 (4), e0124665. doi:10.1371/journal.pone.0124665
Mannheim, J. G., Mamach, M., Reder, S., Traxl, A., Mucha, N., Disselhorst, J. A., et al. (2019). Reproducibility and comparability of preclinical PET imaging data: a multicenter small-animal PET study. J. Nucl. Med. 60, 1483–1491. doi:10.2967/jnumed.118.221994
Marino, M. A., Helbich, T., Baltzer, P., and Pinker-Domenig, K. (2018). Multiparametric MRI of the breast: a review. J. Magn. Reson Imaging 47, 301–315. doi:10.1002/jmri.25790
Matsumoto, K. I., Mitchell, J. B., and Krishna, M. C. (2021). Multimodal functional imaging for cancer/tumor microenvironments based on MRI, EPRI, and PET. Molecules 26 (6), 1614. doi:10.3390/molecules26061614
Navarro, K. L., Huss, M., Smith, J. C., Sharp, P., Marx, J. O., and Pacharinsak, C. (2021). Mouse anesthesia: the art and science. ILAR J. 62 (1-2), 238–273. doi:10.1093/ilar/ilab016
Paszke, A., Gross, S., Massa, F., Lerer, A., Bradbury, J., Chanan, G., et al. (2019). Pytorch: an imperative style, high-performance deep learning library. Adv. neural Inf. Process. Syst. 32. doi:10.48550/arXiv.1912.01703
Ramamonjisoa, N., and Ackerstaff, E. (2017). Characterization of the tumor microenvironment and tumor-stroma interaction by non-invasive preclinical imaging. Front. Oncol. 7, 3. doi:10.3389/fonc.2017.00003
Romeo, V., Helbich, T. H., and Pinker, K. (2023). Breast PET/MRI hybrid imaging and targeted tracers. J. Magn. Reson Imaging 57, 370–386. doi:10.1002/jmri.28431
Schmitz, J., Schwab, J., Schwenck, J., Chen, Q., Quintanilla-Martinez, L., Hahn, M., et al. (2016). Decoding intratumoral heterogeneity of breast cancer by multiparametric in vivo imaging: a translational study. Cancer Res. 76, 5512–5522. doi:10.1158/0008-5472.can-15-0642
Sourbron, S. P., and Buckley, D. L. (2013). Classic models for dynamic contrast-enhanced MRI. NMR Biomed. 26, 1004–1027. doi:10.1002/nbm.2940
Svirkova, A., Turyanskaya, A., Perneczky, L., Streli, C., and Marchetti-Deschmann, M. (2018). Multimodal imaging of undecalcified tissue sections by MALDI MS and μXRF. Analyst 143, 2587–2595. doi:10.1039/c8an00313k
Tuck, M., Grelard, F., Blanc, L., and Desbenoit, N. (2022). MALDI-MSI towards multimodal imaging: challenges and perspectives. Front. Chem. 10, 904688. doi:10.3389/fchem.2022.904688
van der Walt, S., Schonberger, J. L., Nunez-Iglesias, J., Boulogne, F., Warner, J. D., Yager, N., et al. (2014). scikit-image: image processing in Python. PeerJ 2, e453. doi:10.7717/peerj.453
Van Zijl, P. C., and Yadav, N. N. (2011). Chemical exchange saturation transfer (CEST): what is in a name and what isn't? Magn. Reson Med. 65, 927–948. doi:10.1002/mrm.22761
Villano, D., Romdhane, F., Irrera, P., Consolino, L., Anemone, A., Zaiss, M., et al. (2021). A fast multislice sequence for 3D MRI-CEST pH imaging. Magn. Reson Med. 85, 1335–1349. doi:10.1002/mrm.28516
Walter, A., Kleywegt, G. J., and Verkade, P. (2021). “Chapter 17 - correlative multimodal imaging: building a community,” in Methods in cell biology. Editors T. Müller-Reichert, and P. Verkade (Academic Press), 417–430.
Walter, A., Paul-Gilloteaux, P., Plochberger, B., Sefc, L., Verkade, P., Mannheim, J. G., et al. (2020). Correlated multimodal imaging in Life sciences: expanding the biomedical horizon. Front. Phys. 8. doi:10.3389/fphy.2020.00047
Wong, K. P., Sha, W., Zhang, X., and Huang, S. C. (2011). Effects of administration route, dietary condition, and blood glucose level on kinetics and uptake of 18F-FDG in mice. J. Nucl. Med. 52, 800–807. doi:10.2967/jnumed.110.085092
Zaiss, M., Anemone, A., Goerke, S., Longo, D. L., Herz, K., Pohmann, R., et al. (2019). Quantification of hydroxyl exchange of D-Glucose at physiological conditions for optimization of glucoCEST MRI at 3, 7 and 9.4 Tesla. NMR Biomed. 32, e4113. doi:10.1002/nbm.4113
Zaiss, M., and Bachert, P. (2013). Chemical exchange saturation transfer (CEST) and MR Z-spectroscopy in vivo: a review of theoretical approaches and methods. Phys. Med. Biol. 58 (22), R221–R269. doi:10.1088/0031-9155/58/22/r221
Glossary
ACN acetonitrile
ADC apparent diffusion coefficient
BC breast cancer
BOLD-MRI blood oxygen level-dependent MRI
CEST chemical exchange saturation transfer
CMI correlative multimodal imaging
CT computed tomography
D diffusion coefficient
D* perfusion coefficient
DCE-MRI dynamic contrast-enhanced MRI
EPI echo planar imaging
EtOH ethanol
FBS fetal bovine serum
[18F]FDG 2-[18F]Fluor-2-desoxy-D-glucose
fIVIM IVIM fraction
FLASH fast low-angle shot
[18F]FMISO 1H-1-(3-[18F]fluoro-2-hydroxypropyl)-2-nitroimidazole
FOV field of view
GS/s gigasamples per s
H&E hematoxylin and eosin
HCCA α-cyano-4-hydroxycinnamic acid
IVIM-MRI intravoxel incoherent motion MRI
ktrans transfer constant between intravascular and extravascular space
m/z mass-to-charge ratio
MALDI IMS matrix-assisted laser desorption/ionization imaging mass spectrometry
ML machine learning
mpMRI multiparametric MRI
MRI magnetic resonance imaging
MTRasym magnetization transfer ratio asymmetry
PBS phosphate-buffered saline
PET positron emission tomography
RARE rapid acquisition with relaxation enhancement
ROI region of interest
SUVbw standardized uptake value (body weight)
TA acquisition time
TE echo time
TFA trifluoroacetic acid
TIC total ion count
TR repetition time
ve extravascular volume fraction
vp plasma volume fraction
VOI volume of interest
VTR variable repetition time
WASSR water saturation shift referencing
Keywords: PET/MRI, proteomics, molecular imaging, MALDI IMS, correlative multimodal imaging, machine learning
Citation: Bartsch SJ, Brožová K, Fürböck C, Friske J, Laimer-Gruber D, Helbich TH, Hacker M, Kuntner C, Kratochwill K, Kenner L, Langs G, Pinker K and Wanek T (2024) Methodological aspects of correlative, multimodal, multiparametric breast cancer imaging: from data acquisition to image processing for AI-based radioproteomics in a preclinical setting. Front. Biomater. Sci. 3:1420114. doi: 10.3389/fbiom.2024.1420114
Received: 19 April 2024; Accepted: 24 September 2024;
Published: 25 October 2024.
Edited by:
Alfonso Mastropietro, National Research Council (CNR), ItalyReviewed by:
Sandeep Kumar Mishra, Yale University, United StatesLydia Wachsmuth, University of Münster, Germany
Copyright © 2024 Bartsch, Brožová, Fürböck, Friske, Laimer-Gruber, Helbich, Hacker, Kuntner, Kratochwill, Kenner, Langs, Pinker and Wanek. This is an open-access article distributed under the terms of the Creative Commons Attribution License (CC BY). The use, distribution or reproduction in other forums is permitted, provided the original author(s) and the copyright owner(s) are credited and that the original publication in this journal is cited, in accordance with accepted academic practice. No use, distribution or reproduction is permitted which does not comply with these terms.
*Correspondence: Thomas Wanek, dGhvbWFzLndhbmVrQG1lZHVuaXdpZW4uYWMuYXQ=
†These authors have contributed equally to this work