- 1Department for General Physiology and Biophysics, University of Belgrade, Belgrade, Serbia
- 2Instituto de Tecnología del Conocimiento, Complutense University of Madrid, Madrid, Spain
Introduction
The exact neurophysiological mechanisms of repetitive transcranial magnetic stimulation (rTMS) and transcranial direct current stimulation (tDCS) for treating patients diagnosed with depression are still not clear. Results of previous structural and functional MRI studies showed an aberated functional connectivity in major depressive disorder (MDD) (Vederine et al., 2011; de Kwaasteniet et al., 2013). Those, as well as several connectivity studies (Bluhm et al., 2009; Berman et al., 2011; Zhang et al., 2011; Kim et al., 2013; Chen et al., 2015) seem to support the hypothesis that aberrant functional connectivity within fronto-limbic system underlies the pathophysiology of depression. It should be noted that antidepressant application of both rTMS and tDCS is based on previous findings that these two methods help in the case of hypoactivity of the left dorsolateral prefrontal cortex (DLPFC) (Grimm et al., 2006). Those structural and functional differences probably introduce abnormal physiological complexity demonstrated in electroencephalographic (EEG) (Ahmadlou et al., 2012; Bachmann et al., 2013; Hosseinifard et al., 2014; De la Torre-Luque and Bornas, 2017; Jaworska et al., 2018; Lebiecka et al., 2018) as well as in electrocardiographic (ECG) signals in depression (Migliorinni et al., 2012; Rossi et al., 2016; Iseger et al., 2019).
TDCS is low-intensity modality of transcranial electrical stimulation (TES) which induces very mild sensations in the skin (Stagg and Nitsche, 2011). Much later developed TMS primarily uses a strong magnetic field to induce an electric field in the cortex painlessly, initiating optimally focused activation of neural structures (Barker et al., 1985). Some of its modalities used in psychiatry are repetitive TMS (rTMS) and intermittent theta burst TMS (iTBS). In the present abundant literature about both rTMS and tDCS, there is scarce evidence of why these two techniques are capable of ameliorating depressive symptoms. We still don't know what precise mechanisms behind them are. Only a fraction of published research (Amassian et al., 1989; Maccabee et al., 1990; Wassermann and Grafman, 2005; Miranda et al., 2009; Ilmoniemi and Kičić, 2010; Alam et al., 2016) describe the theoretical background of those mechanisms from electromagnetics/physics point of view. The majority of published studies are based on multi-centric comparisons of clinical efficiency (Brunoni et al., 2016; Antal et al., 2017; Mutz et al., 2018) and computational methods-or simulations (Miranda et al., 2001, 2006; Wagner et al., 2007; Huang et al., 2017). Recently, a team of leading researchers in low intensity electrical transcranial stimulation reviewed clinical outcomes for 8,000 people (Antal et al., 2017) confirming its safety and effectiveness, and defined the regulatory and application guidelines for future research.
A term “non-invasive” (attached to both rTMS and tDCS) stems from obsolete medical point of view that the stimulating electrodes do not enter the crania (and the stimulation is performed either via small electrical charges in case of tDCS or via Faraday's induction). The real effect of “non-invasive” electromagnetic stimulation (rTMS and tDCS) cannot be measured directly due to their non-invasive nature. Opitz stated in recent research, that the important point is in interpretability of stimulation effects (Opitz et al., 2015): “if electric fields are delivered inconsistently, but effects are observed nevertheless, the results are more difficult to interpret because effect could be driven by other incidentally affected brain regions.” Both tDCS and TMS are shown to initiate these “unintended” effects: Bestmann showed using MRI that TMS of motor cortex below the threshold power can activate some other deeper structures, contrary to previous belief and Li showed similar phenomena in the case of tDCS (Bestmann et al., 2003, 2004; Li et al., 2018).
The hypothesis here is that both non-invasive electromagnetic modalities of brain stimulation, rTMS and tDCS, are efficient in depression treatments because of their proven ability to decrease the physiological complexity (Čukić et al., 2013, 2019a; Lebiecka et al., 2018; Zuchowicz et al., 2019). The hallmark of MDD is elevated physiological complexity of EEG measured by various entropy measures, fractal dimension, symbolic dynamic approach measures, geometric techniques like recurrence plots and other measures stemming from complex systems dynamics theory (De la Torre-Luque and Bornas, 2017). There are also findings that link changes in heart rhythm complexity with depression (Migliorinni et al., 2012) and the outcomes of rTMS treatment(Royster et al., 2012; Lebiecka et al., 2018; Iseger et al., 2019).
The evidence supporting the close relationship between the electrophysiological complexity, depressive symptoms, and rTMS and tDCS treatment is sufficient but veiled. First, in our 2011 study we showed that even a single pulse transcranial magnetic stimulation (spTMS) can decrease the complexity of electrophysiological signal (Čukić et al., 2012, 2013). Second, Mutanen et al. (2013) used Global Recurrence analysis on concurrently recorded EEG to show that TMS is capable of inducing a “brain-shift” after the stimulation., that is moving the system of brain networks to higher-energy less-probable state in healthy controls. Based on this work we applied the same method but with tDCS (Čukić et al., 2018b, 2019a,b). Čukić et al. (2018b) showed for the first time the graphical representations of tDCS-induced “brain-shift” obtained by principal component analysis (PCA) applied on raw EEG signal samples. PCA was used in our data mining projects to check for separability of data for later classification. This study re-used EEG signals from 16 healthy controls recorded during cathodal and anodal tDCS stimulation protocols from Pelliciari et al. (2013) (which is also elaborating on the difference between cathodal and anodal stimulation). Obtained PCA plots are showing that more than a half an hour post stimulation the system is still in higher-energy lower-probable state “brain-shift” due to the tDCS stimulation. The first three principal components of raw EEG samples before and after tDCS stimulation are illustrating that they belong to separate parts of the phase space. One of participants PCA plot after cathodal stimulation is shown in Figure 1.
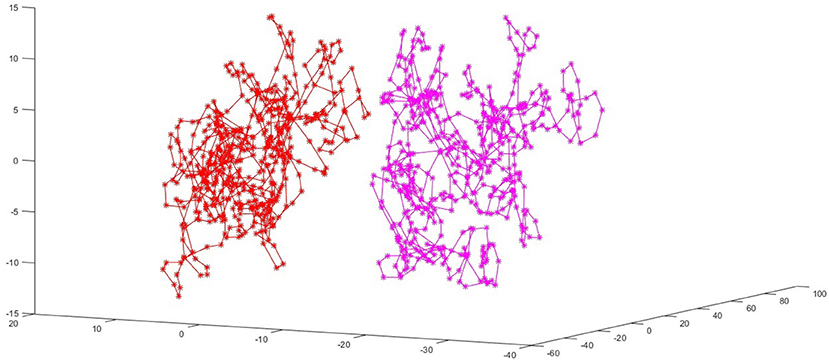
Figure 1. The red voltage samples are taken from the EEG recording before the stimulation, and pink ones from 32 min after the tDCS stimulation. The first three principal components of raw EEG samples before and after tDCS stimulation are illustrating that they belong to separate parts of the phase space. Here is a PCA plot for person number 8, with cathodal (C) stimulation. This figure is part of results published in Chapter 3 in book (Čukić Radenković, 2019), but this particular PCA plot is not displayed before (due to limited space in previous publication).
Several researchers who used various non-linear measures of complexity of EEG confirmed that physiological complexity is elevated in MDD (Ahmadlou et al., 2012; Bachmann et al., 2013, 2015, 2018; Faust et al., 2014; Hosseinifard et al., 2014; Akar et al., 2015; Čukić et al., 2018a, 2019a; Lebiecka et al., 2018). One of the most inclusive review studies on various spectral, fractal and other non-linear measures of relationship between physiological complexity and MDD, concluded that EEG signals in MDD are “probably more random than more complex” compared to those of healthy persons (De la Torre-Luque and Bornas, 2017). This might be due to impaired intrinsic feedback mechanisms important for many regulatory functions (Goldberger et al., 2002). This kind of abnormal functional connectivity is reported in several research papers from seemingly unrelated disciplines, like graph theory application in EEG connectomics (Lee et al., 2011; Van Essen et al., 2012; Castellanos et al., 2013; Kim et al., 2013), and Granger causality applied on fMRI signals (Hamilton et al., 2011). The fMRI and Fractional anisotropy (FA) research also found that within fronto-lymbic system there is abnormal functional connectivity in MDD (Vederine et al., 2011; de Kwaasteniet et al., 2013). De Kwaasteniet found that uncinate fasciculus, important for connecting prefrontal with limbic system, is not fully functional in MDD patients (de Kwaasteniet et al., 2013). Moreover, several studies examining connectivity in MDD found a different dynamical features, and several different regions (anterior cingulate cortex, insula, cingulate and hippocampal network) were confirmed as candidates for these differences (Mayberg, 1997; Mayberg et al., 1997, 1999; Bluhm et al., 2009; Berman et al., 2011; Ge et al., 2019). It is challenging to compare these findings since their methodological approaches are different in so many aspects. Also, Mendez et al. (2012) detected a higher focus on local connections than on global ones in MDD. This can also be seen in persons with depression in remission: previously detected abnormal functional connectivity decreases (Mendez et al., 2012). Lebiecka et al. (2018) showed that elevated physiological complexities diminished after treatment in those MDD patients that reacted well on rTMS (as measured by the decrease in complexity corresponding to remission scores after the treatment was measured) (see also Jaworska et al., 2018). Iseger et al. (2019) also revealed the connection between successful iTBS applied to the DLPFC and modulation of autonomic nervous system (Iseger et al., 2019).
Bestmann et al. (2004) demonstrated that with TMS application below the motor threshold power, MRI can detect a response from areas that were not intended to be stimulated (Bestmann et al., 2004). Li et al. (2018) were the first research group to demonstrate that tDCS can activate some structures within DMN. Opitz et al. (2015) conclude in their work that even the conductivity constants (dielectric constants for tissue types) used for calculating the effect of stimulation, or simulation, are not adequate for describing the much more demanding reality. Opitz's team detected both higher and lower actual values measured directly (with the array of implanted electrodes in patients that were candidates for surgical intervention on epileptic foci) than those predicted with standard simulation procedures for TES (Opitz et al., 2015, 2018). The effect of a stimulation can depend on the geometrical shape of the surface of sulci, which cannot be monitored during the use of a non-invasive procedure, and that also can lead to major miss-predictions (Čukić, 2006; Čukić et al., 2009; Saturnino et al., 2015; Alekseichuk et al., 2018; Opitz et al., 2018).
Although it can seem impossible to compare the two non-invasive brain stimulation techniques that are so different in the sense of their electromagnetic properties and the level of power they can induce in the living tissue, we can still recognize the same functional pattern. In many review papers exploring the efficiency of both rTMS and tDCS in clinical applications (Brunoni et al., 2016; Antal et al., 2017; Mutz et al., 2018), the conclusions are in line: they are effective, and tDCS can be applied even in primary care, but also as a maintenance treatment for already successful rTMS (Mutz et al., 2018). In a study examining the effect of electroconvulsive therapy, it is demonstrated that multiscale entropy is changed after the treatment (Okazaki et al., 2013), pointing again at the link between complexity changes and the effective treatment for depression. Zuchowicz et al. (2019) reported on detected synchronization of EEG as a feature of successful rTMS which is pointing at reduction of complexity, too.
For all electromagnetic stimulation treatments, the effect is of temporary nature. The rationale is that they can at least ameliorate the symptoms for a limited time; after which they need to be repeated. The common advantage of non-invasive brain stimulation techniques over medications is that there are no foreseeable harmful side-effects (Antal et al., 2017).
Although study of physiological complexity changes is still in the realm of research and mainly not in use in clinical setting, it is expected that soon clinicians would start using varying electromagnetic modalities of stimulation with better understanding of how they work—as means to decrease complexity characteristic of depression. Further research based on empirical data is necessary before making the final conclusion that non-invasive brain stimulation treatments may work through changing physiological complexity.
Conclusion
To conclude, after all above mentioned results of various lines of research that tried to bring us closer to understanding various aberrations of depression, both rTMS and tDCS might be efficient because of their ability to decrease characteristically elevated levels of physiological complexity in depression.
Author Contributions
MČ conceived the idea about the article, performed a literature research and wrote entire text.
Conflict of Interest
The author declares that the research was conducted in the absence of any commercial or financial relationships that could be construed as a potential conflict of interest.
Acknowledgments
MČ thankful to colleagues Prof. Carlo Miniussi, Debora Brignani Ph.D., and Maria Concceta Pelliciari Ph.D. for sharing their data with me and for valuable discussions, and also to Prof. Danka Savić for valuable advice on imrpoving the text of this manuscript. Part of this work is supported by RISEWISE (H2020-MSCA-RISE-2015-690874).
References
Ahmadlou, M., Adeli, H., and Adeli, A. (2012). Fractality analysis of frontal brain in major depressive disorder. Int. J. Psychophysiol. 85, 206–211. doi: 10.1016/j.ijpsycho.2012.05.001
Akar, S. A., Kara, S., Agambayev, S., and Bilgic, V. (2015). “Nonlinear analysis of EEG in major depression with fractal dimensions,” in 2015 37th Annual International Conference of the IEEE Engineering in Medicine and Biology Society (EMBC) (IEEE).
Alam, M., Truong, D. Q., Khadka, N., and Bikson, M. (2016). Spatial and polarity precision of concentric high-definition transcranial direct current stimulation (HD-tDCS). Phys. Med. Biol. 61, 4506–4521. doi: 10.1088/0031-9155/61/12/4506
Alekseichuk, I., Mantell, K., Shirinpour, S., and Opitz, A. (2018). Comparative modeling of transcranial magnetic and electric stimulation in mouse, monkey, and human. bioRxiv. [Preprint]. doi: 10.1101/442426
Amassian, V. E., Cracco, R. Q., and Maccabee, P. J. (1989). Focal stimulation of human cerebral cortex with the magnetic coil: a comparison with electrical stimulation. Electroencephalogr. Clin Neurophysiol. 74, 401–416. doi: 10.1016/0168-5597(89)90029-4
Antal, A., Alekseichuk, I., Bikson, M., Brockmöller, J., Brunoni, A. R., Chen, R., et al. (2017). Low intensity transcranial electric stimulation: safety, ethical, legal regulatory and application guidelines. Clin. Neurophysiol. 128, 1774–1809. doi: 10.1016/j.clinph.2017.06.001
Bachmann, M., Kalev, K., Suhhova, A, Lass, J, and Hinrikus, H. (2015). “Lempel Ziv complexity of EEG in depression,” in IFMBE Proceedings. doi: 10.1007/978-3-319-11128-5_15
Bachmann, M., Lass, J., Suhhova, A., and Hinrikus, H. (2013). Spectral asymmetry and Higuchi's fractal dimension measures of depression electroencephalogram. Comput. Math. Methods Med. 2013, 1–8. doi: 10.1155/2013/251638
Bachmann, M., Päeske, L., Kalev, K., Aarma, K., Lehtmets, A., Ööpik, P., et al. (2018). Methods for classifying depression in single channel EEG using linear and nonlinear signal analysis. Comput. Methods Prog. Biomed. 155, 11–17.
Barker, A. T., Jalinous, R., and Freeston, I. L. (1985). Non-invasive magnetic stimulation of human motor cortex. Lancet 325, 1106–1107. doi: 10.1016/S0140-6736(85)92413-4
Berman, M. G., Peltier, S., Nee, D. E., Kross, E., Deldin, P. J., and Jonides, J. (2011). Depression, rumination and the default network. SCAN 6, 548–555. doi: 10.1093/scan/nsq080
Bestmann, S., Baudewig, J., Siebner, H. R., Rothwell, J. C., and Frahm, J. (2003). Subthreshold high-frequency TMS of human primary motor cortex modulates interconnected frontal motor areas as detected by interleaved fMRI-TMS. Neuroimage 20, 1685–1696. doi: 10.1016/j.neuroimage.2003.07.028
Bestmann, S., Baudewig, J., Siebner, H. R., Rothwell, J. C., and Frahm, J. (2004). Functional MRI of the immediate impact of transcranial magnetic stimulation on cortical and subcortical motor circuits. Eur. J. Neurosci. 19, 1950–1962. doi: 10.1111/j.1460-9568.2004.03277.x
Bluhm, R., Williamson, P., Lanius, R., Théberge, J., Densmore, M., Bartha, R., et al. (2009). Resting state default-mode network connectivity in early depression using a seed region-of-interest analysis: decreased connectivity with caudate nucleus. Psychiatr. Clin. Neurosci. 63, 754–761. doi: 10.1111/j.1440-1819.2009.02030.x
Brunoni, A. R., Moffa, A. H., Fregni, F., Palm, U., Padberg, F., Blumberger, D. M., et al. (2016). Transcranial direct current stimulationfor acute major depressive episodes: meta-analysis of individual patient data. Br. J. Psychiatr. 208, 1–10. doi: 10.1192/bjp.bp.115.164715
Castellanos, F. X., Di Martino, A., Craddock, R. C., Mehta, A. D., and Milham, M. P. (2013). Clinical applications of the functional connectome. Neuroimage 80, 527–540. doi: 10.1016/j.neuroimage.2013.04.083
Chen, C., Takahashi, T., Nakagawa, S., Inouea, T., and Kusumia, I. (2015). Reinforcement learning in depression: a review of computational research. Neurosci. Biobehav. Rev. 55, 247–267 doi: 10.1016/j.neubiorev.2015.05.005
Čukić Radenković, M. (2019). Novel Approaches in Treating Major Depressive Disorder (Depression). New York, NY: NOVA Scientific Publishers Ltd.
Čukić, M. (2006). The Influence of configuration and geometric characteristics of induced electrical field induced by transcranial magnetic stimulation on basic physiological parameters of excitability of motor cortex of man (Magisterium thesis). Department for Physiology and Biophysics, University of Belgrade, School of Biology, Belgrade, Serbia.
Čukić, M., Kalauzi, A., Ilić, T., Mišković, M., and Ljubisavljević, M. (2009). The influence of coil-skull distance on transcranial magnetic stimulation motor evoked responses. Exp. Brain Res. 192, 53–60. doi: 10.1007/s00221-008-1552-0
Čukić, M., Oommen, J., Mutavdzić, D., Jorgovanović, V., and Ljubisavljevic, M. (2013). The effect of single-pulse transcranial magnetic stimulation and peripheral nerve stimulation on complexity of EMG signal: fractal analysis. Exp. Brain Res. 228, 97–104. doi: 10.1007/s00221-013-3541-1
Čukić, M., Platiša, M., Ljubisavljević, M., and Kostić, V. (2012). “Complexity changes in TMS induced surface EMG. CCS2012,” in 4th International Interdisciplinary Chaos Symposium on Chaos and Complex Systems, April 29-May 02, 2012 (Antalya).
Čukić, M., Pokrajac, D., Stokić, M., Simić, S., Radivojević, V., and Ljubisavljević, M. (2018a). EEG Machine Learning With Higuchi Fractal Dimension and Sample Entropy as Features for Successful Detection of Depression. Arxive.org/Cornell repository for Statistics/Machine learning. Available online at: https://arxiv.org/abs/1803.05985 (accessed March 15, 2018).
Čukić, M., Stokić, M, Radenković, S., Ljubisavljević, M., and Pokrajac, D. (2019a). The Shift in Brain-State Induced by tDCS: an EEG Study (A Chapter 3 in Book NOVEL Approaches in Treating Major Depressive Disorder. New York, NY: NOVA Scientific Publishers Ltd.
Čukić, M., Stokić, M., Radenković, S., Ljubisavljević, M., and Pokrajac, D. (2018b). The Shift in Brain-State Induced by tDCS: An EEG Study. Cornell Repository, Arxiv.org. Available online at: https://arxiv.org/abs/1812.01342 (accessed December 4, 2018). [Epub ahead of print].
Čukić, M., Stokić, M., Radenković, S., Ljubisavljević, M., Simić, S., and Savić, D. (2019b). Nonlinear analysis of EEG complexity in episode and remission phase of recurrent depression. Int. J. Methods Psychiatr. Res. doi: 10.1002/mpr.1816. [Epub ahead of print].
de Kwaasteniet, B., Ruhe, E., Caan, M., Rive, M., Olabarriaga, S., Groefsema, M., et al. (2013). Relation between structural and functional connectivity in major depressive disorder. Biol. Psychiatr. 74, 40–47. doi: 10.1016/j.biopsych.2012.12.024
De la Torre-Luque, A., and Bornas, X. (2017). Complexity and irregularity in the brain oscillations of depressive patients: a systematic review. Neuropsychiatry 5, 466–477. doi: 10.4172/Neuropsychiatry.1000238
Faust, O., Chuan, P., Ang, A., Puthankattil, S. D., and Joseph, P. K. (2014). Depression diagnosis support system based on EEG signal entropies. J. Mech. Med. Biol. 14:1450035. doi: 10.1142/S0219519414500353
Ge, R., Torres, I., Brown, J. J., Gregory, E., McLellan, E., Downar, J. H., et al. (2019). Functional disconnectivity of the hippocampal network and neural correlates of memory impairment in treatment-resistant depression. J Affect Disord. 253, 248–256. doi: 10.1016/j.jad.2019.04.096
Goldberger, A. L., Peng, C.-K., and Lipsitz, L. A. (2002). What is physiologic complexity and how does it change with aging and disease? Neurobiol. Aging 23, 23–26. doi: 10.1016/S0197-4580(01)00266-4
Grimm, S., Schmidt, C. F., Bermpohl, F., Heinzel, A., Dahlem, Y, Wyss, M., et al. (2006). Segregated neural representation of distinc emotion dimensions in the prefrontal cortex-and fMRI study. Neuroimage 30, 325–340. doi: 10.1016/j.neuroimage.2005.09.006
Hamilton, J. P., Chen, G., Thomason, M. E., Schwartz, M. E., and Gotlib, I. H. (2011). Investigating neural primacy in major depressive disorder: multivariate granger causality analysis of resting-state fMRI time-series data. Mol. Psychiatr. 16, 763–772. doi: 10.1038/mp.2010.46
Hosseinifard, B., Moradi, M. H., and Rostami, R. (2014). Classifying depression patients and normal subjects using machine learning techniques and nonlinear features from EEG signal. Comput. Methods Prog. Biomed. 109, 339–345. doi: 10.1016/j.cmpb.2012.10.008
Huang, Y., Liu, A. A., Lafon, B., Friedman, D., Dayan, M., Wang, X., et al. (2017). Measurements and models of electroc fields in the in vivo human brain during transcranial electric stimulation. eLife 6:e18834. doi: 10.7554/eLife.18834
Ilmoniemi, R., and Kičić, D. (2010). Methodology for combined TMS and EEG. Brain Topogr. 22, 233–248. doi: 10.1007/s10548-009-0123-4
Iseger, T., Arns, M., Downar, J., Blumberger, D. M., Daskalakis, Z. J., and Vila-Rodriguez, F. (2019). Cardiovascular differences between sham and active iTBS related to treatment response in MDD. Brain Stimul. 13, 167–174. doi: 10.1016/j.brs.2019.09.016
Jaworska, N., Wangb, H., Smithc, D. M., Bliera, P., Knotta, V., and Protznerb, A. B. (2018). Pre-treatment EEG signal variability is associated with treatment success in depression. NeuroImage 17, 368−377. doi: 10.1016/j.nicl.2017.10.035
Kim, D., Bolbecker, A. R., Howell, J., Rass, O., Sporns, O., Hetrick, W. P., et al. (2013). Disturbed resting state EEG synchronization in bipolar disorder: a graph-theoretic analysis. NeuroImage Clin. 2, 414–423. doi: 10.1016/j.nicl.2013.03.007
Lebiecka, K., Zuchowicz, U., Wozniak-Kwasniewska, A., Szekely, D., Olejarczyk, E., and David, O. (2018). Complexity analysis of EEG data in persons with depression subjected to transcranial magnetic stimulation. Front Physiol. 9:1385. doi: 10.3389/fphys.2018.01385
Lee, T. W., Wu, Y. T., Yu, Y. W., Chen, M. C., and Chen, T. J. (2011). The implication of functional connectivity strength in predicting treatment response of major depressive disorder: a resting EEG study. Psychiatr. Res. 194, 372–377 doi: 10.1016/j.pscychresns.2011.02.009
Li, L. M., Violante, I. R., Leech, R., Ross, E., Hampshire, A., Opitz, A., et al. (2018). Brain state and polarity dependent modulation of brain networks by transcranial current stimulation. Human Brain Mapp. 40, 904–915. doi: 10.1002/hbm.24420
Maccabee, P. J., Eberle, L., Amassian, V. E., Cracco, R. Q., Rudell, A., and Jayachandra, M. (1990). Spatial distribution of the electric field induced in volume by round and figure ‘8’ magnetic coils: relevance to activation of sensory nerve fibers. Electroencephalogr. Clin. Neurophysiol. 76, 131–141. doi: 10.1016/0013-4694(90)90211-2
Mayberg, H. S. (1997). Limbic-cortical dysregulation: a proposed model of depression. J. Neuropsychiatr. Clin. Neurosci. 9, 471–481. doi: 10.1176/jnp.9.3.471
Mayberg, H. S., Brannan, S. K., Mahurin, R. K., Jerabek, P. A., Brickman, J. S., Tekell, J. L., et al. (1997). Cingulate function in depression: a potential predictor of treatment response. Neuroreport 8, 1057–1061. doi: 10.1097/00001756-199703030-00048
Mayberg, H. S., Liotti, M., Brannan, S. K., McGinnis, S., Mahurin, R. K., Jerabek, P. A., et al. (1999). Reciprocal limbic-cortical function and negative mood: converging PET findings in depression and normal sadness. Am. J. Psychiatr. 156, 675–682.
Mendez, A., Zuluaga, M., Hornero, P., Gomez, R., Escudero, C., Rodriguez-Palancas, J., et al. (2012). Complexity analysis of spontaneous brain activity: effects of depression and antidepressant treatment. J. Psychopharmacol. 26, 636–643. doi: 10.1177/0269881111408966
Migliorinni, M., Mendez, M. O., and Bianchi, A. B. (2012). Study of heartrate variability in bipolar disorder: linear and non-linear parameters during sleep. Front. Neuroeng. 4:22. doi: 10.3389/fneng.2011.00022
Miranda, P. C., Faria, P., and Hallet, M. (2009). What does the ration of injected current to electrode area tell us about current density in the brain during tDCS? Clin. Neurophysiol. (2009) 120, 1183–1187. doi: 10.1016/j.clinph.2009.03.023
Miranda, P. C., Lomarev, M., and Hallet, M. (2006). Modeling the current distribution during transcranial direct current stimulation. Clin. Neurophysiol. 117, 1623–1629. doi: 10.1016/j.clinph.2006.04.009
Miranda, P. C., Pajevic, S., Pierpaoli, C., Hallett, M., and Basser, P. J. (2001). The distribution of currents induced in the brain by magnetic stimulation: a finite element analysis incorporating DT-MRI-derived conductivity data. Proc. Int. Soc. Magn. Reson. Med. 9.
Mutanen, T., Nieminen, J. O., and Ilmoniemi, R. (2013). TMS-evoked changes in brain-state dynamics quantified by using EEG data. Front. Hum. Neurosci. 7:155. doi: 10.3389/fnhum.2013.00155
Mutz, J., Edgcumble, D. R., Brunoni, A. R., and Fu, C. H. Y. (2018). Efficacy and acceptability of non-invasive brain stimulation for the treatment of adult unipolar and bipolar depression: a systematic review and meta-analysis of randomized sham-controlled trials. Neurosci. Biobehav. Rev. 92, 291–303. doi: 10.1016/j.neubiorev.2018.05.015
Okazaki, R., Takahashi, T., Ueno, K., Takahashi, K., Higashima, M., and Wada, Y. (2013). Effects of electroconvulsive therapy on neural complexity in patients with depression: report of three cases. J. Affect Disord. 150, 389–392. doi: 10.1016/j.jad.2013.04.029
Opitz, A., Paulus, W., Will, S., Antunes, A., and Thielscher, A. (2015). Determinants of the electric field during transcranial direct current stimulation. Neuroimage 109, 140–150. doi: 10.1016/j.neuroimage.2015.01.033
Opitz, A., Yeagle, E., Thielscher, A., Schroeder, C., Mehta, A. D, and Milham, M. P. (2018). On the importance of precise electrode placement for targeted transcranial electric stimulation. Neuroimage 181, 560–567. doi: 10.1016/j.neuroimage.2018.07.027
Pelliciari, M. C., Brignani, D., and Miniussi, C. (2013). Excitability modulation of the motor system induced by transcranial direct current stimulation: a multimodal approach. Neuroimage 83, 569–580. doi: 10.1016/j.neuroimage.2013.06.076
Rossi, S., Santarnecchi, E., Valenya, G, and Ulliveli, M. (2016). The heart side of brain neuromodulation. Philos. Trans. A Math. Phys. Eng. Sci. 384:20150187. doi: 10.1098/rsta.2015.0187
Royster, E. B., Trimble, L. M., Cotsonis, G., Schmotzer, B., Manatunga, A., Rushing, N. N., et al. (2012). Changes in heart rate variability of depressed patients after electroconvulsive therapy. Cardiovasc. Psychiatr. Neurol. 2012:794043. doi: 10.1155/2012/794043
Saturnino, G. B., Antunes, A., and Thielscher, A. (2015). On the importance of electrode parameters for shaping electric field patterns generated by tDCS. Neuroimage 120, 25–35. doi: 10.1016/j.neuroimage.2015.06.067
Stagg, C. J., and Nitsche, M. A. (2011). Physiological basis of transcranial direct current stimulation. Neuroscientist 17, 37–53. doi: 10.1177/1073858410386614
Van Essen, D. C., Ugurbil, K., Auerbach, E., Barch, D., Behrens, T. E., Bucholz, R., et al. (2012). The Human Connectome Project: a data acquisition perspective. Neuroimage 62, 2222–2231. doi: 10.1016/j.neuroimage.2012.02.018
Vederine, F. E., Wessa, M., Leboyer, M., and Houenou, J. A. (2011). meta-analysis of whole-brain diffusion tensor imaging studies in bipolar disorder. Prog. Neuropsychopharmacol. Biol. Psychiatr. 35, 1820–1826. doi: 10.1016/j.pnpbp.2011.05.009
Wagner, T., Fregni, F., Fecteau, S., Grodzinsky, A., Zahn, M., and Pascueal-Leone, A. (2007). Transcranial direct current stimulation: a computer-based human model study. NeuroImage 35, 1113–1124. doi: 10.1016/j.neuroimage.2007.01.027
Wassermann, E. M., and Grafman, J. (2005). Recharging the cognition with DC brain polarization. Trends Cogn. Sci. 9, 503–505. doi: 10.1016/j.tics.2005.09.001
Zhang, J., Wang, J., Wu, Q., Kuang, W., Huang, X., He, Y., et al. (2011). Disrupted Brain connectivity networks in drug-naive, first-episode major depressive disorder. Biol. Psychiatr. 70, 334–342. doi: 10.1016/j.biopsych.2011.05.018
Keywords: physiological complexity, rTMS, tDCS, depression, efficiency of treatment, neuromodulation
Citation: Čukić M (2020) The Reason Why rTMS and tDCS Are Efficient in Treatments of Depression. Front. Psychol. 10:2923. doi: 10.3389/fpsyg.2019.02923
Received: 03 October 2019; Accepted: 10 December 2019;
Published: 13 January 2020.
Edited by:
Qing Zhao, Second Affiliated Hospital of Guangzhou Medical University, ChinaReviewed by:
Raquel Chacon Ruiz Martinez, Hospital Sírio-Libanês, BrazilCopyright © 2020 Čukić. This is an open-access article distributed under the terms of the Creative Commons Attribution License (CC BY). The use, distribution or reproduction in other forums is permitted, provided the original author(s) and the copyright owner(s) are credited and that the original publication in this journal is cited, in accordance with accepted academic practice. No use, distribution or reproduction is permitted which does not comply with these terms.
*Correspondence: Milena Čukić, bWljdSYjeDAwMDQwOzNlZ2Eubmw=; bWljdWtpYyYjeDAwMDQwO3VjbS5lcw==