- 1Developmental Neuropsychology Laboratory, Biological Sciences Institute, Neurosciences Department, Universidade Federal de Minas Gerais, Belo Horizonte, Brazil
- 2Mathematics Education, School for Mathematics and Computer Science, Institute of Mathematics, Osnabrück University, Osnabrueck, Germany
- 3Post-Graduate Program of Education, School of Education, Universidade Federal Do Rio Grande Do Sul, Porto Alegre, Brazil
- 4Department of Special Education, The University of Texas at Austin, Austin, TX, United States
- 5Department of Psychology, Universidade Federal de Minas Gerais, Belo Horizonte, Brazil
- 6Neurocognition Department, Universidad Católica del Uruguay, Montevideo, Uruguay
- 7Post-Graduate Program of Psychology, Psychology Institute, Universidade Federal Do Rio Grande Do Sul, Porto Alegre, Brazil
- 8Centre for Mathematical Cognition, Loughborough University, Loughborough, United Kingdom
- 9Leibniz-Institut für Wissensmedien, Tübingen, Germany
- 10Department of Psychology, University of Tübingen, Tübingen, Germany
- 11LEAD Graduate School & Research Network, University of Tübingen, Tübingen, Germany
Introduction
Numeracy is critically associated with personal and vocational life-prospects (Evans et al., 2017; Grotlüschen et al., 2019); yet, many adults and children lack a basic level of proficiency (Jonas, 2018). At the same time, research interest in numerical cognition, and its neuro-cognitive foundations (e.g., Cohen Kadosh and Dowker, 2015), as well as in mathematics education (e.g., Dennis et al., 2016) continues to grow. In this opinion, we argue that more intensive discussion across the disciplines is necessary to answer the question how results from basic research can make it to the classroom, how classroom practices can be validated by research, and discuss a theoretical framework for guiding future transfer endeavors.
Transferring basic research results to educational praxis is not a new challenge. As early as 1899, James (1958) noted the difficulty of directly deriving suggestions for pedagogical practice from psychological research. Even when successful, research in psychology might not be enough to derive effective suggestions or direct conclusions for educational practice without considering environmental challenges and requirements of teaching. Clearly not all basic research aims at informing educational practice; however, failure of important results from research to successfully impact practice reflects missed opportunities at some point during dissemination—as is failing to validate effective existing practices through research to allow for what may be called practice-based evidence.
Basic Research, Applied Research, and Use-Inspired Basic Research
To illustrate possible barriers for moving basic research results on numerical cognition into the classroom, Stokes' Quadrant Model of Scientific Research (Stokes, 1997) may be considered. Agnostic to a specific discipline, Stokes offered two dimensions to visualize goals of research: research inspired by the quest for fundamental understanding vs. research specifically designed with consideration of use. Stokes emphasized that the two dimensions do not describe two opposite poles on a linear scale because if so, the quest for fundamental understanding and consideration of use would drift apart, or at least would not be connected. Additionally, Stokes described a category syncing basic research with more applied research which he termed use-inspired basic research. Research in this category is inspired by the quest for fundamental understanding, with the idea to explicitly consider usefulness for practical needs.
Disciplinary fields such as the learning sciences, cognitive science, neuroscience, and educational psychology may overlap in terms of more basic or more applied research. For reasons of parsimony, we conceptualized more basic research as that conducted in the disciplines of neuroscience, cognitive science, biology, and genetics. In contrast, we conceptualized more applied research as research in the disciplines of mathematics education, educational psychology, and the learning sciences. Moreover, we operationalized use-inspired basic research as research conducted by any of the above disciplines explicitly for use in educational contexts. Of course, each of these disciplines operates on different levels of observation (e.g., brain, individual, classroom) and therefore contributes considerably to our understanding of numerical cognition from the neuro-cognitive foundations to the acquisition and teaching of numerical skills. In the following, we provide examples of research from several fields.
More Basic and More Applied Research on Numerical Cognition
The number of meta-analyses published since 2015 manifests the contributions from both more basic and more applied research. Examples of more applied research on numerical cognition include evaluations of effectiveness of interventions in early childhood (Mononen et al., 2014; Wang et al., 2016; Christodoulou et al., 2017; Nelson and McMaster, 2019); for older students (Jitendra et al., 2018; Stevens et al., 2018); across age groups (Dennis et al., 2016); and across different regions of the world (Conn, 2017). Other examples include interventions for students with emotional difficulties (Losinski et al., 2019); math anxiety (Namkung et al., 2019); or on attitudes toward achievement (Savelsbergh et al., 2016); the impact of homework (Fan et al., 2017); and specific teaching strategies (Capar and Tarim, 2015; Rittle-Johnson et al., 2017; Guillaume and Van Rinsveld, 2018).
On the other hand, meta-analyses of more basic research include synthesized results on the association of numerical and spatial cognition (Hawes et al., 2019); magnitude understanding (Vanbinst and De Smedt, 2016; Sokolowski et al., 2017); rapid automatized naming (Koponen et al., 2017); specific brain regions associated with numerical cognition (Yeo et al., 2017); specific numerical processes (Arsalidou et al., 2018); specific cognitive functions (Peng et al., 2016); different numerical representations (Schneider et al., 2017); and genetic influences (Chen et al., 2017; King et al., 2019).
The above list is far from exhaustive. Synthesizing the entire corpus of work-to-date to create a holistic understanding of what we currently do and do not know on numerical cognition, and then disseminating that work across disciplines and to educators, is a substantial challenge for moving research results into the classroom. Looking at just 15 evidence-based instructional practices, using three different procedures for either early or late implementation, Koedinger et al. (2013) explained that an educator would have to consider 205 trillion options; and the effectiveness of these instructional practices is susceptible to contextual variables (e.g., Dunlosky et al., 2013; Davenport et al., 2019).
Research and Noisy Application in Classrooms
But how can research then come to influence classroom practice? And how can classroom practice influence what is researched? In our opinion, suggestions for two-way bridges over research-to-practice gaps (e.g., Bowers, 2016; Reynvoet et al., 2016; Mackey, 2019; Thomas, 2019) require more in-depth analysis. Where (Stokes, 1997) provides a macro-view, Connell's Adaptation Loop (2012, see Figure 1) provides a closer look.
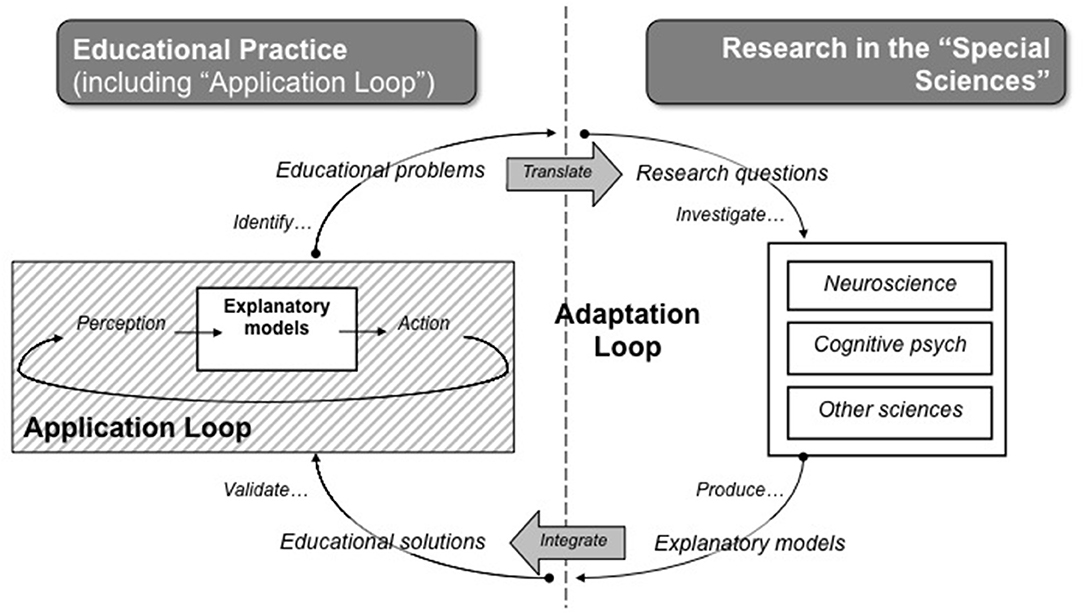
Figure 1. Adaptation Loop (Source: Connell et al., 2012, Reproduced with permission of Michael W. Connell ©2010–2014. All rights reserved. Author contact: Michael.W.Connell@gmail.com).
The right chart of Figure 1 reflects research, whereas the left represents educational practice. Moving in clockwise direction, starting at the top left corner of the diagram, the process of research and adaptation illustrates recognizing a problem, translating the problem into research questions, investigating questions by scientific domain, providing explanations, designing solutions, validating solutions in the educational environment, and then repeating the process.
However, within the domain of educational practice, the application loop indicates the iterative nature of changes within educational settings and reflects the necessity for further adaptation during the validation process. We suggest the Application Loop model as an accurate reflection of what occurs within education. Education is not a unitary system, but a system made up of different sub-systems with hierarchies of stakeholders (i.e., policymakers, administrators, teachers, students). Implementation of explanatory models or interventions previously proven effective in basic research often fails to produce similar results in educational practice because, at each hierarchical level, humans make decisions which introduce new variables. While researchers are cognizant of some of these variables, and often consider these as noise with the aim to control these through experimental design or statistical models, this noise may be the key to the comprehension of use-inspired basic research.
Listen to the Noise
Collaboration, whether across disciplines or within educational contexts, with the explicit aim of conducting use-inspired research, is not easy. Berliner claimed that education research “is the hardest science of all” (Berliner, 2002, p. 18). Below we discuss a few issues critical for researchers to consider when planning to conduct use-inspired research.
First, research in the classroom interrupts daily business of teachers and students. Moreover, testing in classrooms and controlled interventions change the typical dynamic of teaching and learning. Evaluating the effectiveness of interventions may necessitate students' absence from the classroom. Such interruptions not only let students miss instruction but may also disturb learning progress of other students. Students may either come to resent being pulled from their classroom or resent not being pulled when not assigned to the treatment group. These circumstances reflect conflicts of goals between the parties involved in use-inspired research, which may lead to tensions.
Additionally, researchers are interested in publishing their work, thus strive for theoretically and methodologically sound but also positive results. Therefore, they have to include multiple and different measures to evaluate effects of interest, or to control for potential moderators, mediators, or confounds. However, time in classrooms is limited and a precious resource. Schools have demands, schedules, and goals, which are different from those of researchers. This discrepancy often leads to a zero-sum game, in which compromises to meet the needs and interests of both schools and researchers may impact outcomes. Careful consideration of the cost/benefit of variables likely to inform research results requires balancing the cost to the students/teachers/schools and the benefits to science.
How to Increase Use-Inspired Basic Research
There have been others advocating for use-inspired basic research with careful consideration of how to increase implementation and ecological validity of research (e.g., Cai et al., 2017, 2018, 2019). For example, Smolkowski et al. (2019) provided suggestions on levels of implementation, and Higgins et al. (2019) focused on how research can become more use-inspired:
• Choose outcome measures that matter to educators in their context
• Include educators and students in the research process (i.e., researching with them not on them)
• Be flexible and sensitive to time and schedules
• Consider that research that was effective in the lab may not be effective in the classroom
• Ask questions educators want and need to have answered
• Disseminate findings in non-academic media (i.e., social media, websites); attend educator specific conferences.
Space limitations do not allow us to provide multiple successful examples of such use-inspired research (e.g., Hawes et al., 2020), of research partnerships (e.g., Kaplan et al., 2019), or of societies actively promoting and including educators during their annual conferences (e.g., The Math Cognition Learning Society, The International Mind, Brain, and Education Society, The Earli SIG 22). We recommend readers consider the above citations as references for how to reframe perspectives of what it means to conduct use-inspired research. Additionally, researchers interested in what teachers are doing in the (math) classroom can follow the Twitter hashtags #mtbos, #iteachmath, and #SwDMathChat. These clearly indicate that educators often ask the same questions as researchers; although usually without the benefit of being able to validate their work beyond their personal and peer experiences. Collaborative work is happening, though not yet at scale. For example, educator Simon Gregg and researcher Tali Leibovich-Raveh co-authored a paper on numerical magnitude understanding after several discussions on Twitter (preprint: https://osf.io/ndyb6/). Sharing preprints via social media, talking to educators face-to-face, going to educator focused conferences, or any other means of closing feedback loops are examples of ways to move research on numerical cognition forward within and across disciplines.
Interdisciplinary, Collaborative Research: A Way to Bridge the Gap?
More than 100 years ago, James (1958) not only described the difficulty of directly deriving suggestions from psychological research to pedagogical practice; he also claimed that research must include the expertise of educators to respect the complexity of teaching in classrooms. A first step would be when basic and more applied research on numerical cognition find a shared vocabulary and bring their expertise together to do interdisciplinary use-inspired basic research (i.e., Stokes, 1997). Moreover, going from the lab to the classroom and vice versa could offer new perspectives for teaching and learning. Connell et al. (2012) idea of application loops points to the next steps by indicating the necessity of iterations at the application stage to consider contextual demands of classroom practice. To illustrate, imagine various entities in Connell et al. (2012) as overlapping concentric circles in a Venn Diagram: circles for each domain of applied and basic research, and also circles for the different stakeholders in educational practice. Maybe, any two circles will overlap, or some may overlap with more than one other circle, but in the best case, all circles should overlap at a shared core. Each circle is necessary, but the point at which all circles overlap is where use-inspired, contextually relevant research occurs. There will always be a need for basic research, which may not directly impact use, and many open questions remain for researchers to explore. In contrast, classroom teachers have context-specific and practice-relevant questions for research. We propose that results from research should find their way into classrooms, but we need more integration of different perspectives and fruitful collaborations between researchers of different disciplines with educators. Only then we may have a chance to bring results from basic research into educational practice. However, as Minshall (2009) put it, “knowledge transfer is a ‘contact sport'; it works best when people meet to exchange ideas, … and spot new opportunities.”
Author Contributions
Each of the authors contributed equally to the theoretical framing of this article and contributed to edits throughout the writing process. HS wrote the initial draft based on notes from members of our workshop held at the Brazilian-German Numerical Cognition Winter School, Belo Horizonte, Brazil, August 2019. To acknowledge his work in transcribing our workshop notes, HS was considered the first author. HG located the call for manuscripts at Frontiers, managed the contributions of co-authors and finalized the manuscript. Therefore, we listed her as the second author.
Conflict of Interest
The authors declare that the research was conducted in the absence of any commercial or financial relationships that could be construed as a potential conflict of interest.
Acknowledgments
We extend our gratitude to Michael W. Connell for his permission to use the figure. We also extend our gratitude to the organizers (VH and KM) and all participants at the Brazilian-German Winter School 2019 for their constructive feedback on our workshop presentation. We acknowledge support by Deutsche Forschungsgemeinschaft (DFG) and Open Access Publishing Fund of Osnabrück University.
References
Arsalidou, M., Pawliw-Levac, M., Sadeghi, M., and Pascual-Leone, J. (2018). Brain areas associated with numbers and calculations in children: meta-analyses of fMRI studies. Dev. Cogn. Neurosci. 30, 239–250. doi: 10.1016/j.dcn.2017.08.002
Berliner, D. C. (2002). Comment: educational research: the hardest science of all. Educ. Res. 31, 18–20. doi: 10.3102/0013189X031008018
Bowers, J. S. (2016). Psychology, not educational neuroscience, is the way forward for improving educational outcomes for all children: Reply to Gabrieli (2016) and Howard-Jones et al. (2016). Psychol. Rev. 123, 628–635. doi: 10.1037/rev0000043
Cai, J., Morris, A., Hohensee, C., Hwang, S., Robison, V., and Hiebert, J. (2017). A future vision of mathematics education research: blurring the boundaries of research and practice to address teachers' problems. J. Res. Math. Educ. 48, 466–473. doi: 10.5951/jresematheduc.48.5.0466
Cai, J., Morris, A., Hohensee, C., Hwang, S., Robison, V., and Hiebert, J. (2018). Using data to understand and improve students' learning: empowering teachers and researchers through building and using a knowledge base. J. Res. Math. Educ. 49, 362–372. doi: 10.5951/jresematheduc.49.4.0362
Cai, J., Morris, A., Hohensee, C., Hwang, S., Robison, V., and Hiebert, J. (2019). Research pathways that connect research and practice. J. Res. Math. Educ. 50, 2–10. doi: 10.5951/jresematheduc.50.1.0002
Capar, G., and Tarim, K. (2015). Efficacy of the cooperative learning method on mathematics achievement and attitude: a meta-analysis research. Educ. Sci. Theory Pract. 15, 553–559. doi: 10.12738/estp.2015.2.2098
Chen, H., Gu X, H, Zhou, Y., Ge, Z., Wang, B., et al. (2017). A genome-wide association study identifies genetic variants associated with mathematics ability. Sci. Rep. 7:40365. doi: 10.1038/srep40365
Christodoulou, J., Lac, A., and Moore, D. S. (2017). Babies and math: a meta-analysis of infants' simple arithmetic competence. Dev. Psychol. 53, 1405–1417. doi: 10.1037/dev0000330
Cohen Kadosh, R., and Dowker, A. (Eds.). (2015). The Oxford Handbook of Numerical. Oxford: Oxford Library of Psychology.
Conn, K. M. (2017). Identifying effective education interventions in sub-Saharan Africa: a meta-analysis of impact evaluations. Rev. Educ. Res. 87, 863–898. doi: 10.3102/0034654317712025
Connell, M., Stein, Z., and Gardner, H. (2012). “Bridging between brain science and educational practice with design patterns,” in Neuroscience in Education: The Good, the Bad, and the Ugly, eds S. D. Sella and M. Anderson (Oxford: Oxford University Press), 267–285.
Davenport, J. L., Kao, Y. S., Matlen, B. J., and Schneider, S. A. (2019). Cognition research in practice: engineering and evaluating a middle school math curriculum. J. Exp. Educ. 1–20. doi: 10.1080/00220973.2019.1619067
Dennis, M. S., Sharp, E., Chovanes, J., Thomas, A., Burns, R. M., Custer, B., et al. (2016). A meta-analysis of empirical research on teaching students with mathematics learning difficulties. Learn. Disabil. Res. Pract. 31, 156–168. doi: 10.1111/ldrp.12107
Dunlosky, J., Rawson, K. A., Marsh, E. J., Nathan, M. J., and Willingham, D. T. (2013). Improving students' learning with effective learning techniques: promising directions from cognitive and educational psychology. Psychol. Sci. Public Int. 14, 4–58. doi: 10.1177/1529100612453266
Evans, J., Yasukawa, K., Mallows, D., and Creese, B. (2017). Numeracy skills and the numerate environment: affordances and demands. Adults Learn. Math. Int. J. 12, 17–26. Available online at: https://files.eric.ed.gov/fulltext/EJ1159235.pdf
Fan, H., Xu, J., Cai, Z., He, J., and Fan, X. (2017). Homework and students' achievement in math and science: a 30-year meta-analysis, 1986–2015. Educ. Res. Rev. 20, 35–54. doi: 10.1016/j.edurev.2016.11.003
Grotlüschen, A., Buddeberg, K., Redmer, A., Ansen, H., and Dannath, J. (2019). Vulnerable subgroups and numeracy practices: how poverty, debt, and unemployment relate to everyday numeracy practices. Adult Educ. Q. 69, 251–270. doi: 10.1177/0741713619841132
Guillaume, M., and Van Rinsveld, A. (2018). Comparing numerical comparison tasks: a meta-analysis of the variability of the Weber Fraction relative to the generation algorithm. Front. Psychol. 9:1694. doi: 10.3389/fpsyg.2018.01694
Hawes, Z., Cain, M., Jones, S., Thomson, N., Bailey, C., Seo, J., et al. (2020). Effects of a teacher-designed and teacher-led numerical board game intervention: A randomized controlled study with 4- to 6-year olds. Mind Brain Educ. 14, 71–80. doi: 10.1111/mbe.12215
Hawes, Z., Sokolowski, H. M., Ononye, C. B., and Ansari, D. (2019). Neural underpinnings of numerical and spatial cognition: an fMRI meta-analysis of brain regions associated with symbolic number, arithmetic, and mental rotation. Neurosci. Biobehav. Rev. 103, 316–336. doi: 10.1016/j.neubiorev.2019.05.007
Higgins, E. J., Dettmer, A. M., and Albro, E. R. (2019). Looking back to move forward: a retrospective examination of research at the intersection of cognitive science and education and what it means for the future. J. Cognit. Dev. 2, 278–297. doi: 10.1080/15248372.2019.1565537
James, W. (1958). Talks to Teachers–On Psychology and To Students of Some Life's Ideals. New York, NY: W.W. Norton.
Jitendra, A. K., Lein, A. E., Im, S. H., Alghamdi, A. A., Hefte, S. B., and Mouanoutoua, J. (2018). Mathematical interventions for secondary students with learning disabilities and mathematics difficulties: a meta-analysis. Except. Child. 84, 177–196. doi: 10.1177/0014402917737467
Jonas, N. (2018). Students' Numeracy Skills and Practices. Adult Skills in Focus. Paris: OECD Publishing.
Kaplan, R. G., Riedy, R., Van Horne, K., and Penuel, W. (2019). Going on a statewide listening tour: involving education leaders in the process of research to enhance the practical value of qualitative research. Evid. Policy 15, 171–196. doi: 10.1332/174426518X15193816575650
King, M. J., Katz, D. P., Thompson, L. A., and Macnamara, B. N. (2019). Genetic and environmental influences on spatial reasoning: a meta-analysis of twin studies. Intelligence 73, 65–77. doi: 10.1016/j.intell.2019.01.001
Koedinger, K. R., Booth, J. L., and Klahr, D. (2013). Instructional complexity and the science to constrain it. Science 342, 935–937. doi: 10.1126/science.1238056
Koponen, T., Georgiou, G., Salmi, P., Leskinen, M., and Aro, M. (2017). A meta-analysis of the relation between RAN and mathematics. J. Educ. Psychol. 109, 977–992. doi: 10.1037/edu0000182
Losinski, M. L., Ennis, R. P., Sanders, S. A., and Nelson, J. A. (2019). A meta-analysis examining the evidence-base of mathematical interventions for students with emotional disturbances. J. Spec. Educ. 52, 228–241. doi: 10.1177/0022466918796200
Mackey, A. P. (2019). Commentary: Broadening the scope of educational neuroscience, reflections on Thomas, Ansari, and Knowland (2019). J. Child Psychol. Psychiatry 60, 493–495. doi: 10.1111/jcpp.13030
Minshall, T. (2009). What is knowledge transfer? Available online at: https://www.cam.ac.uk/research/news/what-is-knowledge-transfer
Mononen, R., Aunio, P., Koponen, T., and Aro, M. (2014). A review of early numeracy interventions for children at risk in mathematics. Int. J. Early Childh. Spec. Educ. 6, 25–54. doi: 10.20489/intjecse.14355
Namkung, J. M., Peng, P., and Lin, X. (2019). The relation between mathematics anxiety and mathematics performance among school-aged students: a meta-analysis. Rev. Educ. Res. 89, 459–496. doi: 10.3102/0034654319843494
Nelson, G., and McMaster, K. L. (2019). The effects of early numeracy interventions for students in preschool and early elementary: a meta-analysis. J. Educ. Psychol. 111, 1001–1022. doi: 10.1037/edu0000334
Peng, P., Namkung, J., Barnes, M., and Sun, C. (2016). A meta-analysis of mathematics and working memory: moderating effects of working memory domain, type of mathematics skill, and sample characteristics. J. Educ. Psychol. 108, 455–473. doi: 10.1037/edu0000079
Reynvoet, B., Smets, K., and Sasanguie, D. (2016). Number sense: what's in a name and why should we bother?” in Continuous Issues in Numerical Cognition. How Many or How Much, ed A. Henik (London; San Diego, CA; Cambridge, Oxford: Elsevier), 195–214.
Rittle-Johnson, B., Loehr, A. M., and Durkin, K. (2017). Promoting self-explanation to improve mathematics learning: a meta-analysis and instructional design principles. ZDM 49, 599–611. doi: 10.1007/s11858-017-0834-z
Savelsbergh, E. R., Prins, G. T., Rietbergen, C., Fechner, S., Vaessen, B. E., Draijer, J. M., et al. (2016). Effects of innovative science and mathematics teaching on student attitudes and achievement: a meta-analytic study. Educ. Res. Rev. 19, 158–172. doi: 10.1016/j.edurev.2016.07.003
Schneider, M., Beeres, K., Coban, L., Merz, S., Schmidt, S. S., Stricker, J., et al. (2017). Associations of non-symbolic and symbolic numerical magnitude processing with mathematical competence: a meta-analysis. Dev. Sci. 20:e12372. doi: 10.1111/desc.12372
Smolkowski, K., Crawford, L., Seeley, J. R., and Rochelle, J. (2019). Introduction to implementation science for research on learning disabilities. Learn. Disab. Q. 42, 192–203. doi: 10.1177/0731948719851512
Sokolowski, H. M., Fias, W., Ononye, C. B., and Ansari, D. (2017). Are numbers grounded in a general magnitude processing system? A functional neuroimaging meta-analysis. Neuropsychologia 105, 50–69. doi: 10.1016/j.neuropsychologia.2017.01.019
Stevens, E. A., Rodgers, M. A., and Powell, S. R. (2018). Mathematics interventions for upper elementary and secondary students: a meta-analysis of research. Remed. Spec. Educ. 39, 327–340. doi: 10.1177/0741932517731887
Stokes, D. E. (1997). Pasteur's Quadrant. Basic Science and Technological Innovation. Washington, DC: Brookings Institution Press.
Thomas, M. S. (2019). Response to Dougherty and Robey (2018) on neuroscience and education: enough bridge metaphors—interdisciplinary research offers the best hope for progress. Curr. Direct. Psychol. Sci. 4, 337–340. doi: 10.1177/0963721419838252
Vanbinst, K., and De Smedt, B. (2016). Individual differences in children's mathematics achievement: the roles of symbolic numerical magnitude processing and domain-general cognitive functions. Prog. Brain Res. 227, 105–130. doi: 10.1016/bs.pbr.2016.04.001
Wang, A. H., Firmender, J. M., Power, J. R., and Byrnes, J. P. (2016). Understanding the program effectiveness of early mathematics interventions for prekindergarten and kindergarten environments: a meta-analytic review. Early Educ. Dev. 27, 692–713. doi: 10.1080/10409289.2016.1116343
Keywords: numerical cognition, transfer of knowledge, applied science, math education, evidence-based practice, research-to-practice
Citation: Simplicio H, Gasteiger H, Dorneles BV, Grimes KR, Haase VG, Ruiz C, Liedtke FV and Moeller K (2020) Cognitive Research and Mathematics Education—How Can Basic Research Reach the Classroom? Front. Psychol. 11:773. doi: 10.3389/fpsyg.2020.00773
Received: 29 November 2019; Accepted: 30 March 2020;
Published: 23 April 2020.
Edited by:
Karin Binder, University of Regensburg, GermanyReviewed by:
Philipp Alexander Schroeder, University of Tübingen, GermanyDelphine Sasanguie, Hogeschool Gent, Belgium
Copyright © 2020 Simplicio, Gasteiger, Dorneles, Grimes, Haase, Ruiz, Liedtke and Moeller. This is an open-access article distributed under the terms of the Creative Commons Attribution License (CC BY). The use, distribution or reproduction in other forums is permitted, provided the original author(s) and the copyright owner(s) are credited and that the original publication in this journal is cited, in accordance with accepted academic practice. No use, distribution or reproduction is permitted which does not comply with these terms.
*Correspondence: Hedwig Gasteiger, hedwig.gasteiger@uni-osnabrueck.de