- 1Centro de Bioinformática y Biología Integrativa, Facultad de Ciencias de la Vida, Universidad Andrés Bello, Santiago, Chile
- 2Facultad de Enfermería, Universidad Andrés Bello, Santiago, Chile
- 3Computational Biology Institute, Department of Biostatistics and Bioinformatics, The George Washington University, Washington, DC, United States
- 4Departamento de Microbiología, Facultad de Ciencias de la Salud, Universidad de Talca, Talca, Chile
- 5Centro de Ecología Integrativa, Universidad de Talca, Talca, Chile
Around 155 million people worldwide suffer from asthma. In Chile, the prevalence of this disease in children is around 15% and has a high impact in the health system. Studies suggest that asthma is caused by multiple factors, including host genetics, antibiotic use, and the development of the airway microbiota. Here, we used 16S rRNA high-throughput sequencing to characterize the nasal and oral mucosae of 63 asthmatic and 89 healthy children (152 samples) from Santiago, Chile. We found that the nasal mucosa was dominated by a high abundance of Moraxella, Dolosigranulum, Haemophilus, Corynebacterium, Streptococcus, and Staphylococcus. In turn, the oral mucosa was characterized by a high abundance of Streptococcus, Haemophilus, Gemella, Veillonella, Neisseria, and Porphyromonas. Our results showed significantly (P < 0.001) lower alpha diversity and an over-abundance of Streptococcus (P < 0.01) in nasal samples from asthmatics compared to samples from healthy subjects. Community structure, as revealed by co-occurrence networks, showed different microbial interactions in asthmatic and healthy subjects, particularly in the nasal microbiota. The networks revealed keystone genera in each body site, including Prevotella, Leptotrichia, and Porphyromonas in the nasal microbiota, and Streptococcus, Granulicatella, and Veillonella in the oral microbiota. We also detected 51 functional pathways differentially abundant on the nasal mucosa of asthmatic subjects, although only 13 pathways were overrepresented in the asthmatic subjects (P < 0.05). We did not find any significant differences in microbial taxonomic (composition and structure) and functional diversity between the oral mucosa of asthmatic and healthy subjects. This study explores for the first time the relationships between the upper respiratory airways bacteriome and asthma in Chile. It demonstrates that the nasal cavity of children from Santiago harbors unique bacterial communities and identifies potential taxonomic and functional biomarkers of pediatric asthma.
Introduction
The World Health Organization estimates that around 250,000 people die each year globally due to asthma and that its prevalence will increase to 100 million people by 2025 (1). Asthma affects all age ranges, but it is a common chronic disease in children and adolescents (2–4); being their most common chronic respiratory condition worldwide, with around 14% of children and young people affected (4). In Chile, the prevalence of pediatric asthma is 15.5% (5, 6) and has a high economic impact on the health system with approximately 15 million dollars spent in treatment per year (7).
Many factors are involved in the onset and incidence of asthma, including host genetics (8), ethnic components (9), degrees of urbanization (10), gender (11), and environmental factors, e.g., dust exposure (12), living in a farm-like environment (13), antibiotic use during infancy (14), and mode of delivery (15). All of the aforementioned factors also contribute to the microbiota establishment, where an imbalance of the microbiota can lead to disease (16–19).
Several studies have described a relationship between the airway microbiota and the development of asthma, the vast majority revealing an imbalance or dysbiosis (17, 20–23). Some of those studies have also highlighted that the most predominant bacterial genera in the respiratory tract of asthmatic patients are Moraxella, Haemophilus, and Streptococcus, with Moraxella catarrhalis, Haemophilus influenzae, and Streptococcus pneumoniae being dominant in children with asthma (20, 24–28). While many of those studies have focused on the dysbiosis of healthy subjects compared to asthmatics and concurred that this dysbiosis is related to Moraxella, Haemophilus, and Streptococcus, fewer studies have explored changes in microbial interactions (29, 30).
The nostrils are a repository and point of entry of multiple pathogens to the lower respiratory tract (31). However, the oral cavity is the initial interface between allergens, microbiome, and mucosal immunity. The anatomical connection between the oral cavity and the lungs provides many opportunities for the oral microbiota to affect the lung microbiota in different situations (32–34). In fact, recent studies have found differences in the dental microbiota of subjects with asthma and atopy compared to healthy subjects (35). However, studies are still limited and often include few subjects.
Current studies on respiratory airway microbiota have predominantly focused on populations from the northern hemisphere, including the USA (26), Taiwan (16), China (36) and Portugal (30). However, some evidence indicates that the microbiota is affected by factors such as ethnicity, diet, and geographic zone (37, 38). Consequently, the relationships between certain bacteria and asthma in northern populations might not hold for other populations around the world. For instance, the Human Microbiome Project showed that bacterial taxa found in healthy US subjects are not universal, neither in the body sites studied nor in the subjects (39). In Ecuador, for example, members of the genus Streptococcus are detected in oropharyngeal samples in higher abundances than in Europe or the USA, although the implications of this are not yet fully understood (40). In part this has prompted the rise of microbiome centers with a regional component such the American Gut (41) and MetaHIT Consortium (42). Geographic variation is also true for environmental microbiomes as demonstrated for example by the MetaSUB Consortium (43). Studies related to asthma in Chile are limited to socioeconomic factors (44) and prevalence (6, 45), are clinically descriptive (46), and address treatments (47) related to the disease. Therefore, the diversity of the airway microbiota in healthy and asthmatic Chileans is still unknown.
In this study, we assessed whether the oral and nasal microbiota vary in composition, structure, and function between healthy and asthmatic children from Santiago de Chile. Moreover, we explored microbial interactions in both groups to identify relevant (keystone) taxa in the structure of each microbiota. Toward these goals, we characterized the nasal and oral microbiota of 152 subjects using 16S rRNA gene amplicon sequencing and compared their taxonomic and functional diversity.
Materials and methods
Study population and sample collection
All study participants were volunteers. Swab samples were collected at the Amador Neghme primary health center in Santiago, Chile. Written consent was obtained from parents or legal guardians of the volunteers before collecting samples. The study and consent documents were approved by the Ethics Committee of “Servicio de Salud Metropolitano Sur”.
Healthy and asthmatic children from the Santiago area were enrolled in the fall and winter of 2016, 2017 and 2018 to participate in this study. Asthmatics were diagnosed according to AUGE diagnostic clinical guidelines of the Ministry of Health, Chile for children under 15 years of age, which in turn are based on The Agency for Healthcare Research and Quality (48). In brief, the evaluation criteria are a clinical history of the disease, difficulty in breathing, spirometry evaluation with bronchodilator response, and increased forced expiratory volume (equal to or greater than 12% after receiving the application of 400 µg of salbutamol after 15 min). Patients who did not present these characteristics or presented any of the exclusion criteria (see Supplementary File S1) were not eligible for the study.
Swab samples were taken from both the nostrils and oral cavity of asthmatic patients and healthy subjects following and adapting the sample extraction protocol of the Human Microbiome Project (HMP) (49). Sterile swabs were rubbed against the walls of the mouth (∼4 cm2) or both nostrils for 20 to 40 s to ensure transfer of microorganisms to the TD1 buffer solution of the Ultra Clean Tissue and Cell kit (MoBio Laboratories). Swabs were immersed in 700 µl of TD1 buffer (UltraClean Cell and Tissue DNA Isolation Kit) and stored at −20°C until the DNA extraction procedure. Oral samples from individuals with “chronic dry mouth”, periodontal lesions, oral abscesses, or evidence of candidiasis were discarded. Similarly, only nasal samples from individuals vaccinated with a live attenuated influenza vaccine administered through the nose at least 28 days ago, no signs of inflammation, polyps or masses, and no infection of the nasal cavity and upper respiratory tract were considered. The healthy subjects enrolled in the study met the following criteria: not presenting any disorder or disease of the upper or lower respiratory tract, not having active antibiotic or antibiotic treatment for 2 months prior to the sampling and not presenting any exclusion criteria from the study (Supplementary File S1).
DNA extraction and sequencing
Total DNA was extracted using the UltraClean Tissue & Cells DNA Isolation Kit (Cat No. 12334-S, MO BIO Laboratories, Inc.). Samples were homogenized on a horizontal Vortex Adapter (Catalog #13000-V1, MO BIO Laboratories, Inc.) following the manufacturer’s instructions. The concentration of DNA was quantified by a Qubit® 3.0 Fluorometer (Invitrogen), using a Qubit dsDNA HS Assay Kit (Cat N° Q32854). Each DNA sample was amplified for the V4 region of the 16S rRNA gene and libraries were prepared and sequenced using the Schloss MiSeq_WetLab_SOP protocol (50). Twenty nasal and oral samples were sequenced at The Microbial Systems Molecular Biology Laboratory (MSMBL) sequencing group (University of Michigan, Ann Arbor, MI, USA), while 160 samples (nasal and oral mucosa) were sequenced at The Environmental Sample Preparation and Sequencing Facility at Argonne National Laboratory (Lemont, IL, USA). The sequencing facilities used in this study used negative and positive controls in their protocols. Positive controls (Zymo microbiomics) were verified, though were not formally included in the analysis. After quality control and filtering 28 samples were discarded and 152 samples were analyzed. All sequence data was deposited in the NCBI under Bioproject accession number PRJNA446042. All R code and metadata are available in GitHub (https://github.com/ramostapiai/16s-Analysis).
Microbiota analysis
Forward and reverse reads were trimmed at 150 bp to maintain quality over PHRED 25 and filtered using the following parameters: maxN = 0, maxEE = c(2,2), truncQ = 0, rm.phix = TRUE. Error rate learning, dereplication, and read merging were performed using default settings. Taxonomy was assigned using the Silva database for the16S rRNA gene (51) (version 132). A multiple sequence alignment was carried out using the R DECIPHER package (52) (version 2.7.3) and the phylogenetic tree was inferred using FastTree (53) (version 2.1.10). We created a phyloseq object for subsequent microbial analyses using the Phyloseq package (version 1.23.1). Sixteen-S rRNA–V4 amplicon sequence variants (ASV) in each sample were inferred using DADA2 version 1.18 (54). Following the author’s recommendations, we discarded samples of <1,000 reads, uncharacterized (NA) phylum-level taxa, ASVs with an average relative abundance <1 × −5 and ASVs that were not observed more than 2 times in at least 10% of the samples. To avoid batch effects, the sequence files coming from each sequencing facility were processed separately using DADA2 under default parameters (54). We then combined the inferred ASVs and generated a single phyloseq object according to the author’s recommendations (55). We normalized our samples using the negative binomial distribution as recommended by McMurdie and Holmes (56) as implemented in the Bioconductor package DESeq2 (57).
Taxonomic alpha diversity (Chao1, ACE and Shannon) was estimated in R using the phyloseq function estimate_richness (Phyloseq version 1.23.1). Phylogenetic diversity was estimated with estimate_pd (btools package of R version 0.0.1). Statistical differences between groups were assessed using Linear Mixed Effects (LME) model as implemented in the lme4 R package (v1.1–21) (58). In our LME model we included alpha diversity indices and taxa (phyla and genera) abundances (response variables) and health status (healthy or asthmatic; predictors), while accounting for non-independence of subjects (random effects). We included random effects in our LME models since some participants were sampled twice during the study. We also considered the potential contribution of clinical characteristics of the cohort on the composition of the microbiota. Therefore, other covariables were also initially included in our LME analyses (sample collection date, use of drugs, age, condition of the host, and gender). We also included “sequencing facility” to further account for potential batch effects. To avoid redundancy, we did not include three variables (use of drugs, age, and gender) used to predict health status (healthy or asthmatic). We also tested LME models with random intercepts and random slopes and different orders of factors. Initial LME models including the variables listed above were compared using the function lmerTest, which performs automatic backward elimination of factors. ANOVA type III tests with Satterthwaite approximation for degrees of freedom were also carried out for hypothesis testing.
Beta diversity was estimated using weighted and unweighted Unifrac distances. Dissimilarity between samples was explored using Principal Coordinates Analysis (PCoA). Indices were compared using permutational multivariate analysis of variance (adonis2) as implemented in the vegan R package version 2.5–6 (59). Models were compared using the Akaike Index Criterion (60) and significance was determined through 10,000 permutations. We also included “sequencing facility” as a factor in the permutational analysis to account for potential batch effects (R2 < 0.02).
Community interactions among bacterial taxa were inferred using the network approach implemented in the SPIEC-EASI R package version 1.0.5 (SParse InversE Covariance Estimation for Ecological Association Inference) (61) under the neighborhood selection (mb) model. Keystone species at the ASV level (hub nodes) were calculated using the node degree and node centrality metrics (Degree >5 and Betweenness >200). The degree value of a node represents the number of edges connected to the node. Betweenness is also a measure of centrality of the nodes that make up the network. The betweenness value of a node is calculated as the total number of shortest paths from all nodes to all other nodes that pass through the node in question.
Microbial functional signatures (i.e., metabolic pathways) based on 16S rRNA gene sequences were predicted using Phylogenetic Investigation of Communities by Reconstruction of Unobserved States (PICRUSt2) version 2.2.0-b (62). Differentially represented pathways were calculated via the Welch’s t-test (63) (CI = 0.95) with Benjamini-Hochberg correction-FDR (64) as implemented in the STAMP software (65).
Visualizations of alpha and beta diversity indices, microbial relative abundances, and co-occurrence networks were carried out in RStudio (version 1.2.1335) and R (version 3.6.1).
Results
Microbiome composition and diversity
After filtering and quality control, 152 samples (69 oral and 83 nasal) were obtained (Table 1 and Supplementary Table S1), which included a total of 4,921,605 sequences (mean = 27,001, median = 23,430), ranging from 2,023 to 91,604 sequences per sample and 120 ASV.
Nasal microbiomes included sequences corresponding to four dominant phyla (>1%): Firmicutes (41%), Proteobacteria (41.8%), Actinobacteria (15.5%) and Bacteroidetes (1.3%). These phyla comprise 7 dominant genera (>1%) genera: Moraxella (24.1%), Dolosigranulum (17.4%), Corynebacterium_1 (13.9%), Streptococcus (10.8%), Staphylococcus (10.5%), Haemophilus (9.8%) and Veillonella (1%). On the other hand, the oral microbiome included sequences that corresponded to five dominant phyla (>1%): Firmicutes (53.2%), Proteobacteria (31.4%), Bacteroidetes (9%), Actinobacteria (3.1%) and Fusobacteria (3.1%). Those phyla comprised 7 dominant (>1%) genera: Streptococcus (35.7%), Haemophilus (16.5%), Gemella (7.4%), Veillonella (6.5%), Neisseria (5.5%), Porphyromonas (3.9%) and Moraxella (1.2%) (Table 2, Supplementary Figures S1, S2).
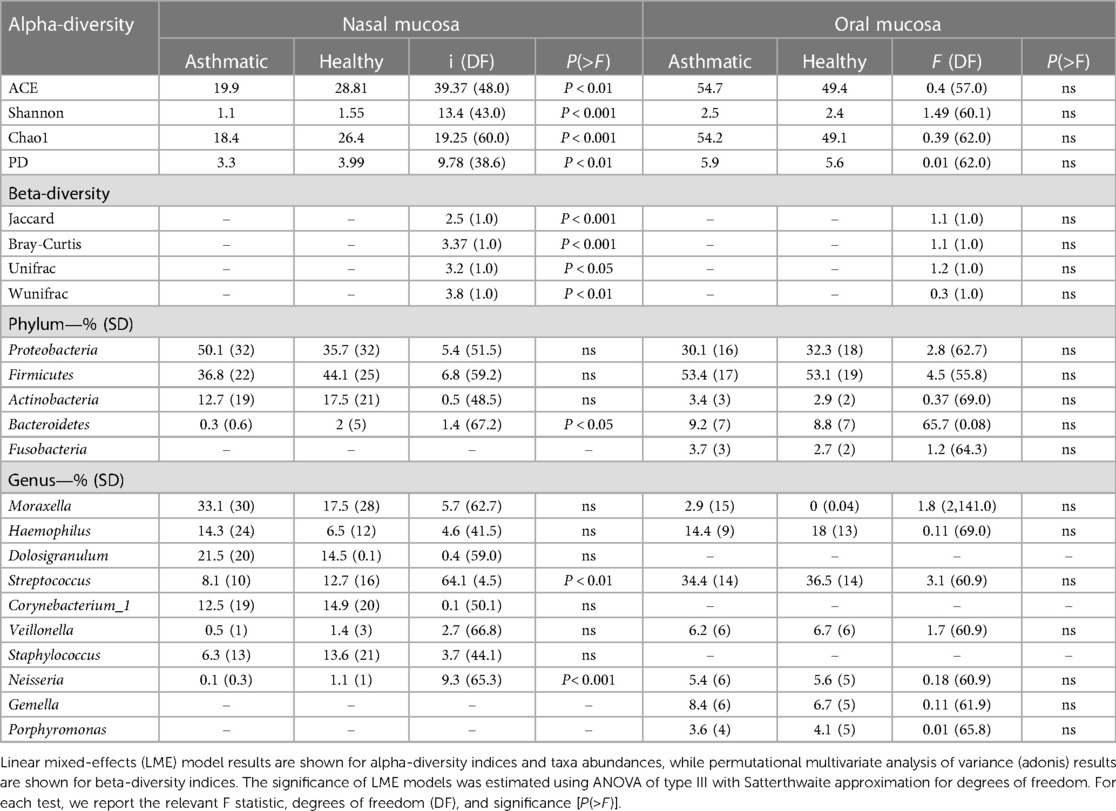
Table 2. Mean alpha-diversity indices, beta diversity indices, and mean relative abundance of dominant phyla and genera (>1%) of nasal and oral samples.
The nasal and oral microbiomes differ greatly in species turnover as indicated by the PCoA of weighted Unifrac distances and confirmed by the Permutational Multivariate Analysis of Variance (PERMANOVA) (P = 9.999 × −5, R2 = 0.26966). While the oral mucosa samples are similar to each other, the nasal microbiome exhibits a wide dispersion among samples, which indicates that its composition, phylogenetic, and taxonomic structures are more variable (Figure 1). Likewise, we did not find any clear patterns structuring oral microbiomes regarding their disease status, i.e., microbial communities coming from asthmatic patients did not seem to differ substantially in species turnover from healthy individuals (Supplementary Figure S3).
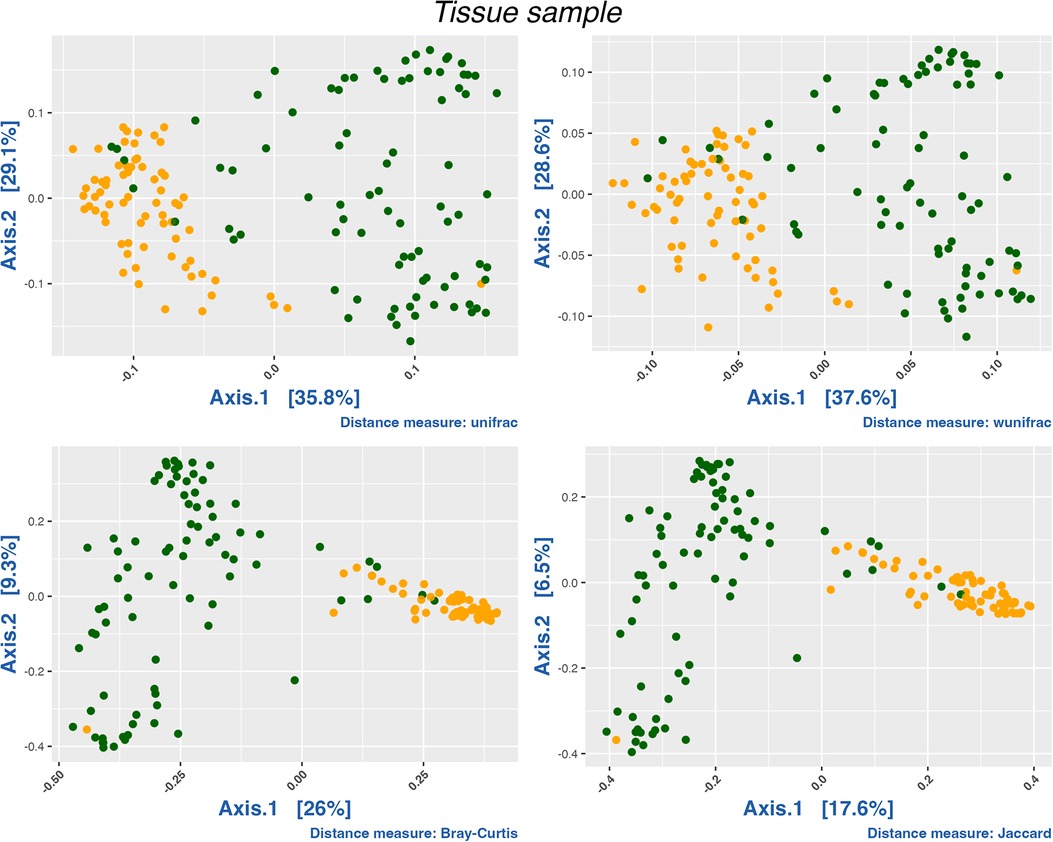
Figure 1. PCoA analysis of all samples by compartment. Oral samples in orange and nasal samples in green. We conducted a PERMANOVA test using distance matrices (Jaccard, Bray-Curtis, Unifrac and Wunifrac; P < 0.0001). R2 for Jaccard (0.14097), Bray-Curtis (0.21004), Unifrac (0.26966) and wUnifrac (0.20951).
The microbiota of asthmatic and healthy children in both the nasal and oral compartments shared many bacterial genera, but differed in their relative proportions, especially in the nasal compartment. Our LME analyses of nasal samples from healthy and asthmatic subjects showed significant differences in the phylum Bacteroidetes (P = 0.03787), and the genera Streptococcus (P = 0.011443) and Neisseria (P = 0.0002554) (Table 2). Similarly, our LME analyses of the oral mucosa did not show differences in the abundances of the phyla or genera (Table 2). The oral microbiome did not show significant differences in alpha diversity between asthmatic and healthy subjects (Table 2 and Figure 2).
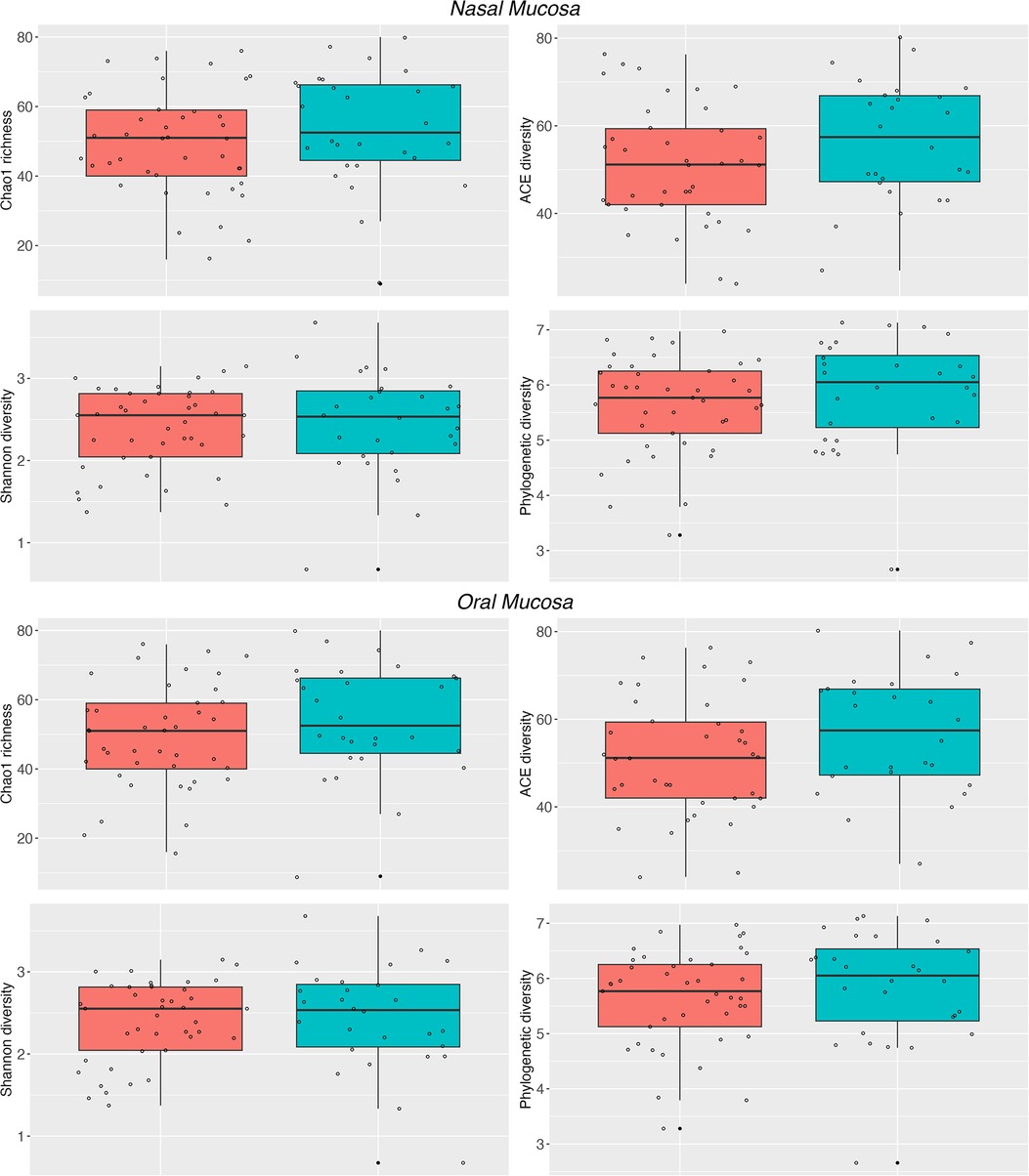
Figure 2. Alpha diversity of the nasal and oral microbiota. Nasal (top panel) and oral (bottom panel) microbiota from healthy subjects (cyan) and asthmatic patients (red). The Chao1, ACE, Shannon and PD indices show differences between the asthmatic and healthy nasal microbiota. See Table 2 for detailed statistics.
Conversely, the results in the nasal communities showed that the diversity between asthmatic and healthy subjects varies greatly (Permanova, Unifrac, wUnifrac, Bray-Curtis and Jaccard index; P > 0.01) (Table 2 and Supplementary Figure S4). In particular, nasal microbiomes showed significant differences in alpha diversity between the asthmatic and healthy subjects in indices Chao1 (P = 4.699 × −5), ACE (P = 9.513 × −8), Shannon (P = 0.0006668), and phylogenetic diversity (P = 0.0033340) (Table 2). Altogether, these results suggest that the microbiota is structured differently in the upper airways and that there is a significant difference between asthmatic and healthy subjects in the nasal mucosa. We did not observe significant differences in the diversity of the oral microbiota and results show no evidence of community differences between asthmatic and healthy subjects in the oral samples, except for a trend in the abundance of Firmicutes.
Microbiome interactions
To infer interactions between bacteria and identify keystone taxa (ASVs), we performed a co-occurrence network analysis. The nasal microbiome networks showed clear differences in topology and number of keystone taxa between asthmatic and healthy subjects (Figure 3). The oral microbiota was characterized by keystone ASVs of the genera Leptotrichia (ASV27: degree = 27 and betweenness = 726; ASV60: degree = 8 and betweenness = 2,250; ASV105: degree = 6 and betweenness = 491), Porphyromonas (ASV2007: degree = 5 and betweenness = 764), Prevotella_6 (ASV2081: degree = 5 and betweenness = 661), and Kingella (ASV3054: degree = 5 and betweenness = 985). In turn, the nasal microbiome network of healthy children included keystone ASVs of the genera Prevotella_2 (ASV2059: degree = 7 and betweenness = 160) and Prevotella_7 (ASV2128: degree = 7 and betweenness = 146). The nasal microbiome networks also showed differences in topology between asthmatic and healthy subjects, but the keystone taxa did not vary much; a ASV of the genus Streptococcus (ASV203: degree = 11 and betweenness = 1,085) was observed in both healthy and asthmatic children, while two ASVs of the genera Granulicatella (ASV296: degree = 6 and betweenness = 777) and Veillonella (ASV441: degree = 6 and betweenness = 900) were found only in the asthmatic subjects.
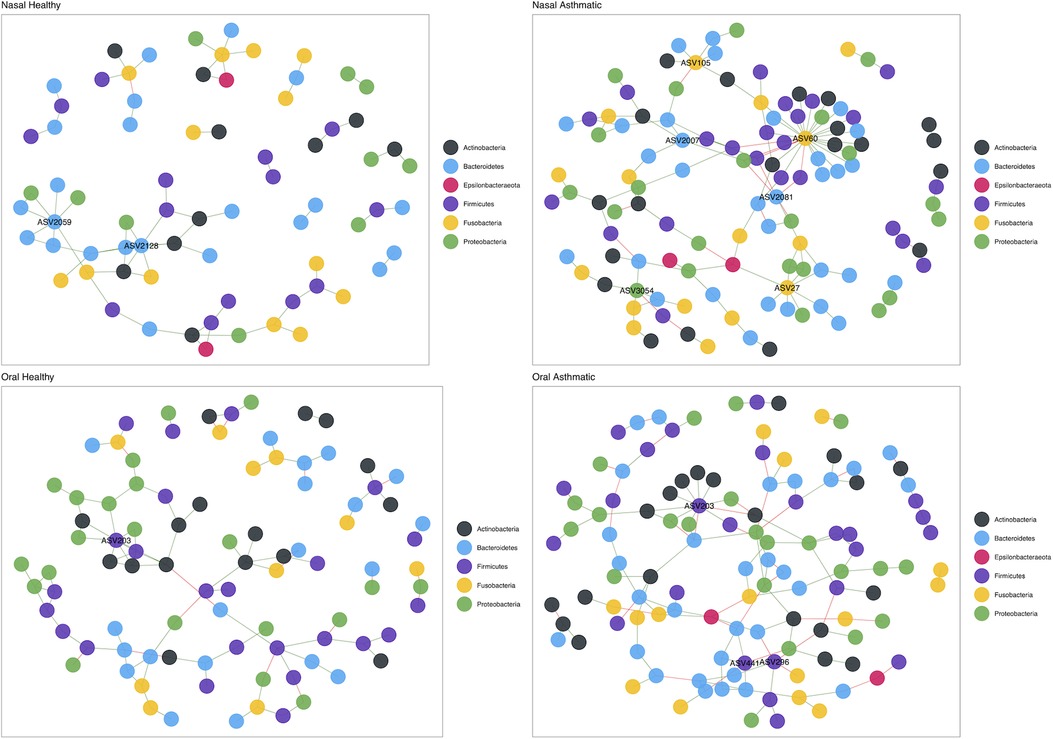
Figure 3. Structure of the nasal microbiota in health and disease. Upper panel shows nasal microbiota co-occurrence networks in healthy (left) and asthmatic (right) subjects. Bottom panel shows oral mucosa co-occurrence network in healthy (left) and asthmatic (right) subjects. Colors represent taxa at the phylum level.
Microbiome functional profiles
We found significant differences in the relative abundances of metabolic pathways between nasal microbiomes of asthmatic and healthy subjects (Figure 4 and Supplementary Table S2). We identified a total of 51 metabolic pathways, of which a total of 38 pathways were significantly underrepresented in asthmatics; these pathways are related to the degradation and synthesis of amino acids, nucleotides, and carbon sources. Only 13 pathways were significantly overrepresented in asthmatics, including tRNA charging pathway, L-alanine biosynthesis, pyrimidine deoxyribonucleotides biosynthesis/degradation and purine nucleotides biosynthesis. With respect to the oral mucosa, we did not detect any significant differences in metabolic pathways between healthy and asthmatic subjects.
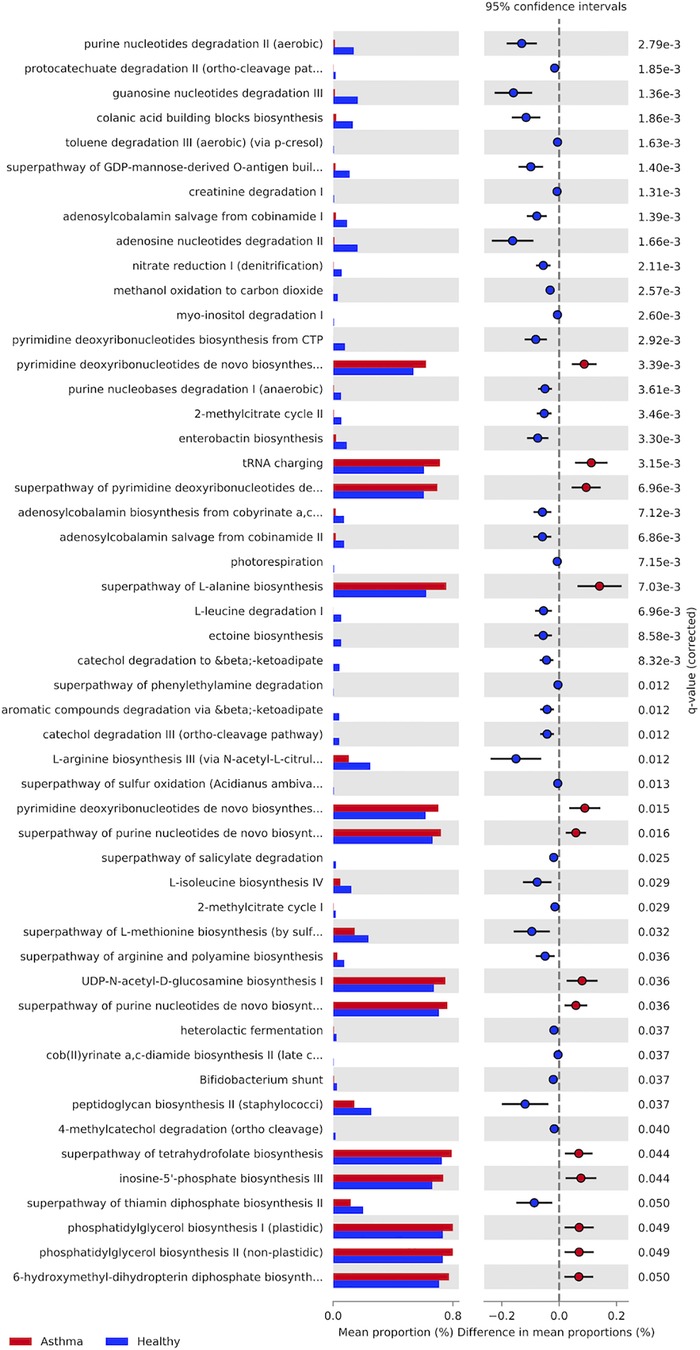
Figure 4. Metabolic pathways present in the asthmatics and healthy subjects’ nasal microbiota. Asthmatic subjects in red and healthy subjects in blue. Last column shows P-value (P > 0.05).
Discussion
Asthma is a condition that imparts large economic burdens to individuals and society. While previous studies indicate that multiple factors are related to the onset and development of asthma, emerging evidence suggest that asthma is intimately linked to alterations of the upper airway microbiota. In this cross-sectional study, we applied 16S rRNA gene sequencing to a cohort of children and adolescents from Santiago de Chile. We identified distinct taxonomic and functional profiles, as well as significant differences in the structure of the microbiota and key bacterial taxa.
Diversity and composition of the nasal and oral microbiotas in asthmatic and health subjects
Our results show differences in diversity and composition in the nasal microbiota of asthmatic subjects compared to healthy subjects (Table 2). We detected a decrease in alpha diversity in the nasal microbiota of asthmatic individuals (Figure 1). Decreased microbial diversity has also been observed in other human diseases, e.g., intestinal failure (66), diarrheagenic infection (67) or intestinal inflammation (68), including some related to the airways, such as rhinitis, bronchiolitis, and cystic fibrosis (18, 30, 69, 70). Our results also agree with previous studies in other populations that show that microbial communities of asthmatics are less diverse than those of healthy individuals (25, 26, 71, 72), which we interpret as a result of the overdominance of some taxonomic groups, and the resulting changes in community structure and microbial interactions over the whole of the community.
Regarding the diversity of the oral microbiota, our analyses did not show significant differences in any diversity index evaluated, indicating that there is no significant variation in the oral microbiota among asthmatic subjects compared to healthy individuals. These results suggest that the oral microbiota seems to be resistant in this particular cohort. We chose the oral mucosa as the relevant sample to study because relationships between the oral microbiota and its involvement in respiratory diseases or complications are unknown. Associations between microbes in the oral cavity and respiratory diseases have been identified in other studies. In pneumonia, for example, respiratory pathogens such as Haemophilus influenzae and Klebsiella pneumoniae are readily detected (73); Staphylococcus aureus (74) and SARS-CoV-2 have also been detected recently (75). In addition, other studies have shown that subjects with COPD have poor oral health and, in consequence, alterations in the oral microbiota (76, 77). Also, recent studies show significant changes in composition and structure of the microbial communities in asthmatics, rhinitis patients, and controls (78). Although certain microorganisms have been found that could be associated with allergies or asthma, such as Gemella or Lactobacillus (79); Veillonella, and Streptococcus (80); and Aggregatibacter (81), they have not shown dysbiosis in the oral microbiota during asthma.
Previous studies have also shown that the composition of the microbiota varies across human populations (37, 39, 82). Likewise, the oral and nasal microbiota, either in health or disease, have been shown to vary across populations (83–86). In this study, we found that the nasal microbiota of asthmatic subjects has a high relative abundance of Moraxella and Haemophilus (Table 2). These results partially agree with previous studies on cohorts from different countries. For instance, in the USA the most abundant taxa in asthmatic subjects are Moraxella, Streptococcus and Haemophilus (24–26). In one population in Europe, the more common taxa are Streptococcus, Veillonella, Haemophilus, Prevotella, and Rothia (87), while in Portugal high abundant taxa in asthmatic subjects were Dolosigranulum, Haemophilus, Lawsonella, Moraxella, and Neisseria (30). Therefore, the same microorganisms have been detected, however, they differ in their relative abundances, which suggests that this depends on each population under study. The overabundant taxa possibly involved in dysbiosis (Moraxella and Haemophilus) are shared with other respiratory diseases such as bronchitis (28) or rhinitis (88), which are chronic diseases of the respiratory tract, producing inflammation and dysfunction of the nasal mucosa (89, 90).
In terms of composition, our findings show large variability across subjects with and without asthma (Table 2). However, the genera Moraxella, Dolosigranulum, Haemophilus, Corynebacterium_1, Streptococcus and Staphylococcus dominated most of the samples of the asthmatic nasal mucosa. In studies related to asthma in children, the same consortium of bacteria (Moraxella, Streptococcus, Haemophilus, Corynebacterium_1, Dolosigranulum and Staphylococcus) has been reported in other tissues and samples, frequently associated with respiratory tract diseases (17, 25–28, 91, 92). In the same way, the nasal mucosa of healthy children is characterized by the presence of the same genera listed above, all previously reported in the literature (26, 71, 88).
For the oral microbiota in both types of individuals, the most prevalent and abundant genera were Streptococcus, Haemophilus, Gemella, Vellionella, Neisseria, and Porphyromonas. Only the phylum Firmicutes varied significantly in abundance between healthy and asthmatic subjects. This could be, at least in part, because the oral microbiota is associated with other human diseases such as periodontitis, refractory periodontal disease, caries, and odontogenic infection (93). Other works associate early changes in the oral microbiota with maturation of the immune system and the development of allergies or asthma (79). However, a study on an African American cohort showed significant changes in the composition of the microbiota in saliva samples (oral cavity) with an increase in the genera Streptococcus and Veillonella in asthmatic subjects (80).
The characterization of the oral microbiota is consistent with other studies. For instance, studies in adults using oropharynx and oral wash samples and 454 pyrosequencing detected a high relative abundance of Streptococcaceae, Veillionellaceae, Fusobacteriaceae and Neisseriaceae (94). Hilty and collaborators in 2010 reported the same phyla and genera in oropharynx samples, one of the first studies related to asthma and microbiota (20). Other studies have found similar results than those reported here, e.g., high abundances of Streptococcus, Prevotella, and Veillonella, which comprise 70% of the oral microbiota (95). However, more studies of the oral microbiota in children or adolescents are required, since recent studies have shown that the microbiota of children is more diverse than that of adults; however, the predominant microorganisms of the oral microbiota have similar abundances (96).
Furthermore, in our study we have observed limited variability of the oral microbiota between subjects, as shown by alpha and beta indices (Figures 1, 2). In contrast, other studies of different diseases or conditions, like children with obesity (97), have revealed changes in the diversity of the microbiota compared to children with normal weight, or changes in microbial diversity associated with gingival bleeding in children (98). In our cohort, we did not detect any evidence of change or alteration in composition or difference in diversity in the oral microbiota. We expect that a new analysis using a greater number of individuals may increase the power to detect variation in this compartment.
Finally, in oral samples, our results only showed a trend between healthy and asthmatic members of the Firmicutes phylum. This is not analogous to what has been suggested by other studies on asthma in children and young people carried out in a different cohort (African Americans) (80). This result reinforces the importance of local studies; cross-sectional studies in addition to longitudinal studies in different populations will be decisive in providing information linking oral microbiota dysbiosis with asthma.
Nasal microbial function varies between asthmatic and healthy subjects
We detected differences in the representation of metabolic pathways on nasal mucosa in asthmatic subjects, e.g., in metabolic pathways related to nucleotide synthesis such as pyrimidine biosynthesis and purine biosynthesis, and pathways related to amino acids, metabolism and transport. These results suggest that the functional potential of the nasal microbiota could be subject to an imbalance as a result of microbial differences in composition between asthmatic and healthy subjects. Previous studies have shown similar results in unrelated populations (30, 99–103). Studies focused on other respiratory illnesses, such as cystic fibrosis have also shown major changes in the representation of metabolic pathways, e.g., enrichment in degradation of aminobenzoate, geraniol, lysine, benzoate, valine leucine and isoleucine; metabolism of beta-alanine, propanoate, tryptophan, butanoate and fatty acid (70).
Like asthma, rhinitis is a disorder in which immunoglobulin E (IgE) and Th2 lymphocytes mediate responses to a small numbers of allergens (104–106). Some studies estimate that 38% of subjects with asthma have rhinitis and that both conditions can coexist in the same patient (106). Metabolomic studies in allergic rhinitis show that deoxyuridine and inosine compounds (pyrimidine metabolism and purine metabolism, respectively), are mostly present in subjects with this disease (107). These same pathways are also overrepresented in asthmatic subjects (Figure 4). In addition, studies of allergic rhinitis with other cohorts show that the same metabolic pathways are increased in cases compared to controls (30, 108).
The alanine synthesis pathway also shows an overrepresentation in asthmatic subjects. Amino acids such as lysine, histidine, and tyrosine, among others, have been studied and are related to IgE sensitivity and response in mild and severe asthma (109). In the case of cystic fibrosis, changes in carbon sources are correlated with a dysbiosis in the microbiota, which might reflect changes in energy requirements in metabolizing carbohydrates (70). Similarly, pyrimidine and purine nucleoside triphosphates serve as precursors of DNA and RNA (110), which also suggest that an enrichment in these pathways is related to changing energy requirements. We speculate that the microbiota in asthma is more energy demanding than in the healthy microbiota, possibly due to challenges imposed by the host or by members of the microbiota.
These results suggest that in the studied cohort, metabolites from members of the microbiota could be related to inflammation in the upper pathways. Further studies using metabolomics or metatranscriptomics will be needed to identify which metabolites or active metabolic pathways are up- or down-regulated in asthmatic subjects. For example, certain compounds can modulate the microbiota and its metabolic activity (111) or how certain taxa contribute to asthma (112) or other diseases (COPD). Different omics approaches are being integrated to establish connections between microbiome-metabolome and the host (113).
Bacterial interactions in the nasal and oral microbiotas of asthmatic and healthy subjects
We found four keystone species in the nasal microbiota of asthmatic subjects, i.e., Leptotrichia, Porphyromonas, Prevotella_6, and Kingella. These results suggest that differences in microbial diversity and composition may cause a restructuring of the microbial interactions in the nose and mouth, so different taxa may adopt key roles in the microbiota. Other studies addressing microbiota structure in asthma have also reported keystone species. In a study from a population in the USA that used a metatranscriptomic approach, researchers reported Moraxella, Alloiococcus, and Corynebacterium as keystone species (27), none of which were detected in our dataset. A study of a population from northern Portugal (30) using more than 300 samples also examined community structure using networks and reported that subjects with allergic rhinitis with and without asthma have more complex networks with more connected nodes, which is in accordance with what we report in this study, where the networks of asthmatic subjects have more connections and are more complex (Figure 4). In addition, the key taxa identified in the Portuguese cohort such as Leptotrichia and Veillonela were also detected in this study, though the Chilean population also identified the genus Prevotella as keystone, which was absent in the Portuguese population. This suggests that asthma has key and shared mechanisms at the microbiota level and that the diversity of the microbiota is a key point to consider in future studies. However, other studies in asthma have also revealed significant differences in co-occurrence networks when studied fungal and bacterial composition between endotypes of asthma (114) and asthmatic vs. controls (115, 116).
Overall, these results are consistent with the available literature, which suggests that Prevotella is a commensal bacterial genus, but in some cases exhibits pathobiont properties (117). Prevotella abundance has been reported to be reduced in subjects with COPD and with asthma (20). The genus Prevotella is associated with establishing tolerance in the respiratory airways as symbiotic bacteria and could partially reduce Haemophilus-induced IL-12 production by dendritic cells (118). In our case, we see Prevotella replaced by other key taxa, which could suggest that inflammation caused by changes in composition and the overabundance of certain taxa displaces its participation (119). This likely reflects different symbiotic interactions between pathogenic and commensal bacteria in the nose as seen in other respiratory diseases (120, 121). Nonetheless, microbial taxa co-occurrences shown here using 16S rRNA data need to be confirmed using more powerful shotgun metagenomic and RNASeq technologies (122).
Our findings are also in agreement with other studies that document that less abundant taxa may have a high degree of connectivity (123), as is the case of Prevotella_2 and Prevotella_7 in healthy nasal mucosa samples and Leptotrichia, Porphyromonas, Prevotella_6, and Kingella in the asthmatic nasal mucosa, which are identified as key nodes (i.e., keystone taxa). Interactions within communities are the fundamental support for their development and maintenance. Non-asthma studies using interaction networks within the microbiota have established that rare taxa play a central role in communities, for example, the genus Symbiodinium which plays a central role in coral robustness (124), or rare and low abundance taxa that contribute significantly to plant rhizospheres (125). This suggests that a particular node may play an irreplaceable role within the community by maintaining key interactions or relationships for its structure, regardless of their abundance.
The Streptococcus genus, one of the main culprits in respiratory tract diseases like pharyngitis and pneumonia (126) show a significant difference between healthy and asthmatic subjects (Table 2). However, we have not detected a high degree of connectivity or centrality within the network of this particular genus. Studies carried out in US American populations involving the use of metatranscriptomics have depicted Streptococcus as a hub that is negatively associated with all other members of the microbiota (27). However, traditional correlation analysis of microbial population amplicon data is likely to produce poor results (127). Studies based on Pearson’s correlation, as is the case of the study by Chun et al., do not account for independence between samples and, being purely compositional, are biased by the fact that, since they must sum to 1, the fractions are not independent and tend to be negatively correlated, regardless of the true correlation between the underlying absolute abundances (128). Therefore, estimates of correlations often reflect the nature of the composition of the data and are not indicative of underlying biological processes (129, 130). Recent methods that take into account the limitations of the techniques used and analyze the samples more independently generate a better representation of the microbial network (127, 131, 132). Our results do not consider the Streptococcus genus as a key node within the microbiota, which may be due to the variability of the microbiota across subjects. Future studies should estimate co-occurrence networks in the microbiota throughout the development of the disease. A study of disease dynamics and progression from a health to a disease would provide key information to assess how the interactions are modulated from a healthy microbiota to an imbalanced one (i.e., dysbiosis) (133).
In summary, this cross-sectional study characterizes for the first time the microbiota of the upper respiratory tract of Chilean children with and without asthma. We found diversity, compositional, and structural differences between asthmatic and healthy children in this particular cohort. The detected bacterial phyla and genera with differential abundance from those described in other cohorts of children with asthma. This reinforces the importance of comparative studies of the microbiome across human populations to discover differences and similarities and make more informed decisions about public health interventions.
Data availability statement
The datasets presented in this study can be found in online repositories. All sequence data was deposited in the NCBI under Bioproject accession number PRJNA446042. All R code and metadata are available in GitHub (https://github.com/ramostapiai/16s-Analysis).
Ethics statement
The studies involving human participants were reviewed and approved by MINISTRY OF HEALTH, CHILE. Written informed consent to participate in this study was provided by the participants’ legal guardian/next of kin.
Author contributions
Conceptualization, IR-T, KR, MP-L and EC-N; methodology, IR-T, MP-L and EC-N; formal analysis, IR-T; resources, EC-N; data curation, IR-T, MP-L and EC-N; writing—original draft preparation, IR-T, MP-L and EC-N; writing—review and editing, IR-T, MP-L and EC-N; visualization, IR-T, MP-L and EC-N; supervision, EC-N; project administration, KR and EC-N; funding acquisition, EC-N. All authors have read and agreed to the published version of the manuscript. All authors contributed to the article and approved the submitted version.
Funding
This research was funded by “ANID Proyecto de atracción e inserción PAI 82140008”, by FONDECYT 1200834, and by ANID ANILLO ACT192057.
Acknowledgments
We thank Felipe Gutiérrez and David Vargas from the Amador Neghme medical center for their help during sample collection and storage.
Conflict of interest
The authors declare that the research was conducted in the absence of any commercial or financial relationships that could be construed as a potential conflict of interest.
Publisher's note
All claims expressed in this article are solely those of the authors and do not necessarily represent those of their affiliated organizations, or those of the publisher, the editors and the reviewers. Any product that may be evaluated in this article, or claim that may be made by its manufacturer, is not guaranteed or endorsed by the publisher.
Supplementary material
The Supplementary Material for this article can be found online at: https://www.frontiersin.org/articles/10.3389/falgy.2023.1223306/full#supplementary-material
References
1. World Health Organization. Global Surveillance, Prevention and Control of CHRONIC RESPIRATORY DISEASES (2006).
2. Vos T, Lim SS, Abbafati C, Abbas KM, Abbasi M, Abbasifard M, et al. Global burden of 369 diseases and injuries in 204 countries and territories, 1990–2019: a systematic analysis for the global burden of disease study 2019. Lancet. (2020) 396(10258):1204–22. doi: 10.1016/S0140-6736(20)30925-9
3. Asher MI, Rutter CE, Bissell K, Chiang C-Y, Sony AE, Ellwood E, et al. Worldwide trends in the burden of asthma symptoms in school-aged children: global asthma network phase I cross-sectional study. Lancet. (2021) 398(10311):1569–80. doi: 10.1016/S0140-6736(21)01450-1
4. Martin J, Townshend J, Brodlie M. Diagnosis and management of asthma in children. BMJ Paediatr Open. (2022) 6(1):e001277. doi: 10.1136/bmjpo-2021-001277
5. Herrera AM, Cavada Ch G, Mañalich J. Hospitalizaciones Por Asma Infantil En Chile: 2001–2014. Rev Chil Pediatr. (2017) 88(5):602–7. doi: 10.4067/S0370-41062017000500005
6. Garcia-Marcos L. Asthma prevalence trends in Chile: a prelude of the global asthma network findings? Allergol Immunopathol (Madr). (2019) 47(4):311–12. doi: 10.1016/j.aller.2019.06.001
7. Alvear S, Canteros J, Jara J, Rodríguez P. Costos Reales de Tratamientos Intensivos Por Paciente Y Día Cama. Rev Med Chil. (2013) 141:202–8. doi: 10.4067/S0034-98872013000200009
8. Song SH, Wang XQ, Shen Y, Hong SL, Ke X. Association between PTPN22/CTLA-4 gene polymorphism and allergic rhinitis with asthma in children. Iran J Allergy Asthma Immunol. (2016) 15(5):413–19.27917628
9. Hunninghake GM, Soto-Quirós ME, Avila L, Su J, Murphy A, Demeo DL, et al. Polymorphisms in IL13, total IgE, eosinophilia, and asthma exacerbations in childhood. J Allergy Clin Immunol. (2007) 120(1):84–90. doi: 10.1016/j.jaci.2007.04.032
10. Baumann LM, Romero KM, Robinson CL, Hansel NN, Gilman RH, Hamilton RG, et al. Prevalence and risk factors for allergic rhinitis in two resource-limited settings in Peru with disparate degrees of urbanization. Clin Exp Allergy. (2015) 45(1):192–99. doi: 10.1111/cea.12379
11. Yao T-C, Ou L-S, Yeh K-W, Lee W-I, Chen L-C, Huang J-L, et al. Associations of age, gender, and BMI with prevalence of allergic diseases in children: PATCH study. J Asthma. (2011) 48(5):503–10. doi: 10.3109/02770903.2011.576743
12. Fu X, Norbäck D, Yuan Q, Li Y, Zhu X, Hashim JH, et al. Indoor microbiome, environmental characteristics and asthma among junior high school students in Johor Bahru, Malaysia. Environ Int. (2020) 138:105664. doi: 10.1016/j.envint.2020.105664
13. Ege MJ, Mayer M, Normand A-C, Genuneit J, Cookson WOCM, Braun-Fahrländer C, et al. Exposure to environmental microorganisms and childhood asthma. N Engl J Med. (2011) 364(8):701–9. doi: 10.1056/NEJMoa1007302
14. Toivonen L, Schuez-Havupalo L, Karppinen S, Waris M, Hoffman KL, Camargo CA, et al. Antibiotic treatments during infancy, changes in nasal Microbiota, and asthma development: population-based cohort study. Clin Infect Dis. (2021) 72(9):1546–54. doi: 10.1093/cid/ciaa262
15. Schoos A-MM, Kragh M, Ahrens P, Kuhn KG, Rasmussen MA, Chawes BL, et al. Season of birth impacts the neonatal nasopharyngeal Microbiota. Children. (2020) 7(5):45. doi: 10.3390/children7050045
16. Chiu C-Y, Chan Y-L, Tsai Y-S, Chen S-A, Wang C-J, Chen K-F, et al. Airway microbial diversity is inversely associated with mite-sensitized rhinitis and asthma in early childhood. Sci Rep. (2017) 7(1):1820. doi: 10.1038/s41598-017-02067-7
17. Zhou Y, Jackson D, Bacharier LB, Mauger D, Boushey H, Castro M, et al. The upper-airway microbiota and loss of asthma control among asthmatic children. Nat Commun. (2019) 10(1):5714. doi: 10.1038/s41467-019-13698-x
18. Park H-K, Choi Y, Lee D-H, Kim S, Lee J-M, Choi SW, et al. Altered gut Microbiota by azithromycin attenuates airway inflammation in allergic asthma. J Allergy Clin Immunol. (2020) 145(5):1466–69.e8. doi: 10.1016/j.jaci.2020.01.044
19. Park YM, Lee SY, Kang MJ, Kim BS, Lee MJ, Jung SS, et al. Imbalance of gut streptococcus, clostridium, and akkermansia determines the natural course of atopic dermatitis in infant. Allergy Asthma Immunol Res. (2020) 12(2):322–37. doi: 10.4168/aair.2020.12.2.322
20. Hilty M, Burke C, Pedro H, Cardenas P, Bush A, Bossley C, et al. Disordered microbial communities in asthmatic airways. PLoS One. (2010) 5(1):e8578. doi: 10.1371/journal.pone.0008578
21. Durack J, Boushey HA, Lynch SV. Airway Microbiota and the implications of dysbiosis in asthma. Curr Allergy Asthma Rep. (2016) 16(8):52. doi: 10.1007/s11882-016-0631-8
22. Chung KF. Airway microbial dysbiosis in asthmatic patients: a target for prevention and treatment? J Allergy Clin Immunol. (2017) 139(4):1071–81. doi: 10.1016/j.jaci.2017.02.004
23. Majak P, Molińska K, Latek M, Rychlik B, Wachulec M, Błauż A, et al. Upper-airway dysbiosis related to frequent sweets consumption increases the risk of asthma in children with chronic rhinosinusitis. Pediatr Allergy Immunol. (2021) 32:489–500. doi: 10.1111/pai.13417
24. Teo SM, Mok D, Pham K, Kusel M, Serralha M, Troy N, et al. The infant nasopharyngeal microbiome impacts severity of lower respiratory infection and risk of asthma development. Cell Host Microbe. (2015) 17(5):704–15. doi: 10.1016/j.chom.2015.03.008
25. Castro-Nallar E, Shen Y, Freishtat RJ, Pérez-Losada M, Manimaran S, Liu G, et al. Integrating microbial and host transcriptomics to characterize asthma-associated microbial communities. BMC Med Genomics. (2015) 8:50. doi: 10.1186/s12920-015-0121-1
26. Pérez-Losada M, Alamri L, Crandall KA, Freishtat RJ. Nasopharyngeal microbiome diversity changes over time in children with asthma. PLoS One. (2017) 12(1):e0170543. doi: 10.1371/journal.pone.0170543
27. Chun Y, Do A, Grishina G, Grishin A, Fang G, Rose S, et al. Integrative study of the upper and lower airway microbiome and transcriptome in asthma. JCI Insight. (2020) 5(5):e133707. doi: 10.1172/jci.insight.133707
28. Raita Y, Pérez-Losada M, Freishtat RJ, Hahn A, Castro-Nallar E, Ramos-Tapia I, et al. Nasopharyngeal metatranscriptome profiles of infants with bronchiolitis and risk of childhood asthma: a multicentre prospective study. Eur Respir J. (2022) 60(1):2102293. doi: 10.1183/13993003.02293-2021
29. Pérez-Losada M, Authelet KJ, Hoptay CE, Kwak C, Crandall KA, Freishtat RJ. Pediatric asthma comprises different phenotypic clusters with unique nasal microbiotas. Microbiome. (2018) 6(1):179. doi: 10.1186/s40168-018-0564-7
30. Pérez-Losada M, Castro-Nallar E, Boechat JL, Delgado L, Rama TA, Berrios-Farías V, et al. Nasal bacteriomes of patients with asthma and allergic rhinitis show unique composition, structure, function and interactions. Microorganisms. (2023b) 11(3):683. doi: 10.3390/microorganisms11030683
31. Zhou Y, Gao H, Mihindukulasuriya KA, La Rosa PS, Wylie KM, Vishnivetskaya T, et al. Biogeography of the ecosystems of the healthy human body. Genome Biol. (2013) 14(1):R1. doi: 10.1186/gb-2013-14-1-r1
32. Gaeckle NT, Pragman AA, Pendleton KM, Baldomero AK, Criner GJ. The oral-lung axis: the impact of oral health on lung health. Respir Care. (2020) 65(8):1211–20. doi: 10.4187/respcare.07332
33. Imai K, Iinuma T, Sato S. Relationship between the oral cavity and respiratory diseases: aspiration of oral Bacteria possibly contributes to the progression of lower airway inflammation. Jpn Dent Sci Rev. (2021) 57:224–30. doi: 10.1016/j.jdsr.2021.10.003
34. Dong J, Li W, Wang Q, Chen J, Zu Y, Zhou X, et al. Relationships between oral microecosystem and respiratory diseases. Front Mol Biosci. (2021) 8:718222. doi: 10.3389/fmolb.2021.718222
35. Arweiler NB, Rahmel V, Alhamwe BA, Alhamdan F, Zemlin M, Boutin S, et al. Dental biofilm and saliva microbiome and its interplay with pediatric allergies. Microorganisms. (2021) 9(6):1330. doi: 10.3390/microorganisms9061330
36. Wang H, Dai W, Feng X, Zhou Q, Wang H, Yang Y, et al. Microbiota composition in upper respiratory tracts of healthy children in Shenzhen, China, differed with respiratory sites and ages. BioMed Res Int. (2018) 2018:6515670. doi: 10.1155/2018/6515670
37. Ursell LK, Clemente JC, Rideout JR, Gevers D, Gregory Caporaso J, Knight R. The interpersonal and intrapersonal diversity of human-associated microbiota in key body sites. J Allergy Clin Immunol. (2012) 129(5):1204–8. doi: 10.1016/j.jaci.2012.03.010
38. Bäckhed F, Fraser CM, Ringel Y, Sanders ME, Balfour Sartor R, Sherman PM, et al. Defining a healthy human gut microbiome: current concepts, future directions, and clinical applications. Cell Host Microbe. (2012) 12(5):611–22. doi: 10.1016/j.chom.2012.10.012
39. Human Microbiome Project Consortium. Structure, function and diversity of the healthy human microbiome. Nature. (2012) 486(7402):207–14. doi: 10.1038/nature11234
40. Cardenas PA, Cooper PJ, Cox MJ, Chico M, Arias C, Moffatt MF, et al. Upper airways microbiota in antibiotic-naïve wheezing and healthy infants from the tropics of rural Ecuador. PLoS One. (2012) 7(10):e46803. doi: 10.1371/journal.pone.0046803
41. McDonald D, Hyde E, Debelius JW, Morton JT, Gonzalez A, Ackermann G, et al. American gut: an open platform for citizen science microbiome research. mSystems. (2018) 3(3):10–1128. doi: 10.1128/mSystems.00031-18
42. Ehrlich SD. MetaHIT: the European union project on metagenomics of the human intestinal tract. In: Nelson KE, editor. Metagenomics of the human body. New York, NY: Springer New York (2011). p. 307–16.
43. Danko D, Bezdan D, Afshin EE, Ahsanuddin S, Bhattacharya C, Butler DJ, et al. A global metagenomic map of urban microbiomes and antimicrobial resistance. Cell. (2021) 184(13):3376–93.e17. doi: 10.1016/j.cell.2021.05.002
44. Corvalán C, Amigo H, Bustos P, Rona RJ. Socioeconomic risk factors for asthma in chilean young adults. Am J Public Health. (2005) 95(8):1375. doi: 10.2105/AJPH.2004.048967
45. Mallol J, Aguirre V, Aguilar P, Calvo M, Amarales L, Arellano P, et al. Cambios En La Prevalencia de Asma En Escolares Chilenos Entre 1994 Y 2002: international study of asthma and allergies in childhood (ISAAC)—chile phases I and III. Rev Méd Chile. (2007) 135(5):580–86. doi: 10.4067/S0034-98872007000500005
46. Herrera AM, Brand P, Cavada G, Koppmann A, Rivas M, Mackenney J, et al. Hospitalizations for asthma exacerbation in chilean children: a multicenter observational study. Allergol Immunopathol (Madr). (2018) 46(6):533–38. doi: 10.1016/j.aller.2018.02.006
47. Herrera AM, Brand P, Cavada G, Koppmann A, Rivas M, Mackenney J, et al. Treatment, outcomes and costs of asthma exacerbations in chilean children: a prospective multicenter observational study. Allergol Immunopathol (Madr). (2019) 47(3):282–88. doi: 10.1016/j.aller.2018.10.003
48. Agency for Healthcare Research and Quality: A Profile. (2002). Rockville, MD. August 1, 2002. Available at: https://www.ahrq.gov/cpi/about/profile/index.html
49. Versalovic J, Keitel W, Petrosino J, University W, Watson M, Dunne M, et al. “Human Microbiome Project—Core Microbiome Sampling Protocol A.” 2010. (2010). Available at: http://hmp2.org/doc/HMP_Protocol_Version_9_032210.pdf
50. Kozich JJ, Westcott SL, Baxter NT, Highlander SK, Schloss PD. Development of a dual-index sequencing strategy and curation pipeline for analyzing amplicon sequence data on the MiSeq illumina sequencing platform. Appl Environ Microbiol. (2013) 79(17):5112–20. doi: 10.1128/AEM.01043-13
51. Quast C, Pruesse E, Yilmaz P, Gerken J, Schweer T, Yarza P, et al. The SILVA ribosomal RNA gene database project: improved data processing and web-based tools. Nucleic Acids Res. (2012) 41(D1):D590–96.23193283
52. Wright ES. DECIPHER: harnessing local sequence context to improve protein multiple sequence alignment. BMC Bioinformatics. (2015) 16:322. doi: 10.1186/s12859-015-0749-z
53. Price MN, Dehal PS, Arkin AP. Fasttree 2–approximately maximum-likelihood trees for large alignments. PLoS One. (2010) 5(3):e9490. doi: 10.1371/journal.pone.0009490
54. Callahan BJ, McMurdie PJ, Rosen MJ, Han AW, Johnson AJA, Holmes SP. DADA2: high-resolution sample inference from illumina amplicon data. Nat Methods. (2016) 13(7):581–83. doi: 10.1038/nmeth.3869
55. McMurdie PJ, Holmes S. Phyloseq: an R package for reproducible interactive analysis and graphics of microbiome census data. PLoS One. (2013) 8(4):e61217. doi: 10.1371/journal.pone.0061217
56. McMurdie PJ, Holmes S. Waste not, want not: why rarefying microbiome data is inadmissible. PLoS Comput Biol. (2014) 10(4):e1003531. doi: 10.1371/journal.pcbi.1003531
57. Love MI, Huber W, Anders S. Moderated estimation of fold change and dispersion for RNA-Seq data with DESeq2. Genome Biol. (2014) 15(12):550. doi: 10.1186/s13059-014-0550-8
58. Bates D, Mächler M, Bolker B, Walker S. “Fitting Linear Mixed-Effects Models Using lme4.” arXiv [stat.CO]. arXiv (2014). http://arxiv.org/abs/1406.5823
59. Oksanen J, Kindt R, Legendre P, O’Hara B, Stevens MHH, Oksanen MJ, et al. The vegan package. Community Ecol. (2007) 10(631–637):719.
60. Bozdogan H. Model selection and Akaike’s information criterion (AIC): the general theory and its analytical extensions. Psychometrika. (1987) 52(3):345–70. doi: 10.1007/BF02294361
61. Kurtz ZD, Müller CL, Miraldi ER, Littman DR, Blaser MJ, Bonneau RA. Sparse and compositionally robust inference of microbial ecological networks. PLoS Comput Biol. (2015) 11(5):e1004226. doi: 10.1371/journal.pcbi.1004226
62. Douglas GM, Maffei VJ, Zaneveld J, Yurgel SN, Brown JR, Taylor CM, et al. PICRUSt2 for prediction of metagenome functions. Nat Biotechnol. (2020 38(6):685–88. doi: 10.1101/672295
63. Bluman AG. Elementary statistics: A step by step approach. New York, NY: McGraw-Hill Higher Education (2009).
64. Benjamini Y, Hochberg Y. Controlling the false discovery rate: a practical and powerful approach to multiple testing. J R Stat Soc Series B Stat Methodol. (1995) 57(1):289–300. doi: 10.1111/j.2517-6161.1995.tb02031.x
65. Parks DH, Tyson GW, Hugenholtz P, Beiko RG. STAMP: statistical analysis of taxonomic and functional profiles. Bioinformatics. (2014) 30(21):3123–24.
66. Wang P, Wang Y, Lu L, Yan W, Tao Y, Zhou K, et al. Alterations in intestinal microbiota relate to intestinal failure-associated liver disease and central line infections. J Pediatr Surg. (2017) 52(8):1318–26. doi: 10.1016/j.jpedsurg.2017.04.020
67. Gallardo P, Izquierdo M, Vidal RM, Chamorro-Veloso N, Rosselló-Móra R, O’Ryan M, et al. Distinctive gut microbiota is associated with diarrheagenic Escherichia Coli infections in chilean children. Front Cell Infect Microbiol. (2017) 7:424. doi: 10.3389/fcimb.2017.00424
68. Ossa JC, Yáñez D, Valenzuela R, Gallardo P, Lucero Y, Farfán MJ. Intestinal inflammation in chilean infants fed with bovine formula vs. breast milk and its association with their gut microbiota. Front Cell Infect Microbiol. (2018) 8:190. doi: 10.3389/fcimb.2018.00190
69. Zhang X, Zhang X, Zhang N, Wang X, Sun L, Chen N, et al. Airway microbiome, host immune response and recurrent wheezing in infants with severe respiratory syncytial virus bronchiolitis. Pediatr Allergy Immunol. (2020) 31(3):281–89. doi: 10.1111/pai.13183
70. Kirst ME, Baker D, Li E, Abu-Hasan M, Wang GP. Upper versus lower airway microbiome and metagenome in children with cystic fibrosis and their correlation with lung inflammation. PLoS One. (2019) 14(9):e0222323. doi: 10.1371/journal.pone.0222323
71. Biswas K, Hoggard M, Jain R, Taylor MW, Douglas RG. The nasal microbiota in health and disease: variation within and between subjects. Front Microbiol. (2015) 9:134. doi: 10.3389/fmicb.2015.00134
72. Depner M, Ege MJ, Cox MJ, Dwyer S, Walker AW, Birzele LT, et al. Bacterial microbiota of the upper respiratory tract and childhood asthma. J Allergy Clin Immunol. (2017) 139(3):826–34.e13. doi: 10.1016/j.jaci.2016.05.050
73. Sumi Y, Miura H, Sunakawa M, Michiwaki Y, Sakagami N. Colonization of denture plaque by respiratory pathogens in dependent elderly. Gerodontology. (2002) 19(1):25–9. doi: 10.1111/j.1741-2358.2002.00025.x
74. Heo S-M, Haase EM, Lesse AJ, Gill SR, Scannapieco FA. Genetic relationships between respiratory pathogens isolated from dental plaque and bronchoalveolar lavage fluid from patients in the intensive care unit undergoing mechanical ventilation. Clin Infect Dis. (2008) 47(12):1562–70. doi: 10.1086/593193
75. To KK-W, Tsang OT-Y, Leung W-S, Tam AR, Wu T-C, Lung DC, et al. Temporal profiles of viral load in posterior oropharyngeal Saliva samples and serum antibody responses during infection by SARS-CoV-2: an observational cohort study. Lancet Infect Dis. (2020) 20(5):565–74. doi: 10.1016/S1473-3099(20)30196-1
76. Wang Z, Zhou X, Zhang J, Zhang L, Song Y, Hu FB, et al. Periodontal health, oral health behaviours, and chronic obstructive pulmonary disease. J Clin Periodontol. (2009) 36(9):750–55. doi: 10.1111/j.1600-051X.2009.01448.x
77. Zhou X, Wang J, Liu W, Huang X, Song Y, Wang Z, et al. Periodontal status and microbiologic pathogens in patients with chronic obstructive pulmonary disease and periodontitis: a case-control study. Int J Chron Obstruct Pulmon Dis. (2020) 15:2071–79. doi: 10.2147/COPD.S266612
78. Pérez-Losada M, Castro-Nallar E, Boechat JL, Delgado L, Rama TA, Berrios-Farías V, et al. The oral bacteriomes of patients with allergic rhinitis and asthma differ from that of healthy controls. Front Microbiol. (2023) 14:1197135. doi: 10.3389/fmicb.2023.1197135
79. Dzidic M, Abrahamsson TR, Artacho A, Collado MC, Mira A, Jenmalm MC. Oral microbiota maturation during the first 7 years of life in relation to allergy development. Allergy. (2018) 73(10):2000–11. doi: 10.1111/all.13449
80. Espuela-Ortiz A, Lorenzo-Diaz F, Baez-Ortega A, Eng C, Hernandez-Pacheco N, Oh SS, et al. Bacterial salivary microbiome associates with asthma among African American children and young adults. Pediatr Pulmonol. (2019) 54(12):1948–56. doi: 10.1002/ppul.24504
81. Durack J, Christian LS, Nariya S, Gonzalez J, Bhakta NR, Mark Ansel K, et al. Distinct associations of sputum and oral microbiota with atopic, immunologic, and clinical features in mild asthma. J Allergy Clin Immunol. (2020) 146(5):1016–26. doi: 10.1016/j.jaci.2020.03.028
82. Fujio-Vejar S, Vasquez Y, Morales P, Magne F, Vera-Wolf P, Ugalde JA, et al. The gut microbiota of healthy chilean subjects reveals a high abundance of the phylum verrucomicrobia. Front Microbiol. (2017) 8:1221. doi: 10.3389/fmicb.2017.01221
83. Gupta VK, Paul S, Dutta C. Geography, ethnicity or subsistence-specific variations in human microbiome composition and diversity. Front Microbiol. (2017) 8:1162. doi: 10.3389/fmicb.2017.01162
84. Pedro N, Brucato N, Cavadas B, Lisant V, Camacho R, Kinipi C, et al. First insight into oral microbiome diversity in Papua New Guineans reveals a specific regional signature. Mol Ecol. (2023) 32:2551–64. doi: 10.1111/mec.16702
85. Ogbanga N, Nelson A, Ghignone S, Voyron S, Lovisolo F, Sguazzi G, et al. The oral microbiome for geographic origin: an Italian study. Forensic Sci Int Genet. (2023) 64:102841. doi: 10.1016/j.fsigen.2023.102841
86. Vogtmann E, Chaturvedi AK, Blaser MJ, Bokulich NA, Gregory Caporaso J, Gillison ML, et al. Representative oral microbiome data for the US population: the national health and nutrition examination survey. Lancet Microbe. (2023) 4(2):e60–61. doi: 10.1016/S2666-5247(22)00333-0
87. Thorsen J, Stokholm J, Rasmussen MA, Roggenbuck-Wedemeyer M, Vissing NH, Mortensen MS, et al. Asthma and wheeze severity and the oropharyngeal microbiota in children and adolescents. Ann Am Thorac Soc. (2022) 19(12):2031–43. doi: 10.1513/AnnalsATS.202110-1152OC
88. Marazzato M, Zicari AM, Aleandri M, Conte AL, Longhi C, Vitanza L, et al. 16S metagenomics reveals dysbiosis of nasal core microbiota in children with chronic nasal inflammation: role of adenoid hypertrophy and allergic rhinitis. Front Cell Infect Microbiol. (2020) 10:458. doi: 10.3389/fcimb.2020.00458
89. Steelant B, Seys SF, Van Gerven L, Van Woensel M, Farré R, Wawrzyniak P, et al. Histamine and T helper cytokine-driven epithelial barrier dysfunction in allergic rhinitis. J Allergy Clin Immunol. (2018) 141(3):951–63.e8. doi: 10.1016/j.jaci.2017.08.039
90. Savouré M, Bousquet J, Jaakkola JJK, Jaakkola MS, Jacquemin B, Nadif R. Worldwide prevalence of rhinitis in adults: a review of definitions and temporal evolution. Clin Transl Allergy. (2022) 12(3):e12130. doi: 10.1002/clt2.12130
91. Chochua S, D’Acremont V, Hanke C, Alfa D, Shak J, Kilowoko M, et al. Increased nasopharyngeal density and concurrent carriage of Streptococcus Pneumoniae, haemophilus influenzae, and moraxella catarrhalis are associated with pneumonia in febrile children. PLoS One. (2016) 11(12):e0167725. doi: 10.1371/journal.pone.0167725
92. Simpson JL, Daly J, Baines KJ, Yang IA, Upham JW, Reynolds PN, et al. Airway dysbiosis: haemophilus influenzae and tropheryma in poorly controlled asthma. Eur Respir J. (2016) 47(3):792–800. doi: 10.1183/13993003.00405-2015
93. Krishnan K, Chen T, Paster BJ. A practical guide to the oral microbiome and its relation to health and disease. Oral Dis. (2017) 23(3):276–86. doi: 10.1111/odi.12509
94. Charlson ES, Bittinger K, Haas AR, Fitzgerald AS, Frank I, Yadav A, et al. Topographical continuity of bacterial populations in the healthy human respiratory tract. Am J Respir Crit Care Med. (2011) 184(8):957–63. doi: 10.1164/rccm.201104-0655OC
95. Nasidze I, Li J, Quinque D, Tang K, Stoneking M. Global diversity in the human salivary microbiome. Genome Res. (2009) 19(4):636–43. doi: 10.1101/gr.084616.108
96. Burcham ZM, Garneau NL, Comstock SS, Tucker RM, Knight R, Metcalf JL, et al. Patterns of oral Microbiota diversity in adults and children: a crowdsourced population study. Sci Rep. (2020) 10(1):2133. doi: 10.1038/s41598-020-59016-0
97. Ma T, Wu Z, Lin J, Shan C, Abasijiang A, Zhao J. Characterization of the oral and gut microbiome in children with obesity aged 3 to 5 years. Front Cell Infect Microbiol. (2023) 13:1102650. doi: 10.3389/fcimb.2023.1102650
98. Bertelsen RJ, Barrionuevo AMP, Shigdel R, Lie SA, Lin H, Real FG, et al. Association of oral bacteria with oral hygiene habits and self-reported gingival bleeding. J Clin Periodontol. (2022) 49(8):768–81. doi: 10.1111/jcpe.13644
99. Pérez-Losada M, Castro-Nallar E, Bendall ML, Freishtat RJ, Crandall KA. Dual transcriptomic profiling of host and microbiota during health and disease in pediatric asthma. PLoS One. (2015) 10(6):e0131819. doi: 10.1371/journal.pone.0131819
100. Li K-J, Chen Z-L, Huang Y, Zhang R, Luan X-Q, Lei T-T, et al. Dysbiosis of lower respiratory tract microbiome are associated with inflammation and microbial function variety. Respir Res. (2019) 20(1):272. doi: 10.1186/s12931-019-1246-0
101. Hu Q, Jin L, Zeng J, Wang J, Zhong S, Fan W, et al. Tryptophan metabolite-regulated treg responses contribute to attenuation of airway inflammation during specific immunotherapy in a mouse asthma model. Hum Vaccin Immunother. (2020) 16(8):1891–99. doi: 10.1080/21645515.2019.1698900
102. Samra MS, Lim DH, Han MY, Jee HM, Kim YK, Kim JH. Bacterial microbiota-derived extracellular vesicles in children with allergic airway diseases: compositional and functional features. Allergy Asthma Immunol Res. (2021) 13(1):56–74. doi: 10.4168/aair.2021.13.1.56
103. Li Y, Zou C, Li J, Wang W, Guo Y, Zhao L, et al. Upper respiratory tract microbiota is associated with small airway function and asthma severity. BMC Microbiol. (2023) 23(1):13. doi: 10.1186/s12866-023-02757-5
104. Bergeron C, Hamid Q. Relationship between asthma and rhinitis: epidemiologic, pathophysiologic, and therapeutic aspects. Allergy Asthma Clin Immunol. (2005) 1(2):81–7. doi: 10.1186/1710-1492-1-2-81
105. Kim H, Bouchard J, Renzi PM. The link between allergic rhinitis and asthma: a role for antileukotrienes? Can Respir J. (2008) 15(2):91–8. doi: 10.1155/2008/416095
106. Acevedo-Prado A, Seoane-Pillado T, López-Silvarrey-Varela A, Salgado F-J, Cruz M-J, Faraldo-Garcia A, et al. Association of rhinitis with asthma prevalence and severity. Sci Rep. (2022) 12(1):6389. doi: 10.1038/s41598-022-10448-w
107. Yuan Y, Wang C, Wang G, Guo X, Jiang S, Zuo X, et al. Airway microbiome and serum metabolomics analysis identify differential candidate biomarkers in allergic rhinitis. Front Immunol. (2021) 12:771136. doi: 10.3389/fimmu.2021.771136
108. Chiang T-Y, Yang Y-R, Zhuo M-Y, Yang F, Zhang Y-F, Fu C-H, et al. Microbiome profiling of nasal extracellular vesicles in patients with allergic rhinitis. World Allergy Organ J. (2022) 15(8):100674. doi: 10.1016/j.waojou.2022.100674
109. Chiu C-Y, Cheng M-L, Chiang M-H, Wang C-J, Tsai M-H, Lin G. Integrated metabolic and microbial analysis reveals host-microbial interactions in IgE-mediated childhood asthma. Sci Rep. (2021) 11(1):23407. doi: 10.1038/s41598-021-02925-5
110. Caspi R, Billington R, Fulcher CA, Keseler IM, Kothari A, Krummenacker M, et al. The MetaCyc database of metabolic pathways and enzymes. Nucleic Acids Res. (2018) 46(D1):D633–39. doi: 10.1093/nar/gkx935
111. Wang X, Xu X, Chen Y, Li Z, Zhang M, Zhao C, et al. Liu shen capsule alters airway microbiota composition and metabolite profiles in healthy humans. Front Pharmacol. (2021) 12:824180. doi: 10.3389/fphar.2021.824180
112. Chiu C-Y, Chou H-C, Chang L-C, Fan W-L, Dinh MCV, Kuo Y-L, et al. Integration of metagenomics-metabolomics reveals specific signatures and functions of airway microbiota in mite-sensitized childhood asthma. Allergy. (2020) 75(11):2846–57. doi: 10.1111/all.14438
113. Wang Z, Yang Y, Yan Z, Liu H, Chen B, Liang Z, et al. Multi-omic meta-analysis identifies functional signatures of airway microbiome in chronic obstructive pulmonary disease. ISME J. (2020) 14(11):2748–65. doi: 10.1038/s41396-020-0727-y
114. Sharma A, Laxman B, Naureckas ET, Kyle Hogarth D, Sperling AI, Solway J, et al. Associations between fungal and bacterial microbiota of airways and asthma endotypes. J Allergy Clin Immunol. (2019) 144(5):1214–27.e7. doi: 10.1016/j.jaci.2019.06.025
115. Huang C, Yu Y, Du W, Liu Y, Dai R, Tang W, et al. Fungal and bacterial microbiome dysbiosis and imbalance of trans-kingdom network in asthma. Clin Transl Allergy. (2020) 10:42. doi: 10.1186/s13601-020-00345-8
116. Kim YH, Jang H, Kim SY, Jung JH, Kim GE, Park MR, et al. Gram-negative microbiota is related to acute exacerbation in children with asthma. Clin Transl Allergy. (2021) 11(8):e12069. doi: 10.1002/clt2.12069
117. Larsen JM. The immune response to prevotella bacteria in chronic inflammatory disease. Immunology. (2017) 151(4):363–74. doi: 10.1111/imm.12760
118. Larsen JM, Steen-Jensen DB, Laursen JM, Søndergaard JN, Musavian HS, Butt TM, et al. Divergent pro-inflammatory profile of human dendritic cells in response to commensal and pathogenic bacteria associated with the airway microbiota. PLoS One. (2012) 7(2):e31976. doi: 10.1371/journal.pone.0031976
119. Yadava K, Pattaroni C, Sichelstiel AK, Trompette A, Gollwitzer ES, Salami O, et al. Microbiota promotes chronic pulmonary inflammation by enhancing IL-17A and autoantibodies. Am J Respir Crit Care Med. (2016) 193(9):975–87. doi: 10.1164/rccm.201504-0779OC
120. Hasegawa K, Mansbach JM, Ajami NJ, Espinola JA, Henke DM, Petrosino JF, et al. Association of nasopharyngeal microbiota profiles with bronchiolitis severity in infants hospitalised for bronchiolitis. Eur Respir J. (2016) 48(5):1329–39. doi: 10.1183/13993003.00152-2016
121. Einarsson GG, Comer DM, McIlreavey L, Parkhill J, Ennis M, Tunney MM, et al. Community dynamics and the lower airway microbiota in stable chronic obstructive pulmonary disease, smokers and healthy non-smokers. Thorax. (2016) 71(9):795–803. doi: 10.1136/thoraxjnl-2015-207235
122. Franzosa EA, Hsu T, Sirota-Madi A, Shafquat A, Abu-Ali G, Morgan XC, et al. Sequencing and beyond: integrating molecular “omics” for microbial community profiling. Nat Rev Microbiol. (2015) 13(6):360–72. doi: 10.1038/nrmicro3451
123. Xue Y, Chen H, Yang JR, Liu M, Huang B, Yang J. Distinct patterns and processes of abundant and rare eukaryotic plankton communities following a reservoir cyanobacterial bloom. ISME J. (2018) 12(9):2263–77. doi: 10.1038/s41396-018-0159-0
124. Ziegler M, Eguíluz VM, Duarte CM, Voolstra CR. Rare symbionts may contribute to the resilience of coral-algal assemblages. ISME J. (2018) 12(1):161–72. doi: 10.1038/ismej.2017.151
125. Shi S, Nuccio EE, Shi ZJ, He Z, Zhou J, Firestone MK. The interconnected rhizosphere: high network complexity dominates rhizosphere assemblages. Ecol Lett. (2016) 19(8):926–36. doi: 10.1111/ele.12630
126. Patterson MJ. Streptococcus. In: Baron S, editor. Medical Microbiology. 4th edition. Galveston (TX): University of Texas Medical Branch at Galveston (1996). Chapter 13. Available at: https://www.ncbi.nlm.nih.gov/books/NBK7611/
127. Friedman J, Alm EJ. Inferring correlation networks from genomic survey data. PLoS Comput Biol. (2012) 8(9):e1002687. doi: 10.1371/journal.pcbi.1002687
128. Aitchison J. The statistical analysis of compositional data. J R Stat Soc. (1982) 44(2):139–60. doi: 10.1111/j.2517-6161.1982.tb01195.x
129. Pearson K. Mathematical contributions to the theory of evolution.—on a form of spurious correlation which may arise when indices are used in the measurement of organs. Proc R Soc London. (1897). 60:489–98. doi: 10.1098/rspl.1896.0076
130. Pawlowsky-Glahn V, Buccianti A. Compositional data analysis: Theory and applications. Chichester: Wiley (2011).
131. Faust K, Fah Sathirapongsasuti J, Izard J, Segata N, Gevers D, Raes J, et al. Microbial co-occurrence relationships in the human microbiome. PLoS Comput Biol. (2012) 8(7):e1002606. doi: 10.1371/journal.pcbi.1002606
132. Alshawaqfeh M, Serpedin E, Younes AB. Inferring microbial interaction networks from metagenomic data using SgLV-EKF algorithm. BMC Genomics. (2017) 18(Suppl 3):228. doi: 10.1186/s12864-017-3605-x
Keywords: 16S rRNA, asthma, nasal bacteriome, Chilean microbiota, upper respiratory tract
Citation: Ramos-Tapia I, Reynaldos-Grandón KL, Pérez-Losada M and Castro-Nallar E (2023) Characterization of the upper respiratory tract microbiota in Chilean asthmatic children reveals compositional, functional, and structural differences. Front. Allergy 4:1223306. doi: 10.3389/falgy.2023.1223306
Received: 15 May 2023; Accepted: 20 July 2023;
Published: 28 July 2023.
Edited by:
Ramcés Falfán-Valencia, National Institute of Respiratory Diseases-Mexico (INER), MexicoReviewed by:
Christopher McMullen, Feedlot Health Management Services Ltd, CanadaVinícius Molla, Hospital Nove De Julho, Brazil
© 2023 Ramos-Tapia, Reynaldos-Grandón, Pérez-Losada and Castro-Nallar. This is an open-access article distributed under the terms of the Creative Commons Attribution License (CC BY). The use, distribution or reproduction in other forums is permitted, provided the original author(s) and the copyright owner(s) are credited and that the original publication in this journal is cited, in accordance with accepted academic practice. No use, distribution or reproduction is permitted which does not comply with these terms.
*Correspondence: Eduardo Castro-Nallar ZWNhc3Ryb25AdXRhbGNhLmNs