- Department of Electrical and Computer Engineering, McGill University, Montreal, QC, Canada
Optical neural networks implemented with Mach-Zehnder Interferometer (MZI) arrays are a promising solution to enable fast and energy-efficient machine learning inference, yet finding a practical application has proven challenging due to sensitivity to thermal noise and loss. To leverage the distinct advantages of integrated optical processors while avoiding its shortcomings given the current state of optical computing, we propose the binary optical trigger as a promising field of application. Implementable as small-scale application-specific circuitry on edge devices, the binary trigger runs binary classification tasks and output binary signals to decide if a subsequent energy intensive system should activate. Motivated by the limited task complexity, constrained area and power budgets of binary triggers, we perform 1) systematic, application-specific hardware pruning by physically removing specific MZIs, and 2) application-specific optimizations in the form of false negative reduction and weight quantization, as well as 3) sensitivity studies capturing the effect of imperfections in real optical components. The result is a customized MZI-mesh topology, MiniBokun Mesh, whose structure provides adequate performance and robustness for a targeted task complexity. We demonstrate in simulation that the pruning methodology achieves at least 50% less MZI usage compared to Clements and Reck meshes with the same input size, translating to at least between 4.6% and 24.2% savings in power consumption and a 40% reduction in physical circuitry footprint compared to other proposed unitary MZI topologies, sacrificing only 1%–2% drop in inference accuracy.
1 Introduction
Optical processors are known for their fast, efficient computation-by-propagation and high energy efficiency. Applying optical processing to machine learning is particularly promising: while optical processors are sensitive to noise, crosstalk, and optical signal attenuation, machine learning is error-tolerant by definition, and benefits substantially from the low-power matrix-vector multiplication (MVM) made possible by optical neural networks (ONNs) (McMahon, 2023).
Previous studies on ONN focused on implementing arbitrary weight matrices (Miller, 2013; Shen et al., 2017; Zhang et al., 2021; Banerjee et al., 2023) similar to the multi-layer perceptron (MLP) (Delashmit et al., 2005) implemented on a digital computer through singular value decomposition (SVD). This is achieved by inserting a diagonal matrix [
To make the most of ONN’s low power operation while avoiding the above-mentioned caveats, using the ONN in some efficiency-demanding edge computing tasks is a promising application. Ideally, the task should have low complexity so that a small-scale ONN can be employed. For this reason, we propose using the ONN as an activation trigger for any subsequent energy-intensive system in an edge environment. Similar to the multi-stage architecture for facial recognition proposed in (Bong et al., 2018) but implemented using optical components, the optical processor will act as an ultra-lightweight neural network that responds to a particular input event (i.e., specific objects appearing in the input image), while being more sophisticated than a conventional motion- or proximity-based detector (Gazivoda and Bilas, 2022) to avoid unnecessary activation caused by any input fluctuation, such as newly present objects of noninterest in the monitoring area. For example, consider a smart door lock facing a busy pedestrian street: the system ignores passersby, and only activates an energy-intensive system (e.g., face recognition for authentication) when someone directly faces the sensor. By triggering the subsequent complex system only when it is needed, energy consumption can be dramatically reduced.
With the target application in mind, further efforts can be explored to construct a tailored ONN for the task. First, the edge execution environment would benefit tremendously from reduced active component usage and reduced control circuitry bit precision that is constantly drawing power. Second, trade-offs can be made between the rate of false activation and the rate of trigger miss, depending on the specific application. To reduce the number of active components, we explored a pruning approach inspired by machine learning, where low-saliency components with minimal impact on overall system performance are removed from an initially over-parametrized optical neural network while maintaining prediction accuracy. Regarding application-specific trade-offs, we examined methods for reducing false negatives and quantization for the proposed binary optical trigger.
In this paper, given the ONN’s fast and efficient computation ability yet with low scalability, we propose the binary optical trigger, a lightweight optical neural network designed for binary classification. The binary optical trigger has a structure similar to a traditional fully connected neural network but is composed of a mesh of MZIs, where the weight matrix is controlled by phase values programmed into the phase shifters. Our proposed ONN application and its associated optimizations diverge from previously reported efforts aimed at moderate classification tasks beyond MNIST, which often results in impractically large photonic circuits or extensive component reuse. Instead, our work focuses on binary classification tasks to trigger subsequent energy-intensive systems. Given the promising energy efficiency of ONNs, despite their early stage of development, this niche and innovative application effectively leverages their advantages in a practical, targeted manner. We then systematically explore the pruning of well-established unitary MZI topologies to optimize it as a trigger, leading to a new, application-specific topology named MiniBokun. Through simulation, we demonstrate that MiniBokun, when used as the binary optical trigger, prunes away at least 50% of the MZIs from a standard unitary at the cost of 1%–2% accuracy impairment—leading to a conservatively estimated power saving of 24% and an area reduction of 40%. The paper is structured as follows: we first cover the ONN background in Section 2. Our experimental setup, application-specific optimization, pruning approach, phase noise considerations, and estimations regarding a physical system (power, latency and area) are described in Section 3. The results, obtained through the methodology described in Section 3, are presented and analyzed in Section 4. Followed by the conclusion in Section 5.
2 Background
2.1 MZI basics
Our optical processor adheres to an MZI-based architecture, taking advantage of its capability of realizing signed, complex-valued weights (Mourgias-Alexandris et al., 2022). The MZI-based neural network accelerator consists of a mesh of
with
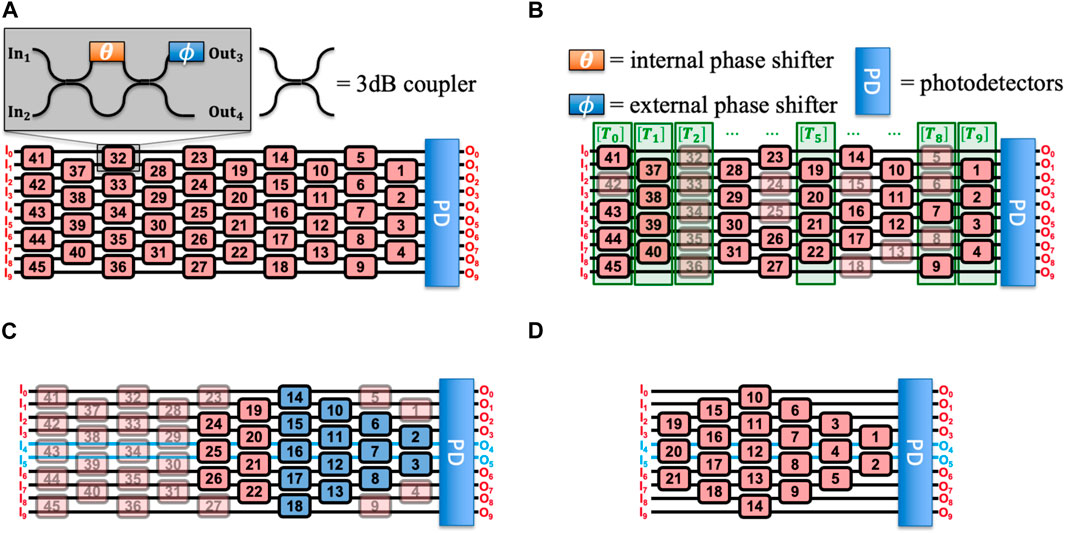
Figure 1. Structure of an MZI and MZI-based ONN. (A) A
2.2 MZI-based optical processor topology and mathematical model
Larger transformation matrices can be realized by organizing these
Considering the specific topology, the transformation matrix,
where,
In Equation 2,
At most
Given an arbitrary mesh formed by the removal of a subset of MZIs from a full
The interpretation in Equation 4 gives insight into the transformation performed by each MZI column while providing a clearer picture of how each MZI is ordered in Equation 2.
2.3 Signal basics
2.3.1 Phasor term
A phasor is a scalar, complex value sufficient to describe the steady state of a mono-frequency sinusoidal waveform. In the context of an optical signal, this represents the electric field component of the monochromatic laser. A phasor term takes the form of:
In Equation 5,
2.3.2 Value representation and importance of coherency
Due to difficulties of controlling the absolute phase of optical signal (Ip et al., 2008), the incoming data (i.e., feature vectors of each data sample) will be solely represented by the intensity
In Equation 7, only the
For the correct functioning of any trained network, not only the zero phase difference across input channels is required, but the absolute phase of each input signal should also remain constant throughout the network’s training and operation. As the MZI mesh works by the principle of interference, an incoherent or varying initial phase difference between signals will affect the intended splitting ratio learned from network training, making the resulting output signal array drastically different from the expectation.
2.4 Imperfect operation
The actual implementation of optical processors faces various aspects of imperfections, and the presence of imperfections significantly degrades the computation accuracy of ONNs (Shafiee et al., 2024; Gu et al., 2020b). In this work, our investigation of the impacts of imperfections focuses on two main sources, optical loss and phase value programming deviations.
2.4.1 Optical loss
During ONN inference, when light couples through the waveguide, the processor suffers from inherent propagation loss. The propagation loss eventually leads to a challenging optical power budget, limiting the signal-to-noise ratio at the photodetectors and reducing the classification accuracy of ONN. The linear loss values
2.4.2 Phase shifter programming deviation
The programmed phase shift can deviate from its intended value due to thermal crosstalk (Shafiee et al., 2024). When programming a targeted waveguide, the heat from resistive heaters can propagate to other waveguides, creating unintended phase changes. To capture these imperfections, we model the programmed phases with a Gaussian distribution
2.5 Neural network pruning
In practice, pruning often implies the removal of neurons and weighted connections in a structured or unstructured fashion (Nagel et al., 2021). For neural networks (NNs) implemented by digital processors, network pruning has been known for its benefits of simplifying NN’s architecture, reducing computation workload and memory footprint, and subsequently improving inference speed and efficiency. ONNs, on the other hand, though composed of physically integrated photonics components (e.g., MZIs), benefit from an analogous set of advantages (Banerjee et al., 2023). First, pruning in the hardware context means the direct removal of photonic integrated circuit (PIC) components. The feasibility of the layout is not only subject to the number of on-chip components but also complicated by the requirement of a voltage supply line to each active component (e.g., for the thermo-optic phase shifter). As the number of components grows, this poses a significant challenge for the layout routability and manufacturability in a two-dimensional circuit board. The removal of PIC components immediately reduces layout complexity and manufacturing costs. Second, each component introduces loss to the propagating optical signal to various extents. Reducing the number of components on one optical path reduces the total amount of accumulated loss experienced by that signal, improving signal-to-noise ratio (SNR) at detection. Third, reducing the number of active components naturally leads to less power consumption during operation.
The pruning of MZI-based ONNs was explored in previous works. Banerjee et al. (2023) introduced a pruning algorithm and its variants targeting large-scale SVD-based ONNs for multi-class classification. The algorithm is demonstrated via simulation on networks comprising at least four unitary meshes connected by a non-linear activation function, with 64 as the minimum network width. In particular, their pruning is realized via power-gating or removal of phase shifters, not the entire MZI. This implies that imprecise beam splitters are still present in the actual physical system. Training-time structured pruning was also conducted in the tile-based ONN, such as the block-circulant unit in Gu et al. (2020a). However, to the best of our knowledge, no direct MZI-level pruning on well-established unitary meshes was explored. Specifically, we focused on removing entire MZIs from a unitary structure rather than power-gating active components in SVD-based setups or setups involving component reuse. Our pruning strategy enables a reduction in optical depth and insertion loss compared to these previous configurations. Though unitary meshes already have limited expressivity compared to an arbitrary linear weight matrix, our study showed that the application as a binary optical trigger allowed for an ultra-lightweight ONN that is pruned into deep sub-unitary space without significantly affecting classification accuracy.
3 Materials and methods
3.1 Neuroptica
We use Neuroptica (Bartlett et al., 2019) to evaluate the simulated performance of our ONN architectures. Neuroptica is a Python simulation platform for coherent optical neural networks built with integrated components, such as MZI. The platform allows one to explore ONN architecture design, ex-situ ONN training (Mojaver et al., 2023), and noise/loss robustness simulation of trained ONNs. In addition to the components simulated within the ONN mesh area, we assume the presence of a laser source and variable optical attenuators at the ONN’s input side to produce feature values for each data sample, though these components are not explicitly simulated.
3.1.1 Hyperparameter selection and training
We first evaluate networks with input sizes
The limited size of the ONN we are evaluating and the resolution of the images in the selected datasets mean that data must first be compressed in some way prior to inference. Therefore, we use Principal Components Analysis (PCA) to perform dimensionality reduction. Mathematically, PCA maps
We make two assumptions about the input range of ONN based on laser power consumption. The first assumption assumes a fixed per-channel laser input range (
With these training setups, training time ranged from two to three minutes for
3.2 Datasets
3.2.1 MNIST
The MNIST dataset (Deng, 2012) consists of
3.2.2 CIFAR-10
The CIFAR-10 dataset (Krizhevsky and Hinton, 2009) contains
3.2.3 Task complexity and pruning efficacy
In both datasets, the original images have sufficient pixels to clearly depict the represented objects. This ensures that the complexity of any formulated task comes from the intrinsic difficulty of distinguishing objects across different classes rather than from low image resolution. Depending on the specific task complexity and the degree of over-parametrization in the network model, varying levels of pruning can be carried out. As a result, our aggregated classification tasks of both datasets provide meaningful task complexity and serve as effective benchmarks for evaluating the ONN capability and the efficacy of the pruning process.
3.3 Application-specific optimization
Apart from a grid search of hyperparameters, we consider the following application-specific optimization methods to further enhance the performance of the models. These optimization methods focus on actual implementation challenges and adapt the trained models to real-world conditions.
3.3.1 False negative reduction
The binary optical trigger structure is anticipated to be used in event-triggered structures, where the ONN activates the rest of a system when a pre-defined event takes place (e.g., a vehicle is detected by ONN after training on the CIFAR-10 dataset). A key challenge in the implementation of such a system is the optical trigger false negatives: the pre-defined event happens, but the ONN does not send a trigger signal, and the rest of the system fails by default. Therefore, one goal of our work is to minimize the number of False Negatives (FN) while maintaining the classification accuracy of ONN.
The FN reduction method considered in this work changes the weight assigned to each label class in the loss function. We penalize FN more severely, and the binary cross-entropy loss becomes
where
where
During implementations in this work, in order to strike a trade-off between FN reduction and the classification performance, we train ONN models with different weights
3.3.2 Post-training quantization (PTQ)
Programming the MZI-based building block of ONNs involves configuring their phase shifters to form a desired transfer matrix. In this work, we consider MZIs with phase shifters controlled by thermally changing the phase using resistive heaters tuned by a voltage supply (Masood et al., 2013). The relationship between the heater control voltage
where
Practical voltage sources have limited resolution, meaning they can be adjusted only to a finite number of discrete voltage levels equally spaced between the maximum and minimum values. A
Models with selected hyperparameters from the previous steps are quantized by rounding the trained phase shifts to their nearest quantized phase values. We choose the least voltage resolution (in
3.4 Hardware pruning
In this section, we will use the
Upon deciding on port selection and pruning of redundant MZIs, we then perform layer-wise pruning. At each pruned step, we monitor the network performance by performing the same training and testing process and record the testing accuracy. The layer-wise pruning stops until we obtain a minimal network topology that still ensures all input signals are able to reach the center two waveguides. This topology consists of MZIs colored in blue in Figure 1C, which is a triangle mesh that marginally allows the diversion of optical power from top/bottom waveguides to the center waveguides, any further removal of MZIs on this topology will either result in wasted waveguide channels, or isolation between two-halves of the input vector causing unwanted dependence in the network’s decision making.
3.4.1 Expressivity study (fidelity analysis)
To gain insight into the trade-off between reducing component usage and disruption in network expressivity, we look for a suitable metric to evaluate the pruned mesh’s expressivity. In previous works, the concept of fidelity was employed (Feng et al., 2022; Zhang et al., 2021) for evaluating the similarity between two complex density matrices. Similar metrics include the Frobenius norm, cosine similarity, and correlation coefficients. However, we note the unsuitability of a simple similarity metric in our particular case, as the goal of pruning is not to produce an optical mesh capable of approximating the original unitary matrix. Rather, given the relative simplicity of the binary trigger task and monitoring of only two entries in the output vector, fully unitary ONNs are likely over-parameterized, and completely different sets of optimal weight may exist in the sub-unitary space that have little to no relation to the unitary weight matrices producing similar classification accuracy.
For this reason, we employ a sampling-based benchmark to evaluate the signal routing ability of
3.5 Imperfection study (sensitivity analysis)
All the previous training and tuning of ONNs are conducted with the assumption of perfect operating conditions. However, in reality, ONNs suffer from various aspects of imperfections. To test the resilience of ONNs to imperfections, we inject and vary the magnitude of the optical loss and phase programming deviations to trained ONN models and check their response. The optical loss, defined at the dB-scale
To quantify the tolerance of ONNs to imperfections, we define two Figures of Merits (FoMs) on two sets of imperfect scenarios. The first imperfect scenario assumes only phase programming deviations (Phi-Theta case),
The second imperfect scenario (Loss-Phase Uncertainty case) considers both optical loss and phase deviations. We assume
3.6 Power, latency, and area estimations
The power consumption estimation of ONN takes into account power consumed by the laser, the memory for storing phase shifter values and input, the digital-to-analog and analog-to-digital conversions, optical input modulation, phase programming, and output optical-electrical signal conversion, as expressed in Equation 12.
Similarly, the latency of one inference on ONN considers the time spent when the electrical and optical signal propagates through the system during one pass of calculation (or one inference). Assuming an always-on laser, the latency is expressed as:
In Equation 13,
The area estimations focus on the layout area of the optical meshes, containing only the MZIs and their connecting waveguides. The length of the mesh is determined by the maximum number of MZIs and waveguide sections connected in series, and the width is the separation distance between waveguides times the number of gaps between input/output ports.
We account for a 10 dBm C-band laser with a wall plug efficiency of 10% (Al-Qadasi et al., 2022). This single laser source provides sufficient optical power to all input ports of ONNs while meeting the minimum required optical power sensitivity of the photodetector. The modulation of laser input (or the electrical-to-optical, EO conversion) is assumed to operate at approximately 20 fJ/bit with a rate of 2.5 Gb/s (Demirkiran et al., 2023).
The phase programming power estimation is divided into two scenarios: a conservative estimation using doped-Si heaters without insulation and an aggressive estimation considering heaters with thermal insulation trenches (formed by deep etching) (Masood et al., 2013). Without the insulation, the heaters consume
The input to ONN is fixed at 8-bit resolution while the phase value resolution will be determined by the post-training quantization. The estimations of digital-to-analog converters (DACs) power consumption patterns are also done in two ways: 1) a conservative FoM-based performance approximation which allows us to consider high-speed DACs (data rate = 10 GSamples/s) (Demirkiran et al., 2023), and 2) an aggressive performance estimation using established commercial products with low power consumption.
The optical-to-electrical (OE) circuit at each output port contains a photodetector with a responsivity of 1 A/W and a trans-impedance amplifier. Each channel of EO conversion consumes 100 mW of power with a group delay of 100 ps (Williamson et al., 2020). The subsequent comparator and analog-to-digital converter (ADC) circuit requires only binary resolution and consumes only 325
According to Al-Qadasi et al. (2022), the input to the ONN is stored in DRAMs while the phase values to be programmed are stored in SRAMs. The ONNs considered in this work are small, with each of them containing less than 256 bytes in total for both input and phase values. Despite this, we set the SRAM size to 16 KB and the DRAM size to 64 KB to sufficiently hold more than 100 copies of ONNs and a few thousand input samples after dimensionality reduction. The power and latency numbers are calculated based on modeling data from Cacti 7.0 (Thoziyoor et al., 2008).
4 Results and discussion
4.1 Architectural analysis of optical meshes
The architectural parameters of the three topologies are summarized in Table 1. Among the three topologies, the MiniBokun shown in Figure 1D, resulting from the pruning process, achieved minimum component usage while demonstrating a size-invariant path length difference of only two MZIs.
4.1.1 Pruning with accuracy monitoring
Following the method discussed in Section 3.4, the monitored average binary MNIST accuracy per
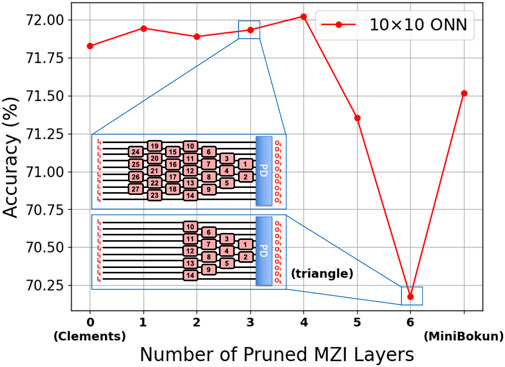
Figure 2. Accuracy Variation as the Clements topology is Pruned column by column, starting with the column closest to the input.
4.1.2 The MiniBokun topology
Similar to the full-size Bokun Mesh proposed in (Mojaver et al., 2023), MiniBokun provides diagonal access for all MZIs in the mesh for practical calibration consideration, yet with no MZI wasted due to being used solely for calibration purposes. Two simple observations can be made for a sufficient formal definition of MiniBokun topology, regardless of network size
• There are always two MZI columns before the widest column, each containing
• The last MZI column always contains two MZIs.
The placement of each MZI is thus well-defined, and the number of MZIs in a
4.1.3 Expressivity analysis
Using the benchmark method presented in Section 3.4.1. We perform statistical analysis on the collected power distribution at each output port for
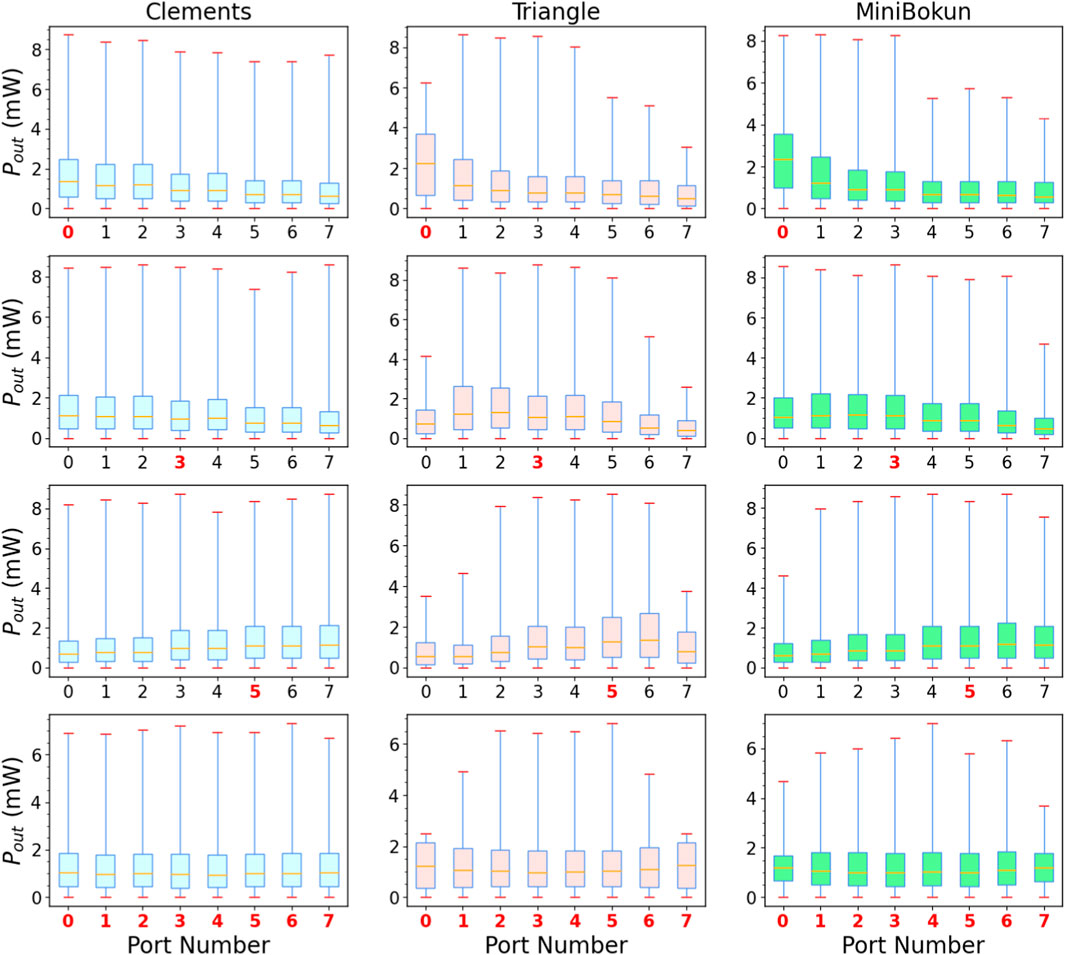
Figure 3. Expressivity of three different meshes. Blue, red and green box plots denote the registered power distribution at each of the eight output ports for Clements, Triangle and MiniBokun mesh. The port number in red indicates the corresponding input port had the highest optical power among all input ports, while the last row of figures denotes the case of equally distributed power among the input (at 1.25 mW per port). The whiskers on each box instance, from top to bottom, are: {max, third quartile, median, first quartile, min}.
As we are not assuming any loss, the average total output power, as expected, sums to 10 mW, which matches the total input power assumption. All tested topology-input combinations managed to achieve near 0 mW output in all output ports. The maximum registered maximum power difference among tested samples were 1.330, 6.205 and 4.080 mW for Clements (port 0), triangle (port 3) and MiniBokun (port 5), respectively. Unitary structures such as the Clements mesh provide full signal routing between any input-output waveguide pairs, thus giving a relatively uniform power distribution profile across each port, even when facing input with power concentrated on particular ports. On the other hand, sub-unitary topologies provide limited signal routing paths, in triangle topology, given the imbalanced number of MZI across different paths and the unbiased random phase setting, the biased weight space manifests as mismatching of maximum detected output power across different output, as well as the varying interquartile ranges. In particular, the low maximum power on edge ports (0, 7) indicates an impaired ability to discard unwanted power as part of the inference process. MiniBokun topology also shows such bias in its power distribution, but to a lighter extent, attributing to the two additional columns providing extra paths to disregard power from inputs 1 through 6, leading to a larger expressible space. This can be validated by the classification accuracy difference between a triangle mesh and a MiniBokun mesh in Figure 2.
4.2 Performance of optical meshes in ONN
4.2.1 Hyperparameter selection
We observe that topology sizes of
Under the assumption of constant per-channel input power, all three metrics, the accuracy of each model on both validation and test set and the F1 score, increase as
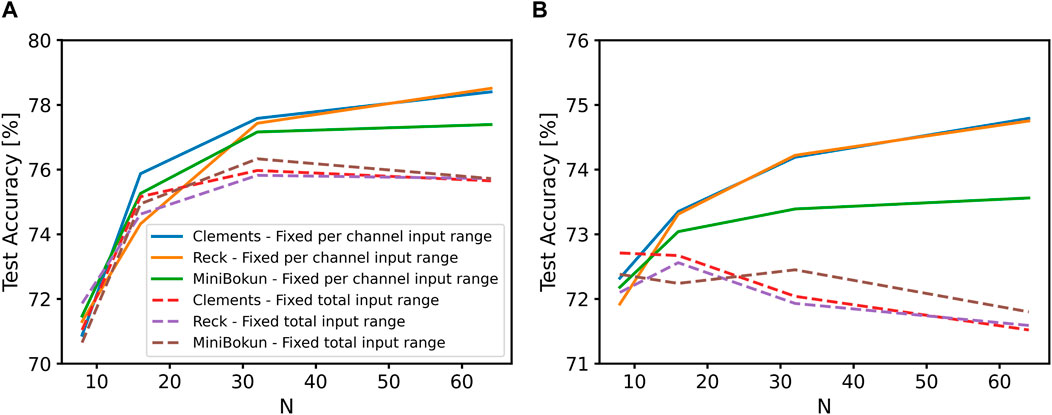
Figure 4. The variation in test accuracy of ONN models with different topology and input power assumptions trained on (A) MNIST dataset, and (B) CIFAR-10 dataset. The same set of legends is used in both figures.
When the assumption changes to fixed laser power, the actual per-channel input power range decreases as the optical mesh scales up. As
The increase in ONN classification accuracy with
4.2.2 Application-specific optimization
The weighted class method effectively reduces the number of FNs made by ONNs after training. As shown in Figures 5A, C, the FN count decreases from more than 1,000 to fewer than 10 as
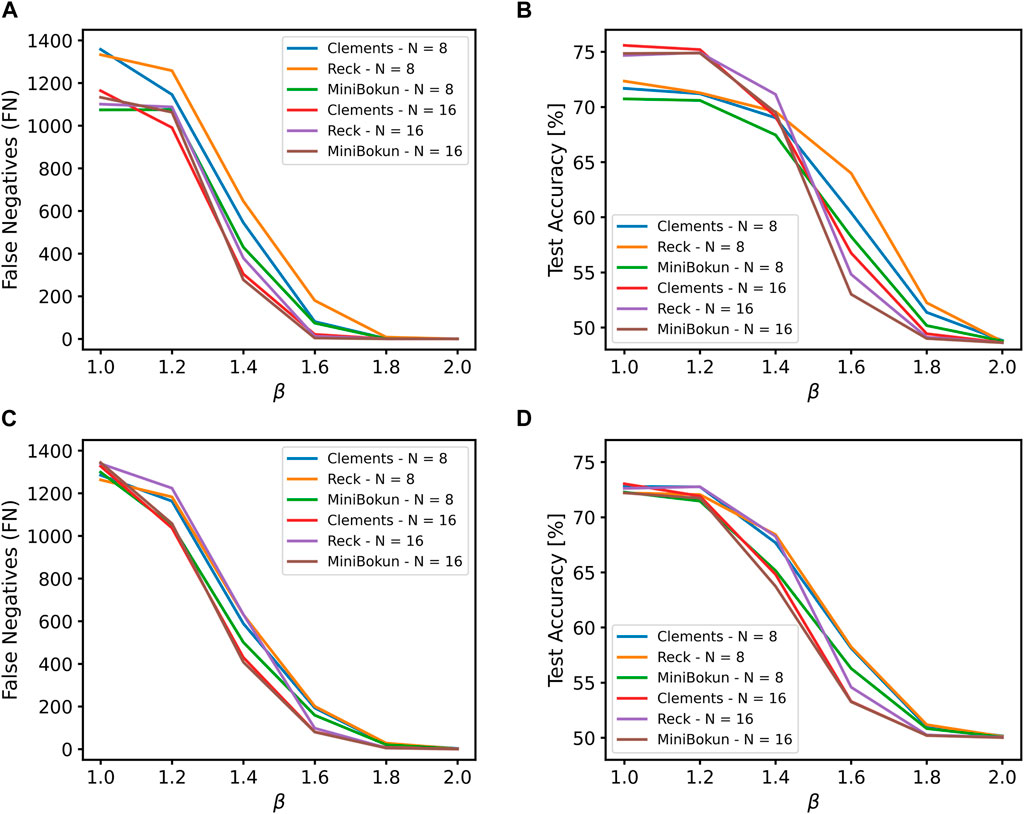
Figure 5. (A) The variation in false negative numbers and (B) the test accuracy of ONN models with different topology and input sizes on the MNIST dataset as a result of the weighted class method. (C) The variation in false negative numbers and (D) the test accuracy of ONN models with different topology and input sizes on the CIFAR-10 dataset as a result of the weighted class method.
We also find that an 8-bit voltage supply resolution is sufficient for models with the selected hyperparameters to achieve similar accuracy to those trained with full precision (32-bit), using a voltage supply setting of
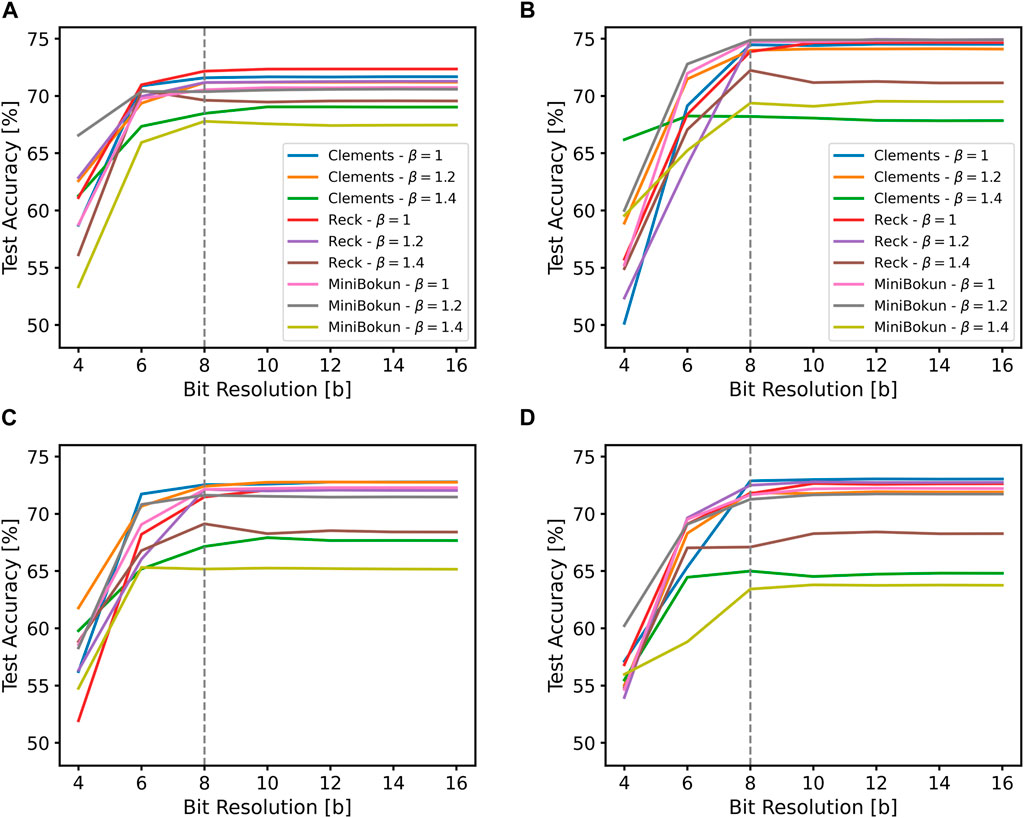
Figure 6. The change in test accuracy of ONN models as a result of post-training quantization (A) on the MNIST dataset with N = 8, (B) on the MNIST dataset with N = 16, and (C) on the CIFAR-10 dataset with N = 8, (D) on the CIFAR-10 dataset with N = 16. The same legend is kept across all four figures.
4.2.3 Impact of pruning on classification performance
Based on the selected hyperparameters and optimization parameters, we summarized the accuracy and F1 score of all the models with different topologies in Table 2. The numbers labeled in bold are the best-performing topology in each category.
In the
The performance gap between MiniBokun and the other meshes further closes when
4.3 Sensitivity analysis
Figure 7 shows the tolerance of investigated topologies towards phase shifter noise and propagation loss in optical components. The models subjected to the analysis are trained with an FN reduction factor
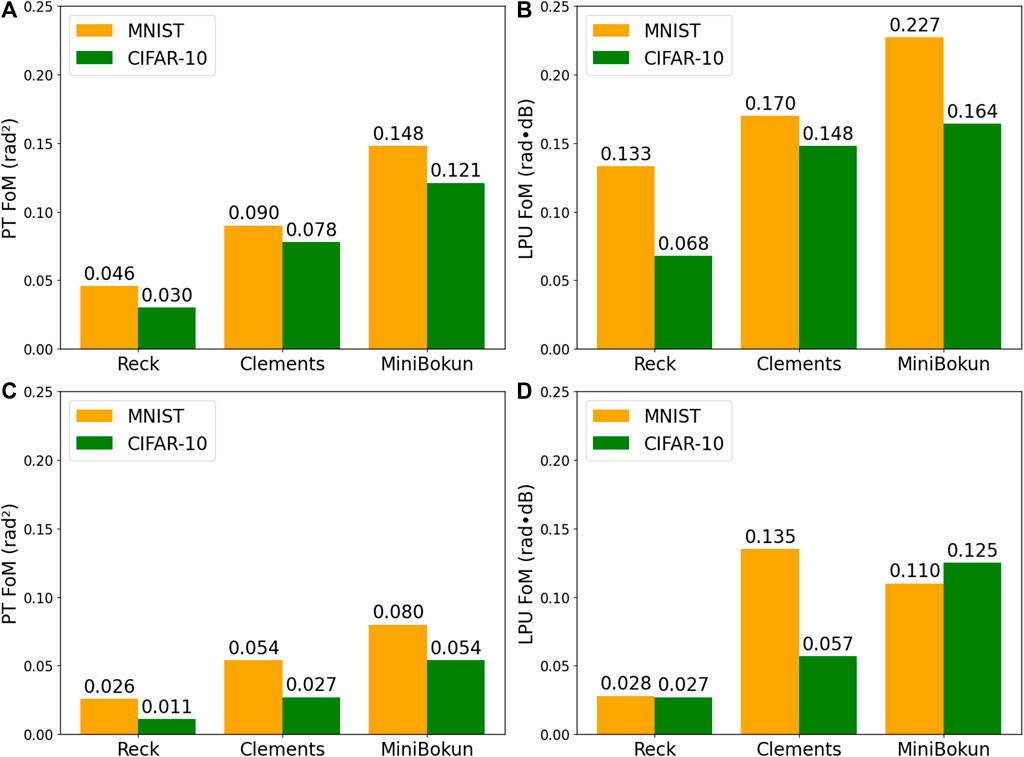
Figure 7. The FoMs of three network topologies: (A)
In contrast to
In almost all cases except for the LPU analysis for
These improvements in the FoMs suggest that the increase in individual weight importance that comes naturally with a pruned neural network is negligible for topologies used in this study. The original network is over-parameterized enough for the pruning benefits to outweigh the errors imposed on the high-saliency phase shifter values.
4.4 Power, latency, and area estimations
Table 3 summarizes the power, latency, and area consumed by each topology with different sizes. Note that the estimation “conservative” and “aggressive” are subject to the overall power consumption. Both the ONN input and the phase value programming require 8-bit DACs (Texas Instruments, 2013) after PTQ.
4.4.1 Power and latency consumption
The pruned MiniBokun mesh has demonstrated a strong capability in reducing overall power consumption. Compared to the conventional Clements and Reck topology, the MiniBokun mesh saves 4.6% power in the aggressive case and 24.2% in the conservative case when
If we take a closer look at the component-wise power and latency consumption, the phase value programming dominates both calculations. Assuming uniform phase distribution, the programming power is directly proportional to the number of MZIs in a mesh (Al-Qadasi et al., 2022). Without insulation (the “conservative” approach), the programming power can take up to 39.8% of the total power consumption in Reck and Clements topology when
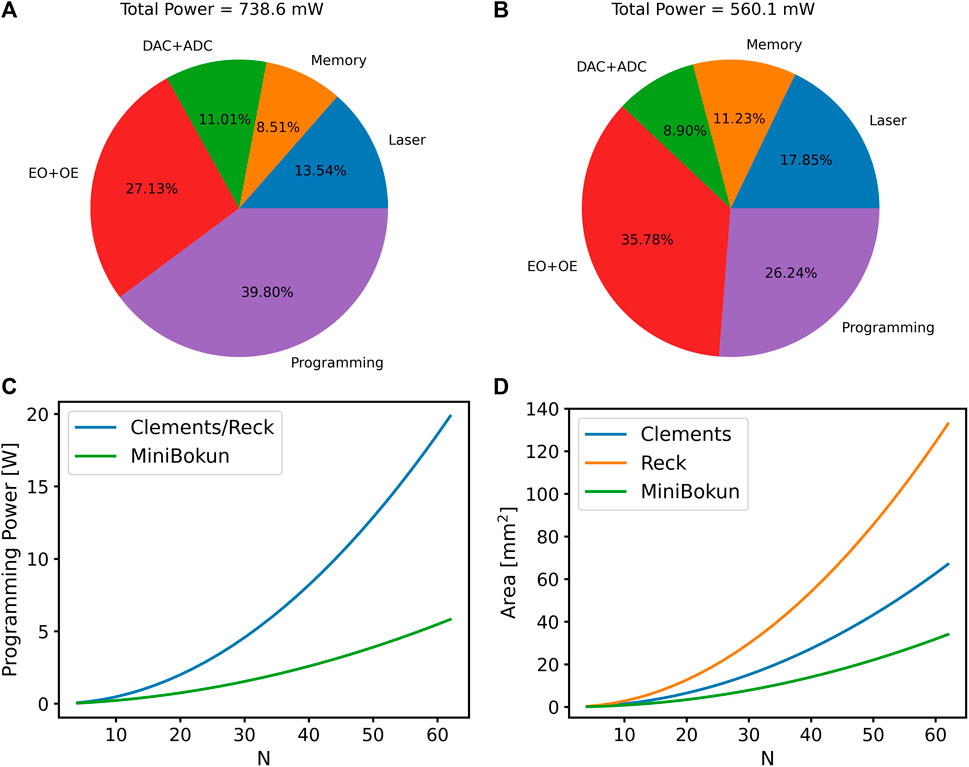
Figure 8. The power breakdown of (A) Clements/Reck topology, (B) the MiniBokun topology with
In the latency calculation, the pruned MiniBokun mesh effectively shortens the optical path length that light propagates through and lowers the number of memory read by reducing the number of phase values to be programmed. However, these savings (
4.4.2 Layout area
The pruning strategy significantly reduces the area of the optical mesh by placing fewer MZIs horizontally along the optical path. As shown in Table 3, the MiniBokun mesh employs 50% fewer MZIs when
For practical deployment, a typical smart lock uses a microcontroller comparable in size to an Arduino chip (Arduino, 2014), implying area constraints on the order of several square centimeters (Motwani et al., 2021). In contrast, modern smartphone processors, facing stricter area limitations, typically have a footprint of over 100 mm2 (Yang et al., 2024). By comparison, our estimated mesh area is 2.4 mm2 for the
4.5 Limitations and future work
Imperfect operating conditions are obstacles to the deployment of proposed systems in real-world applications. In this work, we characterize the resilience of different topologies to two sources of error: optical loss and phase deviations. To achieve a more comprehensive evaluation of the model performance in the future, the model needs to take into account more factors, including the direct impact of fabrication non-uniformity (Mirza et al., 2022), input phase mismatch (Fang et al., 2019), and other sources of crosstalk (Shafiee et al., 2024).
The proposed pruning strategy is tested with well-established image classification datasets, and its performance is compared with existing optical mesh topologies. Although these datasets are sufficiently complex to provide insights into how the ONN trades off accuracy against power/area consumption, the comparisons lack real-world proximity. Future work will explore the use of datasets closer to the actual implementation of the binary optical trigger, for example, face recognition systems (Bong et al., 2018). These experiments can better reveal the benefits of the pruning strategy and the optical trigger structure itself when compared with existing digital electronic products.
Alternative pruning strategies, such as train-time or post-training pruning, consider parameter saliency before deciding which components to remove. However, further investigation is needed to assess these alternatives. Although these methods could offer comparable power savings while reducing the accuracy loss, they have the potential to create sparse network meshes with less clustered MZI removal and thus may provide limited area savings compared to the current pre-training pruning approach.
5 Conclusion
In this work, we propose a pre-training pruning strategy over established optical processor topology subject to the binary optical trigger structure. Motivated by the need for a low-power binary trigger to support machine learning at the edge of the Internet, the pruned structure, “MiniBokun” mesh, removed at least 50% of MZIs from a standard unitary topology and shortened the optical path length by half. The effect of pruning was tested with the binarized version of two benchmark datasets, MNIST and CIFAR-10, in which we only observed 1%–2% accuracy degradation and less than 1% drop in F1 score compared to the unpruned Clements and Reck topologies. In consideration of the practical deployment environment, the impact of limited voltage control precision and the robustness of ONNs toward component imperfections were investigated via weight quantization and a sensitivity study. The MiniBokun mesh showed
Data availability statement
The datasets presented in this study can be found in online repositories. The names of the repository/repositories and accession number(s) can be found below: https://github.com/Xoreus/neuroptica/tree/6c56736010dcfc271724b10a34c849fed349a598.
Author contributions
BZ: Conceptualization, Data curation, Methodology, Software, Visualization, Writing–original draft, Writing–review and editing. XD: Data curation, Methodology, Software, Visualization, Writing–original draft, Writing–review and editing, Conceptualization. KR: Supervision, Writing–review and editing. BM: Supervision, Writing–review and editing. OL-L: Supervision, Writing–review and editing.
Funding
The author(s) declare that financial support was received for the research, authorship, and/or publication of this article. This research is supported by Natural Science and Engineering Research Council of Canada (NSERC) through grants RGPIN-2018-05668 and RGPIN-2021-03480.
Conflict of interest
The authors declare that the research was conducted in the absence of any commercial or financial relationships that could be construed as a potential conflict of interest.
Publisher’s note
All claims expressed in this article are solely those of the authors and do not necessarily represent those of their affiliated organizations, or those of the publisher, the editors and the reviewers. Any product that may be evaluated in this article, or claim that may be made by its manufacturer, is not guaranteed or endorsed by the publisher.
References
Al-Qadasi, M., Chrostowski, L., Shastri, B., and Shekhar, S. (2022). Scaling up silicon photonic-based accelerators: challenges and opportunities. Apl. Photonics 7, 020902. doi:10.1063/5.0070992
Banerjee, S., Nikdast, M., Pasricha, S., and Chakrabarty, K. (2023). Pruning coherent integrated photonic neural networks. IEEE J. Sel. Top. Quantum Electron. 29, 1–13. doi:10.1109/JSTQE.2023.3242992
Bartlett, B., Minkov, M., Hughes, T., and Williamson, I. A. D. (2019). Neuroptica: flexible simulation package for optical neural networks. Available at: https://github.com/fancompute/neuroptica.
Bong, K., Choi, S., Kim, C., Han, D., and Yoo, H.-J. (2018). A low-power convolutional neural network face recognition processor and a cis integrated with always-on face detector. IEEE J. Solid-State Circuits 53, 115–123. doi:10.1109/JSSC.2017.2767705
Clements, W. R., Humphreys, P. C., Metcalf, B. J., Kolthammer, W. S., and Walsmley, I. A. (2016). Optimal design for universal multiport interferometers. Optica 3, 1460–1465. doi:10.1364/OPTICA.3.001460
Delashmit, W., Missiles, L., and Manry, M. (2005). “Recent developments in multilayer perceptron neural networks,” in Proceedings of the seventh annual memphis area engineering and science conference, MAESC, 7, 33.
Demirkiran, C., Eris, F., Wang, G., Elmhurst, J., Moore, N., Harris, N. C., et al. (2023). An electro-photonic system for accelerating deep neural networks. J. Emerg. Technol. Comput. Syst. 19, 1–31. doi:10.1145/3606949
Deng, L. (2012). The mnist database of handwritten digit images for machine learning research [best of the web]. IEEE signal Process. Mag. 29, 141–142. doi:10.1109/msp.2012.2211477
Fang, M. Y.-S., Manipatruni, S., Wierzynski, C., Khosrowshahi, A., and DeWeese, M. R. (2019). Design of optical neural networks with component imprecisions. Opt. express 27, 14009–14029. doi:10.1364/oe.27.014009
Feng, C., Gu, J., Zhu, H., Ying, Z., Zhao, Z., Pan, D. Z., et al. (2022). A compact butterfly-style silicon photonic–electronic neural chip for hardware-efficient deep learning. ACS Photonics 9, 3906–3916. doi:10.1021/acsphotonics.2c01188
Gazivoda, M., and Bilas, V. (2022). Always-on sparse event wake-up detectors: a review. IEEE Sensors J. 22, 8313–8326. doi:10.1109/JSEN.2022.3162319
Gu, J., Zhao, Z., Feng, C., Liu, M., Chen, R. T., and Pan, D. Z. (2020a). “Towards area-efficient optical neural networks: an fft-based architecture,” in 2020 25th asia and south pacific design automation conference (ASP-DAC), 476–481. doi:10.1109/ASP-DAC47756.2020.9045156
Gu, J., Zhao, Z., Feng, C., Zhu, H., Chen, R. T., and Pan, D. Z. (2020b). “Roq: a noise-aware quantization scheme towards robust optical neural networks with low-bit controls,” in Date (IEEE), 1586–1589.
Ip, E., Lau, A. P. T., Barros, D. J. F., and Kahn, J. M. (2008). Coherent detection in optical fiber systems. Opt. Express 16, 753–791. doi:10.1364/OE.16.000753
Masood, A., Pantouvaki, M., Lepage, G., Verheyen, P., Van Campenhout, J., Absil, P., et al. (2013). “Comparison of heater architectures for thermal control of silicon photonic circuits,” in 10th international conference on group IV photonics, 83–84.
McMahon, P. L. (2023). The physics of optical computing. Nat. Rev. Phys. 5, 717–734. doi:10.1038/s42254-023-00645-5
Miller, D. A. B. (2013). Self-configuring universal linear optical component [invited]. Phot. Res. 1, 1–15. doi:10.1364/PRJ.1.000001
Mirza, A., Shafiee, A., Banerjee, S., Chakrabarty, K., Pasricha, S., and Nikdast, M. (2022). Characterization and optimization of coherent mzi-based nanophotonic neural networks under fabrication non-uniformity. IEEE Trans. Nanotechnol. 21, 763–771. doi:10.1109/TNANO.2022.3223915
Mojaver, K. H. R., Zhao, B., Leung, E., Safaee, S. M. R., and Liboiron-Ladouceur, O. (2023). Addressing the programming challenges of practical interferometric mesh based optical processors. Opt. Express 31, 23851–23866. doi:10.1364/OE.489493
Motwani, Y., Seth, S., Dixit, D., Bagubali, A., and Rajesh, R. (2021). Multifactor door locking systems: a review. Mater. Today Proc. 46, 7973–7979. doi:10.1016/j.matpr.2021.02.708
Mourgias-Alexandris, G., Moralis-Pegios, M., Tsakyridis, A., Simos, S., Dabos, G., Totovic, A., et al. (2022). Noise-resilient and high-speed deep learning with coherent silicon photonics. Nat. Commun. 13, 5572. doi:10.1038/s41467-022-33259-z
Nagel, M., Fournarakis, M., Amjad, R. A., Bondarenko, Y., Van Baalen, M., and Blankevoort, T. (2021). A white paper on neural network quantization. arXiv preprint arXiv:2106.08295.
Reck, M., Zeilinger, A., Bernstein, H., and Bertani, P. (1994). Experimental realization of any discrete unitary operator. Phys. Rev. Lett. 73, 58–61. doi:10.1103/physrevlett.73.58
Shafiee, A., Banerjee, S., Chakrabarty, K., Pasricha, S., and Nikdast, M. (2024). “Analysis of optical loss and crosstalk noise in mzi-based coherent photonic neural networks,” in Journal of lightwave technology, 1–16.
Shen, Y., Harris, N. C., Skirlo, S., Prabhu, M., Baehr-Jones, T., Hochberg, M., et al. (2017). Deep learning with coherent nanophotonic circuits. Nat. photonics 11, 441–446. doi:10.1038/nphoton.2017.93
Shokraneh, F., Nezami, M. S., and Liboiron-Ladouceur, O. (2020). Theoretical and experimental analysis of a 4 × 4 reconfigurable MZI-based linear optical processor. J. Light. Technol. 38, 1258–1267. doi:10.1109/JLT.2020.2966949
Texas Instruments (2013). DAC081S101 8-bit micro power digital-to-analog converter with rail-to-rail output. (Rev. C).
Texas Instruments (2018). LMV7235 and LMV7239 75-ns, ultra low power, low voltage, rail-to-rail input comparator with open-drain and push-pull output. (Rev. O).
Thoziyoor, S., Ahn, J. H., Monchiero, M., Brockman, J. B., and Jouppi, N. P. (2008). “A comprehensive memory modeling tool and its application to the design and analysis of future memory hierarchies,” in 2008 international symposium on computer architecture, 51–62. doi:10.1109/ISCA.2008.16
Williamson, I. A. D., Hughes, T. W., Minkov, M., Bartlett, B., Pai, S., and Fan, S. (2020). Reprogrammable electro-optic nonlinear activation functions for optical neural networks. IEEE J. Sel. Top. Quantum Electron. 26, 1–12. doi:10.1109/JSTQE.2019.2930455
Yang, Z., Zhang, W., Ji, S., Zhou, P., and Jones, A. K. (2024). “Reducing smart phone environmental footprints with in-memory processing,” in International conference on hardware/software codesign and system synthesis (CODES+ISSS).
Zhang, H., Thompson, J., Gu, M., Jiang, X. D., Cai, H., Liu, P. Y., et al. (2021). Efficient on-chip training of optical neural networks using genetic algorithm. ACS Photonics 8, 1662–1672. doi:10.1021/acsphotonics.1c00035
Zhao, Z., Liu, D., Li, M., Ying, Z., Zhang, L., Xu, B., et al. (2019). “Hardware-software co-design of slimmed optical neural networks,” in Proceedings of the 24th asia and south pacific design automation conference (New York, NY, USA: Association for Computing Machinery), 705–710. ASPDAC ’19. doi:10.1145/3287624.3287720
Keywords: optical neural network, Mach-Zehnder interferometer, pruning, edge computing, event-based trigger
Citation: Zhao B, Dong X, Rahbardar Mojaver K, Meyer BH and Liboiron-Ladouceur O (2025) Pruning and optimization of optical neural network as a binary optical trigger. Adv. Opt. Technol. 13:1501208. doi: 10.3389/aot.2024.1501208
Received: 24 September 2024; Accepted: 12 December 2024;
Published: 07 January 2025.
Edited by:
Roberto Morandotti, Université du Québec, CanadaReviewed by:
Lianghua Wen, Yibin University, ChinaShuang Chang, Vanderbilt University, United States
Copyright © 2025 Zhao, Dong, Rahbardar Mojaver, Meyer and Liboiron-Ladouceur. This is an open-access article distributed under the terms of the Creative Commons Attribution License (CC BY). The use, distribution or reproduction in other forums is permitted, provided the original author(s) and the copyright owner(s) are credited and that the original publication in this journal is cited, in accordance with accepted academic practice. No use, distribution or reproduction is permitted which does not comply with these terms.
*Correspondence: Bokun Zhao, Ym9rdW4uemhhb0BtYWlsLm1jZ2lsbC5jYQ==