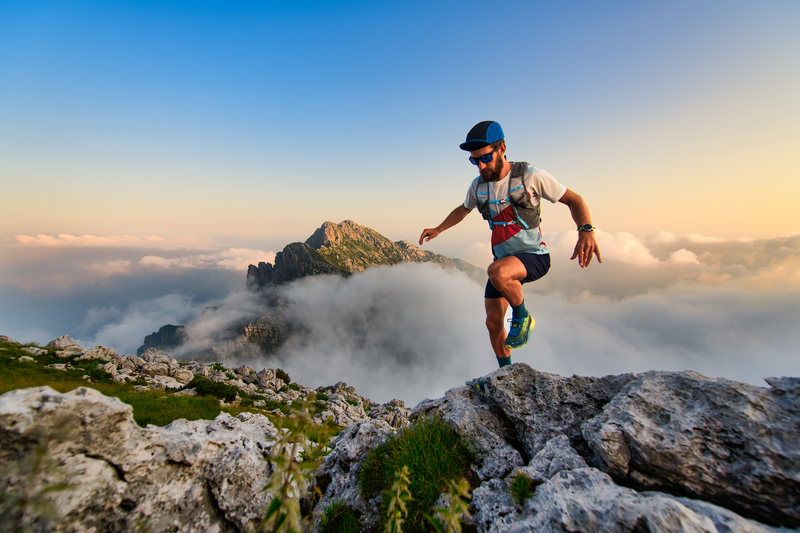
94% of researchers rate our articles as excellent or good
Learn more about the work of our research integrity team to safeguard the quality of each article we publish.
Find out more
ORIGINAL RESEARCH article
Front. Bioinform. , 06 February 2025
Sec. Drug Discovery in Bioinformatics
Volume 5 - 2025 | https://doi.org/10.3389/fbinf.2025.1499255
This article is part of the Research Topic Integrating Bioinformatics and AI to Natural Product-based Drug Discovery and Development View all articles
Background: Diabetes remains a leading cause of morbidity and mortality due to various complications induced by hyperglycemia. Inhibiting Aldose Reductase (AR), an enzyme that converts glucose to sorbitol, has been studied to prevent long-term diabetic consequences. Unfortunately, drugs targeting AR have demonstrated toxicity, adverse reactions, and a lack of specificity. This study aims to explore African indigenous compounds with high specificity as potential AR inhibitors for pharmacological intervention.
Methodology: A total of 7,344 compounds from the AfroDB, EANPDB, and NANPDB databases were obtained and pre-filtered using the Lipinski rule of five to generate a compound library for virtual screening against the Aldose Reductase. The top 20 compounds with the highest binding affinity were selected. Subsequently, in silico analyses such as protein-ligand interaction, physicochemical and pharmacokinetic profiling (ADMET), and molecular dynamics simulation coupled with binding free energy calculations were performed to identify lead compounds with high binding affinity and low toxicity.
Results: Five natural compounds, namely, (+)-pipoxide, Zinc000095485961, Naamidine A, (−)-pipoxide, and 1,6-di-o-p-hydroxybenzoyl-beta-d-glucopyranoside, were identified as potential inhibitors of aldose reductase. Molecular docking results showed that these compounds exhibited binding energies ranging from −12.3 to −10.7 kcal/mol, which were better than the standard inhibitors (zopolrestat, epalrestat, IDD594, tolrestat, and sorbinil) used in this study. The ADMET and protein-ligand interaction results revealed that these compounds interacted with key inhibiting residues through hydrogen and hydrophobic interactions and demonstrated favorable pharmacological and low toxicity profiles. Prediction of biological activity highlighted Zinc000095485961 and 1,6-di-o-p-hydroxybenzoyl-beta-d-glucopyranoside as having significant inhibitory activity against aldose reductase. Molecular dynamics simulations and MM-PBSA analysis confirmed that the compounds bound to AR exhibited high stability and less conformational change to the AR-inhibitor complex.
Conclusion: This study highlighted the potential inhibitory activity of 5 compounds that belong to the African region: (+)-Pipoxide, Zinc000095485961, Naamidine A, (−)-Pipoxide, and 1,6-di-o-p-hydroxybenzoyl-beta-d-glucopyranoside. These molecules inhibiting the aldose reductase, the key enzyme of the polyol pathway, can be developed as therapeutic agents to manage diabetic complications. However, we recommend in vitro and in vivo studies to confirm our findings.
Diabetes Mellitus (DM) is a chronic metabolic disorder characterized by hyperglycemia due to absolute lack, inadequate insulin production, or insulin resistance (i.e., the cells of the body become unresponsive to the insulin’s effects) (Sapra and Bhandari, 2021). Globally, the incidence of diabetes mellitus has surged to epidemic levels, especially in lower and middle-income countries. According to the 2021 International Diabetes Federation (IDF) report, DM affects approximately 575 million adults (20–79 years), who make up 10.5% of the world’s population, and is the seventh leading cause of death worldwide (Vos et al., 2012; IDF Diabetes Atlas, 2021). The complications associated with diabetes include micro- and macrovascular damage (such as diabetic nephropathy), retinopathy, and neuropathy. In addition to these common complications, emerging issues like cancer, liver disease, and cognitive disability also contribute to deaths associated with diabetes (Tomic et al., 2022). Previous studies have examined possible factors linked to the risk of type 1 diabetes (Abolo et al., 2024). High glucose levels in both type 1 and type 2 diabetes activate several metabolic pathways, producing toxic byproducts, which cause pathological and functional changes in various tissues (Forbes and Cooper, 2013). One of the metabolic pathways identified to contribute to the development of many diabetic consequences is the polyol pathway (Srikanth and Orrick, 2022).
The polyol pathway is a two-step metabolic pathway involved in converting glucose to sorbitol through the action of aldose reductase (AR), followed by the conversion of sorbitol to fructose via sorbitol dehydrogenase (Low, 2005). Usually, below normal glucose concentrations, most cellular glucose is directed toward the glycolytic pathway, with only a fraction entering the polyol pathway. However, in hyperglycemic conditions, such as that observed in diabetes, there is a notable increase in the flux through the polyol pathway, accounting for over thirty percent of glucose metabolism (Tang et al., 2012). This heightened activity of the polyol pathway under elevated glucose levels results in a substantial diversion of glucose towards sorbitol production, facilitated by AR, at the expense of cellular nicotinamide adenine dinucleotide phosphate (NADPH), which is a cofactor of AR (Singh et al., 2021a; Gupta, 2023). Given the essential role of NADPH in generating glutathione (GSH), an intracellular antioxidant, the depletion of NADPH by the AR can compromise the cellular antioxidant defense mechanism. Subsequently, sorbitol is converted to fructose by sorbitol dehydrogenase, accompanied by the generation of nicotinamide adenine dinucleotide (NADH), which may contribute to increased reactive oxygen species (ROS) production via NADH oxidase. This cascade of events, including sorbitol accumulation and oxidative stress, is implicated in the pathogenesis of diabetic complications (Tang et al., 2012). The significance of oxidative stress in diabetic complications is highlighted by increased levels of oxidized DNA, proteins, and lipids, which have been extensively studied (Wiernsperger, 2003). Targeting the polyol pathway, specifically by inhibiting AR using aldose reductase inhibitors (ARIs), has emerged as a potential therapeutic strategy for managing diabetic complications (Julius and Hopper, 2019). Clinical studies have demonstrated that AR inhibitors such as sorbinil, tolrestat, and zopolrestat reduce the occurrence of various diabetic complications, including atherothrombotic cardiovascular disease, myocardial ischemia, retinopathy, nephropathy, and neuropathy (Hotta, 2010; Gamal and Munusamy, 2017; Chang et al., 2019; Gopal et al., 2023). However, despite promising preclinical data, the clinical effectiveness of ARIs remains uncertain, and concerns persist about adverse effects such as hepatic damage and neuropathy (Hotta, 2010).
Natural compounds derived from plants, microorganisms, and marine organisms have shown diverse biological activities and unique chemical structures, making them a promising basis for developing new therapeutics (Mishra and Tiwari, 2011). The advancement of genomics, transcriptomics, and proteomics has been crucial in studying biomarkers and genes related to the development of complex traits (Wesonga and Awe, 2022; Nzungize et al., 2022; Chikwambi et al., 2023; El Abed et al., 2023; Nyamari et al., 2023; Ogbodo et al., 2023; Omar et al., 2024; Alaya et al., 2024; Aribi et al., 2024). Natural products have long been an important source of potential drugs for various diseases and conditions. Approximately 35% of modern medicines are estimated to be derived from natural products. Examples include the anti-cancer drug Taxol from the Pacific yew tree and the anti-malarial drug artemisinin from the Artemisia annua plant (Calixto, 2019). Bioinformatics and in silico approaches have experienced huge developments, thereby enabling their applications in various fields such as in identifying potential lead compounds (Enejoh et al., 2025; Hanson et al., 2024), comparative genomics (Awe et al., 2023; Mwanga et al., 2023; Obura et al., 2022) and pipeline development (Ather et al., 2018; Die et al., 2019) and protein structure prediction (Pawar et al., 2024). Natural compounds from Africa present a rich resource for discovering active pharmaceutical ingredients. For example, metformin, a widely used oral antidiabetic drug, originates from Galegaofficinalis, a plant traditionally used across North Africa, the Middle East, and Europe to alleviate diabetes symptoms. The discovery of galegine, an active compound extracted from Galegaofficinalis, led to the development of metformin as an active ingredient (Witters, 2001; Brasileira De Farmacognosia et al., 2006).
Building on the understanding of aldose reductase’s role in diabetic mechanisms and the potential of natural compounds as therapeutic agents, this study utilized computer-aided drug design methods to identify potential inhibitors. These techniques are valuable tools in pharmaceutical research and allow for cost-effective identification and optimization of potential drug candidates, bypassing the limitations of traditional laboratory-based approaches (Zuhri et al., 2022). We employed a virtual screening approach to screen a database of African natural compounds against aldose reductase to identify the most appropriate inhibitors that could serve as potential therapeutics to treat diabetes and its consequences. The molecular docking approach enabled us to assess the binding affinity and interactions between the selected compounds and the enzyme. To validate the reliability of these interactions, molecular dynamics simulations were performed to provide insights into the stability of the protein-ligand complex over time.
This study employed a systematic methodology to identify potent aldose reductase (AR) inhibitors using computational techniques (Figure 1). The process begins with the preparation of the AR protein and a diverse compound library sourced from AfroDB, NANPDB, and EANPDB. The docking protocol was validated by redocking the experimental ligand and receiver operating characteristic (ROC) curve analysis. Virtual screening was conducted via molecular docking to identify compounds with significant interaction potential for inhibiting AR. Following the initial screening, selected compounds underwent pharmacological evaluation, including ADMET (Absorption, Distribution, Metabolism, Excretion, and Toxicity) predictions and analysis of protein-ligand interactions to ensure favorable drug-like properties. To further investigate the interactions, molecular dynamics simulations, and MM-PBSA calculations are performed to assess the stability and binding free energies of the selected complexes.
Figure 1. A graphic illustration of the study’s methodology. The methods involved pre-filtering ligands using Lipinski’s rule of five. Molecular docking, pharmacokinetics profiling by assessing the absorption, distribution, metabolism, excretion, and toxicity (ADMET), protein-ligand interaction analysis, and biological activity predictions were used to identify promising leads.
The three-dimensional (3D) x-ray diffraction structure of the human AR protein was retrieved from the Research Collaboratory for Structural Bioinformatics (RCSB) Protein Data Bank (PDB) (https://www.rcsb.org/structure/1us0; PDB ID: 1US0) (Berman et al., 2000). The 1US0 structure resolved at 0.66 Å was co-crystalized with its cofactor, the Nicotinamide-adenine dinucleotide phosphate (NADP+), and the inhibitor IDD 594 (Howard et al., 2004). The existing inhibitor and water molecules were removed from the protein’s structure using the PyMOL version 3.0.0 software (DeLano, 2002) and saved in the. pdb format. The SwissPDB viewer (Guex et al., 2009) was used to check and resolve the missing residues. Energy minimization was carried out using GROMACS version 2024 (Abraham et al., 2015), and the output. gro file was converted to a PDB file using PyMOL.
The compounds used for this study were retrieved from the AfroDB Database (Ntie-Kang et al., 2013), the Northern African Natural Products Database (NANPDB), and the East African Natural Product Database (EANPDB) (Ntie-Kang et al., 2017). The AfroDB is a library of natural products containing diverse and highly potent molecules from African medicinal plants. The NANPDB and EANPDB are databases comprising natural products from Northern African and Eastern African sources, respectively. A total of 7,344 compounds obtained from combining the databases were pre-filtered based on Lipinski’s rule of five (Lipinski, 2004) using the Data Warrior software (v.06.01.00) (Sander et al., 2015). Lipinski’s rule of five includes a molecular weight of approximately 500 Da, a partition coefficient (cLogP) of less than five, and the ability to form hydrogen bonds (with no more than five hydrogen bond donors and ten hydrogen bond acceptors). Five standard aldose reductase inhibitors (ARIs), namely, zopolrestat, epalrestat, IDD594, tolrestat, and sorbinil were obtained from the chemistry database PubChem (https://pubchem.ncbi.nlm.nih.gov) and incorporated into the pre-filtered compound libraries.
In order to validate the docking protocol, the ligand IDD594 was extracted from the co-crystallized structure of aldose reductase obtained from PDB (1US0) and re-docked into the binding site using Autodock Vina software. The docked binding pose of the IDD594 ligand was then superimposed with the experimentally determined pose of the co-crystallized structure by LigPlot+ (v2.2) (Laskowski and Swindells, 2011) and PyMOL.
To further validate the docking protocol,250 decoys of five aldose reductase inhibitors were obtained from the Directory of Useful Decoys and enhanced (DUD-E) web server to generate the ROC curve (Mysinger et al., 2012). Decoys and compounds have similar physicochemical properties but different 2D topologies to the selected inhibitors. The inhibitors comprised mycretin, tolrestat, IDD594, epalrestat, and sorbinil. The area under the curve (AUC) for the ROC curve was generated by screening a total of 250 decoys and five inhibitors against AR using easyROC version 1.3 (Goksuluk et al., 2016).
Virtual screening of the pre-filtered compounds and the standard ARIs was performed using the Autodock Vina interface via PyRx software v0.8 (Trott and Olson, 2010; Dallakyan and Olson, 2015). The pre-filtered compounds were obtained as 3D data files (sdf) and then uploaded to PyRx’s Open Babel Converter (O’Boyle et al., 2011). The compounds were energy minimized using the default parameters of the Universal Force Field (UFF) and conjugate gradients for the optimization procedure, which consisted of 200 steps. The. sdf files were then converted to protein data bank partial charge and atom type (.pdbqt) files using Open Babel Converter. The energy-minimized AR protein in. pdb format was also imported into PyRx and converted to. pdbqt. A grid box with dimensions of X = 29.3 Å, Y = 25.1 Å, and Z = 28.4 Å, centered at coordinates X = 40.03 Å, Y = 35.18 Å, and Z = 35.97 Å was used to cover the binding site precisely. The exhaustiveness parameter was maintained at its default value of 8 to ensure efficient docking. The protein was kept in a rigid conformation during the docking process while the ligands were treated as flexible entities during the docking simulations, allowing AutoDock Vina to generate up to 9 conformers for each compound. Five standard AR inhibitors namely, epalrestat, IDD594, sorbinil, tolrestat, and zopolrestat were docked against the AR protein to serve as a benchmark. After virtual screening, compounds that performed better than the standard inhibitors were chosen for further investigation.
SwissADME (Daina et al., 2017) and AdmetSAR (Cheng et al., 2012a; Yang et al., 2019) tools were used for Absorption, Distribution, Metabolism, Excretion, and Toxicity (ADMET) predictions. Ligands in SMILES format were used to generate pharmacological profiles. SwissADME and AdmetSAR provided access to parameters and predictive models for the computation of pharmacokinetics, physicochemical properties, drug-likeness, and toxicity of the preselected compounds.
The hydrogen and hydrophobic interactions between AR and the molecules that passed the ADMET test were assessed by LigPlot + using default settings. The protein-ligand complexes generated by PyMOL were saved as. pdb and loaded into Ligplot + to generate 2D schematic representations of the structures and their interactions.
The Prediction of Activity Spectra for Substances (PASS) (Filimonov et al., 2014) was employed to predict the biological activities of the selected compounds based on Bayesian models. PASS uses a training set of 26,000 compounds with known activities to generate the probability of activity (Pa) and inactivity (Pi) for each compound on a scale from 0.000 to 1.000 (Parasuraman, 2011; Agyapong et al., 2021). A compound with Pa > Pi is considered to have a higher likelihood of the predicted activity. The biological activities assessed in this study were aldose reductase inhibition, antidiabetic, anti-inflammatory, and antioxidant properties. Additionally, structural similarity analysis was conducted using the DrugBank tool (Knox et al., 2024), which provides comprehensive drug data, including over 7,800 drugs, to identify compounds with structural similarities to FDA-approved and experimental drugs. This analysis helped determine whether the selected compounds share common structural features with known bioactive molecules, suggesting potential pharmacological properties.
Molecular dynamics (MD) simulations were conducted for 100 nanoseconds (ns), employing the CHARMM36 all-atom force field (July 2022) and the CHARMM-modified three-point transferable intermolecular potential (TIP3P) water model, within the GROMACS software platform, version 2024 (Abraham et al., 2015). The simulations were executed on the high-performance computing (HPC) infrastructure hosted at the West African Centre for Cell Biology of Infectious Pathogens (WACCBIP), at the University of Ghana. The initial MD simulation focused on the unbound aldose reductase protein, utilizing the aldose reductase raw coordinate file obtained from the Protein Data Bank (PDB) as the starting configuration. Subsequently, MD simulations were conducted for the five docked complexes of aldose reductase. Each simulation employed a dodecahedron box with dimensions of 1.0 nm and was solvated with the SPC water model, which was pre-neutralized. Topology files of the compounds were generated using the CHARMM General Force Field (CGenFF) (Vanommeslaeghe and MacKerell, 2012). Energy minimization was performed over 1,000 steps using the Steepest Descent (SD) algorithm. Position restraints were applied to the AR protein and the ligands, followed by temperature equilibration at 300 K and pressure equilibration at 1 bar, each conducted for 50,000 picoseconds. Subsequently, production MD runs were carried out for 100 nanoseconds, with temperature and pressure maintained at 300 K and 1 bar, respectively. After MD, the output files were visualized and analyzed for the radius of gyration (Rg), root mean square deviations (RMSD), and root mean square fluctuations (RMSF) of the atoms for each amino acid residue using XMGRACE, Version 5.1.19 (Turner, 2005).
The Molecular Mechanics Poisson-Boltzmann Surface Area (MM-PBSA) technique was utilized to compute the binding free energies of the protein-ligand complexes (Wang et al., 2017). Molecular mechanics and continuum solvent models are combined in MM-PBSA to yield the binding energy components and the individual energy contributions of the AR residues. The graphs from the MM-PBSA computations were then plotted using the RStudio programming software version 2023.6.0.421 (Posit team, 2023).
In this study, we considered the aldose reductase (AR) protein structure 1US0 with the lowest structure resolution of 0.66 Å and an R-value of 0.094 compared with other AR proteins in the protein database. The selected AR structure was compared with equally solved structures such as 4IGS, 1PWM, and 4LBS, with resolutions 0.85, 0.92, and 0.76 Å, respectively, and R-values of 0.143, 0.129, and 0.134, respectively. A highly resolved structure has well-defined substructures and features, including the active site region, bond density, and significant deviations from standard stereochemistry (Howard et al., 2004). The AR protein with PDB ID: 1US0 exists as a 37.4 kDa monomeric protein with 316 amino acids and an active site that is bound to cofactor NADP+ and inhibitor IDD594 which were removed to make the active site available for molecular docking of the compounds in this study.
In preparing the EANPDB, NANPD, and AfroDB compound libraries for molecular docking, Lipinski’s rule of five was applied to pre-filter compounds eliminating those that failed to meet Lipinski’s criteria. Lipinski’s rule of five is a widely accepted method for evaluating the drug-likeness of compounds. It combines computational and experimental techniques to assess the likelihood of absorption or permeation of the compound (Mahgoub et al., 2022). The rule states that a compound is more likely to be a viable drug candidate if it satisfies certain criteria, which include having less than five hydrogen bond donors, less than 10 hydrogen bond acceptors, a molecular weight greater than 500, and a calculated Log P (cLogP) value exceeding five (or MlogP > 4.15) (Lipinski, 2004; Devadasu et al., 2018). After pre-filtering, 2,968 out of 7,344 compounds in compound libraries satisfied the rule and were therefore considered for docking.
Validation of a docking protocol is a crucial step in any in silico study that utilizes molecular docking. Several studies have shown that the validation process is useful for assessing the accuracy and robustness of a chosen protocol for the specific system being studied (Shivanika et al., 2022; Granchi et al., 2015). Validation is generally achieved using various procedures some of which include, re-docking, super-imposition, use of docking decoys, comparison with other docking programs, and so on (Hevener et al., 2009). However, for this study, validation by superimposition and validation by ROC curve analysis using docking decoys were used to assess the docking protocol.
This validation method involves extracting the inhibitor (IDD594) from the experimental crystal (1US0) and re-docking it into the binding site of the aldose reductase protein. The re-docked structure was superimposed using PyMOL (v.3.0.0) on the co-crystallized protein-ligand complex resulting in an RMSD of 0.211Å (Figure 2A). Using LigPlot (v.2.2.8), IDD594 was observed to bind into the binding site via hydrogen bonding with His110, Trp111, Tyr48, Thr113 and hydrophobic interactions with Trp20, Trp219, Phe122, and Leu300 as shown in Figure 2B. The validation process with an RMSD of 0.000 is indicative of a very high degree of similarity between the docked ligand pose and the reference ligand pose (Hevener et al., 2009), thereby suggesting a high degree of accuracy in predicting the ligand’s binding pose with similarity in hydrogen and hydrophobic bond interactions.
Figure 2. Validation of docking protocol by superimposition (A) Superimposed image showing the structural alignment of the docked complex of native IDD594 ligand (green) and re-docked ligand (blue) with protein represented in cartoon and ligand represented in sticks. (B) LigPlot+ of superimposition between the co-crystallized ligand of aldose reductase (1US0) and the re-docked IDD594 ligand. Red circles represent the superimposed molecular interactions between the co-crystallized and the re-docked ligands.
To further validate the docking protocol used in the study, the Area under the curve (AUC) of the Receiver Operating Characteristic (ROC) curve was used. This is an indicator for assessing a docking model’s capacity to differentiate between docked decoys and active ligands (Bowers and Zhou, 2019). When tested against the aldose reductase protein (1US0), the ROC curve depicts the overall docking performance in distinguishing between active and decoy ligands (Figure 3). An AUC of ROC closer to 1 indicates that the model can differentiate between active ligands and decoys more effectively (Guterres and Im, 2020). The results showed an AUC value of 0.773 for the ROC curve when evaluating the 5 active inhibitors and 250 decoys independently against the aldose reductase model 1US0.
Figure 3. An ROC curve generated after screening 255 compounds consisting of five inhibitors and 250 decoys against the 3D model of the aldose reductase. An acceptable AUC of 0.773 was obtained.
Molecular docking is a method for predicting the binding mode and binding affinity of a small molecule compound to the binding site of a target protein based on its structural properties (Guterres and Im, 2020; Khan et al., 2023). The binding affinities for the 2,968 pre-filtered compounds ranged from −12.3 to −3.6 kcal/mol. The more negative the affinity score, the stronger the bond between the compound and the protein. Zopolrestat, a standard inhibitor, had the highest binding affinity of −9.9 kcal/mol and was used as the benchmark for selecting the best compounds. This resulted in the selection of 105 compounds (Supplementary Table S1). However, due to computational limitations, only the best 20 compounds were selected for downstream analysis. The molecular docking results for the top 20 compounds and 5 standard aldose reductase inhibitors are shown in Table 1. The affinity scores of these 20 compounds ranged between −12.3 kcal/mol and −10.7 kcal/mol. The compound 4,5-di-p-trans-coumaroylquinic_acid had the highest binding affinity score of −12.3 kcal/mol, while 1,6-di-o-p-hydroxybenzoyl-beta-d-glucopyranoside had the lowest binding affinity score of −10.7 kcal/mol. All top 20 compounds had a higher binding affinity than the known standard inhibitors, with binding affinity ranging between −9.9 kcal/mol and −7.7 kcal/mol.
ADMET predictions were performed on the top 20 compounds to assess their drug-like properties. A potential lead compound should have a favorable ADMET profile, including appropriate absorption, distribution, metabolism, excretion, and toxicity properties. The results from SwissADME describe the absorption, distribution, and metabolism of the ligands in this study which are illustrated in Tables 2, 3.
Table 3. Pharmacokinetics properties of the predicted compounds and standard inhibitors. The pharmacokinetics properties comprised cytochrome inhibition, the blood-brain barrier permeability (BBB), P-glycoprotein (P-gp) substrates, and gastrointestinal (GI) absorption and log Kp.
All the compounds including the standard inhibitors complied with Lipinski’s rule of five as shown in Table 2, because the compound library was prefiltered based on Lipinski’s rule of 5. The bioavailability score is the likelihood that a substance will possess an oral bioavailability of no less than 10% in rats and be detected by Caco-2 permeability (Stelzl et al., 2005). This is represented by a value range of 0–1 in SwissADME, which can be translated to a percentage (Martin, 2005). All the ligands in this research study have a bioavailability score of 0.55 except 4,5-di-p-trans-coumaroylquinic acid, which has a bioavailability score of 0.11, and (+)-strigol has 0.56; this could be translated to a bioavailability score of 55%, 11%, and 56%, respectively (Table 2). However, all the standards had a bioavailability score of 0.55 except for epalrestat and sorbinil which had a score of 0.56.
As indicated in Table 3, compounds that were not inhibitors of any cytochrome P450 enzymes include 4,5-di-p-trans-coumaroylquinic acid, Zinc000095485961, Lactupicrin, (+)-strigol, and Zinc000095485890. These results suggest that they are less likely to be involved in drug-drug interactions and have adequate drug elimination properties via metabolic biotransformation. All the predicted ligands exhibited high gastrointestinal absorption except 4,5-di-p-trans-coumaroylquinic acid. Zinc000000134782, Abyssinone II, and (+)-pipoxide-2-methyl ether compounds were found to show potential permeability through the blood-brain barrier among all the compounds from the SwissADME prediction. In vivo and in vitro studies have shown that P-glycoprotein is important for drug absorption and clearance in the liver and kidney. In the brain, it also acts as a rate-limiting factor for drug uptake from blood circulation into the brain; in the intestinal lumen, it is a binding agent for drug absorption into epithelial cells (Lin and Yamazaki, 2003). Drugs that bind to P-glycoprotein (Pgp) are regarded as Pgp substrates. 4,5-di-p-trans-coumaroylquinic acid, Zinc000095485961, Tricoccin_s13_acetate, Lactupicrin, Naamidine A, Isosamarcandin, and 1,6-di-o-p-hydroxybenzoyl-beta-d-glucopyranoside show potential to bind to P-glycoprotein from the SwissADME prediction. Log Kp (expressed in cm/s) represents a crucial indicator of a drug or ligand’s ability to permeate the skin, especially if the mode of administration is transdermal (Chen et al., 2018). A compound is typically considered to have limited skin permeability when its log Kp is greater than −2.5 cm/s (Pires et al., 2015). In the study, all the predicted compounds have values ranging from −8.43 to −5.20 cm/s, indicating extremely low skin permeability.
The prediction of the toxicological properties of the ligands used for docking was achieved using admetSAR 2.0 (Cheng et al., 2012b). Hepatotoxicity, acute oral toxicity, nephrotoxicity, carcinogenicity, and mutagenicity toxicological properties were recorded, as shown in Table 4. The results from the admetSAR also showed that none of the ligands is carcinogenic (Table 4). The compounds that exhibited positive toxicology predictions for hepatotoxicity, acute oral toxicity, nephrotoxicity, carcinogenicity, and mutagenesis were eliminated leaving eight compounds namely, (+)-pipoxide, Zinc000095485961, Naamidine A, Sigmoidin B 4′-methylether diacetate, (−)-pipoxide, (+)-strigol, Isosamarcandin, and 1,6-di-O-p-hydroxybenzoyl-beta-D-glucopyranoside. These selected compounds stand out due to their high bioavailability, minimal Central Nervous System (CNS) side effects, low drug-drug interaction potential, and excellent safety profiles across key toxicological endpoints, making them promising candidates for targeting aldose reductase in managing diabetic complications. Additionally, epalrestat and IDD549, where the standard inhibitors showed no toxicity for the selected toxicity profiles.
Table 4. The toxicology of the ligands is based on the linear regression model prediction from admetSAR. The negative symbol (−) indicates a negative prediction of being toxic while the positive symbol (+) indicates a negative prediction of being non-toxic.
The eight compounds and the standard inhibitor (epalrestat) identified through the ADMET studies underwent analysis to assess their interactions with the active site of the aldose reductase protein (Table 5). The amino acids comprising this active site were determined from literature reviews, as follows: His110, Asp43, Lys77, Cys298, and Tyr48 (Tarle et al., 1993); and Trp111, Trp20, Phe122, Thr113, Leu300, Ser210, and Trp219 (Ashik et al., 2022). Notably, 1,6-di-o-p-hydroxybenzoyl-beta-d-glucopyranoside, (+)-pipoxide, and (+)-strigol exhibited hydrogen bonding interactions with residue Trp111, with bond lengths of 3.03, 3.16, and 3.29, respectively. Naamidine A formed hydrogen bonds with Trp20 and Thr113, with bond lengths of 3.01 and 3.13, respectively. Moreover, 1,6-di-o-p-hydroxybenzoyl-beta-d-glucopyranoside and Zinc000095485961 were observed to interact with Ile260, His110, and Thr113 via hydrogen bonds (Table 5). However, Sigmoidin-b-4′-methylether diacetate and (+)-strigol, despite forming hydrogen bonds, did not interact with the crucial residues of the aldose reductase active site, leading to their exclusion from further studies. Isosamarcandin was also excluded due to its lack of interaction with any residues via hydrogen bonding. Selection of compounds solely based on interactions with only the critical residues of the aldose reductase active site resulted in five compounds being selected as the top compounds. Notably, the standard inhibitor interacted with the active site solely through hydrophobic interactions implying that our selected compounds may form stronger interactions when bound to AR than the standard epalrestat (Table 5; Figure 4).
Table 5. This table depicts the interactions between AR binding site residues and the top 20 compounds via hydrogen bonding and hydrophobic interactions. The length of the hydrogen bonds’ interactions is also shown.
Figure 4. Epalrestat docked firmly in the binding pocket and interacting with critical residues. (A) Surface view of the AR protein and epalrestat (blue). (B) 2D diagram of the protein-ligand interaction generated using LigPlot+.The ligand is colored in purple, and hydrophobic contacts are represented as red spoke arcs.
The PASS software predicted the biological activities of the five selected compounds. Zinc000095485961 and 16-di-o-p-hydroxybenzoyl-beta-D-glucopyranoside were predicted to possess all four biological activities which were aldose reductase inhibition, antidiabetic, anti-inflammatory, and antioxidant with high Pa values for each activity (Table 6). Zinc000095485961 had Pa values of 0.679 for antioxidant, 0.636 for anti-inflammatory, 0.596 for antidiabetic, and 0.109 for aldose reductase inhibition, all greater than their Pi values (Table 6). Similarly, 16-di-o-p-hydroxybenzoyl-beta-D-glucopyranoside exhibited Pa values of 0.724 for anti-inflammatory, 0.681 for antioxidant, 0.568 for antidiabetic, and 0.205 for aldose reductase inhibition, also showing strong potential for these activities. (+)-Pipoxide and (−)-Pipoxide were predicted to have anti-inflammatory (Pa = 0.327) and antioxidant (Pa = 0.162) activities, although they were not predicted to have aldose reductase inhibitory or antidiabetic properties. Naamidine A did not exhibit significant activity in any of the assessed biological categories according to the PASS predictions, but its known anticancer properties suggest other potential uses.
Table 6. The table shows the names of the compounds and their predicted biological activity with their corresponding probability of activity (Pa) and the probability of inactivity (Pi). Selected pharmacological activity in this study includes aldose reductase inhibition, anti-inflammatory, antidiabetic, and antioxidant activity. Additionally, the table shows structural similarity scores for compounds compared to known drugs.
In the structural similarity analysis (Table 6), Zinc000095485961 showed a high similarity score with Acteoside (0.891) and Echinacoside (0.887), both of which are known bioactive compounds. Also, 16-di-o-p-hydroxybenzoyl-beta-D-glucopyranoside exhibited notable structural similarity to Beta-12346-Penta-O-Galloyl-D-Glucopyranose (0.873) and Tannic Acid (0.739). These findings suggest that these compounds may share pharmacological properties with known drugs. In contrast, (+)-Pipoxide, (−)-Pipoxide, and Naamidine A did not demonstrate significant structural similarity to any known drugs in the DrugBank database.
An RMSD plot over simulation time revealed the backbones of the five complexes after 100 ns in comparison to the unbound AR protein and a standard inhibitor (Epalrestat-AR) complex (Figure 5A). The unbound protein at the initial start of the simulation had an RMSD of 0.11 nm that gradually increased to 0.16 nm and after 16 ns decreased steadily till 22 ns where it attained an RMSD of 0.14 nm. A steady increase was observed after the 22 ns time and maintained a relatively steady RMSD of 0.24 nm at 38 ns till the end of the 100 ns simulation. The standard inhibitor (Epalrestat-AR) complex had an initial RMSD of 0.09 nm which increased steadily to 0.17 nm at 40 ns and then decreased to 0.14 nm at 65 ns after RMSD was maintained at an average RMSD of 0.14 nm in the remaining simulation time. Zinc0009548961-AR complex rose from 0 nm to 0.08 nm at the start of the simulation and then maintained an average RMSD of 0.12 nm over the remaining simulation time. The 1,6-di-o-p-hydroxybenzoyl-beta-d-glucopyranoside (1,6 DHG)-AR complex had an RMSD of 0.11 at the start of the simulation and peaked at 0.15 nm/25 ns; then it decreased to 0.125 at a maintained RMSD. It peaked around 70 ns and later averaged at about 0.12 nm over the remaining simulation time. For (+)- pipoxide, the RMSD started at 0.1 nm and steadily increased to 0.15 nm within the first 12 ns. It then declined to 0.125 nm after 25 ns. The RMSD was maintained at 0.125 nm till 50 ns where it increased to 0.18 nm and then maintained an average RMSD of 0.18 nm till the end of the simulation. (−)- Pipoxide initially had an RMSD of 0.15 nm at the start of the simulation which then declined to 0.13 nm. An average RMSD of 0.13 nm was then maintained throughout the simulation. The Naamidine-AR complex showed the most stable conformation by maintaining an RMSD of 0.1 nm in the 100 ns simulation.
Figure 5. MD simulations on five selected natural compounds and the standard inhibitor (epalrestat). A 100 ns MD simulation was performed to analyze the structural stability and conformational changes of aldose reductase when bound to the complexes. The parameters considered shown in the graphs include (A) the root mean square deviation (RMSD), (B) the radius of gyration (Rg), and (C) the root mean square fluctuation (RMSF).
The compactness of the complexes was evaluated using the radius of gyration (Rg). The Rg values obtained from the simulations showed that all AR-complexes including the unbound protein (Figure 5B) remained in their compact (folded) form throughout 100 ns. All AR complexes maintained their Rg from the beginning of the simulation (0 ns) to the end (100 ns) within or fluctuations. The average Rg of the unbound protein and AR-complexes, epalrestat, (+)-pipoxide, Zinc000095485961, 1,6-di-o-p-hydroxybenzoyl-beta-d-glucopyranoside, Naamidine A and (−)-pipoxide were 1.940, 1.937, 1.929, 1.937, 1.929, 1.941 and 1.943 nm, respectively. The differences in Rg between the AR-complexes and the standard inhibitors were small however, Zinc000095485961, Naamidine A, and 1,6-di-o-p-hydroxybenzoyl-beta-d-glucopyranoside had a slightly lower Rg compared to the unbound protein and epalrestat.
The stability of the individual residues was assessed using their Root Mean Square Fluctuation (RMSF) plots (Figure 5C). All the complexes possessed similar residue fluctuations within the same regions, with little deviation from the unbound protein and the epalrestat-AR complex (Figure 5C). High residue fluctuations were observed within amino acid residues at positions 2, 8, 25, 118, 125, 173, 224, 225, 264, and 312. Residue fluctuations between 0.1 and 0.2 nm were generally observed within the residues. However, a rapid increase to 0.5 nm RMSF within residues 205–225 was observed and this might be indicative of the region with the highest flexibility.
The study employed the MM-PBSA approach to elucidate the binding free energies of the top five selected compounds: (+)-pipoxide, Zinc000095485961, Naamidine A, (−)-pipoxide, and 1,6-di-o-p-hydroxybenzoyl-beta-d-glucopyranoside compared to the standard inhibitor (epalrestat). These compounds exhibited binding free energies of −115.073, −106.483, −98.523, −93.751, and −92.007 kJ/mol, respectively Notably, all five compounds displayed higher binding free energy, Van der Waals energy, electrostatic energy, polar solvation energy, and Solvent-Accessible Surface Area (SASA) when compared to epalrestat (Table 7). Additionally, the contribution of each residue’s energy via MM-PBSA decomposition was determined to identify the active site residues of AR involved in ligand binding. Residues with energy contribution exceeding >5.0 or < −5.0 are considered critical residues for protein-ligand binding (Kwofie et al., 2019). For Zinc000095485961 Trp20, Trp111, and Trp219 were the identified critical residues with binding energies of 9.453, 8.032, and 5.031 kJ/mol (Figure 6), respectively. Asp43, a critical residue contributed a high energy of 15.089 kJ/mol in all complexes. For 1,6-di-o-p-hydroxybenzoyl-beta-d-glucopyranoside, Trp20, Trp111, and Leu300 were identified as critical residues with contributed energies of −6.564, −8.187, and 5.342 kJ/mol, respectively (Supplementary Figure S1). Asp43 also had a significantly high energy of 18.011 kJ/mol. Trp208 was not a critical residue but contributed less than −5 kJ/mol. Regarding (+)-pipoxide, Trp20, Trp111, and Phe122 were the critical residues with energies exceeding 5 and below −5 (Supplementary Figure S2). Although Trp43 is not a critical residue, it showed a significant contribution of −5.956 kJ/mol. For (−)- pipoxide-AR complex, Trp20 contributed low energy of −7.654 kJ/mol, with Trp48 also peaking at −9.765 kJ/mol (Supplementary Figure S3). Asp 43 contributed an energy of 9.678 kJ/mol, although it is not a critical residue. Finally, in the naamidine-AR complex, Trp20, Trp111, and Leu300 were the critical residues with a low binding affinity of −6.987, −7.865, and −6.132 kJ/mol, respectively (Supplementary Figure S4). Chemical structures for the five leads and epalrestat are shown in Table 8.
Table 7. The table below shows the binding energies and the contributing energy terms of the AR-ligand complexes from the MMPBSA calculation. The values are presented in average ± standard deviations in kJ/mol.
Table 8. Chemical structures of (A–E) the top 5 identified compounds and (F) Epalrestat (Standard Inhibitor).
Figure 6. Molecular Mechanics Poisson-Boltzmann Surface Area (MMPBSA) plot of binding free energy contribution per residue of AR- Zinc000095485961 complex. Fluctuations by selected critical residues of AR are shown in red.
The use of natural bioactive compounds including flavones, flavonoids, and coumarins derived from naturally occurring plants, for aldose reductase enzyme inhibition has gained traction in recent years. Numerous studies have investigated the inhibitory effects of synthetic and natural compounds on aldose reductase to mitigate the chronic complications of diabetes, such as nephropathy, retinopathy, and neuropathy (Gamal and Munusamy, 2017; Dănilă et al., 2024). Compounds like epalrestat, sorbinil, tolrestat, and fidarestat have demonstrated significant inhibitory activity against the enzyme in animal models. However, many of these compounds were withdrawn from the market due to adverse effects and lack of selectivity for enzymes sharing sequence homology with aldose reductase, such as aldehyde reductase (Antony and Vijayan, 2015). Among them, only epalrestat, a synthetic aldose reductase inhibitor has successfully undergone clinical trials and is commercially available for treating diabetic neuropathy in Japan and other regions (Zhu and Zhu, 2013). Given the rising prevalence of diabetic complications worldwide, there is an urgent need for alternative and more effective aldose reductase inhibitors to manage diabetic complications. This study is thus aimed to identify natural compounds from the African region with inhibitory activity against aldose reductase.
After screening the pre-filtered library of 2,968 compounds of African origin against aldose reductase (PDB: 1US0), the predicted binding affinities ranged from −12.3 to −3.6 kcal/mol, showcasing a wide range of interaction strengths with the aldose reductase protein. This range highlights the diversity of the compound library and the ability of the docking protocol to discern variations in binding strengths. Among the standard inhibitors screened, zopolrestat, a known aldose reductase inhibitor, had the highest binding affinity of −9.9 kcal/mol and was used as a benchmark. Out of the screened library, 105 compounds displayed binding affinities equal to or better than zopolrestat. This represents approximately 3.5% of the screened compounds, indicating a stringent selection process. To ensure computational feasibility, the top 20 compounds were prioritized for further analysis. These compounds demonstrated binding affinities ranging from −12.3 to −10.7 kcal/mol, significantly outperforming zopolrestat. Such a clear improvement over the benchmark compound indicates the potential of these molecules as aldose reductase inhibitors.
The selected standard inhibitors of aldose reductase include epalrestat, IDD594, sorbinil, tolrestat, and zopolrestat with respective binding affinities of −8.8, −8.1, −7.4, −7.6, and −9.9 kcal/mol, and have been shown to have considerably high inhibitory activity both in vitro and in vivo. Based on bioactivity studies, sorbinil has been shown to have an IC50 of 3.14 μM (Shehzad et al., 2021). In a study involving streptozotocin diabetic mice, zopolrestat was studied to inhibit at a low IC50 of 0.004 μM (Mylari et al., 2003). IDD594 was studied to have an IC50 of 0.030 μM and is known to be an effective inhibitor of AR (Podjarny et al., 2004). Tolrestat has been studied to have an effective IC50 of 0.0012 µM in a streptozotocin-induced diabetic rat model (Van Zandt et al., 2005). These studies suggest that these standards exhibit notable inhibitory effects on aldose reductase. Nonetheless, the selected top 20 compounds had a higher binding affinity for AR binding sites than all the standard ARIs used in this study highlighting a high potential of the selected compounds as an ARI.
To develop innovative therapeutic agents, it is important to have a thorough understanding of the complex pharmacokinetic dynamics, thereby elucidating the compound’s behavior within the biological environment. This process involves assessing Absorption, Distribution, Metabolism, Excretion, and Toxicity (ADMET) parameters to screen compounds for favorable physicochemical properties (Flores-Holguín et al., 2021; Tian et al., 2015). In this study, compounds exhibiting favorable solubility, pharmacokinetic profiles, and toxicity profiles were meticulously selected. Based on these stringent criteria, eight compounds, namely, (+)-pipoxide, Zinc000095485961, Naamidine A, Sigmoidin-b-4′-methylether diacetate, (−)-pipoxide, (+)-strigol, Isosamarcandin, and 1,6-di-o-p-hydroxybenzoyl-beta-d-glucopyranoside, were identified as possessing drug-like properties with suitable pharmacokinetics and low toxicity. These compounds performed better than some of the standard inhibitors namely, sorbinil, tolrestat, and zopolrestat used in this study.
Intermolecular interactions like hydrogen bonding and hydrophobic interactions play pivotal roles in stabilizing energetically favorable ligands within the open conformational environment of protein structures (Coimbra et al., 2020; Varma et al., 2010). These interactions enhance ligand stability at the target site, influencing binding affinity and drug efficacy (Lou and Martin, 2021). Specifically, (+)-pipoxide, Zinc000095485961, Naamidine A, (−)-pipoxide, and 1,6-di-o-p-hydroxybenzoyl-beta-d-glucopyranoside were chosen from the 8 compounds due to their interaction with specific active site residues of aldose reductase through multiple hydrogen bonds (Coimbra et al., 2020). Hydrogen bonding is essential in drug design, influencing structural stability, enzyme catalysis, and drug partitioning and permeability. Functional groups capable of forming hydrogen bonds in a drug increase their ability to interact with biomolecular targets, enhancing binding and selectivity (Coimbra et al., 2020). Hydrophobic interactions significantly improve inhibitor affinity and selectivity in drug design, with even minor modifications such as adding a methyl group yielding substantial effects (Lou and Martin, 2021). The Hydroxyl groups, benzene rings, and glycosidic linkages of these compounds catalyze the hydrogen bonding and the interactions with the catalytic residues of AR (Lou and Martin, 2021). Zinc000095485961 and Naamidine A’s glycosidic linkage and hydroxyl groups enable strong hydrogen bonding with His110 and Tyr48 (Kingsley et al., 2013) while 1,6-di-o-p-hydroxylbenzoyl-beta-D-glucopyranoside benzene ring and hydroxyl group catalyzes hydrophobic interactions with Trp111 and Trp20. The study showed that the selected compounds have stronger interactions in the AR binding site making them potentially higher inhibitors than the known drug epalrestat.
The integration of PASS and structural similarity analysis provided valuable insights into the biological potential of selected compounds. Our study focused on aldose reductase inhibition, antidiabetic, anti-inflammatory, and antioxidant properties, essential for managing diabetes by addressing glycemic control, oxidative stress, and inflammation. Zinc000095485961 and 1,6-di-o-p-hydroxybenzoyl-beta-D-glucopyranoside were predicted to possess all four activities. Zinc000095485961, identified as eutigoside A, is a natural product from Stereospermum acuminatissimum, used for its hemostatic and antiseptic properties in African countries (Leutcha et al., 2023; Sob et al., 2011). Its antidiabetic potential may be attributed to eutigoside A (Kingsley et al., 2013). High structural similarity to drug molecules such as acteoside and echinacoside further supports its potential for drug development. Similarly, 1,6-di-o-p-hydroxybenzoyl-beta-D-glucopyranoside, derived from Tabebuia species, has been studied to demonstrate antioxidant, anti-inflammatory, and antidiabetic properties (Govindappa et al., 2013; Murugan et al., 2017; Jimenez-Gonzalez et al., 2018). The lead compound (−)-Pipoxide, from Uvaria dependens and Uvaria dependensis, traditionally used to treat malaria, exhibited anti-inflammatory, antifungal, antioxidant, and antiviral properties (Nkunya et al., 1993; Mayeka et al., 2024). Similarly, its enantiomer, (+)-pipoxide, derived from Monanthotaxis buchananii, displayed similar properties (Liang et al., 1988; Mayeka et al., 2024). While Naamidine A did not exhibit predicted biological activity in our selected parameters, it has been extensively reported for its anticancer activity (LaBarbera et al., 2009; Vaden et al., 2019; LaBarbera et al., 2009; Vaden et al., 2019). Also, (+)-pipoxide, (−)-pipoxide and Naamidine-A showed no significant structural resemblance to known drugs. This highlights the novelty of these compounds and the need for further exploration and testing.
To further validate the selected compounds as lead candidates in this study, we performed molecular dynamics simulations (MDS). MDS serves as a computational tool to elucidate the impact of atomic alterations within a molecular system (Knapp et al., 2011). These simulations provide insights into atomic movements and intermolecular interactions over time, capturing the dynamic behavior and positional changes of atoms (Adelusi et al., 2022). Post-simulation analysis conducted, such as Root Mean Square Deviations (RMSD), Root Mean Square Fluctuations (RMSF), Radius of Gyration (Rg), and hydrogen bonds offer valuable insights into the stability of protein-ligand complexes throughout the simulation period (Arthur et al., 2024; Hanson et al., 2025). These analyses are pivotal for assessing their functional reliability within living systems, thereby influencing the efficacy of drug candidates, a critical aspect in drug discovery (Adelusi et al., 2022; Ashley et al., 2024). In our molecular dynamics (MD) analysis, we compared selected compounds to the standard aldose reductase inhibitor, epalrestat, which is clinically approved for treating diabetic peripheral neuropathy symptoms (Zhu and Zhu, 2013; Ramirez and Borja, 2008).
The Root Mean Square Deviation (RMSD) serves as an indicator of the structural stability of the aldose reductase (AR)-ligand complex (Kuzmanic and Zagrovic, 2010). This study revealed a consistent RMSD profile throughout the 100 ns simulation, suggesting that all the selected compounds maintain the structural stability of the protein upon binding. Literature suggests that RMSD values between 0.15 and 0.25 nm indicate enhanced stability (Arnittali et al., 2021), further emphasizing the stable conformation of all five selected compounds having an average RMSD below 0.2 nm. A stable conformation results in increased affinity to AR thereby increasing the efficacy as a drug (Maruyama et al., 2023). Furthermore, the Radius of Gyration (Rg) analysis highlighted stable protein compactness, indicating that the ligands effectively maintain the protein’s compact conformation upon binding. A fluctuating Rg reveals the lack of compactness which may be due to protein unfolding (Jiang et al., 2019; Kwofie et al., 2022). In this study, all compounds showed a stable Rg and did not differ from the unbound protein which means that the ligands can maintain the compact conformation of aldose reductase when bound to the binding site. Similarly, the Root Mean Square Fluctuation (RMSF) analysis elucidates changes in conformation induced by compound binding, particularly with specific amino acid residues of the protein (Abdullah et al., 2023). A fluctuating or unstable binding site results in a weak binding which increases the energy required for effective binding, making the interaction thermodynamically unfavorable (Du et al., 2016). The highest fluctuation was between residues 210 to 230. Within this region is the loop region (214–230) which exhibits significant flexibility facilitates the binding of diverse substrates and enables conformational adjustments essential for AR’s functional promiscuity. Notably, the observed fluctuations did not significantly differ from those of the unbound protein and the standard inhibitor, indicating minimal influence of the selected compounds on protein conformation. This finding underscores the stability of the AR-ligand complex and the potential for effective inhibition against AR.
The MM-PBSA was calculated to elucidate the binding free energies of five selected compounds. Results indicated high binding free energies, which validate the docking results as the lead compounds’ high binding free energies coincide with the molecular docking’s high binding affinity. Van der Waals energy and the electrostatic energy of the top-selected compounds show strong indicators of stable binding energy to aldose reductase, with an effect stronger than the standard inhibitor. The polar solvation and SASA energy of the compounds are well-balanced, indicating effective hydrophobic packing and solvent exclusion during their inhibitory activities in biological phenomena with aldose reductase. The top five selected ligands exhibit optimal molecular interactions, characterized by strong binding, specificity, and stability, indicating their potential therapeutic effectiveness in effectively inhibiting aldose reductase.
To effectively inhibit aldose reductase, the selected compounds should dock within the binding site and interact with critical residues involved in protein-ligand interactions. Upon evaluating the energy decomposition and binding interactions of the amino acid residues of AR and the ligand complex, it was observed that there were significant binding interactions with critical residues such as His110, Tyr48, Trp111, Trp20, Phe122, Asp43, Cys298, and Trp219, which have also been previously reported in literature as active site residues of AR (Singh et al., 2021b; Ashik et al., 2022). These interactions highlight important inhibitory mechanisms of the selected compounds on aldose reductase. Comparatively, the energy contribution of the critical residues of the selected five compounds is considerably higher than that of the standard inhibitor highlighting the potential of the selected compounds as effective inhibitors.
In this study, out of 7,344 African compounds being studied, (+)-pipoxide, Zinc000095485961, 1,6-di-o-p-hydroxybenzoyl-beta-d-glucopyranoside and Naamidine A, (−)-pipoxide were concluded as the top lead compound with molecular binding to aldose reductase, no toxicity and inhibitory activity against aldose reductase. Molecular docking studies predicted a low binding energy of the successful compounds compared to the standard ARIs, suggesting that the predicted compounds have a higher binding affinity to the aldose reductase enzyme. These compounds were shown to possess strong interactions with the binding site residues through hydrogen bonding as well as hydrophobic interactions. Also, through ADMET studies, these compounds have revealed favorable pharmacokinetic properties with no predicted toxicity. Molecular dynamics simulations validated these findings by producing a lower and stable RMSD and Radius of gyration. Equally, relatively similar fluctuations in RMSF indicate stable amino acid residue conformation when the ligands bind to aldose reductase. MM-PBSA analysis confirmed the docking results and highlighted energies contributed by interacting residues when AR is bound to the selected compound. With epalrestat, a potent known inhibitor used as a standard and a benchmark, this study has strongly emphasized the potential of natural African compounds as potential therapeutics in managing diabetic complications. While computational methods such as molecular docking and dynamics simulations provide valuable insights, they are inherently limited by the potential for false positives in predicting compound efficacy. These computational predictions may not accurately reflect the complex biological interactions in vivo, necessitating further validation through experimental studies. In vitro and in vivo studies are necessary steps to prove the effectiveness of these compounds as potential therapeutic AR inhibitors to mitigate complications in diabetic patients.
The original contributions presented in the study are included in the article/Supplementary Material, further inquiries can be directed to the corresponding author.
MG: Formal Analysis, Methodology, Supervision, Writing–original draft. SA: Writing–original draft. TJ: Formal Analysis, Methodology, Writing–original draft. GH: Formal Analysis, Methodology, Resources, Writing–original draft. SM: Writing–original draft. FM: Writing–original draft, Writing–review and editing. OB: Writing–original draft. PT: Writing–original draft. MS: Writing–original draft. HT: Conceptualization, Writing–original draft. OA: Conceptualization, Project administration, Resources, Supervision, Writing–review and editing.
The author(s) declare that no financial support was received for the research, authorship, and/or publication of this article.
The authors thank the National Institutes of Health (NIH) Office of Data Science Strategy (ODSS) for their immense support before and during the April 2024 Omics codeathon organized by the African Society for Bioinformatics and Computational Biology (ASBCB).
The authors declare that the research was conducted in the absence of any commercial or financial relationships that could be construed as a potential conflict of interest.
The author(s) declare that no Generative AI was used in the creation of this manuscript.
All claims expressed in this article are solely those of the authors and do not necessarily represent those of their affiliated organizations, or those of the publisher, the editors and the reviewers. Any product that may be evaluated in this article, or claim that may be made by its manufacturer, is not guaranteed or endorsed by the publisher.
The Supplementary Material for this article can be found online at: https://www.frontiersin.org/articles/10.3389/fbinf.2025.1499255/full#supplementary-material
ADMET, Absorption, Distribution, Metabolism, Excretion, and Toxicity; AR, Aldose Reductase; ARI, Aldose Reductase Inhibitor; AUC, Area Under the Curve; CHARMM, Chemistry at HARvard Molecular Mechanics; DUD-E, Directory of Useful Decoys, Enhanced; PASS, Prediction of Activity Spectra for Substances; RCSB, Research Collaboratory for Structural Bioinformatics; RMSD, Root Mean Square Deviation; RMSF, Root Mean Square Fluctuation; ROC, Receiver Operating Curve; RoG, Radius of Gyration; SPC, Simple Point Charge; UFF, Universal Force Field; MM-PBSA, Molecular Mechanics Poisson-Boltzmann Surface Area.
Abdullah, A., Biswas, P., Sahabuddin, M., Mubasharah, A., Khan, D. A., Hossain, A., et al. (2023). Molecular dynamics simulation and pharmacoinformatic integrated analysis of bioactive phytochemicals from azadirachtaindica (Neem) to treat diabetes mellitus. J. Chem., 1–19. doi:10.1155/2023/4170703
Abolo, L., Ssenkaali, J., Mulumba, O., and Awe, O. I. (2024). Exploring the causal effect of omega-3 polyunsaturated fatty acid levels on the risk of type 1 diabetes: a mendelian randomization study. Front. Genet. 15, 1353081. doi:10.3389/fgene.2024.1353081
Abraham, M. J., Murtola, T., Schulz, R., Páll, S., Smith, J. C., Hess, B., et al. (2015). GROMACS: high-performance molecular simulations through multi-level parallelism from laptops to supercomputers. SoftwareX 1–2, 19–25. doi:10.1016/J.SOFTX.2015.06.001
Adelusi, T. I., Oyedele, A. Q. K., Boyenle, I. D., Ogunlana, A. T., Adeyemi, R. O., Ukachi, C. D., et al. (2022). Molecular modeling in drug discovery. Inf. Med. Unlocked 29, 100880. doi:10.1016/J.IMU.2022.100880
Agyapong, O., Asiedu, S. O., Kwofie, S. K., Miller, W. A., Parry, C. S., Sowah, R. A., et al. (2021). Molecular modelling and de novo fragment-based design of potential inhibitors of beta-tubulin gene of Necatoramericanus from natural products. Inf. Med. Unlocked 26, 100734. doi:10.1016/J.IMU.2021.100734
Alaya, F., Baraket, G., Adediran, D. A., Cuttler, K., Ajiboye, I., Kivumbi, M. T., et al. (2024). Multiple sclerosis stages and their differentially expressed genes: a bioinformatics analysis. bioRxiv. doi:10.1101/2024.01.20.576448
Antony, P., and Vijayan, R. (2015). Identification of novel aldose reductase inhibitors from spices: a molecular docking and simulation study. PloS One 10 (9), e0138186. doi:10.1371/JOURNAL.PONE.0138186
Aribi, H. B., Abassi, N., and Awe, O. I. (2024). NeuroVar: an open-source tool for the visualization of gene expression and variation data for biomarkers of neurological diseases. Gigabyte, gigabyte143. doi:10.46471/gigabyte.143
Arnittali, M., Rissanou, A. N., Amprazi, M., Kokkinidis, M., and Harmandaris, V. (2021). Structure and thermal stability of wtRop and RM6 proteins through all-atom molecular dynamics simulations and experiments. Int. J. Mol. Sci. 22 (11), 5931. doi:10.3390/ijms22115931
Arthur, M. N., Hanson, G., Broni, E., Sakyi, P. O., Mensah-brown, H., Iii, W. A. M., et al. (2024). Natural product identification and molecular docking studies of leishmania major pteridine reductase inhibitors. Pharmaceuticals 18, 6–24. doi:10.3390/ph18010006
Ashik, M. A., Islam, T., Fujii, M., Alam, M. M., and Hossain, M. N. (2022). Interaction pattern of aldose reductase with β-glucogallin: active site exploration and multiple docking analyses. Inf. Med. Unlocked 30, 100938. doi:10.1016/J.IMU.2022.100938
Ashley, C. N., Broni, E., Wood, C. M., Okuneye, T., Ojukwu, M. P. T., Dong, Q., et al. (2024). Identifying potential monkeypox virus inhibitors: an in silico study targeting the A42R protein. Front. Cell. Infect. Microbiol. 14 , 1351737–1351819. doi:10.3389/fcimb.2024.1351737
Ather, S. H., Awe, O. I., Butler, T. J., Denka, T., Semick, S. A., Tang, W., et al. (2018). SeqAcademy: an educational pipeline for RNA-Seq and ChIP-Seq analysis. F1000Research 7, 628. doi:10.12688/f1000research.14880.4
Awe, O. I., En najih, N., Nyamari, M. N., and Mukanga, L. B. (2023). Comparative study between molecular and genetic evolutionary analysis tools using African SARS-CoV2 variants. Inf. Med. Unlocked 36, 101143. doi:10.1016/j.imu.2022.101143
Berman, H. M., Westbrook, J., Feng, Z., Gilliland, G., Bhat, T. N., Weissig, H., et al. (2000). The protein Data Bank. Nucleic Acids Res. 28 (1), 235–242. doi:10.1093/NAR/28.1.235
Bowers, A. J., and Zhou, X. (2019). Receiver operating characteristic (ROC) area under the curve (AUC): a diagnostic measure for evaluating the accuracy of predictors of education outcomes. J. Educ. Students Placed A. T. Risk 24 (1), 20–46. doi:10.1080/10824669.2018.1523734
Brasileira De Farmacognosia, R., Funke, I., and elzig, M. F. (2006). Traditionally used plants in diabetes therapy: phytotherapeutics as inhibitors of alpha-amylase activity. Rev. Farmacogn. 16 (1), 1–5. doi:10.1590/S0102-695X2006000100002
Calixto, J. B. (2019). The role of natural products in modern drug discovery. An. Acad. Bras. Ciencias 91 (3), e20190105. doi:10.1590/0001-3765201920190105
Chang, K. C., Shieh, B., and Petrash, J. M. (2019). Role of aldose reductase in diabetes-induced retinal microglia activation. Chemico-Biological Interact. 302, 46–52. doi:10.1016/J.CBI.2019.01.020
Chen, C. P., Chen, C. C., Huang, C. W., and Chang, Y. C. (2018). Evaluating molecular properties involved in transport of small molecules in stratum corneum: a quantitative structure-activity relationship for skin permeability. Molecules 23 (4), 911. doi:10.3390/MOLECULES23040911
Cheng, F., Li, W., Zhou, Y., Shen, J., Wu, Z., Liu, G., et al. (2012a). admetSAR: a comprehensive source and free tool for assessment of chemical ADMET properties. J. Chem. Inf. Model. 52 (11), 3099–3105. doi:10.1021/CI300367A
Cheng, F., Li, W., Zhou, Y., Shen, J., Wu, Z., Liu, G., et al. (2012b). admetSAR: a comprehensive source and free tool for assessment of chemical ADMET properties. J. Chem. Inf. Model. 52 (11), 3099–3105. doi:10.1021/CI300367A
Chikwambi, Z., Hidjo, M., Chikondowa, P., Afolabi, L., Aketch, V., Jayeoba, G., et al. (2023). Multi-omics data integration approach identifies potential biomarkers for Prostate cancer. bioRxiv. doi:10.1101/2023.01.26.522643
Coimbra, J. T. S., Feghali, R., Ribeiro, R. P., Ramos, M. J., and Fernandes, P. A. (2020). The importance of intramolecular hydrogen bonds on the translocation of the small drug piracetam through a lipid bilayer. RSC Adv. 11 (2), 899–908. doi:10.1039/D0RA09995C
Daina, A., Michielin, O., and Zoete, V. (2017). SwissADME: a free web tool to evaluate pharmacokinetics, drug-likeness and medicinal chemistry friendliness of small molecules. Sci. Rep. 2017 7 (1), 42717–42813. doi:10.1038/srep42717
Dallakyan, S., and Olson, A. J. (2015). Small-molecule library screening by docking with PyRx. Methods Mol. Biol. 1263, 243–250. doi:10.1007/978-1-4939-2269-7_19
Dănilă, A.-I., Ghenciu, L. A., Stoicescu, E. R., Bolintineanu, S. L., Iacob, R., Săndesc, M.-A., et al. (2024). Aldose reductase as a key target in the prevention and treatment of diabetic retinopathy: a comprehensive review. Biomedicines 12 (4), 747. doi:10.3390/BIOMEDICINES12040747
DeLano, W. L. (2002). Pymol: an open-source molecular graphics tool. CCP4 Newsl. Protein Crystallogr. 40, 1–8. Available at: http://www.pymol.org/pymol.
Devadasu, V. R., Deb, P. K., Maheshwari, R., Sharma, P., and Tekade, R. K. (2018) “Physicochemical, pharmaceutical, and biological considerations in GIT absorption of drugs,” in Dosage form design considerations, I. Elsevier Inc, 149–178. doi:10.1016/B978-0-12-814423-7.00005-8
Die, J. V., Elmassry, M. M., LeBlanc, K. H., Awe, O. I., Dillman, A., and Busby, B. (2019). geneHummus: an R package to define gene families and their expression in legumes and beyond. BMC Genomics 20, 591. doi:10.1186/s12864-019-5952-2
El Abed, F., Baraket, G., Nyamari, M. N., Naitore, C., and Awe, O. I. (2023). Differential expression analysis of miRNAs and mRNAs in epilepsy uncovers potential biomarkers. bioRxiv. doi:10.1101/2023.09.11.557132
Enejoh, O. A., Okonkwo, C. H., Nortey, H., Kemiki, O. A., Ainembabazi, M., Mbaoji, F. N., et al. (2025). Machine learning and molecular dynamics simulations predict potential TGR5 agonists for type 2 diabetes treatment. Front. Chem. 12, 1503593. doi:10.3389/fchem.2024.1503593
Filimonov, D. A., Lagunin, A. A., Gloriozova, T. A., Rudik, A. V., Druzhilovskii, D. S., Pogodin, P. V., et al. (2014). Prediction of the biological activity spectra of organic compounds using the pass online web resource. Chem. Heterocycl. Compd. 50 (3), 444–457. doi:10.1007/s10593-014-1496-1
Flores-Holguín, N., Frau, J., and Glossman-Mitnik, D. (2021). In silico pharmacokinetics, ADMET study and conceptual DFT analysis of two plant cyclopeptides isolated from rosaceae as a computational peptidology approach. Front. Chem. 9, 708364. doi:10.3389/fchem.2021.708364
Forbes, J. M., and Cooper, M. E. (2013). Mechanisms of diabetic complications. Physiol. Rev. 93 (1), 137–188. doi:10.1152/PHYSREV.00045.2011
Gamal, H., and Munusamy, S. (2017). Aldose reductase as a drug target for treatment of diabetic nephropathy: promises and challenges. Protein Peptide Lett. 24 (1), 71–77. doi:10.2174/0929866523666161128153548
Goksuluk, D., Korkmaz, S., Zararsiz, G., and Karaagaoglu, A. E. (2016). EasyROC: an interactive web-tool for roc curve analysis using r language environment. R J. 8 (2), 213–230. doi:10.32614/RJ-2016-042
Gopal, K., Karwi, Q. G., Tabatabaei Dakhili, S. A., Wagg, C. S., Zhang, L., Sun, Q., et al. (2023). Aldose reductase inhibition alleviates diabetic cardiomyopathy and is associated with a decrease in myocardial fatty acid oxidation. Cardiovasc. Diabetol. 22 (1), 73–12. doi:10.1186/s12933-023-01811-w
Govindappa, M., Channabasava, R., Kumar, K. R. S., and Pushpalatha, K. C. (2013). Antioxidant activity and phytochemical screening of crude endophytes extracts of tabebuiaargentea bur. and K. Sch. Am. J. Plant Sci. 04 (08), 1641–1652. doi:10.4236/AJPS.2013.48198
Granchi, C., Capecchi, A., Del Frate, G., Martinelli, A., Macchia, M., Minutolo, F., et al. (2015). Development and validation of a docking-based virtual screening platform for the identification of new lactate dehydrogenase inhibitors. Molecules 20 (5), 8772–8790. doi:10.3390/molecules20058772
Guex, N., Peitsch, M. C., and Schwede, T. (2009). Automated comparative protein structure modeling with SWISS-MODEL and Swiss-PdbViewer: a historical perspective. ELECTROPHORESIS 30 (S1), S162–S173. doi:10.1002/ELPS.200900140
Gupta, J. K. (2023). The role of aldose reductase in polyol pathway: an emerging pharmacological target in diabetic complications and associated morbidities. Curr. Pharm. Biotechnol. 25, 1073–1081. doi:10.2174/1389201025666230830125147
Guterres, H., and Im, W. (2020). Improving protein-ligand docking results with high-throughput molecular dynamics simulations. J. Chem. Inf. Model. 60 (4), 2189–2198. doi:10.1021/ACS.JCIM.0C00057
Hanson, G., Adams, J., Kepgang, D. I. B., Zondagh, L. S., Bueh, L. T., Asante, A., et al. (2024). Machine learning and molecular docking prediction of potential inhibitors against dengue virus. Front. Chem. 12, 1510029. doi:10.3389/fchem.2024.1510029
Hanson, G., Asmah, N. E., Nyarko, G. A., Broni, E., Adzagbo, M. A., Wilson, M. D., et al. (2025). Structure-based design of potential anti-dengue compounds against the envelope glycoprotein of dengue 2 virus. J. Comput. Biophys. Chem. (January), 1–22. doi:10.1142/S2737416524500704
Hevener, K. E., Zhao, W., Ball, D. M., Babaoglu, K., Qi, J., White, S. W., et al. (2009). Validation of molecular docking programs for virtual screening against dihydropteroate synthase. J. Chem. Inf. Model. 49 (2), 444–460. doi:10.1021/CI800293N
Hotta, N. (2010). Aldose reductase inhibitor. Nippon Rinsho. Jpn. J. Clin. Med. 68 (Suppl. 9), 147–154. doi:10.1016/B978-0-444-53717-1.00240-7
Howard, E. I., Sanishvili, R., Cachau, R. E., Mitschler, A., Chevrier, B., Barth, P., et al. (2004). Ultrahigh resolution drug design I: details of interactions in human aldose reductase–inhibitor complex at 0.66 Å. Proteins Struct. Funct. Bioinforma. 55 (4), 792–804. doi:10.1002/PROT.20015
IDF Diabetes Atlas (2021). Available at: https://diabetesatlas.org/atlas/tenth-edition/?dlmodal=active&dlsrc=https%3A%2F%2Fdiabetesatlas.org%2Fidfawp%2Fresource-files%2F2021%2F07%2FIDF_Atlas_10th_Edition_2021.pdf.
Jiang, Z., You, L., Dou, W., Sun, T., and Xu, P. (2019). Effects of an electric field on the conformational transition of the protein: a molecular dynamics simulation study. Polymers 11 (2), 282. doi:10.3390/POLYM11020282
Jimenez-Gonzalez, F., Vélez-Gómez, J., Melchor-Moncada, J., Veloza, L., and Sepúlveda-Arias, J. (2018). Antioxidant, anti-inflammatory, and antiproliferative activity of extracts obtained from Tabebuia Rosea (Bertol.) DC. Pharmacogn. Mag. 14 (55), S25–S31. doi:10.4103/PM.PM_624_17
Julius, A., and Hopper, W. (2019). A non-invasive, multi-target approach to treat diabetic retinopathy. Biomed. and Pharmacother. 109, 708–715. doi:10.1016/J.BIOPHA.2018.10.185
Khan, S., Ullah, H., Rahim, F., Hussain, R., Khan, Y., Khan, M. S., et al. (2023). Synthesis, biological evaluation and molecular docking study of pyrimidine based thiazolidinone derivatives as potential anti-urease and anti-cancer agents. J. Saudi Chem. Soc. 27 (4), 101688. doi:10.1016/J.JSCS.2023.101688
Kingsley, R. B., Mishra, M., Brindha, P., and Subramoniam, A. (2013). Anti-diabetic activity of active fractions of Stereospermumtetragonum DC and isolation of active principles. J. Young Pharm. JYP 5 (1), 7–12. doi:10.1016/J.JYP.2012.09.002
Knapp, B., Frantal, S., Cibena, M., Schreiner, W., and Bauer, P. (2011). Is an intuitive convergence definition of molecular dynamics simulations solely based on the root mean square deviation possible? J. Comput. Biol. 18 (8), 997–1005. doi:10.1089/CMB.2010.0237
Knox, C., Wilson, M., Klinger, C. M., Franklin, M., Oler, E., Wilson, A., et al. (2024). DrugBank 6.0: the DrugBank knowledgebase for 2024. Nucleic acids Res. 52 (D1), D1265–D1275. doi:10.1093/nar/gkad976
Kuzmanic, A., and Zagrovic, B. (2010). Determination of ensemble-average pairwise root mean-square deviation from experimental B-factors. Biophysical J. 98 (5), 861–871. doi:10.1016/J.BPJ.2009.11.011
Kwofie, S. K., Broni, E., Teye, J., Quansah, E., Issah, I., Wilson, M. D., et al. (2019). Pharmacoinformatics-based identification of potential bioactive compounds against Ebola virus protein VP24. Comput. Biol. Med. 113, 103414. doi:10.1016/J.COMPBIOMED.2019.103414
Kwofie, S. K., Hanson, G., Sasu, H., Enninful, K. S., Mensah, F. A., Nortey, R. T., et al. (2022). Molecular modelling and atomistic insights into the binding mechanism of MmpL3 mtb. Chem. Biodivers. 19 (9), e202200160. doi:10.1002/cbdv.202200160
LaBarbera, D. V., Modzelewska, K., Glazar, A. I., Gray, P. D., Kaur, M., Liu, T., et al. (2009). The marine alkaloid naamidine A promotes caspase-dependent apoptosis in tumor cells. Anti-Cancer Drugs 20 (6), 425–436. doi:10.1097/CAD.0B013E32832AE55F
Leutcha, P. B., Jouda, J. B., Tsague Tankeu, V. F., Magnibou, L. M., Choudhry, M. I., Lannang, A. M., et al. (2023). Secondary metabolites from the stem bark of Stereospermum acuminatissimum and their antimicrobial activity. Biochem. Syst. Ecol. 109, 104648. doi:10.1016/J.BSE.2023.104648
Liang, G. Y., Gray, A. I., Thomas, D. W., and Waterman, P. G. (1988). Polyoxygenated cyclohexane epoxide derivatives from the stem bark of Monanthotaxisbuchananii. Phytochemistry 27 (12), 3857–3860. doi:10.1016/0031-9422(88)83032-2
Lin, J. H., and Yamazaki, M. (2003). Role of P-glycoprotein in pharmacokinetics: clinical implications. Clin. Pharmacokinet. 42 (1), 59–98. doi:10.2165/00003088-200342010-00003
Lipinski, C. A. (2004). Lead- and drug-like compounds: the rule-of-five revolution. Drug Discov. Today. Technol. 1 (4), 337–341. doi:10.1016/J.DDTEC.2004.11.007
Lou, L. L., and Martin, J. C. (2021). Selected thoughts on hydrophobicity in drug design. Molecules 26 (4), 875. doi:10.3390/MOLECULES26040875
Low, P. A. (2005). Oxidative stress and excitatory neurotoxins in neuropathy. Peripher. Neuropathy 2-Volume Set Expert Consult Basic, 509–533. doi:10.1016/B978-0-7216-9491-7.50026-0
Mahgoub, R. E., Atatreh, N., and Ghattas, M. A. (2022). Using filters in virtual screening: a comprehensive guide to minimize errors and maximize efficiency. Annu. Rep. Med. Chem. 59, 99–136. doi:10.1016/BS.ARMC.2022.09.002
Martin, Y. C. (2005). A bioavailability score. J. Med. Chem. 48 (9), 3164–3170. doi:10.1021/JM0492002
Maruyama, Y., Igarashi, R., Ushiku, Y., and itsutake, A. (2023). Analysis of protein folding simulation with moving root mean square deviation. J. Chem. Inf. Model. 63 (5), 1529–1541. doi:10.1021/acs.jcim.2c01444
Mayeka, J. G., Nyandoro, S. S., and Munissi, J. J. E. (2024). Genus Monanthotaxis: a review on distribution, ethnomedicinal uses and phytochemistry. Nat. Prod. Res. 38, 4494–4510. doi:10.1080/14786419.2024.2301743
Mishra, B. B., and Tiwari, V. K. (2011). Natural products: an evolving role in future drug discovery. Eur. J. Med. Chem. 46 (10), 4769–4807. doi:10.1016/J.EJMECH.2011.07.057
Murugan, K. K., Poojari, C. C., Ryavalad, C., Lakshmikan, R. Y., Satwadi, P. R., Vittal, R. R., et al. (2017). Anti-diabetic activity of endophytic fungi, penicillium species of Tabebuia argentea; in silico and experimental analysis. Res. J. Phytochemistry 11 (2), 90–110. doi:10.3923/RJPHYTO.2017.90.110
Mwanga, M. J., Obura, H. O., Evans, M., and Awe, O. I. (2023). Enhanced deep convolutional neural network for SARS-CoV-2 variants classification. bioRxiv. doi:10.1101/2023.08.09.552643
Mylari, B. L., Armento, S. J., Beebe, D. A., Conn, E. L., Coutcher, J. B., Dina, M. S., et al. (2003). A highly selective, non-hydantoin, non-carboxylic acid inhibitor of aldose reductase with potent oral activity in diabetic rat models: 6-(5-chloro-3-methylbenzofuran-2-sulfonyl)-2-H-pyridazin-3-one. J. Med. Chem. 46 (12), 2283–2286. doi:10.1021/jm034065z
Mysinger, M. M., Carchia, M., Irwin, J. J., and Shoichet, B. K. (2012). Directory of useful decoys, enhanced (DUD-E): better ligands and decoys for better benchmarking. J. Med. Chem. 55 (14), 6582–6594. doi:10.1021/jm300687e
Nkunya, M. H. H., Waibel, R., and Achenbach, H. (1993). Three flavonoids from the stem bark of the antimalarial Uvariadependens. Phytochemistry 34 (3), 853–856. doi:10.1016/0031-9422(93)85372-X
Ntie-Kang, F., Telukunta, K. K., Döring, K., Simoben, C. V., Moumbock, A. F. A., Malange, Y. I., et al. (2017). NANPDB: a resource for natural products from northern African sources. J. Nat. Prod. 80 (7), 2067–2076. doi:10.1021/ACS.JNATPROD.7B00283
Ntie-Kang, F., Zofou, D., Babiaka, S. B., Meudom, R., Scharfe, M., Lifongo, L. L., et al. (2013). AfroDb: a select highly potent and diverse natural product library from African medicinal plants. PloS One 8 (10), e78085. doi:10.1371/JOURNAL.PONE.0078085
Nyamari, M. N., Omar, K. M., Fayehun, A. F., Dachi, O., Bwana, B. K., and Awe, O. I. (2023). Expression level analysis of ACE2 receptor gene in african-American and non-African-American COVID-19 patients. bioRxiv. doi:10.1101/2023.09.11.557129
Nzungize, L., Kengne-Ouafo, J. A., Wesonga, M. R., Umuhoza, D., Murithi, K., Kimani, P., et al. (2022). Transcriptional profiles analysis of COVID-19 and malaria patients reveals potential biomarkers in children. bioRxiv, doi:10.1101/2022.06.30.498338
O’Boyle, N. M., Banck, M., James, C. A., Morley, C., Vandermeersch, T., and Hutchison, G. R. (2011). Open Babel: an open chemical toolbox. J. Cheminformatics 3 (10), 33–14. doi:10.1186/1758-2946-3-33
Obura, H. O., Mlay, C. D., Moyo, L., Karumbo, B. M., Omar, K. M., Sinza, E. M., et al. (2022). Molecular phylogenetics of HIV-1 subtypes in african populations: a case study of sub-saharan African countries. bioRxiv. doi:10.1101/2022.05.18.492401
Ogbodo, U. C., Enejoh, O. A., Okonkwo, C. H., Gnanasekar, P., Gachanja, P. W., Osata, S., et al. (2023). Computational identification of potential inhibitors targeting cdk1 in colorectal cancer. Front. Chem. 11, 1264808. doi:10.3389/fchem.2023.1264808
Omar, K. M., Kitundu, G. L., Jimoh, A. O., Namikelwa, D. N., Lisso, F. M., Babajide, A. A., et al. (2024). Investigating antimicrobial resistance genes in Kenya, Uganda and Tanzania cattle using metagenomics. PeerJ 12, e17181. doi:10.7717/peerj.17181
Parasuraman, S. (2011). Prediction of activity spectra for substances. J. Pharmacol. Pharmacother. 2 (1), 52–53. doi:10.4103/0976-500X.77119
Pawar, S. V., Banini, W. S. K., Shamsuddeen, M. M., Jumah, T. A., Dolling, N. N. O., Tiamiyu, A., et al. (2024). Prostruc: an open-source tool for 3D structure prediction using homology modeling. Front. Chem. 12, 1509407. doi:10.3389/fchem.2024.1509407
Pires, D. E. V., Blundell, T. L., and Ascher, D. B. (2015). pkCSM: predicting small-molecule pharmacokinetic and toxicity properties using graph-based signatures. J. Med. Chem. 58 (9), 4066–4072. doi:10.1021/acs.jmedchem.5b00104
Podjarny, A., Cachau, R. E., Schneider, T., Van Zandt, M., and Joachimiak, A. (2004). Subatomic and atomic crystallographic studies of aldose reductase: implications for inhibitor binding. Cell. Mol. Life Sci. CMLS 61 (7–8), 763–773. doi:10.1007/S00018-003-3404-1
Ramirez, M. A., and Borja, N. L. (2008). Epalrestat: an aldose reductase inhibitor for the treatment of diabetic neuropathy. Pharmacotherapy 28 (5), 646–655. doi:10.1592/phco.28.5.646
Sander, T., Freyss, J., Von Korff, M., and Rufener, C. (2015). DataWarrior: an open-source program for chemistry aware data visualization and analysis. J. Chem. Inf. Model. 55 (2), 460–473. doi:10.1021/ci500588j
Sapra, A., and Bhandari, P. (2021). Diabetes mellitus. StatPearls. Available at: http://www.ncbi.nlm.nih.gov/pubmed/31855345.
Shehzad, M. T., Imran, A., Hameed, A., Rashida, M. al, Bibi, M., Uroos, M., et al. (2021). Exploring synthetic and therapeutic prospects of new thiazoline derivatives as aldose reductase (ALR2) inhibitors. RSC Adv. 11 (28), 17259–17282. doi:10.1039/D1RA01716K
Shivanika, C., Deepak Kumar, S., Ragunathan, V., Tiwari, P., Sumitha, A., and Brindha Devi, P. (2022). Molecular docking, validation, dynamics simulations, and pharmacokinetic prediction of natural compounds against the SARS-CoV-2 main-protease. J. Biomol. Struct. and Dyn. 40 (2), 585–611. doi:10.1080/07391102.2020.1815584
Singh, M., Kapoor, A., and Bhatnagar, A. (2021a). Physiological and pathological roles of aldose reductase. Metabolites 11 (10), 655. doi:10.3390/METABO11100655
Singh, M., Kapoor, A., and Bhatnagar, A. (2021b). Physiological and pathological roles of aldose reductase. Metabolites 11 (10), 655. doi:10.3390/METABO11100655
Sob, S. V. T., Wabo, H. K., Tang, C. P., Tane, P., Ngadjui, B. T., and Ye, Y. (2011). Phenol esters and other constituents from the stem barks of Stereospermum acuminatissimum. J. Asian Nat. Prod. Res. 13 (12), 1128–1134. doi:10.1080/10286020.2011.619182
Srikanth, K. K., and Orrick, J. A. (2022). Biochemistry, polyol or sorbitol pathways. StatPearls. Available at: https://www.ncbi.nlm.nih.gov/books/NBK576381/.
Stelzl, U., Worm, U., Lalowski, M., Haenig, C., Brembeck, F. H., Goehler, H., et al. (2005). A human protein-protein interaction network: a resource for annotating the proteome. Cell 122 (6), 957–968. doi:10.1016/J.CELL.2005.08.029
Tang, W. H., Martin, K. A., and Hwa, J. (2012). Aldose reductase, oxidative stress, and diabetic mellitus. Front. Pharmacol. 3, 87. doi:10.3389/FPHAR.2012.00087
Tarle, I., Borhani, D. W., Wilson, D. K., Quiocho, F. A., and Petrash, J. M. (1993). Probing the active site of human aldose reductase: site-directed mutagenesis of ASP-43, TYR-48, LYS-77, and HIS-110. J. Biol. Chem. 268 (34), 25687–25693. doi:10.1016/S0021-9258(19)74444-5
Tian, S., Wang, J., Li, Y., Li, D., Xu, L., and Hou, T. (2015). The application of in silico drug-likeness predictions in pharmaceutical research. Adv. Drug Deliv. Rev. 86, 2–10. doi:10.1016/J.ADDR.2015.01.009
Tomic, D., Shaw, J. E., and Magliano, D. J. (2022). The burden and risks of emerging complications of diabetes mellitus. Nat. Rev. Endocrinol. 18 (9), 525–539. doi:10.1038/S41574-022-00690-7
Trott, O., and Olson, A. J. (2010). AutoDock Vina: improving the speed and accuracy of docking with a new scoring function, efficient optimization and multithreading. J. Comput. Chem. 31 (2), 455–461. doi:10.1002/JCC.21334
Turner, P. J. (2005). XMGRACE (version 5.1. 19). Cent. Coast. Land-Margin Res. Or. Graduate Inst. Sci. Technol.
Vaden, R. M., Guillen, K. P., Salvant, J. M., Santiago, C. B., Gibbons, J. B., Pathi, S. S., et al. (2019). A cancer-selective Zinc ionophore inspired by the natural product naamidine A. ACS Chem. Biol. 14 (1), 106–117. doi:10.1021/ACSCHEMBIO.8B00977
Vanommeslaeghe, K., and MacKerell, A. D. (2012). Automation of the CHARMM general force field (CGenFF) I: bond perception and atom typing. J. Chem. Inf. Model. 52 (12), 3144–3154. doi:10.1021/ci300363c
Van Zandt, M. C., Jones, M. L., Gunn, D. E., Geraci, L. S., Jones, J. H., Sawicki, D. R., et al. (2005). Discovery of 3-[(4,5,7-trifluorobenzothiazol-2-yl)methyl]indole-N-acetic acid (lidorestat) and congeners as highly potent and selective inhibitors of aldose reductase for treatment of chronic diabetic complications. J. Med. Chem. 48 (9), 3141–3152. doi:10.1021/JM0492094
Varma, A. K., Patil, R., Das, S., Stanley, A., Yadav, L., and Sudhakar, A. (2010). Optimized hydrophobic interactions and hydrogen bonding at the target-ligand interface leads the pathways of drug-designing. PloS One 5 (8), e12029. doi:10.1371/JOURNAL.PONE.0012029
Vos, T., Flaxman, A. D., Naghavi, M., Lozano, R., Michaud, C., Ezzati, M., et al. (2012). Years lived with disability (YLDs) for 1160 sequelae of 289 diseases and injuries 1990-2010: a systematic analysis for the Global Burden of Disease Study 2010. Lancet London, Engl. 380 (9859), 2163–2196. doi:10.1016/S0140-6736(12)61729-2
Wang, C., Greene, D., Xiao, L., Qi, R., and Luo, R. (2017). Recent developments and applications of the MMPBSA method. Front. Mol. Biosci. 4 (JAN), 87. doi:10.3389/FMOLB.2017.00087
Wesonga, R. M., and Awe, O. I. (2022). An assessment of traditional and genomic screening in newborns and their applicability for Africa. Inf. Med. Unlocked 32, 101050. doi:10.1016/j.imu.2022.101050
Wiernsperger, N. F. (2003). Oxidative stress as a therapeutic target in diabetes: revisiting the controversy. Diabetes and Metabolism 29 (6), 579–585. doi:10.1016/S1262-3636(07)70072-1
Witters, L. A. (2001). The blooming of the French lilac. J. Clin. Investigation 108 (8), 1105–1107. doi:10.1172/JCI14178
Yang, H., Lou, C., Sun, L., Li, J., Cai, Y., Wang, Z., et al. (2019). admetSAR 2.0: web-service for prediction and optimization of chemical ADMET properties. Bioinformatics 35 (6), 1067–1069. doi:10.1093/BIOINFORMATICS/BTY707
Zhu, C., and Zhu, C. (2013). Aldose reductase inhibitors as potential therapeutic drugs of diabetic complications. Diabetes Mellitus - Insights Perspect. doi:10.5772/54642
Keywords: diabetes mellitus, aldose reductase, molecular docking, pharmacokinetics, molecular dynamics simulations
Citation: Gakpey MEL, Aidoo SA, Jumah TA, Hanson G, Msipa S, Mbaoji FN, Bukola O, Tjale PC, Sangare M, Tebourbi H and Awe OI (2025) Targeting aldose reductase using natural African compounds as promising agents for managing diabetic complications. Front. Bioinform. 5:1499255. doi: 10.3389/fbinf.2025.1499255
Received: 23 October 2024; Accepted: 17 January 2025;
Published: 06 February 2025.
Edited by:
Cristiano Valim Bizarro, Pontifical Catholic University of Rio Grande do Sul, BrazilReviewed by:
Debanjan Kundu, Georgia Institute of Technology, United StatesCopyright © 2025 Gakpey, Aidoo, Jumah, Hanson, Msipa, Mbaoji, Bukola, Tjale, Sangare, Tebourbi and Awe. This is an open-access article distributed under the terms of the Creative Commons Attribution License (CC BY). The use, distribution or reproduction in other forums is permitted, provided the original author(s) and the copyright owner(s) are credited and that the original publication in this journal is cited, in accordance with accepted academic practice. No use, distribution or reproduction is permitted which does not comply with these terms.
*Correspondence: Miriam E. L. Gakpey, bGF3c21pbWk0MEBnbWFpbC5jb20=
†ORCID: Miriam E. L Gakpey, orcid.org/0009-0005-6595-5910; Shadrack A. Aidoo, orcid.org/0009-0003-3895-909X; Toheeb A. Jumah, orcid.org/0000-0001-5227-1118; George Hanson, orcid.org/0009-0007-2720-9102; Siyabonga Msipa, orcid.org/0000-0002-6800-752X; Florence N. Mbaoji, orcid.org/0000-0002-9705-3250; Omonijo Bukola, orcid.org/0009-0006-6692-3387; Palesa C. Tjale, orcid.org/0000-0002-3227-8387; Mamadou Sangare, orcid.org/0000-0002-8300-8205; Hedia Tebourbi, orcid.org/0009-0009-9382-671X; Olaitan I. Awe, orcid.org/0000-0002-4257-3611
Disclaimer: All claims expressed in this article are solely those of the authors and do not necessarily represent those of their affiliated organizations, or those of the publisher, the editors and the reviewers. Any product that may be evaluated in this article or claim that may be made by its manufacturer is not guaranteed or endorsed by the publisher.
Research integrity at Frontiers
Learn more about the work of our research integrity team to safeguard the quality of each article we publish.