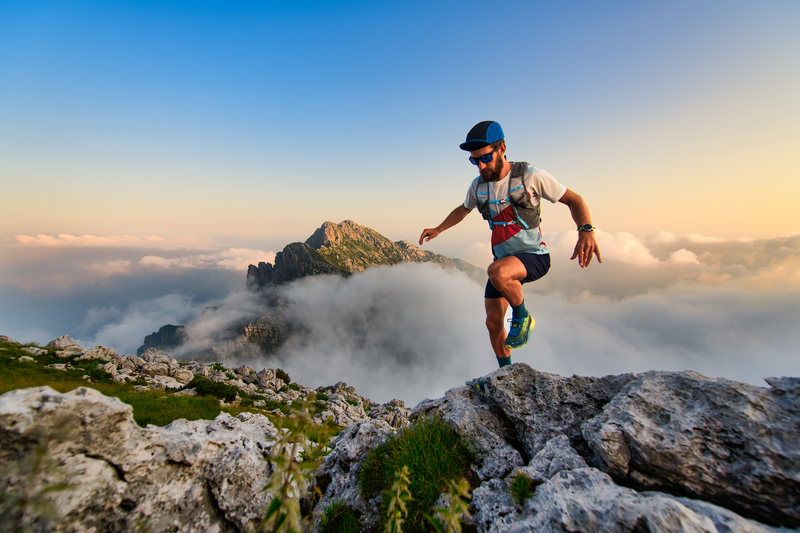
95% of researchers rate our articles as excellent or good
Learn more about the work of our research integrity team to safeguard the quality of each article we publish.
Find out more
ORIGINAL RESEARCH article
Front. Bioeng. Biotechnol. , 09 November 2023
Sec. Biomechanics
Volume 11 - 2023 | https://doi.org/10.3389/fbioe.2023.1288134
Purpose: This study aimed to investigate the features of progressive keratoconus by means of machine learning.
Methods: In total, 163 eyes from 127 patients with at least 3 examination records were enrolled in this study. Pentacam HR was used to measure corneal topography. Steepest meridian keratometry (K1), flattest meridian keratometry (K2), steepest anterior keratometry (Kmax), central corneal thickness (CCT), thinnest corneal thickness (TCT), anterior radius of cornea (ARC), posterior elevation (PE), index of surface variation (ISV), and index of height deviation (IHD) were input for analysis. Support vector machine (SVM) and logistic regression analysis were applied to construct prediction models.
Results: Age, PE, and IHD showed statistically significant differences as the follow-up period extended. K2, PE, and ARC were selected for model construction. Logistic regression analysis presented a mean area under the curve (AUC) score of 0.780, while SVM presented a mean AUC of 0.659. The prediction sensitivity of SVM was 52.9%, and specificity was 79.0%.
Conclusion: It is feasible to use machine learning to predict the progression and prognosis of keratoconus. Posterior elevation exhibits a sensitive prediction effect.
Keratoconus is a corneal disease featuring progressive ectasia and thinning and typically occurs in teenagers. Aggravating irregular corneal astigmatism can lead to vision loss and compromise of life quality with the progression of keratoconus (Ferdi et al., 2019). It is one of the major causes of blindness.
The effects of various interventions, including rigid gas-permeable contact lenses (RGP), intrastromal corneal rings, corneal cross-linking (CXL), and corneal graft, have been broadly investigated over the years. A previous study indicated a remarkable improvement in best-corrected visual acuity (BCVA) in patients who wear RGP on a long-term basis, while they show no influence on the progression of keratoconus (Araki et al., 2021). Intrastromal corneal rings were shown to profoundly promote visual quality but bring in glare and night vision loss at the same time (Daxer et al., 2010). Not only did CXL surgery improve visual quality and prove its safety, but slowed the progression of keratoconus as well (Hashemi et al., 2013; Hersh et al., 2017; Wang et al., 2018). The effect of CXL with epithelial removal using transepithelial phototherapeutic keratectomy (t-PTK) appeared most notable above all (Kymionis et al., 2012).
Different parameters have been used to evaluate the progression of keratoconus. Chan et al. pointed out that steeper keratometry and younger age could lead to more radical progression (Chan et al., 2020). Another study indicated longitudinal monitoring of corneal topography as a vital perspective (Choi and Kim, 2012). Moreover, predictors with higher efficiency acquired by formulas have shown a new way forward (Shajari et al., 2019; Kato et al., 2021). Various devices play a vital role in the diagnosis and evaluation of keratoconus. The most commonly used protocol is corneal biomechanics combined with topography, including corneal pachymetry, aberration analysis, and optical coherence tomography (OCT) (Martinez-Abad and Pinero, 2017).
With the development of artificial intelligence, prediction efficiency can now be elevated further. Kato et al. used deep learning to predict the need for CXL and found that pachymetry mapping combined with age worked as ideal indicators (Kato et al., 2021), while Ruiz Hidalgo et al. reached a similar conclusion with machine learning (Ruiz Hidalgo et al., 2016). Al-Timemy et al. reported high accuracy in the detection of keratoconus with a time-friendly hybrid deep-learning model (Al-Timemy et al., 2021). The application of machine learning for keratoconus has shown preliminary results and needs further investigation.
The most severe stage of keratoconus usually appears in the second decade of life in Asia. It is inclined to stabilize in the fourth decade of life (Saini et al., 2004; Duncan et al., 2016). With an early onset, keratoconus raises the common concerns of progression and prognosis. Although several studies have investigated single parameters of keratoconus, a precise prediction model remains to be developed. This study aims to investigate the progression and prognosis of keratoconus via machine learning.
This is a retrospective study in compliance with the tenets of the Declaration of Helsinki and the request of the Ethics Committee of the Eye and ENT Hospital of Fudan University (Shanghai, China). Informed consent was obtained from the subjects after an explanation of the nature and possible consequences of the study. In total, 163 eyes from 127 patients with keratoconus were recruited. The inclusion criteria were primary keratoconus with no less than 3 examination records on Pentacam HR in the Eye and ENT Hospital of Fudan University and the interval of each follow-up visit should be no less than 1 month. The exclusion criteria were as follows: ectasia after refractive surgery, corneal perforation, history of corneal collagen crosslinking surgery, and history of systematic diseases and other ocular diseases.
Pentacam HR (Oculus, Wetzlar, Germany) was used to analyze the corneal topography. Based on Scheimpflug rotating technology, it reconstructs the anterior segment and exhibits corneal features. Parameters including steepest meridian keratometry (K1), flattest meridian keratometry (K2), steepest anterior keratometry (Kmax), central corneal thickness (CCT), thinnest corneal thickness (TCT), anterior radial curvature (ARC), posterior elevation (PE), index of surface variation (ISV), index of height deviation (IHD), and staging were input for analysis.
Clustering and machine learning were combined to construct a prediction model based on the patients’ group level. A variety of regression algorithms of machine learning including regression trees model (CART), support vector machine (SVM), random forest (RF), and extreme gradient boosting (XGBoost) was conducted. Ten-fold cross-validation was used for model training. Of the data, 70% were randomly chosen for the test set and 30% randomly for the training set. There are 2 sets in 10-fold cross-validation. The training set was created for learning and the test set for validation, which tests the efficiency of models. Performing 10-fold cross-validation is meant to provide a reliable estimate of prediction accuracy, even though it may require longer processing time. The LASSO (Least Absolute Shrinkage and Selection Operator) model was used to select the parameters with the highest performance in SVM and logistic regression, while the RFE (Recursive Feature Elimination) model was used to do the same in CART and RF. The parameters of XGBoost were selected in the model automatically. Mutual parameters shared by at least four models were defined as the parameters with the highest performance.
Statistical analysis was performed using R version 4.1.12. Continuous variables were presented as mean ± standard deviation (SD), and categorical variables were presented as frequency and percentage. Machine learning was processed with Python (3.0).
In total, 163 eyes from 127 patients (male, 99; female, 28) were enrolled in this study with no corneal infection or perforation. The mean age of diagnosis was 20.1 ± 5.4 years old, ranging from 7 to 34 years old. The mean follow-up time was 55.50 ± 27.75 months.
Parameters fluctuated during follow-up (Table 1; Figure 1). K1, K2, and Kmax showed a down-up-down trend, while CCT and TCT shared the same trend. ISV and IHD varied similarly and simultaneously. The changes in age (p < 0.001), PE (p = 0.009), and IHD (p = 0.033) were statistically significantly different.
Among all the parameters input, K2, PE, and ARC showed the highest performance in most models and were screened for machine learning and logistic regression. The AUC scores of three out of five prediction models were above 0.500 (Table 2), while the logistic regression model presented a higher AUC value of 0.780 (Figure 2), followed by SVM with 0.659. The prediction sensitivity of the logistic regression model reached 0.842, followed by XGBoost (0.640) and CART (0.619), while SVM showed the highest prediction specificity with 0.790, followed by logistic regression (0.737) and RF (0.684).
FIGURE 2. Performance of prediction models. LR, Logistic regression; Purple line, reference line, showing AUC score of 0.500.
Leading to blindness, keratoconus has an overall incidence of 1/2000 (Gomes et al., 2015). While a consensus on diagnosis and treatment has been reached over the past 20 years, the criteria of progression and prognosis remain to be discussed. To our knowledge, this study predicted the development of keratoconus with Pentacam via machine learning for the first time.
It was revealed in our study that changes in age, PE, and IHD showed statistical significance in the progression of keratoconus, which has been proved in previous studies. Serving as indications of elevation, PE shows the variation directly, while IHD does so with a processed index, which implies the indication effect of posterior elevation in the progression and prognosis of keratoconus. Younger age and severity of onset indicate more radical development (Meyer et al., 2021; Ferdi et al., 2022). Moreover, it was implied that a steeper Kmax is a risk factor for the progression of keratoconus (Hamilton et al., 2016). This could be attributed to the interaction between Kmax and other parameters such as TCT, which becomes thinner with the steepening of Kmax. While the change is reflected in staging, it fails to indicate the progression of the disease. Age and PE are relatively more sensitive and could serve as early warning parameters.
It has been pointed out that ISV is also a sensitive predictor of the progression of keratoconus (Kanellopoulos and Asimellis, 2013), which could be related to its role in the staging algorithm of Pentacam. It was included in the machine learning input to improve the accuracy of prediction.
The AUC value of the logistic regression model was higher than that of the SVM model. This could be related to the appropriate condition of both algorithms. While the SVM model is a deep learning method that is relatively well adapted to limited samples, a multi-classification task remains a problem. On the contrary, logistic regression analysis is an ideal method for the prediction of complex prognosis, which explains the higher AUC value compared to the SVM model.
The limitations of our study are 1) The sample size is relatively small and 2) the eye-rubbing habits and genetic backgrounds of patients remain to be taken into consideration for better stability of prediction.
In conclusion, posterior elevation serves as a sensitive predictor of the progression of keratoconus. It is feasible to predict the development of keratoconus via machine learning.
The raw data supporting the conclusion of this article will be made available by the authors, without undue reservation.
The studies involving humans were approved by the Ethics Committee of the Eye and ENT Hospital of Fudan University (Shanghai, China). The studies were conducted in accordance with the local legislation and institutional requirements. The participants provided their written informed consent to participate in this study.
XH: Writing–original draft. YS: Writing–original draft. DG: Writing–original draft. XiaZ: Writing–review and editing. LS: Writing–review and editing. ZC: Writing–review and editing. XinZ: Writing–review and editing.
The author(s) declare financial support was received for the research, authorship, and/or publication of this article. National Natural Science Foundation of China (Grant No. 82101183). National Natural Science Foundation of China (Grant No. 81770955). Research Project Grant of Shanghai Municipal Commission of Health and Family Planning (20204Y0058). Project of Shanghai Science and Technology (Grant No. 20410710100). Clinical Research Plan of SHDC (SHDC2020CR1043B). Project of Shanghai Xuhui District Science and Technology (2020-015). Project of Shanghai Xuhui District Science and Technology (XHLHGG202104). Shanghai Engineering Research Center of Laser and Autostereoscopic 3D for Vision Care (20DZ2255000). Construction of a 3D digital intelligent prevention and control platform for the whole life cycle of highly myopic patients in the Yangtze River Delta (21002411600).
The authors appreciate the contributions of all patients who participated in this study, as well as the staff at the Eye and ENT Hospital of Fudan University for their support.
The authors declare that the research was conducted in the absence of any commercial or financial relationships that could be construed as a potential conflict of interest.
All claims expressed in this article are solely those of the authors and do not necessarily represent those of their affiliated organizations, or those of the publisher, the editors and the reviewers. Any product that may be evaluated in this article, or claim that may be made by its manufacturer, is not guaranteed or endorsed by the publisher.
Al-Timemy, A. H., Mosa, Z. M., Alyasseri, Z., Lavric, A., Lui, M. M., Hazarbassanov, R. M., et al. (2021). A hybrid deep learning construct for detecting keratoconus from corneal maps. Transl. Vis. Sci. Technol. 10 (14), 16. doi:10.1167/tvst.10.14.16
Araki, S., Koh, S., Kabata, D., Inoue, R., Morii, D., Maeda, N., et al. (2021). Effect of long-term rigid gas-permeable contact lens wear on keratoconus progression. Br. J. Ophthalmol. 105 (2), 186–190. doi:10.1136/bjophthalmol-2020-315942
Chan, E., Yip, H., Vogrin, S., and Sahebjada, S. (2020). Factors affecting keratoconus progression and corneal collagen cross-linking. Clin. Exp. Ophthalmol. 48 (4), 531–532. doi:10.1111/ceo.13723
Choi, J. A., and Kim, M. S. (2012). Progression of keratoconus by longitudinal assessment with corneal topography. Invest. Ophthalmol. Vis. Sci. 53 (2), 927–935. doi:10.1167/iovs.11-8118
Daxer, A., Mahmoud, H., and Venkateswaran, R. S. (2010). Intracorneal continuous ring implantation for keratoconus: one-year follow-up. J. Cataract. Refract Surg. 36 (8), 1296–1302. doi:10.1016/j.jcrs.2010.03.039
Duncan, J. K., Belin, M. W., and Borgstrom, M. (2016). Assessing progression of keratoconus: novel tomographic determinants. Eye Vis. (Lond). 3, 6. doi:10.1186/s40662-016-0038-6
Ferdi, A., Nguyen, V., Kandel, H., Tan, J. C. K., Arnalich-Montiel, F., Abbondanza, M., et al. (2022). Predictors of progression in untreated keratoconus: a save sight keratoconus registry study. Br. J. Ophthalmol. 106 (9), 1206–1211. doi:10.1136/bjophthalmol-2020-317547
Ferdi, A. C., Nguyen, V., Gore, D. M., Allan, B. D., Rozema, J. J., and Watson, S. L. (2019). Keratoconus natural progression: a systematic review and meta-analysis of 11 529 eyes. Ophthalmology 126 (7), 935–945. doi:10.1016/j.ophtha.2019.02.029
Gomes, J. A., Tan, D., Rapuano, C. J., Belin, M. W., Ambrosio, R., Guell, J. L., et al. (2015). Global consensus on keratoconus and ectatic diseases. Cornea 34 (4), 359–369. doi:10.1097/ico.0000000000000408
Hamilton, A., Wong, S., Carley, F., Chaudhry, N., and Biswas, S. (2016). Tomographic indices as possible risk factors for progression in pediatric keratoconus. J. AAPOS 20 (6), 523–526. doi:10.1016/j.jaapos.2016.08.006
Hashemi, H., Seyedian, M. A., Miraftab, M., Fotouhi, A., and Asgari, S. (2013). Corneal collagen cross-linking with riboflavin and ultraviolet a irradiation for keratoconus: long-term results. Ophthalmology 120 (8), 1515–1520. doi:10.1016/j.ophtha.2013.01.012
Hersh, P. S., Stulting, R. D., Muller, D., Durrie, D. S., Rajpal, R. K., Binder, P. S., et al. (2017). United States multicenter clinical trial of corneal collagen crosslinking for keratoconus treatment. Ophthalmology 124 (9), 1259–1270. doi:10.1016/j.ophtha.2017.03.052
Kanellopoulos, A. J., and Asimellis, G. (2013). Revisiting keratoconus diagnosis and progression classification based on evaluation of corneal asymmetry indices, derived from Scheimpflug imaging in keratoconic and suspect cases. Clin. Ophthalmol. 7, 1539–1548. doi:10.2147/opth.s44741
Kato, N., Masumoto, H., Tanabe, M., Sakai, C., Negishi, K., Torii, H., et al. (2021). Predicting keratoconus progression and need for corneal crosslinking using deep learning. J. Clin. Med. 10 (4), 844. doi:10.3390/jcm10040844
Kymionis, G. D., Grentzelos, M. A., Kounis, G. A., Diakonis, V. F., Limnopoulou, A. N., and Panagopoulou, S. I. (2012). Combined transepithelial phototherapeutic keratectomy and corneal collagen cross-linking for progressive keratoconus. Ophthalmology 119 (9), 1777–1784. doi:10.1016/j.ophtha.2012.03.038
Martinez-Abad, A., and Pinero, D. P. (2017). New perspectives on the detection and progression of keratoconus. J. Cataract. Refract Surg. 43 (9), 1213–1227. doi:10.1016/j.jcrs.2017.07.021
Meyer, J. J., Gokul, A., Vellara, H. R., and McGhee, C. N. J. (2021). Progression of keratoconus in children and adolescents. Br. J. Ophthalmol. 107, 176–180. doi:10.1136/bjophthalmol-2020-316481
Ruiz Hidalgo, I., Rodriguez, P., Rozema, J. J., Ni Dhubhghaill, S., Zakaria, N., Tassignon, M. J., et al. (2016). Evaluation of a machine-learning classifier for keratoconus detection based on Scheimpflug tomography. Cornea 35 (6), 827–832. doi:10.1097/ico.0000000000000834
Saini, J. S., Saroha, V., Singh, P., Sukhija, J. S., and Jain, A. K. (2004). Keratoconus in Asian eyes at a tertiary eye care facility. Clin. Exp. Optom. 87 (2), 97–101. doi:10.1111/j.1444-0938.2004.tb03155.x
Shajari, M., Steinwender, G., Herrmann, K., Kubiak, K. B., Pavlovic, I., Plawetzki, E., et al. (2019). Evaluation of keratoconus progression. Br. J. Ophthalmol. 103 (4), 551–557. doi:10.1136/bjophthalmol-2017-311651
Keywords: cornea, keratoconus, posterior elevation, corneal topography, Pentacam, machine learning
Citation: Han X, Shen Y, Gu D, Zhang X, Sun L, Chen Z and Zhou X (2023) Prediction of posterior elevation stability in keratoconus. Front. Bioeng. Biotechnol. 11:1288134. doi: 10.3389/fbioe.2023.1288134
Received: 03 September 2023; Accepted: 25 October 2023;
Published: 09 November 2023.
Edited by:
Yang Liu, Hong Kong Polytechnic University, Hong Kong SAR, ChinaReviewed by:
Naiyang Li, Zhongshan People’s Hospital (ZSPH), ChinaCopyright © 2023 Han, Shen, Gu, Zhang, Sun, Chen and Zhou. This is an open-access article distributed under the terms of the Creative Commons Attribution License (CC BY). The use, distribution or reproduction in other forums is permitted, provided the original author(s) and the copyright owner(s) are credited and that the original publication in this journal is cited, in accordance with accepted academic practice. No use, distribution or reproduction is permitted which does not comply with these terms.
*Correspondence: Xingtao Zhou, ZG9jdHpob3V4aW5ndGFvQDE2My5jb20=; Zhi Chen, cGV0ZXI0NTlAYWxpeXVuLmNvbQ==
†These authors have contributed equally to this work and share first authorship
Disclaimer: All claims expressed in this article are solely those of the authors and do not necessarily represent those of their affiliated organizations, or those of the publisher, the editors and the reviewers. Any product that may be evaluated in this article or claim that may be made by its manufacturer is not guaranteed or endorsed by the publisher.
Research integrity at Frontiers
Learn more about the work of our research integrity team to safeguard the quality of each article we publish.