- 1Department of Agricultural Sciences, University of Naples Federico II, Portici, Italy
- 2Interdepartmental Research Centre on the “Earth Critical Zone”, University of Naples Federico II, Naples, Italy
- 3Research Center for Cereal and Industrial Crops – Council for Agricultural Research and Economics (CREA), Caserta, Italy
- 4Research Centre for Cereal and Industrial Crops – Council for Agricultural Research and Economics (CREA), Foggia, Italy
Durum wheat, a staple crop in Italy, faces substantial challenges due to increasing droughts and rising temperatures. This study examines the grain yield, agronomic traits, and quality of 41 durum wheat varieties over ten growing seasons in Southern Italy, utilizing a randomized complete block design. Notably, most varieties were not repeated between trials and 45% of the data was missing. The results indicate that the interaction between genotype and environment (GEI) significantly impacted all traits. High temperatures, elevated vapor pressure deficit (VPD), and water deficits severely affected yield and quality during warm years, while cooler years with favorable water availability promoted better growth and higher yields. Broad-sense heritability (H²) was generally low, suggesting that environmental factors played a major role in the observed traits. However, some traits, such as grain yield, ears per square meter, plant height, bleached wheat, thousand-grain weight, and hectoliter weight exhibited moderate to high heritability of the mean genotype (h²mg), indicating their potential for effective selection in breeding programs. Correlation analyses revealed strong connections between certain traits, such as protein content, and gluten index as well as between grain yield, and spike per square meter. Using the Multi-Trait Mean Performance Selection (MTMPS) index, the study identified six top-performing varieties. Among these, Antalis (G4) and Core (G18) consistently demonstrated strong adaptability and stability across different environments, particularly in hotter, drier conditions. Furio Camillo (G31) also exhibited valuable traits. This study highlights the challenges and complexities of breeding durum wheat for improved yield and quality in the face of climate change.
1 Introduction
Durum wheat (Triticum turgidum L.subsp. durum (Desf.) Husn.) is one of the most vital cereal crops globally, serving as a staple food that provides essential nutrients to a significant portion of the world’s population. In the 2022/23 season, global durum wheat production was projected to rise by 10% to 33.9 million metric tons (MMT), driven by significant contributions from North American countries such as the United States, Canada, and Mexico (Euronext, 2023). However, this increase was not uniform across all regions. While North America experienced favorable production levels, Europe – especially Spain and France – and North Africa faced declines due to adverse weather conditions, with the European Union’s production forecasted to drop to historically low levels (Euronext, 2023). Italy, the largest producer of durum wheat in Europe, has averaged around 4.26 million tons annually over the past decade. Despite this strong production history, Italy’s durum wheat output was estimated to decrease by 8% to 3.5 MMT in 2023/24 due to severe drought conditions (Italianfood, 2024). This shortfall has necessitated increased imports, particularly from the United States, to meet domestic demand. Durum wheat holds a prominent place in Italian agriculture due to its economic importance and cultural value. It is a key ingredient in traditional Italian cuisine, particularly as the exclusive raw material for pasta production – a main component of the Mediterranean diet, which has gained increasing global appreciation (Viggiani, 2009; Bux et al., 2022). The growing consumption of pasta has transformed it into a significant export product and a major symbol of the “Made in Italy” brand (Nicola and Scaccia, 2021; Boncinelli et al., 2023).
The Mediterranean climate of Southern Italy, characterized by hot, dry summers and mild, wet winters, is conducive to durum wheat cultivation. However, this climate is also highly variable, with recent trends indicating rising temperatures and shifting precipitation patterns, posing challenges to wheat yields and quality (Tataw et al., 2016; Carucci et al., 2023; Bonfil et al., 2023).
Climate change has various effects on wheat plant breeding by inducing heat stress, drought, and elevated CO2 levels. These factors lead to reduced grain quality, altered phenology, and decreased yield potential. Heat stress during the reproductive phase causes morphophysiological alterations, biochemical disruptions, and a reduction in the genetic potential of wheat (Kumar et al., 2017; Bishwas et al., 2021; Farhad et al., 2023). Moreover, drought and heat stress increase phytate contents, limiting essential mineral bioavailability, while elevated CO2 concentrations and other climatic events alter grain quality components and composition (Zahra et al., 2022). Rising temperatures and increased aridity also affect wheat phenological development by shortening the life cycle and impacting gluten accumulation, ultimately influencing grain quality (Elahi et al., 2022).
It is crucial to develop heat-tolerant wheat varieties and improve crop management practices to mitigate these adverse effects (Khalid et al., 2022). Achieving this requires a comprehensive understanding of GEI and the application of advanced breeding techniques that incorporate climate resilience traits. Scholars such as Mehmet et al. (2011); Karaman et al. (2023) and Ninou et al. (2024) have documented significant GEI for grain yield in wheat, highlighting the importance of understanding these interactions to optimize wheat breeding strategies and enhance yield stability and adaptability across diverse environments.
Evaluating GEI is particularly critical on a smaller scale, where the goal is to select varieties that not only perform consistently across multiple years but are also uniquely adapted to their specific local environments (Hanif et al., 2021; Kunze et al., 2024). A significant challenge in utilizing multiyear data is the inherent imbalance, as underperforming varieties are often excluded from further evaluation while promising ones continue to be assessed (Yan, 2015; Lado et al., 2016; Bernardo, 2020). This imbalance complicates analysis, necessitating the use of robust statistical methods to accurately interpret data and make informed breeding decisions.
Several statistical models have been developed to estimate genotype-environment interactions (GEI) in multi-environment trials (METs), with the additive main effects and multiplicative interaction (AMMI) model and the genotype main effects + genotype × environment interaction effects (GGE) model being particularly prominent (Scavo et al., 2023). The AMMI model combines additive main effects for genotypes and environments with multiplicative terms for G×E interaction, allowing for a comprehensive analysis of both main effects and interactions (Bishwas et al., 2021; Etana and Merga, 2021). The GGE model, on the other hand, focuses on genotype and G×E effects together, removing the environment main effects to emphasize genotype performance and stability across environments (Olanrewaju et al., 2021). Both models utilize biplot visualization techniques to aid in the interpretation of complex GEI patterns. Additionally, mixed model approaches using restricted maximum likelihood/best linear unbiased prediction (REML/BLUP) have gained traction for their ability to handle unbalanced data and incorporate pedigree information (Rout et al., 2022; Sugasawa and Kubokawa, 2023). These powerful methodologies are widely employed in plant breeding programs to estimate genetic parameters, predict genotypic values, and select superior genotypes for further cultivation (Lanna et al., 2022; Tajalifar and Rasooli, 2022; Costa et al., 2023). The choice between these models often depends on the specific research objectives and the nature of the data, with some researchers opting to use multiple approaches complementarily to gain a more comprehensive understanding of GEI patterns.
Traditional breeding programs often focused on single traits, primarily yield. However, this approach can led to unexpected problems such as increased susceptibility to diseases or reduced quality traits. Multi-trait selection, which considers a suite of desirable traits, offers a more holistic approach. This method aims to balance yield with other critical traits such as disease resistance, drought tolerance, and grain quality (Arnold, 2023). Integrating multi-trait selection into breeding programs can lead to the development of more resilient and high-performing wheat varieties.
The multi-trait mean performance and stability index (MTMPS) proposed by Olivoto et al. (2021) is a valuable tool in plant breeding for identifying genotypes with consistent performance across multiple environments. This index uses factor analysis to assess each ideotype’s scores, considering both desirable and undesirable traits. By computing the Euclidean distance between accessions and the ideotype, a spatial probability is derived, facilitating accession ranking. Varieties with the lowest MTMPS values are closer to the ideotype, indicating superior mean performance and stability across all analyzed variables (Olivoto et al., 2019).
Optimizing multi-trait selection indices allows breeders to address trade-offs between traits like grain yield and protein content, as well as combine traits of interest like yield and weed competitive ability. This approach can lead to continued genetic gain while maintaining genetic diversity in wheat populations (Silva et al., 2023). Additionally, including drought-tolerance indices in multi-trait selection strategies has been shown to provide superior gains in grain yield under stress conditions, emphasizing the importance of considering multiple traits in wheat breeding to enhance resilience to environmental challenges.
Therefore, this study aims to: (i) evaluate the GEI in wheat varieties across multiple traits, and (ii) identify superior varieties that demonstrate high performance and genotypic stability in Southern Italy under diverse environmental conditions, utilizing the MTMPS index.
This study adds significant value to the existing body of knowledge by employing advanced statistical techniques, addressing the complexities of long-term and unbalanced datasets, and providing a comprehensive multi-trait evaluation of durum wheat performance under Mediterranean climatic conditions. These contributions highlight the importance of multi-environment trials and advanced selection indices in breeding programs, offering practical insights for enhancing durum wheat resilience and productivity.
2 Materials and methods
2.1 Experimental site
A field screening experiment was conducted over ten years (2013–2022) at the experimental research station of the Department of Agriculture, University of Naples Federico II, located in Sant’Angelo dei Lombardi (40°55’12.0”N, 15°07’12.0”E, 667 m above sea level). This rural site, situated in the foothills of the Apennine Mountain range in the province of Avellino, southern Italy, experiences a warm-summer Mediterranean climate (Csb) as per the Köppen-Geiger classification (Beck et al., 2018). Utilizing 43 years of daily climatic data from the NASA-Power project (https://power.larc.nasa.gov/) covering the period from 1981 to 2023, this region displays an average daily temperature of 14.02°C (± 0.60°C) and an average annual precipitation of 572.36 mm (± 119.38 mm). The soil in this area is predominantly clay loam in texture (clay, sand, and silt: 38.8%, 36.9%, and 24.3%, respectively). The chemical and physical characteristics of the soil at the beginning of the experiment at 0.20 m of soil depth were as follows: pH 8.07, Kjeldahl total nitrogen 0.97 g kg−1, phosphorus pentoxide (P2O5: Olsen method) 14.4 mg kg−1, and soil organic matter (SOM) content of 1.16%.
2.2 Climate influence assessment
The daily key weather parameters for each wheat growing season at the Sant’Angelo dei Lombardi location, including, precipitation, relative humidity, air temperature, and solar radiation, along with 11 additional climatic variables (Table 1), were obtained from the NASA-Power project (https://power.larc.nasa.gov/). The weather regime, including monthly precipitation (P) and minimum and maximum temperatures (Tmin and Tmax) for the ten growing seasons, is detailed in Supplementary Table S1. These data are compared against the historical means from 1981 to 2023. To precisely reflect the temporal dynamics of environmental conditions throughout crop development, the crop cycles were segmented into five main phenological stages based on days after sowing (DAS): 0 to 21 days (Emergence), 22 to 110 days (Tillering), 111 to 140 days (Jointing), 141 to 184 days (Heading), and 185 to 217 days (Maturity). These intervals were determined through field observations of various wheat varieties grown in Sant’Angelo dei Lombardi over ten growing seasons. This approach resulted in the generation of 900 combinations of environmental covariates (18 weather parameters) across growing seasons (10 years) and main phenological stages (5 time periods), providing a comprehensive dataset for analyzing climate impacts on wheat development.
2.3 Agronomic management and experimental design
Eight-nine varieties were investigated. These varieties were certified by ENSE (Ente Nazionale Sementi Elette) and registered in either the national or community variety registry. Forty-eight varieties tested for less than three years were excluded from the analysis, leaving 41 varieties for genotype-by-environment interaction assessment. Not all varieties were evaluated every year, just 5 varieties were shared between all years (Figure 1). Consequently, the dataset is highly unbalanced, with 45% of the data missing. Comprehensive genealogy records, crop cycles, and breeding institutions for all 41 durum wheat varieties are detailed in Supplementary Table S2. Each year, a set of 23 ± 3 varieties was evaluated, ensuring that at least five varieties were common across all years (Figure 1). The trials were implemented using an experimental block design (RCBD), wherein varieties were randomized across the plots and replicated three times. The size of the plots was 5 m × 2 m. Sowing density was standardized at 400 grains per square meter for each variety. All varieties were grown under rainfed conditions. Each year, broad beans served as the preceding crop for wheat. Prior to sowing, phosphorus (P) and potassium (K) were applied in the form of superphosphate and potassium sulfate, respectively, at a rate of 100 kg ha−1. The specific amounts of nitrogen applied as urea, as well as the sowing and harvest dates, are detailed in Table 2.
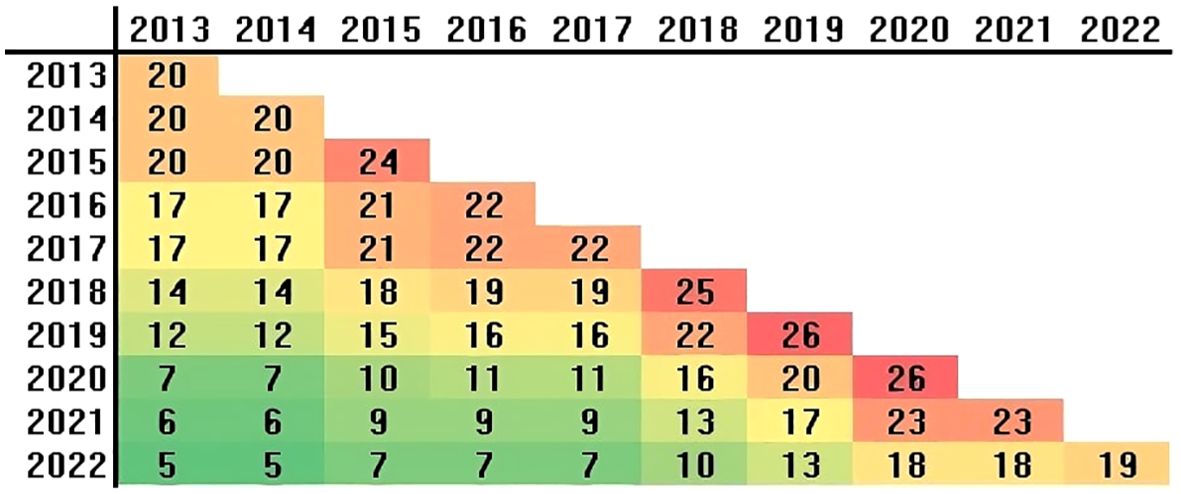
Figure 1. Connectivity of wheat varieties, measured by the number of shared varieties between years (below diagonal) and the total number of varieties per year (diagonal).
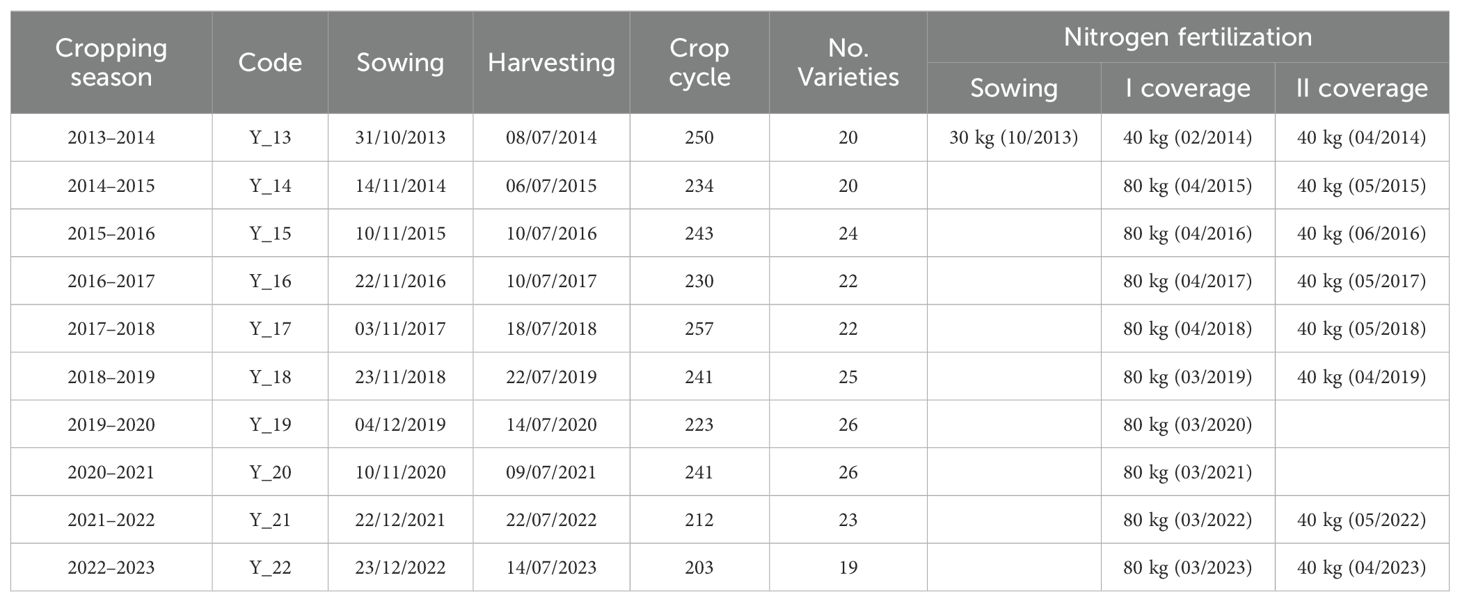
Table 2. Sowing date, harvesting, crop cycle and number of varieties, as well as amounts of nitrogen at the experimental site.
2.4 Phenotypic and quality traits
At harvest, a 1 square meter subplot in the center of each plot was hand-harvested to determine nine phenotypic and quality traits: 1) grain yield (GY), adjusting the moisture to 13% and converting the unit to tons per hectare (t ha−1), 2) plant height (Alt), measured using a graduated scale from ground level to the tip of the tallest spikelet and is expressed in meters (m), 3) Thousand-gain weight (TGW), is expressed in gram (g), 4) number of ears per square meter (Sp, num m−2), 5) hectoliter weight (Pe) was determined according to the AACC method 55-10.01 (Cereals and Grains Association, 2024) and reported in kilograms per hectoliter (kg/hL), 6) crude protein concentration (Pro), determined using the Kjeldahl method according to AACC 46-10.01 (Cereals and Grains Association, 2024). The Jones factor was used to convert it to protein (6.25) (Mariotti et al., 2008) and expressed in percentage (%), 7) gluten index value (Glu), analyzed according to AACC 38-12.02 (Cereals and Grains Association, 2024), and expressed in percentage (%), 8) shriveled wheat grain (Cs), referring to grain that is underdeveloped, small, and often misshapen expressed in percentage (%), and 9) bleached wheat grain (Cb), referring to wheat grain that has been exposed to environmental conditions causing them to lose their natural color, resulting in a paler, often whitish appearance, expressed in percentage (%).
2.5 Statistical analysis
2.5.1 Climatic impact on crop development
Principal component analysis (PCA) using the correlation matrix was employed to elucidate the characteristics of each of the ten consecutive growing seasons in relation to their associated environmental variables. These variables were collected using the `get_weather()` function from the R package EnvRtype (Costa-Neto et al., 2021). The PCA was conducted with the software packages FactoMineR (Husson et al., 2014) and psych (Revelle, 2024) within the RStudio environment (R Core Team, 2013). Hierarchical cluster analysis (HCA) was also performed using the `pheatmap` package (Kolde, 2019) in RStudio. This analysis utilized a single linkage approach and Euclidean distances to determine the similarities between the ten growing seasons. For a more detailed analysis of the climate data throughout the study duration, we utilized the env_typing() function from the EnvRtype package (Costa-Neto et al., 2021) to establish environmental patterns using quantile thresholds of 18 environmental covariates collected from sowing to harvest each growing season. Frequency distributions for each year and main phenological stage combination were computed using the 0.25, 0.50, and 0.75 quantiles. This approach facilitated the identification of extreme values for each environmental variable.
2.5.2 Variance estimation with random effects model
A comprehensive evaluation was conducted on 41 durum wheat varieties to estimate variance components and predict genotypic values along with genotype-by-environment interactions (GEI). The random model applied in this analysis was as follows:
where is the response variable (e.g., grain yield) observed in the kth block of the ith genotype in the jth year (i = 1, 2, …, g; j = 1, 2, …, e; k = 1, 2, …, b); μ is the general mean; is the random effect of the ith genotype; is the random effect of the jth year; is the random interaction effect of the ith genotype with the jth year; } is the random effect of the kth replicate within the jth environment; and ϵijk is the random error.
The Restricted Maximum Likelihood (REML) method (Dempster et al., 1977) was employed for variance component analysis. The significance of the random effects was evaluated using the likelihood ratio test (LRT). This test compares −2 times the residual log-likelihood (−2(REG)log) of two models: one model contains all the random effects, whereas the other model omits a single random effect. Additionally, four heritability estimates were obtained to assess the genetic contribution to observed phenotypic variance. These estimates include:
Broad-sense heritability (H2), which is the ratio of genetic variance to total phenotypic variance, indicates the proportion of observed variation in a trait that is due to genetic factors:
where: is the genetic variance, representing the portion of phenotypic variance attributed to genetic differences among individuals. is the total phenotypic variance, which includes both genetic and environmental variance ().
Heritability of mean genotype (h2gm), also known as the broad-sense heritability for the mean genotype, can be calculated using the formula:
where e and b are the numbers of environments and blocks, respectively.
Heritability of Cullis et al. (2006) is a robust methodology for estimating heritability in the presence of unbalanced data and can be calculated using the formula:
where is the mean standard error of the genotype BLUPs and Heritability of Piepho and Möhring (2007) is a robust methodology for estimating heritability in the presence of unbalanced data and can be calculated using the formula:
where is the mean variance of a difference of two best linear unbiased estimators (BLUE).
The REML/BLUP methodology was conducted using the metan package in RStudio (R Core Team, 2013), employing the functions gamem_met() (Olivoto and Lúcio, 2020). Heritability estimates were determined using the H2cal() function from the inti package (Lozano-Isla, 2021).
2.5.3 Mean performance and stability analyses
Durum wheat varieties were ranked using the Mean Performance and Stability (MPS) method for an individual trait across varying environments, which incorporates a linear mixed-effects model (LMM) structure to offer innovative graphical insights for concurrent selection. In this investigation, this method evaluates genotype-by-environment interactions (GEI) employing the stability approach proposed by Eberhart and Russell (1966), which is particularly effective for analyzing unbalanced data (Pour-Aboughadareh et al., 2022). According to Sampaio Filho et al. (2023), performance and stability were assessed using the Weighted Average Absolute Scores of BLUPs (WAASB) method, with stability adjustments considering genotypic stability of deviations from regression (S2di), the root mean square error of the regression (RMSE), and the coefficient of determination (R2). The main goal here is to rank different genotypes based on their stability and performance in terms of one specific trait, such as yield, protein content, or any other trait of interest, which limits its application when multiple traits need to be considered simultaneously. Before calculating the MPS index, we adjusted (rescaled) the agronomic performance and stability matrix so they could be easily compared using the following model:
For agronomic performance:
For the stability index:
where nmax and nmin are the new maximum and minimum values of the variables and the MPS index (S2di, RMSE, and R2) after rescaling; and are the original values for the response variable and MPS index of the genotype i, respectively.
For the S2di and RMSE indices, genotypes with values of zero for both were ranked as the most stable. To adjust the environmental index, ERmax was set to 0 and ERmin to 100. On the other hand, for the R2 parameter, genotypes with R2 values close to 1 were considered well-adapted, so the values were set to and . As a result, a genotype with the highest average and highest R2 would receive = =100. An exception applies to the “Alt,” “Cb,” and “Cs” traits, where smaller values are better, so Ymax=0 and Ymin=100 for these traits.
After adjusting the values for mean performance and stability (), the best-performing genotype was given a score of 100, while the worst-performing one received a score of 0. Likewise, the genotype with the most stability was also scored 100, and the least stable genotype received a score of 0. This created a two-way table with scores ranging from 0 to 100 across the columns, matching the direction of the selection process. After that, the MPS was computed using this formula:
where refers to the superiority index for genotype I, which weights between mean performance and stability; the values and represent the weights for mean performance and stability, respectively; the terms and are the rescaled values for mean performance and stability (), respectively of the genotype i. In this context, we used =70 and =30 to give more importance to mean performance at the MTMPS index.
Next, the MTMPS index was used to calculate the mean performance and stability of multiple traits. The MTMPS index extends the MPS approach by integrating multiple traits into the stability analysis. This index provides a more holistic evaluation of genotypes compared to the single-trait MPS approach. This approach is based on factor analysis and ideotype design, where the factorial scores of each ideotype are designed according to the desirable and undesirable factors. Then, a spatial probability is estimated based on genotype-ideotype distance, enabling genotype ranking. The results allowed for conducting a single and straightforward selection process of durum wheat varieties. The MTMPS index was computed using this model:
The refers to the multi-trait stability index for each genotype, while Fij is the j-th score for the i-th genotype, with i ranging from 1 to g (the total number of genotypes) and j ranging from 1 to f (the number of factors). Fj represents the j-th score of the ideal genotype. This approach aimed to identify varieties with superior values (positive gains) for grain yield (GY), thousand-grain weight (TGW), spike number (Sp), plant height (Pe), protein content (Pro), and gluten content (Glu). Consequently, the variety with the lowest MTMPS score is considered closest to the ideotype, demonstrating high performance and stability in all measured traits. Additionally, the study calculated the selection differential () for the chosen varieties, assuming a selection intensity of 15%. The calculation used the formula:
where is the value of the selected genotypes and is the average value of the population. We employed the mps() and mtmps() functions from the metan package (Olivoto and Lúcio, 2020) in RStudio (R Core Team, 2013) to perform this analysis.
3 Results
3.1 Climatic impact on crop development
Figure 2 provides a comprehensive multivariate analysis of weather parameters and wheat traits over several years. The first two principal components (Dims), which have eigenvalues greater than one, account for 88.58% of the total variance after varimax rotation. Dim1 explains 59.24% of the variance, while Dim2 accounts for 29.34% (Figure 2A). Dim1 is positively correlated (>0.5) with most major weather parameters except for precipitation (PRECTOT), wind speed (WS), and dew-point temperature (T2MDEW), which are positively correlated with Dim2 (Supplementary Table S3). This analysis highlights significant climatic variability between growing seasons. A positive correlation was identified among minimum temperature (Tmin), fraction of radiation use efficiency (FRUE), vapor pressure deficit (VPD), maximum temperature (Tmax), mean temperature (Tmed), growing degree days (GDD), evapotranspiration (ETP), sunshine duration (N), and radiation at the top of the atmosphere (RTA). Conversely, these climate parameters showed a negative correlation with total precipitation (PRECTOT), precipitation deficit (PETP), and relative humidity (RH) (Supplementary Table S4).
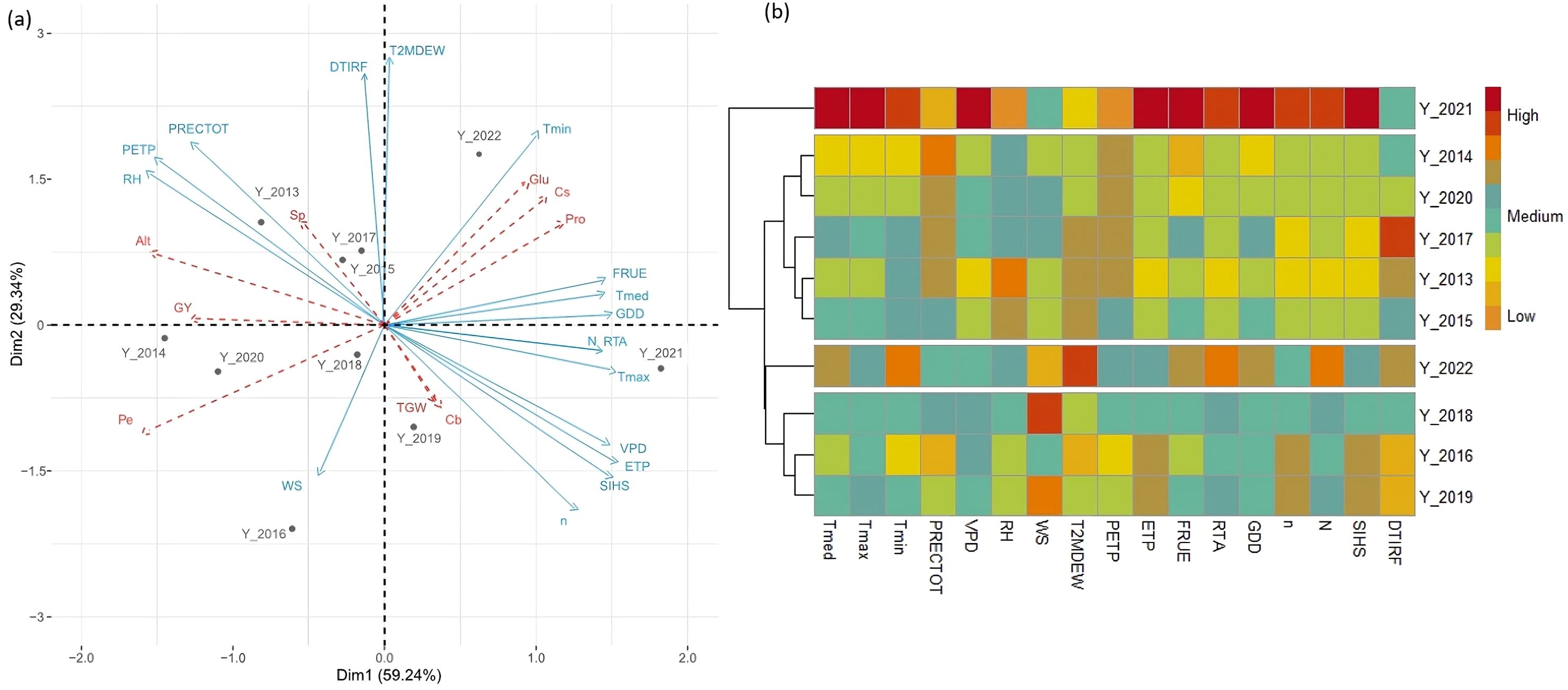
Figure 2. (A) Principal component analysis (PCA) biplot of weather parameters as a function of cropping seasons. Principal variables are indicated by blue arrows, while supplementary variables (morphologic and quality traits) are represented by dotted red arrows. For weather parameter abbreviations, refer to Table 1. Key traits include: GY, grain yield; Alt, plant height; TGW, 1000-gain weight; Sp, number of ears per square meter; Pe, hectoliter weight; Pro, crude protein concentration; Glu, gluten index value; Cs, shriveled wheat grain; and Cb, bleached wheat grain. (B) Heat map showing the similarity between ten consecutive cropping seasons (2013–2014 to 2022–2023) based on 17 environmental covariates.
Based on the hierarchical cluster analysis of 17 weather parameters, four distinct groups of growing seasons were identified, each characterized by specific climatic conditions (Figure 2B). Group 1 (Y_2021) is marked by high values for most weather parameters, including maximum and minimum temperatures (Tmax, Tmin), average temperature (Tmed), vapor pressure deficit (VPD), evapotranspiration (ETP), fractional radiation use efficiency (FRUE), radiation absorption (RTA), growing degree days (GDD), daylight hours (N), actual duration of sunshine (n), and insolation incident (SIHS), further signifying very warm and dry conditions. Group 2, comprising the seasons Y_2014, Y_2020, Y_2017, Y_2013, and Y_2015, shows lower temperatures and higher precipitation (PRECTOT), relative humidity (RH), and a precipitation deficit (PETP), suggesting a cooler environment with favourable water availability. Group 3 (Y_2022) is distinguished by high values for dew-point temperature (T2MDew), the effect of temperature on radiation use efficiency (FRUE), sunshine duration (N), radiation at the top of the atmosphere (RTA), Growing Degree Day (GDD), minimum and mean temperatures (Tmin, Tmed), and thermal infrared (longwave) radiative flux (DTIRF). These high values indicate a warm environment during this growing season. Finally, Group 4 (Y_2018, Y_2019, Y_2016) is characterized by higher wind speed (WS), evapotranspiration (ETP), sunshine duration (N), and insolation incident (SIHS), along with moderate temperatures, precipitation, and humidity, delineating an intermediate environment.
During the warm years (2021 and 2022), higher vapor pressure deficit (VPD) values were frequently observed, particularly during the heading and maturity stages, with the maximum VPD reaching 2.83 kPa day−1 (Figure 3A). High values of maximum temperature (Tmax) ranging from 22.4°C to 35.9°C were evident during almost the entire heading and maturity stages (Figure 3C). Both high VPD and Tmax values, causing heat and drought stress, can impair photosynthesis, reduce pollen viability, decrease grain setting, and ultimately impact yield. The precipitation deficit (PETP) data during these years show notable deficits, with values ranging from −13.9 to −9.35 mm day−1 observed on approximately more than 80% of the days during the heading and maturity stages (Figure 3B). This exacerbates the effect of both heat and drought stress, and consequently reducing grain yield.
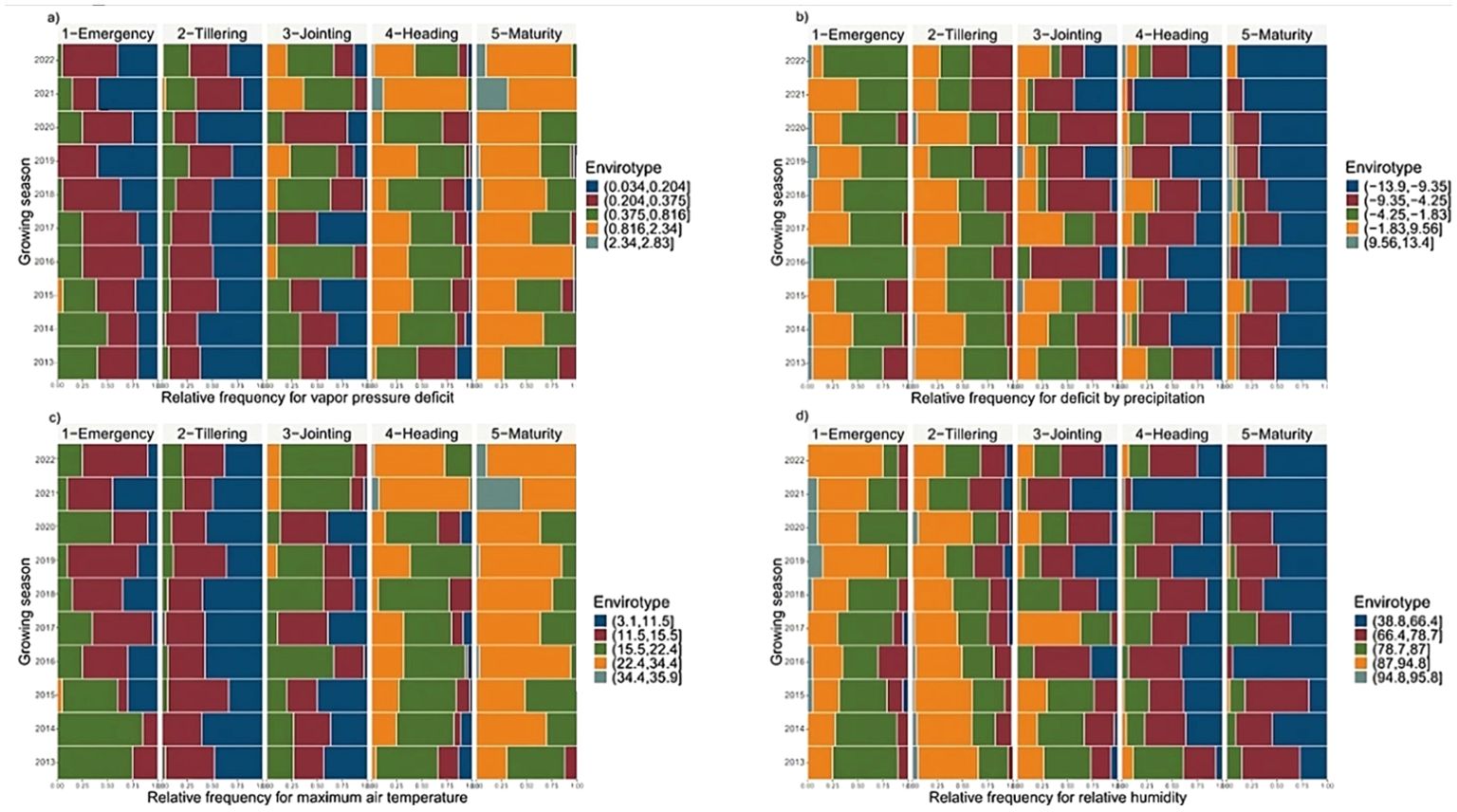
Figure 3. Framework for envirotyping analysis of: (A) vapor pressure deficit (kPa day−1), (B) deficit by precipitation (mm day−1), (C) maximum air temperature (Tmax, °C day−1), and (D) relative humidity (%) impact on durum wheat across 10 growing seasons and 5 growth stages (emergency, tillering, jointing, heading and maturity).
In contrast, cooler years (2013, 2014, 2015, 2017, and 2020) exhibited lower VPD and Tmax values during almost 80% of the heading stage, with values ranging from 0.38 to 0.82 kPa day−1 and 15.5 to 22.4°C, respectively (Figures 3A, C). This suggests reduced stress from high temperatures and dry air. These conditions are more conducive to optimal plant development and grain filling. Additionally, in these years, PETP values ranging from −1.83 to 13.4 mm day−¹ were displayed on 13% of days during the heading and maturity stages, indicating a certain availability of water in these two critical phenological stages (Figure 3B). Higher relative humidity (RH) observed during the cooler years, particularly at the emergence and tillering stages, showed maximum frequency distributions between 78.7% and 95.8% (Figure 3D), which can foster better early growth and establishment.
3.2 Variance estimation with random effects model
The likelihood-ratio test (LRT) revealed that only six durum wheat traits (GY, Sp, TGW, Alt, Pe, and Cb) had significant effects of environment, genotype, and genotype by environment interaction effects (Table 3). Additionally, all traits, except gluten, grain protein, and shriveled wheat grain, showed significant genotypic effects (Table 3). The genotype × environment interaction was significant for all traits, demonstrating the varying responses of different varieties to distinct growing seasons (Table 3).
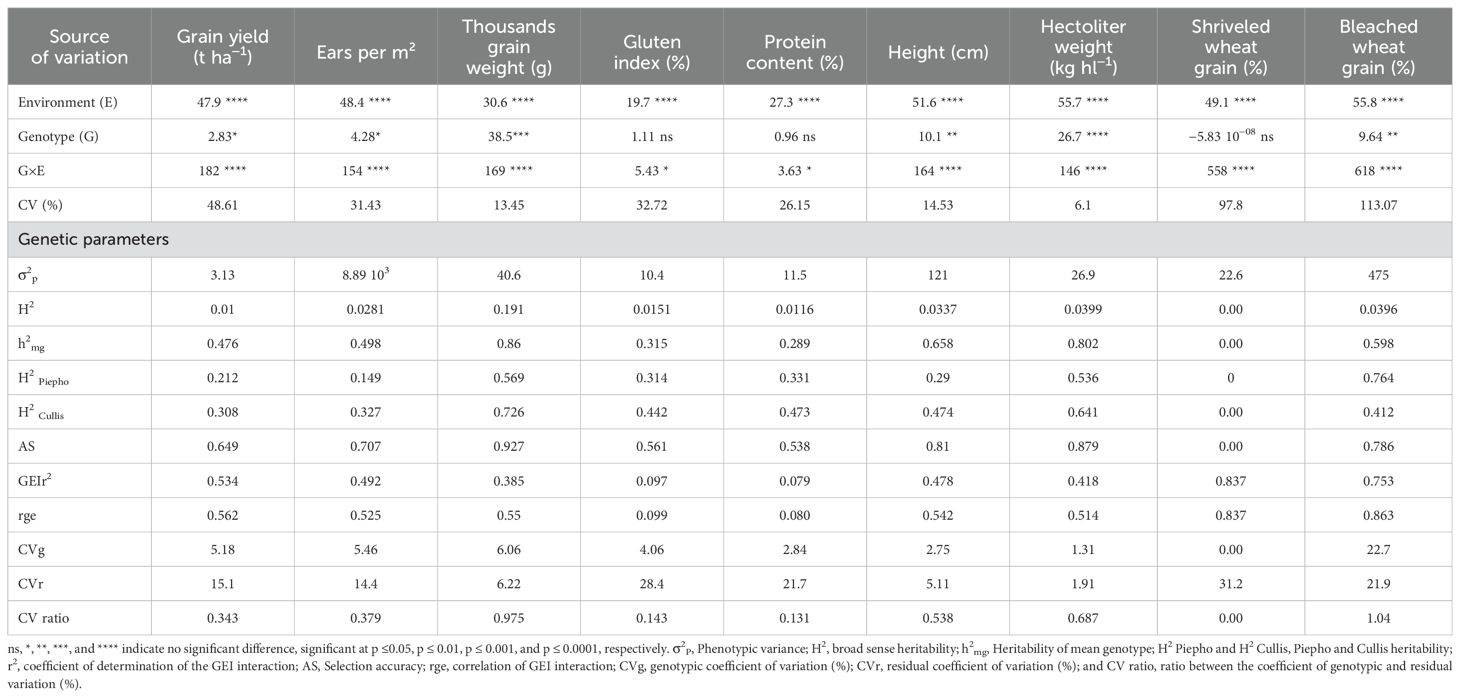
Table 3. Likelihood ratio test, estimated variance components and genetic parameters for nine traits of 41 wheat varieties. evaluated in 10 growing seasons.
In the analysis of durum wheat traits, it was observed that, with the exception of gluten index, grain protein content, and shriveled wheat grain, the environmental variance (σe2) contributed substantially more to the total phenotypic variance (σ2P) than any other traits examined (Figure 4). The genotype-by-environment interaction (GEI) variance was stronger on shriveled wheat grain traits, accounting for 50% of the overall phenotypic variation. For gluten content and grain protein, the residual variance (σr2) was more substantial than the other variance components (Figure 4).
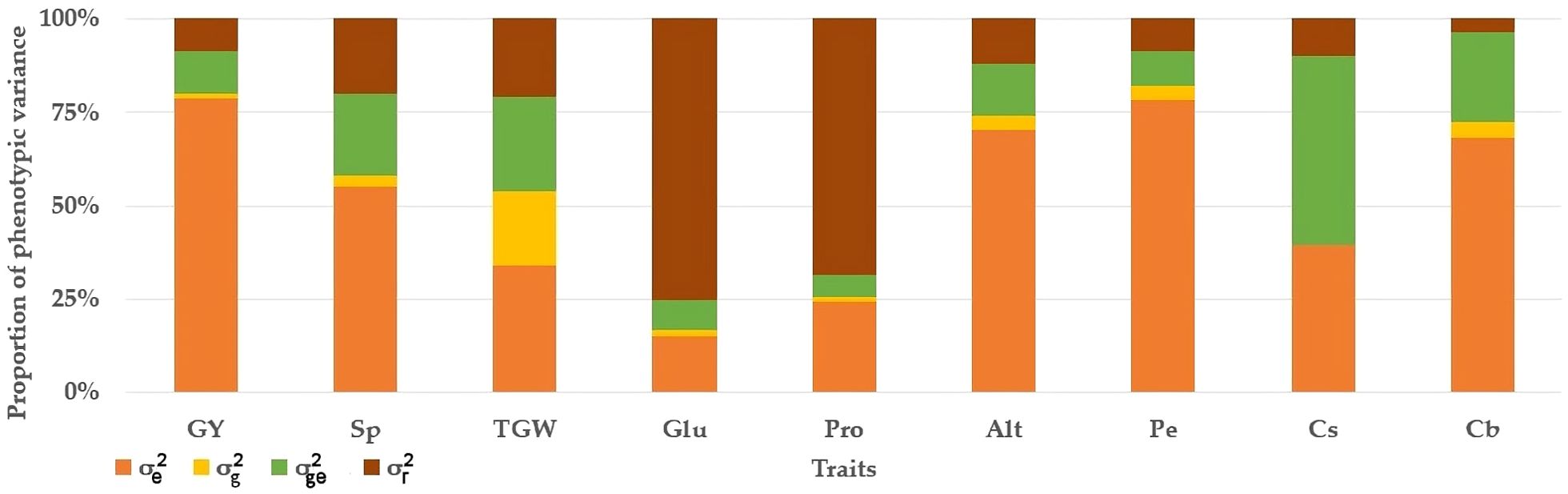
Figure 4. Proportion of the phenotypic variance for nine wheat traits evaluated during ten consecutive growing seasons. GY, grain yield; Sp, ears per m2; TGW, 1000 grain weight; Glu, gluten index; Pro, protein content; Alt, height; Pe, hectoliter weight; Cs, shriveled wheat grain; Cb, bleached wheat grain. σe2, environment variance; σg2, genotype variance; σge2, interaction g × e variance; σr2, residual variance.
The distribution of grain yield for each variety is illustrated in Figure 5. Wheat grain yield varied significantly across years and varieties, with an average yield of 3.42 ± 1.66 t ha−1 among the 41 tested varieties. The lowest yield was recorded in 2021 at 1.66 t ha⁻¹, while the highest yield was observed in 2014 at 6.09 t ha⁻¹ (Supplementary Figure S1). Grain yield (GY) ranged from 1.09 t ha⁻¹ (variety G75 in 2018) to 7.22 t ha⁻¹ (variety G65 in 2014), with a coefficient of variation (CV) of 48.61%. The coefficient of variation for durum wheat traits between varieties over the ten growing seasons showed maximum variability for traits such as bleached wheat grain (113.07%), shriveled wheat grain (97.8%), gluten content (32.72%), spikes per m2 (31.43%), and grain protein (26.15%). Minimum variability was observed for hectoliter weight (6.1%), thousand-grain weight (13.45%), and plant height (14.53%).
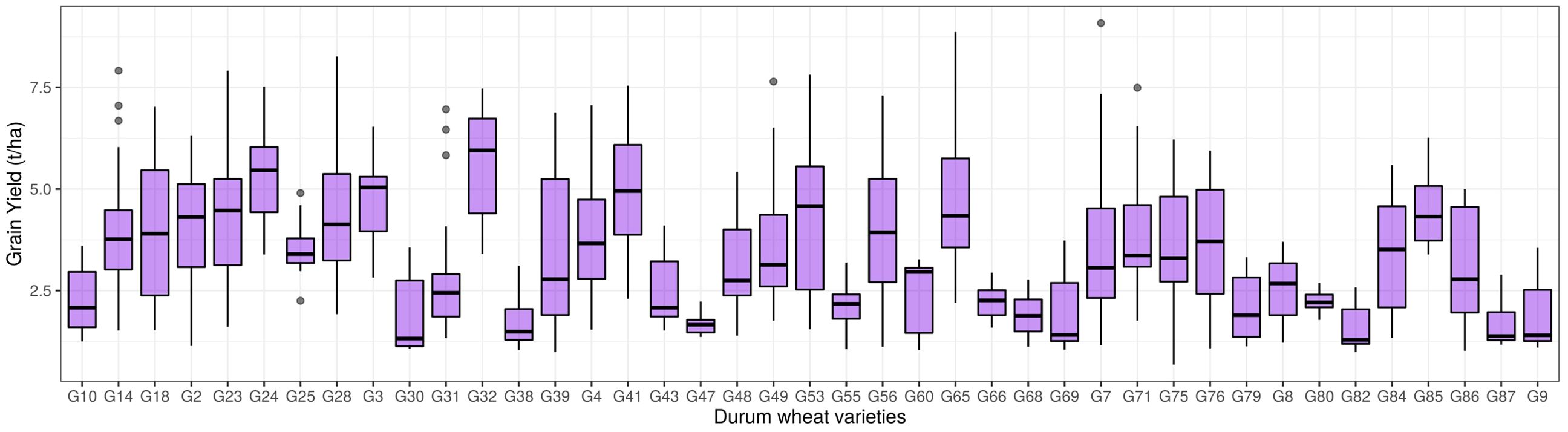
Figure 5. Distribution of grain yield (t ha⁻¹) for 41 wheat varieties test across ten consecutive crop seasons (2013–2014 to 2022–2023) in southern Italy.
The broad-sense heritability (H²) values for all traits were generally low, ranging from 0 for shriveled wheat grain to 0.19 for thousand-grain weight, indicating that most of the variation in these traits is likely due to environmental or other non-genetic factors. Conversely, the estimate heritability of the mean genotype (h²mg) varied, being low for gluten index, grain protein, and shriveled wheat grain (ranging from 0 to 0.32), moderate for grain yield, ears per square meter, height, and bleached wheat grain (ranging from 0.48 to 0.66), and high for thousand-grain weight and hectoliter weight (ranging from 0.80 to 0.86).
Regarding Piepho heritability (H² Piepho), only thousand-grain weight and ears per square meter showed moderate heritability (ranging from 0.54 to 0.57), while bleached wheat grain exhibited high heritability (0.76), with the remaining traits showing low heritability. For Cullis heritability (H² Cullis), five traits – gluten index, grain protein, height, ears per square meter, and bleached wheat grain – had moderate heritability (ranging from 0.41 to 0.64), with thousand-grain weight showing high heritability (0.72), and the remaining traits displaying low heritability. These differing heritability estimates highlight the complex nature of heritability and the importance of considering multiple perspectives when evaluating genetic contributions to trait variation.
The selection accuracy (AS) exceeded 0.70 for all traits except gluten index, protein content, and shriveled wheat grain. This high selection accuracy indicates reliable identification and selection of superior genotypes for breeding, ensuring effective genetic improvement in these traits.
The correlation of genotype–environment interaction effects (rge) varied in magnitude, being low for gluten index, grain protein, and thousand-grain weight (ranging from 0.08 to 0.39), moderate for grain yield, spikes per square meter, height, and ears per square meter (ranging from 0.42 to 0.53), and high for shriveled wheat grain and bleached wheat grain (ranging from 0.75 to 0.84). The genotypic coefficient of variation (CVg) exhibited a wide range, from 0% for Cs to 22.7% for Cb, demonstrating significant genetic diversity among the varieties. Conversely, the residual coefficient of variation (CVr) ranged from 1.91% for ears per square meter (Pe) to 31.2% for shriveled wheat grain (Cs). Additionally, the ratio between the genotypic and residual coefficients of variation (CVratio) was below 1 for all traits except for bleached wheat grain (Cb).
3.3 Factor analysis of durum wheat traits
Due to the data being unbalanced, Sampaio Filho et al. (2023) recommend evaluating performance and genotypic stability using factorial analysis, and they suggest focusing on the deviations from regression (S2di), the coefficient of determination (R²), and the root mean square error of the regression (RMSE). This approach is preferred over the originally proposed WAASB index method. According to Table 4, three factor components (FA) were identified, explaining 68.61%, 63.87%, and 69.55% of the total variance for S2di, R², and RMSE, respectively, in durum wheat traits.
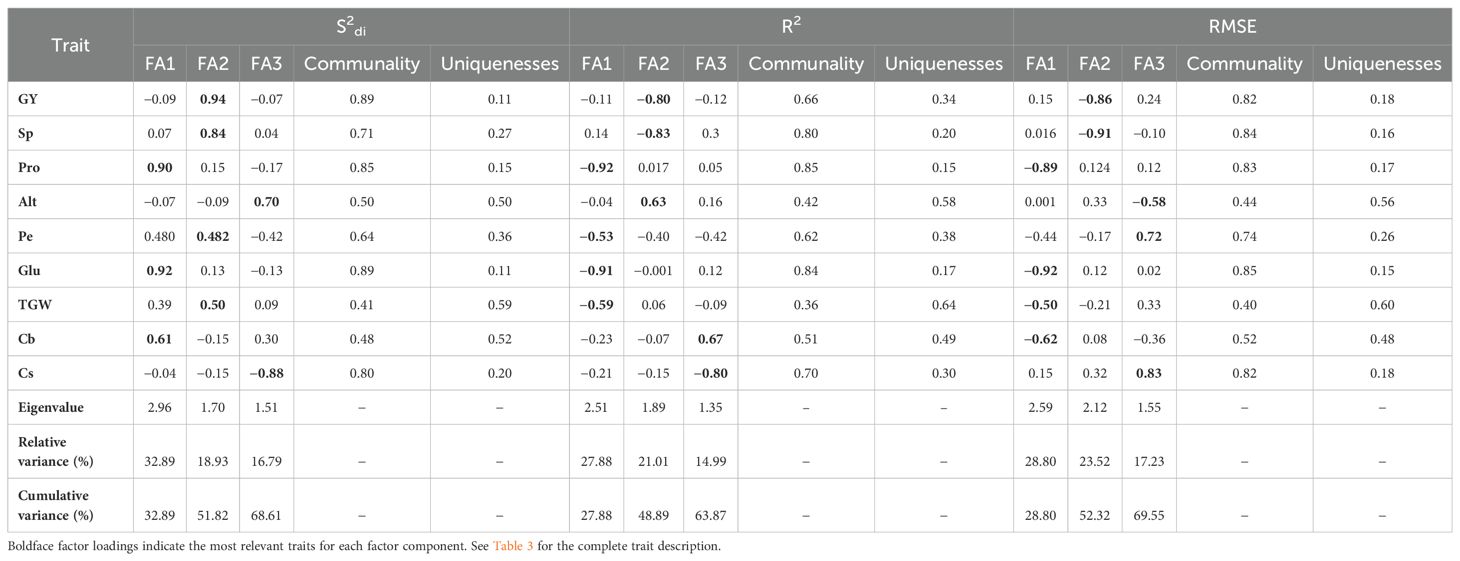
Table 4. Factorial analysis of 9 durum wheat traits: assessing regression deviations (S2di), regression determination coefficient (R2), and square root mean square error (RMSE) with a weighted emphasis of 70% on performance and 30% on stability.
In our factor analysis, we evaluated the communality (h2i) and uniqueness (u2i) of each variable to understand how well they are represented by the common factors. Communality indicates the proportion of a variable’s variance explained by these factors, while uniqueness shows the variance not explained by them. The average h2i values were 0.69 for S2di, 0.64 for R², and 0.70 for RMSE. For S2di, all durum wheat traits had h2i values greater than 0.5 except for TGW and Cb. For R² and RMSE, all traits had h2i values above 0.5 except for Alt and TGW. This indicates that most traits are well represented by the common factors in our analysis.
The nine durum wheat traits were grouped into three factors (FA) for each stability parameter. For S2di, the first factor (FA1) showed a strong positive correlation with important quality traits, such as the gluten index (0.92) and protein content (0.90), and a moderate correlation with bleached wheat grain (0.61). The second factor (FA2) included traits related to plant productivity, like grain yield (0.94) and ears per m² (0.84), and some quality traits such as thousand-grain weight (0.50) and hectoliter weight (0.48). The third factor (FA3) had a strong negative correlation with the quality trait shriveled wheat grain (−0.88) and a significant positive correlation with the morphological trait of plant height (0.70).
For R² and RMSE parameters, FA1 was associated with quality traits, including protein content (−0.92), gluten index (−0.91), and thousand-grain weight (−0.59) for R², and gluten index (−0.92), protein content (−0.89), bleached wheat grain (−0.61), and thousand-grain weight (−0.50) for RMSE. The second factor (FA2) grouped productive traits (grain yield (−0.80) and ears per m² (−0.83)) and the morphological trait of plant height (0.62) for R², and productive traits (grain yield (−0.86) and ears per m² (−0.91)) for RMSE. Finally, FA3 showed a high correlation with quality traits (bleached wheat grain (0.67) and shriveled wheat grain (−0.80)) for R², and with the morphological trait plant height (−0.58), and quality traits such as shriveled wheat grain (0.83) and protein content (0.72) for RMSE.
3.4 Correlations between agronomic and quality traits
Figure 6 illustrates Pearson’s correlation coefficients between various agronomic and quality traits. These correlations illustrate the relationships among traits using the mean performance and stability (MPS) index, which was established based on three stability parameters (S2di, R², and RMSE). Approximately 25% of the correlations were significant for both R² and RMSE, while 30.56% were significant for S2di. Traits show varying degrees of correlation depending on the stability parameter considered. Notably, protein content and gluten index exhibited a highly positive correlation (>0.88) across all stability parameters. Additionally, grain yield and spike per square meter (Sp) demonstrated a moderate positive correlation, with coefficients ranging from 0.52 to 0.70. There was a low positive correlation between shriveled wheat grain (Cs) and hectoliter weigh (Pe), with values ranging from 0.33 to 0.43. Conversely, plant height showed a low negative correlation with Cs for S2di (−0.36), Pe for RMSE (−0.33), and Sp for R² (−0.34). Thousand-grain weight (TGW) exhibited low positive correlations with several traits: grain yield (0.40), Pe (0.37), and gluten index (0.35) for S2di. For RMSE, TGW was positively correlated with Pe (0.46), and for R², it correlated positively with protein content (0.39) and gluten index (0.35). Pe also had low positive correlations with grain yield (0.35) and protein content (0.38) for R², with protein content (0.35) for RMSE, and with grain yield (0.38), Sp (0.38), and protein content (0.44) for S2di. Additionally, a low but significant positive correlation between bleached wheat grain (Cb) and both protein content and gluten index (0.32 and 0.40, respectively) was observed for the RMSE parameter.
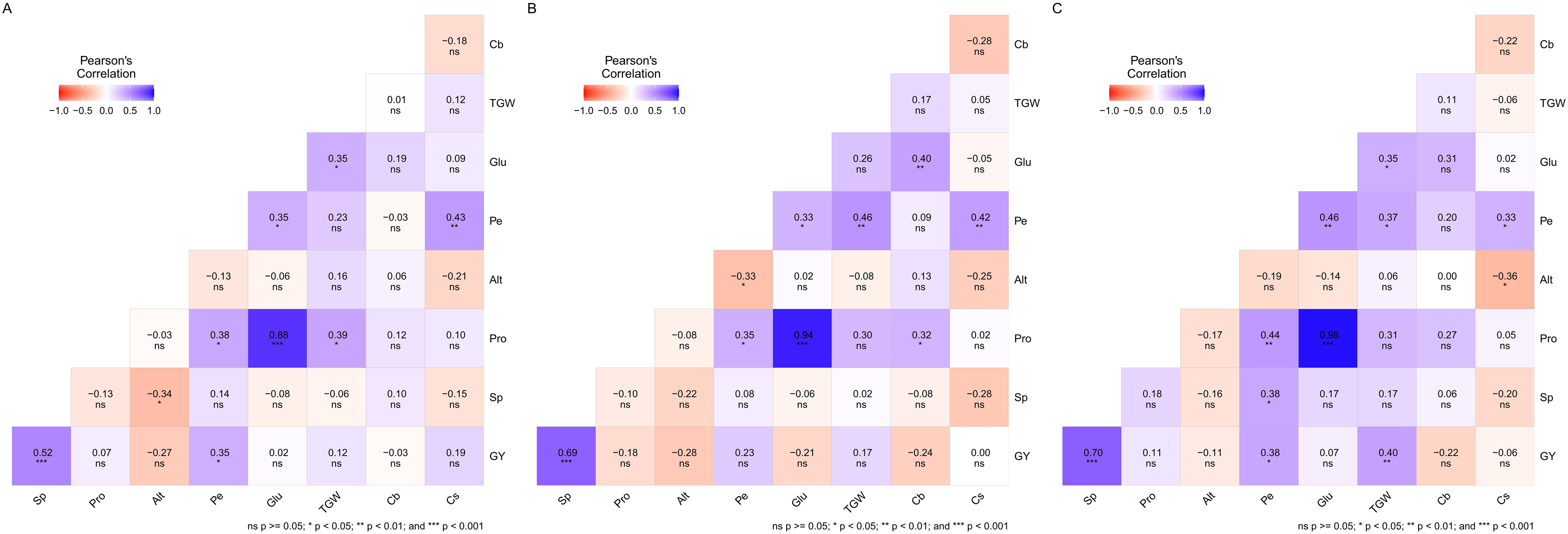
Figure 6. Pearson’s correlation coefficients between agronomic and quality traits for the mean performance and stability (MPS) index, with a 70% emphasis on performance and 30% on stability, for regression parameters R2 (A), RMSE (B), and S2di (C). Refer to Table 3 for the complete trait description.
3.5 Identifying superior genotypes with the MTMPS index
The ranking of the 41 durum wheat varieties based on the Multi-Trait Index (MTMPS) analysis, with a 15% selection intensity, is illustrated in Figure 7. Among the 41 varieties evaluated, six were selected for each of the three regression variance parameters (S2di, R², and RMSE). Regarding the R² parameter, the varieties with lower MTMPS values were Core (G18), Furio Camillo (G31), Antalis (G4), Bering (G9), Dylan (G24), Gibraltar (G32), and Anco Marzio (G3) (Figure 7A). For the RMSE parameter, Core (G18), Aureo (G7), Svevo (G76), Antalis (G4), Bering (G9), and Marco Aurelio (G49) were selected (Figure 7B). In the S2di parameter, the varieties with lower MTMPS values were Core (G18), Aureo (G7), Marco Aurelio (G49), Antalis (G4), Furio Camillo (G31), Claudio (G14), and Svevo (G76) (Figure 7C). These six varieties selected across three regression parameters demonstrated satisfactory mean grain yield performance. Specifically, their yields ranged from 3.41 t/ha in R², to 3.91 t/ha in RMSE, and 3.61 t/ha in S2di. Additionally, these varieties exhibited better stability in grain yield across different growing seasons, highlighting their resilience and consistency under varying environmental conditions.
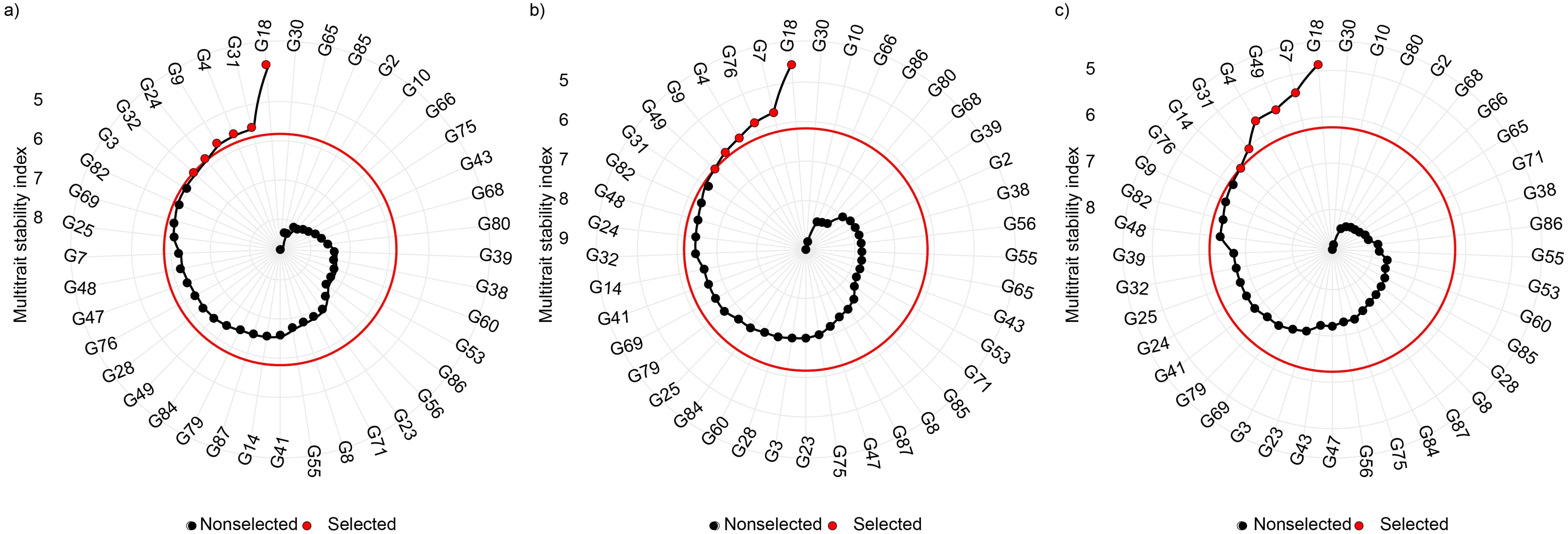
Figure 7. Multi-trait index (MTMPS) analysis of durum wheat varieties with 15% selection intensity (red circle), emphasizing 70% performance and 30% stability for regression parameters: R2 (A), RMSE (B), and S2di (C). Based on the Eberhart and Russell (1966) model. See Supplementary Table S1 for the complete variety description.
The multi-trait mean performance and stability index (MTMPS) proved highly effective in selecting traits with selection gain (SG) for all durum wheat traits, achieving a success rate of 77.77% (7 out of 9 traits) based on RMSE and R², and 88.89% (8 out of 9 traits) based on S2di, with a few exceptions. Notably, the trait Cb showed reductions of 4.95%, 10.17%, and 13.55% for S2di, RMSE, and R², respectively, indicating its favorable desirability as a wheat trait in this context. Specifically, the trait Cb exhibited reductions of 4.95%, 10.17%, and 13.55% for S2di, RMSE, and R², respectively, which is desirable wheat trait in this context. Additionally, Cs showed a slight reduction of 0.04% for S2di. Conversely, the trait Alt experienced increased gains of 0.33%, 1.21%, and 1.50% for RMSE, S2di, and R², respectively, which are not suitable for mechanized harvesting. For the parameters R² and RMSE, Cs increased by 1.92% and 4.46%, respectively, which negatively impacts durum wheat quality. Regarding S2di, the selection gain percentages for traits with desirable values ranged from 0.70% for Pe to 3.65% for TGW (Table 5). Similarly, the MTMPS index gains for the R² and RMSE parameters followed a comparable pattern, with increases in selection gains for six desirable wheat traits. For R², the gains ranged from 1.28% for Pe to 6.08% for Sp, while for RMSE, the gains ranged from 0.19% for Pe to 3.67% for Glu (Table 5).
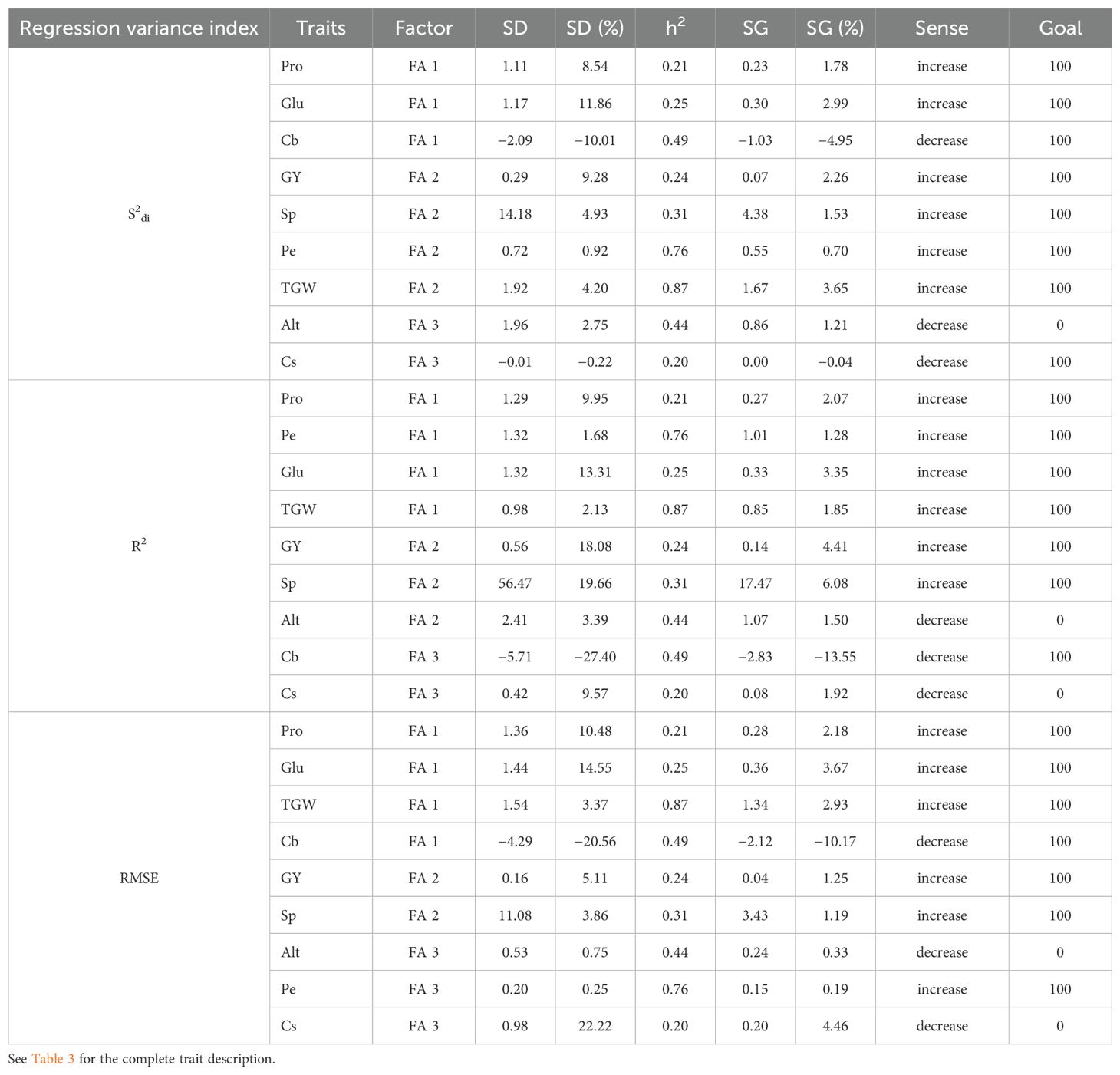
Table 5. Durum wheat traits, selection differential (SD), heritability (h2), and selection gain (SG) of selected varieties for the MPS index using three regression variance parameters (S2di, R2 and RMSE).
Figure 8 illustrates the strengths and weaknesses of selected durum wheat varieties identified over ten consecutive growing seasons. Based on the R² parameter, the “G31” variety exhibits strengths in FA1, indicating high performance in quality wheat traits such as protein (Pro), gluten (Glu), thousand-grain weight (TGW), and plant height (Pe). The variety “G4” shows strengths associated with FA2, which includes grain yield (GY), ears per m² (Sp), and plant height (Alt), while the variety “G9” excels in FA3, encompassing traits like shriveled wheat grain (Cs) and bleached wheat grain (Cb) (Figure 8A). For the RMSE parameter, FA1, which primarily relates to Pro, Glu, TGW, and Cb, significantly influences the deviation of the “G49” variety from the ideotype, suggesting areas for improvement in these traits. Meanwhile, the “G4” variety demonstrates strengths in both FA2 (GY and Sp) and FA3 (Alt, Pe, and Cs), indicating a well-rounded performance across these factors (Figure 8B). Regarding the S2di parameter, the “G18” variety performs very well in FA1, showing strong traits in protein (Pro), gluten (Glu), and bleached wheat grain (Cb). The “G14” variety shows robustness in FA2, associated with GY, Sp, and Pe. On the other hand, the “G31” variety presents a positive gain in traits related to Alt and Cs (Figure 8C).
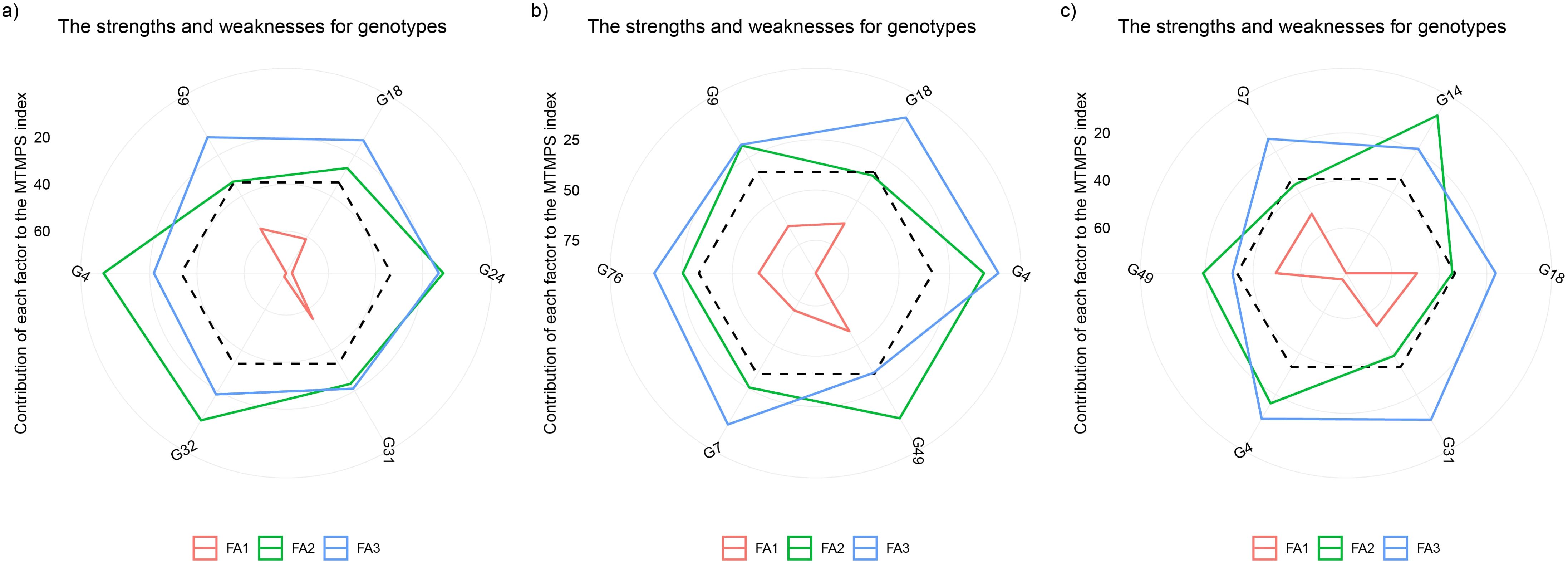
Figure 8. Comparative analysis of strengths and weaknesses of stable landraces across environments: proportion of each factor in the computed MTMPSI. Smaller proportions (closer to the outer edge) indicate greater similarity to the ideotype for the trait within that factor. The dashed line represents the expected value if all factors contributed equally. Refer to Table 4 for a detailed description of the factor analysis (FA). The 20, 25, 40, 50, 60, and 75 value indicate the contribution of each factor to the MTMPSI for each. Regression parameters: R2 (A), RMSE (B), and S2di (C), as per the Eberhart and Russell (1966) model.
4 Discussion
In recent years, Italy a country that suffers from drought for most of the year and where agriculture requires large amounts of water, especially for wheat cultivation, has been working for many years on developing new varieties of durum wheat that can resist drought and high temperatures due to the phenomenon of global warming, which has become a global concern.
In this study, the grain yield, agronomic traits, and quality parameters of 41 durum wheat varieties were assessed over ten consecutive growing seasons in Southern Italy, particularly in the foothills of the Apennine Mountains. The likelihood-ratio test indicated a significant effect of genotype by environment interaction (GEI) for all traits, demonstrating that the performance of these varieties was strongly influenced by different growing conditions. GEI significantly impacts grain yield in wheat, highlighting the complexity of breeding programs aimed at improving yield stability across diverse environments. Studies have consistently shown that wheat genotypes exhibit variable performance under different environmental conditions, underscoring the necessity of multi-environment trials to accurately assess their yield potential (Eltaher et al., 2021; Gupta et al., 2022; Khare et al., 2024).
The contrasting environmental conditions during warm and cooler years had a significant impact on wheat grain yield, agronomic traits, and quality parameters. The observed differences can be attributed to the interplay between various climatic factors and their effects on wheat development stages.
In warm and dry environments like those in 2021 and 2022, high temperatures, high vapor pressure deficit (VPD), and water deficit had profound impacts on agronomic and quality traits. Water stress, occurring on more than 20% of days during the tillering stage, reduced the number of tillers by 21%, flag leaf area by 43%, plant height by 12%, and dry matter by 19% compared to control treatments, highlighting the sensitivity of this stage to water deficits (Zabn and Alsajri, 2022). High temperatures and water stress during the jointing stage accelerated the development process, leading to shorter periods for biomass accumulation and potentially reducing overall plant height and yield (Djanaguiraman et al., 2020). The combination of high temperatures (Tmax ranging from 22.4°C to 35.9°C) and high vapor pressure deficit (VPD up to 2.83 kPa day⁻¹) during the critical heading and maturity stages created significant heat and drought stress. During the grain-filling stage, high temperatures accelerated the process, resulting in shorter maturation times and smaller grains, leading to higher concentrations of proteins, including gluten-forming proteins like gliadins and glutenins (Gagliardi et al., 2020; Djanaguiraman et al., 2020; Yang et al., 2023; Sihag et al., 2024). Elevated temperatures and high VPD can alter the balance of protein fractions in the grain, increasing the proportion of gliadins relative to glutenins, which enhances the gluten index and overall protein content (Torbica and Mastilović, 2008). These environmental stresses also influence the synthesis and assembly of glutenin polymers, crucial for dough strength and elasticity (Torbica and Mastilović, 2008; Wehrli et al., 2021). Additionally, studies have shown that high VPD can activate nitrogen metabolism, as indicated by increased expression of nitrogen transporter genes and higher concentrations of amino acids such as glutamine, aspartate, and serine in the leaves (Fakhet et al., 2021). These amino acids play crucial roles in regulating nitrate reductase gene expression and overall nitrogen assimilation, which can shift the balance of protein fractions in the plant. The combined effects of elevated temperatures and high VPD on stomatal conductance, photosynthesis, and nitrogen metabolism lead to significant changes in the balance of protein fractions in wheat, necessitating careful nitrogen management to optimize protein quality and maintain crop productivity in warm environments (Fakhet et al., 2021; El Haddad et al., 2021).
Our study found that the residual variance of quality traits, such as gluten index and protein content, is often higher than other phenotypic variances due to the inherent complexity and variability associated with nitrogen management strategies (Table 2). Environmental variability, measurement errors, genotype by environment interactions, and temporal variability all contribute to this increased residual variance. Understanding and managing these sources of variability is crucial for improving the accuracy and reliability of field trial results in plant breeding and agronomic research (Geiler-Samerotte et al., 2013; Wang et al., 2023). However, during heading and maturity, these conditions (elevated temperatures and high VPD) also impair photosynthesis and reduce pollen viability, leading to lower grain yield and reduced hectoliter weight due to the production of shriveled grains (Dupont and Altenbach, 2003; Jamieson et al., 2004; Triboï et al., 2003). Water deficit exacerbates these effects by limiting the plant’s ability to uptake water, further increasing the relative protein content compared to carbohydrates (Guttieri et al., 2000; 2001; Gulati et al., 2014).
In the cooler years such as 2013, 2014, 2015, 2017, and 2020, characterized by favorable water availability and lower vapor pressure deficit (VPD), wheat plants experience reduced stress across all phenological stages, including emergence, tillering, jointing, heading, and maturity. These conditions promote better early growth and establishment due to higher relative humidity during the emergence and tillering stages, which enhances seedling vigor and tiller formation (Farooq et al., 2009). Adequate water availability and moderate temperatures during the jointing stage support robust stem elongation and biomass accumulation, setting a strong foundation for subsequent growth phases (Shang et al., 2020). During the critical heading and maturity stages, cooler temperatures and sufficient precipitation reduce heat and drought stress, allowing for optimal photosynthesis, pollen viability, and grain setting. This results in higher grain yield, increased hectoliter weight, and more spikes per square meter (Cetin et al., 2022). The cooler temperatures also lead to a more prolonged grain filling period, enabling the development of plumper, denser grains with lower protein and gluten content. This dilution effect occurs because the grains have more time to accumulate biomass, which dilutes the concentration of proteins within the grain (Lama et al., 2022; Zhang et al., 2022). Furthermore, the high precipitation and lower VPD conditions in cooler environments enhance plant water status by maintaining higher stomatal conductance and reducing water loss, which supports better growth and biomass accumulation and reduces the incidence of shriveled grains, as the plants were not concentrating their resources on survival but rather on maximizing growth and yield (Gibberd et al., 2000; Stoddard et al., 2006; Fakhet et al., 2021). The negative correlation between precipitation and grain protein concentration further supports this, as cooler, wetter conditions typically result in lower protein content (Fowler, 2003; Orlandini et al., 2011).
Traditionally, plant breeders have focused on single-trait analysis, primarily yield, due to its direct economic impact, simplicity, and ease of measurement (Luby and Shaw, 2009; Yan, 2021; Beavis and Mahama, 2023). Yield-focused breeding is less resource-intensive and easier to implement, particularly in early breeding stages or regions with limited resources. This approach has a strong historical precedent due to the straightforward nature of phenotypic measurement and selection processes (Luby and Shaw, 2009). However, as the need for crops with multiple desirable traits like disease resistance, drought tolerance, and nutritional quality grows, the limitations of single-trait analysis are becoming clear (Merrick et al., 2022). Multi-trait analysis, which considers genetic correlations between traits, enhances predictive accuracy and efficiency and offers a more comprehensive and sustainable approach. With advanced statistical tools and computational resources becoming more accessible, multi-trait analysis is increasingly adopted to address the complex challenges of modern agriculture effectively (Guo et al., 2014; Merrick et al., 2022).
The MTMPS index is a sophisticated quantitative genetic technique used for the selection of suitable varieties across various crop species such as bread wheat (Farhad et al., 2022), elephant grass (Rocha et al., 2018), and maize (Olivoto et al., 2021; Yue et al., 2022) and is free from the multicollinearity problem (Sampaio Filho et al., 2023). According to the MTMPS results, two varieties, Antalis (G4) and Core (G18), were consistently selected across all regression parameters, indicating their broad adaptability and robust performance across diverse environments, particularly in drier and warmer conditions. Additionally, variety Furio Camillo (G31) was positioned near the selection intensity threshold for the RMSE parameter and ranked among the top six varieties for both R² and S²di, suggesting it possesses valuable traits. Overall, Antalis (G4) and Core (G18) varieties were consistently selected across all regression parameters, showcasing their unique strengths. The Antalis (G4) variety is optimal for grain yield and number of ears per m², making it a top choice for high-yield production. In contrast, the Core (G18) variety excels in protein content and gluten index, underscoring its potential for producing high-quality wheat. However, both varieties exhibit the undesired trait of high shriveled wheat grain, which should be addressed in future breeding programs to enhance their overall quality.
5 Conclusions
This study underscores the critical influence of environmental factors on the performance of durum wheat varieties in Southern Italy, emphasizing the importance of genotype by environment interaction (GEI) in breeding programs. The significant GEI effects observed highlight the necessity for multi-environment trials to accurately evaluate and enhance yield stability and quality traits across diverse conditions. Our findings indicate that warm years characterized by high temperatures, elevated vapor pressure deficit (VPD), and water deficits adversely affect grain yield and quality, leading to lower yields, reduced hectoliter weight, and increased grain shriveling. Conversely, cooler years with sufficient water availability and lower VPD foster better plant development, resulting in higher yields, increased hectoliter weight, and a greater number of spikes per square meter. The application of the Multi-Trait Mean Performance Selection (MTMPS) index proved to be an effective tool for identifying durum wheat varieties with superior performance and stability, particularly under varying environmental conditions. Varieties such as Antalis (G4) and Core (G18) demonstrated broad adaptability and robust performance, making them valuable candidates for high-yield and quality wheat production. These findings suggest that future breeding efforts should focus on multi-trait analysis to balance yield with other critical traits such as disease resistance. In conclusion, the integration of advanced statistical tools and computational resources in multi-trait selection can significantly improve the resilience and productivity of durum wheat varieties. Addressing undesired traits like high grain shriveling in future breeding programs will further enhance the overall quality and stability of wheat crops, ensuring sustainable agricultural practices in the face of climate change.
Data availability statement
The raw data supporting the conclusions of this article will be made available by the authors, without undue reservation.
Author contributions
MHS: Data curation, Formal analysis, Investigation, Methodology, Software, Supervision, Validation, Visualization, Writing – original draft, Writing – review & editing. IDM: Data curation, Formal analysis, Investigation, Project administration, Resources, Writing – review & editing. LO: Data curation, Formal analysis, Investigation, Project administration, Resources, Writing – review & editing. EC: Formal analysis, Investigation, Project administration, Resources, Writing – review & editing. PDV: Conceptualization, Supervision, Writing – review & editing. MM: Conceptualization, Resources, Supervision, Writing – review & editing, Project administration.
Funding
The author(s) declare that no financial support was received for the research, authorship, and/or publication of this article.
Conflict of interest
The authors declare that the research was conducted in the absence of any commercial or financial relationships that could be construed as a potential conflict of interest.
Publisher’s note
All claims expressed in this article are solely those of the authors and do not necessarily represent those of their affiliated organizations, or those of the publisher, the editors and the reviewers. Any product that may be evaluated in this article, or claim that may be made by its manufacturer, is not guaranteed or endorsed by the publisher.
Supplementary material
The Supplementary Material for this article can be found online at: https://www.frontiersin.org/articles/10.3389/fagro.2024.1466040/full#supplementary-material
References
Allen R. G., Pereira L. S., Raes D., Smith M. (1998). “Crop evapotranspiration – guidelines for computing crop water requirements,” in FAO irrigation and drainage paper 56/food and agriculture organization of the united nations. Rome.
Arnold S. J. (2023). The selection surface and adaptive landscape for multiple traits (Oxford University Press eBooks), 58–82. doi: 10.1093/oso/9780192859389.003.0005
Beavis W., Mahama A. A. (2023).Plant breeding basics. In: Quantitative genetics for plant breeding (Iowa State University Digital Press). Available online at: https://iastate.pressbooks.pub/quantitativegenetics/back-matter/plant-breeding-basics/ (Accessed 12 Jun 2024).
Beck H. E., Zimmermann N. E., McVicar T. R., Vergopolan N., Berg A., Wood E. F. (2018). Present and future köppen-geiger climate classification maps at 1-km resolution. Sci. Data 5, 180214. doi: 10.1038/sdata.2018.214
Bernardo R. (2020). Breeding for quantitative traits in plants. 3rd (Woodbury, Minnesota: Stemma Press).
Bishwas K. C., Poudel M. R., Regmi D. (2021). AMMI and GGE biplot analysis of yield of different elite wheat line under terminal heat stress and irrigated environments. Heliyon 7, p.e07206. doi: 10.1016/j.heliyon.2021.e07206
Boncinelli F., Dominici A., Bondioni F., Marone E. (2023). Consumers bahavior towards the country-of-origin labeling policy: The case of the pasta market in Italy. Agribusiness. 40(1), 46–69. doi: 10.1002/agr.21831
Bonfil D. J., Abbo S., Degen D., Simchon Y., Ben-David R. (2023). Towards stable wheat grain yield and quality under climatic instability. Agron. J 115, 1622–1639. doi: 10.1002/agj2.21351
Bux C., Lombardi M., Varese E., Amicarelli V. (2022). Economic and environmental assessment of conventional versus organic durum wheat production in southern Italy. Sustainability 14, p.9143. doi: 10.3390/su14159143
Carucci F., Gatta G., Gagliardi A., Bregaglio S., Giuliani M. M. (2023). Individuation of the best agronomic practices for organic durum wheat cultivation in the Mediterranean environment: a multivariate approach. Agric. Food Secur 12, 12. doi: 10.1186/s40066-023-00417-5
Cereals and Grains Association (2024). American association of cereal chemists (AACC) approved methods of analysis. Available online at: https://www.cerealsgrains.org/resources/Methods/Pages/default.aspx (Accessed 23 May 2024).
Cetin O., Yildirim M., Akinci C., Yarosh A. (2022). Critical threshold temperatures and rainfall in declining grain yield of durum wheat (Triticum durum desf.) during crop development stages. Romanian Agric. Res 39, 247–257. doi: 10.59665/rar3924
Costa C., Costa A. E. D. S., Neto F. P. L., de Lima M. A. C., Martins L. S. S., Musser R. S. (2023). Repeatability coefficient for fruit quality and selection of mango hybrids using REML/BLUP analysis. Euphytica 219, 120. doi: 10.1007/s10681-023-03249-3
Costa-Neto G., Galli G., Carvalho H. F., Crossa J., Fritsche-Neto R. (2021). EnvRtype: a software to interplay enviromics and quantitative genomics in agriculture. G3 Genes|Genomes|Genetics 11, jkab040. doi: 10.1093/g3journal/jkab040
Cullis B. R., Smith A. B., Coombes N. E. (2006). On the design of early generation variety trials with correlated data. J. Agricultural Biological Environ. Stat 11, 381–393. doi: 10.1198/108571106x154443
Dempster A. P., Laird N. M., Rubin D. B. (1977). Maximum likelihood from incomplete data via the EM algorithm. J. R. Stat. Society: Ser. B (Methodological) 39, 1–22. doi: 10.1111/j.2517-6161.1977.tb01600.x
Djanaguiraman M., Narayanan S., Erdayani E., Prasad P. V. V. (2020). Effects of high temperature stress during anthesis and grain filling periods on photosynthesis, lipids and grain yield in wheat. BMC Plant Biol 20, 1–12. doi: 10.1186/s12870-020-02479-0
Dupont F. M., Altenbach S. B. (2003). Molecular and biochemical impacts of environmental factors on wheat grain development and protein synthesis. J. Cereal Sci 38, 33–146. doi: 10.1016/S0733-5210(03)00030-4
Eberhart S. A., Russell W. A. (1966). Stability parameters for comparing varieties 1. Crop Sci 6, 36–40. doi: 10.2135/cropsci1966.0011183x000600010011x
Elahi I., Saeed U., Wadood A., Abbas A., Nawaz H., Jabbar S. (2022). Effect of climate change on wheat productivity. Wheat-Recent Advances. Ansari M. R. (London: IntechOpen). doi: 10.5772/intechopen.103780
El Haddad N., Choukri H., Ghanem M. E., Smouni A., Mentag R., Rajendran K., et al. (2021). High-temperature and drought stress effects on growth, yield and nutritional quality with transpiration response to vapor pressure deficit in lentil. Plants 11, p.95. doi: 10.3390/plants11010095
Eltaher S., Baenziger P. S., Belamkar V., Emara H. A., Nower A. A., Salem K. F. M., et al. (2021). GWAS revealed effect of genotype × environment interactions for grain yield of Nebraska winter wheat. BMC Genomics 22, 1–14. doi: 10.1186/s12864-020-07308-0
Etana D., Merga D. (2021). Additive main effect and multiplicative interaction model (AMMI) in plant breeding stability analysis: review. J. Agric. Res. Pesticides Biofertilizers 2. Available at: https://aditum.org/images/currentissue/1628678596Galley_Proof.pdf.
Euronext (2023). World durum wheat market focus. Available online at: https://www.euronext.com/en/news/world-durum-wheat-market-focus (Accessed 20 Jul. 2024).
Fakhet D., Morales F., Jauregui I., Erice G., Aparicio-Tejo P. M., González-Murua C., et al. (2021). Short-term exposure to high atmospheric vapor pressure deficit (VPD) severely impacts durum wheat carbon and nitrogen metabolism in the absence of edaphic water stress. Plants 10, 120–120. doi: 10.3390/plants10010120
Farhad M., Kumar U., Tomar V., Bhati P. K., Krishnan J. N., Kishowar-E-Mustarin, et al. (2023). Heat stress in wheat: a global challenge to feed billions in the current era of the changing climate. Front. Sustain. Food Syst 7. doi: 10.3389/fsufs.2023.120372
Farhad M., Tripathi S. B., Singh R. P., Joshi A. K., Bhati P. K., Vishwakarma M. K., et al. (2022). Multi-trait selection of bread wheat ideotypes for adaptation to early sown condition. Crop Sci 62, 67–82. doi: 10.1002/csc2.20628
Farooq M., Wahid A., Kobayashi N., Fujita D., Basra S. M. A. (2009). Plant drought stress: effects, mechanisms and management. Agron. Sustain. Dev 29, 185–212. doi: 10.1051/agro:2008021
Fowler D. B. (2003). Crop nitrogen demand and grain protein concentration of spring and winter wheat. Agron. J 95, 260–265. doi: 10.2134/agronj2003.2600
Gagliardi A., Carucci F., Masci S., Flagella Z., Gatta G., Giuliani M. M. (2020). Effects of genotype, growing season and nitrogen level on gluten protein assembly of durum wheat grown under mediterranean conditions. Agronomy 10, 755–755. doi: 10.3390/agronomy10050755
Geiler-Samerotte K., Bauer C., Li S., Ziv N., Gresham D., Siegal M. (2013). The Details in the Distributions: Why and how to study phenotypic variability. Curr. Opin. Biotechnol 24, 752–759. doi: 10.1016/j.copbio.2013.03.010
Gibberd M. R., Turner N. C., Loveys B. R. (2000). High vapour pressure deficit results in a rapid decline of leaf water potential and photosynthesis of carrots grown on free-draining, sandy soils. Aust. J. Agric. Res 51, 839. doi: 10.1071/ar00037
Gulati A., Asthir B., Bains N. S. (2014). Controlling water deficit by osmolytes and enzymes: Enhancement of carbohydrate mobilization to overcome osmotic stress in wheat subjected to water deficit conditions. Afr. J. Biotechnol 13, 2072–2083. doi: 10.5897/ajb2013.13153
Guo G., Zhao F., Wang Y., Zhang Y., Du L., Su G. (2014). Comparison of single-trait and multiple-trait genomic prediction models. BMC Genet 15, p.30. doi: 10.1186/1471-2156-15-30
Gupta V., Kumar M., Singh V., Chaudhary L., Yashveer S., Sheoran R., et al. (2022). Genotype by Environment Interaction Analysis for Grain Yield of Wheat (Triticum aestivum (L.) em.Thell) Genotypes. Agriculture 12, p.1002. doi: 10.3390/agriculture12071002
Guttieri M. J., Ahmad R., Stark J. C., Souza E. (2000). End-use quality of six hard red spring wheat cultivars at different irrigation levels. Crop Sci 40, 631–635. doi: 10.2135/cropsci2000.403631x
Guttieri M. J., Stark J. C., O’Brien K., Souza E. (2001). Relative sensitivity of spring wheat grain yield and quality parameters to moisture deficit. Crop Sci 41, 327–335. doi: 10.2135/cropsci2001.412327x
Hanif U., Gul A., Amir R., Munir F., Sorrells M. E., Gauch H. G., et al. (2021). Genetic gain and G × E interaction in bread wheat cultivars representing 105 years of breeding in Pakistan. Crop Sci. 62(1), 178–191. doi: 10.1002/csc2.20655
Husson F., Josse J., Le S., Mazet J. (2014).Facto mine R: multivariate exploratory data analysis and data mining with R. In: R package version.1 2014. Available online at: http://factominer.free.fr/ (Accessed 1 March 2024).
Italianfood (2024). Production of durum wheat surges in non-EU countries. Available online at: https://news.italianfood.net/2024/05/16/production-of-durum-wheat-surges-in-non-eu-countries (Accessed 20 Jul. 2024).
Jamieson P. D., Martre M., Samoil V., Porter J. R., Zyskowski R. L., Semenov M., et al. (2004).Modelling protein content and composition in wheat. In: Proceedings of the 4th international crop science congress brisbane, Australia, 26. Available online at: https://agronomyaustraliaproceedings.org/images/sampledata/2004/poster/2/8/475_jamieson.pdf (Accessed 17 May 2024).
Karaman M., Bayram S., Şatana E. (2023). genotypes (Triticum aestivum L.) with GGE biplot and AMMI model in multiple environments. Romanian Agric. Res 40, 189–198. doi: 10.59665/rar4018
Khalid S., Imran N., Khan H. A., Arif M., Abdel, Adnan M., et al. (2022). Effect of climate change on cereal crops (Floride, United States: CRC Press eBooks), 113–121. doi: 10.1201/9781003286417-6
Khare V., Shukla R. S., Pandey S., Singh S. K., Singh C. (2024). Exploring the genotype-environment interaction of bread wheat in ambient and high-temperature planting conditions: a rigorous investigation. Sci. Rep 14, 2402. doi: 10.1038/s41598-024-53052-w
Kolde R. (2019).pheatmap: pretty heatmaps. In: R package version 1.0. 12. Available online at: https://CRAN.R-project.org/package=pheatmap (Accessed 23 May 2024).
Kumar S., Kumari J., Bansal R., Kuri B. R., Upadhyay D., Srivastava A., et al. (2017). Multi-environmental evaluation of wheat genotypes for drought tolerance. Indian J. Genet. Plant Breed. (The) 78, p.26. doi: 10.5958/0975-6906.2018.00004.4
Kunze K. H., Meints B., Massman C., Gutiérrez L., Hayes P. M., Smith K. P., et al. (2024). Genotype × environment interactions of organic winter naked barley for agronomic, disease, and grain quality traits. Crop Sci. 64(2), 678–696. doi: 10.1002/csc2.21195
Lado B., Barrios P. G., Quincke M., Silva P., Gutiérrez L. (2016). genotype × Environment interaction for genomic selection with unbalanced data from a wheat breeding program. Crop Sci 56, 2165–2179. doi: 10.2135/cropsci2015.04.0207
Lama S., Vallenback P., Hall S. A., Kuzmenkova M., Kuktaite R. (2022). Prolonged heat and drought versus cool climate on the Swedish spring wheat breeding lines: Impact on the gluten protein quality and grain microstructure. Food Energy Security. 11(2), e736. doi: 10.1002/fes3.376
Lanna J., De M., Mendes T., Da L., Lucia R., Celis Â. (2022). Lima bean populations assessments via reml/blup methodology. Rev. Caatinga 35, 783–790. doi: 10.1590/1983-21252022v35n405rc
Lozano-Isla F. (2021).inti: Tools and statistical procedures in plant science. In: R package version 0.1 3. Available online at: https://cran.r-project.org/web/packages/inti/vignettes/heritability.html (Accessed 23 May 2024).
Luby J. J., Shaw D. V. (2009). Plant breeders’ Perspectives on improving yield and quality traits in horticultural food crops. HortScience 44, 20–22. doi: 10.21273/hortsci.44.1.20
Mariotti F., Tomé D., Mirand P. P. (2008). Converting nitrogen into protein—Beyond 6.25 and jones’ Factors. Crit. Rev. Food Sci. Nutr 48, 177–184. doi: 10.1080/10408390701279749
Mehmet A. S., Cuma A., Oral D., Emin D. (2011). Assessment of genotype x environment interaction on yield and yield components of durum wheat genotypes by multivariate analyses. Afr. J. Biotechnol 10, 2875–2885. doi: 10.5897/ajb10.2197
Merrick L. F., Burke A. B., Zhang Z., Carter A. H. (2022). Comparison of single-trait and multi-trait genome-wide association models and inclusion of correlated traits in the dissection of the genetic architecture of a complex trait in a breeding program. Front. Plant Sci 12. doi: 10.3389/fpls.2021.772907
Nicola F. G., Scaccia G. (2021). It’s all about the pasta: protectionism, liberalization, and the challenge for quality and sustainability of made in Italy. FIU Law Rev 14, 479. doi: 10.25148/lawrev.14.3.7
Ninou E., Tsivelika N., Sistanis I., Katsenios N., Korpetis E., Vazaneli E., et al. (2024). Assessment of durum wheat cultivars’ Adaptability to mediterranean environments using G x E interaction analysis. Agronomy 14, 102–102. doi: 10.3390/agronomy14010102
Olanrewaju O. S., Oyatomi O., Babalola O. O., Abberton M. (2021). GGE biplot analysis of genotype × Environment interaction and yield stability in bambara groundnut. Agronomy 11, 1839. doi: 10.3390/agronomy11091839
Olivoto T., Lúcio A. D. (2020). metan: An R package for multi-environment trial analysis. Methods Ecol. Evol 11, 783–789. doi: 10.1111/2041-210x.13384
Olivoto T., Lúcio A. D. C., Silva J. A. G., Marchioro V. S., Souza V. Q., Jost E. (2019). Mean performance and stability in multi-environment trials I: combining features of AMMI and BLUP techniques. Agron. J 111, pp.2949–2960. doi: 10.2134/agronj2019.03.0220
Olivoto T., Nardino M., Meira D., Meier C., Follmann D. N., Souza V. Q., et al. (2021). Multi-trait selection for mean performance and stability in maize. Agron. J 113, 3968–3974. doi: 10.1002/agj2.20741
Orlandini S., Mancini M., Daniele G., Orlando F., Dalla Marta A., Capecchi V. (2011). Integration of meteo-climatic and remote sensing information for the analysis of durum wheat quality in Val d’Orcia (Tuscany, Italy). Q. J. Hungarian Meteorological Service 115, 233–245.
Piepho H.-P., Möhring J. (2007). Computing heritability and selection response from unbalanced plant breeding trials. Genetics 177, 1881–1888. doi: 10.1534/genetics.107.074229
Pour-Aboughadareh A., Khalili M., Poczai P., Olivoto T. (2022). Stability indices to deciphering the Genotype-by-Environment Interaction (GEI) effect: An applicable review for use in plant breeding programs. Plants 11, p.414. doi: 10.3390/plants11030414
R Core Team (2013).R: A language and environment for statistical computing. In: R foundation for statistical computing (Vienna, Austria). Available online at: https://www.scirp.org/(S(vtj3fa45qm1ean45vvffcz55))/reference/ReferencesPapers.aspx?ReferenceID=1742158 (Accessed 1 March 2021).
Revelle W. (2024). psych: procedures for psychological, psychometric, and personality research. R-packages. Available online at: https://cran.r-project.org/web/packages/psych/index.html (Accessed 23 May 2024).
Rocha J., MaChado J. C., Carneiro P. C. S. (2018). Multitrait index based on factor analysis and ideotype-design: proposal and application on elephant grass breeding for bioenergy. GCB Bioenergy 10, 52–60. doi: 10.1111/gcbb.12443
Rout N., Mishra D., Mallick M. K., Mallick P. K. (2022). Dealing with Imbalanced Data. In: Electronic Systems and Intelligent Computing: Proceedings of ESIC 2021. Singapore: Springer Nature Singapore. 2022, 383–390. doi: 10.1007/978-981-16-9488-2_35
Sampaio Filho J. S., Olivoto T., Campos M. D. S., Oliveira E. J. D. (2023). Multi-trait selection in multi-environments for performance and stability in cassava genotypes. Front. Plant Sci 14. doi: 10.3389/fpls.2023.1282221
Scavo A., Mauromicale G., Ierna A. (2023). Genotype × environment interactions of potato tuber quality characteristics by AMMI and GGE biplot analysis. Scientia Hortic 310, 111750. doi: 10.1016/j.scienta.2022.111750
Shang Y., Lin X., Li P., Gu S., Lei K., Wang S., et al. (2020). Effects of supplemental irrigation at the jointing stage on population dynamics, grain yield, and water-use efficiency of two different spike-type wheat cultivars. PloS One 15, e0230484. doi: 10.1371/journal.pone.0230484
Sihag P., Kumar U., Sagwal V., Kapoor P., Singh Y., Mehla S., et al. (2024). Effect of terminal heat stress on osmolyte accumulation and gene expression during grain filling in bread wheat (Triticum aestivum L.). Plant Genome 17, e20307. doi: 10.1002/tpg2.20307
Silva C. M., Mezzomo H. C., Ribeiro J. P. O., De Freitas D. S., Nardino M. (2023). Multi-trait selection of wheat lines under drought-stress condition. Bragantia 82, e20220254. doi: 10.1590/1678-4499.20220254
Soltani A., Sinclair T. R. (2012). Modeling physiology of crop development, growth and yield. Wallingford CA: CAB Int. Press pp, 322. doi: 10.1079/9781845939700.0000
Sparks A. (2018). nasapower: A NASA POWER global meteorology, surface solar energy and climatology data client for R. J. Open-Source Software 3, 1035. doi: 10.21105/joss.01035
Stoddard F. L., Balko C., Erskine W., Khan H. R., Link W., Sarker A. (2006). Screening techniques and sources of resistance to abiotic stresses in cool-season food legumes. Euphytica 147, 167–186. doi: 10.1007/s10681-006-4723-8
Sugasawa S., Kubokawa T. (2023). “General mixed-effects models and BLUP,” in Mixed-effects models and small area estimation (Springer Briefs in Statistics, Springer, Singapore). doi: 10.1007/978-981-19-9486-9_2
Tajalifar M., Rasooli M. (2022). Importance of BLUP method in plant breeding. J. Plant Sci. Phytopathol 6, 040–042. doi: 10.29328/journal.jpsp.1001072
Tataw J. T., Baier F., Krottenthaler F., Pachler B., Schwaiger E., Wyhlidal S., et al. (2016). Climate change induced rainfall patterns affect wheat productivity and agroecosystem functioning dependent on soil types. Ecol. Res 31, 203–212. doi: 10.1007/s11284-015-1328-5
Torbica A., Mastilović J. (2008). Influence of different factors on wheat proteins quality. Food Feed Res 35, 47–52.
Triboï E., Martre P., Triboï-Blondel A.-M. (2003). Environmentally-induced changes in protein composition in developing grains of wheat are related to changes in total protein content. J. Exp. Bot 54, 1731–1742. doi: 10.1093/jxb/erg183
Viggiani P. (2009). ““Frumento in italia“,” in Il grano. Eds. Viggiani P., D'Egidio M. G., Saviotti B., Angelini R., Milano Di F. N. (Bayer CropScience Press, Italy), 1–91.
Wang L., Ma L., Li Y., Geilfus G. M., Wei J., Zheng F., et al. (2023). Managing nitrogen for sustainable crop production with reduced hydrological nitrogen losses under a winter wheat–summer maize rotation system: an eight-season field study. Front. Plant Sci 14. doi: 10.3389/fpls.2023.1274943
Wehrli M. C., Kratky T., Schopf M., Scherf K. A., Becker T., Jekle M. (2021). Thermally induced gluten modification observed with rheology and spectroscopies. Int. J. Biol. Macromolecules 173, 26–33. doi: 10.1016/j.ijbiomac.2021.01.008
Yan W. (2015). Mega-environment analysis and test location evaluation based on unbalanced multiyear data. Crop Sci 55, 113–122. doi: 10.2135/cropsci2014.03.0203
Yan W. (2021). A systematic narration of some key concepts and procedures in plant breeding. Front. Plant Sci 12. doi: 10.3389/fpls.2021.724517
Yang J., Feng Y., Chi T., Wen Q., Liang P., Wang A., et al. (2023). Mitigation of Elevated CO2 Concentration on Warming-Induced Changes in Wheat Is Limited under Extreme Temperature during the Grain Filling Period. Agronomy 13, 1379–1379. doi: 10.3390/agronomy13051379
Yue H., Olivoto T., Bu J., Li J., Wei J., Xie J., et al. (2022). Multi-trait selection for mean performance and stability of maize hybrids in mega-environments delineated using envirotyping techniques. Front. Plant Sci 13. doi: 10.3389/fpls.2022.1030521
Zabn K., Alsajri F. (2022). Effect of stopping irrigation at different growth stages in wheat growth and dry matter accumulation. Iraqi J. desert Stud 12, 18–30. doi: 10.36531/ijds.2022.176691
Zahra N., Hafeez M. B., Wahid A., Al Masruri M. H., Ullah A., Siddique K. H. M., et al. (2022). Impact of climate change on wheat grain composition and quality. J. Sci. Food Agric 103, 2745–2751. doi: 10.1002/jsfa.12289
Keywords: durum wheat, genotype by environment interaction, mixed model, MTMPS, unbalanced data
Citation: Sellami MH, Di Mola I, Ottaiano L, Cozzolino E, De Vita P and Mori M (2024) Assessing temporal variability in durum wheat performance and stability through multi-trait mean performance selection in Mediterranean climate. Front. Agron. 6:1466040. doi: 10.3389/fagro.2024.1466040
Received: 17 July 2024; Accepted: 14 October 2024;
Published: 06 November 2024.
Edited by:
John R. Porter, University of Copenhagen, DenmarkReviewed by:
Tiago Olivoto, Federal University of Santa Catarina, BrazilAurelio Scavo, University of Messina, Italy
Leyla Nazari, Education and Extension Organization (AREEO), Iran
Copyright © 2024 Sellami, Di Mola, Ottaiano, Cozzolino, De Vita and Mori. This is an open-access article distributed under the terms of the Creative Commons Attribution License (CC BY). The use, distribution or reproduction in other forums is permitted, provided the original author(s) and the copyright owner(s) are credited and that the original publication in this journal is cited, in accordance with accepted academic practice. No use, distribution or reproduction is permitted which does not comply with these terms.
*Correspondence: Ida Di Mola, aWRhLmRpbW9sYUB1bmluYS5pdA==