- 1Farm Animal Behavior and Husbandry Section, University of Kassel, Kassel, Germany
- 2Friedrich-Loeffler Institute of Animal Welfare and Animal Husbandry, Celle, Germany
- 3Thünen Institute of Organic Farming, Westerau, Germany
- 4Association for Technology and Structures in Agriculture, Darmstadt, Germany
One approach to strengthening the involvement of farmers or stockpersons in the evaluation and improvement of animal welfare is the implementation of an on-farm self-assessment. A valid comparison of the results with reference values, between or within farms, requires that training of the farmers and reliability testing have taken place. We investigated two different training methods (online vs. live) with a total of 146 livestock farmers from farms with dairy cows and calves, beef cattle, sows and suckling piglets, weaners and fattening pigs, laying hens, broiler chickens, and turkeys from all over Germany. Online tests were conducted by assessing photos/videos of each indicator of the assessment scheme to estimate the inter-rater reliability (prevalence-adjusted and bias-adjusted kappa, PABAK). The farmers were requested to provide information on their professional background and rate their motivation to participate in the training and their subjective training success, meaning their confidence in assessing each indicator later on-farm. They evaluated the feasibility of the training and its impact on their views and attitudes. In general, farmers achieved at least substantial inter-rater reliability (PABAK ≥ 0.61) in 86.8% of all initial tests; 13.4% of the tests were repeated once or more times, resulting in a significant improvement of the agreement, with 90.9% of the tests reaching a PABAK ≥ 0.61. However, reliability was higher for indicators with a lower number of score levels. The subjective evaluation of training success was, on average, positive (score = 74.8 out of 100). No effects of the training method or the farmers’ professional background on the inter-rater reliability or the subjective training success were detected. Furthermore, for both methods, farmers moderately agreed that the training had sharpened their views on the animals, encouraged them to implement the assessment on their farm, and made it clear that self-assessment supports animal management. Although the reported costs and time investment for the online training were significantly lower, the effort required for both methods and the ease of integration into the workflow were ranked as similarly acceptable. Overall, both training methods appear feasible for the training of farmers/stockpersons on the assessment of animal-based indicators.
Introduction
Various animal welfare assessments for different farm animals, such as the Welfare Quality® protocols (Welfare Quality® Consortium, 2009a; Welfare Quality® Consortium, 2009b; Welfare Quality® Consortium, 2009c) and AWIN protocols (Ferrante et al., 2015) or on-farm assurance schemes (e.g., Main et al., 2012), have been developed for the certification of animal welfare on-farm or for use in scientific studies. These approaches are usually applied by trained inspectors or scientists. However, when farmers apply a systematic assessment of animal welfare themselves, it may increase their awareness of the welfare state of their animals and contribute to an increased motivation to intervene in case of welfare problems. Indeed, the animal welfare status on-farm or its improvement largely depends on the farmers’ attitudes, motivation (Ivemeyer et al., 2015; Pol et al., 2021), and their empathy toward animals (Kielland et al., 2010). Several approaches emphasizing the importance of farmers’ empowerment in the improvement of animal welfare have been developed over the past years. Among them are stable schools (Vaarst and Fisker, 2013; March et al., 2014; Ivemeyer et al., 2015) and practice-led innovation networks (van Dijk et al., 2019) or approaches such as “hazard analysis and critical control point” (HACCP)-based risk management programs for health and welfare (Rousing et al., 2020). Although animal monitoring is routinely carried out by farmers during their daily inspections as an important and time-intensive activity (Dockès and Kling-Eveillard, 2006), it often lacks a more detailed inspection or systematic observation of animals and a regular evaluation of animal welfare. Consequently, it was found that farmers underestimate the prevalence of animal welfare problems, such as lameness in dairy cows (Whay et al., 2003; Beggs et al., 2019) or in sheep (Liu et al., 2018).
In Germany, an obligation to conduct on-farm self-assessments came into force in the German Animal Welfare Act in 2014 (TierSchG, 2006). Proposals for on-farm self-assessment protocols were subsequently elaborated between 2014 and 2016 in different expert panels together with different stakeholders, including livestock farmers. The proposed indicators were selected and adapted for farmers’ use to detect the most relevant animal welfare problems as they arise on their farms. All indicators were defined based on existing assessment protocols (e.g., Welfare Quality® Consortium, 2009a; Welfare Quality® Consortium, 2009b; Welfare Quality® Consortium, 2009c) while striving for simple assessment schemes as much as possible (Zapf et al., 2015). These practical guides were published in 2016 and are now available in a revised version for dairy cows, calves, and beef cattle (Brinkmann et al., 2020); for sows and suckling piglets; weaning and fattening pigs (Schrader et al., 2020); and for laying hens, broiler chickens, and turkeys (Knierim et al., 2020).
The outcomes of the on-farm self-assessments by the farmers need to be sufficiently reliable to allow valid evaluations using reference values or comparisons with previous recordings and the results of other farms (benchmarking). For scientific investigations, it is well documented that adequate inter-rater reliability is supported by training (Brenninkmeyer et al., 2007; March et al., 2007; Gibbons et al., 2012; Vasseur et al., 2013; Vieira et al., 2015; Croyle et al., 2018; Jung et al., 2021). Furthermore, inter-rater reliability should be tested and should reach certain minimum values (Knierim and Winckler, 2009). This does similarly apply to self-assessments by farmers who, moreover, may not necessarily have been trained within their vocational education to assess animal-based welfare indicators.
Training of animal welfare assessment is mostly conducted live or on-farm with animals (e.g., Heerkens et al., 2016; Oliveira et al., 2017). This provides a realistic setting, allowing the use of all senses and training on how to handle the animals. However, the disadvantages of the live or on-farm training are the time and travel expenses. In contrast, an online training is not tied to a specific location and may fit better into farmers’ work schedules, as it is permanently available. Moreover, it is easier to equally demonstrate and test all levels of an indicator’s scoring system using pictures and videos, whereas the range may be limited in live training. Several online training courses for the recording of animal welfare indicators are available (AssureWel, 2016; AWARE, 2017; Bio Austria, 2021; University of California, 2021). It has already been shown that indicators for on-farm assessments can successfully be trained using pictures for body condition scoring (BCS) in dairy cows (Ferguson et al., 2006) and in goats (Vieira et al., 2015). The same applies to lameness in dairy cows by using videos (Garcia et al., 2015). However, information on training for farmers is lacking.
The aim of this study was to compare the training success of online and live courses developed for livestock farmers to learn the assessment of animal-based indicators. Various potentially influencing factors such as the complexity of the indicators or the farmers’ professional backgrounds, including whether they worked with animals for breeding (including egg or milk production) or fattening purposes, were considered. We were also interested whether the two training methods differ with regard to their feasibility (costs, required time, adequacy of the effort, and the integration into the workflow) and their impact on the farmers’ views on the animals and attitudes toward animal welfare assessment (encouragement to apply the indicators on farm and the evaluation of the value of on-farm self-assessment for animal management). Our hypotheses were that live training results in a higher reliability of the assessments due to direct contact with a supervisor, and a greater impact on the farmer, but is less feasible in terms of cost and time efforts and integration into the workflow. Additionally, we expected the reliability to be influenced by the professional background of the farmers and the simplicity of the scoring system.
Materials and methods
Involved livestock farmers
In total, 146 livestock farmers from 136 farms participated voluntarily in the study and completed the training. The term farmer is used here in the sense of any participating stockperson working on a farm regardless of whether they are the farm owners or employees. They worked with altogether seven specific types of farm animals lumped into two categories of livestock: 78 farmers from breeding farms (36 for dairy cows with calves, 21 for sows and suckling piglets, and 21 for laying hens) and 68 farmers from fattening farms (23 for beef cattle, 23 for fattening pigs, 11 for broiler chickens, and 11 for fattening turkeys). This included seven farrow-to-finish pig farms, each with two participating farmers (one from the breeding and one from the fattening unit), and three dairy farms with two farmers each (one responsible for the cows and one for the calves). All farmers were recruited through advertisements in agricultural journals and recommendations from agricultural advisors or farming associations. A precondition for participation was that the farmer had no experience in the systematic assessment of animal welfare indicators. The farms were distributed all over Germany and differed regarding their number of animals and their management or housing systems. Most of the farms operated conventionally, 23 farms were organic and two poultry farms had organic and conventional herds. In further analyses, the farmers from all farms were included and no distinction was made between organic and conventional farms.
Indicator sets and training methods
The investigated training material covered all animal welfare indicators in the first edition of the practical guide for the on-farm self-assessment of cattle, pigs, and poultry (Brinkmann et al., 2016; Knierim et al., 2016; Schrader et al., 2016;). From these protocols, this study focused on the indicators that are directly recorded on the animal (Table 1). They are predominantly scored with two levels (yes/no alteration; n = 29) or three levels (no/slight/severe alteration; n = 18; for stereotypies in sows sham chewing/bar biting/tongue rolling). Exceptions were signs of ectoparasites in pigs, which had four levels, and underconditioning in sows, with five levels. All investigated indicators are described in detail in Supplementary Table S1.
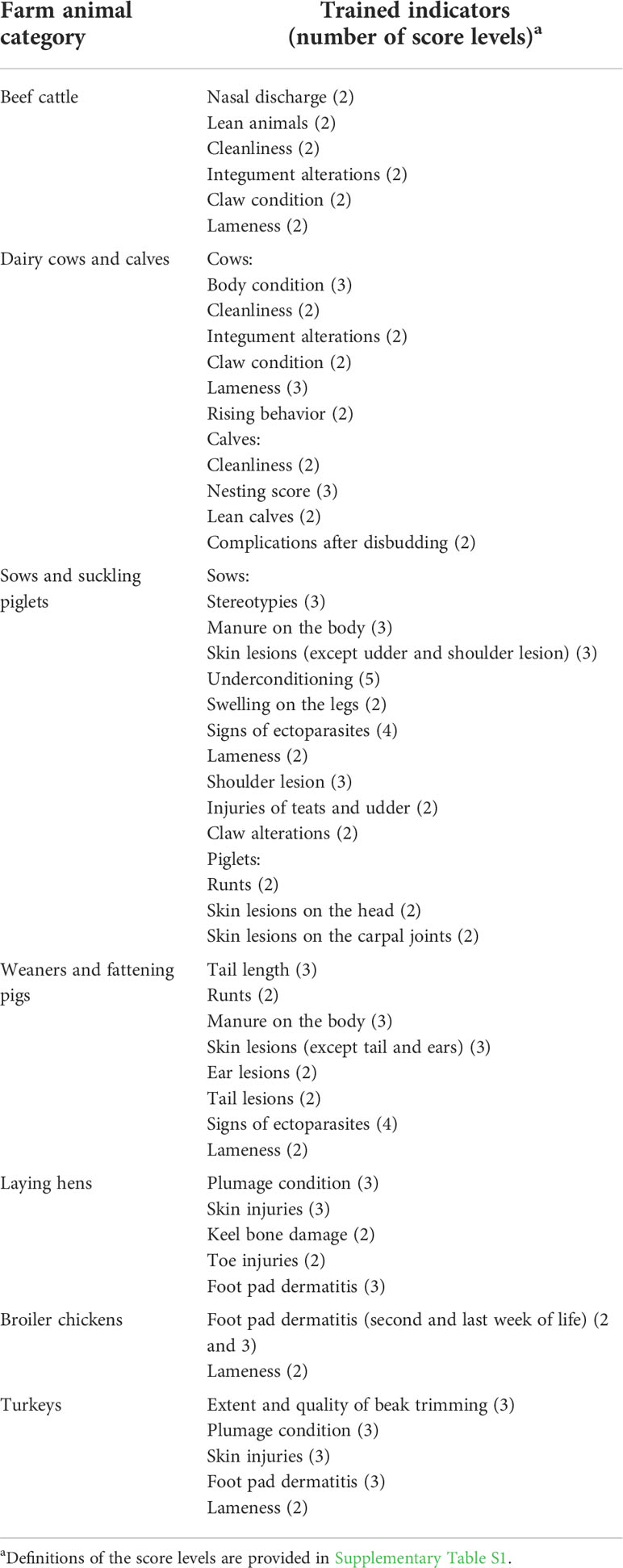
Table 1 Investigated animal-based indicators of the “Animal Welfare Indicators: Practical Guide—Cattle/Pigs/Poultry” (Brinkmann et al., 2016; Knierim et al., 2016; Schrader et al., 2016).
The online and live trainings were developed and conducted according to a joint training concept by one trainer for pig farmers, two trainers for poultry farmers, and three trainers for cattle farmers. The poultry live training included an on-farm practice with bird handling, while the cattle and pig live groups were trained to perform the assessment using pictures and videos. All trainers were scientists in the field of animal welfare with extensive practice in the assessment of indicators or had been trained by experienced animal welfare scientists reaching substantial to very good inter-rater reliability (following the interpretation of kappa values by Landis and Koch, 1977). The prevalence-adjusted and bias-adjusted kappa (PABAK) values (Byrt et al., 1993) ranged from 0.65 to 1.0 for the trainers of the poultry farmers and from 0.60 to 1.0 for the trainers of the cattle farmers. The pictures and videos used for the exercises and tests had been scored by the trainers. These scorings were used as a reference for the agreement tests (silver standard). For cattle and poultry, at least two trainers had scored the material, and pictures/videos were only selected when an agreement on the scoring was reached. Pretests of the training contents and functionality were carried out with external scientists, advisers, agricultural students, and farmers who were not participants of the study.
The farmers were assigned to a training method, considering the ease of access to the location of the live training and, for cattle, also the farmers’ preferences. All farmers received the practical guide, which contained comprehensive information on all indicators, including pictures and web addresses to videos. The 1-day live training was conducted with 70 farmers in groups of up to nine participants on a total of 16 training dates. Four poultry farmers received an individual live training because they were otherwise unable to attend. The training took place at experimental and educational facilities or participants’ farms. It comprised an oral presentation with PowerPoint® slides elucidating the definitions and scoring methods for each indicator. The farmers practiced the assessment of the indicators individually with previously selected pictures or videos (5–10 for each indicator) and received feedback from the trainer. Poultry farmers additionally received training in the handling of the birds and assessed about five birds in pairs on-farm with feedback from the trainers. The online training was provided for 76 farmers on a web-based learning platform [Moodle, version 3.5 (long-term support release, LTS)] with unlimited access, but a recommended time period for completion. The structure of the platform was kept simple and contained a short introduction about how to use it. The farmers could navigate individually and self-paced through the definitions and the scoring methods of each indicator, including exercises with integrated feedback on their scoring. These exercises could be repeated without limit. The media presented in the online and live training exercises came from the same pool of material. However, the farmers who trained online decided themselves how many pictures or videos they assessed for training purposes.
Inter-rater reliability tests
After completing both training methods, all farmers were invited to test their inter-rater reliability on the online learning platform. While farmers in the online training group had access since the beginning of their training, farmers who did the live training received access after completion of their training. The online test consisted of individual tests for each indicator with 20 pictures or videos per indicator, previously scored by the trainers. For a few indicators, suitable material was not available in sufficient quantity. In these cases, the tests comprised only 10 videos (lameness in sows and fattening pigs) or 15 pictures (ectoparasites, runts, stereotypies, and underconditioning in pigs). For integument alterations in cattle and cleanliness in dairy cows, 21 pictures (presenting three different body parts) were provided. The aim was to equally represent all score levels in the tests, but this was not completely achieved for lameness and footpad dermatitis in turkeys and for tail length, ectoparasites, stereotypies, shoulder lesions, and underconditioning in pigs.
The farmers’ results in the online test were compared with the assessment of the trainers by calculating the PABAK value as a measure of the inter-rater reliability. Based on the interpretation of kappa coefficients by Landis and Koch (1977), the farmers received feedback on the achieved agreement after each completion of an individual test: “well done, you can apply that indicator on your farm” for PABAK values of 0.61–1.0, “some more training is needed” for PABAK values of 0.41–0.60, or “you should review the theory and the assessment of that indicator” if the PABAK was 0.40 or less. All individual tests of a farm animal type had to be completed by the farmer. It was possible to repeat each test without limit, with the presented material changing randomly out of a selection of 20–222 pictures or videos per indicator.
Online survey
Following the training and tests, an online survey (LimeSurvey, version 3.22.9 + 200317) was conducted to explore possible influences on the training’s success and to have the farmers subjectively rate the training (Table 2). It included questions on the farmers’ current motivation to participate in the training (factors included interest, challenge, anxiety, and success, modified from Freund et al., 2011 and Lenski et al., 2016) since motivation can influence performance (Eklöf, 2010). The farmers were asked about the feasibility of the training (i.e., ease of integration into their workflow, incurred costs, required time, and the adequacy of the effort) and its perceived impact (whether their animal observation skills were sharpened, whether it encouraged them to assess the indicators on the farm, and whether it made it clear that self-assessment supports animal management). The incurred costs and required time were measured by extracting data on the amount of euro and the number of hours from the open-answer format. In addition, the farmers were asked, for each indicator, to rank how well prepared they felt to assess that indicator later on their own farm (subjective training success). Lastly, the farmers gave information about their professional background, i.e., their highest agricultural education, their position at the farm, and the number of years of experience with the respective species.
Data analysis
The dataset was analyzed using the statistical program R version 3.6.1. Firstly, three multilevel mixed models were applied with the PABAK values from the first and the last test of all participants and the subjective training success ratings as dependent variables. Due to non-normally distributed residuals (Shapiro–Wilk test) and the many outliers in the dataset [quantile–quantile (Q–Q) plots], a robust mixed model with a Huber k-estimation was selected (“robustlmm” package). Here, outliers exceeding a distance k = 1.345 are not weighted quadratically (Koller, 2016). The robust mixed model was applied.
where is the dependent variable. Fixed factors (denoted by x with the corresponding parameter β) included the training method, xtr; the number of score levels, xns; years of experience, xex; the highest agricultural educational degree of the farmer, xed; the position of the farmer, xpf; and the livestock category, xlc. The random factors (denoted by z, with the corresponding parameter u) included the indicator zin and the individual farmer zfa (being the experimental unit). Both were nested in the farm animal type (e.g., dairy cows or fattening pigs), leading to crossed random effects between them. The farm animal type was not required as an additional random factor since the individual farmers and the indicators were uniquely assigned to the farm animal type and therefore already contained this information. The random error of the model is ϵi.
To check for differences in the farmers’ professional backgrounds between the online and live groups, a two-tailed Mann–Whitney U test was calculated since the data were not normally distributed (Shapiro–Wilk test).
Possible differences between the results of the first and last inter-rater reliability tests in the case of repeated tests were analyzed using the Wilcoxon signed-rank test since the dataset was not normally distributed (Shapiro–Wilk test). The hypothesis was one-tailed, expecting the reliability to be higher in the last tests. For the Wilcoxon test and the Mann–Whitney U test, the W value denoted the standardized test statistic and r the effect size (Cohen, 1988). Furthermore, Spearman’s correlation analysis was used to test for associations between the number of repeated tests per indicator and improvements in the PABAK value.
Regarding the farmers’ evaluation of the feasibility and the impact of the training, the following robust mixed models were applied:
The dependent variable represents the rating concerning feasibility (ease of integration into the workflow and adequacy of effort), incurred costs and required time, and the three ratings concerning the impact of the training (animal observation skills, encouragement to apply the self-assessment, and the perception of the self-assessment as a management tool). The fixed factor was the training method, xtr, and the random factor was the farm animal type, zft.
P-values were calculated for all models using Satterthwaite approximations of degrees of freedom (Luke, 2017). Effect sizes were calculated according to Field et al. (2012). The significance level was set at 0.05.
Results
Farmers’ professional background and motivation
The overall response rate to the online questionnaire was 97.3% (n = 142 out of 146; online n = 73, live n = 69), but single questions were not answered by all respondents. The farmers’ experience with the farm animal type ranged from 1 to 48 years (mean = 18.2 years, n = 140). About two-thirds of the farmers (93 of 142) held a master’s craftsman certificate or academic degrees (engineering school, university of applied sciences, or university). In addition, most of the farmers (n = 77) were the head of the farm. Table 3 shows more information on the professional background of the farmers. The professional background did not differ significantly between the participants of the online or the live training (Mann–Whitney U test).
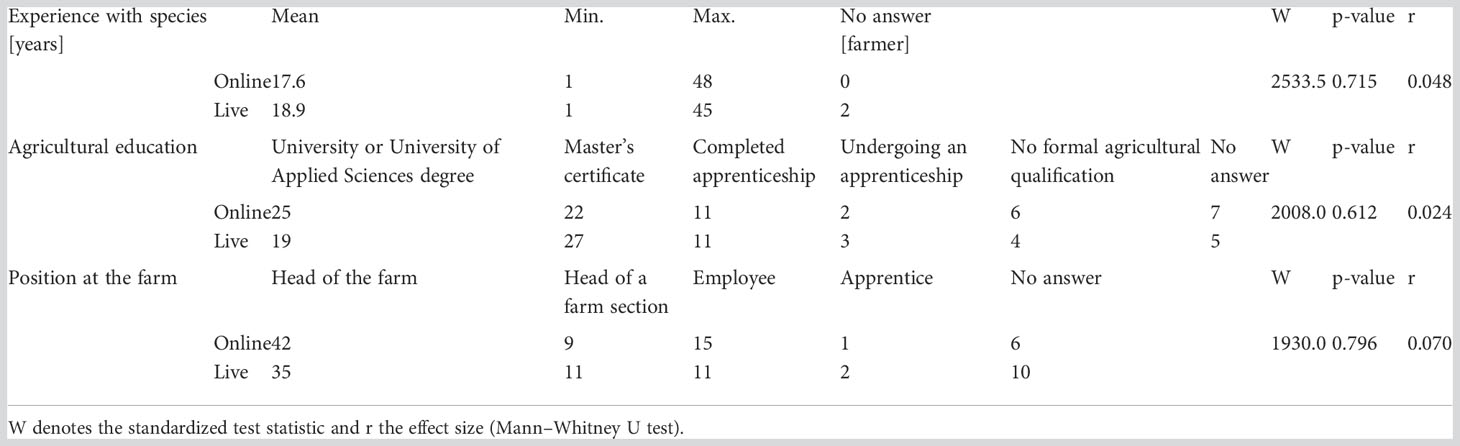
Table 3 Comparison of the farmers’ professional backgrounds between the online and live training groups.
To validate the farmers’ test outputs, we averaged their responses to the four questions on the current motivation to participate in the training (Freund et al., 2011). Both the online (n = 72) and live (n = 68) groups assigned, on average, 2.9 points (on the scale from 1 = less motivated to 4 = motivated).
Training success
After the training, 146 farmers completed, in total, 1,416 individual tests (online = 733, live = 683). After the online training, a PABAK of 0.79 ± 0.016 (least square means ± standard error) was reached in the first tests and a value of 0.83 ± 0.013 in the last tests; after live training, values of 0.81 ± 0.017 and 0.84 ± 0.014 were reached. The PABAK values did not significantly differ between the online and live training (Table 4).
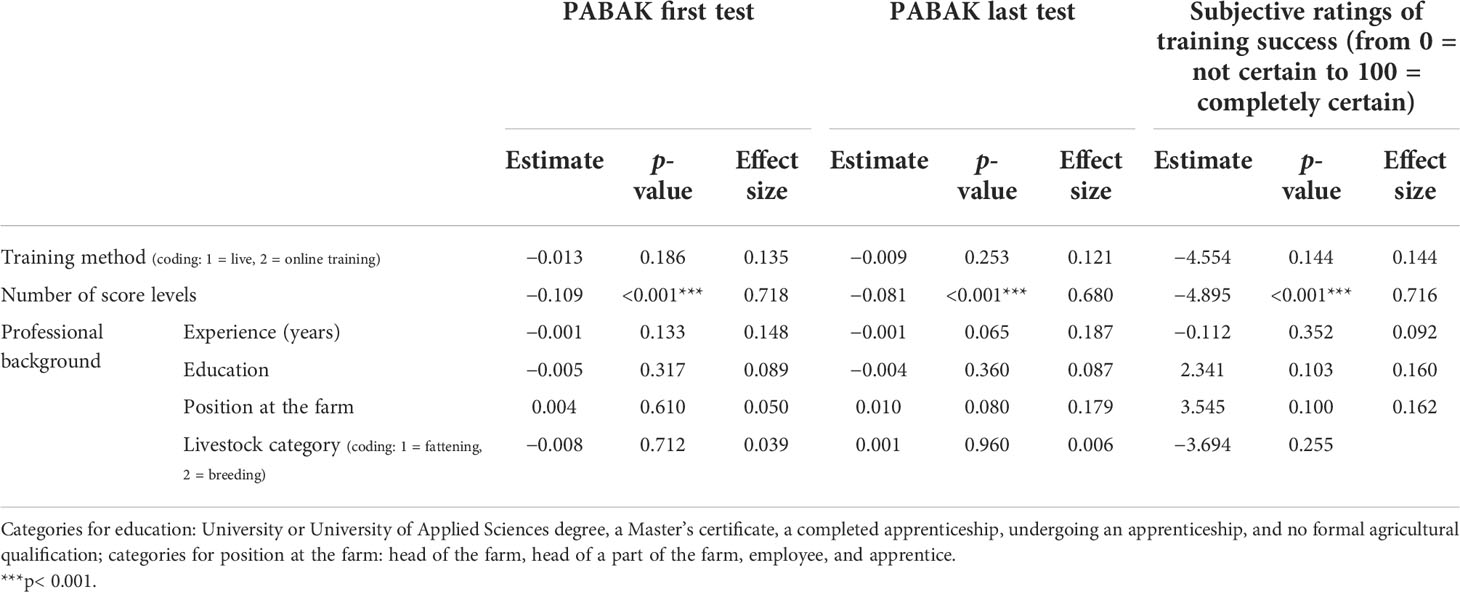
Table 4 Effects of the training method, number of score levels, and the professional background, including livestock category on inter-rater reliability (prevalence-adjusted and bias-adjusted kappa, PABAK) in the first and last online tests and on the subjective ratings of the training success for all indicators (robust mixed model).
The ratings of the subjective training success (from 0 to 100) also did not differ, with 72.4 ± 3.5 (n = 529) after online training and 80.3 ± 3.8 (n = 464) after live training. No significant influence of the farmers’ professional background or the livestock category (fattening = 563 tests, breeding = 853 tests) could be detected. However, significantly lower PABAK values and subjective training success ratings were obtained for indicators with a higher number of score levels (Table 4). The indicators underconditioning in sows and skin lesions in turkeys (both mean PABAK = 0.65) had the lowest mean PABAK values in the last test. Moreover, underconditioning in sows reached a low mean for subjective training success (56.1 points). The lowest value for subjective training success was ranked for extent and quality of beak trimming in turkeys (mean = 50.5), although the PABAK value was 0.82. All PABAK results are presented in Supplementary Table S2.
After the first individual tests (n = 1,148), 154 tests were repeated by the farmers (13.4%). Of these, 58 tests were repeated two or more times (range = 2–20 times). Online-trained farmers repeated tests more often than those trained live (58.4% vs. 41.6% of the tests for online and live, respectively).
The farmers reached PABAK values ≥0.61 in 86.8% of the first tests. For repeated tests, a significant improvement was realized (Wilcoxon test: W = 264.0, p< 0.001, r = 0.655; n = 154), with an improvement from 22.7% of the first tests to 90.9% of the last tests, resulting in PABAK ≥ 0.61. The number of repeated tests per indicator was positively correlated with the improvement of the PABAK value (Spearman’s correlation test: p = 0.774, p< 0.001; n = 154). On average, the improvement in the PABAK value between the first and the last repeated tests was +0.23 (from −0.23 to 0.80).
Feasibility and impact of the training methods on views and attitudes
Farmers who trained online invested significantly less money and time than did farmers who participated in the live training (Table 5). The costs reported by the farmers were mainly from travel expenses and working time. However, both groups of farmers rated the investments as equally adequate and also did not judge the ease of integration of the training into their everyday work differently (Table 5).
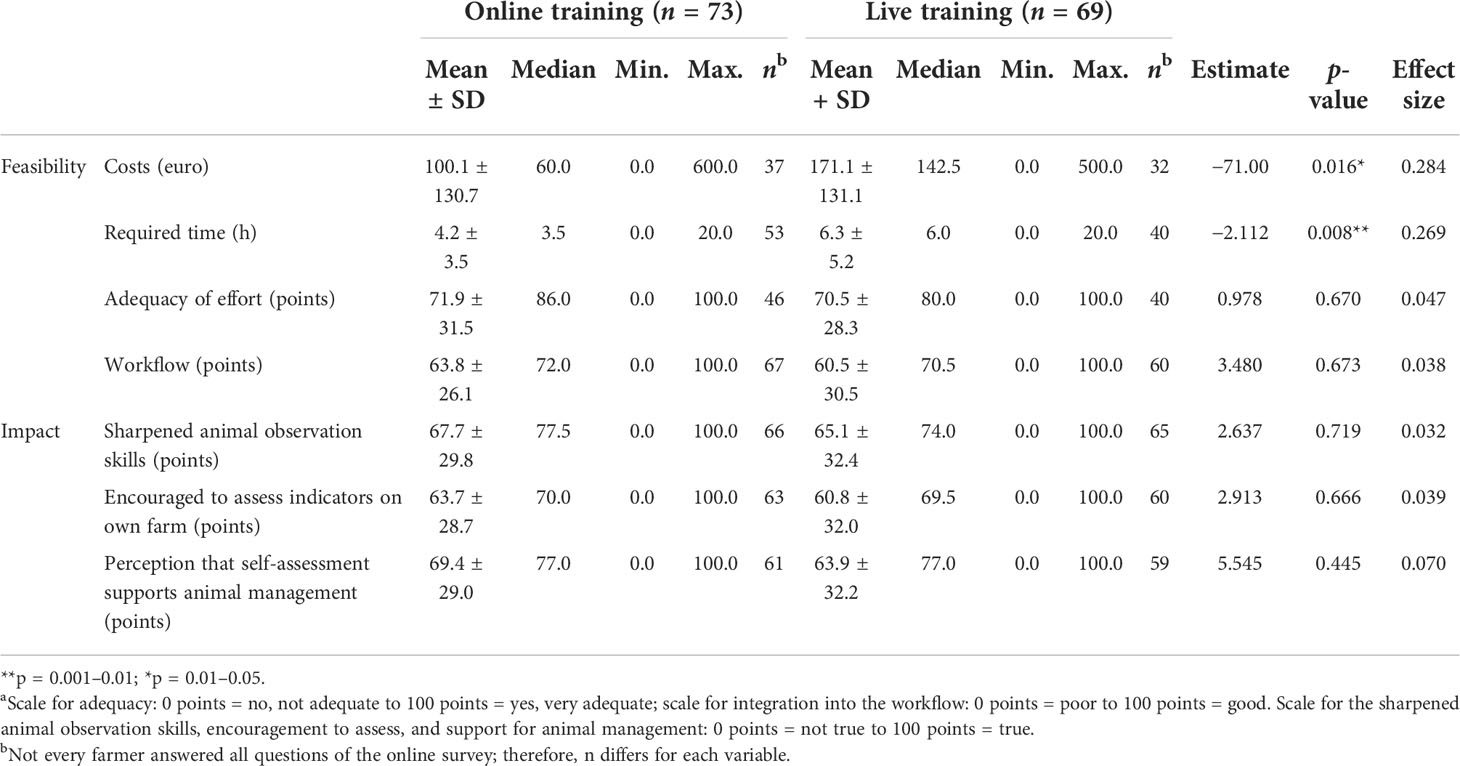
Table 5 Farmers’ ratings of the feasibility and impact of the online and live training (robust mixed model)a.
Regarding the impact of training on views and attitudes, on average, the farmers’ responses indicated a medium effect of the training, with a broad range from 0 to 100 points. On average, 66.4 points were awarded for the statement “the training sharpened the farmers’ animal observation skills,” 62.3 points for “the training encouraged them to implement the assessment on their farms,” and 66.7 points for “the training made it clear that self-assessment supports the animal management,” with no impact of the training method on the ratings (Table 5).
Discussion
The aim of this study was to compare the training success of farmers attending online or live courses on animal welfare self-assessment regarding important animal welfare problems. We hypothesized that live training results in a higher reliability of the assessments and has greater impact on the farmers, but is less feasible, for instance in terms of costs and work organization. Additionally, we expected the reliability to be influenced by the simplicity of the scoring system and the farmers’ professional background, including whether they worked with animals kept for breeding or fattening purposes (livestock category).
Used methods
The combination of an objective inter-rater reliability test with a subjective evaluation of the training success by the person tested is described as a suitable approach to determining the success of a method (Clasen, 2010). In addition, information about the farmers’ current motivation to participate in the training was assessed since it can influence the willingness to perform well (Eklöf, 2010). The average score of 2.9 (range = 1–4) given by the farmers in both training groups reflected a moderately high motivation to participate in the training. Therefore, it appears permissible to use their test performance for the evaluation of the two training methods.
While a great number of different reliability measures are available and controversies about their relative merits have arisen (e.g., Friedrich et al., 2020; Giammarino et al., 2021), we decided to calculate the PABAK, which is not affected by an unbalanced distribution of scores in a sample (Byrt et al., 1993) that otherwise would have led to low kappa values even in cases of high agreement (Feinstein and Cicchetti, 1990). Although we aimed at an equal distribution of the different score levels, our picture and video sets did not reach equipartition for all indicators. Additionally, the PABAK allows comparisons with later on-farm reliability tests, where unbalanced score distributions are to be expected due to low or high prevalence of the assessed welfare aspects.
Training success
On average, we found an equally good inter-rater reliability following both training methods. The same applied to the farmers’ ranking of their subjective training success. Here, the mean points over all indicators were in the upper quartile of the range, although the range was very wide, from 0 to 100. Thus, despite individual variations, the farmers were, on average, rather confident about their future assessments after completing both methods. Although the didactic approach of the live training was standardized across farm animal types, except for the practical training contents for poultry, the training settings varied even within farm animal types, e.g., with regard to group size. It was not possible to consider this statistically, but an exploration of the data revealed that there was no effect of this variation on the training success. In principle, live training allows direct interaction with the trainer, provides the opportunity to discuss clinical findings, and facilitates exchange with colleagues. In line with Strong et al. (2010), we expected this active approach to contribute to a higher training success for farmers. However, this hypothesis was not supported by our results. Nevertheless, it cannot be excluded that the success of the methods might have differed if the inter-rater reliability was tested on-farm rather than media-based. Online tests in general might lead to higher reliability due to the pre-selection of the assessment material, which tends to reduce the number of ambiguous cases. Additionally, adverse environmental conditions such as poor light and moving animals can impair the recognition of certain findings or distract the assessor on-farm. Some indicators, such as keel bone damage in laying hens (Jung et al., 2021) or underconditioning in sows (Welfare Quality® Consortium, 2009b) are commonly assessed by palpation, and some findings might appear differently from the three-dimensional view in the two-dimensional pictures. Therefore, a final evaluation of the two methods should additionally take into account an on-farm inter-rater reliability assessment. In addition, trainees’ individual preferences for online or live training might have affected the success of the different training methods. For instance, the results may depend on the extent of experience with web applications and online learning. However, possible effects on the inter-rater reliability might have been reduced as all farmers had to complete the reliability test online.
Picture- and video-based reliability tests have already been used in various studies (Mullan et al., 2011; Gibbons et al., 2012; Vasseur et al., 2013; Staaf Larsson et al., 2021). In addition to allowing the presentation of a wide and evenly distributed range of findings, media-based tests can be completed at different times and locations. Furthermore, an instant and automated reliability feedback, based on earlier assessments of the same pictures and videos by experts, is possible. In the case of test repetitions, different materials can also be provided immediately. Although only 13.4% of tests had been repeated in our study, these repetitions resulted in better inter-rater reliability (mean + 0.23). Of these tests, more were repeated by farmers who did online training than by those who trained live. Perhaps this indicates that the online training better familiarized the farmers with the media-based tests and, therefore, a repetition was more likely or that the exercises of the live training were more effective so that the farmers felt less need for improvement in the tests. A test repetition of the media-based tests can therefore be recommended in case of insufficient reliability or if there is willingness to improve and learn from mistakes (Croyle et al., 2018). The farmers’ professional backgrounds had no influence of on the inter-rater reliability and the subjective training success. This is in line with findings of Garcia et al. (2015), where the raters’ professions (veterinarians, farmers, veterinary students, researchers, and assessors) and years of experience did not influence the inter-rater reliability (Gibbons et al., 2012; Garcia et al., 2015). Since all participants in the present study had worked at least 1 year with the specific farm animal type, it remains open how well the training works for inexperienced stockpersons. Nevertheless, we can conclude that it is possible to successfully train farmers within the investigated wide range of professional backgrounds, from employees and no formal education to heads of farms and academic degrees and with 1 to 48 years of work experience.
In addition, we looked at possible differences between farmers working with animals kept for breeding and those farming for fattening purposes. Farmers from the fattening sector usually work with animals with shorter lifetimes and have less direct interactions with these animals compared to farmers working with breeding animals (including egg- or milk-producing animals). The former have been reported to be more detached from their animals (Wilkie, 2005; Bock et al., 2007), while farmers working with sows and dairy cows are closer to their animals (Kling-Eveillard et al., 2020). Therefore, it seemed possible that farmers working with breeding animals are more motivated to learn the self-assessment or that the approach is more familiar to them. In the present study, however, no differences in the training success were found between the farmers working with breeding animals and farmers working with animals for fattening purposes.
On the individual test level, the farmers reached mean PABAK values representing substantial to almost perfect agreement. The PABAK means did not differ substantially compared to those reported from reliability tests performed by scientists (using PABAK), for example for keel bone damage in laying hens (Jung et al., 2021); manure on the body of pigs (Wimmler et al., 2021); body condition, lameness, and cleanliness in dairy cows (Wagner et al., 2021); footpad dermatitis in turkeys (Stracke et al., 2020) or broilers (Piller et al., 2020; slightly higher in Louton et al., 2019); and stereotypies, skin lesions, underconditioning, and lameness in sows (Friedrich, 2018). For some of the indicators in our study, the reliability was relatively low and showed potential for improvement by using fewer score levels or modified definitions. The indicator underconditioning in sows had the highest number of score levels (five levels) and reached the lowest mean PABAK. This largely contributed to the overall pattern of a negative correlation between the number of scores and reliability. This association has already been highlighted in the literature (Brenninkmeyer et al., 2007; March et al., 2007; Knierim and Winckler, 2009; Mullan et al., 2011). Another influence may be the need to palpate the sows to properly assess their body condition (Welfare Quality® Consortium, 2009b). Low agreement was also achieved for skin lesions in turkeys. Here, a complex definition of the scores with different lesion sizes depending on the body region may have been the cause and calls for simplification of the score definitions.
Feasibility of the training
The feasibility is important to facilitate participation in the self-assessment training. According to the farmers’ responses, those who trained online spent on average about 2 h less on the training with lower estimated expenses (mean = 100€ vs. 171€) while reaching similar test results. This higher efficiency can be explained by the absence of travel hours and travel expenses in the online training, which was also mentioned by Brown (2000). In our study, farmers had been allocated to the live training also depending on the ease of access to the training location, so that the costs and travel hours might even be higher. For instance, Macurik et al. (2008) recommend the use of online training when the trainer or the participants need to travel far or when more frequent training is required. In the current study, the participants considered live training to be as appropriate as the online training, despite the need of more time and higher costs. Therefore, either the farmers were willing to invest more money and time in a live training or the threshold at which they perceived the costs as no longer appropriate was not reached.
Both online- and live-trained farmers, on average, ranked the ease of integration of the training into their workflow equally in the upper half of the range. Thus, the more flexible use of online training in terms of direct and permanent access to the content did not appear to provide any significant advantage for the integration into the workflow. Similarly to the costs, a 1-day live training could be well enough integrated into the work routine so that the online training did not bring any advantage in this regard.
Further advantages of online training compared to live training include the reduction of stress for the animals, if animal handling is part of the live training, and the avoidance of biosecurity problems on-farm and for farmers themselves. Especially in circumstances such as the coronavirus disease 2019 (COVID-19) pandemic, online training can be useful and effective for information transfer (Quayson et al., 2020).
Concerning feasibility, it appears that both training methods with their specific characteristics can play an important role in the training of farmers on the assessment of animal welfare indicators. Possibly, even a choice or a combination of the two methods is advantageous, addressing different individual conditions, preferences, and circumstances. For instance, according to Main et al. (2012) and Vasseur et al. (2013), online training is particularly suited for regular refresher training. This may also be beneficial for animal welfare self-assessment since the recommended intervals between assessments for cattle and pigs amount to half a year (Brinkmann et al., 2016; Schrader et al., 2016; Brinkmann et al., 2020; Schrader et al., 2020).
Impact of the training methods on views and attitudes
For each indicator, the training concept included background information on the respective animal welfare issues. Thus, we expected the training to sharpen the farmers’ animal observation skills, encourage them to conduct on-farm self-assessment to improve animal welfare, and enhance their perception of self-assessment supporting the animal management. However, although the means of the participants’ responses for each of these aspects were in the upper half of the range, they revealed only a moderate impact of the training, without differences between training methods. A possible reason for the moderate impact could be the lack of practical components in the training, except for the poultry live training. Since farming is a strongly practice-based profession, Strong et al. (2010) explicitly recommend hands-on experiences and instructions for farmers. Another reason could be the rather controversial debate in the German farming sector about the value of the legal obligation to perform on-farm self-assessments of animal welfare, which may have influenced some of the responses.
Conclusion
The online and live training methods were equally effective in teaching the assessment of animal-based welfare indicators for on-farm self-assessment, with no impact of the professional background of the farmers and the livestock category. Both the inter-rater reliability and the subjective rating to assess the indicators on their farms showed good results on average. However, it was also confirmed that scores with fewer levels lead to better training success. Although online training required less input in terms of time and costs, farmers in both training methods ranked the effort and ease of integration into the workflow as equally acceptable. Similarly, the farmers attributed an equally moderate positive impact to both training methods in that their views on the animals changed, they were encouraged to assess the indicators on their farm, and that they perceived the self-assessment as a support for their animal management. In summary, we conclude that both the online and live training concepts are feasible and allow for the successful training of farmers from a variety of farm animal types and professional backgrounds to apply the proposed animal welfare on-farm self-assessment protocols.
Data availability statement
The raw data supporting the conclusions of this article will be made available by the authors, without undue reservation.
Ethics statement
Ethical review and approval was not required for the study on human participants in accordance with the local legislation and institutional requirements. The participants provided written informed consent to participate in this study.
Author contributions
All authors except SL contributed to the conception of the training. AS, DG, KC, RZ, JB, SoM, and SaM elaborated and conducted the training. All authors contributed to the conception and design of the study. SaM organized the database, performed the statistical analysis, and wrote the first draft of the manuscript. All authors contributed to manuscript revision, read, and approved the submitted version.
Funding
The present study is part of the project “Feasibility of Animal Welfare Indicators for the On-Farm Self-Assessment, Development of an Orientation Framework With Reference Values and Technical Implementation in Digital Applications (EiKoTiGer)” at the University of Kassel, Friedrich-Loeffler Institute of Animal Welfare and Animal Husbandry, Thünen Institute of Organic Farming and Association for Technology and Structures in Agriculture, Germany. The project was funded by the German Federal Ministry of Food and Agriculture (BMEL) based on a decision of the Parliament of the Federal Republic of Germany, granted by the Federal Office for Agriculture and Food (www.ble.de/ptble/innovationsfoerderung-bmel; grant nos. 2817900915, 2817901015, 2817901115, and 2817901215) under the Innovation Scheme. The funder had no role in the study design, data collection and analysis, decision to publish, or preparation of the manuscript.
Acknowledgments
We gratefully acknowledge all participating farmers for their contributions. Our special thanks also go to our colleagues and students for pretesting the training and giving helpful feedback and to Carlo Michaelis (University of Göttingen, Third Institute of Physics) for advice on statistical analyses.
Conflict of interest
The authors declare that the research was conducted in the absence of any commercial or financial relationships that could be construed as a potential conflict of interest.
Publisher’s note
All claims expressed in this article are solely those of the authors and do not necessarily represent those of their affiliated organizations, or those of the publisher, the editors and the reviewers. Any product that may be evaluated in this article, or claim that may be made by its manufacturer, is not guaranteed or endorsed by the publisher.
Supplementary material
The Supplementary Material for this article can be found online at: https://www.frontiersin.org/articles/10.3389/fanim.2022.915708/full#supplementary-material
References
AssureWel (2016) AssureWel training tool. Available at: http://www.assurewel.org/training.html (Accessed March 12, 2022).
AWARE (2017) E-learning. Available at: http://www.organic-animal-welfare.eu/de/e-learning (Accessed March 12, 2022).
Beggs D., Jongman E., Hemsworth P., Fisher A. (2019). Lame cows on Australian dairy farms: a comparison of farmer-identified lameness and formal lameness scoring, and the position of lame cows within the milking order. J. Dairy Sci. 102 (2), 1522–1529. doi: 10.3168/jds.2018-14847
Bio Austria (2021) Tierwohl auf einen blick. Available at: https://www.bio-austria.at/tierwohl-auf-einen-blick (Accessed March 12, 2022).
Bock B. B., Van Huik M. M., Prutzer M., Kling-Eveillard F., Dockes A. (2007). Farmers’ relationship with different animals: the importance of getting close to the animals. case studies of French, Swedish and Dutch cattle, pig and poultry farmers. Int. J. Sociol. Agric. Food. 15 (3), 108–125. doi: 10.48416/ijsaf.v15i3.290
Brenninkmeyer C., Dippel S., March S., Brinkmann J., Winckler C., Knierim U. (2007). Reliability of a subjective lameness scoring system for dairy cows. Anim. Welfare 16 (2), 127–129.
Brinkmann J., Cimer K., March S., Ivemeyer S., Pelzer A., Schultheiß U., et al. (2020). “Tierschutzindikatoren: Leitfaden für die praxis – rind,” in Vorschläge für die produktionsrichtungen milchkuh, aufzuchtkalb, mastrind (2nd ed.) (Darmstadt: KTBL).
Brinkmann J., Ivemeyer S., Pelzer A., Winckler C., Zapf R. (2016). “Tierschutzindikatoren: Leitfaden für die praxis – rind,” in Vorschläge für die produktionsrichtungen milchkuh, aufzuchtkalb, mastrind (Darmstadt: KTBL).
Brown B. L. (2000). “Web-based-training,” in ERIC digest no. 218 (Washington, DC: Office of Educational Research and Improvements).
Byrt T., Bishop J., Carlin J. B. (1993). Bias, prevalence and kappa. J. Clin. Epidemiol. 46 (5), 423–429. doi: 10.1016/0895-4356(93)90018-v
Clasen H. (2010). “Die messung von lernerfolg: eine grundsätzliche aufgabe der evaluation von lehr-bzw,” in Trainingsinterventionen [dissertation] (Dresden (Germany: University of Dresden).
Cohen J. (1988). Statistical power analysis for the behavioral sciences (2nd ed.) (Hillsdale, N.J: L. Erlbaum Associates).
Croyle S. L., Nash C. G. R., Bauman C., LeBlanc S. J., Haley D. B., Khosa D. K., et al. (2018). Training method for animal-based measures in dairy cattle welfare assessments. J. Dairy Sci. 101 (10), 9463–9471. doi: 10.3168/jds.2018-14469
Dockès A. C., Kling-Eveillard F. (2006). Farmers' and advisers' representations of animals and animal welfare. Livestock Sci. 103 (3), 243–249. doi: 10.1016/j.livsci.2006.05.012
Eklöf H. (2010). Skill and will: test-taking motivation and assessment quality. Assess. Education: Principles Policy Practice 17 (4), 345–356. doi: 10.1080/0969594X.2010.516569
Feinstein A. R., Cicchetti D. V. (1990). High agreement but low kappa: I. the problems of 2 paradoxes. J. Clin. Epidemiol. 43, 543–549. doi: 10.1016/0895-4356(90)90158-L
Ferguson J. D., Azzaro G., Licitra G. (2006). Body condition assessment using digital images. J. Dairy Sci. 89 (10), 3833–3841. doi: 10.3168/jds.S0022-0302(06)72425-0
Ferrante V., Watanabe T. T. N., Marchewka J., Estevez I. (2015). AWIN welfare assessment protocol for turkeys. doi: 10.13130/AWIN_TURKEYS_2015
Field A. P., Miles J., Field Z. (2012). Discovering statistics using r (Great Britain: SAGE Publications). doi: 10.1111/insr.12011_21
Freund P. A., Kuhn J. T., Holling H. (2011). Measuring current achievement motivation with the QCM: Short form development and investigation of measurement invariance. Pers. Individ. Diff. 51 (5), 629–634. doi: 10.1016/j.paid.2011.05.033
Friedrich L. (2018). The welfare quality animal welfare assessment protocol for sows and piglets' - on-farm evaluation and possible improvements of feasibility and reliability (Kiel (Germany: University of Kiel).
Friedrich L., Krieter J., Kemper N., Czycholl I. (2020). Interobserver reliability of measures of the welfare quality® animal welfare assessment protocol for sows and piglets. Anim. Welfare 29 (3), 323–337. doi: 10.7120/09627286.29.3.323
Garcia E., König K., Allesen-Holm B. H., Klaas I. C., Amigo J. M., Bro R., et al. (2015). Experienced and inexperienced observers achieved relatively high within-observer agreement on video mobility scoring of dairy cows. J. Dairy Sci. 98 (7), 4560–4571. doi: 10.3168/jds.2014-9266
Giammarino M., Mattiello S., Battini M., Quatto P., Battaglini L. M., Vieira A. C. L., et al. (2021). Evaluation of inter-observer reliability of animal welfare indicators: which is the best index to use? Animals 11, 1445. doi: 10.3390/ani11051445
Gibbons J., Vasseur E., Rushen J., de Passillé A. M. (2012). A training programme to ensure high repeatability of injury scoring of dairy cows. Anim. Welfare 21 (3), 379–388. doi: 10.7120/09627286.21.3.379
Heerkens J., Delezie E., Rodenburg T. B., Kempen I., Zoons J., Ampe B., et al. (2016). Risk factors associated with keel bone and foot pad disorders in laying hens housed in aviary systems. Poultry Sci. 95 (3), 482–488. doi: 10.3382/ps/pev339
Ivemeyer S., Bell N. J., Brinkmann J., Cimer K., Gratzer E., Leeb C., et al. (2015). Farmers taking responsibility for herd health development —stable schools in research and advisory activities as a tool for dairy health and welfare planning in Europe. Org Agricult. 5 (2), 135–141. doi: 10.1007/s13165-015-0101-y
Jung L., Nasirahmadi A., Schulte-Landwehr J., Knierim U. (2021). Automatic assessment of keel bone damage in laying hens at the slaughter line. Animals 11, 163. doi: 10.3390/ani11010163
Kielland C., Skjerve E., Østerås O., Zanella A. J. (2010). Dairy farmer attitudes and empathy toward animals are associated with animal welfare indicators. J. Dairy Sci. 93 (7), 2998–3006. doi: 10.3168/jds.2009-2899
Kling-Eveillard F., Allain C., Boivin X., Courboulay V., Creach P., Philibert A., et al. (2020). Farmers’ representations of the effects of precision livestock farming on human-animal relationships. Livestock Sci. 238, 104057. doi: 10.1016/j.livsci.2020.104057
Knierim U., Andersson R., Keppler C., Petermann S., Rauch E., Spindler B., et al. (2016). Tierschutzindikatoren: Leitfaden für die praxis - geflügel. vorschläge für die produktionsrichtungen Jung- und legehenne, masthuhn, mastpute (Darmstadt: KTBL).
Knierim U., Gieseke D., Michaelis S., Keppler C., Spindler B., Rauch E., et al. (2020). Tierschutzindikatoren: Leitfaden für die praxis – geflügel. vorschläge für die produktionsrichtungen Jung- und legehenne, masthuhn, mastpute (2nd ed.) (Darmstadt: KTBL).
Knierim U., Winckler C. (2009). On-farm welfare assessment in cattle: validity, reliability and feasibility issues and future perspectives with special regard to the welfare quality® approach. Anim. Welfare 18 (4), 451–458.
Koller M. (2016). Robustlmm: An r package for robust estimation of linear mixed-effects models. J. Stat. Software 75 (6), 1–24. doi: 10.18637/jss.v075.i06
Landis J. R., Koch G. G. (1977). The measurement of observer agreement for categorical data. Biometrics 33 (1), 159–174. doi: 10.2307/2529310
Lenski A. E., Hecht M., Penk C., Milles F., Mezger M., Heitmann P., et al. (2016). IQB-ländervergleich 2012. skalenhandbuch zur dokumentation der erhebungsinstrumente (Berlin: Humboldt-Universität zu Berlin). doi: 10.20386/HUB-42547
Liu N., Kaler J., Ferguson E., O’Kane H., Green L. E. (2018). Sheep farmers’ attitudes to farm inspections and the role of sanctions and rewards as motivation to reduce the prevalence of lameness. Anim. Welfare 27 (1), 67–79. doi: 10.7120/09627286.27.1.067
Louton H., Keppler C., Erhard M., van Tuijl O., Bachmeier J., Damme K., et al. (2019). Animal-based welfare indicators of 4 slow-growing broiler genotypes for the approval in an animal welfare label program. Poultry Sci. 98 (6), 2326–2337. doi: 10.3382/ps/pez023
Luke S. G. (2017). Evaluating significance in linear mixed-effects models in r. Behav. Res. Methods 49 (4), 1494–1502. doi: 10.3758/s13428-016-0809-y
Macurik K. M., O'Kane N. P., Malanga P., Reid D. H. (2008). Video training of support staff in intervention plans for challenging behavior: comparison with live training. Behav. Interventions 23 (3), 143–163. doi: 10.1002/bin.261
Main D., Mullan S., Atkinson C., Bond A., Cooper M., Fraser A., et al. (2012). Welfare outcomes assessment in laying hen farm assurance schemes. Anim. Welfare 21 (3), 389–396. doi: 10.7120/09627286.21.3.389
March S., Brinkmann J., Winckler C. (2007). Effect of training on the inter-observer reliability of lameness scoring in dairy cattle. Anim. Welfare 16 (2), 131–133.
March S., Brinkmann J., Winckler C. (2014). Improvement of animal health in organic dairy farms through ‘stable schools’: selected results of a pilot study in Germany. Org Agricult. 4, 319–323. doi: 10.1007/s13165-014-0071-5
Mullan S., Edwards S. A., Butterworth A., Whay H. R., Main D. (2011). Inter-observer reliability testing of pig welfare outcome measures proposed for inclusion within farm assurance schemes. Veterinary J. 190 (2), e100–e109. doi: 10.1016/j.tvjl.2011.01.012
Oliveira A., Lund V. P., Christensen J. P., Nielsen L. R. (2017). Inter-rater agreement in visual assessment of footpad dermatitis in Danish broiler chickens. Br. Poultry Sci. 58 (3), 224–229. doi: 10.1080/00071668.2017.1293231
Piller A., Bergmann S., Schwarzer A., Erhard M., Stracke J., Spindler B., et al. (2020). Validation of histological and visual scoring systems for foot-pad dermatitis in broiler chickens. Anim. Welfare 29 (2), 185–196. doi: 10.7120/09627286.29.2.185
Pol F., Kling-Eveillard F., Champigneulle F., Fresnay E., Ducrocq M., Courboulay V. (2021). Human-animal relationship influences husbandry practices, animal welfare and productivity in pig farming. Animal 15 (2), 100103. doi: 10.1016/j.animal.2020.100103
Quayson M., Bai C., Osei V. (2020). Digital inclusion for resilient post-COVID-19 supply chains: smallholder farmer perspectives. IEEE Eng. Manage. Rev. 48 (3), 104–110. doi: 10.1109/EMR.2020.3006259
Rousing T., Holm J. R., Krogh M. A., Østergaard S., GplusE Consortium (2020). Expert-based development of a generic HACCP-based risk management system to prevent critical negative energy balance in dairy herds. Prev. Veterinary Med. 175, 104849. doi: 10.1016/j.prevetmed.2019.104849
Schrader L., Czycholl I., Krieter J., Leeb C., Zapf R., Ziron M. (2016). Tierschutzindikatoren: Leitfaden für die praxis - schwein. vorschläge für die produktionsrichtungen sauen, saugferkel, aufzuchtferkel und mastschweine (Darmstadt: KTBL).
Schrader L., Schubbert A., Rauterberg S., Czycholl I., Leeb C., Ziron M., et al. (2020). Tierschutzindikatoren: Leitfaden für die praxis – schwein. vorschläge für die produktionsrichtungen sauen, saugferkel, aufzuchtferkel und mastschweine (2nd ed.) (Darmstadt: KTBL).
Staaf Larsson B., Petersson E., Stéen M., Hultgren J. (2021). Visual assessment of body condition and skin soiling in cattle by professionals and undergraduate students using photo slides. Acta Agricult Scandinavica 70 (1), 31–40. doi: 10.1080/09064702.2020.1849380
Stracke J., Klotz D., Wohlsein P., Döhring S., Volkmann N., Kemper N., et al. (2020). Scratch the surface: histopathology of footpad dermatitis in turkeys (Meleagris gallopavo). Anim. Welfare 29 (4), 419–432. doi: 10.7120/09627286.29.4.419
Strong R., Harder A., Carter H. (2010). Agricultural extension agents' perceptions of effective teaching strategies for adult learners in the master beef producer program. J. Extension 48 (3), 1–7.
TierSchG (2006). Tierschutzgesetz [Animal welfare act], as of 18 May 2006 (BGBl. I p. 1206, 1313), last amended 10 August 2021 (BGBl. I p. 3436). German legislation
University of California (2021) Cow-calf health and handling assessment. Available at: https://www.ucdcowcalfassessment.com (Accessed March 12, 2022).
Vaarst M., Fisker I. (2013). Potential contradictions connected to the inclusion of stable schools in the legislation for danish organic dairy farms. Open Agric. J. 7, 118–124. doi: 10.2174/1874331501307010118
van Dijk L., Buller H. J., Blokhuis H. J., Niekerk T. V., Voslarova E., Manteca X., et al. (2019). HENNOVATION: learnings from promoting practice-led multi-actor innovation networks to address complex animal welfare challenges within the laying hen industry. Animals 9 (1), 24–36. doi: 10.3390/ani9010024
Vasseur E., Gibbons J., Rushen J., de Passillé A. M. (2013). Development and implementation of a training program to ensure high repeatability of body condition scoring of dairy cows. J. Dairy Sci. 96 (7), 4725–4737. doi: 10.3168/jds.2012-6359
Vieira A., Brandão S., Monteiro A., Ajuda I., Stilwell G. (2015). Development and validation of a visual body condition scoring system for dairy goats with picture-based training. J. Dairy Sci. 98 (9), 6597–6608. doi: 10.3168/jds.2015-9428
Wagner K., Brinkmann J., Bergschmidt A., Renziehausen C., March S. (2021). The effects of farming systems (organic vs. conventional) on dairy cow welfare, based on the welfare quality® protocol. Animal 15 (8), 100301. doi: 10.1016/j.animal.2021.100301
Welfare Quality® Consortium (2009a). Welfare quality® assessment protocol for cattle (Lelystad, the Netherlands).
Welfare Quality® Consortium (2009b). Welfare quality® assessment protocol for pigs (Lelystad, the Netherlands).
Welfare Quality® Consortium (2009c). Welfare quality® assessment protocol for poultry (Lelystad, the Netherlands).
Whay H. R., Main D., Green L. E., Webster A. (2003). Assessment of the welfare of dairy cattle using animal-based measurements: direct observations and investigation of farm records. Veterinary Rec. 153 (7), 197–202. doi: 10.1136/vr.153.7.197
Wilkie R. (2005). Sentient commodities and productive paradoxes: the ambiguous nature of human–livestock relations in northeast Scotland. J. Rural Stud. 21 (2), 213–230. doi: 10.1016/j.jrurstud.2004.10.002
Keywords: animal welfare, training, animal-based indicators, self-assessment, reliability, feasibility
Citation: Michaelis S, Schubbert A, Gieseke D, Cimer K, Zapf R, Lühken S, March S, Brinkmann J, Schultheiß U and Knierim U (2022) A comparison of online and live training of livestock farmers for an on-farm self-assessment of animal welfare. Front. Anim. Sci. 3:915708. doi: 10.3389/fanim.2022.915708
Received: 08 April 2022; Accepted: 19 October 2022;
Published: 14 November 2022.
Edited by:
Ruth Catriona Newberry, Norwegian University of Life Sciences, NorwayReviewed by:
Andrea Bragaglio, Council for Agricultural and Economics Research (CREA), ItalyLuiene Moura Rocha, Centre de Développement du Porc du Québec, Canada
Cristian Larrondo, Universidad de las Américas, Chile
Copyright © 2022 Michaelis, Schubbert, Gieseke, Cimer, Zapf, Lühken, March, Brinkmann, Schultheiß and Knierim. This is an open-access article distributed under the terms of the Creative Commons Attribution License (CC BY). The use, distribution or reproduction in other forums is permitted, provided the original author(s) and the copyright owner(s) are credited and that the original publication in this journal is cited, in accordance with accepted academic practice. No use, distribution or reproduction is permitted which does not comply with these terms.
*Correspondence: Sarina Michaelis, bWljaGFlbGlzQHVuaS1rYXNzZWwuZGU=