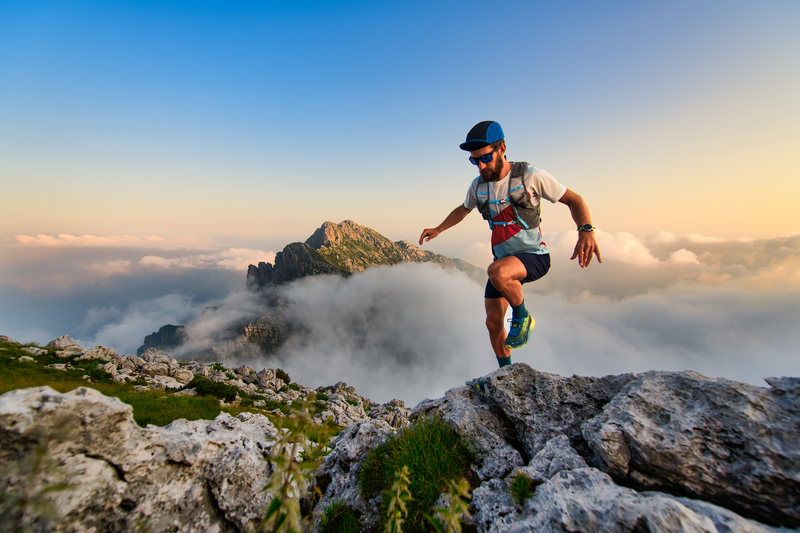
94% of researchers rate our articles as excellent or good
Learn more about the work of our research integrity team to safeguard the quality of each article we publish.
Find out more
ORIGINAL RESEARCH article
Front. Anesthesiol. , 05 December 2024
Sec. General Pharmacology and Pharmacokinetics
Volume 3 - 2024 | https://doi.org/10.3389/fanes.2024.1464004
Background: Immunomodulation is widely invoked to explain possible effects of anesthetic/analgesic drugs on recurrence and survival in cancer patients. By analogy with immune checkpoint inhibitors, which enhance anti-tumor actions of immune cells in the tumor microenvironment (TME), we aim to develop a precision approach to immunomodulation by anesthetic/analgesic drugs. We explore biomarkers predictive of immunotherapy response [tumor mutational burden (TMB)] and resistance [fraction genome altered (FGA)] in relation to anesthetic/analgesic dose to survival response and the expression of drug target receptor genes.
Methods: Two local clinical cohorts [lung adenocarcinoma (LUAD) and colon adenocarcinoma (COAD) patients] were analyzed retrospectively to yield statistical interactions between drugs, outcomes, and TMB/FGA (extending previously reported results). Bulk tumor gene expression data for solid tumors from 6,488 patients across 18 solid tumor types was obtained from The Cancer Genome Atlas (TCGA) and normalized by tumor type. TMB and FGA for each TCGA patient sample was extracted from cBioPortal. DeSeq was employed to quantify differential gene expression of target receptors of 79 common anesthetic/analgesic drugs for high/low TMB and FGA. Localization of these receptors to specific immune cells was estimated using CIBERSORT.
Results: Increased TMB and FGA magnified opioid pro-tumor effects on overall survival in LUAD, while increased TMB reduced ketamine anti-tumor effects on recurrence and did not affect ketorolac anti-tumor effects on recurrence. In COAD, increased TMB (DNA mismatch repair deficiency) magnified opioid anti-tumor effects on recurrence. Drug target receptor gene expression (and immune cell-type specificity) correlated with both TMB and FGA as a function of cancer type.
Conclusions: TMB and FGA may have utility as biomarkers predictive of individual cancer patient response to anesthetic/analgesic dose effects on survival due to immunomodulation. Correlation across cancer types of anesthetic/analgesic target receptor gene expression with TMB and FGA and with TME immune cell types suggests molecular/omics level targets for further mechanistic exploration. A precision oncoanalgesia approach in the cancer patient may ultimately be warranted to optimize oncological outcomes.
The question of whether anesthetic or analgesic drugs can have oncological effects remains very much incompletely answered (1) despite being raised over four decades ago (2). A recent line of inquiry maintains that the way to approach this question is from the vantage point of modern oncology (3, 4), to focus on the genomics (and transcriptomics, etc.) from which emerge the differences between cancer types generally and between individual patient tumors more specifically, and which are key determinants in the relationships between drug perturbations and oncological outcomes such as recurrence and survival (5).
Immunomodulation has long been invoked as the primary mechanism underlying anesthetic/analgesic drug effects on cancer (6). The specific formulation of this idea has historically been that of opioid-induced immunosuppression (7): opioids suppress immune function and enable tumor cells to escape immune-surveillance, leading to disease progression. The precision oncological approach has demonstrated the possibility that in fact opioids specifically may be either pro- or anti-tumor, depending on cancer type and variation in individual patient-specific tumor genomics, and furthermore that mechanisms underlying these effects may be immune-mediated as well as resulting from drug action on oncogenic pathways in tumor cells (8–13).
Ultimate validation of this precision approach is prediction of individual patient susceptibility to oncological effects of analgesic agents. Recent retrospective work integrating clinicopathologic and omics data has generated hypotheses for specific tumor genomic mutations that may be able to predict opioid, ketamine, and ketorolac effects on oncological outcomes for specific patients, pending further prospective validation, through direct on-tumor effects (11–13). The aim of the present work is to identify factors that may do the same based on immune-mediated effects of analgesic agents, i.e., to articulate a precision approach to analgesic-induced immunomodulation.
From the oncological viewpoint, an analogy can be made between anesthetic/analgesic drugs and immunotherapeutic agents, in particular immune checkpoint inhibitors (ICI), which enhance anti-tumor actions of immune cells in the tumor microenvironment (TME) (14). Recognizing that a particular anesthetic/analgesic drug may be pro- or anti-tumor depending on the context (cancer type and individual patient-specific tumor omics), the common factor here is immunomodulation at the cellular level, i.e., action on immune cells in the TME. As with ICI, characteristics of the TME may render a specific patient's tumor more susceptible to drug effects on TME immune cells. Practically, specific biomarkers may reflect these characteristics and enable prediction of drug effects.
The fundamental characteristic determining ICI efficacy is tumor immunogenicity, which can be defined as a tumor's ability to induce anti-tumor immune response (15), reflected by high levels of tumor-infiltrating lymphocytes (TILs). A major contributor to immunogenicity is tumor mutational burden (TMB), defined as the number of somatic mutations per megabase of genomic sequence (16). TMB captures the number of non-silent mutated single base pairs in tumor DNA, meaning that these mutations result in tumor cells producing neoantigens, i.e., new molecules translated from the mutated DNA that bind to T-cell receptors, are recognized as “non-self”, and therefore stimulate anti-tumor immune activity (17). TMB has been extensively studied as a predictive biomarker for response to ICI (18, 19) and is used clinically to guide ICI treatment (20), though challenges remain in terms of its general implementation across cancer types and individuals (21).
We hypothesize that immunomodulation by anesthetic/analgesic drugs should similarly depend on tumor immunogenicity since these drugs need to have a substrate (i.e., TILs) to modulate to exert on-tumor effects (either pro- or anti-tumor). We therefore hypothesize that TMB may serve as a predictive biomarker for individual patient susceptibility to immunomodulation by these drugs, manifesting as a change in the dose-response curves for associations between drug dose and oncological outcome. We further explore anesthetic/analgesic target receptor gene expression in relation to individual patient immunogenicity, as represented by TMB. In addition to TMB, we explore dose-response and receptor gene expression in the context of another ICI biomarker, fraction genome altered (FGA), which measures copy number variation (deletion or new copies of entire genome segments). FGA reflects duplication or deletion of DNA in lengths from single genes to entire chromosomes, in contrast to the single base pair changes captured by TMB, and in contrast to TMB appears to predict resistance to ICI (22–24).
We previously retrospectively analyzed two large prospectively collected datasets for early-stage patients presenting for primary tumor resection at Memorial Sloan Kettering Cancer Center: 740 patients with lung adenocarcinoma (MSK-LUAD) (11) and 1,157 patients with colon adenocarcinoma (MSK-COAD) (12). In the present work we revisited these datasets to further probe relationships between TMB, FGA, drugs, and outcomes.
The Cancer Genome Atlas (TCGA) (16) contains clinicopathologic and bulk RNA sequencing gene expression data for over 20,000 primary cancer samples from 33 cancer types. We focused on 18 TCGA solid tumor cohorts, corresponding to 6,488 patients (see Supplementary Table S1 for a breakdown of total samples by cancer type).
The MSK-LUAD cohort featured next-generation sequencing (MSK-IMPACT) (25) of tumor samples removed during surgery, which enabled calculation of TMB and FGA (among other genomic factors). The MSK-COAD cohort featured immunohistochemical determination of DNA mismatch repair (MMR) status [either deficient (dMMR) or proficient (pMMR)] (26). MMR status correlates with TMB, where dMMR patients have high TMB and pMMR patients have low TMB (27, 28). TCGA cohorts featured TMB and FGA values for patient tumor samples, which were obtained through cBioPortal (29–31). TMB in TCGA was determined by the total number of nonsynonymous coding mutations.
Distributions for TMB and FGA were calculated separately for the MSK and TCGA cohorts by plotting log TMB or unnormalized FGA vs. TMB/FGA rank. Since TMB distributions are known to differ by cancer type (32), we calculated separate distributions for the different TCGA cohorts. Consistent with existing literature and clinical algorithms for immunotherapy response prediction (33, 34), these distributions were not linear but rather featured breakpoints dividing the distributions into high (hypermutators) (35) and low TMB and high, mid, and low FGA. Three breakpoints were computed for TMB, while two were computed from FGA. Because TMB was binarized, the choice between the 2nd and 3rd breakpoints was manual; the 1st breakpoint was discarded as it represented samples with virtually no mutations and so was too low to be the hypermutation breakpoint. Breakpoints were calculated using the R package Segmented (36) with the mixed-linear segmented models.
The multivariable regression models developed previously (11–13) were employed to calculate predicted 5-year survival estimates for model patients for specific TMB and FGA values.
Bulk RNA sequencing data was analyzed for the same TCGA patients described above. HTSeq files containing raw RNA counts were downloaded from the National Institutes of Health/National Cancer Institute Genomic Data Commons public database (37). Standard DESeq2 workflow (38) was employed to normalize the data and calculate differential gene expression for the TMB and FGA categories described above (i.e., high vs. low TMB and high vs. low FGA). In brief, genes were prefiltered by cancer to only include genes where at least 50% of the samples had non-zero count data. Standard differential expression using DESeq2 was carried out and log2 fold-change estimate shrinkage was carried out using the APEGLM method (39). P-values were automatically adjusted in DESeq2 for multiple testing correction. Count data was normalized using the variance-stabilized transform algorithm, which decreases the variance of counts as compared to the mean of counts for a given gene, along with outputting expression in log scale.
A list of 79 genes corresponding to target receptors (or subunits where applicable) for common anesthetic and analgesic drugs was compiled, listed in Supplementary Table S2.
Computational estimates for immune cell-type proportions for each patient sample in the TCGA cohorts were previously computed by Wang and colleagues (40) using the default LM22 immune composition expression matrices (41) in CIBERSORT (42).
Increased intraoperative opioid dose was previously found to be associated with worse overall survival (OS) in the MSK-LUAD cohort (11), while intraoperative ketamine (vs. either dexmedetomidine or no adjunct) (11) and intraoperative ketorolac were associated with higher recurrence-specific survival (RSS) (13). TMB was previously found to interact with opioid dose to modify the opioid-OS association (11), though not with ketorolac (13). Further analysis of this dataset in this work demonstrated that TMB interacted with ketamine to modify the ketamine-RSS association (see below). FGA was previously found to modify the opioid-OS association (11).
To probe these interactions further, we calculated predicted 5-year survival estimates for model patients with different TMB values, derived from the TMB distribution for MSK-LUAD cohort (Figure 1A) as explained in Figure 1B (TMB values: 1.8 (17th percentile), 3.9 (41st percentile), 10.8 (83rd percentile), and 16.7 (92nd percentile)). The 10.8 muts/mb cutoff separating hypermutators from non-hypermutators is consistent with the FDA-defined cutoff (10 muts/mb) above which patients are considered hypermutators and approved to receive pembrolizumab (20). TMB interacted with ketamine such that increased TMB [hypermutation (HM)] opposed ketamine's effect to reduce recurrence (Figure 1C, TMB = 1.8: predicted 5-year RSS = 0.94 with ketamine vs. 0.78 for no adjunct; 3.9: 0.93 vs. 0.78; 10.8: 0.87 vs. 0.78; 16.7: 0.80 vs. 0.77). TMB did not significantly interact with ketorolac, illustrated by the overlapping curves for TMB values (Figure 1D, TMB = 1.8: predicted 5-year RSS 0.81 with ketorolac vs. 0.71 without; 3.9: 0.80 vs. 0.71; 10.8: 0.79 vs. 0.70; 16.7: 0.78 vs. 0.69). TMB increase magnified the opioid-OS association such that OS further worsened at higher opioid dose (Figure 1E).
Figure 1. TMB and FGA modify anesthetic/analgesic dose to survival response curves in two cancer types: TMB distribution for the MSK-LUAD cohort (A); predicted 5-year survival estimates for model patients with derived TMB values (B) plotted for RSS for ketamine vs. no adjunct (C), RSS for ketorolac vs. no ketorolac (D), and OS vs. opioid dose (E); FGA distribution for the MSK-LUAD cohort (F); predicted 5-year OS estimates across a range of opioid doses for model patients with derived FGA values (G); MMR status distribution for the MSK-COAD cohort (H) and predicted 5-year OS estimates across a range of opioid doses for model patients based on MMR status (I). FGA, fraction genome altered; HM, hypermutator (high TMB); MME, oral morphine milligram equivalents; MMR, DNA mismatch repair (pMMR, MMR-proficient; dMMR, MMR-deficient), MSK-COAD, Memorial Sloan Kettering colon adenocarcinoma cohort; MSK-LUAD, Memorial Sloan Kettering lung adenocarcinoma cohort; OS, overall survival; RSS, recurrence-specific survival; TMB, tumor mutational burden.
For FGA, the median of each group (high, mid, and low) was selected from the distribution (Figure 1F) for further analysis; these were 0.0135 (31st percentile), 0.1442 (78th percentile) and 0.3975 (96th percentile). Like TMB, FGA increase magnified the opioid-OS association such that OS further worsened at higher opioid dose (Figure 1G). Note that the error on these predicted values increases for higher opioid dose and FGA (Supplementary Figure S1) owing to the decreasing number of patients at both higher MME and high FGA, and so the observed near 100 percent mortality at high FGA and high opioid dose should be interpreted in that context, with a focus on the trend of increasing mortality for a given opioid dose as FGA increases.
In the MSK-COAD cohort, increased intraoperative opioid dose was associated with lower risk of recurrence (12) (highlighting that oncological effects of opioids may depend on cancer type/subtype). For this analysis, a correlate of TMB, MMR status, was used to determine hypermutation high-TMB status (Figure 1H). Hypermutation (dMMR) further decreased recurrence at higher opioid dose. (Figure 1I, modified from the original figure).
Distributions for TMB and FGA by cancer type are shown for the TCGA lung adenocarcinoma cohort (TCGA-LUAD) in Figures 2A,B and for the TCGA colon adenocarcinoma cohort (TCGA-COAD) in Figures 3A,B (and for all 18 TCGA cancer types in Supplementary Figure S2, S3 for TMB and FGA respectively with breakpoints provided in Supplementary Table S3, S4 for TMB and FGA respectively). For TCGA-LUAD, the breakpoint separating high and low TMB was 23.57 muts/mb. The breakpoints separating low to mid FGA and mid to high FGA were 0.318 and 0.494, respectively. For TCGA-COAD, the TMB breakpoint was 5.9 muts/mb, while the breakpoints separating low to mid FGA and mid to high FGA were 0.004 and 0.465, respectively. Note that these values are determined from whole-exome sequencing and do not correspond exactly to those calculated from a targeted oncology panel like MSK-IMPACT [the harmonization of different panel assays and whole exome sequencing is the subject of ongoing research (43, 44)].
Figure 2. TMB and FGA modify expression of anesthetic/analgesic target receptor genes in lung adenocarcinoma: Distributions for TMB (A) and FGA (B) and differential gene expression for high vs. low TMB (C) and FGA (D) plotted for the TCGA-LUAD cohort (horizontal line denotes p = 0.05, vertical lines denote fold change = ±1 left and right, respectively). FGA, fraction genome altered; HM, hypermutator (high TMB); TCGA-LUAD, The Cancer Genome Atlas lung adenocarcinoma cohort; TMB, tumor mutational burden.
Figure 3. TMB and FGA modify expression of anesthetic/analgesic target receptor genes in colon adenocarcinoma: Distributions for TMB (A) and FGA (B) and differential gene expression for high vs. low TMB (C) and FGA (D) plotted for the TCGA-COAD cohort (horizontal line denotes p = 0.05, vertical lines denote fold change = ±1 left and right, respectively). FGA, fraction genome altered; HM, hypermutator (high TMB); TCGA-COAD, The Cancer Genome Atlas colon adenocarcinoma cohort; TMB, tumor mutational burden.
Differential gene expression for TCGA-LUAD is illustrated in the volcano plot Figure 2C for high vs. low TMB and in Figure 2D for high vs. low FGA. For the TMB differential expression, 6,469 of a total 31,521 genes (20.5%) were significantly differentially expressed, with 18 of the 59 expressed anesthetic receptor genes (30.5%) being differentially expressed. For the FGA differential expression, 16,082 total genes were differentially expressed (51.0%) with 38 of anesthetic target receptors (64.4%) being differentially expressed. Differential gene expression for TCGA-COAD is shown similarly in Figures 3C,D. For TCGA-LUAD, 12,492 of 28,711 (43.5%) total genes were found to be differentially expressed for TMB with 32 of the 58 (55.2%) expressed anesthetic receptors being differentially expressed. In the FGA comparison, 7,479 (26.0%) of all genes were differentially expressed, and 12 of the 58 (20.7%) of the anesthetic genes had differential expression. Note that while this demonstrates overrepresentation of the receptor genes, more relevant to the current analysis is that there is differential expression of these genes (and the specific genes that are differentially expressed).
Anesthetic/analgesic target receptor genes are highlighted throughout. In TCGA-LUAD, an increase in TMB or FGA generally causes a subset of same genes to be upregulated (e.g., OPRD1, GRIN2D, GRIN1, GRIN2C) and downregulated (e.g., GRIN2A, GABRP, CHRNA2), though some may switch, such as CHRNA9. However, in TCGA-COAD, most of the genes switch up- or downregulation when comparing an increase in TMB vs. FGA (e.g., GABRB3, HTR7, CHRNA7, GABRE, CHRNG, GLRA2). It should also be noted that FGA for TCGA-LUAD and TMB for TCGA-COAD showed an increase in differential expression in both the background genes, as well as the target receptor genes, as compared to TMB and FGA, respectively. As a check, differential expression between FGA medium and high was carried out for TCGA-COAD and TCGA-LUAD (Supplementary Figure S4). This showed in general less differential expression than the FGA high vs. low comparisons, and no additional genes were found to be enriched.
Dot plots showing differential target receptor gene expression for all 18 TCGA cancer types for both TMB and FGA are displayed in Figure 4. For TMB (Figure 4A), the genes that are upregulated significantly most frequently are GRIN1 (5 cancer types), CHRNA5 (4), PTGS2, HTR3A, HTR1D, GRIN3A, GRIN2D, and GRIN2B (3). Similarly, the genes that are downregulated significantly the most frequently are HTR2B, GRIN2A (7), HTR3A, GABRP, GABRG3, GABRA2, and CHRNA1 (5). With the same determination for FGA (Figure 4B), the upregulated genes are GABRE (8), OPRK1, GRIN2D, GABRA3, CHRNA5 (7), HTR3A, GRIN1, ADRA2B, and GABRQ (6), and the downregulated genes are TLR4 (12), HTR7 (11), CNRIP1 (10), PTGS1, CNR2 (9), HT2RB (8), HT2RA, and GABRP (7). When comparing pan-cancer if genes were alternatively down- and upregulated by increasing TMB or FGA, 7 TCGA cancer types were found to have at least 5 significant genes that were common between the TMB differential expression experiment and the FGA differential expression experiment. The 7 types were BRCA, COAD, GBM, LUAD, LUSC, STAD, and UCEC. For these types, GBM had 0/10 genes (0%) swap, BRCA had 6/34 (17.6%), LUAD had 3/15 (20%), LUSC had 2/5 (40%), STAD had 12/21 (57.1%), UCEC had 17/21(81.0%), and COAD had 8/8 (100%) common genes swap whether they were up- or downregulated between high/low TMB/FGA. Differential expression results for all cancer types may be found in the Supplementary Data File.
Figure 4. Pan-cancer correlation of TMB and FGA with anesthetic/analgesic target receptor gene expression: Dot plots for differential gene expression of target receptor genes (vertical axis) separated by TCGA cancer type (x-axis) for high vs. low TMB (A) and FGA (B) Greyed out dots represent non-significant differential expressions. For gene-cancer type pairs without a dot, DESeq2 did not produce a valid p-value or not enough expression of the gene was found.
Consistent with existing literature (45–47), variation in immune composition correlated with TMB and FGA. TCGA-LUAD immune composition is shown in Figures 5A,B for TMB and FGA variation, respectively, while TCGA-COAD immune composition is shown in Figures 5C,D, respectively. For hypermutated tumors (high TMB), most cancer types showed a significant increase in CD8+ T-cells and macrophages, while noting that many subtypes showed exception to this trend. For high FGA, CD8+ T-cells, macrophages (all subtypes but specifically M2 macrophages), and CD4+ memory resting T-cells are significantly decreased. One exception to this rule is TCGA-PRAD (figures and data for all cancer types can be found in Supplementary Data).
Figure 5. TME immune cell composition correlation with TMB and FGA: Computational estimates for 22 immune cell-type populations (measured by CIBERSORT) are plotted as a function of high vs. low TMB (A) and FGA (B) for TCGA-LUAD and for TCGA-COAD (C,D). Bolded x-axis labels indicate significantly different immune cell-type populations by the Kruskal-Wallis test. CIBERSORT, cell-type identification by estimating relative subsets of ribonucleic acid transcripts; FGA, fraction genome altered; TCGA-COAD, The Cancer Genome Atlas colon adenocarcinoma cohort; TCGA-LUAD, The Cancer Genome Atlas lung adenocarcinoma cohort; TMB, tumor mutational burden.
The anesthetic/analgesic target receptor genes found to be significantly correlated with TMB or FGA in TCGA-COAD and TCGA-LUAD were assessed for correlation with the 22 immune cell populations in each cancer type. Correlation was assessed using Spearman's correlation and p-values were computed using the permutation test from the R package coin (48). Comparison was done using Spearman's correlation so as to make no assumption of the linearity when analyzing the correlation of immune cell composition with the receptor genes. Data is provided in Supplementary Data File for the correlation between all significant genes and immune types with resulting p-values that were adjusted for multiple hypothesis testing within each immune cell type for each cancer type. Sample correlation plots are displayed in Figure 6. Data is shown for the expression of 3 example genes that were significantly differentially expressed for the TCGA-COAD TMB and TCGA-LUAD FGA categories (GRIN3A, OPRD1, and GRIN1). There is no clear functional significance at this time for selection of these genes; they are used to illustrate how differential expression may be affecting different immune cells in similar or different ways in various tumor types. These were compared to the presence of M2-type macrophages and CD8+ T-cells, also significantly different when comparing TCGA-COAD TMB and TCGA-LUAD FGA categories. As shown, GRIN3A is highly expressed in both TCGA-LUAD and TCGA-COAD across a wide variety of immune cells (Figures 6A–D, p < 1e-11 for all 4). However, OPRD1 shows a significant decreasing expression (p = 0.006) in TCGA-LUAD with an increase in M2 macrophages, but no change in expression in TCGA-COAD (p = 0.97). In contrast, GRIN1 shows no significant change in expression based on CD8+ T-cell level in TCGA-LUAD (p = 0.58), but an increase in expression is found with an increase in CD8+ T-cells in TCGA-COAD (p = 0.0004).
Figure 6. Immune cell-type specificity of anesthetic/analgesic target receptor genes: Correlation of expression of highlighted genes with specific immune cell types for TCGA-LUAD and TCGA-COAD [GRIN3A (A–D), OPRD1 (E–F), GRIN1 (G–H)]. Outliers on the x-axis that were greater than 2 interquartile ranges above the upper quartile were removed to allow for better visualization. These were still included in the correlation calculations. TCGA-LUAD, The Cancer Genome Atlas lung adenocarcinoma cohort; TCGA-COAD, The Cancer Genome Atlas colon adenocarcinoma cohort; FGA, fraction genome altered; HM, hypermutator (high TMB); TMB, tumor mutational burden.
Heatmaps displaying Spearman's rho for TCGA-LUAD and TCGA-COAD are shown for the significant genes for TMB and FGA in Figure 7. These plots are both clustered by cell type and gene expression. As evidenced, specific genes that may be descriptive of a broader cell type such as GRIN3A or HTR7 for macrophages are generally upregulated in samples with estimated higher macrophages, while other genes that may be differentially expressed in a cancer type due to underlying genomic events in the tumor such as hypermutation or genomic alteration can cause significant differences to appear.
Figure 7. Immune cell-type specificity of anesthetic/analgesic target receptor gene expression: Heatmaps illustrating correlations of target receptor gene expression and immune cell type in TCGA-LUAD for receptors correlated with TMB (A) and FGA (B) and in TCGA-COAD (C,D). FGA, fraction genome altered; HM, hypermutator (high TMB); TCGA-COAD, The Cancer Genome Atlas colon adenocarcinoma cohort; TCGA-LUAD, The Cancer Genome Atlas lung adenocarcinoma cohort.
The aim of this study is to begin to develop a precision approach to immunomodulation by anesthetic and analgesic drugs to affect outcomes in cancer. At a molecular level, the idea of immunomodulation translates to action on lymphocytes in the TME (i.e., TILs), and so analogy is made to immunotherapy, in particular ICI, which also act on TILs to enhance immune response against tumor cells. We reason that anesthetic/analgesic immunomodulation would be sensitive to the level of TILs in the TME just as ICI are, and would therefore correlate with tumor immunogenicity, captured by the TMB biomarker. Following the analogy made to ICI, we also explored FGA, associated with ICI resistance, even though its relation to TME immune composition is not as defined.
The first major finding of this paper is that there is in fact evidence for TMB and FGA modifying the dose-response curves for analgesics and survival: specifically, TMB and FGA modify the opioid-OS association in LUAD, TMB modifies the ketamine-RSS association in LUAD, and TMB modifies the opioid-recurrence association in COAD. This fundamentally supports the hypothesis that these drugs may have effects on recurrence and survival through immunomodulation. Further, the magnitude of this effect may depend on individual patient tumor immunogenicity and TILs infiltration.
The second major finding is that gene expression of common anesthetic/analgesic drugs correlates with TMB (and FGA) and that the pattern of this relationship (and the immune cell types correlated with receptor expression) depends on specific cancer type. Just as ICI target receptor PD-1 is expressed on TILs (and both target receptors PD-1 and PDL-1 and are themselves biomarkers of response) (49), the expression levels of various anesthetic/analgesic target receptors appear to vary with immunogenicity and may account at least partially for the observed drug interactions with TMB and FGA (Table 1). Regarding opioids, the canonical receptor OPRD1 is correlated with TMB for both LUAD and COAD, though in opposite directions (upregulated in LUAD and downregulated in COAD) (Figures 2, 3). This is consistent with TMB modification of the opioid survival/recurrence curves for both cancers as well as the difference in directionality of opioid effect (pro-tumor in LUAD, anti-tumor in COAD) (Figures 1E,I). Upregulation of OPRD1 and OPRK1 with increasing FGA (Figure 2) is consistent with FGA modification of the opioid-survival curves in LUAD (Figure 1G). Increased TMB and FGA are both correlated with upregulation of OPRD1 in LUAD, consistent with both TMB and FGA magnifying the pro-tumor opioid-survival association in LUAD (Figures 1E,G). Regarding ketamine, TMB modification of the dose-RSS curves in LUAD (Figure 1C) is consistent with its correlation with GRIN gene expression (Figure 2). By contrast, ketorolac's target receptors PTGS1 and PTGS2 are not correlated with TMB in LUAD (Figure 4), consistent with TMB not modifying the dose-survival curves (Figure 1D); in this way ketorolac can be seen as a negative control compared with the other drugs.
Table 1. Comparison of clinical (drug) and transcriptomic (receptor gene) interactions with TMB and FGA.
The results in Figure 4 are consistent with data in the literature as well. Variation of target receptor expression with cancer type is consistent with previous work looking more generally at opioid receptor genes and tumor gene expression (not specifically immune gene expression) (50). It is notable that serotonergic receptor differential expression correlates with TMB and FGA in a variety of cancers (Figure 4), considering recent evidence that these drugs may have oncological effects (51) and representation in TCGA-LIHC specifically (Figure 4) is consistent with recent clinical evidence in hepatocellular carcinoma (52).
Interestingly, high TMB and FGA modify the opioid dose to survival response in the same direction in LUAD, whereas high TMB and FGA predict opposite responses for ICI. This does not contradict the underlying hypothesis that opioid immunomodulation acts on the same TILs substrate as immunotherapy but rather highlights the possibility that the specific pathways regulated may be different for different drugs, which may be affected differently with increase in copy number alteration. Similarly, increased TMB magnifies the pro-tumor opioid-OS association in TCGA-LUAD while reducing the anti-tumor ketamine-RSS association. This likely reflects different pathways of interaction between these specific drugs and TILs, not to mention differences in direct on-tumor effects of drugs (vs. drug regulation of TILs action to affect tumor). These findings underscore that immunomodulation is nuanced, a finding consistent with recent epidemiological evidence that opioids may decrease ICI efficacy (53–56) while evincing [in the case of COAD or triple-negative breast cancer (TNBC)] anti-tumor effect.
We recently proposed a mechanism for such opioid-ICI interaction, based on analysis of gene expression and drug-induced transcriptomic signatures, suggesting that opioids and ICI regulate a common gene network in CD8+ T-cells in the TME, but in opposite directions (57). Drug interaction with ICI may depend on pathways separable from drug immunomodulation more generally with possibly opposing effects on survival. This may partially explain our findings that ketamine can be simultaneously anti-tumor (i.e., lower recurrence risk) due to direct on-tumor effects, that this may be mitigated through ketamine interaction with TILs (i.e., reducing the RSS improvement with increasing TMB), and also be neutral with respect to ICI efficacy (predicted in the mechanistic modeling of drug-ICI interaction).
Limitations of this study include the following. First, the clinical data related to opioids and ketamine derives from intraoperative dosing. While it is reasonable that these effects extend (and are perhaps even more pronounced) in chronic dosing for cancer pain, this remains speculative. Second, at present we have limited data relating drugs, outcomes, and TMB and FGA. Consequently, we have focused on opioids, ketamine, and ketorolac in analysis of the clinical data supporting TMB and FGA modification of drug-survival dose-response, while the target receptor exploration is broader. Ideally, we would have the requisite data to similarly analyze other anesthetic/analgesic agents for dose-response and TMB/FGA modification. Similarly, the clinical data is limited to two cancer types (LUAD and COAD). A third limitation is that we have employed bulk gene expression data to make inferences about immune cells specifically. Ideally, we would demonstrate these relationships in single-cell data derived from specific immune cell populations in the TME (in combination with the bulk data, which is needed for calculation of TMB and FGA). This data, however, is limited and not sufficient for pan-cancer analysis. Furthermore, it is often derived from only a few patients, whereas elucidating correlation with TMB and FGA requires larger patient samples. Future research could employ single-cell immune cell data to corroborate expression of target receptors on TILs, as we have already done for TNBC (9). Finally, while the exploratory analysis of target receptor gene expression (and correlation with TMB/FGA) suggests an underlying mechanism to explain individual patient variation in immunomodulation by anesthetic/analgesic drugs, this is beyond the scope of the present work. The possible regulation by these receptors of specific downstream oncogenic pathways is not elucidated here, nor is the relation between expression level and degree of regulation, both of which are necessary to formulate a mechanism for variation of immunomodulation by the drugs to affect outcomes. Additionally, the current exploration focuses specifically on immunomodulation, while noting that these drugs may have direct on-tumor effects as well.
Future research should aim to understand whether TMB and other markers related to immunogenicity and TILs infiltration can modify relationships between other anesthetic/analgesic drugs and outcomes and in other cancer types, whether those drugs are dosed in the intraoperative or chronic settings. Models for studying immunotherapy are well described and may be applicable here; these include syngeneic mouse tumor models, genetically engineered mouse models (GEMMs), cell line-derived xenografts (CDX), patient-derived xenografts (PDX), humanized mouse models, and tumor organoids/spheroids (58). Ultimately, prospective validation is important, which could definitively establish these markers as predictive. As the cost of acquiring omics data continues to decrease (59), its incorporation in prospective studies will become more feasible. Throughout, it is imperative to look at specific cancer types and subtypes individually, as immunogenicity fundamentally varies between cancer types (60). Our results argue strongly for a precision approach to understanding immunomodulation by anesthetic/analgesic drugs in cancer to inform future research and ultimately for application at the clinical level.
The data analyzed in this study is subject to the following licenses/restrictions: TCGA data is publicly available. Data in the MSK patient cohorts is not publicly available. Requests to access these datasets should be directed to bWluY2VyakBtc2tjYy5vcmc=.
The studies involving humans were approved by Memorial Sloan Kettering Cancer Center IRB. The studies were conducted in accordance with the local legislation and institutional requirements. Written informed consent for participation was not required from the participants or the participants' legal guardians/next of kin in accordance with the national legislation and institutional requirements.
HG: Conceptualization, Data curation, Formal Analysis, Investigation, Methodology, Software, Visualization, Writing – original draft, Writing – review & editing. KT: Formal Analysis, Investigation, Methodology, Visualization, Writing – review & editing, Data curation. GF: Conceptualization, Investigation, Methodology, Project administration, Resources, Supervision, Writing – review & editing. JM: Conceptualization, Data curation, Formal Analysis, Investigation, Methodology, Project administration, Resources, Supervision, Visualization, Writing – original draft, Writing – review & editing.
The author(s) declare financial support was received for the research, authorship, and/or publication of this article. This work was supported in part by the National Institutes of Health/National Cancer Institute Memorial Sloan Kettering Cancer Center Support Grant (P30 CA008748). HG received support from the Medical Scientist Training Program (MSTP) at the Albert Einstein College of Medicine.
JSM acknowledges Sahrena London for helpful conversations.
The authors declare that the research was conducted in the absence of any commercial or financial relationships that could be construed as a potential conflict of interest.
All claims expressed in this article are solely those of the authors and do not necessarily represent those of their affiliated organizations, or those of the publisher, the editors and the reviewers. Any product that may be evaluated in this article, or claim that may be made by its manufacturer, is not guaranteed or endorsed by the publisher.
The Supplementary Material for this article can be found online at: https://www.frontiersin.org/articles/10.3389/fanes.2024.1464004/full#supplementary-material
1. Murphy O, Forget P, Ma D, Buggy DJ. Tumour excisional surgery, anaesthetic-analgesic techniques, and oncologic outcomes: a narrative review. Br J Anaesth. (2023) 131(6):989–1001. doi: 10.1016/j.bja.2023.07.027
2. Shapiro J, Jersky J, Katzav S, Feldman M, Segal S. Anesthetic drugs accelerate the progression of postoperative metastases of mouse tumors. J Clin Invest. (1981) 68(3):678–85. doi: 10.1172/JCI110303
3. Mincer JS, Buggy DJ. Anaesthesia, analgesia, and cancer outcomes: time to think like oncologists? Br J Anaesth. (2023) 131(2):193–6. doi: 10.1016/j.bja.2023.02.001
4. Pedoto A, Fischer GW, Mincer JS. The current (and possible future) role of opioid analgesia in lung cancer surgery. Best Pract Res Clin Anaesthesiol. (2024) 38(1):74–80. doi: 10.1016/j.bpa.2024.05.001
5. Wahida A, Buschhorn L, Fröhling S, Jost PJ, Schneeweiss A, Lichter P, et al. The coming decade in precision oncology: six riddles. Nat Rev Cancer. (2023) 23(1):43–54. doi: 10.1038/s41568-022-00529-3
6. Smith L, Cata JP, Forget P. Immunological insights into opioid-free anaesthesia in oncological surgery: a scoping review. Curr Oncol Rep. (2022) 24(10):1327–36. doi: 10.1007/s11912-022-01300-5
7. Bettinger JJ, Friedman BC. Opioids and immunosuppression: clinical evidence, mechanisms of action, and potential therapies. Palliat Med Rep. (2024) 5(1):70–80. doi: 10.1089/pmr.2023.0049
8. Silagy AW, Hannum ML, Mano R, Attalla K, Scarpa JR, DiNatale RG, et al. Impact of intraoperative opioid and adjunct analgesic use on renal cell carcinoma recurrence: role for onco-anaesthesia. Br J Anaesth. (2020) 125(5):e402–e4. doi: 10.1016/j.bja.2020.06.036
9. Montagna G, Gupta HV, Hannum M, Tan KS, Lee J, Scarpa JR, et al. Intraoperative opioids are associated with improved recurrence-free survival in triple-negative breast cancer. Br J Anaesth. (2021) 126(2):367–76. doi: 10.1016/j.bja.2020.10.021
10. Scarpa JR, DiNatale RG, Mano R, Silagy AW, Kuo F, Irie T, et al. Identifying clear cell renal cell carcinoma coexpression networks associated with opioid signaling and survival. Cancer Res. (2021) 81(4):1101–10. doi: 10.1158/0008-5472.CAN-20-1852
11. Connolly JG, Tan KS, Mastrogiacomo B, Dycoco J, Caso R, Jones GD, et al. Intraoperative opioid exposure, tumour genomic alterations, and survival differences in people with lung adenocarcinoma. Br J Anaesth. (2021) 127(1):75–84. doi: 10.1016/j.bja.2021.03.030
12. Yuval JB, Lee J, Wu F, Thompson HM, Verheij FS, Gupta HV, et al. Intraoperative opioids are associated with decreased recurrence rates in colon adenocarcinoma: a retrospective observational cohort study. Br J Anaesth. (2022) 129(2):172–81. doi: 10.1016/j.bja.2022.04.024
13. Connolly JG, Scarpa JR, Gupta HV, Tan KS, Mastrogiacomo B, Dycoco J, et al. Intraoperative ketorolac may interact with patient-specific tumour genomics to modify recurrence risk in lung adenocarcinoma: an exploratory analysis. Br J Anaesth. (2021) 127(3):e82–e5. doi: 10.1016/j.bja.2021.05.032
14. Butterfield LH, Najjar YG. Immunotherapy combination approaches: mechanisms, biomarkers and clinical observations. Nat Rev Immunol. (2024) 24(6):399–416. doi: 10.1038/s41577-023-00973-8
15. Blankenstein T, Coulie PG, Gilboa E, Jaffee EM. The determinants of tumour immunogenicity. Nat Rev Cancer. (2012) 12(4):307–13. doi: 10.1038/nrc3246
16. Sha D, Jin Z, Budczies J, Kluck K, Stenzinger A, Sinicrope FA. Tumor mutational burden as a predictive biomarker in solid tumors. Cancer Discov. (2020) 10(12):1808–25. doi: 10.1158/2159-8290.CD-20-0522
17. Xie N, Shen G, Gao W, Huang Z, Huang C, Fu L. Neoantigens: promising targets for cancer therapy. Signal Transduct Target Ther. (2023) 8(1):9. doi: 10.1038/s41392-022-01270-x
18. Wang X, Lamberti G, Di Federico A, Alessi J, Ferrara R, Sholl ML, et al. Tumor mutational burden for the prediction of PD-(L)1 blockade efficacy in cancer: challenges and opportunities. Ann Oncol. (2024) 35(6):508–22. doi: 10.1016/j.annonc.2024.03.007
19. Samstein RM, Lee CH, Shoushtari AN, Hellmann MD, Shen R, Janjigian YY, et al. Tumor mutational load predicts survival after immunotherapy across multiple cancer types. Nat Genet. (2019) 51(2):202–6. doi: 10.1038/s41588-018-0312-8
20. Marcus L, Fashoyin-Aje LA, Donoghue M, Yuan M, Rodriguez L, Gallagher PS, et al. FDA approval summary: pembrolizumab for the treatment of tumor mutational burden-high solid tumors. Clin Cancer Res. (2021) 27(17):4685–9. doi: 10.1158/1078-0432.CCR-21-0327
21. Jardim DL, Goodman A, de Melo Gagliato D, Kurzrock R. The challenges of tumor mutational burden as an immunotherapy biomarker. Cancer Cell. (2021) 39(2):154–73. doi: 10.1016/j.ccell.2020.10.001
22. Chang TG, Cao Y, Shulman ED, Ben-David U, Schäffer AA, Ruppin E. Optimizing cancer immunotherapy response prediction by tumor aneuploidy score and fraction of copy number alterations. NPJ Precis Oncol. (2023) 7(1):54. doi: 10.1038/s41698-023-00408-6
23. Rizvi H, Sanchez-Vega F, La K, Chatila W, Jonsson P, Halpenny D, et al. Molecular determinants of response to anti-programmed cell death (PD)-1 and anti-programmed death-ligand 1 (PD-L1) blockade in patients with non-small-cell lung cancer profiled with targeted next-generation sequencing. J Clin Oncol. (2018) 36(7):633–41. doi: 10.1200/JCO.2017.75.3384
24. Asleh K, Ouellette RJ. Tumor copy number alteration burden as a predictor for resistance to immune checkpoint blockade across different cancer types. Cancers (Basel). (2024) 16(4):732. doi: 10.3390/cancers16040732
25. Cheng DT, Mitchell TN, Zehir A, Shah RH, Benayed R, Syed A, et al. Memorial sloan kettering-integrated mutation profiling of actionable cancer targets (MSK-IMPACT). J Mol Diagn. (2015) 17(3):251–64. doi: 10.1016/j.jmoldx.2014.12.006
26. Vilar E, Gruber SB. Microsatellite instability in colorectal cancer—the stable evidence. Nat Rev Clin Oncol. (2010) 7(3):153–62. doi: 10.1038/nrclinonc.2009.237
27. Kang YJ, O'Haire S, Franchini F, IJzerman M, Zalcberg J, Macrae F, et al. A scoping review and meta-analysis on the prevalence of pan-tumour biomarkers (dMMR, MSI, high TMB) in different solid tumours. Sci Rep. (2022) 12(1):20495. doi: 10.1038/s41598-022-23319-1
28. Zhou Z, Xie X, Wang X, Zhang X, Li W, Sun T, et al. Correlations between tumor mutation burden and immunocyte infiltration and their prognostic value in colon cancer. Front Genet. (2021) 12:623424. doi: 10.3389/fgene.2021.623424
29. Cerami E, Gao J, Dogrusoz U, Gross BE, Sumer SO, Aksoy BA, et al. The cBio cancer genomics portal: an open platform for exploring multidimensional cancer genomics data. Cancer Discov. (2012) 2(5):401–4. doi: 10.1158/2159-8290.CD-12-0095
30. de Bruijn I, Kundra R, Mastrogiacomo B, Tran TN, Sikina L, Mazor T, et al. Analysis and visualization of longitudinal genomic and clinical data from the AACR project GENIE biopharma collaborative in cBioPortal. Cancer Res. (2023) 83(23):3861–7. doi: 10.1158/0008-5472.CAN-23-0816
31. Gao J, Aksoy BA, Dogrusoz U, Dresdner G, Gross B, Sumer SO, et al. Integrative analysis of complex cancer genomics and clinical profiles using the cBioPortal. Sci Signal. (2013) 6(269):l1. doi: 10.1126/scisignal.2004088
32. McGrail DJ, Pilié PG, Rashid NU, Voorwerk L, Slagter M, Kok M, et al. High tumor mutation burden fails to predict immune checkpoint blockade response across all cancer types. Ann Oncol. (2021) 32(5):661–72. doi: 10.1016/j.annonc.2021.02.006
33. Sholl LM, Hirsch FR, Hwang D, Botling J, Lopez-Rios F, Bubendorf L, et al. The promises and challenges of tumor mutation burden as an immunotherapy biomarker: a perspective from the international association for the study of lung cancer pathology committee. J Thorac Oncol. (2020) 15(9):1409–24. doi: 10.1016/j.jtho.2020.05.019
34. Li W, Zhao Y, Zhang H, Zheng W, Wang R, Gu X. Predictive value of tumor mutational burden for PD-1/PD-L1 inhibitors in NSCLC: a meta-analysis. Medicine (Baltimore). (2023) 102(40):e34990. doi: 10.1097/MD.0000000000034990
35. Campbell BB, Light N, Fabrizio D, Zatzman M, Fuligni F, de Borja R, et al. Comprehensive analysis of hypermutation in human cancer. Cell. (2017) 171(5):1042–1056.e10. doi: 10.1016/j.cell.2017.09.048
36. Muggeo VM. Estimating regression models with unknown break-points. Stat Med. (2003) 22(19):3055–71. doi: 10.1002/sim.1545
37. Grossman RL, Heath AP, Ferretti V, Varmus HE, Lowy DR, Kibbe WA, et al. Toward a shared vision for cancer genomic data. N Engl J Med. (2016) 375(12):1109–12. doi: 10.1056/NEJMp1607591
38. Love MI, Huber W, Anders S. Moderated estimation of fold change and dispersion for RNA-Seq data with DESeq2. Genome Biol. (2014) 15(12):550. doi: 10.1186/s13059-014-0550-8
39. Zhu A, Ibrahim JG, Love MI. Heavy-tailed prior distributions for sequence count data: removing the noise and preserving large differences. Bioinformatics. (2019) 35(12):2084–92. doi: 10.1093/bioinformatics/bty895
40. Wang X, Chen L, Liu W, Zhang Y, Liu D, Zhou C, et al. TIMEDB: tumor immune micro-environment cell composition database with automatic analysis and interactive visualization. Nucleic Acids Res. (2023) 51(D1):D1417–D24. doi: 10.1093/nar/gkac1006
41. Chen B, Khodadoust MS, Liu CL, Newman AM, Alizadeh AA. Methods in molecular biology. Methods Mol Biol. (2018) 1711:243–59. doi: 10.1007/978-1-4939-7493-1_12
42. Newman AM, Liu CL, Green MR, Gentles AJ, Feng W, Xu Y, et al. Robust enumeration of cell subsets from tissue expression profiles. Nat Methods. (2015) 12(5):453–7. doi: 10.1038/nmeth.3337
43. Vega DM, Yee LM, McShane LM, Williams PM, Chen L, Vilimas T, et al. Aligning tumor mutational burden (TMB) quantification across diagnostic platforms: phase II of the friends of cancer research TMB harmonization project. Ann Oncol. (2021) 32(12):1626–36. doi: 10.1016/j.annonc.2021.09.016
44. Li Y, Luo Y. Optimizing the evaluation of gene-targeted panels for tumor mutational burden estimation. Sci Rep. (2021) 11(1):21072. doi: 10.1038/s41598-021-00626-7
45. Xie F, Bai Y, Yang X, Long J, Mao J, Lin J, et al. Comprehensive analysis of tumour mutation burden and the immune microenvironment in hepatocellular carcinoma. Int Immunopharmacol. (2020) 89(Pt A):107135. doi: 10.1016/j.intimp.2020.107135
46. Wang X, Li M. Correlate tumor mutation burden with immune signatures in human cancers. BMC Immunol. (2019) 20(1):4. doi: 10.1186/s12865-018-0285-5
47. Chen H, Zhang T, Zhang Y, Wu H, Fang Z, Liu Y, et al. Deciphering the tumor microenvironment cell-infiltrating landscape reveals microenvironment subtypes and therapeutic potentials for nonsquamous NSCLC. JCI Insight. (2022) 7(12):e152815. doi: 10.1172/jci.insight.152815
48. Hothorn T, Hornik K, van de Wiel MA, Zeileis A. A lego system for conditional inference. Am Stat. (2006) 60(3):257–63. doi: 10.1198/000313006X118430
49. Wojtukiewicz MZ, Rek MM, Karpowicz K, Górska M, Polityńska B, Wojtukiewicz AM, et al. Inhibitors of immune checkpoints-PD-1, PD-L1, CTLA-4-new opportunities for cancer patients and a new challenge for internists and general practitioners. Cancer Metastasis Rev. (2021) 40(3):949–82. doi: 10.1007/s10555-021-09976-0
50. Belltall A, Zúñiga-Trejos S, Garrido-Cano I, Eroles P, Argente-Navarro MP, Buggy DJ, et al. Solid tumor opioid receptor expression and oncologic outcomes: analysis of the cancer genome atlas and genotype tissue expression project. Front Oncol. (2022) 12:801411. doi: 10.3389/fonc.2022.801411
51. Zheng Y, Chang X, Huang Y, He D. The application of antidepressant drugs in cancer treatment. Biomed Pharmacother. (2023) 157:113985. doi: 10.1016/j.biopha.2022.113985
52. Huang KL, Chen YL, Stewart R, Chen VC. Antidepressant use and mortality among patients with hepatocellular carcinoma. JAMA Netw Open. (2023) 6(9):e2332579. doi: 10.1001/jamanetworkopen.2023.32579
53. Botticelli A, Cirillo A, Pomati G, Cerbelli B, Scagnoli S, Roberto M, et al. The role of opioids in cancer response to immunotherapy. J Transl Med. (2021) 19(1):119. doi: 10.1186/s12967-021-02784-8
54. Miura K, Sano Y, Niho S, Kawasumi K, Mochizuki N, Yoh K, et al. Impact of concomitant medication on clinical outcomes in patients with advanced non-small cell lung cancer treated with immune checkpoint inhibitors: a retrospective study. Thorac Cancer. (2021) 12(13):1983–94. doi: 10.1111/1759-7714.14001
55. Ju M, Gao Z, Liu X, Zhou H, Wang R, Zheng C, et al. The negative impact of opioids on cancer patients treated with immune checkpoint inhibitors: a systematic review and meta-analysis. J Cancer Res Clin Oncol. (2023) 149(6):2699–708. doi: 10.1007/s00432-022-04513-0
56. Mao Z, Jia X, Jiang P, Wang Q, Zhang Y, Li Y, et al. Effect of concomitant use of analgesics on prognosis in patients treated with immune checkpoint inhibitors: a systematic review and meta-analysis. Front Immunol. (2022) 13:861723. doi: 10.3389/fimmu.2022.861723
57. Scarpa JR, Montagna G, Plitas G, Gulati A, Fischer GW, Mincer JS. Opioids and immune checkpoint inhibitors differentially regulate a common immune network in triple-negative breast cancer. Front Oncol. (2023) 13:1267532. doi: 10.3389/fonc.2023.1267532
58. Franklin MR, Platero S, Saini KS, Curigliano G, Anderson S. Immuno-oncology trends: preclinical models, biomarkers, and clinical development. J Immunother Cancer. (2022) 10(1):e003231. doi: 10.1136/jitc-2021-003231
59. Cuppen E, Elemento O, Rosenquist R, Nikic S, IJzerman M, Zaleski ID, et al. Implementation of whole-genome and transcriptome sequencing into clinical cancer care. JCO Precis Oncol. (2022) 6:e2200245. doi: 10.1200/PO.22.00245
Keywords: anesthesia, analgesia, opioids, ketamine, ketorolac, immunomodulation, immunotherapy, precision medicine & genomics
Citation: Gupta HV, Tan KS, Fischer GW and Mincer JS (2024) Towards a precision approach to anesthetic/analgesic immunomodulation in cancer. Front. Anesthesiol. 3:1464004. doi: 10.3389/fanes.2024.1464004
Received: 12 July 2024; Accepted: 20 November 2024;
Published: 5 December 2024.
Edited by:
Zong Sheng Guo, University at Buffalo, United StatesReviewed by:
Nicholas Perry, Institute of Cancer Research (ICR), United KingdomCopyright: © 2024 Gupta, Tan, Fischer and Mincer. This is an open-access article distributed under the terms of the Creative Commons Attribution License (CC BY). The use, distribution or reproduction in other forums is permitted, provided the original author(s) and the copyright owner(s) are credited and that the original publication in this journal is cited, in accordance with accepted academic practice. No use, distribution or reproduction is permitted which does not comply with these terms.
*Correspondence: Joshua S. Mincer, bWluY2VyakBtc2tjYy5vcmc=
Disclaimer: All claims expressed in this article are solely those of the authors and do not necessarily represent those of their affiliated organizations, or those of the publisher, the editors and the reviewers. Any product that may be evaluated in this article or claim that may be made by its manufacturer is not guaranteed or endorsed by the publisher.
Research integrity at Frontiers
Learn more about the work of our research integrity team to safeguard the quality of each article we publish.