- 1Department of Affective Neuroscience and Psychophysiology, Institute for Psychology, University of Goettingen, Goettingen, Germany
- 2Leibniz Science “Primate Cognition”, Goettingen, Germany
The conflicting findings from the few studies conducted with regard to gender differences in the recognition of vocal expressions of emotion have left the exact nature of these differences unclear. Several investigators have argued that a comprehensive understanding of gender differences in vocal emotion recognition can only be achieved by replicating these studies while accounting for influential factors such as stimulus type, gender-balanced samples, number of encoders, decoders, and emotional categories. This study aimed to account for these factors by investigating whether emotion recognition from vocal expressions differs as a function of both listeners' and speakers' gender. A total of N = 290 participants were randomly and equally allocated to two groups. One group listened to words and pseudo-words, while the other group listened to sentences and affect bursts. Participants were asked to categorize the stimuli with respect to the expressed emotions in a fixed-choice response format. Overall, females were more accurate than males when decoding vocal emotions, however, when testing for specific emotions these differences were small in magnitude. Speakers' gender had a significant impact on how listeners' judged emotions from the voice. The group listening to words and pseudo-words had higher identification rates for emotions spoken by male than by female actors, whereas in the group listening to sentences and affect bursts the identification rates were higher when emotions were uttered by female than male actors. The mixed pattern for emotion-specific effects, however, indicates that, in the vocal channel, the reliability of emotion judgments is not systematically influenced by speakers' gender and the related stereotypes of emotional expressivity. Together, these results extend previous findings by showing effects of listeners' and speakers' gender on the recognition of vocal emotions. They stress the importance of distinguishing these factors to explain recognition ability in the processing of emotional prosody.
Introduction
The ability to accurately perceive the emotional states of others is a fundamental socio-cognitive ability for the successful regulation of our interpersonal relationships (Levenson and Ruef, 1992; Fischer and Manstead, 2008) and it relies on the integration of several information cues such as facial expressions, tone of voice (prosody), words or body language (Van den Stock et al., 2007; Jessen and Kotz, 2011). Although there is a consensus among researchers that the recognition of emotions is facilitated by the availability of additional sensory channels (de Gelder and Vroomen, 2000; Paulmann and Pell, 2010; Klasen et al., 2014), it has also been shown that using just one channel (e.g., the voice) is more than sufficient at deciphering a person's emotional state well above chance (Apple and Hecht, 1982; Juslin and Laukka, 2001; Thompson and Balkwill, 2006, 2009; Jürgens et al., 2018).
The voice is a highly complex tool of communication or, as already Darwin (1872/1998) pointed out, the most indicative of an individual's emotional state. Our voice discloses information not only about our biological, psychological or social status (e.g., Azul, 2013) but also expresses emotions using different domains such as prosody, semantics or non-speech sounds (i.e., affect bursts; e.g., Schwartz and Pell, 2012; Kraus, 2017). Several studies have demonstrated that the main and most obvious function of prosody and non-speech sounds is that of facilitating interaction and communication (see for example, Belin, 2006; Hawk et al., 2009; Belin et al., 2011; Paulmann et al., 2012; Pell et al., 2015; Fischer and Price, 2017, for details). One of the methodological challenges when studying prosody in human speech is how to isolate processes related to the encoding (expressing) and decoding (judging) of emotions from those of processing semantic information carried by, for example, words or sentences. To circumvent this problem, researchers used either pseudo-speech or affect bursts (e.g., simulated laughter, crying) as stimulus material. While the former captures the pure effects of emotional prosody independent of lexical-semantic cues, the latter has been argued to have an adaptive value (Fischer and Price, 2017) and to be an ideal tool when investigating the expression of emotional information when there is no concurrent verbal information present (Pell et al., 2015).
In the context of nonverbal communication (e.g., vocal affect, facial expressions, body language), gender has been repeatedly proposed as an important factor that might influence the accuracy of performance in emotion recognition tasks (e.g., Hall, 1978, 2006; Hall et al., 2000; Sokolov et al., 2011; Fischer and Evers, 2013; Thompson and Voyer, 2014; Santos and Osório, 2015). One can distinguish two major lines of research. One line assumes that females and males differ in their emotionality, personality, abilities, attitudes or behavioral tendencies (gender differences hypothesis; Gray, 1992) and that women are “emotional experts”, more inclined to pay attention to their own and others' feelings and intuitions (Hess et al., 2000; Shields, 2002; Timmers et al., 2003). Several studies have shown that both genders differ in the way they express (e.g., Barett and Bliss-Moreau, 2009; Parkins, 2012; McDuff et al., 2017), experience (e.g., Šolcová and Lacev, 2017), and decode or encode emotions with females outperforming males when completing tasks designed to measure non-verbal communication ability (e.g., Zuckerman et al., 1975; Ambady and Rosenthal, 1998; MacAdams, 2012; Wells et al., 2016; Wingenbach et al., 2018). In addition, meta-analytic reviews, summarizing work on gender differences concerning the ability to recognize non-verbal expressions of emotion, also reported a female advantage for emotion recognition tasks with effect sizes ranging from small to medium (e.g., Hall, 1984; McClure, 2000). Explanations for these gender-based behavior patterns range from socio-cultural influences and psychological dispositions to evolutionary perspectives (see Briton and Hall, 1995; Brody, 1997; Eagly and Wood, 1999; Davis et al., 2012; for more detailed explanations). For instance, it has been suggested that females, due to their responsibility for child rearing, are expected to be prosocial and nurturing and, thus, more responsive and accurate in judging other people's emotions (Hall, 1984; Babchuk et al., 1985; Schirmer, 2013).
Conversely, the other line of research has emphasized the homogeneity between genders across various domains (e.g., non-verbal communication, social and personality variables, psychological well-being) based on evidence from meta-analyses. For instance, Richard et al. (2003) examined gender differences across domains by using a second order meta-analysis (see Schmidt and Oh, 2013; Zell and Krizan, 2014, for details) to characterize the average difference between males and females. With regard to nonverbal communication the authors aggregated the data from a series of experiments conducted by Rosenthal and DePaulo (1979) and found that the correlation coefficients between genders were small, ranging from r = 0.16, for facial cues, r = 0.11, for body cues to r = 0.06, for vocal cues. Furthermore, Hyde (2005, 2014) observed 78% of effect sizes to be small or close to zero, leading her to conclude that in many cases females and males are rather similar on most psychological dimensions (gender similarity hypothesis). The results of these meta-analytic reviews are useful for estimating the overall magnitude and variability of female-male comparisons across various domains. However, this line of research might under-interpret the differences between females and males for emotion recognition by failing to consider modality specific effects (Abelson, 1985; Thompson and Voyer, 2014). A comprehensive conclusion cannot be drawn when the vast majority of evidence comes from studies that assess gender effects mainly within only one modality (e.g., Hyde, 2005) or by employing only one test (Profile of Nonverbal Sensitivity, Rosenthal and DePaulo, 1979) to assess performance accuracy for decoding nonverbal cues (e.g., Richard et al., 2003). Thus, until further evidence on the similarities and differences between genders within specific sensory modalities is provided, the direction of these effects remains an open question.
Contrary to the growing field of research examining gender effects in the recognition of emotions within the visual modality, where researchers are working toward improving methodology by either including facial expressions with varying intensity (Wingenbach et al., 2018), dynamically rising expressions (e.g., Recio et al., 2011, 2014), or different stimulus types such as avatars, human faces or icons (Fischer et al., 2018), the investigation of these effects within the vocal domain is still understudied. This paucity persists despite a common consensus that the voice is an important source of social information (e.g., Latinus and Belin, 2011; Morningstar, 2017). Research comparing auditory, visual, and audio-visual modalities reported significant main effects of gender (Scherer and Scherer, 2011), with females outperforming males in all three conditions of stimulus presentation (Collignon et al., 2010). Similarly, Lambrecht et al. (2014) demonstrated a significant female advantage in emotion recognition which was however restricted to vocal emotions. A female advantage was also found in studies investigating emotion recognition purely within the vocal domain (e.g., Scherer et al., 2001; Toivanen et al., 2005; Paulmann and Uskul, 2014; Demenescu et al., 2015). These findings were corroborated by Keshtiari and Kuhlmann (2016), who investigated how gender affects the recognition of vocal expressions of emotion. Participants listened to sentences spoken in five different emotions (angry, disgust, fear, happiness, and sadness) or in a neutral tone of voice and made a decision on the emotional category the presented utterances corresponded to. Results revealed a significant main effect of gender with an overall recognition advantage for females, confirming in this way the consistency of findings in past research. Other studies, however, reported either only a small overall advantage in favor of females in the recognition of non-verbal (auditory, visual, audio-visual) displays of emotion (Kret and de Gelder, 2012; Thompson and Voyer, 2014) or even equal performance accuracy for male and female participants in identifying emotions from both, speech-embedded (e.g., Raithel and Hielscher-Fastabend, 2004; Paulmann et al., 2008; Sauter et al., 2013) and non-speech sounds (e.g., Hawk et al., 2009; Lima et al., 2014).
To address these diverging findings, it has been suggested that instead of examining gender effects across emotions, specific emotion categories should be considered separately (de Gelder, 2016). For instance, in a behavioral study Bonebright et al. (1996) examined participants' ability to decode emotions from vocal cues. They instructed trained actors to record paragraph-long stories, each time using their voice to portray a specified emotion (i.e., anger, fear, happiness, sadness, and neutral). Subsequently, undergraduate students listened to each recorded paragraph and tried to determine which emotion the speaker was trying to portray. Females were significantly more accurate than males in decoding voices that expressed fear, happiness, and sadness. These gender differences were small but consistent. No gender differences were found for emotional expressions uttered in an angry or neutral tone of voice. Subsequent evidence showed that females outperform males for utterances spoken in a fearful (Demenescu et al., 2015; Zupan et al., 2016), happy (Fujisawa and Shinohara, 2011; Lambrecht et al., 2014; Demenescu et al., 2015; Zupan et al., 2016), and sad (Fujisawa and Shinohara, 2011; Zupan et al., 2016) tone of voice. While both genders were found to perform equally well when identifying angry (Fujisawa and Shinohara, 2011; Lambrecht et al., 2014; Demenescu et al., 2015; Zupan et al., 2016), and neutral (Demenescu et al., 2015) prosody, other investigators failed to replicate these findings and found higher accuracy for females in correctly recognizing neutral vocalizations (Lambrecht et al., 2014), or no gender differences in the recognition of sad prosody (Demenescu et al., 2015). That the accuracy of performance varies across discrete emotion categories (e.g., fear, sadness or happiness was argued to play a greater role in women, whereas anger and disgust in men) might be the result of biological or environmental factors, which are likely to trigger “qualitatively” different emotional experiences for men and women (see Schirmer, 2013, for a comprehensive review).
The above-mentioned studies do not show a consistent gender pattern either regarding overall effects in the performance accuracy of decoding vocal emotions or emotion specific categories [see Table 1 (a1) for overall effects in decoding vocal emotions and (a2) for decoding performance accuracy by emotion categories]. There are several likely sources for these inconsistencies. One of the reasons may have been the large variety of different types of vocal stimuli (e.g., words, pseudo-words, sentences, pseudo-sentences, affect bursts). Other methodological differences that might have been responsible for these conflicting results are related either to the number of emotions studied [which vary from two (e.g., Collignon et al., 2010) to nine (e.g., Belin et al., 2008)], the language under investigation (e.g., Scherer et al., 2001; Keshtiari and Kulhmann, 2016), the population in question [children (e.g., Fujisawa and Shinohara, 2011; Sauter et al., 2013), young adults (e.g., Scherer et al., 2001; Paulmann and Uskul, 2014), older adults (e.g., Lima et al., 2014), clinical populations (e.g., Zupan et al., 2016)], unbalanced gender groups [e.g., 71F/50M (Hawk et al., 2009)], and the sample size [which range from 24 (e.g., Raithel and Hielscher-Fastabend, 2004) to 428 (e.g., Scherer et al., 2001)]. The gender of the actor/actress portraying different emotions is a further variable of interest that has been proposed to influence the overall performance accuracy when identifying emotions from the voice (e.g., Scherer et al., 2001). In a validation study concerning the identification of vocal emotions, Belin et al. (2008) tested for differences in performance accuracy based on listeners' as well as speakers' gender. Participants were asked to evaluate actors' vocalizations on three emotional dimensions: valence, arousal, and intensity. Results showed higher mean identification rates (for intensity and arousal dimensions) across all emotion categories when spoken by female actors. Similar to other findings (e.g., Bonebright et al., 1996; Lambrecht et al., 2014), Belin et al. (2008) found no significant interaction between listeners' gender, speakers' gender and emotions, but a significant main effect for listeners' and speakers' gender. These findings indicate that females compared to males were not only better at decoding but also at identifying emotions in the female voice. Considering emotion-specific effects, it has been shown that vocal portrayals of anger and fear have higher mean identification rates when spoken by male actors (Bonebright et al., 1996), whereas happy (Bonebright et al., 1996), and neutral expressions (Young et al., 2017) were better identified from female voices. In contrast, other investigators observed that fear and disgust were better identified when spoken by a female (though a response bias toward disgust when an actor portrayed the emotion and, fear when an actress expressed the emotion was reported; see Collignon et al., 2010, for details). Further research that includes speakers' gender as an additional factor, reports that while gender differences might exist for identifying emotions from speakers' voice, these are not systematic and vary for specific emotions (Pell et al., 2005; Hawk et al., 2009) or occur regardless of the actors' gender (Schirmer and Kotz, 2003; Riviello and Esposito, 2016). Similar to the performance accuracy of decoding emotions, the evidence with regard to speaker's gender as a relevant factor for identifying emotions from the voice is inconsistent [see Table 1 (b1) for overall identification rates by speakers' gender and (b2) for identification rates by speakers' gender and emotion category]. The discrepancies in these findings are likely to be attributable to a number of methodological differences, such as recording conditions (e.g., Burkhardt et al., 2005), number of speakers which vary from 2 (e.g., Demenescu et al., 2015) to 14 (Toivanen et al., 2005) or validity of prosodic stimuli derived from the simulation of emotional expressions (see Hawk et al., 2009; Jürgens et al., 2015, for a discussion whether authentic vs. play acted emotional speech may lower ecological validity).
A seemingly inevitable conclusion after reviewing past work on gender differences in the recognition of vocal expressions of emotion is that conflicting findings have left the exact nature of these differences unclear. Although accuracy scores from some prior studies suggest that females are overall better than males at decoding and encoding vocal emotions, independent of the stimulus type, other studies do not confirm these findings. Likewise, the question whether women are consistently better than men at decoding and identifying emotions such as happiness, fear, sadness or neutral expressions when spoken by a female, while men have an advantage for anger and disgust, remains unresolved. The absence of consistent gender effects for the encoding and decoding of emotional vocal expressions might be a result of the selected stimuli, either speech-embedded (pseudo/words, pseudo/sentences) or non-verbal vocalizations (affect bursts). Thus, it has been suggested that a comprehensive understanding of gender differences in vocal emotion recognition can only be achieved by replicating these studies while accounting for influential factors such as stimulus type, gender-balanced samples, number of encoders, decoders, and emotional categories (Bonebright et al., 1996; Pell, 2002; Lambrecht et al., 2014; Ba̧k, 2016).
To address some of these limitations, the present study aimed at investigating, across a large set of speech-embedded stimuli (i.e., words, pseudo-words, sentences, pseudo-sentences) and non-verbal vocalizations (i.e., affect bursts) whether emotion recognition of vocal expressions differs as a function of both decoders' and encoders' gender and to provide parameter estimates on the magnitude and direction of these effects. To date, no extensive research on differences between males and females in the recognition of emotional prosody has been conducted and, thus, we based our approach for investigating these effects on the patterns observed in the majority of the aforementioned studies. We first examined whether there are any differences in the performance accuracy of decoding vocal emotions based on listeners' gender (i.e., across all stimuli and for each stimulus type; across all emotions and for each emotion category). Specifically, we expected an overall female advantage when decoding vocal emotions, and that they would be more accurate than males when categorizing specific emotions such as happiness, fear, sadness, or neutral expressions. No gender differences were expected to manifest for emotions uttered in an angry and disgusted tone of voice. Secondly, we tested whether there are any differences for identifying vocal emotions based on speakers' gender (i.e., across all stimuli and for each stimulus type; across all emotions and for each emotion category). We hypothesized that vocal portrayals of emotion would have overall significantly higher hit rates when spoken by female than by male actors. Considering emotion-specific effects, we expected that anger and disgust would have higher identification rates when spoken by male actors, whereas portrayals of happiness, fear, sadness, and neutral would be better identified when spoken by female actors. Finally, we investigated potential interactions between listeners' and speakers' gender for the identification of vocal emotions across all stimuli and for each stimulus type.
Methods
The study was conducted in accordance with the ethical principles formulated in the Declaration of Helsinki and approved by the ethics committee of the Georg-Elias-Müller- Institute of Psychology, University of Goettingen, Germany.
Participants
Participants were N = 302 volunteers (age range 18–36) from the University of Goettingen and the local community. They were recruited through flyers distributed at the University campus, the ORSEE database for psychological experiments (http://www.orsee.org/web/), postings on the social media site Facebook and the online platform Schwarzes Brett Goettingen (https://www.uni-goettingen.de/en/644.html). Inclusion criteria for participation in the study were: native speakers of German, aged above eighteen, normal hearing, not currently taking medication affecting the brain and no self-reported mental health problems. Twelve participants who reported hearing disorders (e.g., tinnitus), psychiatric/neurological disorders or the intake of psychotropic medication were not eligible to participate. This left a total of 290 participants (143 female, 147 male) with a mean age of 23.83 years (SD = 3.73). To assess the performance accuracy between females and males within different types of vocal stimuli (i.e., words, pseudo-words, sentences, pseudo-sentences, affect bursts) and to reduce the length of the experiment participants were randomly allocated to two groups of equal size. This allowed us to have a higher number of stimuli in each group resulting in a higher precision of estimated gender or emotion differences within one database and respectively within one of the groups. One group classified words and pseudo-words stimuli (n = 145, Mage = 24.00, SD = 3.67), whereas the other group was presented with stimuli featuring sentences, pseudo-sentences, and affect bursts (n = 145, Mage = 23.66, SD = 3.80). To assess whether there were any age differences in the two groups a Wilcoxon-Mann-Whitney test was conducted. The results indicated a significant age difference between females and males in both groups (GroupWords: z = −2.91, p = 0.004; GroupSentences: z = −2.79, p = 0.005). Participants' demographic characteristics are presented in Table 2.
Throughout the article these two groups will be referred to as Group-Words and Group-Sentences. Participants were reimbursed with course credit or 8 Euros.
Materials and Stimuli Selection
The speech/non-speech embedded stimuli were extracted from well-established and validated databases or provided by researchers who developed their own stimulus materials [see Table 3 for a brief description on the features of the selected databases (e.g., stimuli types, number of speakers)].
To be included in the present study the stimuli had to satisfy the following criteria: (1) be spoken in a neutral tone (i.e., baseline expression) or in one of the emotion categories of interest (i.e., happiness, surprise, anger, fear, sadness, disgust), (2) to be recorded under standardized conditions, (3) to have at least two encoders (i.e., male/female), and (4) to be produced by human expressers.
We decided to use a wide variety of stimuli representing the spectrum of materials used in emotional prosody research (i.e., for speech: words, lexical and neutral sentences; pseudo-speech: pseudo-words/sentences; for non-speech: vocalizations). For economic reasons, only a sub-set of stimuli from each database was selected. For Anna and Montreal Affective Voices (MAV) databases all speakers for the emotion category of interest were chosen. This resulted in a total number of 88 Stimuli for Anna [4 Emotions (anger, happiness, sadness, neutral) × 22 Speakers] and 70 Stimuli for MAV [7 Emotions (anger, disgust, fear, happiness, sadness, surprise, neutral) × 10 Speakers]. The stimuli from the remaining other three databases were ordered randomly and the first 10 items per database were selected. Stimulus selection resulted in a total number of 280 stimuli from the Paulmann Prosodic Stimuli set [10 Pseudo-sentences × 7 Emotions (anger, disgust, fear, happiness, sadness, surprise, and neutral) × 2 Speakers; 10 Lexical Sentences × 7 Emotions (anger, disgust, fear, happiness, sadness, surprise, and neutral) × 2 Speakers], 120 stimuli from the Berlin Database of Emotional Speech [10 Semantic Neutral Sentences × 6 Emotions (anger, disgust, fear, happiness, sadness, and neutral) × 2 Speakers] and 480 Stimuli from the Magdeburg Prosody Corpus [10 Pseudo-words × 6 Emotions (anger, disgust, fear, happiness, sadness, and neutral) × 2 Speakers; 10 Semantic positive nouns/10 Semantic negative nouns/10 Semantic neutral nouns × 6 Emotions (anger, disgust, fear, happiness, sadness, and neutral) × 2 Speakers]. The nouns extracted from the Magdeburg Prosody Corpus were additionally controlled for valence, arousal, and word frequency according to the Berlin Affective Word List Reloaded (Võ et al., 2009).
Acoustic Analysis
The extraction of amplitude (dB), duration, and peak amplitude of all 1,038 original stimuli was conducted using the phonetic-software Praat (Boersma, 2001). As the stimuli used for this study came from different databases with different recording conditions, we controlled for acoustic parameters, including the minimum, maximum, mean, variance, and standard deviation of the amplitude. The results of our analyses indicated that the variation coefficient (CV) for amplitude between the stimuli was high (s2 = 71.92, M = 63.06, CV = 13.45%). Therefore, the stimuli were normalized with regards to loudness by applying the Group Waveform Normalization algorithm of Adobe Audition CC (Version 8.1, Adobe Systems, 2015, San Jose, CA) that uniformly matches the loudness based on the root-mean-square (RMS) levels. To control whether normalization worked, the stimuli were re-uploaded in Praat, which indicated that the variation coefficient between the stimuli was reduced by roughly 40% (s2 = 24.97, M = 61.07, CV = 8.18%) by this procedure.
Physical volume of stimulus presentation across the four PCs' used in the experiment was controlled by measuring sound volume of the practice trials with a professional sound level meter, Nor140 (Norsonic, 2010, Lierskogen, Norway). No significant difference in volume intensity was observed [F(3, 27) = 0.53, p = 0.668].
Procedure
Participants were tested in groups of up to four members. At arrival, each participant was seated in front of a Dell OptiPlex™ 780 Desktop-PC. All participants were provided with individual headphone devices (Bayerdynamic DT 770 PRO). After signing a consent form and completing a short demographic questionnaire concerning age, gender1 and education level, participants were informed that they would be involved in a study evaluating emotional aspects of vocal stimulus materials. Afterwards, they were told to put on headphones and carefully read the instructions presented on the computer screen. Before the main experiment, participants were familiarized with the experimental setting in a short training session comprised of 10 stimuli, which were not presented in the main experiment. They were instructed to carefully listen to the presented stimuli as they would be played only once and that the number of emotions presented might vary form the number of categories given as possible choices (see Design and Randomization for an argument related to this approach). Each trial began with a white fixation-cross presented on a gray screen, which was shown until participants' response had been recorded. The presentation of the stimuli was initiated by pressing the Enter-key. After stimulus presentation, participants had to decide as accurately as possible, in a fixed-choice response format, which of the 7 emotional categories (i.e., anger, disgust, fear, happiness, sadness, surprise, neutral) the emotional prosody of the presented stimulus corresponded to. Following their emotion judgment, they were asked the correctness of their answer on a 7-point Likert scale, where “1” corresponded to not at all confident and “7” corresponded to extremely confident. The responses were made using the marked computer keyboard (Z to M for the emotion judgments, which were labeled corresponding to the emotion categories, and 1 to 7 for confidence). There was no time limit for emotion judgments or confidence ratings. At the end of each block a visual message in the center of the screen instructed participants to take a break if they wished to or to press the Spacebar to proceed with the next block. The 568 stimuli for Group Words had a mean duration of 1.03 ± 0.36 s, whereas in Group Sentences the mean duration of the 470 stimuli was 2.66 ± 1.01 s. Testing took approximately 60 min for both groups.
Design and Randomization
We fitted a balanced design to allow for a separate analysis of effects across the recognizability of emotional expressions, skill in judging emotional expressions, and the interaction between encoding and decoding (the assumptions of such an approach were justified by Elfenbein and Ambady, 2002a,b). Following the argumentation of Wagner (1993), participants were provided with the same number of judgment categories, independent of the given emotion categories within the included databases. This approach guarantees that, the response probabilities are not influenced by the different number of emotional categories (i.e., the probability of correct/false recognition of emotions by random choice is equal).
The set of stimuli for the Group Words was split into three blocks (Anna, Pseudo-words, and Nouns) while the set of stimuli for the Group Sentences was split into four blocks (Pseudo-sentences, Lexical Sentences, Neutral sentences, and Affect bursts). Each block as well as the stimuli within each block were randomized using the software Presentation (Version 14.1, Neurobehavioral Systems Inc., Albany, CA).
Sample Size Calculations
A target sample size of 134 participants per group (67F/67M) was determined using Wilcoxon Mann-Whitney two-tailed test (d = 0.50; α = 0.05; 1–β = 0.80). Assuming 67 participants in each gender group and the minimum number of observations per participant (i.e., 70) we further investigated, via a two-sample binomial test, whether the determined sample size possessed enough statistical power to assess the size of females'/males' differences in detecting vocal emotions. This argument indicated that at 80% recognition probability the sample size was powered enough to detect small differences as 2.3%. To take account of possible attrition the sample size was increased by at least 10%.
Statistical Analysis
The data was analyzed by a generalized linear model (quasi-binomial logistic regression) for the binary response variable emotion recognition. As individual effects (e.g., fatigue, boredom) might impact cognitive performance, we treated participants as a confounder in our model. In addition, we controlled for confidence, as a confounder, shown to impact on performance accuracy in emotion recognition tasks (e.g., Beaupré and Hess, 2006; Rigoulot et al., 2013). Our analysis on the baseline characteristics of the study population indicated a significant age effect between males and females and, therefore, we additionally included this factor as a confounder in our model. Listeners' gender, speakers' gender, emotions and stimulus type were included as predictor variables. Age was included as a quantitative variable. Listeners' gender and speakers' gender were included as binary variables and confidence, participant, emotion, and stimulus type were included as nominal variables. The dispersion parameter of the quasi-binomial model and the nominal variable participants accounted for dependencies caused by repeated measurements within the participants. First order interactions were fitted between listeners' gender and speakers' gender, age and stimuli types, confidence and stimuli types, speakers' gender and stimuli types, listeners' gender and stimuli types, emotions and stimuli types, age and speakers' gender, age and emotions, listeners' gender and emotions, speakers' gender and emotions, confidence and participant. A second order interaction was fitted between listeners' gender, speakers' gender, and emotions. Chi-square tests of the deviance analysis (generalized mixed model analysis) were used to analyze additive and interaction effects.
Means, standard deviations, z-scores, p-values and effect sizes were calculated to describe the differences between genders in performance accuracy. This descriptive analysis was conducted using the unadjusted group means, which allows the application of non-parametric robust methods, direct illustration and interpretation of the effect sizes and patterns. As emotion recognition is binomial distributed and does not allow the assumption of a normal distribution we used Wilcoxon-Mann-Whitney test for independent samples to analyze the effects of listeners' gender and Wilcoxon-rank-sum test for dependent samples to analyze the effects of speakers' gender. Corrections for multiple testing were implemented using Bonferroni's method for multiple comparisons.
The data was analyzed using the R language and environment for statistical computing and graphics version 3.3.1 (R Core Team, 2016) and the integrated environment R-Studio version 1.0.316. The quasi-binomial logistic regression was fitted using the R function glm. Wilcoxon-Mann-Whitney and Wilcoxon-rank-sum were performed with the R package coin introduced by Hothorn et al. (2008).
Results
Emotion Effects on Performance Accuracy
The quasi-binomial logistic models revealed significant interactions between emotions and stimuli types in both groups [Group Words: = 1097.80, p < 0.001; Group Sentences: = 1990.40, p < 0.001]. Main effects of emotion were observed across all stimuli [Group Words: = 4853.80, p < 0.001; Group Sentences: = 6956.00, p < 0.001] and for each stimulus type [Anna: = 2463.87, p < 0.001; pseudo-words: = 1060.19, p < 0.001; semantic positive nouns: = 616.96, p < 0.001; semantic negative nouns: = 735.54, p < 0.001; semantic neutral nouns: = 1603.56, p < 0.001; pseudo-sentences: = 2784.06, p < 0.001; lexical sentences: = 3745.60, p < 0.001; neutral sentences: = 1332.93, p < 0.001; and affect bursts: = 1113.20, p < 0.001]. The full models across all databases and for each stimulus type are presented in Supplementary Material (see Tables S1a,b, S2a–i).
Decoding Performance Accuracy by Listeners' Gender
Significant first order interactions between listeners' gender and stimuli types were observed for both groups [Group Words: = 16.40, p = 0.038; Group Sentences: = 22.80, p < 0.001]. A significant main effect of gender was found across the stimuli types for Group Words [ = 51.70, p < 0.001] but not for Group Sentences [ = 5.20, p = 0.332]. Main effects of gender were revealed for the following stimulus sub-sets: pseudo-words [ = 29.18, p < 0.001], semantic positive nouns [ = 20.14, p < 0.001], semantic negative nouns [ = 8.38, p = 0.046] and semantic neutral nouns [ = 9.13, p = 0.029]. No main effects of gender were found for Anna [ = 0.03, p = 1.00], pseudo-sentences [ = 7.44, p = 0.068], lexical sentences [ = 5.50, p = 0.211], neutral sentences [ = 5.35, p < 0.243] and affect bursts [ = 2.83, p = 0.698].
The quasi-binomial logistic models showed significant first order interactions between listeners' gender and emotions for both groups [Group Words: = 26.60, p < 0.001; Group Sentences: = 19.60, p = 0.029]. When testing the performance accuracy by stimulus type there were no significant interactions between listeners' gender and emotions [Anna: = 7.61, p = 0.644; pseudo-words: = 15.18, p = 1.00; semantic positive nouns: = 6.73, p = 1.00; semantic negative nouns: = 14.96, p = 0.124; semantic neutral nouns: = 3.12, p = 1.00; pseudo-sentences: = 17.36, p = 0.075; lexical sentences: = 9.60, p = 1.00; neutral sentences: = 4.05, p = 1.00; affect bursts: = 5.90, p = 0.848]. Figures 1A,B illustrate the performance accuracy by listeners' gender and emotion categories, separated for both, Group-Words and Group-Sentences.
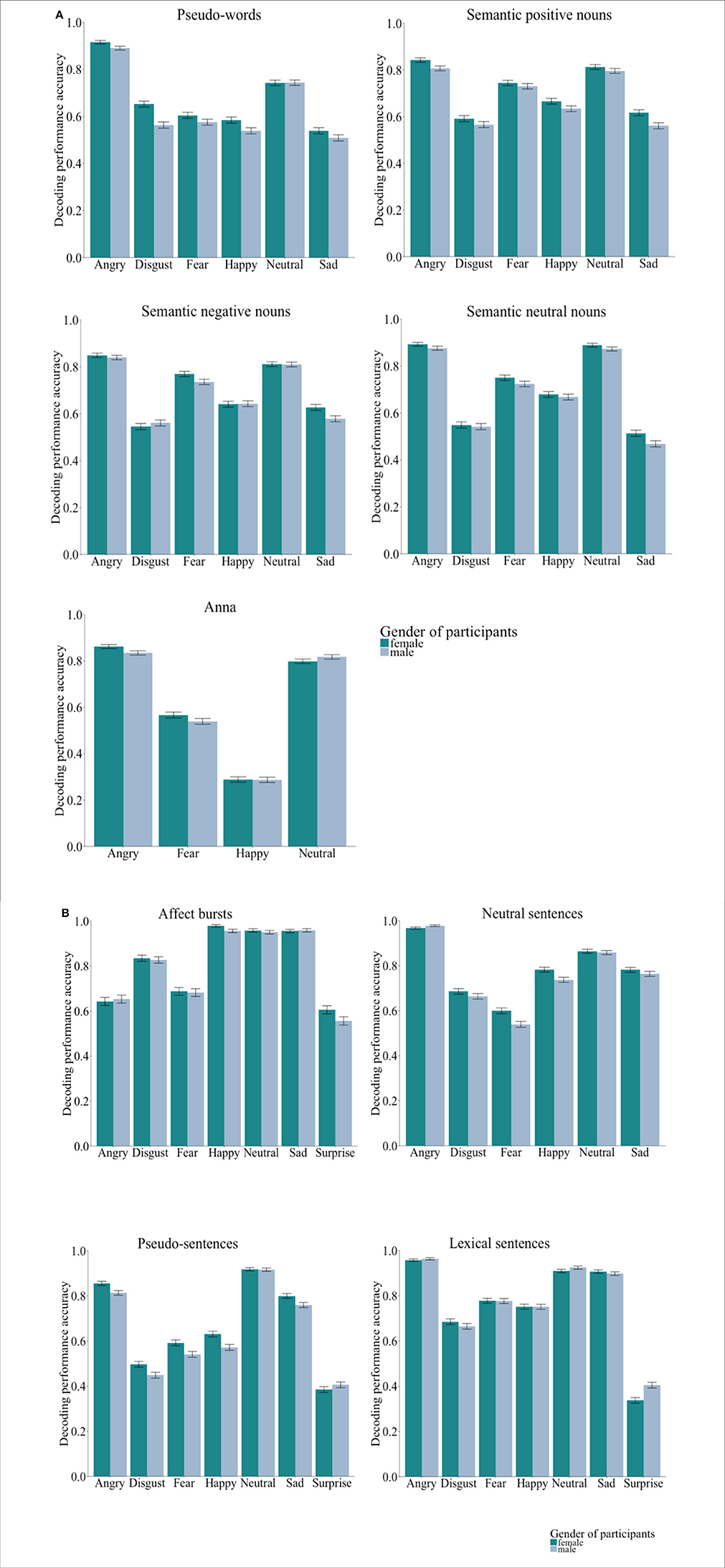
Figure 1. (A) Group words (n = 145, 71 females). Bar charts showing the performance accuracy by listeners' gender. Error bars represent the standard error. As it can be observed, for the majority of emotion categories by databases, females had higher decoding performance accuracy than males. (B) Group sentences (n = 145, 72 females). Bar charts showing the performance accuracy by listeners' gender. Error bars represent the standard error. As it can be observed, for the majority of emotion categories by databases, females had higher decoding performance accuracy than males.
To describe the size of the difference between females and males when decoding emotions, a Wilcoxon Mann-Whitney test was implemented. Results showed that overall females (M = 0.67, SD = 0.06) were significantly better than males (M = 0.64, SD = 0.06) at decoding emotions from pseudo-sentences, z = 2.87, p = 0.033, d = 0.49. No significant differences between females and males by emotion category were observed, with effect sizes close to zero (d ≤ 0.10) or in the small (0.11 < d < 0.35) range. Overall, the results indicated the existence of a small decoding effect favoring females across all emotions (0.15 < d < 0.34) and stimulus types (d = 0.31). The parameter estimates by listeners' gender for each emotion category, across all emotions and stimulus types are presented in Tables 4A,B.
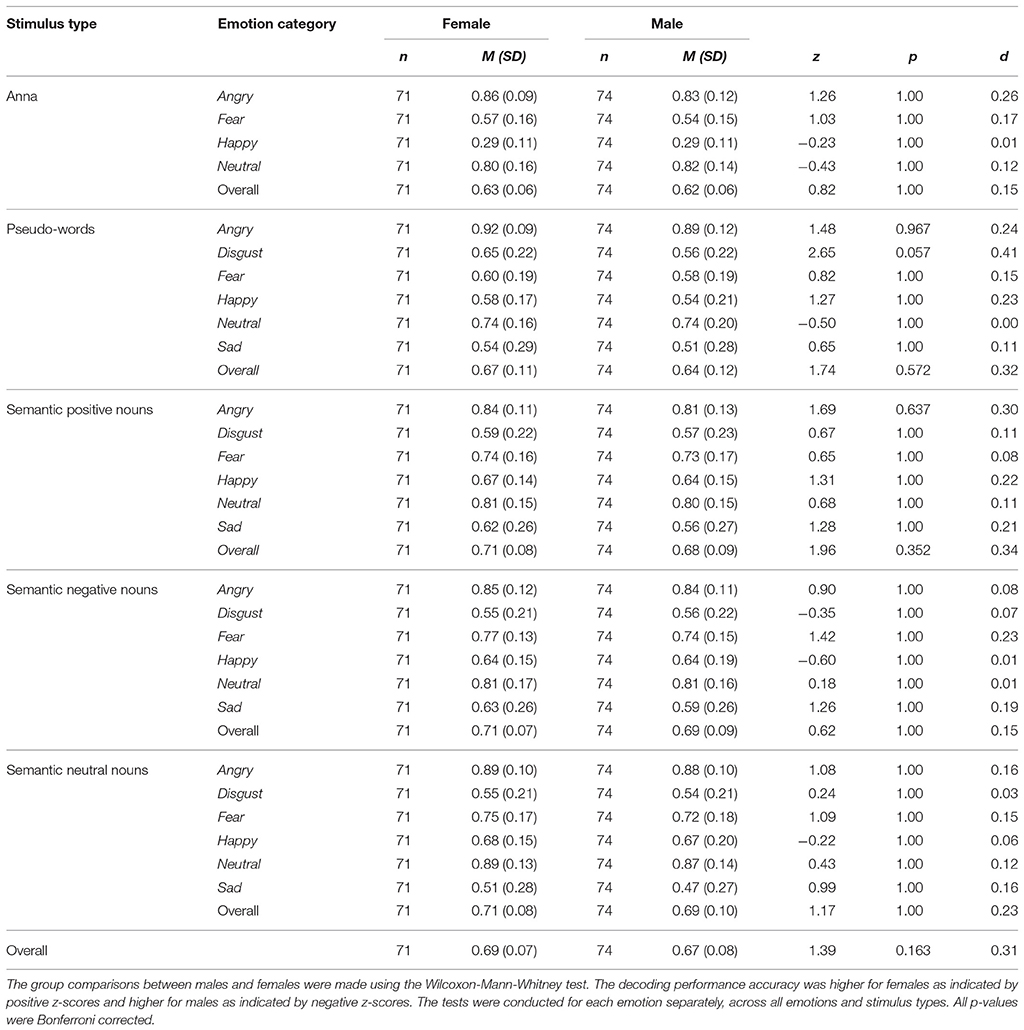
Table 4A. Group words: Means, standard deviations, z-scores, p-values, and effect sizes of performance accuracy by listeners' gender.
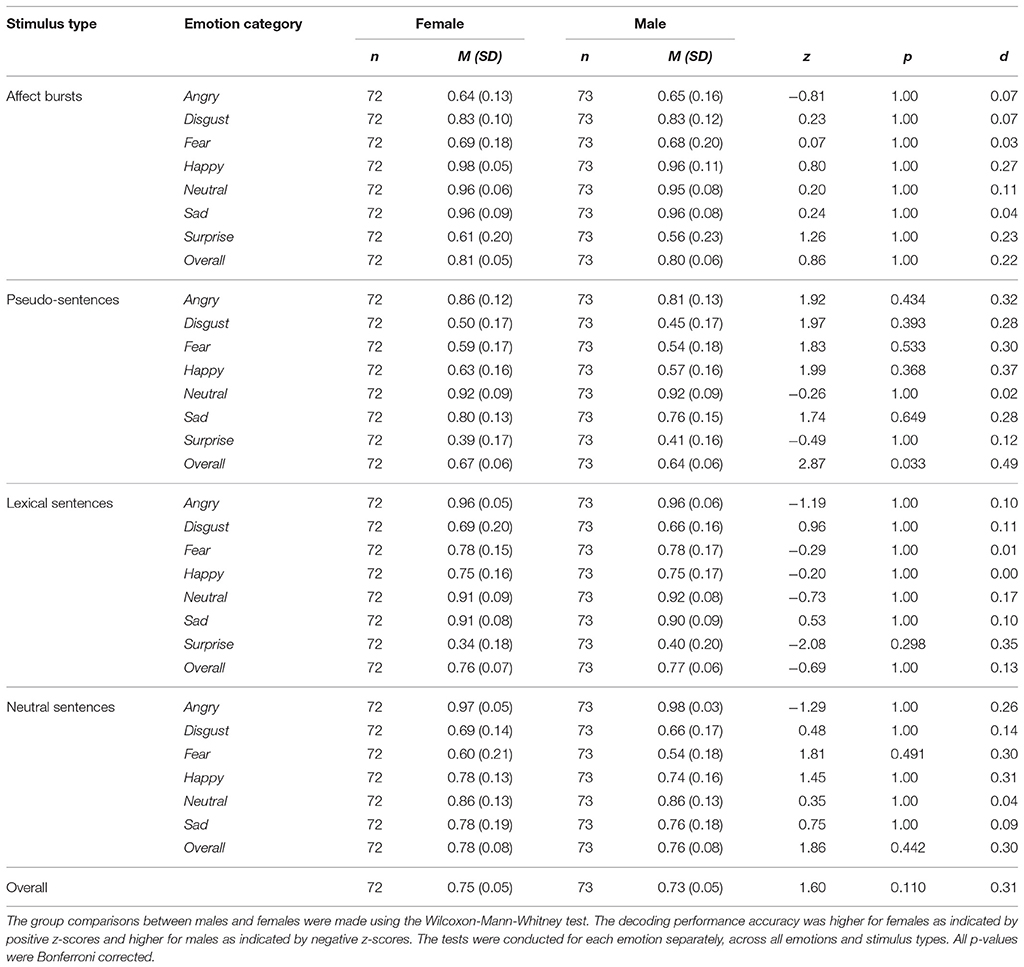
Table 4B. Group Sentences: Means, standard deviations, z-scores, p-values and effect sizes of performance accuracy by listeners' gender.
Performance Accuracy of Identifying Vocal Emotions by Speakers' Gender
The logistic regression models showed significant first order interactions between speaker gender and stimuli types [Group Words: = 142.80, p < 0.001; Group Sentences: = 18.50, p = 0.003]. Main effects of speaker gender were observed across all stimuli types [Group Words: = 42.30, p < 0.001; Group Sentences: = 589.40, p < 0.001], and following stimulus sub-sets: Anna [ = 75.13, p < 0.001], pseudo-words [ = 22.26, p < 0.001], semantic negative nouns [ = 71.74, p < 0.001], pseudo-sentences [ = 173.65, p < 0.001], lexical sentences [ = 154.70, p < 0.001], and affect bursts [ = 40.24, p < 0.001]. No main effects of speaker gender were found for semantic positive nouns [ = 0.43, p = 1.00], semantic neutral nouns [ = 3.05, p = 0.997], and neutral sentences [ = 0.93, p = 1.00].
We observed significant first order interactions between speakers' gender and emotions across all stimuli types [Group Words: = 842.30, p < 0.001; Group Sentences: = 726.70, p < 0.001] and for each stimulus sub-set [Anna: = 211.41, p < 0.001; pseudo-words: = 202.22, p < 0.001; semantic positive nouns: = 462.14, p < 0.001; semantic negative nouns: = 280.14, p < 0.001; semantic neutral nouns: = 465.36, p < 0.001; affect bursts: = 243.28, p < 0.001; pseudo-sentences: = 1276.99, p < 0.001; lexical sentences: = 194.50, p < 0.001; neutral sentences: = 449.41, p < 0.001]. Figures 2A, B display listeners' performance accuracy when identifying emotions from females' and males' voice.
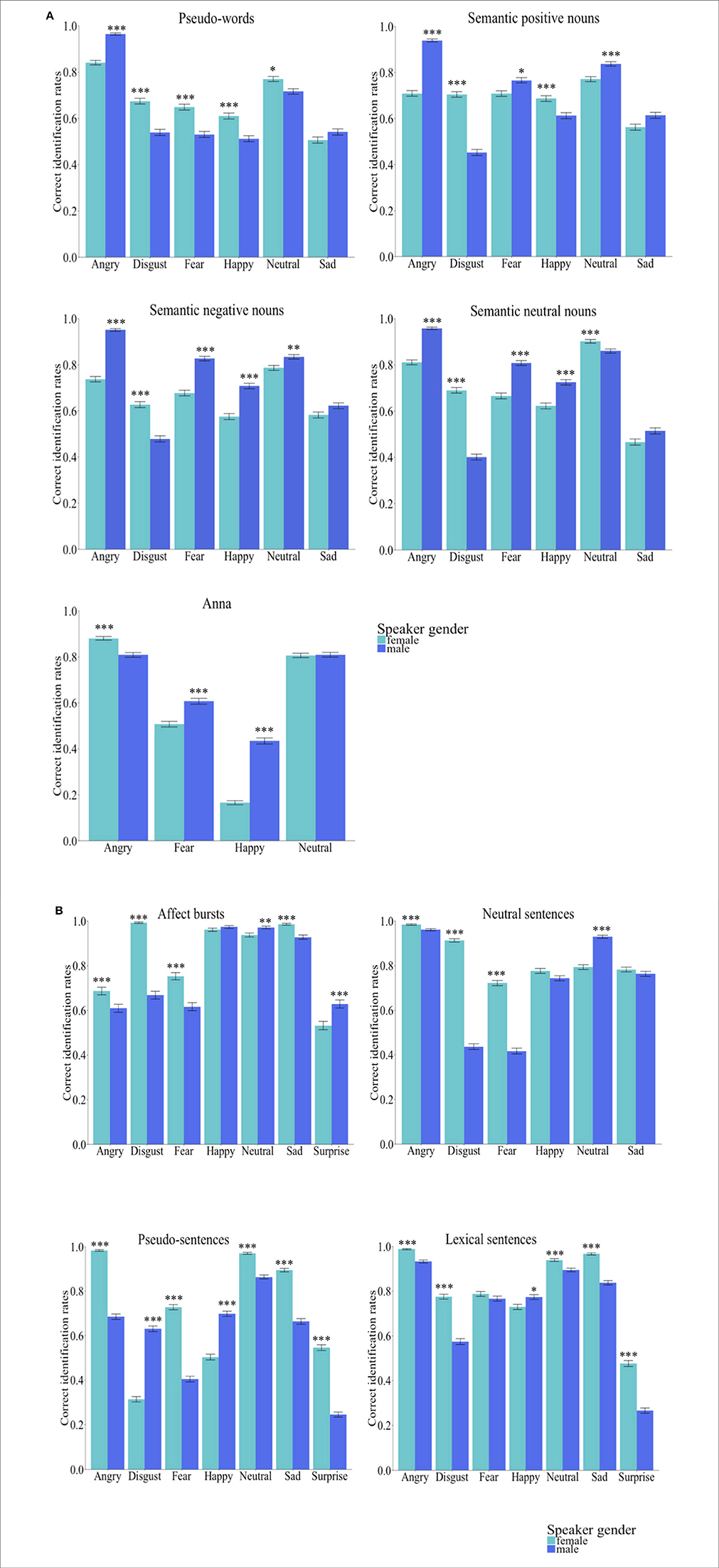
Figure 2. (A) Group words (n = 145, 71 females). Bar charts showing the performance accuracy of identifying emotions by speakers'gender. Error bars represent the standard error. Asterisks mark the significance level: *p < 0.05, **p<0.01, ***p<0.001. As it can be observed, for the majority of emotion categories by databases, the correct identification rates were higher for emotions uttered in a male than a female voice. (B) Group sentences (n = 145, 72 females). Bar charts showing the performance accuracy of identifying emotions by speakers' gender. Error bars represent the standard error. Asterisks mark the significance level: *p < 0.05, **p < 0.01, ***p < 0.001. As it can be observed, for the majority of emotion categories by databases, the correct identification rates were higher for emotion uttered in a female than a male voice.
To analyze whether specific emotions will have higher identification rates when spoken by a female than by a male encoder or vice-versa, a Wilcoxon-rank-sum test was fitted. Results showed that, except pseudo-sentences, in all other types of stimuli disgust was significantly better identified when uttered by a female than by a male (p's < 0.001, 0.42 < d < 2.39). In Group Words, except for the name Anna, angry had higher identification rates in males' than females' voice (p's < 0.001, 0.89 < d < 1.29), whereas in Group Sentences this emotion was better identified when spoken by a female than by a male (p's < 0.001, 0.32 < d < 1.21). For the other emotion categories, the pattern of results was not as clear-cut: in some types of stimuli utterances were significantly better identified when spoken by female than male actors and vice-versa. Across all emotions, Anna (p < 0.001, d = 0.84) and semantic negative nouns (p < 0.001, d = 0.84) were better identified in the male voice, whereas pseudo-words were better identified in the female voice. No significant differences in performance accuracy when male or female actors expressed the emotions were observed for semantic positive nouns (p = 1.00, d = 0.15) and semantic neutral nouns (p = 0.578, d = 0.18). In Group Sentences, however, female utterances were significantly better identified than those spoken by male actors (p's < 0.001, 0.80 < d < 1.62). Across all stimuli types, in Group Words vocal expressions had higher identification rates for male actors' expressions of emotion (p < 0.001, d = 0.40), whereas in Group Sentences these were better identified in the female voice (p < 0.001, d = 2.29). The performance accuracy by speakers' gender for each emotion category, across all emotions and stimulus types is presented in Tables 5A,B.
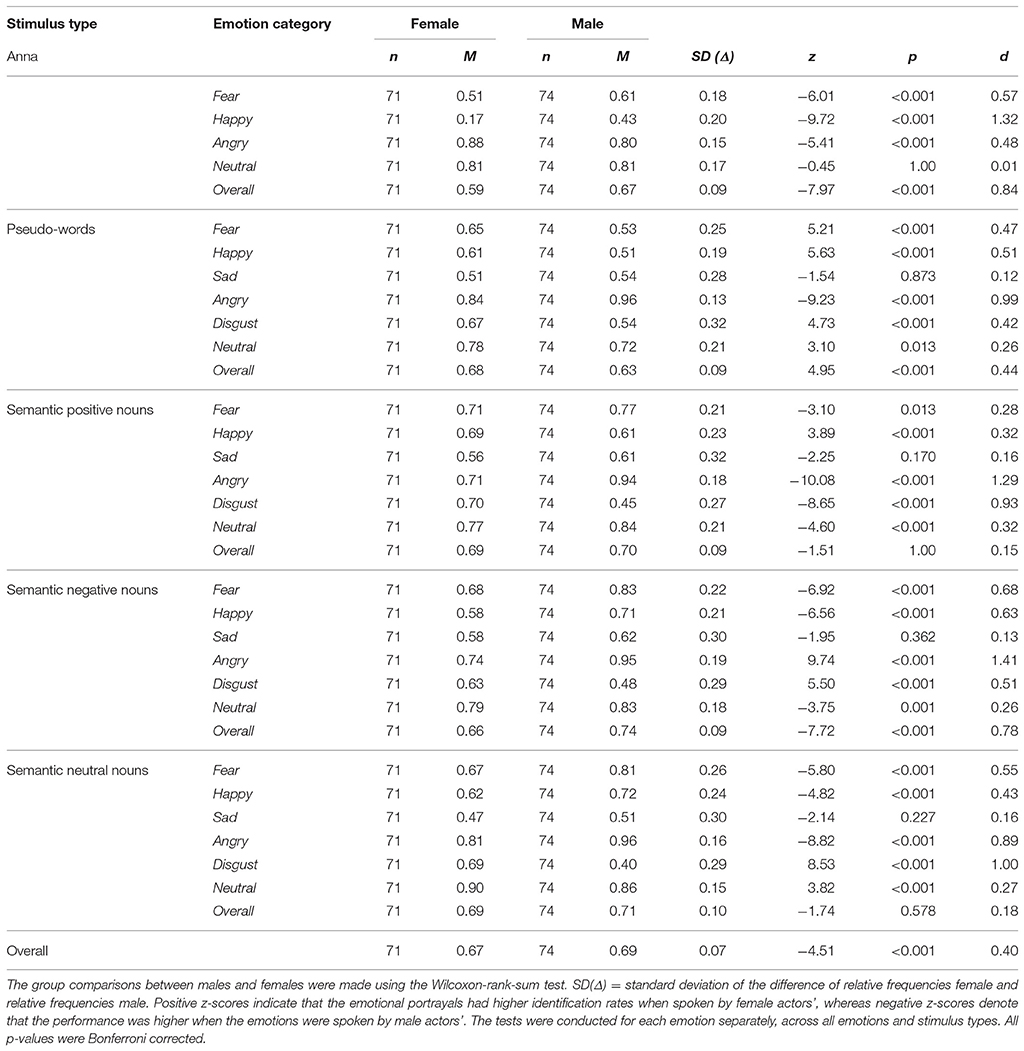
Table 5A. Group Words: Means, standard deviations, z-scores, p-values, and effect sizes of identification rates by speakers' gender.
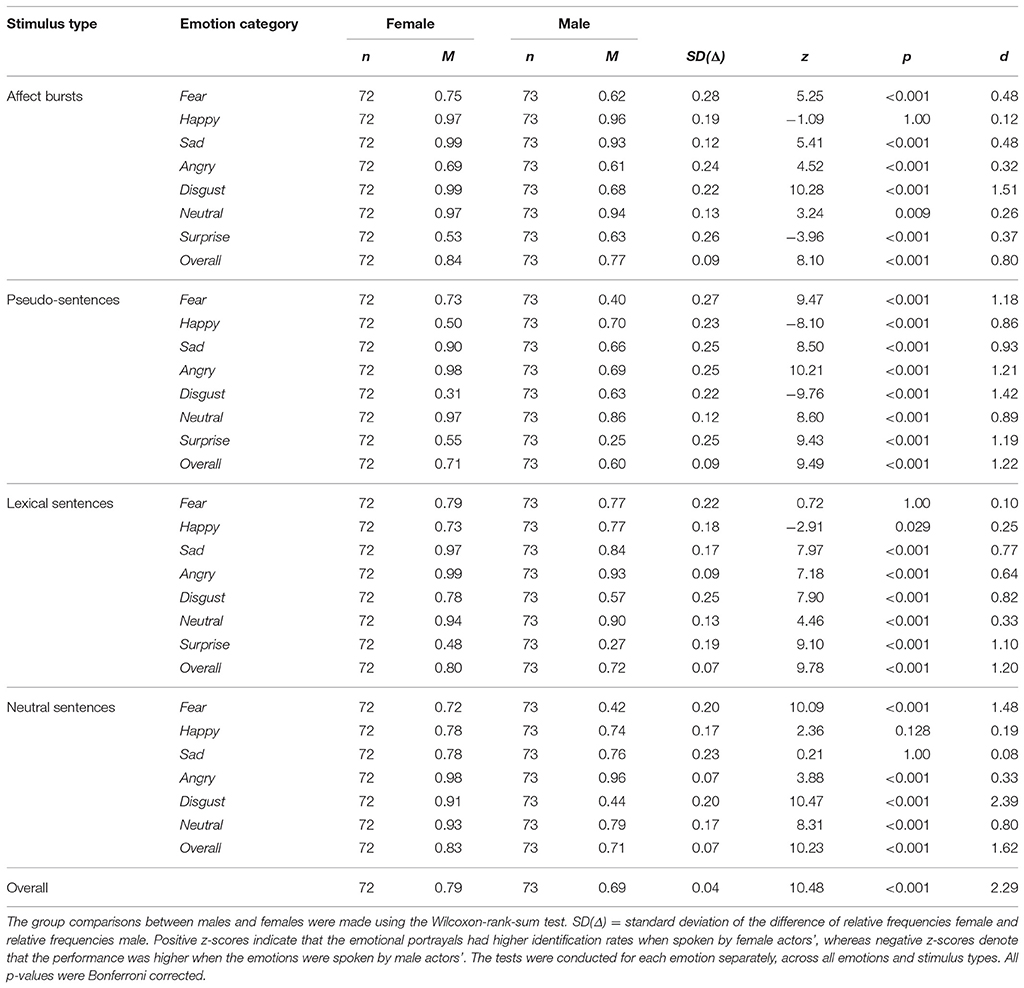
Table 5B. Group Sentences: Means, standard deviations, z-scores, p-values and effect sizes of identification rates by speakers' gender.
Interplay of Decoder and Encoder Gender and Emotion
No interactions between listeners and speakers gender was found across stimuli types [Group Words: = 0.30, p = 1.00; Group Sentences: = 0.10, p = 1.00] or for any of the stimuli sub-sets [Anna: = 1.41, p = 1.00; pseudo-words: = 0.85, p = 1.00; semantic positive nouns: = 0.06, p = 1.00; semantic negative nouns: = 0.25, p = 1.00; semantic neutral nouns: = 2.80, p = 1.00; affect bursts: = 0.21, p = 1.00; pseudo-sentences: = 0.31, p = 1.00; lexical sentences: = 0.20, p = 1.00; neutral sentences: = 1.67, p = 1.00]. The quasi-binomial logistic regression model revealed a significant second order interaction between speaker gender (encoder), listener gender (decoder), and emotion for semantic positive nouns [ = 17.94, p = 0.044]. This second order interaction pattern is explained by the inspection of the average ratings showing different gender patterns conditional on emotion categories (see Figure 3).
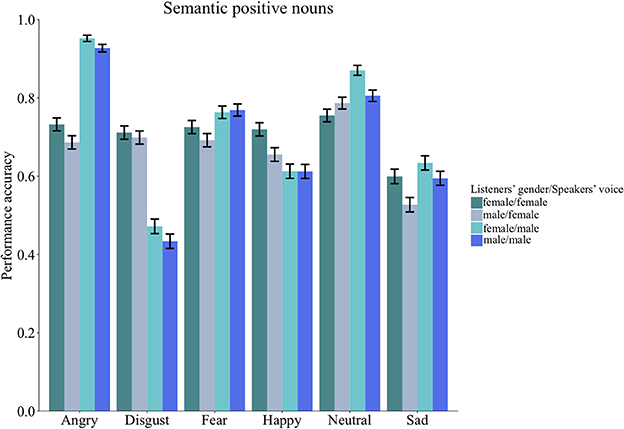
Figure 3. Group words (n = 145, 71 females). Bar charts showing the performance accuracy listeners' gender, speakers' gender and emotion categories. Error bars represent the standard error. As it can be observed, the second order interaction pattern is explained by the inspection of the average ratings showing different gender patterns conditional on emotion categories. For instance, female listeners had higher recognition accuracy for the emotion category happiness encoded in the female voice and higher recognition accuracy for the neutral category encoded in the male voice. In contrast, male listeners had lower recognition accuracy for the emotion category sad when spoken by a female.
No second order interactions were found across stimuli types [Group Words: = 15.00, p = 0.164; Group Sentences: = 4.50, p = 1.00] and for any of the other stimuli sub-sets: Anna [ = 2.57, p = 1.00], pseudo-words [ = 10.04, p = 0.927], semantic negative nouns [ = 6.63, p = 1.00], semantic neutral nouns [ = 2.36, p = 1.00], affect bursts [ = 4.94, p = 1.00], pseudo-sentences [ = 5.64, p = 1.00], lexical sentences [ = 5.70, p = 1.00] and neutral sentences [ = 2.01, p = 1.00].
Discussion
The present study aimed at investigating gender differences in the recognition of vocal emotions. Specifically, we investigated any gender-specific advantage for the decoding of vocal emotions that were presented across a variety of stimulus types and emotion categories. A second objective was to assess whether the speakers' gender impacts on identification accuracy for different types of vocal emotions. Finally, we explored potential interactions between listeners' and speakers' gender for the identification of vocal emotions. The stimuli used in this study included a wide range of vocal utterances (e.g., words/pseudo-words, sentences/pseudo-sentences, affect bursts) that were expressing different emotions [i.e., anger, disgust, happy, fear, sadness, and surprise, or no emotion (neutral)]. These characteristics of the stimulus set allowed us to assess gender differences for the recognition of vocal emotions in a differentiated manner and to provide parameter estimates on the magnitude of these effects. Especially the latter represents a largely neglected aspect within the vocal emotion literature.
Overall, our results showed that in each of the databases there were large differences in the recognition rates between emotions confirming well-established findings that recognition accuracy depends largely on the emotion category concerned (e.g., Scherer et al., 2001). Furthermore, we observed that performance accuracy is modulated by listeners' and speakers' gender and can significantly vary across stimulus types and emotion categories. Finally, we found that speaker gender had a significant impact on how listeners judged specific emotions from the voice. These findings will be discussed in detail in the following sub-sections.
Performance Accuracy by Listeners' Gender
We observed a significant main effect of gender reflecting that females outperformed males at categorizing emotions in vocal stimuli. The direction of this effect is consistent with previous findings on the recognition of non-verbal expressions of emotion (e.g., Hall, 1978; Collignon et al., 2010; Kret and de Gelder, 2012; Thompson and Voyer, 2014; Wingenbach et al., 2018) and emotional prosody in particular (e.g., Bonebright et al., 1996; Scherer et al., 2001; Paulmann and Uskul, 2014; Keshtiari and Kuhlmann, 2016).
An interesting pattern observed in our study is that females outperformed males when listening to emotionally produced pseudo-speech. Although the differences between females and males were not significant for emotion-specific categories the results clearly showed that overall females outperformed males when recognizing emotions from pseudo-sentences and had slightly higher recognition rates when decoding emotions from pseudo-words. As pseudo-speech lacks semantic meaning, one possible explanation for this effect is that women compared to men are better decoders under conditions of minimal stimulus information [see for example, child-rearing hypothesis (Babchuk et al., 1985) according to which females due to their role as primary caretakers have developed “evolved adaptions” hypothesized to include the fast and accurate decoding especially, for negative emotions]. However, the female advantage was significant only for pseudo-sentences and, thus until further evidence is provided on the robustness of these effects, this interpretation should be approached with caution. One example related to this interpretation is a large-sample study (N = 5,872) conducted by Fischer et al. (2018) that failed to replicate earlier findings assuming that females are better than males when categorizing discrete emotions in faces under situations of minimal stimulus information. Previous studies revealed that females score higher than males in decoding specific emotions such as happiness, fear, sadness or neutral expressions and that both genders perform equally well for emotions spoken in an angry and disgusted tone of voice (for an overview see Table 1). Our results partially support these findings. On the one hand, we were able to show that the performance accuracy between females and males did not differ for emotions spoken in an angry and disgusted tone of voice. The absence of a gender specific advantage for decoding a socially salient vocal emotion such as anger may be because humans (and other primates) are biologically prepared or “hard-wired” (Öhman, 1993) to respond rapidly to specific stimuli (e.g., screams; alarm calls) in the environment, independent of gender. Moreover, it has been suggested that anger and disgust are expressions that signal the rejection of something or someone (Schirmer, 2013) and, thus, one could argue that they place an equal demand on attentional resources regardless of gender. On the other hand, we found no evidence that females outperform males when decoding distressing (i.e., sad, fear), happy and neutral emotions from the voice. Similar to other findings on gender differences in emotion recognition (e.g., Fischer et al., 2018), the magnitude between genders we observed for the decoding of vocal emotions was relatively small. In our study, however, the direction of this effect consistently showed a female advantage for these specific emotions. Incorporating this pattern in biological and socialization models, one could assume that due to their ascribed nurturing, affiliative, and less dominant role (see Schirmer, 2013, for a review; Hess et al., 2005) women might have developed a higher sensitivity to minimal affective signals (e.g., recognition of infants' fleeting and subtle emotional signals) which may contribute to their advantage in understanding other persons' emotional states. Nevertheless, the variety of methodologies used in previous research posits difficulties when aiming to draw a conclusion against or in favor of an “female advantage” toward these specific emotions. Although our results partially suggest that females may have an advantage when decoding emotions from the voice, these effects might relate to the specific stimulus sets rather than female sensitivity toward particular emotions. It seems plausible that this finding is attributable to the different number of emotional categories included in the stimulus sets. For instance, some stimulus sets covered less emotional categories (e.g., Anna) than the options participants were offered to choose from. Offering emotional categories not included in the stimulus set, could lead to a systematic error in the face of a dichotomous choice between an emotion included in the set and one not included (e.g., happy vs. surprise). Another possible explanation could be a bias toward “negative” emotions, due to the majority of emotional categories being negative (four out of seven options). In addition, research has shown that speakers' pitch contour largely depends on the type and length of stimuli (e.g., words vs. sentences; see Jürgens et al., 2015, for a discussion) and, thus, one could argue that the results on emotion specific effects were affected by the acoustic properties of stimuli (e.g., pitch, timing, voice quality; see Banse and Scherer, 1996; Juslin and Laukka, 2003, for details), which might have varied between the stimulus sets.
Performance Accuracy of Identifying Vocal Emotions by Speakers' Gender
Despite observing some variability between genders in the expression of emotions for certain types of stimuli (e.g., Anna and nouns with a semantic positive and negative connotation were better identified when spoken by males), overall performance accuracy was significantly higher when females expressed the emotions. While findings for emotion-specific categories are pretty much inconsistent across studies, our results showed significantly higher identification rates for disgust when spoken by a female. However, for the other emotions categories, this pattern was less straightforward than one would expect. For instance, the identification rates for portrayals of anger were not consistently higher when spoken by a male. Likewise, happy, fearful or sad tone of voice were not invariably better identified when the speaker was a female (see Table 1 for an overview on previous findings). Enhanced identification of women compared to men's emotional expressions has been shown in both, facial (e.g., Hall, 1984) and vocal domains (e.g., Scherer et al., 2001; Belin et al., 2008). Previous reviews addressing gender-related patterns for the expression of emotions have suggested that these predispositions emerge as a result of various factors ranging from biologically innate traits, social norms and skills to situational contexts (e.g., see Chaplin, 2015; Fischer and LaFrance, 2015 for an overview). While the overall female advantage in the expression of emotions is advocated across studies on non-verbal communication (Hall, 1984), less clear is the evidence on why females and males differ in how well they can express particular emotions.
In our study, the mixed pattern for emotion-specific effects indicates that in the vocal domain, the reliability of emotion judgments is not systematically influenced by encoders' gender and the related stereotypes of emotional expressivity. Prior studies suggested that encoders' success in the speech channel may vary with the standardized utterance used (Banse and Scherer, 1996; Juslin and Laukka, 2003). As our stimulus sets were standardized utterances selected from validated databases we cannot clearly comment on similarities within or differences between the stimulus sets that might explain the observed mixed-pattern of results. One can assume, however, that in each database the instructions given to encoders' when portraying the emotions were different. This might have increased the chance that encoders differentially produced high- and low-intensity variants of vocal expressions of emotion and, thus one could speculate that, independent of gender, stimuli with higher-intensity were better identified than those with low intensity (Banse and Scherer, 1996; Juslin and Laukka, 2001). Another potential explanation for these variations in performance accuracy is, that in all databases the emotional expressions were recorded in a controlled setting through professional and non-professional actors. They were thus not real-life emotional expressions. While the methods of emotion simulation offer high experimental control, the validity of prosodic stimuli derived from these measures is limited (Scherer, 1986) and may boost recognition accuracy (Sauter and Fischer, 2017). Previous studies found that speakers often portray stereotypes of emotions and might differ in the quality of their emotional portrayals (e.g., one speaker might be very good at portraying happiness but not fear, whereas another speaker's performance might show the opposite pattern; Scherer, 1986; Banse and Scherer, 1996). More recent studies complement this evidence by showing that speakers with less acting experience might encounter difficulties when asked, for instance, to emote in a language devoid of meaning (e.g., Paulmann et al., 2016). Similarly, past work has shown that emotion categories sharing the same dimension of valence (e.g., happiness and surprise) and arousal (e.g., anger and fear) are more likely to be confused (e.g., Banse and Scherer, 1996). Thus, it is plausible that enacted emotions, expressed in isolation (i.e., without situational context) and belonging to the same valence category, might have challenged not only encoders' but also listeners' performance accuracy, thereby leading to ambiguous results. Finally, one could argue that the observed patterns in our results with regard to the identification accuracy of particular emotions from speakers' voice might not only be related to above-mentioned characteristics of our selected databases (e.g., types of stimuli, speakers acting experience, context) but they may also be reflected in the similarities and differences of acoustic and spectral profiles of emotional inflections in spoken language and non-verbal vocalizations (see, Banse and Scherer, 1996; Juslin and Laukka, 2001; Sauter et al., 2010, for details), which can be independent of encoders' gender.
Interplay Between Listeners, Speakers Gender and Emotion Categories
In contrast to previous findings (e.g., Bonebright et al., 1996; Belin et al., 2008) an interesting pattern we observed in our study is related to the significant interaction between listeners' gender, speakers' gender and emotions for sematic positive nouns. This showed that females were more sensitive to happy expressions spoken by a female, while sensitivity increased for angry, neutral, disgust, and sad expressions when spoken by a male. Although recognition accuracy seems to be contingent on the emotion being decoded as well as the speaker's gender, it is not clear whether the influence of encoder gender on these emotions reflects systematic properties of how these emotions are decoded and labeled, or whether certain artifacts may have been introduced by the semantic category of the stimuli (e.g., positive content spoken in an angry voice). As this pattern was present only for this type of stimuli we do not have a clear explanation for this effect. At most, one could speculate that females might be disposed to display fast and accurate decoding strategies in the face of an apparently conflicting message presented through semantics to detect credible cues about a speaker's true attitude and intentions. Words with a positive and negative semantic connotation, for instance, were found to have a processing advantage over neutral words (e.g., Schacht and Sommer, 2009a,b) and, thus one may speculate that this type of stimuli (here meaningful nouns) that either express an emotional state (e.g., happiness) or elicit one (e.g., shame) provoke differential responses in females and males.
Strengths, Limitations and Future Research
As emphasized by previous research and corroborated by our data, there are several advantages to control for factors believed to be central when assessing emotion recognition ability. First, the ecological validity of emotion recognition tasks can be expected to increase when a large number of stimuli containing a wider range of emotional expressions is studied. Second, employing gender-balanced samples allows the control of possible main effects in emotion recognition ability while examining potential interaction effects between decoders' and encoders'. Finally, presenting participants with one out of several emotions reduces the likelihood of judges arriving at the correct answer by using exclusion and probability rules. Given that gender differences in the recognition of emotions are generally reported as small or even absent, the present study extends previous findings to show that the female advantage becomes more evident when using a variety of stimuli, a larger number of speakers and a wider range of emotions. Although, we agree to some extent with proponents of gender similarity hypothesis (e.g., Hyde, 2014) that this female advantage should not be over-interpreted, our results clearly indicate that in the vocal domain, there was an underlying consistency toward a female advantage across a wide range of presented stimuli. Therefore, we believe that before under-interpreting these effects, one should consider them within the larger context of the more recent literature (e.g., Wingenbach et al., 2018), which similar to our study, demonstrated that improved methodologies and analysis (e.g., balanced design) help to assess the differences between genders in a more representative and generalizable fashion.
In our study, results showed some strong differences favoring each gender when decoding specific emotions from speakers' voice yet, this pattern was less straightforward than we expected. Although all selected stimuli were from validated databases, the variations within our results may simply reflect inconsistent procedures attributable to database characteristics (e.g., speakers' training, baseline vocal qualities, recording conditions). Moreover, it should be noted that despite using a variety of stimuli the number of speakers for some stimulus types was quite small (e.g., pseudo-words; lexical sentences). This makes it hard to generalize the effects regarding speaker gender to other speech databases. Future research should, thus, control for these factors and, seek to replicate findings on gender differences in the recognition of vocal emotions by using datasets of stimuli that include fully naturalized speech in emotion-related states to further increase ecological validity.
The absence of certain emotional categories within the databases and the fixed alternatives of emotional categories listeners had to choose from, might have led to lower accuracy in performance due to higher levels of cognitive load imposed by the task format. We chose a fixed-choice response format to compare the results with the majority of prior literature. However, this format may be less ecologically valid (Russel, 1994) and thus, it has been suggested that tasks including “other emotion” as a response alternative (Frank and Stennett, 2001), visual analog scales (Young et al., 2017) or open-ended perspective taking (Cassels and Birch, 2014) may prove more sensitive when measuring individuals' ability to recognize emotions. Moreover, our experiment might have been affected by common method variance such as assessment context (i.e., laboratory), item complexity (e.g., the perception of surprise might be interpreted as positive or negative), and mood state (for a comprehensive review, see Podsakoff et al., 2003).
An unexpected finding within our study was the significant age difference between males and females in both groups. Although several studies demonstrated that advancing age is associated with lower accuracy performance in the recognition of vocal emotions (e.g., Paulmann et al., 2008; Lima et al., 2014), our cohort was rather close in age (i.e., the older adults were not as old as populations reported in the literature). Thus, in future studies it would be interesting to clarify whether there is a critical earlier age period for emotional prosody recognition. This could be done, for example, by testing balanced groups of similar ages (e.g., 18–23; 24–29; 30–35) in order to specify a point of time at which emotional prosody recognition might start to decline with age.
Moreover, it has been suggested that prosodic acoustic parameters (e.g., speech melody, loudness), among other cues (e.g., semantics), provide listeners with a general understanding of the intended emotion and, thus, contribute in a cumulative fashion to the communication and recognition of emotions (Thompson and Balkwill, 2006, 2009). Future studies could explore how much of the variance in recognition rates is explained by similarities or differences in the acoustic attributes of emotive speech and assess the extent to which listeners use these acoustic parameters as a perceptual cue for identifying the portrayed emotion.
The present findings help to establish whether recognition accuracy differs according to listeners' and speakers' gender. Thus, an important step for future research will be to evaluate theories regarding why these differences or similarities may occur by taking into account evolutionary, cognitive-learning, socio-cultural, and expectancy-value theories (Hyde, 2014).
Previous research suggested that the visual-modality conveys higher degrees of positivity-negativity, whereas the voice incorporates higher degrees of dominance-submission (e.g., Hall, 1984). Thus, one interesting line of future investigation could explore whether females specialize in visual and males in vocal communication. Finally, as the present study evidenced some differences in emotion decoding and encoding in the auditory modality, it would be worthwhile to investigate how these differences relate to audio-visual integration of emotional signals among men and women. The combination of recognition data with physiological measures (e.g., peripheral indicators of emotional responses), psychosocial (e.g., personality traits) and demographic variables (e.g., age, education), as well as, self-reported trait measures of emotional intelligence and tests to assess participants' ability for sustained attention during an experiment, could help to assess gender differences in emotion recognition in an even more differentiated manner.
Conclusion
The present study replicates earlier research findings while controlling for several previously unaddressed confounds. It adds to the literature on gender differences for the recognition of vocal emotions by showing a female advantage in decoding accuracy and by establishing that females' emotional expressions are more accurately identified than those expressed by men. Results explain inconsistencies in the past literature in which findings of female superiority for identifying vocal emotions remain mixed by highlighting that the effect emerges for particular stimulus categories and under controlled environments. The partially mixed pattern of results in the current experimental task should be further investigated in natural settings, to assess whether males and females are attuned toward specific emotions in more realistic contexts.
Author Contributions
AL and AS designed the study and wrote the manuscript. AL conducted the experiment and data analysis. Both authors have given approval for the current version to be submitted and agree to be accountable for all aspects of the work in ensuring that questions related to the accuracy or integrity of any part of the work are appropriately investigated and resolved.
Funding
This research was funded by the Deutsche Forschungsgemeinschaft (DFG, German Research Foundation) – Project number 254142454/GRK 2070.
Conflict of Interest Statement
The authors declare that the research was conducted in the absence of any commercial or financial relationships that could be construed as a potential conflict of interest.
Acknowledgments
The authors thank Annika Grass and Florian Niefind for technical support, and Kurt Hammerschmidt and Lars Penke for fruitful discussions. We would further like to thank the editor and reviewers for their helpful comments on earlier versions of our manuscript.
Supplementary Material
The Supplementary Material for this article can be found online at: https://www.frontiersin.org/articles/10.3389/fpsyg.2018.00882/full#supplementary-material
Footnotes
1. ^We decided to use the term “gender” instead of “sex“ because this concept has a wider connotation and is not purely assigned by genetics. Participants had the option to provide a textual answer with regard to their gender (“Geschlecht”), yet all of them identified either as male or female (none of them wrote “sexless,” “I am born male but feel female” etc.).
References
Abelson, R. P. (1985). A variance explanation paradox: when a little is a lot. Psychol. Bull. 97, 129–133. doi: 10.1037/0033-2909.97.1.129
Ambady, N., and Rosenthal, R. (1998). “Nonverbal communication,” in Encyclopedia of Mental Health, 1st Edn, ed. H. Friedman (San Diego, CA: Academic Press), 775–782.
Apple, W., and Hecht, K. (1982). Speaking emotionally: the relation between verbal and vocal communication of affect. J. Pers. Soc. Psychol. 42, 864–875. doi: 10.1037/0022-3514.42.5.864
Azul, D. (2013). “How do voices become gendered? A critical examination of everyday and medical constructions of the relationship between voice, sex and gender identity,” in Challenging Popular Myths on Sex, Gender and Biology, ed. M. Ah-King (Dordrecht: Springer Science and Business Media), 77–89.
Babchuk, W. A., Hames, R. B., and Thompson, R. A. (1985). Sex differences in the recognition of infant facial expressions of emotion: the primary caretaker hypothesis. Ethol. Sociobiol. 6, 89–101. doi: 10.1016/0162-3095(85)90002-0
Ba̧k, H. K. (ed.). (2016). “The state of emotional prosody research—a meta-analysis,” in Emotional Prosody Processing for Non-Native English Speakers (Basel: Springer International Publishing), 79–112.
Banse, R., and Scherer, K. R. (1996). Acoustic profiles in vocal emotion expression. J. Pers. Soc. Psychol. 70, 614–636. doi: 10.1037/0022-3514.70.3.614
Barett, L. F., and Bliss-Moreau, E. (2009). She's emotional. He's having a bad day: attributional explanations for emotion stereotypes. Emotion 9, 649–658. doi: 10.1037/a0016821
Beaupré, M. G., and Hess, U. (2006). An ingroup advantage for confidence in emotion recognition judgments: the moderating effect of familiarity with the expressions of outgroup members. Pers. Soc. Psychol. Bull. 32, 16–26. doi: 10.1177/0146167205277097
Belin, P. (2006). Voice processing in human and non-human primates. Philos. T. R. Soc. B. Biol. Sci. 361, 2091–2107. doi: 10.1098/rstb.2006.1933
Boersma, P. (2001). Praat, a system for doing phonetics by computer. Glot Int. 5, 341–345. Available online at: https://www.researchgate.net/publication/208032992
Belin, P., Bestelmeyer, P. E. G., Latinus, M., and Watson, R. (2011). Understanding voice perception. Brit. J. Psychol. 102, 711–725. doi: 10.1111/j.2044-8295.2011.02041.x
Belin, P., Fillion-Bilodeau, S., and Gosselin, F. (2008). The Montreal affective voices: a validated set of nonverbal affect bursts for research on auditory affective processing. Behav. Res. Psychol. 40, 531–539. doi: 10.3758/BRM.40.2.531
Bonebright, T. L., Thompson, J. L., and Leger, D. W. (1996). Gender stereotypes in the expression and perception of vocal affect. Sex Roles 34, 429–445. doi: 10.1007/BF01547811
Briton, N. J., and Hall, J. A. (1995). Beliefs about female and male nonverbal communication. Sex Roles 32, 79–90.
Brody, L. R. (1997). Gender and emotion: beyond stereotypes. J. Soc. Issues 53, 369–393. doi: 10.1111/j.1540-4560.1997.tb02448.x
Burkhardt, F., Paeschke, A., Rolfes, M., Sendlmeier, W. F., and Weiss, B. (2005). “A database of German emotional speech,” in European Conference on Speech and Language Processing (EUROSPEECH) (Lisbon), 1517–1520.
Cassels, T. G., and Birch, S. A. J. (2014). Comparisons of an open-ended vs. forced-choice ‘mind reading’ task: implications for measuring perspective-taking and emotion recognition. PLoS ONE 9:e93653. doi: 10.1371/journal.pone.0093653
Chaplin, T. M. (2015). Gender and emotion expression: a developmental contextual perspective. Emot. Rev. 7, 14–21. doi: 10.1177/1754073914544408
Collignon, O., Girard, S., Gosselin, F., Saint-Amour, D., Lepore, F., and Lassonde, M. (2010). Women process multisensory emotion expressions more efficiently than men. Neuropsychologia 48, 220–225. doi: 10.1016/j.neuropsychologia.2009.09.007
Darwin, C. (1872/1998). The Expression of Emotion in Man and Animals. London: Harper Collins Publishers.
Davis, E., Greenberger, E., Charles, S., Chen, C., Zhao, L., and Dong, Q. (2012). Emotion ex- perience and regulation in China and the United States: how do culture and gender shape emotion responding? Int. J. Psychol. 47, 230–239. doi: 10.1080/00207594.2011.626043
de Gelder, B. (2016). “Gender, culture and context differences in recognition of bodily expressions,” in Emotions and the Body, 1st Edn, ed. B. de Gelder (New York, NY: Oxford University Press), 163–191.
de Gelder, B., and Vroomen, J. (2000). The perception of emotions by ear and by eye. Cogn. Emotion 14, 289–311. doi: 10.1080/026999300378824
Demenescu, L. R., Kato, Y., and Mathiak, K. (2015). Neural processing of emotional prosody across the adult lifespan. Biomed. Res. Int. 1:9. doi: 10.1155/2015/590216
Eagly, A. H., and Wood, W. (1999). The origins of sex differences in human behavior. Evolved dispositions versus social roles. Am. Psychol. 54, 408–423. doi: 10.1037/0003-066X.54.6.408
Elfenbein, H. A., and Ambady, N. (2002a). On the universality and cultural specificity of emotion recognition: a meta-analysis. Psychol. Bull. 128, 203–235. doi: 10.1037/0033-2909.128.2.203
Elfenbein, H. A., and Ambady, N. (2002b). Is there an in-group advantage in emotion recognition? Psychol. Bull. 128, 243–249. doi: 10.1037/0033-2909.128.2.243
Fischer, A. H., and Evers, C. (2013). “The social basis of emotion in men and women,” in The Sage Handbook of Gender and Psychology, eds M. K. Ryan and N. R. Branscombe (London: Sage), 183–199.
Fischer, A. H., Kret, M. E., and Broekens, J. (2018). Gender differences in emotion perception and self-reported emotional intelligence: a test of the emotion sensitivity hypothesis. PLoS ONE 13:e0190712. doi: 10.1371/journal.pone.0190712
Fischer, A. H., and LaFrance, M. (2015). What drives the smile and the tear: why women are more emotionally expressive than men. Emot. Rev. 7, 22–29. doi: 10.1177/1754073914544406
Fischer, A. H., and Manstead, A. S. R. (2008). “Social functions of emotion,” in Handbook of Emotions, 3rd Edn, eds M. Lewis, J. Haviland-Jones, and L. F. Barrett (New York, NY: Guilford Press), 456–468.
Fischer, J., and Price, T. (2017). Meaning, intention, and inference in primate vocal communication. Neurosci. Biobehav. R. 82, 22–31. doi: 10.1016/j.neubiorev.2016.10.014
Frank, M. G., and Stennett, J. (2001). The forced-choice paradigm and the perception of facial expressions of emotion. J. Pers. Soc. Psychol. 80, 75–85. doi: 10.1037/0022-3514.80.1.75
Fujisawa, T. X., and Shinohara, K. (2011). Sex differences in the recognition of emotional prosody in late childhood and adolescence. J. Physiol. Sci. 61, 429–435. doi: 10.1007/s12576-011-0156-9
Gray, J. (1992). Men are From Mars, Women are From Venus: A Practical Guide for Improving Communication and Getting What You Want in Your Relationships. New York, NY: Harper Collins.
Hall, J. A. (1978). Gender effects in decoding nonverbal cues. Psychol. Bull. 85, 845–857. doi: 10.1037/0033-2909.85.4.845
Hall, J. A. (1984). Non-Verbal Sex Differences. Communication and Expressive Style. London: John Hopkins Press.
Hall, J. A. (2006). Nonverbal behavior, status, and gender: how do we understand their relations? Psychol. Women Q. 30, 384–391. doi: 10.1111/j.1471-6402.2006.00313.x
Hall, J. A., Carter, J. D., and Horgan, T. G. (2000). “Gender differences in the nonverbal communication of emotion,” in Gender and Emotion: Social Psychological Perspectives, ed. A. H. Fischer (Paris: Cambridge University Press), 97–117.
Hammerschmidt, K., and Jürgens, U. (2007). Acoustical correlates of affective prosody. J. Voice 21, 531–540. doi: 10.1016/j.jvoice.2006.03.002
Hawk, S. T., van Kleef, G. A., Fischer, A. H., and van der Schalk, J. (2009). “Worth a thousand words”: absolute and relative decoding of nonlinguistic affect vocalizations. Emotion 9, 293–305. doi: 10.1037/a0015178
Hess, U., Adams, R., and Kleck, R. (2005). Who may frown and who should smile? Dominance, affiliation, and the display of happiness and anger. Cogn. Emot. 19, 515–536. doi: 10.1080/02699930441000364
Hess, U., Senécal, S., Kirouac, G., Herrera, P., Philippot, P., and Kleck, R. E. (2000). Emotional expressivity in men and women: stereotypes and self-perceptions. Cogn. Emot. 14, 609–642. doi: 10.1080/02699930050117648
Hothorn, T., Hornik, K., van de Wiel, M. A., and Zeileis, A. (2008). Implementing a class of permutation tests: the coin package. J. Stat. Softw. 28, 1–23. doi: 10.18637/jss.v028.i08
Hyde, J. S. (2005). The gender similarities hypothesis. Am. Psychol. 60, 581–592. doi: 10.1037/0003-066X.60.6.581
Hyde, J. S. (2014). Gender similarities and differences. Annu. Rev. Psychol. 65, 373–398. doi: 10.1146/annurev-psych-010213-115057
Jessen, S., and Kotz, S. A. (2011). The temporal dynamics of processing emotions from vocal, facial, and bodily expressions. Neuroimage 58, 665–674. doi: 10.1016/j.neuroimage.2011.06.035
Jürgens, R., Fischer, J., and Schacht, A. (2018). Hot speech and exploding bombs: autonomic arousal during emotion classification of prosodic utterances and affective sounds. Front. Psychol. 9:228. doi: 10.3389/fpsyg.2018.00228
Jürgens, R., Grass, A., Drolet, M., and Fischer, J. (2015). Effect of acting experience on emotion expression and recognition in voice: non-actors provide better stimuli than expected. J. Nonverbal Behav. 39, 195–214. doi: 10.1007/s10919-015-0209-5
Juslin, P. N., and Laukka, P. (2001). Impact of intended emotion intensity on cue utilization and decoding accuracy in vocal expression of emotion. Emotion 1, 381–412. doi: 10.1037/1528-3542.1.4.381
Juslin, P. N., and Laukka, P. (2003). Communication of emotions in vocal expression and music performance: different channels, same code? Psychol. Bull. 129, 770–814. doi: 10.1037/0033-2909.129.5.770
Keshtiari, N., and Kuhlmann, M. (2016). The effects of culture and gender on the recognition of emotional speech: evidence from Persian speakers living in a collectivist society. Int. J. Soc. Cult. Lang. 4, 71–86. doi: 10.13140/rg.2.1.1159.0001
Klasen, M., Kreifelts, B., Chen, Y. H., Seubert, J., and Mathiak, K. (2014). Neural processing of emotion in multimodal settings. Front. Hum. Neurosci. 8:822. doi: 10.3389/fnhum.2014.00822
Kraus, M. (2017). Voice-only communication enhances empathic accuracy. Am. Psychol. 72, 644–654. doi: 10.1037/amp0000147
Kret, M. E., and de Gelder, B. (2012). A review on sex differences in processing emotional signals. Neuropsychologia 50, 1211–1221. doi: 10.1016/j.neuropsychologia.2011.12.022
Lambrecht, L., Kreifelts, B., and Wildgruber, D. (2014). Gender differences in emotion recognition: impact of sensory modality and emotional category. Cogn. Emot. 28, 452–469. doi: 10.1080/02699931.2013.837378
Latinus, M., and Belin, P. (2011). Human voice perception. Curr. Biol. 21, 143–145. doi: 10.1016/j.cub.2010.12.033
Levenson, R. W., and Ruef, A. M. (1992). Empathy: a physiological substrate. J. Pers. Soc. Psychol. 63, 234–246. doi: 10.1037/0022-3514.63.2.234
Lima, C. F., Alves, T., Scott, S. K., and Castro, S. L. (2014). In the ear of the beholder: how age shapes emotion processing in nonverbal vocalizations. Emotion 14, 145–160. doi: 10.1037/a0034287
MacAdams, S. (2012). Decoding nonverbal expressions of emotion of men and women. Modern Psychol. Studies 18, 6–17. Available online at: https://scholar.utc.edu/mps/vol18/iss1/3
McClure, E. B. (2000). A meta-analytic review of sex differences in facial expression processing and their development in infants, children, and adolescents. Psychol. Bull. 125, 424–453. doi: 10.1037/0033-2909.126.3.424
McDuff, D., Kodra, E., Kaliouby, R., and LaFrance, M. (2017). A large-scale analysis of sex differences in facial expressions. PLoS ONE 12:e0173942. doi: 10.1371/journal.pone.0173942
Morningstar, M. (2017). Age-Related Differences in the Production and Recognition of Vocal Socio-Emotional Expressions. Dissertation, McGill University, Montreal.
Öhman, A. (1993). “Fear and anxiety as emotional phenomenon: clinical phenomenology, evolutionary perspectives, and information-processing mechanisms,” in Handbook of Emotions, eds M. Lewis and J. M. Haviland (New York, NY: Guildford Press), 511–536.
Parkins, R. (2012). “Gender and emotional expressiveness: an analysis of prosodic features in emotional expression. Griffith work. Papers in Pragmat. Intercult. Commun. 5, 46–54. Available online at: https://www.griffith.edu.au/__data/assets/pdf_file/0026/363680/Paper-6-Parkins-Gender-and-Emotional-Expressiveness_final.pdf
Paulmann, S., Furnes, D., Bøkenes, A. M., and Cozzolino, P. J. (2016). How psychological stress affects emotional prosody. PLoS ONE 11:e0165022. doi: 10.1371/journal.pone.0165022
Paulmann, S., and Kotz, S. A. (2008). An ERP investigation on the temporal dynamics of emotional prosody and emotional semantics in pseudo- and lexical sentence context. Brain Lang. 105, 59–69. doi: 10.1016/j.bandl.2007.11.005
Paulmann, S., and Pell, M. D. (2010). Dynamic emotion processing in Parkinson's disease as a function of channel availability. J. Clin. Exp. Neuropsyc. 32, 822–835. doi: 10.1080/13803391003596371
Paulmann, S., Pell, M. D., and Kotz, S. A. (2008). How aging affects the recognition of emotional speech. Brain Lang. 104, 262–269. doi: 10.1016/j.bandl.2007.03.002
Paulmann, S., Titone, D., and Pell, M. D. (2012). How emotional prosody guides your way: evidence from eye movements. Speech Commun. 54, 92–107. doi: 10.1016/j.specom.2011.07.004
Paulmann, S., and Uskul, A. K. (2014). Cross-cultural emotional prosody recognition: evidence from Chinese and British listeners. Cogn. Emot. 28, 230–244. doi: 10.1080/02699931.2013.812033
Pell, M. D. (2002). Evaluation of nonverbal emotion in face and voice: some preliminary findings on a new battery of tests. Brain Cogn. 48, 499–514.
Pell, M. D., Kotz, S. A., Paulmann, S., and Alasseri, A. (2005). “Recognition of basic emotions from speech prosody as a function of language and sex,” in Abstract Psychonomic Social 46th Annual Meeting 10 (Toronto), 98.
Pell, M. D., Rothermich, K., Liu, P., Paulmann, S., Sethi, S., and Rigoulot, S. (2015). Preferential decoding of emotion from human non-linguistic vocalizations versus speech prosody. Biol. Psychol. 111, 14–25. doi: 10.1016/j.biopsycho.2015.08.008
Podsakoff, P. M., MacKenzie, S. B., Lee, Y. J., and Podsakoff, N. P. (2003). Common method biases in behavioural research: a critical review of the literature and recommended remedies. J. Appl. Psychol. 88, 879–903. doi: 10.1037/0021-9010.88.5.879
R Core Team (2016). R: A Language and Environment for Statistical Computing. Vienna: R Foundation for Statistical Computing. Available online at: https://www.r-project.org
Raithel, V., and Hielscher-Fastabend, M. (2004). Emotional and linguistic perception of prosody. Reception of prosody. Folia Phoniatr. Logo. 56, 7–13. doi: 10.1159/000075324
Recio, G., Schacht, A., and Sommer, W. (2014). Recognizing dynamic facial expressions of emotion: specificity and intensity effects in event-related brain potentials. Biol. Psychol. 96, 111–125. doi: 10.1016/j.biopsycho.2013.12.003
Recio, G., Sommer, W., and Schacht, A. (2011). Electrophysiological correlates of perceiving and evaluating static and dynamic facial expressions. Brain Res. 1376, 66–75. doi: 10.1016/j.brainres.2010.12.041
Richard, F. D., Bond, C. F., and Stokes-Zoota, J. J. (2003). One hundred years of social psychology quantitatively described. Rev. General Psychol. 7, 331–363. doi: 10.1037/1089-2680.7.4.331
Rigoulot, S., Wassiliwizky, E., and Pell, M. D. (2013). Feeling backwards? How temporal order in speech affects the time course of vocal emotion recognition. Front. Psychol. 4:367. doi: 10.3389/fpsyg.2013.00367
Riviello, M. T., and Esposito, A. (2016). “Recognition performance on the American and Italian cross-modal databases,” in On the Perception of Dynamic Emotional Expressions: A Cross-cultural Comparison, eds M. T. Riviello and A. Esposito (Dordrecht: Springer), 9–23.
Rosenthal, R., and DePaulo, B. M. (1979). “Sex differences in accommodation in nonverbal communication,” in Skill in Nonverbal Communication: Individual Differences, ed. R. Rosenthal (Cambridge, MA: Oelgeschlager, Gunn and Hain Publishers), 68–96.
Russel, J. A. (1994). Is there universal recognition of emotion from facial expression? A review of the cross-cultural studies. Psychol. Bull. 115, 102–141. doi: 10.1037/0033-2909.115.1.102
Santos, L. F., and Osório, F. L. (2015). Influence of gender in the recognition of basic facial expressions: a critical literature review. World J. Psychiatr. 22, 342–351. doi: 10.5498/wjp.v5.i3.342
Sauter, D. A., Eisner, F., Calder, A. J., and Scott, S. K. (2010). Perceptual cues in nonverbal vocal expressions of emotion. Q. J. Exp. Psychol. 63, 2251–2272. doi: 10.1080/17470211003721642
Sauter, D. A., and Fischer, A. H. (2017). Can perceivers recognise emotions from sponatneous expressions? Cogn. Emot. 32, 504–515. doi: 10.1080/02699931.2017.1320978
Sauter, D. A., Panattoni, C., and Happé, F. (2013). Children's recognition of emotions from vocal cues. Brit. J. Dev. Psychol. 31, 97–113. doi: 10.1111/j.2044-835X.2012.02081.x
Schacht, A., and Sommer, W. (2009a). Emotions in word and face processing: early and late cortical responses. Brain Cogn. 69, 538–550. doi: 10.1016/j.bandc.2008.11.005
Schacht, A., and Sommer, W. (2009b). Time course and task dependence of emotion effects in word processing. Cogn. Affect. Behav. Neurosci. 9, 28–43. doi: 10.3758/CABN.9.1.28
Scherer, K. R. (1986). Vocal affect expression: a review and a model for future research. Psychol. Bull 99, 143–165.
Scherer, K. R., Banse, R., and Wallbott, H. (2001). Emotion inferences from vocal expression correlate across languages and cultures. J. Cross. Cult. Psychol. 32, 76–92. doi: 10.1177/0022022101032001009
Scherer, K. R., and Scherer, U. (2011). Assessing the ability to recognize facial and vocal expressions of emotion: construction and validation of the emotion recognition index. J. Nonverbal Behav. 35, 305–326. doi: 10.1007/s10919-011-0115-4
Schirmer, A. (2013). “Sex differences in emotion,” in The Cambridge Handbook of Human Affective Neuroscience, 1st Edn, eds. J. Armony and P. Vuilleumier (New York, NY: Cambridge University Press), 591–611.
Schirmer, A., and Kotz, S. A. (2003). ERP evidence for a sex-specific stroop effect in emotional speech. J. Cogn. Neurosci. 15, 1135–1148. doi: 10.1162/089892903322598102
Schmidt, F. L., and Oh, I. S. (2013). Methods for second order meta-analysis and illustrative applications. Organ. Behav. Hum. Decision Proc. 121, 204–218. doi: 10.1016/j.obhdp.2013.03.002
Shields, S. A. (2002). Speaking From the Heart: Gender and the Social Meaning of Emotion. New York, NY: Cambridge University Press.
Schwartz, R., and Pell, M. D. (2012). Emotional speech processing at the intersection of prosody and semantics. PLoS ONE 7:e47279. doi: 10.1371/journal.pone.0047279
Sokolov, A. A., Krüger, S., Enck, P., Mann, I. K., and Pavlova, M. A. (2011). Gender affects body language reading. Front. Psychol. 2:16. doi: 10.3389/fpsyg.2011.00016
Šolcová, I. P., and Lacev, A. (2017). Differences in male and female subjective experience and physiological reactions to emotional stimuli. Int. J. Psychophysiol. 117, 75–82. doi: 10.1016/j.ijpsycho.2017.04.009
Thompson, A. E., and Voyer, D. (2014). Sex differences in the ability to recognize non-verbal displays of emotion: a meta-analysis. Cogn. Emot. 28, 1164–1195. doi: 10.1080/02699931.2013.875889
Thompson, W. F., and Balkwill, L. L. (2006). Decoding speech prosody in five languages. Semiotica 158, 407–424. doi: 10.1515/SEM.2006.017
Thompson, W. F., and Balkwill, L. L. (2009). “Cross-cultural similarities and differences,” in Handbook of Music and Emotion: Theory, Research, Applications, 1st Edn, eds P.N. Juslin and J.A. Sloboda (New York, NY: Oxford University Press), 755–791.
Timmers, M., Fischer, A. H., and Manstead, A. S. R. (2003). Ability versus vulnerability: beliefs about men's and women's emotional behavior. Cogn. Emot. 17, 41–63. doi: 10.1080/02699930302277
Toivanen, J., Väyrynen, E., and Seppänen, T. (2005). “Gender differences in the ability to discriminate emotional content from speech,” in Proceedings FONETHIK, eds A. Eriksson and J. Lindh (Göteborg: Reprocentralen, Humanisten, Göteborg University), 119–123.
Van den Stock, J., Righart, R., and de Gelder, B. (2007). Body expressions influence recognition of emotions in the face and voice. Emotion 7, 487–494. doi: 10.1037/1528-3542.7.3.487
Võ, M. L.-H., Conrad, M., Kuchinke, L., Urton, K., Hofmann, M. J., et al. (2009). The Berlin affective word list reloaded (BAWL-R). Behav. Res. Methods 41, 534–538. doi: 10.3758/BRM.41.2.534
Wagner, H. L. (1993). On measuring performance in category judgement studies of nonverbal behaviour. J. Nonverbal Behav. 17, 3–28. doi: 10.1007/BF00987006
Wells, L. J., Gillespie, S. M., and Rotshtein, P. (2016). Identification of emotional facial expressions: effects of expression, intensity, and sex on eye gaze. PLoS ONE 11:e0168307. doi: 10.1371/journal.pone.0168307
Wendt, B., and Scheich, H. (2002). “The “Magdeburger Prosodie Korpus” - a spoken language corpus for fMRI-Studies,” in Speech Prosody 2002, eds B. Bel and I. Marlien (Aix-en-Provence, SproSIG), 699–701.
Wingenbach, T. S. H., Ashwin, C., and Brosnan, M. (2018). Sex differences in facial emotion recognition across varying expression intensity levels from videos. PLoS ONE 13:e0190634. doi: 10.1371/journal.pone.0190634
Young, K. S., Parsons, C. E., LeBeau, R. T., Tabak, B. A., Sewart, A. R., et al. (2017). Sensing emotion in voices: negativity bias and gender differences in a validation study of the Oxford Vocal (“OxVoc”) Sounds Database. Psychol. Assess. 29, 967–977. doi: 10.1037/pas0000382
Zell, E., and Krizan, Z. (2014). Do people have insight into their abilities? A meta-synthesis. Perspect. Psychol. Sci. 9, 111–125. doi: 10.1177/1745691613518075
Zuckerman, M., Lipets, M. S., Koivumaki, J. H., and Rosenthal, R. (1975). Encoding and decoding nonverbal cues of emotion. J. Pers. Soc. Psychol. 32, 1068–1032. doi: 10.1037/0022-3514.32.6.1068
Keywords: gender differences, emotion recognition accuracy, voice, speech-embedded emotions, affect bursts
Citation: Lausen A and Schacht A (2018) Gender Differences in the Recognition of Vocal Emotions. Front. Psychol. 9:882. doi: 10.3389/fpsyg.2018.00882
Received: 02 February 2018; Accepted: 15 May 2018;
Published: 05 June 2018.
Edited by:
Petri Laukka, Stockholm University, SwedenReviewed by:
Tanja S. H. Wingenbach, Mackenzie Presbyterian University, BrazilKatherine S. Young, University of California, Los Angeles, United States
Copyright © 2018 Lausen and Schacht. This is an open-access article distributed under the terms of the Creative Commons Attribution License (CC BY). The use, distribution or reproduction in other forums is permitted, provided the original author(s) and the copyright owner are credited and that the original publication in this journal is cited, in accordance with accepted academic practice. No use, distribution or reproduction is permitted which does not comply with these terms.
*Correspondence: Adi Lausen, adi.lausen@psych.uni-goettingen.de