- 1South African Grape and Wine Research Institute (SAGWRI), Stellenbosch University, Faculty of AgriSciences, Stellenbosch, South Africa
- 2Department of Plant Pathology, Stellenbosch University, Stellenbosch, South Africa
Avocado production faces a substantial global threat in the form of Phytophthora root rot (PRR). When trees succumb to PRR, their canopy health deteriorates, leading to adverse impacts on production. To effectively implement remedial strategies, infected trees need to be identified, evaluated, and located within the field. The current commercially accepted method for determining PRR severity in canopies consists of a visual estimation using the ‘Ciba-Geigy’ rating scale. This rating scale incorporates a numerical severity ranking system based on a visual approach conducted by trained personnel. However, tracking tree health using visual ratings is a time-consuming process, fraught with practical challenges arising from gradual visual changes, spatial variation, and dimensions of the orchards. To address these limitations, the integration of remote sensor-based methods is proposed as a viable alternative to the visual severity ranking. A field experiment was conducted in two avocado blocks to investigate the effect of spatial resolution, phenological stages, and canopy conditions on the mapping of PRR severity. The results of this study showed that canopy management practices revealed a pronounced influence in the determination of the severity ranking using remote sensing (RS) methods and that these methods can be used as an alternative to visual estimations. Additionally, the spatial resolution of the images emerged as a significant factor, improving the estimation of severity when more detailed spatial data were incorporated into the analysis. In the most favorable scenario, an R2 determination coefficient of 0.80 was achieved. In summary, RS approaches can provide valuable information to mitigate the effect of PRR in avocado production. However, the image characteristics and particular canopy conditions need to be carefully considered in order to deliver a reliable method that can be used for informed decision-making. Nonetheless, the results were promising and could open doors to further investigate RS methods as a subjective and efficient means of PRR severity rankings.
1 Introduction
Diseases in avocado trees negatively affect productivity and may ultimately result in tree mortality over time. Phytophthora root rot (PRR) is a globally significant disease with a wide-ranging host spectrum, affecting nearly 5000 plant species (Haagsma et al., 2020). It is considered a major threat to avocado production globally (Erwin and Ribeiro, 1996). PRR is a soil-borne disease caused by the oomycete Phytophthora cinnamomi which primarily attacks the roots under warm and moist soil conditions. In mature plants, the pathogen causes an over-production of low-weight fruit which is detrimental to the crop yield (Ramírez-Gil et al., 2017). The pathogen is known to attack trees by destroying their small absorbing roots. These roots become brittle and can eventually die, leaving the tree with little nutrient and water uptake ability. In extreme circumstances, PRR-infected trees are reduced to a bare framework of dying branches (Pegg et al., 2002; García-Pineda et al., 2010). In avocado trees afflicted with PRR, canopy health characteristically declines resulting in smaller, wilted leaves with brown tips that are pale green (Marais et al., 2002).
Once PRR is identified, growers have the opportunity to implement remedial strategies that combine cultural practices with chemical control (Eskalen et al., 2017). The most used chemical control method includes treatment with phosphonates (Salgadoe et al., 2018). Phosphonate applications are most effective when applied preventatively or at an early stage of tree decline. Consequently, growers often apply phosphonates preventatively on an annual basis, considering the aggressive and destructive nature of the disease. However, this preventive approach can result in unnecessary expenditure and resource allocation if the tree is not significantly infected. Consequently, some growers opt to implement visual canopy health assessments. This allows them to make informed decisions as to whether phosphonate applications are required and apply chemical control only if deemed necessary.
The current commercially accepted method for estimating PRR severity in canopies consists of a visual estimation using the ‘Ciba-Geigy’ rating scale. This visual rating scale incorporates a numerical severity ranking system that chiefly evaluates canopy porosity, leaf color, and twig die-back and is conducted by trained personnel (Darvas et al., 1984; Nutter et al., 2006). However, tracking changes in tree health due to PRR infections using visual ratings is a time-consuming process and can prove exceptionally challenging in practice. This challenge arises from the gradual nature of visual changes, spatial variation within orchards, and the considerable expanses of land that require monitoring (Garza et al., 2020). Salgadoe et al. (2018) highlighted that despite its common usage, the visual assessment of tree health is inefficient in terms of time, labor, and cost requirements. Mahlein (2016) further emphasizes that visual assessment methods such as these require the involvement of experienced individuals with well-developed skills in diagnostics and disease detection and are thus introducing a potential for human bias. Additionally, the assessments require trained experts to ensure that observations are comparable across orchards and growing seasons, thereby increasing the potential for human bias.
In the context of phosphonate applications, their preventative use necessitates precise timing, usually directly after harvest and during the summer root flush when the leaf flush has hardened off. This adds to the complexity of the process, especially when dealing with extensive hectares that may require phosphonate treatments. This can lead to constraints and complications with time and labor force. Therefore, the need arises for an objective and efficient technique characterized by high sensitivity and reliability to enhance the detection of PRR disease severity in avocado tree canopies.
Remote sensor-based methods offer an alternative to the human vision inspection for disease detection, presenting notable benefits for the management of PRR infections in avocado orchards (e.g., Abdulridha et al., 2018; Salgadoe et al., 2018). These methods facilitate the rapid coverage of larger areas, facilitating quicker and more informed management decisions regarding phosphonate treatments. Among these remote sensors, optical sensors measuring reflectance have emerged as highly promising tools (Chaerle and van der Straeten, 2000; West and Bravo, 2003; Sankaran et al., 2010; Mahlein et al., 2012). Reflectance-based sensors can detect changes in the leaf structure and pigments of plants induced by plant pathogens and diseases. These sensors are installed on multiple platforms, categorized into three major categories: space-borne (satellites), airborne (aircraft, and unmanned aerial vehicles (UAVs)) and ground-based (proximal sensing). Aerial (space-borne and airborne) remote sensing (RS) has recently gained traction in plant disease detection applications with red, green, and blue (RGB) and spectral (multi- and hyperspectral) sensors receiving the most attention thus far (Mahlein, 2016).
RGB and multispectral (MS) images, acquired from aerial platforms at different resolutions, have proven effective in detecting various disease symptoms and health conditions in several tree crops at the orchard or farm level. Examples include banana plants (Selvaraj et al., 2020), macadamia (Johansen et al., 2020), olives (Hornero et al., 2020), avocado (De Castro et al., 2015; Salgadoe et al., 2018; Pérez-Bueno et al., 2019; Tu et al., 2019) and citrus (Kumar et al., 2010; Li et al., 2012; Garcia-Ruiz et al., 2013; Chang et al., 2020; Garza et al., 2020). However, most of these studies focused on fixed/same canopy conditions within a short/single temporal range, often neglecting phenological aspects.
Regarding avocado trees, previous research has not considered canopy conditions nor the impact of orchard management. These studies are predominantly centered on, single orchard studies with short or no temporal aspects considered for disease detection or canopy condition assessment at the orchard or farm level. Additionally, while multiple platforms (i.e., single platform or proximal combined with satellite imagery) have been researched in other crops, the combined use of UAV and satellite imagery remains unexplored in avocado.
In this context, this study aims to investigate different RS techniques for mapping the severity of PRR in avocado orchards by considering the combination and interaction of 3 important factors: i) Spatial resolution (satellite and UAV); ii) Multi-temporal approach (phenological periods); and iii) Canopy conditions (Pruning type and intensity). The RS techniques were two different platforms: UAVs (RGB and MS imagery) and satellites (MS imagery). Spectral properties of target trees were extracted from each technique over four periods and compared to the commercial visual severity rating method, ‘Ciba Geigy’ rating scale, by linear regression. By doing this research, this study contributes to a better understanding of using RS techniques as an alternative to human vision in the detection of PRR in avocado orchards.
2 Material and methods
2.1 Experimental sites
The study was conducted on two commercial avocado farms, ZZ2 and Westfalia Fruit, located in the Tzaneen area of Limpopo Province, Northern South Africa (Figure 1A). This location is classified as having a Monsoon-influenced humid subtropical climate (Regional Weather and Climate of South Africa, 2023). For this study, single PRR-infected orchards within each farm were selected. These orchards shared identical cultivars, rootstock, and planting year, but varied in canopy management practices (Table 1). The selection was based on previous in-house and academic research projects conducted in conjunction with the farm owners and managers (McLeod et al., 2018). Additionally, an RS-based screening analysis was implemented on Sentinel-2A (S2A) imagery to evaluate the intra-block changes in vigor, expressed as normalized difference vegetation index (NDVI) (Figures 1B, C) and to further define the study area. Furthermore, this screening analysis ensured that severity variability was evident in the selected orchard blocks. One of the major symptoms of PRR is the progressive death of shoots and branches which is detectable through NDVI analysis. Orchards with different canopy management practices were selected to create a more “realistic” representation of data, as differing farms/companies often implement different practices.
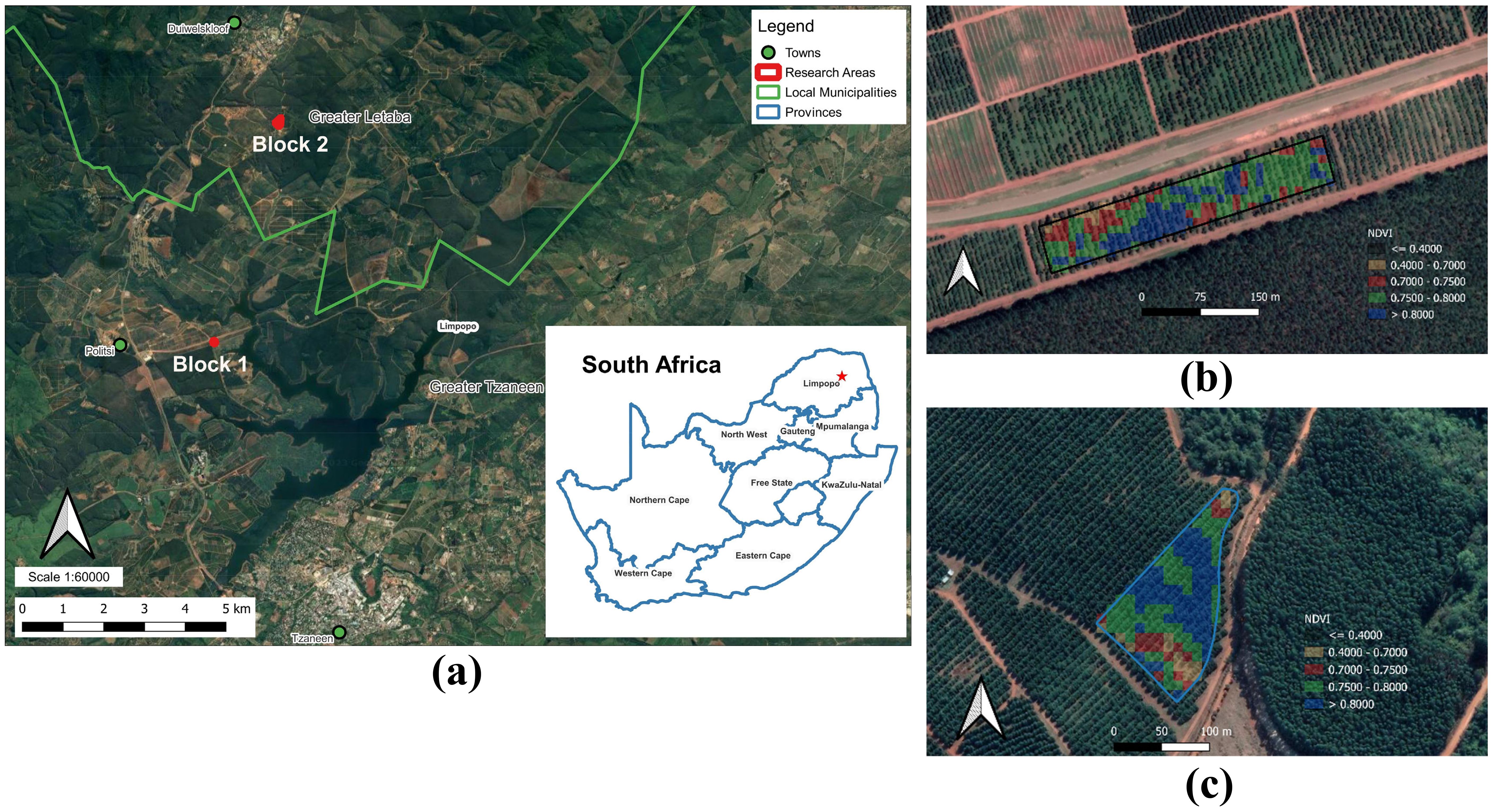
Figure 1. Location of research blocks using imagery from Google Earth (A). Example of the RS-based screening analysis used for the block selection (A, B). Normalized difference vegetation index (NDVI) obtained by satellite S2A (May 2020) (B) Block –1 - Gigas (managed by ZZ2) (C) Block –2 - McNoon Estate (managed by Westfalia Fruit).
2.2 Target tree selection and geolocation
Research blocks containing 13 to 17 rows of trees comprising ±1.18 hectares (ha) for the ZZ2 managed block (block 1) and ±1.85 ha for the Westfalia Fruit managed block (block 2) were selected. Target trees were selected by two experienced personnel from ZZ2 and Westfalia fruit, relying on visual assessments using the ‘Ciba-Geigy’ rating scale. A total of 69 trees were selected for this research: 34 trees in block 1 and 35 trees in block 2. To ensure variability was present in the tree dataset, target trees expressing different levels of canopy decline were selected with dimensionless rankings ranging from 0 to 8. Furthermore, the locations of the target trees were captured using a Global Positioning System (GPS) rover, and their coordinates were saved in the WGS-84 reference system. The positions of the trees were measured in the middle of the rows, perpendicular to the trees. The accuracy of these positions is of utmost importance due to the multi-temporal aspect of this research and the need for accurate integration across the RS techniques utilized.
2.3 Data acquisition
Reference measurements, UAV imagery, and satellite imagery were acquired over 10 months, from May 2021 to February 2022, covering four different phenological periods. Figure 2 summarizes the whole process followed in this study. This comprises the data acquisition from the different platforms/RS techniques and the processing steps to get qualitative results from which the analysis was conducted. Images were acquired from UAV and satellite platforms and then image analysis was conducted.
2.4 Reference measurements – severity rankings
Visual assessments of the target trees in each block were conducted using the ‘Ciba-Geigy’ for each period (Darvas et al., 1984). To ensure consistency within the rankings, the visual assessments were conducted by the same two personnel throughout the duration of the experiment.
2.5 UAV imagery – MS and RGB
Images were captured of both blocks during each of the four data collection periods using a DJI Phantom 4 Pro multirotor (DJI, Shenzhen, China). The multirotor was equipped with a 16-bit RGB camera (model FC6310), and a 12-bit RedEdge™ 3 MS camera (MicaSense Inc., Seattle, U.S.A). The RedEdge™ 3 camera recorded spectral information in blue [B] (465 – 475nm); green [G] (550 – 570nm); red [R] (663 – 673nm); RedEdge [RE] (716 – 722nm) and near-infrared [NIR] (820 – 860nm). Both cameras were stabilized in pitch, roll, and yaw by a three-axis gimbal. To ensure full coverage of the areas, the UAV was flown autonomously along pre-programmed flight paths, with parameters shown in Table 2, set in the DroneDeploy application (California, U.S.A.). The flights took place as close to solar noon as possible to avoid shadows and the UAV was flown along a flight path along-tree rows.
Field marks were positioned in the middle of the rows (at the GPS point locations), next to the target trees for target tree identification and the reflectance panel was imaged immediately before each flight at the respective sites for radiometric calibration. Orthomosaics were generated for both RGB (DJI camera) and MS (RedEdge™ 3 camera) datasets along with RGB-Digital Elevation Models (DEM’s) and MS-DEM’s for each flight were constructed using Agisoft Metashape version 1.7.6 (Agisoft LLC., St. Petersburg, Russia) following the standard Agisoft photogrammetric pipeline that produces dense Structure-from-Motion (SfM) point clouds. The onboard GPS on the UAV supplied sufficient geolocation; therefore, the images weren’t geometrically calibrated in Agifsoft. Before processing, all photos were visually assessed for quality assurance. DEM’s achieved resolutions of 2.0 < 7.0 cm/pix for all 16 datasets (8 x RGB-DEM’s, 8 x MS-DEM’s) and the orthomosaics attained resolutions of 1.0 <4.0 cm/pix for all 16 datasets (8 x RGB, 8 x MS orthomosaics). These results, maintaining a precision level of 5.0 cm, are deemed sufficient for precision farming purposes (Candiago et al., 2015). Upon completion, the DEM imagery was exported as GeoTIFF files and orthomosaics were exported in JPEG format, preserving the B, G, R, RE, and NIR channels for analysis.
Once the orthomosaics and DEM’s were created for the sites, the individual targeted trees were clipped out of the parent images using QGIS. These individual images were saved in tiff format and used for the following analysis. The size of the clipped images was determined by the row and tree spacing, canopy size, and the amount of canopy overlapping. Individual block 1 images were 7.0 m by 5.0 m and block 2 were 8.5 m by 4.0 m. These dimensions were narrower because the trees were growing intertwined, and the researchers endeavored to not have neighboring trees in the image. Additionally, they were longer to accommodate the larger canopies and wider row spacing.
Radiometric calibration was performed on the MS imagery in Agisoft Metashape version 1.7.6 (Agisoft LLC., St. Petersburg, Russia) as part of the workflow before processing the orthomosaics. The software used the images of the white radiometric panel and the panels’ predetermined calibration values (obtained from MicaSense) to convert the raw sensor values into reflectance values.
2.6 Satellite imagery – MS
High-resolution (0.50 – 0.75 m) satellite imagery was acquired from third-party suppliers (Maxar Technologies and GEO Data Design (Pty) LTD. This imagery came pre-georeferenced and had undergone radiometric correction. High-resolution imagery generally has short revisit times, so imagery was acquired with acquisition dates as close to the UAV data collection dates (Table 3).
Medium-resolution (10 m) Sentinel-2B (S2B) imagery was obtained from the European Space Agency (ESA) Copernicus Open Access Hub (https://scihub.copernicus.eu/dhus/#/home). Cloud-free Level-1C (L1C), ortho-rectified imagery expressed in top-of-atmosphere (TOA) reflectance were downloaded and atmospherically corrected to generate Level-2C using the Sen2Cor (version 2.5.5, ESA, Paris, France) in the Sentinel application platform SNAP (version 9.0.0, ESA, Paris, France) using the default parameters. Images were not filtered for cloud cover since no clouds were present over the study areas and the images were not resampled. Finally, the 10 m resolution bands 2, 3, 4, and 8 (B, G, R, and NIR respectively) were exported in the GeoTIFF format as a single image. The individual trees were clipped out of all the parent images using the same method as described above.
2.7 Data analysis – outputs from UAV and satellite imagery
2.7.1 Determination of plant projective cover (fcover) from UAV-RGB imagery
The calculation of plant projective cover (fcover) was performed using DEM’s obtained from UAV-RGB images. These DEM’s allowed for the determination of the canopy extent by considering all points above a certain height threshold and disregarding the points below. Using the individual DEM’s as masks and specifying a predefined height threshold as inputs, the fcover was calculated for each individual image using MATLAB (version 9.11.0.1809720 (R2021b) Update 1, MathWorks, U.S.A.) code and exported as a percentage. The height threshold was based on the elevation/altitude and was determined by measuring the difference in height between the ground and the lowest part of the tree. Subsequently, the fcover was correlated to the ‘Ciba-Geigy’ severity rankings to determine whether there was a relationship between fcover and severity.
2.7.2 Vegetation indices
2.7.2.1 Determination of vegetation indices from UAV-MS imagery
The MS imagery was used to calculate vegetation indices (VI’s). The selected VI’s were determined based on the five spectral bands acquired by the sensor and on relevant literature (Table A1 in Appendix A). DEM’s from the MS imagery were used as masks for the same masking purpose as mentioned above. The individual tree images were analyzed using MATLAB code that calculated the mean reflectance value of each band, and based on these mean values, a total of 45 VI’s utilizing B, G, R, RE, and NIR bands were calculated. The full list of VI’s used in this study is presented in Appendix A (Table A1).
2.7.2.2 Determination of vegetation indices from satellite imagery – high- and medium-resolution
High- and medium-resolution imagery of the individual trees was used to calculate 27 VI’s that utilized B, G, R, and NIR bands. For medium-resolution imagery (S2B), the single-band reflectance values for each tree were extracted using the “Point Sampling Tool” plugin in QGIS (version 3.20.2 Odense, QGIS Development Team). These extractions were carried out at the precise GPS points surveyed in the field to extract the mean reflectance value of the corresponding band. Before calculating the VI’s, the S2B digital numbers (DN) were divided by 1000 to obtain the reflectance, ranging from 0 to 1. The “Point Sampling Tool” was used due to the large pixel size (10 m resolution), making use of a clipping polygon unnecessary. For high-resolution imagery, the clipping method described earlier was employed to extract the individual trees from the parent rasters in QGIS. Subsequently, “zonal statistics” was applied to each of the individual tree rasters, calculating the mean reflectance value for the corresponding band. To avoid having non-canopy features, the polygons were placed so that only the canopy was inside them.
2.8 Statistical analysis
This study aimed to determine trends between the severity rankings and the calculated VI values from different remote sensing platforms, to extract an underlying pattern of behavior over four periods. The trends were determined by a linear regression analysis using as a criterion the visual severity ranging measure by experts in the field experiments and as predictors the remote sensing derived values (fcover and VI values). The VI’s demonstrating the most significant correlations with the visual severity rankings were identified and descriptive statistics were performed on them. The linear regression analysis was performed using R Statistical Software version 4.1.2 (R Core Team, 2021) and GraphPad Prism version 9.0.0 (GraphPad Software, California, U.S.A.) was used to generate scatter plots and boxplots.
3 Results
3.1 Severity rankings
Severity rankings were acquired of each target tree over four phenological periods: post-harvest period (PH), flowering period (F), early fruit development period (ED) and late fruit development period (LD). Figure 3 shows an example of the spatial distribution of the rankings measured in the field during the PH period in May 2021 for block 1 (Figure 3A) and block 2 (Figure 3B). As seen for this period in block 1, trees with higher severity rankings are located on the southwest side of the block, while in block 2 they are more sparsely spatially distributed. Block 1 severity rankings were fairly consistent over the four data collection periods, changing no more than one or two rankings (Figure 4A). More often, the changes were negative as the season progressed resulting in February having the highest severity rankings (Figure 4B). Block 2 severity rankings were relatively similar in consistency, although slightly less, over the four data collection periods (Figures 4C, D). Severity rankings acquired in September, FP, were clearly the highest (Figure 4D).
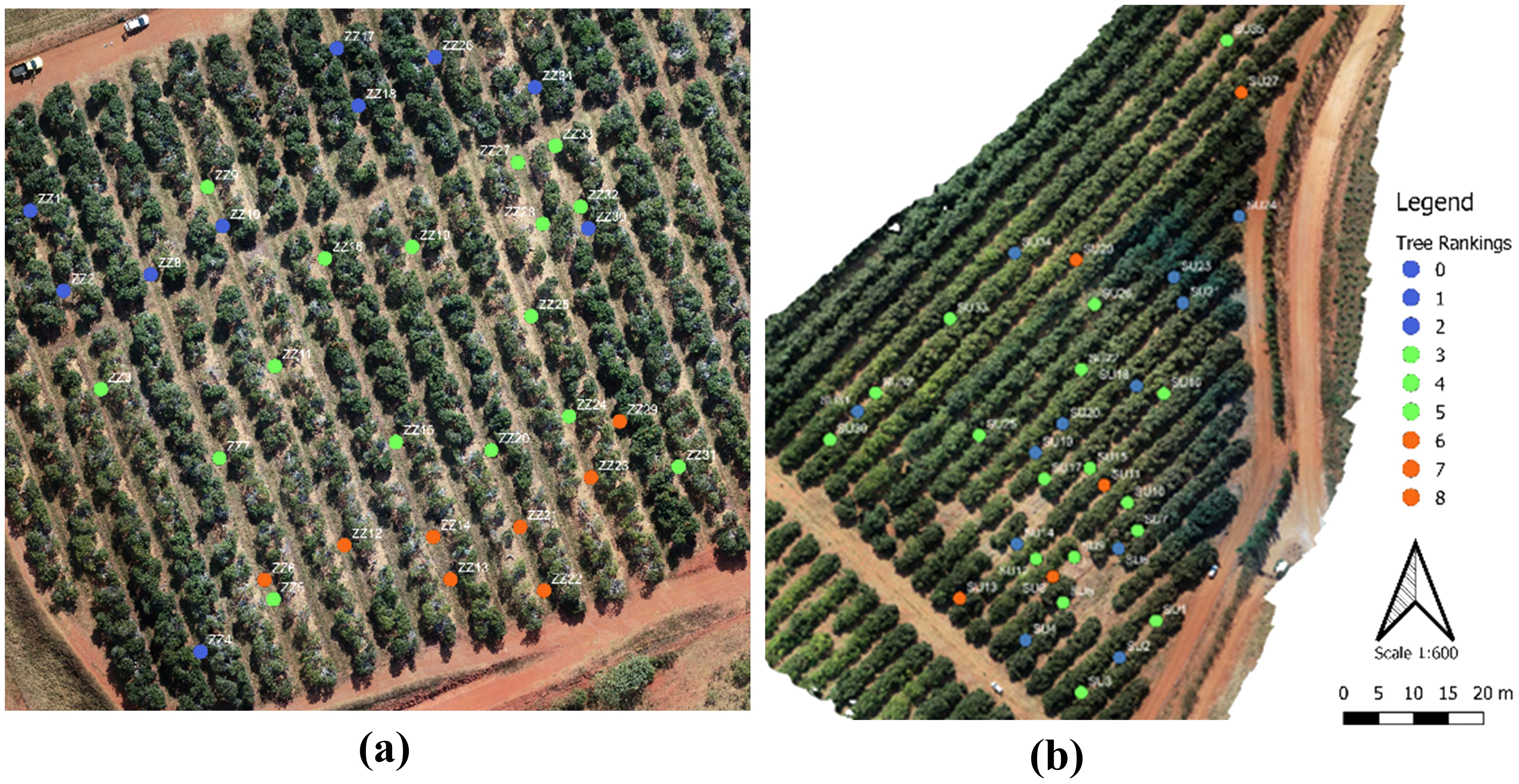
Figure 3. Locations of target trees. (A) Block 1 and (B) Block 2. Images from UAV (acquired in May 2021).
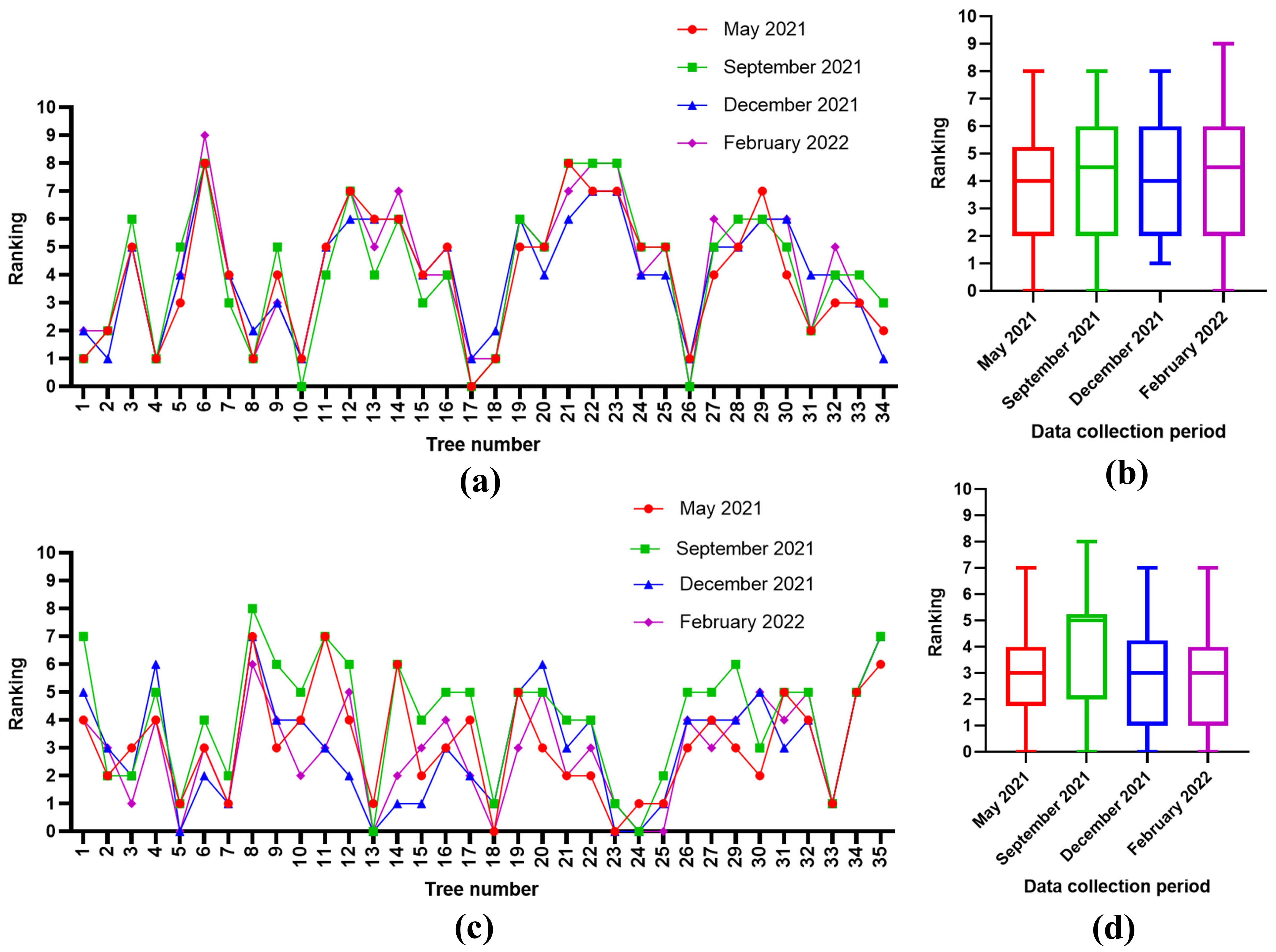
Figure 4. Target tree severity rankings for each tree in block 1 (A) and block 2 (C), and boxplots of the severity rankings for each period in block 1 (B) and block 2 (D).
3.2 Plant projective cover (fcover) (RGB-UAV imagery)
fcover was calculated of each target tree in each period using the RGB-UAV imagery as a percentage of a specific selected rectangle size. Figure 5 shows an example of the tree extraction method used to calculate fcover based on tree height. Figure 5A shows the RGB image of a section of the orchard in block 2 while Figure 5B shows the RGB image of the target tree from which the fcover is calculated. Figures 5C, D, respectively, show the DEM where the ground is eliminated using the height thresholding technique. fcover for block1 ranged between 22% and 100% with a median of 48% (Figure 6A). The standard deviation was 15%, indicating some variation. While fcover for block 2 ranged between 71% and 100% with a median of 83%. The standard deviation was 6%, indicating little variation (Figure 6B).
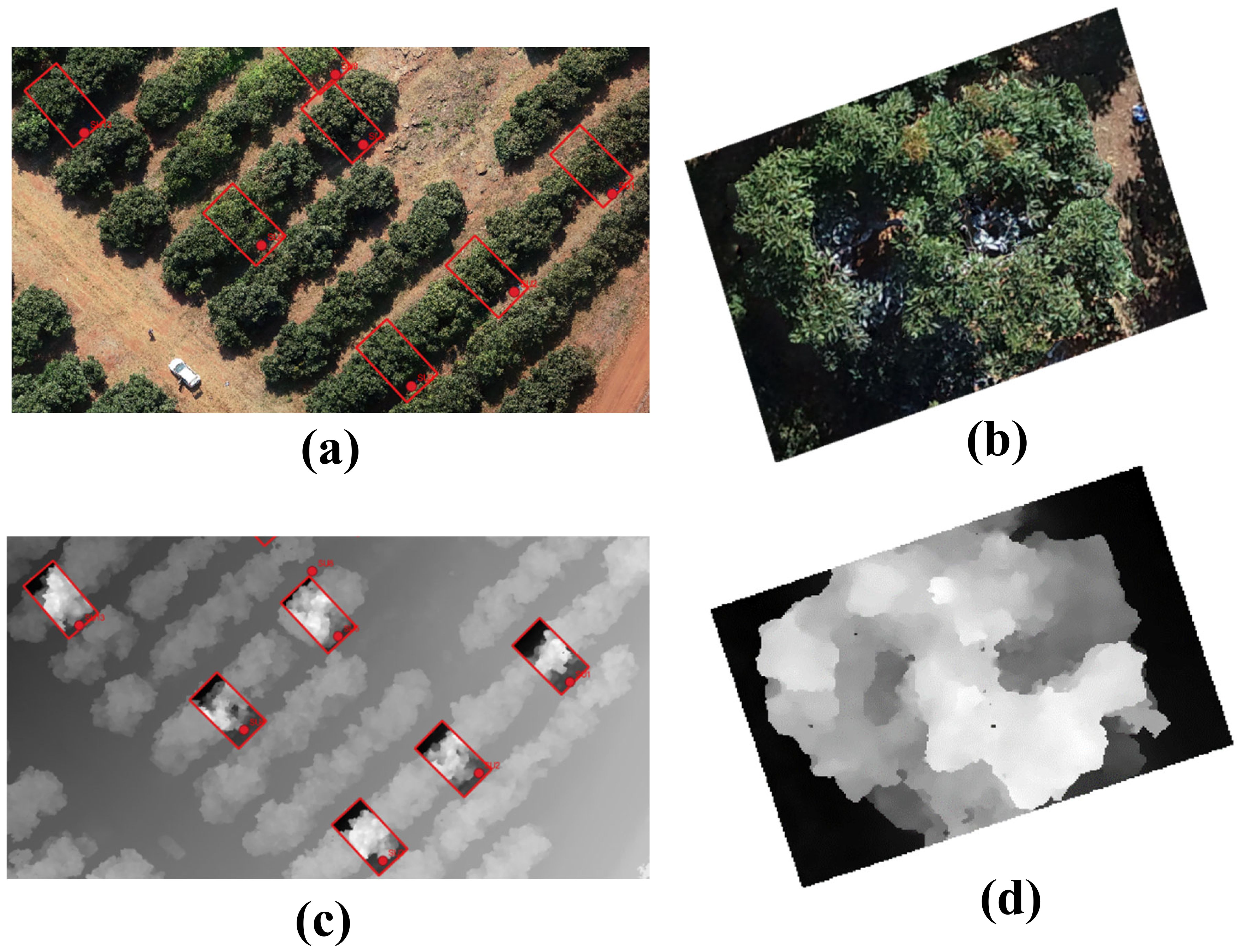
Figure 5. Example of single tree extraction. RGB image of a selected area (A), RGB image of a single tree (B), DEM image of a selected area (C) and DEM image of a single tree (D).
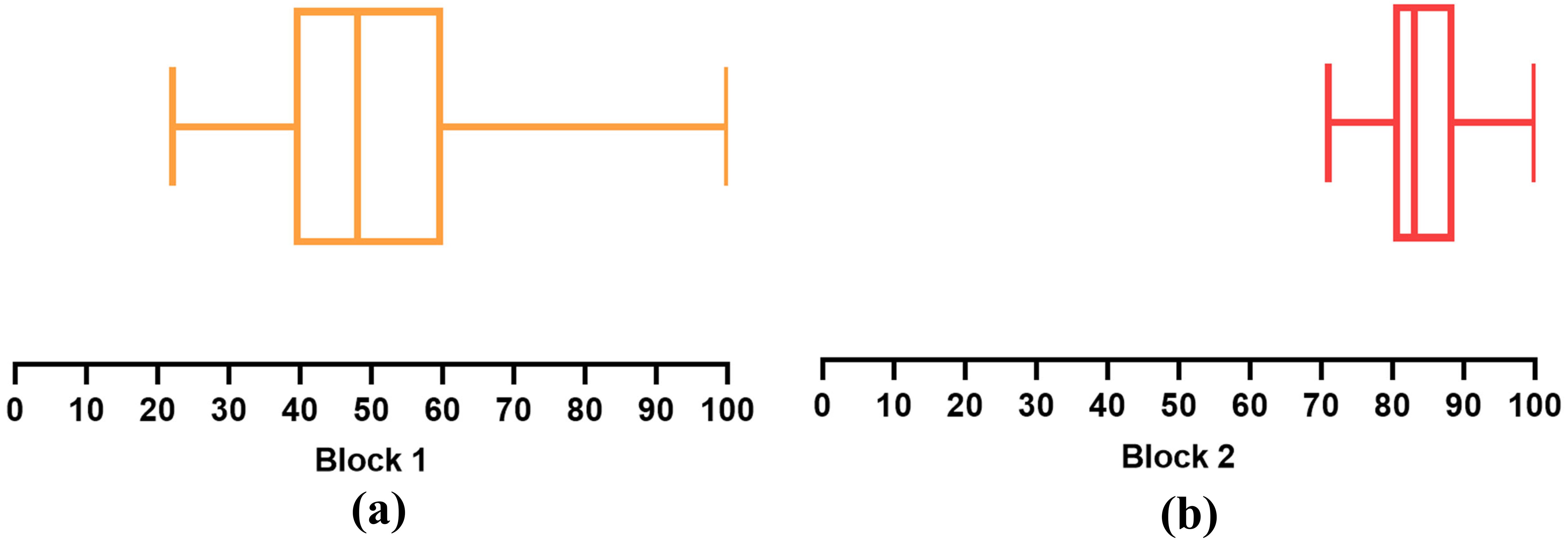
Figure 6. Boxplots of the fcover incorporating all data collection periods for block 1 (A) and block 2 (B).
In block 1, the linear regression analysis indicated a significant correlation between fcover and the severity ranking for all the studied periods with R2 values ranging between 0.52 and 0.65 (Figure 7). Promising results were obtained in the PH period (Figure 7A). The F period also achieved high R2 values (Figure 7B). The ED and LD periods produced the lowest R2 values (Figures 7C, D). Furthermore, in block 2, the regression analysis showed a low correlation with R2 values of, 0.30, 0.01, 0.26, and 0.48 for PH, F, ED, and LD periods, respectively (Figure 8). Better results were achieved in the PH and LD periods (Figure 8). These align with the results from block 1. Block 1 received intense pruning while block 2 received mild pruning and the canopies were kept large. It is important to note that pruning is done to improve the sunlight entering the inside of the canopy and is therefore executed irrespective of PRR-infections.
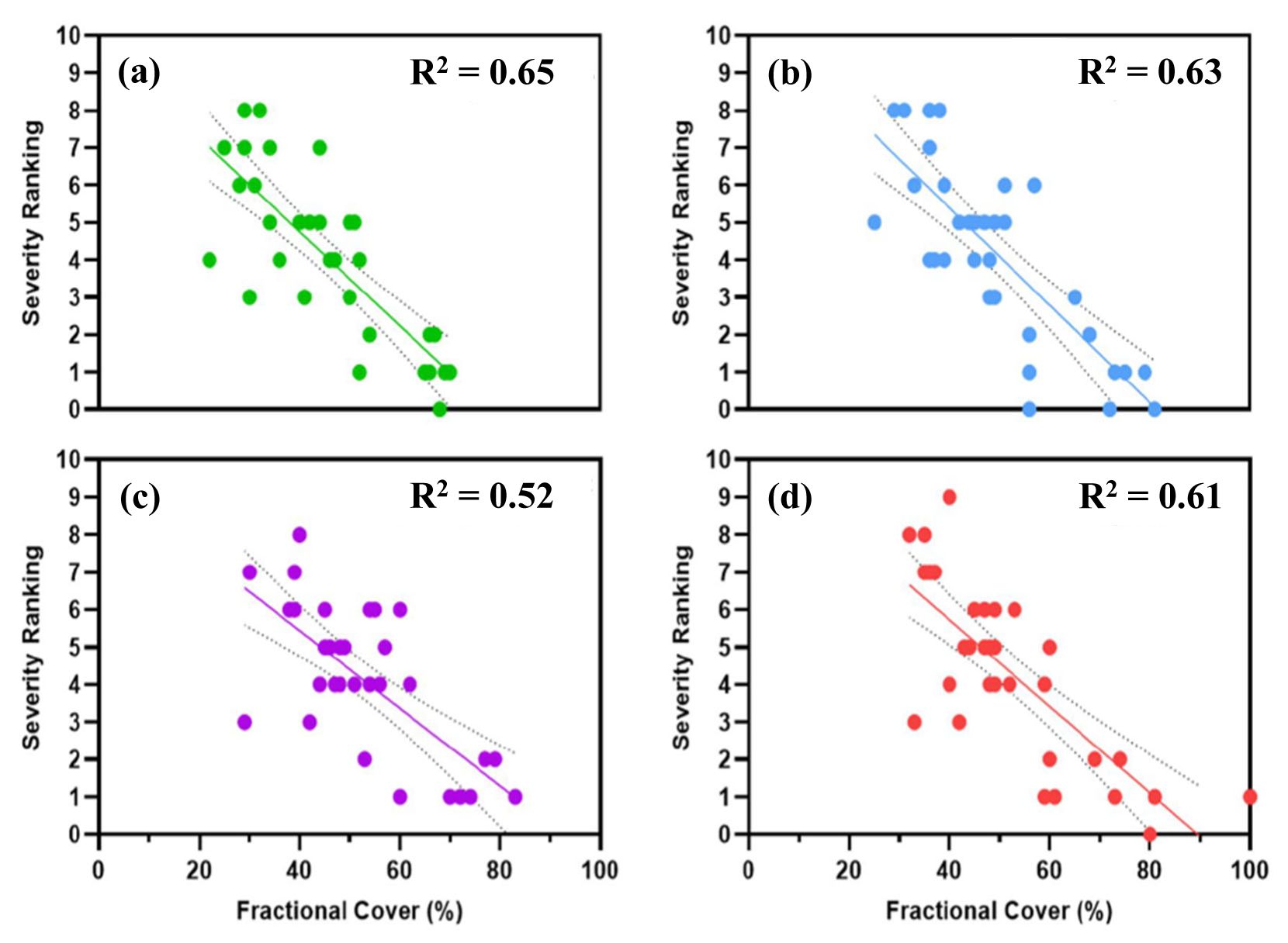
Figure 7. Linear regression between severity ranking and fractional cover of block 1. (A) May 2021, (B) September 2021, (C) December 2021, and (D) February 2022.
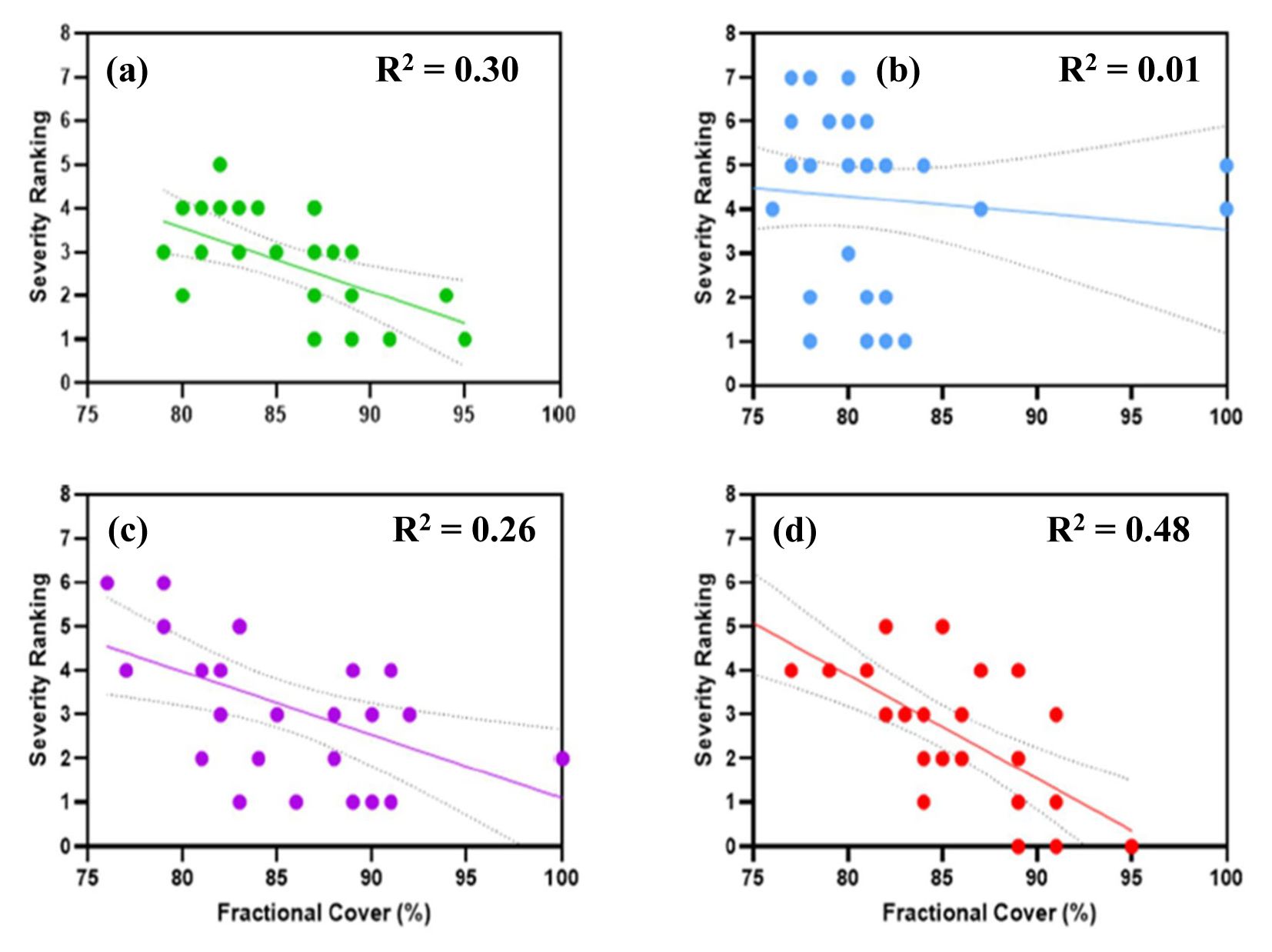
Figure 8. Simple linear regression between severity ranking and fractional cover of block 2. (A) May 2021, (B) September 2021, (C) December 2021, and (D) February 2022.
3.3 Vegetation indices
3.3.1 Super high-resolution (UAV imagery)
In order to simplify the identification, the VI’s that provided a good agreement with the severity ranking, an R2 threshold of 0.65, was defined (Table 4). Block 1 achieved very promising results, specifically during the PH and F periods where 25 and 27 VI’s respectively achieved correlations of 0.65 or more. Block 2 only achieved correlations of 0.65 or more (six VI’s) in the PH period. Furthermore, in block 2, the F period achieved correlations of 0.50 to 0.57 in six VI’s while the remaining two periods only achieved 0.40 as the highest. In both blocks, the individual bands were not good indicators. Although the green band in the PH period, block 2 received a correlation of 0.51.
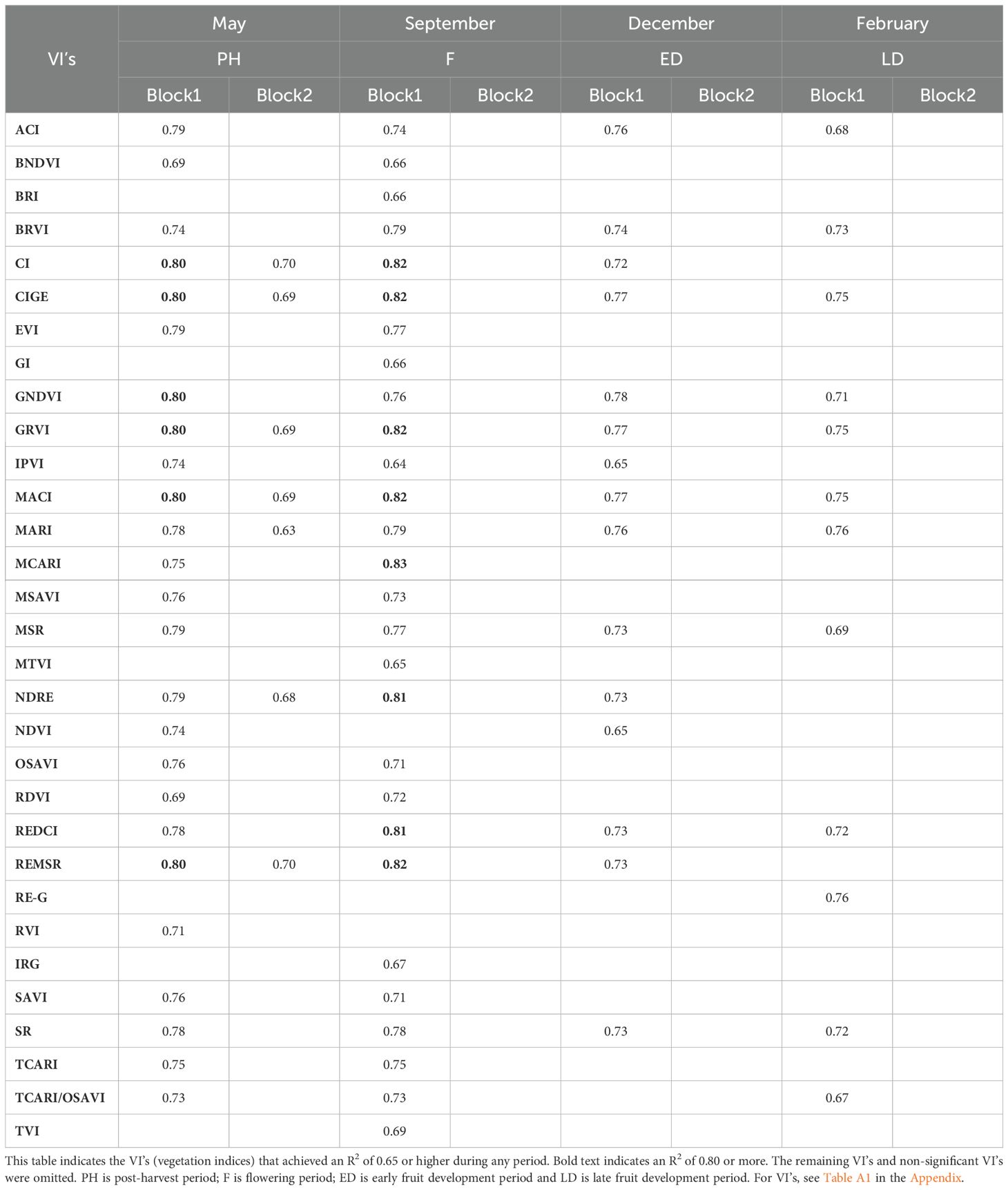
Table 4. Correlation coefficients (R2) of the comparison between severity ranking and VI’s obtained from super high-resolution imagery (UAV).
Six VI’s achieved correlations of 0.80 in the PH period for block 1: GNDVI, REMSR, CI, GRVI, MACI and CIGE. These bands also performed well in block 2 during this period, but not as high. In block 1, potential VI’s across all the periods were ACI, BRVI, CIGE, GNDVI, GRVI, MACI, MARI, MSR, REMSR and SR. They achieved higher than an R2 of 0.70 in each period. However, after performing basic statistical analysis, the top five VI’s (considering standard deviation and R2) all include IPVI, ACI and BNDVI. Although the R2 for block 2 was substantially lower, these VI’s were also amongst the top five for all periods. Block 2 did not achieve any consistently high correlations. Although CI, CIGE, GNVDI, GRVI, MACI, MARI, NDRE and REMSR were relatively consistent from PH to F. Additionally, the R2 values appear to decrease as time progressed.
3.3.2 High-resolution (satellite imagery)
0.50 m March LD and 0.50 m bud development data sets achieved the highest results for block 1 with regression values of 0.64 and higher while 0.75 m LD and 0.50 m F achieved the highest in block 2 with regression values up to 0.55 (Table 5). Although the correlations are not as high as the UAV imagery correlations, they were still relatively high with CIGE, GRVI, and GNDVI (block 1 LD period 0.50m); TCARI, TCARI/OSAVI (block 1 LD period 0.75 m); MSAVI and GNDVI (block 1 bud development period 0.50 m); and TCARI/OSAVI (block 1 F period 0.50 m) being the highest in the respective periods. In block 2, the highest correlation was in the LD period (0.75 m), TCARI, followed by GARI and TCARI/OSAVI in the same period with the latter achieving the same correlation in the F period. Otherwise, block 2 achieved low regression values, with no strong correlations. The indices that achieved high correlations in block 1 correspond to some of the high performers in the UAV imagery. Although no clear pattern is evident, TCARI/OSAVI, CIGE, GRVI, GNDVI, SR, GARI, and REDCI showed some consistency across all the collection periods.
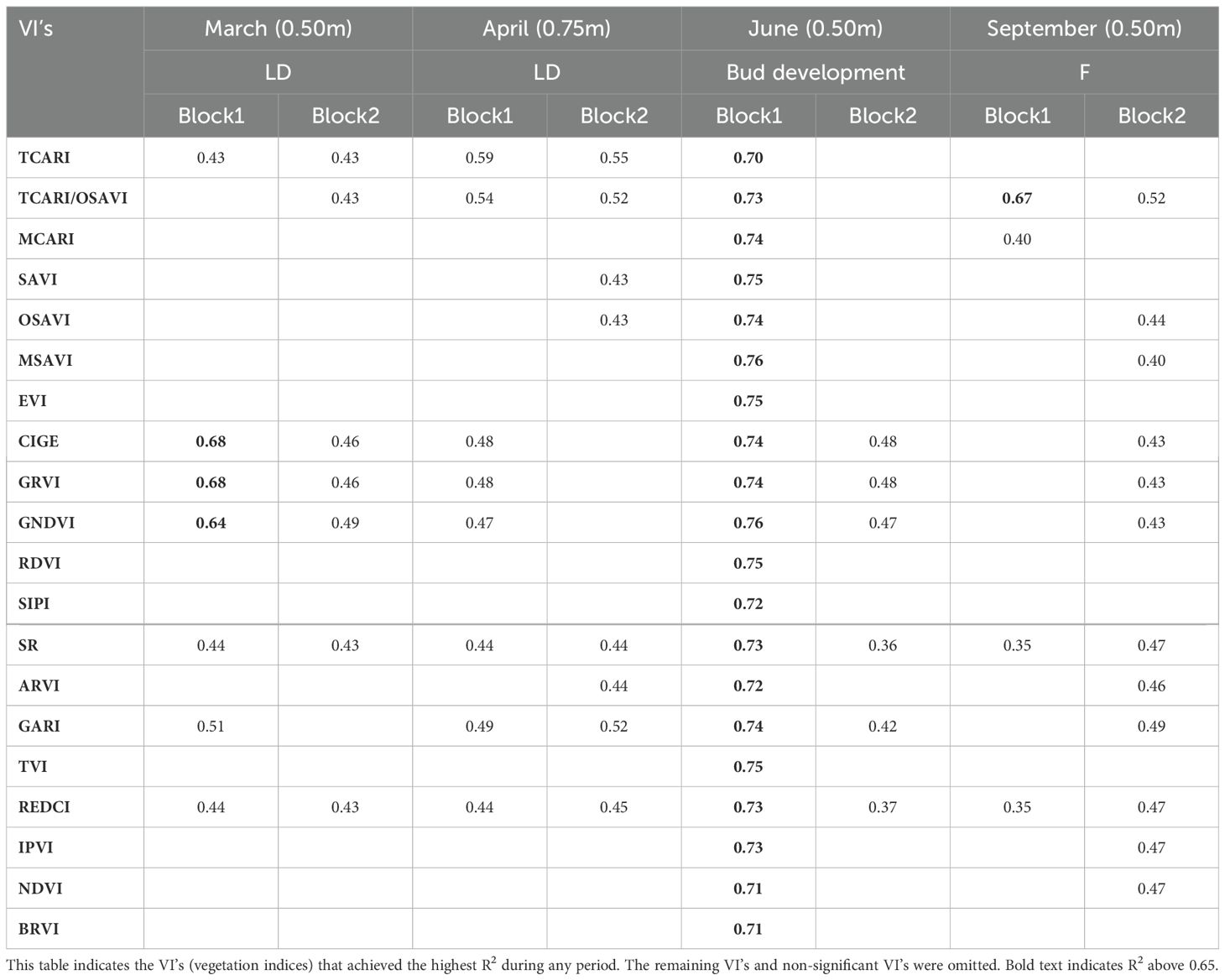
Table 5. Correlation coefficients (R2) of the comparison between severity ranking and VI’s obtained from high-resolution imagery (Satellite).
3.3.3 Medium-resolution (satellite imagery)
Block 1 VI’s showed more promise, however, both blocks achieved low correlation values (Table 6). Again, PH imagery appeared to show the most promise but only in block 1. Block 2 performed extremely poorly. GNDVI seemed to perform the best. Values obtained during LD were significantly lower than in the other periods. In addition to the other RS techniques, CIGE and GRVI also did well in block 1, PH. In addition, RDVI achieved a relatively high correlation. GNDVI, CIGE and GRVI performed well in the UAV imagery, and did relatively well in the high-resolution satellite imagery indices.
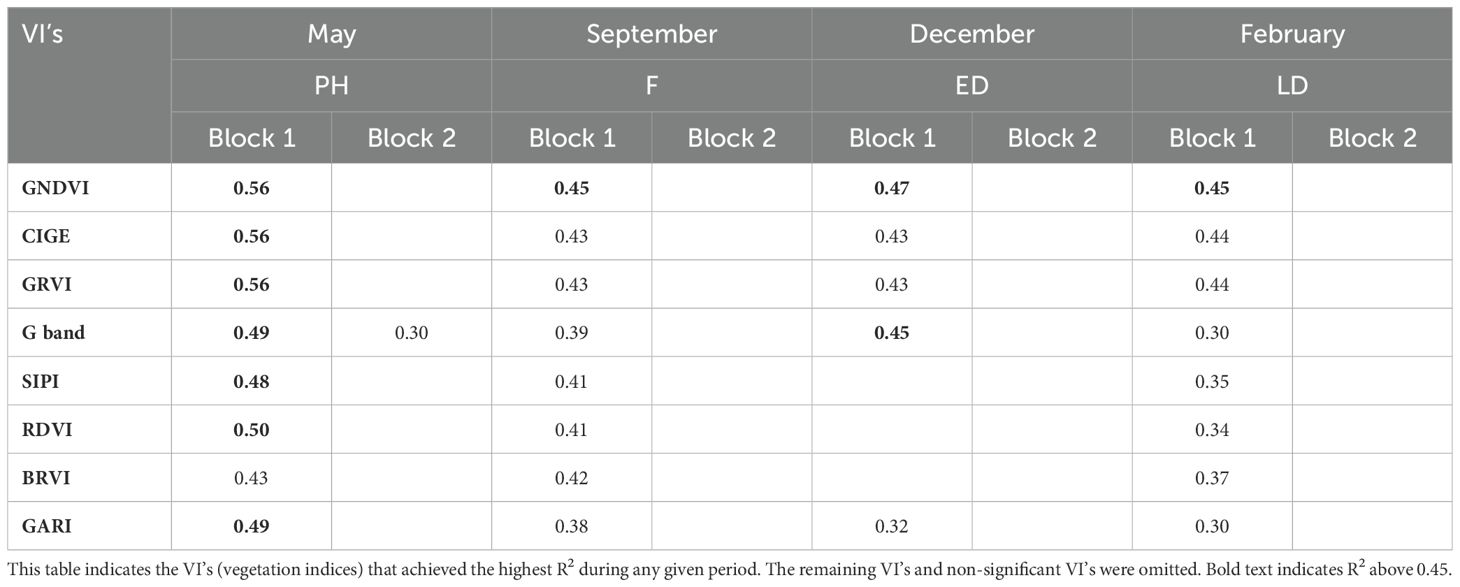
Table 6. Determination coefficients (R2) of the comparison between severity ranking and VI’s obtained from medium-resolution imagery (Satellite).
4 Discussion
4.1 Severity rankings
Severity rankings were acquired from each target tree over four data collection periods by trained agronomists. Severity rankings were consistent in terms of spatial patterns and did not change drastically over the growing season. The lowest severity rankings present in the PH period in both blocks could potentially be due to the presence of matured leaves and no flush or fruit, which may interfere with the structural properties of the canopies (Figure 4C). The presence of fruit and flowers could affect the rankings due to their being high-energy sinks (Rahman et al., 2022). Flushes occur in spring (F period, superimposed on flowering), therefore competition for nutrients and water occurs (Rahman et al., 2022) and summer (LD period).
From a practical point of view, the visual screening of severity rankings in block 1 was simple owing to the flat terrain, smaller canopies, and minimal ground cover vegetation. Symptom expression in this block was clearer, which was potentially due to the smaller, less vigorous canopies that were as a result of intense pruning. Intense pruning on this farm is where major limbs are removed to maintain orchard access between rows and enhance light interception. On the other hand, block 2 was slightly more complex due to the sloped terrain, large and high-vigor canopies, and overgrown ground cover vegetation. The pruning level had a strong influence on the screening process.
Selected blocks were well-irrigated; therefore, it is not likely that canopy decline was a result of water stress. On the contrary, in block 1, high infections may be due to a surplus of water in the south-eastern section of the block, which is close to a water body and at the bottom of a slight slope. This factor, including poor-draining soils and high soil temperatures, is the optimal environment for PRR. Salgadoe et al. (2018) found that the distribution of trees in severe decline was in areas attributed to water logging conditions and lower in elevation, much like block 1. Although mulch is used in this block to help combat PPR infections, it seems they are still present. On the other hand, healthy trees appear to be situated at the northern side of the block, which is at the top of the slight slope. High infections in block 2 do not seem to indicate a distinct pattern.
4.2 Super high-resolution (UAV imagery – fcover)
In our case, super high-resolution refers to pixel size of <0.05m obtained by MS imagery with the sensor mounted on a UAV flown at an altitude of 55m. From this UAV imagery, fcover and VIs were evaluated as indicators of PRR severity. VIs obtained from UAV imagery are discussed in the next section. The fcover obtained by UAV imagery changed over time so the correlation with PRR severity rating varied according to the phenology. In block 1, promising results with high correlations were obtained in the PH period where only mature leaves were evident (Figure 8). The high R2 in the F period could be due to the first flush growth and limb removal having taken place soon after the PH period. The ED and LD periods produced the lowest R2 values which could potentially be due to the trees bearing fruit (thereby slightly stressed) and because limb removal pruning was conducted in November 2021 thereby decreasing the canopy biomass. It is important to note that pruning is done to improve the sunlight entering the inside of the canopy. Pruning is performed for light interception purposes so that fruit set occurs on the inside as well as the outside. Therefore, pruning is independent of PRR severity, i.e., branches with dieback are kept on the tree unless they are blocking the sun from entering the center of the tree.
In block 2, better results were achieved in the PH and LD periods (Figure 8) although correlations were low. These align with the results from block 1. Interestingly, the F period showed no correlation and the fcover values all seem to be slightly lower than the rest of the periods. The poor results of block 2 are potentially due to the low pruning intensity, high vigor and large size of intertwining canopies resulting in minimal changes in fcover over the study period. Additionally, block 2 had high ground cover vegetation and this potentially interfered with the calculation. It is clear that using fcover as a method to determine PPR severity is not adequate in this case of mild pruning and large canopies. The fcover is a good indicator of light interception, which is a crucial determinant of crop growth and important for flowering, fruit maturation, and quality as well as reducing disease and pest incursions for horticultural tree crops (Wu et al., 2020).
Salgadoe et al. (2018) found similar results of a strong linear relationship between canopy porosity percentage and the ‘Ciba-Geigy’ rating scale with an R2 of 0.97 in the study of PRR in avocado. In this study, the canopy porosity was defined as “the proportion of the sky area visible within the perimeter of the crowns of individual plants”. Therefore, the concept is like fcover with the difference being the sky was used as the background instead of the ground. Tu et al. (2019) used the same parameter (with the ground as the background) to investigate a condition ranking strategy on avocado trees where they assessed tree crop condition directly related to canopy structural attributes estimated from UAV image data and used the data to rank avocado tree condition. One of the measured parameters was fcover and the authors produced an R2 value of 0.62 against field data. The fcover relationship with PRR severity ranking will depend on orchard management practices i.e., if farmers only prune branches with severe die-back, it will affect the relationship, therefore it is an important factor to consider when using it to identify the severity of a tree.
4.3 Vegetation indices
4.3.1 Super high-resolution (UAV imagery)
In terms of spectral information, it is well known that the reflectance of visible light is influenced significantly by the concentration of photosynthetic pigments, while the reflectance of NIR is sensitive to the leaf and canopy structure (Rahman et al., 2022). Also, literature indicates that the spectral reflectance of trees is impacted by the orchard location (i.e., topography, slope, sun angle) and growing season (i.e., phenological phase) (Robson et al., 2017). Therefore, VIs can provide valuable information in order to assess PRR severity in avocado orchards.
From our results, VI’s containing the NIR band achieved greater correlations. The six highest correlations in block 1: CI (containing NIR and RE bands) and CIGE (containing NIR and G) determine the amount of chlorophyll in plants and PRR-infected plants display lighter color leaves. GNDVI (containing NIR and G) estimates photosynthetic activity (as well as GRVI (containing NIR and G)) and is commonly used to determine water and nitrogen uptake into the plant. PRR-infected trees have reduced water uptake ability. MACI (containing NIR and G) measures anthocyanin content and weakening vegetation contains higher concentrations of anthocyanins, so this VI is a good indicator of stressed vegetation. REMSR (containing NIR and RE) is a good indicator of stress. A study done on banana trees infected with Fusarium wilt disease also found the CIGE, along with REDCI, NDVI, and NDRE, to easily discriminate between healthy and unhealthy plants (Ye et al., 2020).
All these indices contain a combination of NIR, RE and G which are common in health detection. Stressed vegetation produces lower solar reflectance in NIR and when the tree is infected with PRR, the amount of chlorophyll in the leaves is reduced (Gitelson et al., 2003). Even though the flowers altered the spectral properties of the imagery, the F period still proved to supply high correlation results in block 1. RE wavelength is more sensitive to flushing and thus more effective for the detection of flushing related to tree health (Chang et al., 2020). This could explain why the RE-related VI’s achieved higher correlations in the F period in block 1. The low standard deviation of the VI’s in block 2 were was potentially due to all the canopies being large and having high vigor and therefore not much variation is evident in the VI’s. Calculating the VI’s for block 2 proved to be slightly problematic due to the site being on a relatively steep slope and dense ground cover was present.
The mask technique was used to eliminate spectral disturbances of other coverages such as ground vegetation, shadows, soils, understory (more applicable to sparse trees evident in block 1) etc. A comparison was done between VI correlations calculated using the mask technique versus not using the mask for block 1 (Table 7). Fewer differences occurred in February (wet season, received 13cm of rain) while higher differences occurred in December (wet season) and May (dry season, almost no rain). Except for SAVI, where the highest differences were in February and September. The VI results from the ‘no mask’ analysis were generally worse than those that used the mask (Table 7). Perhaps a slightly lower difference in the IPVI can be attributed to the fact that IPVI comprises of atmospheric and soil corrections (Huete et al., 1999). GNDVI and NDVI also had very small differences while ACI and BNDVI had the largest differences. To conclude, the effect of the mask technique was not great, the soil and ground vegetation did not appear to have much effect on the index in the case of no mask. However, subtle differences are present, and these may have contributed to the favorable results of the high correlations.
Shadows may lead to variation in VI’s. Shadows are evident in the imagery, and these may lead to the overestimation of NDVI, SAVI and SR and an underestimation of some VI’s such as RVI1 (Ranson and Daughtry, 1987) As in the Garcia-Ruiz et al. (2013) study, the shadow might have led to higher variation in some indices in all the imagery.
The VI’s with the most potential, IPVI, ACI and BNDVI, have not been recorded as efficient in the study of PRR in avocado trees. NDVI, GNDVI, and SAVI are some of the most frequently used VI’s in agriculture and of the three, only one (GNDVI) has featured relatively significantly in this research. GNDVI has been found to be a robust spectral feature in the detection of stress in citrus orchards and may also be more effective than NDVI at detecting changes in vegetation caused by factors such as disease (Sankaran et al., 2013). GNDVI is more sensitive to the variation of chlorophyll content, it works well in crops with dense canopies or in more advanced stages of development (Sankaran et al., 2013). De Castro et al. (2015) evaluated the spectral requirements for quick and accurate detection of laurel wilt infected avocado trees using MS imagery. They found GNDVI was among the optimum VI's for detection. Garcia-Ruiz et al. (2013) studied Haunglongbing infections in citrus trees using UAV-MS imagery, finding that healthy canopies are expected to have higher values of NDVI, GNDVI, SAVI and S among others. In this study, GNDVI was one of the most statistically significant VI for block 1 while NDVI, SAVI and SR fared well. Furthermore, they found that SR had a greater difference between healthy and unhealthy trees. This agreed to findings from this study where SR achieved high correlations in block 1. Interestingly, our study did not indicate that NDVI was among the best discriminating factor of severity while a study by Pérez-Bueno et al. (2019) who used UAV-MS imagery to detect white root rot in avocado trees found that NDVI showed significant differences between diseased and healthy avocado trees.
Chang et al. (2020) studied citrus greening disease using airborne MS imagery. Focusing on NDVI, NDRE, MSAVI and CI, they found that RE-related VI's showed a higher capability for citrus orchard monitoring. MSAVI outperformed in quantifying crop status, and CI was the most efficient feature for monitoring citrus greening infection. Additionally, other works done on citrus greening disease by Li et al. (2012) also found that CI discriminated well. These findings agree with this study where NDVI, NDRE, MSAVI and CI achieved relatively high correlations with CI achieving the highest. However, CI wasn’t good statistically.
ACI, developed by van den Berg and Perkins (2004), is a VI that increases in response to increased anthocyanins as green leaf reflectance drops (Roberts et al., 2011). In our study, the values of ACI tend to increase in the LD period (February) and the F period (September), which corresponds to higher severity rankings and bearing large fruit or flowers. Large fruit and flowers may be changing the spectral reflectance values in the LD and F periods, which may add stress to canopies, thereby reducing green leaf reflectance in the leaves. While the absence and small size of fruit in PH and ED periods respectively, may be decreasing the values of ACI. BNDVI, or blue NDVI, is often used for areas sensitive to chlorophyll content and has been found to make the best separation between healthy and leaf curl infected peach trees (Pourazar et al., 2019). PH and LD period have the lowest BNDVI, perhaps this is due to the absence of flush.
Radiometric calibration is a prerequisite when using spectral information, especially when in the case of multi-temporal investigations, it would be needed as the conditions will not be the exact same (e.g., different light conditions) which will affect the data validity (Pourazar et al., 2019; Tu et al., 2019). Even though Pourazar et al. (2019) and Candiago et al. (2015) found that radiometric calibration had an insignificant effect on classification results and a good description of crop condition was still achieved, these authors did not investigate the affect over multiple time periods. Their results were therefore mainly qualitative, unlike the results of this study where the spectral information of the trees was normalized through the use of radiometric calibration.
In terms of flight altitude, the results of this study show that it is a crucial factor for aerial image acquisition due to its impact on spatial resolution and spectral resolution. Lower altitude allows for higher spatial resolution while higher altitude imagery has a lower spatial resolution. Our study shows that 50 to 60 m flight height is suitable for detecting PRR in avocado orchards. Similarly, Sankaran et al. (2013) found that 60 m altitude is more suitable for stress detection in citrus orchards. While De Castro et al. (2015) found that 250 m was adequate in the detection of avocado trees infected with laurel wilt. Furthermore, they argue that any of their tested altitudes (180 m, 250 m, 300 m) would be able to discriminate an avocado tree infected with laurel wilt. However, the accuracy of this statement depends on factors like sensor selection coupled with the selected altitude. A sensor with low spatial resolution acquiring imagery from higher altitudes may result in poor quality imagery (e.g., imagery impacted by shadow effects and pixel-mixing) and therefore may not be able to detect disease accurately.
To quantify leaf and canopy level infections at low altitude, one would need to obtain accurate VI’s (Kubera, 2013). Therefore, one needs good quality MS orthomosaics, and furthermore, good quality greyscale MS images. The reason is because the greyscale MS images are ultimately “stitched” together to create the multi-band orthomosaics from which the VI’s are calculated. Issues like varying canopy height and movement from the wind can reduce the quality of the greyscale image and further reduce the already limited number of tie points (in comparison to RGB imagery) that are inherent in the greyscale images. The lower number of key points is usually the reason for RGB imagery having a higher resolution.
4.3.2 High-resolution (satellite imagery)
In our study, high-resolution refers to pixel size of 0.50 m and 0.75 m obtained by satellites such as SuperView, JPSS1 and ViewReady. The pixel-mixing effect is much stronger in the case of satellite imagery as the masking technique was not used and pixels are larger than those from the UAV imagery. Pixel-mixing with the shadows, soil, understory, adjacent ground cover in the row spaces such as mulch (block 1) and weeds/cover crops (both blocks) was evident, and it is highly likely that it disturbed the spectral values of the canopies. Adding to the complication is the seasonality of the understory or space between rows and management thereof as they change from season to season and depend on management practices and therefore impact multi-temporal spectral datasets (Hornero et al., 2020). In rainy seasons (Summer) it may be fully covered with weeds while in dry season (Winter) it may just be bare earth. This may have an impact on the variability of results where June (dry) achieved better than the other periods in block 1. Shadow effect may also be evident and cause variability and lower correlations. The 0.50 m June imagery having the highest correlations in block 1 aligns closely with the UAV imagery, where the month of May had the highest correlations. Following is the 0.50 m March imagery, which would align with the LD period. This month does not align with the UAV imagery.
Robson et al. (2017) used high-resolution WorldView-2 and WorldView-3 satellite imagery to map yield in avocado orchards. With a multi-temporal approach, they used NDVI to successfully determine three vigor classes, extracted VI’s for each sample tree and determined the yield. In this research, NDVI did not perform well, except in block 1 June imagery. A study done by Salgadoe et al. (2018) investigated the use of WorldView-3 imagery to quantify the severity of PRR in avocado trees. They found that SR (NIR/R) had the best correlation (R2 = 0.96) with the disease rankings, and it was able to detect early decline. The spatial resolution of the WorldView-3 imagery was 1.2 m and the imagery was from the month of April. In this study SR achieved a determination coefficient of 0.73 in June for the 0.50 m resolution satellite imagery, therefore achieving similar results.
A study done by Johansen et al. (2020) found that the NIR band, RDVI, SIPI, GNDVI and NDVI, among others, had the highest importance when investigating macadamia tree condition using UAV images and WorldView-3. Although the analysis method differs to this study (machine learning approach), the results are still telling. RDVI achieved good results in this study in the high- and medium-resolution satellite imagery while GNDVI, NDVI, SIPI achieved promising results in the medium-resolution imagery. A study done on fire blight in pears also found RDVI, along with TCARI among others, to be useful in the early-stage detection there of (Bagheri, 2020).
Other than the 0.50 m imagery acquired from June and the three high correlation indices from March, the VI values for both blocks were relatively similar. This suggests that the effect of canopy management using this technique is not as clear as the UAV technique. This is due to the resolution being too low and not being able to discern the finer details of the canopy. From this section of this research, we can suggest that CIGE, GRVI, GNDVI, MSAVI and OSAVI be further investigated in the PRR detection as they have shown promise in the LD and bud development periods.
4.3.3 Medium-resolution (satellite imagery)
In our case, medium resolution refers to pixel size of 10 m obtained from S2B satellites. Although some correlations were evident, VI results still performed poorly in this case of medium resolution imagery. Medium resolution satellite imagery will not provide telling results due to the lower resolution, especially in crowded orchards, causing low precision and accuracies. One of the main contributors is the mixed-pixel effects. These make it challenging when attempting to separate the contribution of the different canopy-scene components such as soil, shadows, and understory, particularly in open vegetation canopies (Hornero et al., 2020). In terms of avocado trees, they are usually planted close to each other, (specifically in block 2) where their canopies overlap. Along with the pixel-mixing of the trees, there is also the issue of pixel-mixing with the understory such as mulch (block 1) and weeds/cover crops (both blocks). Adding to the complication is the seasonality of the understory or space between rows. In rainy seasons it may be fully covered with weeds (December and February) while in the dry season (May and September) it may just be bare earth. This may partly explain why results from May and September were generally better than those from December and February in block 1. Shadow effects may also interfere with the spectral properties. At this low resolution, canopy management practices may not play a role in the spectral properties as the 10 m pixels are too large to describe these finer details.
In terms of the VI’s, GNDVI seemed to perform the best over all the periods in block 1, this is no surprise as this index is a chlorophyll index and measures the plants’ “greenness” or photosynthetic activity. It is one of the most widely used vegetation indices to determine water uptake in the canopy. This index is mainly used in the intermediate and final stages of the crop cycle therefore proving the highest results in the PH period. In addition, CIGE, used to estimate the content of leaf chlorophyll, and GRVI, sensitive to leaf pigments, did well in block 1, PH period. Perhaps indicating that the mature leaves were not under stress from other factors i.e., fruit or flowers draining energy. GNDVI, CIGE and GRVI did well in the high-resolution imagery for June (a month after PH) and March (two months before PH) and, additionally, did well in the UAV-derived indices for the PH period. This shows that results agree, and that compatibility is highly potential.
Availability of frequent MS imagery data from S2B offers the opportunity to assess both spatial and temporal variation. However, not at tree canopy level but rather at block or orchard level. This RS technique could be used as a ‘pre-screening’ procedure to identify potential diseased trees (Pérez-Bueno et al., 2019). Using S2B data, Hornero et al. (2020) found that VI’s that minimized atmospheric and background effects, ARVI and OSAVI, performed better than the traditional VI’s such as NDVI, RDVI, and MSR. Further, they found that ARVI and OSAVI can be used to monitor orchard-level changes in disease incidence when investigating a xyllela fastidiosa disease in olive trees. However, they also found that the effects of the understory had a considerable impact on the VI’s. Similar results were obtained in this study but for high-resolution satellite imagery (0.50 m). A study done on banana trees by Selvaraj et al. (2020) used similar platforms to this research. They also investigated UAV imagery along with multi-level satellite images. From S2B imagery, they found that it could not map bananas as accurately as UAV and high-resolution satellite imagery. Rahman et al. (2022) investigated the potential of S2B data for monitoring avocado crop phenology. They were able to show statistically significant differences between four phenological periods using EVI. This index did not feature in this present study.
4.4 Comparison among platforms
The super high-resolution imagery obtained the best results, in both blocks, although block 2 was significantly lower. This indicates that UAV-MS imagery can be a good indicator of PRR severity, especially in the PH period, but it still is not good enough to overcome the issue of large canopies where the symptom expression was not clear. Major limitations in UAV-MS imagery include coverage, costs, image processing time and weather conditions. Although UAV’s are not affected by cloud cover as with satellite imagery. High-resolution satellite imagery could prove to be a good indicator of infection at block and orchard level, specifically in the LD and bud development periods. Pixel-mixing, cloud cover and sun angle are major concerns in high-resolution satellite imagery. Image acquisition, temporal resolution, and sensor selection flexibility is less than UAV imagery and cost is around the same. A critical challenge with satellite imagery is spatial resolution (Zhang et al., 2020). Although high-resolution satellite imagery can have spatial resolution up to 0.31 m, the satellite image is still not as good as those acquired through UAV-based sensing and it is not suitable for application where the plant traits are subtle (Zhang et al., 2020). Medium-resolution imagery could provide an indication of high severity on an orchard/farm scale. The resolution is too low to detect single tree, and the pixel-mixing effect is too prominent. Although S2B imagery is free, it is not suitable for precise PRR detection.
5 Conclusions
In this study, we explored the use of RS techniques for mapping the severity of PRR in avocado orchards considering the spatial resolution effect, phenological periods, and canopy conditions modulated by pruning intensity. Ground truthing of healthy and PRR-infected trees with various rankings, along with their GPS position, were recorded and the trees were identified in and segmented from all the RS imagery.
Regarding RGB-UAV imagery, we tested fcover as an indicator of ranking severity. The results show that fcover relationship depends on orchard management practices since this parameter is highly influenced by the level of pruning, vigor size of canopies, and tree spacing. In block 1 with a high pruning level, significant relationships were registered for all phenological periods analyzed. However, in block 2 there were poor results due to the high-vigor and size of canopies. Under these conditions, it is clear that fcover obtained from an aerial view is not an adequate parameter to determine PPR severity due to the lack of sensitivity to canopy changes.
VI’s obtained by MS imagery were also analyzed as potential indicators of PRR ranking severity, in this case, the resolution effect was tested using different platforms to obtain super high-, high- and medium-resolutions. VI’s CIGE, GNDVI, and GRVI, were found to be significantly different, while having a good correlation with the ground truth, at all the spatial resolutions. Therefore, the highest correlation VI’s were recognized as suitable indicators of PRR severity. Prior to statistical analysis, ACI, BNDVI, and IPVI were deemed have the best potential for PRR severity in UAV-based MS data. Furthermore, the results showed that the relationship with top-performing VI’s also depends on orchard management practices since VI’s in block 1, that received limb removal pruning, achieved better results in all the different resolutions tested. The results indicate that UAV-MS imagery can be a good indicator of PRR severity, but it still is not good enough to overcome the issue of large canopies.
High-resolution satellite imagery could prove to be a good indicator of the general area of infection, specifically in the LD and bud development periods. On the other hand, medium-resolution imagery could provide an indication of high severity on an orchard/farm scale. In all cases, the PH period achieved the best results. Health scouting is an important practice in the avocado industry and understanding the spatial and temporal differences of PRR would be useful for disease management. The results of this study show the potential of fcover and VI’s obtained from UAV super high-resolution imagery and VI’s obtained high-resolution satellite imagery in the determination of PRR severity in avocado orchards. These results could be used as a rapid sensing technology to aid in the scouting process by reducing scouting costs and improve efficiency.
Data availability statement
The datasets presented in this article are not readily available because the dataset is private funded by a growers' association. Requests to access the datasets should be directed to Y3BlQHN1bi5hYy56YQ==.
Author contributions
SD: Investigation, Methodology, Visualization, Data curation, Formal analysis, Writing – original draft. AM: Supervision, Writing – review & editing. CP-E: Conceptualization, Funding acquisition, Investigation, Methodology, Project administration, Resources, Supervision, Visualization, Writing – review & editing.
Funding
The author(s) declare financial support was received for the research, authorship, and/or publication of this article. This study was funded by the South African Avocado Growers' Association (SAAGA) through the research project “Study of remote sensing techniques for mapping the severity of Phytophthora Root Rot (PRR) disease in Avocado orchards” (Project 06/22).
Acknowledgments
The authors are grateful to Precious Novela and Zanelle Mufamadi from ZZ2 and Westfalia Fruit for their assistance with the site selections and sampling in the field.
Conflict of interest
The authors declare that the research was conducted in the absence of any commercial or financial relationships that could be construed as a potential conflict of interest.
Publisher’s note
All claims expressed in this article are solely those of the authors and do not necessarily represent those of their affiliated organizations, or those of the publisher, the editors and the reviewers. Any product that may be evaluated in this article, or claim that may be made by its manufacturer, is not guaranteed or endorsed by the publisher.
Supplementary material
The Supplementary Material for this article can be found online at: https://www.frontiersin.org/articles/10.3389/fagro.2024.1419479/full#supplementary-material
References
Abdulridha J., Ampatzidis Y., Ehsani R., de Castro A. I. (2018). Evaluating the performance of spectral features and multivariate analysis tools to detect laurel wilt disease and nutritional deficiency in avocado. Comput. Electron. Agric. 155, 203–2011. doi: 10.1016/j.compag.2018.10.016
Bagheri N. (2020). Application of aerial remote sensing technology for detection of fire blight infected pear trees. Comput. Electron. Agric. 168, 105147. doi: 10.1016/j.compag.2019.105147
Candiago S., Remondino F., De Giglio M., Dubbini M., Gattelli M. (2015). Evaluating multispectral images and vegetation indices for precision farming applications from UAV imagery. Remote Sens. 7, 4026–4047. doi: 10.3390/rs70404026
Chaerle L., van der Straeten D. (2000). Imaging techniques and the early detection of plant stress. Trends Plant Sci. 5, 495–501. doi: 10.1016/S1360-1385(00)01781-7
Chang A., Yeom J., Jung J., Landivar J. (2020). Comparison of canopy shape and vegetation indices of citrus trees derived from UAV multispectral images for characterization of citrus greening disease. Remote Sens. 12, 4122. doi: 10.3390/rs12244122
Darvas J. M., Toerien J. C., Milne D. L. (1984). Control of avocado root rot by trunk injection with phosetyl-Al. Plant Dis. 68, 691–693. doi: 10.1094/PD-68-691
De Castro A. I., Ehsani R., Ploetz R., Crane J. H., Abdulridha J. (2015). Optimum spectral and geometric parameters for early detection of laurel wilt disease in avocado. Remote Sens. Environ. 171, 33–44. doi: 10.1016/j.rse.2015.09.011
Erwin D. C., Ribeiro O. K. (1996). Phytophthora Diseases Worldwide (St. Paul, MN, USA: APS Press), 269–279.
Eskalen A., Bender G., Faber B., Rios S. (2017). Phytophthora root rot of avocado and management strategies. From the Grove. 7 (2), 31–32.
García-Pineda E., Benezer-Benezer M., Gutiérrez-Segundo A., Rangel-Sánchez G., Arreola-Cortés A., Castro-Mercado E. (2010). Regulation of defence responses in avocado roots infected with Phytophthora cinnamomi (Rands). Plant Soil 331, 45–56. doi: 10.1007/s11104-009-0225-5
Garcia-Ruiz F., Sankaran S., Maja J. M., Lee W. S., Rasmussen J., Ehsani R. (2013). Comparison of two aerial imaging platforms for identification of Huanglongbing-infected citrus trees. Comput. Electron. Agric. 91, 106–115. doi: 10.1016/j.compag.2012.12.002
Garza B. N., Ancona V., Enciso J., Perotto-Baldivieso H. L., Kunta M., Simpson C. (2020). Quantifying citrus tree health using true color UAV images. Remote Sens. 12, 170. doi: 10.3390/rs12010170
Gitelson A. A., Gritz Y., Merzlyak M. N. (2003). Relationships between leaf chlorophyll content and spectral reflectance and algorithms for non-destructive chlorophyll assessment in higher plant leaves. J. Plant Physiol. 160, 271–282. doi: 10.1078/0176-1617-00887
Haagsma M., Page G. F. M., Johnson J. S., Still C., Waring K. M., Sniezko R. A., et al. (2020). Using hyperspectral imagery to detect an invasive fungal pathogen and symptom severity in pPinus strobiformis seedlings of different genotypes. Remote Sens. 12, 1–19. doi: 10.3390/rs12244041
Hornero A., Hernández-Clemente R., North P. R. J., Beck P. S. A., Boscia D., Navas-Cortes J. A., et al. (2020). Monitoring the incidence of Xylella fastidiosa infection in olive orchards using ground-based evaluations, airborne imaging spectroscopy and Sentinel-2 time series through 3-D radiative transfer modelling. Remote Sens. Environ. 236, 111480. doi: 10.1016/j.rse.2019.111480
Huete A., Justice C., van Leeuwen W. (1999). MODIS Vegetation Index (MOD 13). Algorithm Theoretical Basis Document. 3 (213), 295–309.
Johansen K., Duan Q., Tu Y. H., Searle C., Wu D., Phinn S., et al. (2020). Mapping the condition of macadamia tree crops using multi-spectral UAV and WorldView-3 imagery. ISPRS J. Photogramm. Remote Sens. 165, 28–40. doi: 10.1016/j.isprsjprs.2020.04.017
Kubera C. (2013). Enhancing Remote Sensing for Agriculture Using Small Unmanned Aerial Systems: San Diego, CA, as a Test Case. Geography and the Environment: Graduate Student Capstones. 42. doi: 10.56902/ETDCRP.2013.15
Kumar A., Lee W. S., Ehsani R., Albrigo L. G., Yang C., Mangan R. L. (2010). “Citrus greening disease detection using airborne multispectral and hyperspectral imaging,” in Proceedings of the 10th International Conference on Precision Agriculture. Denver, Colorado: American Society of Agricultural and Biological Engineers.
Li X., Lee W. S., Li M., Ehsani R., Mishra A. R., Yang C., et al. (2012). Spectral difference analysis and airborne imaging classification for citrus greening infected trees. Comput. Electron. Agric. 83, 32–46. doi: 10.1016/j.compag.2012.01.010
Mahlein A. K. (2016). Plant disease detection by imaging sensors – Parallels and specific demands for precision agriculture and plant phenotyping. Plant Dis. 100, 241–254. doi: 10.1094/PDIS-03-15-0340-FE
Mahlein A. K., Oerke E. C., Steiner U., Dehne H. W. (2012). Recent advances in sensing plant diseases for precision crop protection. Eur. J. Plant Pathol. 1, 197–209. doi: 10.1007/s10658-011-9878-z
Marais L. J., Menge J. A., Bender G. S., Faber B. (2002). Phyophthora root rot. AvoResearch 2, 15–18.
McLeod A., Masikane S. L., Novela P., Ma J., Mohale M., Nyoni M., et al (2018). Quantification of root phosphite concentrations for evaluating the potential of foliar phosphonate sprays for the management of avocado root rot. Crop Protect. 103, 87–97. doi: 10.1016/j.cropro.2019.105008
Nutter F. W., Esker P. D., Netto R. A. C. (2006). Disease assessment concepts and the advancements made in improving the accuracy and precision of plant disease data. Eur. J. Plant Pathol. 115, 95–103. doi: 10.1007/s10658-005-1230-z
Pegg K. G., Coates L. M., Korsten L., Harding R. M. (2002). “Foliar, fruit and soilborne diseases,” in The Avocado: botany, production and uses. Eds. Whiley A. W., Schaffer B., Wolstenholme B. N. (CAB International, Wallingform, Oxfordshire, United Kingdom), 299–338. doi: 10.1079/9780851993577.0299
Pérez-Bueno M. L., Pineda M., Vida C., Fernández-Ortuño D., Torés J. A., de Vicente A., et al. (2019). Detection of white root rot in avocado trees by remote sensing. Plant Dis. 103, 1119–1125. doi: 10.1094/PDIS-10-18-1778-RE
Pourazar H., Samadzadegan F., Dadrass Javan F. (2019). Aerial multispectral imagery for plant disease detection: radiometric calibration necessity assessment. Eur. J. Remote Sens. 52, 17–31. doi: 10.1080/22797254.2019.1642143
Rahman M. M., Robson A., Brinkhoff J. (2022). Potential of time-series sentinel 2 data for monitoring avocado crop phenology. Remote Sens. 14, 5942. doi: 10.3390/rs14235942
Ramírez-Gil J. G., Castañeda-Sánchez D. A., Morales-Osorio J. G. (2017). Production of avocado trees infected with Phytophthora cinnamomi under different management regimes. Plant Pathol. 66, 623–632. doi: 10.1111/ppa.12620
Ranson K. J., Daughtry C. S. T. (1987). Scene shadow effects on multispectral response. IEEE Trans. Geosci. Remote Sens. GE-25, 502–509. doi: 10.1109/TGRS.1987.289863
R Core Team (2021). R: A language and environment for statistical computing (Vienna, Austria: R Foundation for Statistical Computing). Available at: https://www.R-project.org/.
Roberts D. A., Roth K. L., Perroy R. L. (2011). Hyperspectral vegetation indices. In Hyperspectral Remote Sensing of Vegetation. Thenkabail P. S., Lyon J. G., Huete A. Eds. (Boca Raton, FL, USA: CRC Press, Taylor & Francis Group), 309–327.
Robson A., Rahman M. M., Muir J. (2017). Using worldview satellite imagery to map yield in avocado (Persea americana): A case study in Bundaberg, Australia. Remote Sens. 9, 1223. doi: 10.3390/rs9121223
Salgadoe A. S. A., Robson A. J., Lamb D. W., Dann E. K., Searle C. (2018). Quantifying the severity of Phytophthora rot disease in avocado trees using image analysis. Remote Sens. 10, 226. doi: 10.3390/rs10020226
Sankaran S., Khot L. R., Maja J. M., Ehsani R. (2013). “Comparison of two multiband cameras for use on small UAVs in agriculture,” in 2013 5th Workshop on Hyperspectral Image and Signal Processing: Evolution in Remote Sensing (WHISPERS). Piscataway, NJ: IEEE, 1–4. doi: 10.1109/WHISPERS.2013.8080668
Sankaran S., Mishra A., Ehsani R., Davis C. (2010). A review of advanced techniques for detecting plant diseases. Comput. Electron. Agric. 72, 1–13. doi: 10.1016/j.compag.2010.02.007
Selvaraj M. G., Vergara A., Montenegro F., Alonso Ruiz H., Safari N., Raymaekers D., et al. (2020). Detection of banana plants and their major diseases through aerial images and machine learning methods: A case study in DR Congo and Republic of Benin. ISPRS J. Photogramm. Remote Sens. 169, 110–124. doi: 10.1016/j.isprsjprs.2020.08.025
Tu Y. H., Johansen K., Phinn S., Robson A. (2019). Measuring canopy structure and condition using multi-spectral UAS imagery in a horticultural environment. Remote Sens. (Basel) 11, 3. doi: 10.3390/rs11030269
van den Berg A. K., Perkins T. D. (2004). Evaluation of a portable chlorophyll meter to estimate chlorophyll and nitrogen contents in sugar maple (Acer saccharum Marsh.) leaves. For. Ecol. Manage. 200, 113–117. doi: 10.1016/j.foreco.2004.06.005
West J. S., Bravo C. (2003). The potential of optical canopy measurement for targeted control of field crop diseases. Annu. Rev. Phytopathol. 41, 593–614. doi: 10.1146/annurev.phyto.41.121702.103726
Wu D., Johansen K., Phinn S., Robson A. (2020). Suitability of airborne and terrestrial laser Scanning for mapping tree crop structural metrics for improved orchard management. Remote Sens. 12, 1647. doi: 10.3390/rs12101647
Ye H., Huang W., Huang S., Cui B., Dong Y., Guo A., et al. (2020). Recognition of banana fusarium wilt based on UAV remote sensing. Remote Sens. 12, 938–956. doi: 10.3390/rs12060938
Keywords: remote sensing, avocado, disease severity detection, RGB imaging, plant projective cover, multispectral imaging, UAV, satellite
Citation: Duncan S, McLeod A and Poblete-Echeverria C (2024) Application of UAV and satellite technologies for assessing phytophthora root rot severity in avocado orchards. Front. Agron. 6:1419479. doi: 10.3389/fagro.2024.1419479
Received: 18 April 2024; Accepted: 15 November 2024;
Published: 12 December 2024.
Edited by:
Henning Buddenbaum, University of Trier, GermanyReviewed by:
Abel Ramoelo, University of Pretoria, South AfricaEvans Asenso, University of Ghana, Ghana
Copyright © 2024 Duncan, McLeod and Poblete-Echeverria. This is an open-access article distributed under the terms of the Creative Commons Attribution License (CC BY). The use, distribution or reproduction in other forums is permitted, provided the original author(s) and the copyright owner(s) are credited and that the original publication in this journal is cited, in accordance with accepted academic practice. No use, distribution or reproduction is permitted which does not comply with these terms.
*Correspondence: C. Poblete-Echeverria, Y3BlQHN1bi5hYy56YQ==