- 1Istituto Auxologico Italiano IRCCS, Psychology Research Laboratory, San Giuseppe Hospital, Verbania, Italy
- 2Department of Psychology, Catholic University of the Sacred Heart, Milan, Italy
- 3Faculty of Psychology, eCampus University, Como, Italy
- 4Istituto Auxologico Italiano IRCCS, Cardiac Rehabilitation Unit, San Giuseppe Hospital, Verbania, Italy
Objective: Exercise capacity (EC) is a well-established predictor of cardiovascular health. It is notoriously influenced by several factors, but the independent effect of psychological well-being (PWB) on EC has not yet been explored. The present study aims to investigate (1) whether PWB is an independent predictor of EC over and above selected demographic, behavioral, and biomedical parameters in a sample of CR patients with obesity and (2) whether PWB is a stronger predictor of EC than the other variables.
Methods: Data from 1968 patients were collected at the time of their inclusion in a cardiac rehabilitation (CR) program and retrospectively analyzed in a cross-sectional study. Since cardiorespiratory parameters defined in normal weight populations differ from those of their obese counterparts, an ad hoc validated formula taking body mass index (BMI) into consideration was used to predict EC.
Results: A multiple regression analysis revealed left ventricular eject fraction (LVEF) to be the strongest predictor of EC, followed by PWB, type 2 diabetes (DM), smoking status, atrial fibrillation (AF), and education. Bayesian evaluation of informative hypotheses corroborated LVEF as the best predictor of EC, and confirmed the superiority of PWB over and above DM and smoking status in influencing EC.
Conclusion: These findings strengthen the link between psychological and physical health, suggesting a better PWB is associated with greater EC. Prompt screening of a patient’s mood and readiness to perform an active lifestyle would therefore enhance the long-term health benefits of CR.
Introduction
Cardiovascular disease (CVD) is a leading cause of disability and premature death, globally, and substantially contributes to the escalating costs of healthcare (Roth et al., 2017). The American Heart Association (AHA) has established a sedentary lifestyle as a major modifiable risk factor for CVD (Thompson et al., 2003). However, despite overwhelming evidence promoting an active lifestyle in both primary prevention and formal cardiac rehabilitation (CR), evidence suggests that patients with CVD often fail to maintain physical activity (PA) recommendations (Dontje et al., 2014; Yates et al., 2017).
Still, a low level of PA increases the risk of developing other chronic conditions including diabetes, hypertension, obesity (Wing and Hill, 2001), and depression (Pollock, 2001; Dibben et al., 2018). Findings from a meta-analysis of 63 randomized studies evaluating the efficacy of different CR programs–with and without exercise–in patients with documented ischemic heart disease (IHD) showed that exercise alone produced a significant reduction in diverse causes of mortality (Clark et al., 2005). Thus, exercise capacity (EC) is a valuable measure for the control and treatment of CVD, and its assessment provides important information to guide exercise prescription (Demers et al., 2001; Banerjee et al., 2012). Assessment includes subjective evaluation of an individual’s exercise tolerance and objective exercise test (ET) results.
Besides the well-known effect of age and gender, which continue to be the basis of the equation most frequently used to calculate exercise intensity (Ahmadian et al., 2013), evidence exists for the influence of body mass index (BMI, kg/m2) on EC (Gondoni et al., 2010; Gong et al., 2013). Obesity is constantly increasing in prevalence (Pietrabissa et al., 2012; Holmgren et al., 2017) and the cardiovascular benefits obtained from increasing PA are greater than those from dietary control to lose weight.
Sufficient information is also found in the medical literature on the role of atrial fibrillation (AF) (Osbak et al., 2012; Zakeri et al., 2014), left ventricular ejection fraction (LVEF) (Wong and Yeo, 2010), type 2 diabetes (DM) (Awotidebe et al., 2014), smoking status (Mesquita et al., 2015), and educational level (Witham et al., 2006) in predicting aerobic capacity in patients with CVD.
Although evidence exists for the negative impact of impaired EC on perceived psychological well-being (PWB) in the cardiac population (Wang, 2018), the role of psychosocial domains on EC remains unclear (Chiala et al., 2018). Also, to our knowledge, no study has yet tested the independent effect of PWB in determining the maximum amount of physical exertion that a patient with both CVD and obesity can sustain. Understanding this effect could assist in the design of tailored assessments and intervention procedures in CR.
The present cross-sectional study aims to investigate (1) whether PWB is an independent predictor of EC over and above a series of selected demographic, behavioral, and biomedical parameters in a sample of patients with CVD and obesity, and (2) whether PWB is a stronger predictor of EC than the other selected variables.
Materials and Methods
Participants
From January 2012 to June 2019, relevant data were collected from 1968 consecutive patients (1348 males) with CVD and obesity who were referred to a single clinical center (Istituto Auxologico Italiano IRCCS, San Giuseppe Hospital, Verbania, Italy) to attend a comprehensive cardiac and nutritional rehabilitation program (duration 25 ± 3 days).
Inclusion criteria for participating in the study were (1) being 18 or over; (2) presenting a diagnosis of IHD, defined as a history of at least one of the following: myocardial infarction, coronary artery bypass grafting, or percutaneous transluminal coronary angioplasty; (3) presenting a history of congestive heart failure (CHF) with either reduced or preserved EF; (4) having BMI ≥ 30; and (5) having undergone symptom-limited exercise stress testing to define their effort tolerance for exercise.
Patients (1) with recent (less than 2 months) myocardial infarction, coronary artery bypass, or coronary angioplasty or (2) who were unable to perform ET were excluded from the study.
All patients were clinically stable, and none had clinically evident heart failure according to the Framingham criteria (McKee et al., 1971).
The study conformed with the principles outlined in the Declaration of Helsinki and complied with APA ethical standards.
Measures
At the time of inclusion for CR, all subjects underwent a comprehensive routine assessment, including clinical history, physical examination, laboratory tests, and echocardiogram for the calculation of LVEF–which is the percentage of the diastolic left ventricular volume that is pumped out during systole–and exercise stress test. Also, demographic (i.e., age, gender, education) and behavioral (smoking status) parameters were collected at baseline.
Exercise Test Protocol
A Marquette series 2000 motorized treadmill and Marquette Max Personal electrocardiography (ECG) instrumentation (Marquette Medical Systems, Milwaukee, WI, United States) were used to assess patients’ EC for treadmill speed and grade. EC is defined as the maximal oxygen intake for a stated workload, and it is commonly measured in metabolic equivalents (METs).
The test was tailored to patients’ characteristics, and a ramp protocol was used. ET was conducted in the morning, at least 2 h after breakfast and while taking regular medication.
Patients were encouraged to continue exercising until symptoms prevented them from continuing, even after reaching 85% of their maximum predicted heart rate. Reasons for test termination were limiting symptoms (i.e., fatigue, angina, dyspnea, and muscular pain), abnormal ECG, abnormal blood pressure, or choice of the patient.
Since cardiorespiratory parameters defined in normal weight populations differ from those of patients with obesity, the following ad hoc validated formula that takes into account BMI along with other variables (i.e., height, age, and gender) was used to predict EC: Predicted EC (METs) = 14.53 − (0.12 × age in years) −(0.17 × BM in kg/m2I) + (3.16 × height in meters) (+ 0.71 for male patients) (Gondoni et al., 2006). The ratio between measured and predicted values was calculated.
Psychological Assessment
The Italian version of the psychological general well-being index (PGWBI) was used to investigate the patients’ self-evaluation of their perceived PWB. The score is derived from the summary score of six dimensions through 22 items: anxiety, depressed mood, positive well-being, self-control, general health, and vitality (Grossi et al., 2006). The PGWBI has been translated and culturally adapted into several languages across diverse populations of chronic patients and displays high values of Cronbach’s alpha coefficients (range 0.80–0.94). The majority of applications have been in studies involving patients with CVD (Croog et al., 1986). The overall reliability index for the present sample was 0.87. Data were obtained retrospectively from the patients’ medical records by a research assistant.
For the aims of the present study, literature-based selected predictors of EC were perceived PWB, education, LVEF, DM, AF, and smoking status.
Since BMI, height, age, and gender are parameters already included in the equation used to predict EC, these variables were excluded from the analysis.
Statistical Analysis
Data were examined prior to hypothesis testing for identification of any missing data and normality, revealing no missing data that was normally distributed.
Descriptive statistics were performed to summarize the characteristics of the sample.
A multiple regression analysis was performed to evaluate whether DM, LVEF, AF, smoking status, education, and the PGWBI total score were significant and independent predictors of EC. The model was fitted by a forward stepwise method. Parametric assumptions, possible outliers, and influential cases were assessed by inspection of diagnostic plots and calculation of ad hoc statistics. Critical alpha was set at 0.05, if not otherwise specified, and p-values ≤ 0.05 were considered as statistically significant.
A Bayesian method for the evaluation of informative hypotheses on regression coefficients was also used to compare the predictors for statistical differences and identify the most influential ones (Kluytmans et al., 2012).
Descriptive statistics and regression analysis were completed with SPSS 20.0 for windows (Release 20.0.0, SPSS Inc.), while the BIEMS free software package (Mulder et al., 2009) was used to perform Bayesian analysis.
Results
Of 1968 patients, 620 were female (31.5%) and 1348 were male (68.5%). The mean age of the sample was 62.2 (SD = 9.5), and the average BMI was 38.8 (SD = 5.4) (Table 1).
Predictors of EC
The stepwise multiple regression analysis stopped at step 6 and the final model included LVEF, PGWBI, DM, smoking, AF, and education as significant and independent predictors of EC [F(6.1961) = 38.53; p < 0.001]. The multiple correlation coefficient was 0.32, indicating that approximately 10.5% of the variance of EC could be explained by the included variables.
Diagnostic statistics showed no collinearity among the predictors (VIF values were all well below 4 and the tolerance statistics all well above 0.2) (Hair et al., 2010), and the P–P plot, as well as the histogram, suggested normality of residuals. However, a relatively random display of points in the scatterplot of standardized residuals against standardized predicted values did not provide evidence of homogeneity of variance.
The Durbin-Watson statistic was also computed to evaluate independence of errors; the result was equal to 0.21, which was not considered acceptable.
Inspection of standardized residuals revealed that heteroskedasticity did not concern the LVEF and AF variables. With respect to outliers and influential cases, no more than 5% of cases were shown to have absolute standardized residuals above 2 (3.6%; n = 71). Still, 15 cases (0.76%) had standardized residuals equal to or above 3 and could thus be considered as outliers. However, neither the Mahalanobis and Cook distances, nor the DFBeta values, showed any case significantly influencing the estimation of the model parameters.
Bayesian Analysis
Standardized regression coefficients (βs) showed LVEF to be the strongest predictor of EC in the study sample, followed by the PGWBI total score, DM, smoking status, and AF (Table 2).
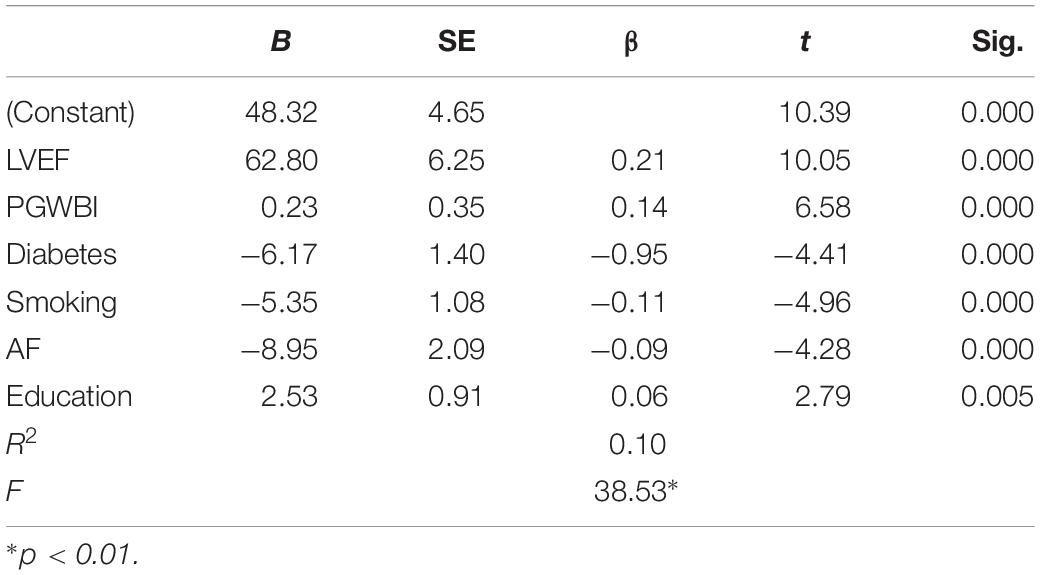
Table 2. Summary of stepwise regression analyses for variables predicting exercise capacity (n = 1968).
However, this ranking is purely descriptive and provides no statistical evidence about the superiority of LVEF over PWB, or of PWB over the other determinants in predicting EC in the present population. Three informative hypotheses were thus formulated: (1) βLVEF > βPWB, (2) βPWB > βDM, and (3) βPWB > βSmoking.
BIEMS generated a default prior and calculated a Bayes Factor for each hypothesis versus its unconstrained alternative (both βs are free to vary from minus to plus infinity).
For the first hypothesis, a Bayes factor of 1.96 was found. This means that the hypothesis stating that LVEF is more important for predicting EC than the PGWBI received almost two times more support from the data than the unconstrained hypothesis.
The second hypothesis received a Bayes factor of 2.00 and the third one received a value of 2.01, indicating that the data supported the superiority of PGWBI over both DM and smoking status in predicting EC.
Discussion
This study targeted a large representative sample of patients with CVD and comorbid obesity referred for CR and revealed PWB to be a strong predictor of EC, second only to LVEF. Indeed, left ventricular dysfunction has been shown to have a largely demonstrated negative impact on exercise performance (von Roeder et al., 2017; Sacre et al., 2018), especially in obese patients (Benge et al., 1980). Moreover, DM, smoking status, AF, and education were significant predictors of EC in the present sample, although to a lesser extent than the subjective well-being.
Consistently, previous studies showed reduced EC in individuals without CVD who had DM (Choe et al., 2018), and a lower improvement of exercise tolerance in CR patients with diabetes compared with their non-diabetic counterparts–independently from their BMI (Verges et al., 2004; Kim et al., 2015).
These findings might be explained by the strong association between subjective well-being and lifestyle habits in people with DM. Diabetes is a chronic disease that affects both health and PWB (Moreno and Pearson, 2011). In fact, diet and exercise are the foundation of most therapies for diabetes (Tonetto et al., 2019), and people with DM often feel challenged by their disease-related features and complications and its day-to-day management demands (Thapa et al., 2019). Fears about the reality of complications (i.e., obesity, CVD, renal failure, etc.) also prevent people from exercising (Stryker, 2016) and are responsible for impaired PWB in persons with DM (Jing et al., 2018). At the same time, feeling good and staying healthy would give a further boost to the subjective well-being of the sufferers and their health-related outcomes. Accurate lifestyle analysis of patients suffering from CVD and other chronic conditions is, therefore, important for a comprehensive assessment in CR and the promotion of disease prevention strategies (Ceccarini et al., 2015; Pietrabissa et al., 2017b). This includes the screening of smoking habits and of socioeconomic status (SES) of the person.
In fact, cigarette smoking is a powerful independent risk factor for CVD and has a significant negative impact on EC (Asthana et al., 2012) and other cardiovascular parameters (Unverdorben et al., 2008). Still, most smokers gain weight after quitting due to increased energy intake and reduced energy expenditure. In order to prevent or reduce the onset of further complications, such as diabetes or obesity, actions for smoking cessation should, therefore, be combined with weight control interventions. These must be tailored for home-based care that would consider social disparities and meet the varying needs (and limitations) of the patients (Favoccia et al., 2014).
In fact, previous research has found inverse relationships between SES–including household income, education, housing status, and occupation–and unhealthy behaviors (Lantz et al., 2001; Pampel et al., 2010). In fact, socioeconomically challenged individuals confront more barriers (e.g., access, cost) to modifying risk behaviors over time such as quitting smoking, improving diet, increasing PA, and adhering to medications (Chandrasekhar, 2019). In particular, low education levels might affect the individuals’ ability to interpret and to comply with health recommendations and to interface effectively with healthcare professionals (Kavanagh et al., 2010; Stringhini et al., 2010; Prag and Subramanian, 2017; Andrade and Mehta, 2018; Vonneilich et al., 2019), therefore constituting a risk factor for adherence to PA programs and functional EC (Anderson et al., 1997; Shishehbor et al., 2006).
Strengths and Limitation of the Study
The strengths of this study include its large sample size and the use of the Bayesian approach, which provides the most theoretically defensible framework that can be used to address probabilistic questions.
However, this work must be considered in light of several potential limitations. Firstly, absence of a comprehensive screening of mental health problems [e.g., eating disorders (Faulconbridge et al., 2012) and depression (Alizai et al., 2015; González-Castro et al., 2019)], readiness to change, and perceived self-efficacy of the participants prevented us from clarifying the role played by emotional factors in influencing the amount of physical effort that an individual with obesity and cardiac complication can sustain. Adaptation to living with CVD may, in fact, differ for each individual, and further studies should also investigate the role played by disease severity/typology (i.e., coronary artery disease–CAD, ischemic heart disease–IHD, or chronic heart failure–CHF) in the relation between PWB and patient-reported EC. Moreover, obesity might affect CR in many ways, but the absence of a control condition did not allow us to investigate the specific impact of PWB on EC during CR in both obese and non-obese people with CVD. It is also possible that other unmeasured modifiable and non-modifiable variables might account for these results, including family history, blood pressure, pulmonary functions, the skeletal muscle status, or the individuals’ perceived social support. Lastly, the use of self-report methods in the assessment of the individuals’ PWB and the absence of a proper cognitive screening in CR might limit the reliability of the research findings. Socially desirable responding, difficulties in assessing themselves accurately or misunderstanding of the meaning of the questions cannot, therefore, be excluded. Still, self-reports represent an inexpensive tool that allows the collection of a large amount of data in a relatively short amount of time and, therefore, are a particularly useful approach in assisting the assessment of CR patients in research and in everyday practice.
Conclusion
The present study showed for the first time the independent impact of PWB on exercise intolerance over and above a series of selected physical and social components in a representative sample of patients with obesity and CVD enrolled in a CR program.
Exercise capacity is a key outcome of CR, and these results highlight the importance for healthcare professionals to properly assess the psychosocial status of the patient in order to promptly address potentially modifiable barriers to PA other than the biomedical ones (Baldasseroni et al., 2016; Cattivelli et al., 2018). Indeed, many patients with obesity and CVD fail to meet recommended daily PA levels (Balady and McInnis, 1996; Ghashghaei et al., 2012), and effective personalized actions aimed at increasing long-term behavioral change should be promoted (Pietrabissa et al., 2013, 2015, 2017a,b; Ceccarini et al., 2015).
Maintenance of healthy behaviors (i.e., PA, smoking, alcohol use, diet) would also increase cardiovascular parameters and reduce severity of comorbid illnesses (i.e., obesity, diabetes, etc.), thus preventing these conditions from further affecting the individuals’ exercise tolerance.
Still, longitudinal studies are needed to examine the mediating effect of health behaviors in the relationship between emotional status and the main clinical parameters of cardiac patients–with and without obesity. Findings from this study should be further explored in investigations targeting different populations. In fact, a research gap still exists in understanding the predictors and barriers to PA adherence in samples with varying background, ethnicity, or SES.
Data Availability Statement
The datasets generated for this study are available on request to the corresponding author.
Ethics Statement
The studies involving human participants were reviewed and approved by the Istituto Auxologico Italiano, IRCCS. The patients/participants provided their written informed consent to participate in this study.
Author Contributions
LG conceived the original idea. GP planned and supervised the work, collected the experimental data, and wrote the manuscript with support from GC and RC. GM performed the analysis. EM supervised the findings of this work. All authors discussed the results and contributed to the final manuscript.
Conflict of Interest
The authors declare that the research was conducted in the absence of any commercial or financial relationships that could be construed as a potential conflict of interest.
The handling Editor declared a shared affiliation, though no other collaboration, with several of the authors GP, GC, RC, EM at the time of the review.
References
Ahmadian, H. R., Sclafani, J. J., Emmons, E. E., Morris, M. J., Leclerc, K. M., and Slim, A. M. (2013). Comparison of predicted exercise capacity equations and the effect of actual versus ideal body weight among subjects undergoing cardiopulmonary exercise testing. Cardiol. Res. Pract. 2013:940170. doi: 10.1155/2013/940170
Alizai, P. H., Akkerman, M. K., Kaemmer, D., Ulmer, F., Klink, C. D., Ernst, S., et al. (2015). Presurgical assessment of bariatric patients with the Patient Health Questionnaire (PHQ)–a screening of the prevalence of psychosocial comorbidity. Health Qual. Life Outcomes 13:80. doi: 10.1186/s12955-015-0278-5
Anderson, R. T., Sorlie, P., Backlund, E., Johnson, N., and Kaplan, G. A. (1997). Mortality effects of community socioeconomic status. Epidemiology 8, 42–47.
Andrade, F. C. D., and Mehta, J. D. (2018). Increasing educational inequalities in self-rated health in Brazil, 1998-2013. PLoS One 13:e0196494. doi: 10.1371/journal.pone.0196494
Asthana, A., Piper, M. E., McBride, P. E., Ward, A., Fiore, M. C., Baker, T. B., et al. (2012). Long-term effects of smoking and smoking cessation on exercise stress testing: three-year outcomes from a randomized clinical trial. Am. Heart J. 163, 81–87.e1. doi: 10.1016/j.ahj.2011.06.023
Awotidebe, T. O., Adedoyin, R. A., Yusuf, A. O., Mbada, C. E., Opiyo, R., and Maseko, F. C. (2014). Comparative functional exercise capacity of patients with type 2-diabetes and healthy controls: a case control study. Pan Afr. Med. J. 19:257. doi: 10.11604/pamj.2014.19.257.4798
Baldasseroni, S., Pratesi, A., Francini, S., Pallante, R., Barucci, R., Orso, F., et al. (2016). Cardiac rehabilitation in very old adults: effect of baseline functional capacity on treatment effectiveness. J. Am. Geriatr. Soc. 64, 1640–1645. doi: 10.1111/jgs.14239
Banerjee, A., Newman, D. R., Van den Bruel, A., and Heneghan, C. (2012). Diagnostic accuracy of exercise stress testing for coronary artery disease: a systematic review and meta-analysis of prospective studies. Int. J. Clin. Pract. 66, 477–492. doi: 10.1111/j.1742-1241.2012.02900.x
Benge, W., Litchfield, R. L., and Marcus, M. L. (1980). Exercise capacity in patients with severe left ventricular dysfunction. Circulation 61, 955–959.
Cattivelli, R., Castelnuovo, G., Musetti, A., Varallo, G., Spatola, C. A. M., Riboni, F. V., et al. (2018). ACTonHEALTH study protocol: promoting psychological flexibility with activity tracker and mHealth tools to foster healthful lifestyle for obesity and other chronic health conditions. Trials 19:659. doi: 10.1186/s13063-018-2968-x
Ceccarini, M., Borrello, M., Pietrabissa, G., Manzoni, G. M., and Castelnuovo, G. (2015). Assessing motivation and readiness to change for weight management and control: an in-depth evaluation of three sets of instruments. Front. Psychol. 6:511. doi: 10.3389/fpsyg.2015.00511
Chandrasekhar, R. (2019). Socioeconomic disparities and health outcomes. Am. J. Respir. Crit. Care Med. 199, 807–808. doi: 10.1164/rccm.201811-2073LE
Chiala, O., Vellone, E., Klompstra, L., Ortali, G. A., Stromberg, A., and Jaarsma, T. (2018). Relationships between exercise capacity and anxiety, depression, and cognition in patients with heart failure. Heart Lung 47, 465–470. doi: 10.1016/j.hrtlng.2018.07.010
Choe, Y., Han, J. Y., Choi, I. S., and Park, H. K. (2018). Improvement of exercise capacity in patients with type 2 diabetes mellitus during cardiac rehabilitation. Eur. J. Phys. Rehabil. Med. 56, 981–983. doi: 10.23736/S1973-9087.18.05250-4
Clark, A. M., Hartling, L., Vandermeer, B., and McAlister, F. A. (2005). Meta-analysis: secondary prevention programs for patients with coronary artery disease. Ann. Intern. Med. 143, 659–672. doi: 10.7326/0003-4819-143-9-200511010-00010
Croog, S. H., Levine, S., Testa, M. A., Brown, B., Bulpitt, C. J., Jenkins, C. D., et al. (1986). The effects of antihypertensive therapy on the quality of life. N. Engl. J. Med. 314, 1657–1664. doi: 10.1056/NEJM198606263142602
Demers, C., McKelvie, R. S., Negassa, A., and Yusuf, S. (2001). Reliability, validity, and responsiveness of the six-minute walk test in patients with heart failure. Am. Heart J. 142, 698–703. doi: 10.1067/mhj.2001.118468
Dibben, G. O., Dalal, H. M., Taylor, R. S., Doherty, P., Tang, L. H., and Hillsdon, M. (2018). Cardiac rehabilitation and physical activity: systematic review and meta-analysis. Heart 104, 1394–1402. doi: 10.1136/heartjnl-2017-312832
Dontje, M. L., van der Wal, M. H., Stolk, R. P., Brugemann, J., Jaarsma, T., Wijtvliet, P. E., et al. (2014). Daily physical activity in stable heart failure patients. J. Cardiovasc. Nurs. 29, 218–226. doi: 10.1097/JCN.0b013e318283ba14
Faulconbridge, L. F., Wadden, T. A., Rubin, R. R., Wing, R. R., Walkup, M. P., Fabricatore, A. N., et al. (2012). One-year changes in symptoms of depression and weight in overweight/obese individuals with type 2 diabetes in the look AHEAD study. Obesity (Silver Spring) 20, 783–793. doi: 10.1038/oby.2011.315
Favoccia, C., Pietrabissa, G., Castelnuovo, G., Manzoni, G. M., Montano, M., Bertone, G., et al. (2014). “Psychosocial Risk Factors in Cardiovascular Disease,” in Myocardial Infarctions: Risk Factors, Emergency Management and Long-Term Health Outcomes, ed. P. Wilkonson, Hauppauge, NY: Nova Science Publishers.
Ghashghaei, F. E., Sadeghi, M., Rabiei, K., Roohafza, H., and Sarrafzadegan, N. (2012). Gender differences in risk factors of obese patients after cardiac rehabilitation program. Iran. J. Nurs. Midwifery Res. 17, 381–385.
Gondoni, L. A., Liuzzi, A., Titon, A. M., Taronna, O., Nibbio, F., Ferrari, P., et al. (2006). A simple tool to predict exercise capacity of obese patients with ischaemic heart disease. Heart 92, 899–904. doi: 10.1136/hrt.2005.064758
Gondoni, L. A., Nibbio, F., Caetani, G., Augello, G., and Titon, A. M. (2010). What are we measuring? Considerations on subjective ratings of perceived exertion in obese patients for exercise prescription in cardiac rehabilitation programs. Int. J. Cardiol. 140, 236–238. doi: 10.1016/j.ijcard.2008.11.068
Gong, S. J., Li, L., Yan, J., Ning, G. M., Yang, G. Q., and Ren, Y. Z. (2013). [A correlation study between body mass index and exercise capacity in patients with chronic systolic heart failure]. Zhonghua Nei Ke Za Zhi 52, 181–184.
González-Castro, T. B., Escobar-Chan, Y. M., Fresan, A., López-Narváez, M. L., Tovilla-Zárate, C. A., Juárez-Rojop, I. E., et al. (2019). Higher risk of depression in individuals with type 2 diabetes and obesity: results of a meta-analysis. J. Health Psychol. doi: 10.1177/1359105319876326
Grossi, E., Groth, N., Mosconi, P., Cerutti, R., Pace, F., Compare, A., et al. (2006). Development and validation of the short version of the psychological general well-being index (PGWB-S). Health Qual. Life Outcomes 4:88. doi: 10.1186/1477-7525-4-88
Hair, J. F., Black, W. C., Babin, B. J., Anderson, R. E., and Tatham, R. L. (2010). Multivariate Data Analysis, 7th Edn. New York, NY: Pearson.
Holmgren, M., de Munter, J., Rasmussen, F., Sandberg, M., and Ahlström, G. (2017). Is obesity more than a double burden among people with mobility disability? the effect of obesity on HRQoL and participation in society. Healthcare 5:E79. doi: 10.3390/healthcare5040079
Jing, X., Chen, J., Dong, Y., Han, D., Zhao, H., Wang, X., et al. (2018). Related factors of quality of life of type 2 diabetes patients: a systematic review and meta-analysis. Health Qual. Life Outcomes 16:189. doi: 10.1186/s12955-018-1021-9
Kavanagh, A., Bentley, R. J., Turrell, G., Shaw, J., Dunstan, D., and Subramanian, S. V. (2010). Socioeconomic position, gender, health behaviours and biomarkers of cardiovascular disease and diabetes. Soc. Sci. Med. 71, 1150–1160. doi: 10.1016/j.socscimed.2010.05.038
Kim, H. J., Joo, M. C., Noh, S. E., and Kim, J. H. (2015). Long-term outcomes of cardiac rehabilitation in diabetic and non-diabetic patients with myocardial infarction. Ann. Rehabil. Med. 39, 853–862. doi: 10.5535/arm.2015.39.6.853
Kluytmans, A., van de Schoot, R., Mulder, J., and Hoijtink, H. (2012). Illustrating bayesian evaluation of informative hypotheses for regression models. Front. Psychol. 3:2. doi: 10.3389/fpsyg.2012.00002
Lantz, P. M., Lynch, J. W., House, J. S., Lepkowski, J. M., Mero, R. P., Musick, M. A., et al. (2001). Socioeconomic disparities in health change in a longitudinal study of US adults: the role of health-risk behaviors. Soc. Sci. Med. 53, 29–40. doi: 10.1016/s0277-9536(00)00319-1
McKee, P. A., Castelli, W. P., McNamara, P. M., and Kannel, W. B. (1971). The natural history of congestive heart failure: the Framingham study. N. Engl. J. Med. 285, 1441–1446. doi: 10.1056/NEJM197112232852601
Mesquita, R., Goncalves, C. G., Hayashi, D., Costa Vde, S., Teixeira Dde, C., de Freitas, E. R., et al. (2015). Smoking status and its relationship with exercise capacity, physical activity in daily life and quality of life in physically independent, elderly individuals. Physiotherapy 101, 55–61. doi: 10.1016/j.physio.2014.04.008
Moreno, M., and Pearson, T. A. (2011). The quality of lifestyle and the quality of life. Arch. Intern. Med. 171, 1819–1820. doi: 10.1001/archinternmed.2011.442
Mulder, J., Klugkist, I., Van de Schoot, R., Selfhout, M., and Hoijtink, H. (2009). Bayesian model selection of informative hypotheses for repeated measurements. J. Math. Psychol. 53, 530–546. doi: 10.1016/j.jmp.2009.09.003
Osbak, P. S., Mourier, M., Henriksen, J. H., Kofoed, K. F., and Jensen, G. B. (2012). Effect of physical exercise training on muscle strength and body composition, and their association with functional capacity and quality of life in patients with atrial fibrillation: a randomized controlled trial. J. Rehabil. Med. 44, 975–979. doi: 10.2340/16501977-1039
Pampel, F. C., Krueger, P. M., and Denney, J. T. (2010). Socioeconomic disparities in health behaviors. Annu. Rev. Sociol. 36, 349–370. doi: 10.1146/annurev.soc.012809.102529
Pietrabissa, G., Ceccarini, M., Borrello, M., Manzoni, G. M., Titon, A., Nibbio, F., et al. (2015). Enhancing behavioral change with motivational interviewing: a case study in a cardiac rehabilitation unit. Front. Psychol. 6:298. doi: 10.3389/fpsyg.2015.00298
Pietrabissa, G., Manzoni, G. M., and Castelnuovo, G. (2013). Motivation in psychocardiological rehabilitation. a book review on how motivation affects cardiovascular response: mechanisms and applications. Front. Psychol. 4:827. doi: 10.3389/fpsyg.2013.00827
Pietrabissa, G., Manzoni, G. M., Corti, S., Vegliante, N., Molinari, E., and Castelnuovo, G. (2012). Addressing motivation in globesity treatment: a new challenge for clinical psychology. Front. Psychol. 3:317. doi: 10.3389/fpsyg.2012.00317
Pietrabissa, G., Manzoni, G. M., Rossi, A., and Castelnuovo, G. (2017a). The MOTIV-HEART study: a prospective, randomized, single-blind pilot study of brief strategic therapy and motivational interviewing among cardiac rehabilitation patients. Front. Psychol. 8:83. doi: 10.3389/fpsyg.2017.00083
Pietrabissa, G., Sorgente, A., Rossi, A., Simpson, S., Riva, G., Manzoni, G. M., et al. (2017b). Stages of change in obesity and weight management: factorial structure of the Italian version of the university of rhode island change assessment scale. Eat. Weight Disord. 22, 361–367. doi: 10.1007/s40519-016-0289-1
Pollock, K. M. (2001). Exercise in treating depression: broadening the psychotherapist’s role. J. Clin. Psychol. 57, 1289–1300. doi: 10.1002/jclp.1097
Prag, P., and Subramanian, S. V. (2017). Educational inequalities in self-rated health across US states and European countries. Int. J. Public Health 62, 709–716. doi: 10.1007/s00038-017-0981-6
Roth, G. A., Johnson, C., Abajobir, A., Abd-Allah, F., Abera, S. F., Abyu, G., et al. (2017). Global, regional, and national burden of Cardiovascular Diseases for 10 Causes, 1990 to 2015. J. Am. Coll. Cardiol. 70, 1–25. doi: 10.1016/j.jacc.2017.04.052
Sacre, J. W., Wong, C., Chan, Y. K., Carrington, M. J., Stewart, S., and Kingwell, B. A. (2018). Left ventricular dysfunction and exercise capacity trajectory: implications for subclinical heart failure staging criteria. JACC Cardiovasc. Imaging. 12, 798–806. doi: 10.1016/j.jcmg.2017.10.023
Shishehbor, M. H., Litaker, D., Pothier, C. E., and Lauer, M. S. (2006). Association of socioeconomic status with functional capacity, heart rate recovery, and all-cause mortality. JAMA 295, 784–792. doi: 10.1001/jama.295.7.784
Stringhini, S., Sabia, S., Shipley, M., Brunner, E., Nabi, H., Kivimaki, M., et al. (2010). Association of socioeconomic position with health behaviors and mortality. JAMA 303, 1159–1166. doi: 10.1001/jama.2010.297
Stryker, L. S. (2016). Modifying risk factors: strategies that work diabetes mellitus. J. Arthroplasty 31, 1625–1627. doi: 10.1016/j.arth.2016.02.084
Thapa, S., Pyakurel, P., Baral, D. D., and Jha, N. (2019). Health-related quality of life among people living with type 2 diabetes: a community based cross-sectional study in rural Nepal. BMC Public Health 19:1171. doi: 10.1186/s12889-019-7506-6
Thompson, P. D., Buchner, D., Pina, I. L., Balady, G. J., Williams, M. A., Marcus, B. H., et al. (2003). Exercise and physical activity in the prevention and treatment of atherosclerotic cardiovascular disease: a statement from the council on clinical cardiology (Subcommittee on Exercise, Rehabilitation, and Prevention) and the council on nutrition, physical activity, and metabolism (Subcommittee on Physical Activity). Circulation 107, 3109–3116. doi: 10.1161/01.CIR.0000075572.40158.77
Tonetto, I. F. A., Baptista, M. H. B., Gomides, D. D. S., and Pace, A. E. (2019). Quality of life of people with diabetes mellitus. Rev. Esc. Enferm. USP 53:e03424. doi: 10.1590/S1980-220X2018002803424
Unverdorben, M., van der Bijl, A., Potgieter, L., Venter, C., Munjal, S., Qiwei, L., et al. (2008). Effects of different levels of cigarette smoke exposure on prognostic heart rate and rate–pressure-product parameters. J. Cardiovasc. Pharmacol. Ther. 13, 175–182. doi: 10.1177/1074248408321571
Verges, B., Patois-Verges, B., Cohen, M., Lucas, B., Galland-Jos, C., and Casillas, J. M. (2004). Effects of cardiac rehabilitation on exercise capacity in Type 2 diabetic patients with coronary artery disease. Diabet. Med. 21, 889–895. doi: 10.1111/j.1464-5491.2004.01262.x
von Roeder, M., Rommel, K. P., Kowallick, J. T., Blazek, S., Besler, C., Fengler, K., et al. (2017). Influence of left atrial function on exercise capacity and left ventricular function in patients with heart failure and preserved ejection fraction. Circ. Cardiovasc. Imaging 10:e005467. doi: 10.1161/CIRCIMAGING.116.005467
Vonneilich, N., Ludecke, D., and von dem Knesebeck, O. (2019). ∗Educational inequalities in self-rated health and social relationships - analyses based on the European Social Survey 2002-2016. Soc. Sci. Med. 112379. doi: 10.1016/j.socscimed.2019.112379
Wang, J. S. (2018). Effect of exercise training on exercise capacity and quality of life in patients with heart failure. Int. J. Cardiol. 261, 144–145. doi: 10.1016/j.ijcard.2018.03.065
Wing, R. R., and Hill, J. O. (2001). Successful weight loss maintenance. Annu. Rev. Nutr. 21, 323–341. doi: 10.1146/annurev.nutr.21.1.323
Witham, M. D., Argo, I. S., Johnston, D. W., Struthers, A. D., and McMurdo, M. E. (2006). Predictors of exercise capacity and everyday activity in older heart failure patients. Eur. J. Heart Fail. 8, 203–207. doi: 10.1016/j.ejheart.2005.03.008
Wong, R. C., and Yeo, T. C. (2010). Left atrial volume is an independent predictor of exercise capacity in patients with isolated left ventricular diastolic dysfunction. Int. J. Cardiol. 144, 425–427. doi: 10.1016/j.ijcard.2009.03.060
Yates, B. C., Pozehl, B., Kupzyk, K., Epstein, C. M., and Deka, P. (2017). Are heart failure and coronary artery bypass surgery patients meeting physical activity guidelines? Rehabil. Nurs. 42, 119–124. doi: 10.1002/rnj.257
Zakeri, R., Borlaug, B. A., McNulty, S. E., Mohammed, S. F., Lewis, G. D., Semigran, M. J., et al. (2014). Impact of atrial fibrillation on exercise capacity in heart failure with preserved ejection fraction: a relax trial ancillary study. Circ. Heart Fail. 7, 123–130. doi: 10.1161/CIRCHEARTFAILURE.113.000568
Keywords: physical activity, exercise capacity, psychological well-being, cardiac rehabilitation, obesity
Citation: Pietrabissa G, Castelnuovo G, Manzoni GM, Cattivelli R, Molinari E and Gondoni LA (2020) Psychological Well-Being as an Independent Predictor of Exercise Capacity in Cardiac Rehabilitation Patients With Obesity. Front. Psychol. 10:2973. doi: 10.3389/fpsyg.2019.02973
Received: 08 July 2019; Accepted: 16 December 2019;
Published: 28 January 2020.
Edited by:
Daniela Villani, Catholic University of the Sacred Heart, ItalyReviewed by:
Volker Max Perlitz, Simplana GmbH, GermanyAntonio Simone Laganà, University of Insubria, Italy
Copyright © 2020 Pietrabissa, Castelnuovo, Manzoni, Cattivelli, Molinari and Gondoni. This is an open-access article distributed under the terms of the Creative Commons Attribution License (CC BY). The use, distribution or reproduction in other forums is permitted, provided the original author(s) and the copyright owner(s) are credited and that the original publication in this journal is cited, in accordance with accepted academic practice. No use, distribution or reproduction is permitted which does not comply with these terms.
*Correspondence: Giada Pietrabissa, Z2lhZGEucGlldHJhYmlzc2FAdW5pY2F0dC5pdA==