- 1The Shraga Segal Department of Microbiology, Immunology and Genetics, Faculty of Health Sciences, Ben-Gurion University of the Negev, Beer Sheva, Israel
- 2National Institute for Biotechnology in the Negev, Ben-Gurion University of the Negev, Beer Sheva, Israel
- 3Department of Otolaryngology-Head and Neck Surgery, Soroka Medical Center and Faculty of Health Sciences, Ben-Gurion University of the Negev, Beer Sheva, Israel
- 4Department of Otolaryngology-Head and Neck Surgery and The Center for Translational Research in Head and Neck Cancer, Rabin Medical Center, Petah Tikva and Sackler Faculty of Medicine, Tel Aviv University, Tel Aviv, Israel
- 5Worldwide Innovative Network Association-WIN Consortium, Villejuif, France
Despite of remarkable progress made in the head and neck cancer (HNC) therapy, the survival rate of this metastatic disease remain low. Tailoring the appropriate therapy to patients is a major challenge and highlights the unmet need to have a good preclinical model that will predict clinical response. Hence, we developed an accurate and time efficient drug screening method of tumor ex vivo analysis (TEVA) system, which can predict patient-specific drug responses. In this study, we generated six patient derived xenografts (PDXs) which were utilized for TEVA. Briefly, PDXs were cut into 2 × 2 × 2 mm3 explants and treated with clinically relevant drugs for 24 h. Tumor cell proliferation and death were evaluated by immunohistochemistry and TEVA score was calculated. Ex vivo and in vivo drug efficacy studies were performed on four PDXs and three drugs side-by-side to explore correlation between TEVA and PDX treatment in vivo. Efficacy of drug combinations was also ventured. Optimization of the culture timings dictated 24 h to be the time frame to detect drug responses and drug penetrates 2 × 2 × 2 mm3 explants as signaling pathways were significantly altered. Tumor responses to drugs in TEVA, significantly corresponds with the drug efficacy in mice. Overall, this low cost, robust, relatively simple and efficient 3D tissue-based method, employing material from one PDX, can bypass the necessity of drug validation in immune-incompetent PDX-bearing mice. Our data provides a potential rationale for utilizing TEVA to predict tumor response to targeted and chemo therapies when multiple targets are proposed.
Introduction
Advances in basic and medical science research led to the discovery of numerous cancer specific-targeted chemotherapy drugs [reviewed in (1)]. The variety of treatment alternatives and the existence of several mutated signaling pathways in each cancer imposes a new challenge to the physicians since omics-based prediction of personalized treatment might not suffice to accurately predict and prioritize the best personalized treatment [reviewed in (2)]. Therefore, additional test of the various omics-predicted targeted therapies, on the specific patient's cancer, is needed. At present, several in vitro 3D test systems, based on human material taken from patient's cancer biopsy or surgery, are investigated for the evaluation of optimal personalized targeted chemotherapy regimen [reviewed in (3, 4)]. Alternatively, growing patient derived xenografts (PDXs) and evaluating response to targeted chemotherapies in vivo is also studied [reviewed in (5)]. Both methods have their limitations; particularly the shortage in human material taken from biopsy/surgery of small lesions (for the in vitro 3D approach) and length of time needed to obtain adequate number of PDX's to evaluate in vivo several targeted drug candidates. Therefore, there is an unmet need of a proper preclinical system that can be employed for assessing the optimal targeted single drug or combination from a list of omics-predicted targeted drugs. In addition, for the scenario that patient's tumor omics do not result in list of available targeted drugs, there is a need to assess the effect of quite a few off-label drugs on the patient's tumor to try to come with a possible candidate drug.
Head and neck cancer (HNC) is the sixth most common cancer worldwide where only 40–50% of the patients have a survival rate of nearly 5-years (6). For early stage disease, surgery and/or radiotherapy are the only standard of care (7). However, for locoregionally advanced stage disease, cisplatin-based chemo-radiotherapy remains the first treatment of choice while cetuximab is for platinum-based chemotherapy resistant patients (8, 9). For recurrent and metastatic disease, addition of cetuximab to platinum-based chemotherapy offers modest survival benefit (10). Cetuximab is the only FDA approved targeted monoclonal antibody against epidermal growth factor receptor (EGFR) for HNC patients. When administered along with platinum-based chemotherapy, it has no correlation to either EGFR copy number or level of EGFR expression in predicting its response (11, 12). Presently, in the myriad of treatment options, no universally agreed second line therapy exists.
In such a scenario, prediction of drug responses by employing patient derived xenograft (PDX) models has been done by many researchers, imparting ingenious advantages as a preclinical model (5, 13–17). Yet, as aforementioned, exploring drug efficiency in mice is costly as well as time taking in clinical decision making. Hence, oncology research with PDX model can be regarded as more suitable in drug validation upon drug screening (18). As discussed, extensive research efforts have been seen to develop ex vivo drug efficacy assays. Such efforts include isolation of fresh tumor cells from patients (19), patient derived 3D tumor spheroids (20, 21), or 3D organoids (22, 23), tumor tissue slices (24–27) and tumor tissue explants (28, 29). However, 3D tumor tissue explant culture seems to be more promising as it retains an intact tumor microenvironment.
In this study, we employed PDXs to develop and optimize a 3D tumor tissue explant culture and named it tumor ex vivo analysis (TEVA). TEVA, which is based on PDXs and 24 h of drug exposure, is reproducible, reliable, efficient, and rapid. The TEVA setting allows testing of numerous drugs and combinations in a robust manner, and predicts multiple drug responses accurately, as compared to in vivo treatment of the PDX. TEVA approach is different from other ex vivo approaches by putting emphasis on both (i) even size and volume of relatively large explants (2 × 2 × 2 mm3) allowing uniformity, reproducibility and a less divergent stroma/tumor ratio among tested samples; and (ii) performing the assay on tumor source taken from PDX (preference to first generation PDX), thus allowing testing numerous single drugs and combinations in a robust manner and after having the genomics data. Overall, our data provides a potential rationale to develop TEVA as a predictive assessment of tumor response to therapy in HNC.
Materials and Methods
Sample Procurement
Six head and neck cancer patients were included in the study. Details of the patients are given in Table 1. Fresh tumor tissue samples were procured just after their surgery with patient consent and with Helsinki approval from Ear Nose and Throat unit, Soroka Medical Center, Israel and Rabin Medical Center, Israel. The numbers of the Ethics Committee approvals are 0372-15-SOR, 0421-16-SOR, 0103-17-SOR, and 0813-16-RMC. The samples were placed in serum free DMEM (Gibco) media for transport and then processed within 2–3 h from harvesting.
Mice and Generation of PDXs
Male NOD/SCID (Envigo-NOD.CB17-Prkdcscid/NCrHsd) mice were used for the study. The patient-derived tumor tissue samples were implanted subcutaneously in dorsal flanks of the mice to form the PDXs. Tumor take rate varied from 1 to 6 months. PDXs were maintained by passing the tumors in mice from first generation to subsequent generations. All animal experiments were done under Institutional Animal Care and Use Committee (IACUC) of Ben-Gurion University of the Negev (BGU's IACUC) according to specified protocols aiming to ensure animal welfare and reduce suffering. The Animal ethical clearance protocol number used for this research is IL-80-12-2015.
Ex vivo Tissue Explant Preparation and Culture
When the PDXs (preferably first generation PDX) reached ~500 mm3, they were subjected to Tumor ex vivo Analysis (TEVA). After excising out the PDXs aseptically from mice they were cut into 2 × 2 × 2 mm3 tissue explants and cultured in 48 well tissue culture plates for specific time point as indicated in the text. The DMEM culture media (Gibco) contained 20% FBS (Gibco), 1 mM sodium pyruvate (Biological Industries), 2 mM L-glutamine (Biological Industries), 1% penicillin/streptomycin/amphotericin (Biological Industries), 0.1 mM MEM non-essential amino acids (Biological Industries), 10 mM HEPES (Biological Industries), 1% BIO-MYC (Biological Industries) and 50 ug/ml gentamycin (Gibco). For drug treatment, the 2 × 2 × 2 mm3 explants were treated with different therapeutic drugs for 24 h in 48 well tissue culture plates in 37°C, 5% CO2.
Therapeutic Agents
BYL719 (2.5 uM) (AdooQ bioscience, A11328), Erlotinib (5 uM) (MedChem Express, HY-50896), Cetuximab (10 ug/ml) (Merck, kindly provided by Soroka Medical Center), Cisplatin (9 uM) (Teva Pharmaceutical Industries), Olaparib (5 uM) (MedChem Express, HY-10162), and 5FU (123 uM) (Sigma, F6627) were used for the study.
Tissue Microarray (TMA)
The treated or untreated 2 × 2 × 2 mm3 tumor tissue explants were then went through formalin fixed paraffin embedding (FFPE) process using automated tissue processing machine (Leica, Biosystems) as we previously described (30, 31). TMA blocks containing up to 24 tissues were made from donor paraffin tissue blocks using 3 mm T-Sue™ punch needles (Simport).
Immunohistochemistry Staining and Quantification
5 μm sections were cut from TMA blocks using fully automated rotary microtome (Leica RM2255) and then Hematoxylin (Mayer) and Eosin (1% alcoholic) [Pioneer research chemical Ltd, UK] staining was performed as described (32). For (IHC) staining, tissue sections were first deparaffinized by two rinses in xylene and 100% ethanol for 10 min each. After subsequent rinses in 70 and 50% ethanol for 5 min tissues were washed in ultrapure water and subjected to antigen retrieval at 95°C for 30 min using antigen unmasking solution, citrate buffer P.H 6.0 (Invitrogen). Sections were then washed and ImmPRESS universal reagent (Vector Laboratories, MP-7500) was used according to manufacturer's protocol for the blocking. After the blocking, sections were incubated with primary antibodies against human cytokeratin 14 (1:2,000, Abcam, cat no-ab181595), vimentin (1:100, Cell signaling Technology, cat no-5741), α-SMA (1:250, Abcam, cat no-ab5694), Ki67 (1:250, Vector laboratories, cat no-VP K451), p-ERK (1:250, Cell signaling Technology, cat no-4370) p-PRAS40 (1:250, Cell signaling Technology, cat no-2997). For the HRP conjugated second antibody step we followed the manufacturer protocol of ImmPRESS universal reagent. Then immunoreactivity was visualized by using DAB substrate kit (Cell Marque, cat no-957D-60), according to manufacturer protocol. TUNEL assay was done according to manufacturer protocol (TREVIGEN, cat no-4815-30-K). TMA images were taken by pannoramic scanner (3D Histech). Images were analyzed by HistoQuant™ software (3D Histech). For the analysis, tumor areas were annotated based upon cytokeratin 14 staining of images of the respective PDXs. For both Ki67 and TUNEL, the software can calculate the number of positive nuclei and the annotated area for each tissue and the value was expressed as object frequency (pcs/mm3). For p-ERK, p-S6, and p-PRAS40, the software calculated proportion of the stained area within the annotated area for each tissue, and the value were expressed as relative mask area (%).
Western Blotting
2 × 2 × 2 mm3 tumor tissue explants were lysed group wise with 50 mM HEPES (pH 7.4), 100 mMNaCl, 0.1% CHAPS, 1 mM DTT, and 0.1 mM EDTA and protease inhibitor cocktail (Calbiochem) containing buffer to extract the total cellular protein. Protein concentration was determined by Bradford assay (BIO-RAD, Protein Assay, cat no-500-0006). 30 μg of total cellular protein from each group were electrophoresed on 10% SDS–polyacrylamide gels followed by their transfer onto nitrocellulose membranes. The membranes were blocked with 10% skimmed milk in Tris Buffered Saline with Tween 20 (TBST) for 2 h at room temperature, followed by overnight incubation at 4°C with p-AKT (Cell signaling Technology, cat no-4060), p-ERK and β-actin (MP Biomedicals, cat no-0869100) diluted 1:1,000 in 1% BSA in TBST. Blots are then washed with TBST followed by incubation with Goat Anti-Rabbit IgG Polyclonal Antibody (HRP) (KPL) and Peroxidase AffiniPure Rabbit Anti-Mouse IgG (H + L) (Jackson ImmunoResearch LABORATORIES, INC.) at a dilution of 1:5,000 in TBST. SuperSignal West Pico Chemiluminescent Substrate (Thermo Scientific™) was used for developing blots and the images were taken using XRS+ imaging system (Bio-Rad). Densitometry analysis of the blots was done using ImageJ software. Values of p-AKT and p-ERK were represented normalized to β-actin (33).
In vivo Drug Efficacy
Patient derived xenografts were re-implanted subcutaneously into mice and when the tumors reached ~100 mm3, mice were randomized keeping two restrictions: average PDX size and SD in the different groups should be similar, and SD should be kept lower thus excluding from the experiment, prior to its beginning, mice bearing PDX with volumes at the edges of the PDX volume range. The mice were treated as follows: BYL719 (25 mg/kg, daily, orally) (AdooQ bioscience, A11328), erlotinib (50 mg/kg, daily, orally) (Glentham Life Sciences, GP3306), cetuximab (10 mg/kg, once every 5 days, ip) (Merck, kindly provided by Soroka Medical Center), cisplatin (2 mg/kg, twice weekly, ip) (Teva Pharmaceutical Industries), olaparib (50 mg/kg, daily, orally) (Glentham Life Sciences, GP0126), and 5FU (8 mg/kg, thrice weekly, ip) (Sigma, F6627). Tumor volume was measured on day 1 and thereafter every 3 days using digital calipers. Formula used for tumor volume calculation is (L × W × W) × (π/6) in which W is the smaller measurement of tumor size.
In vivo and ex vivo Scoring
Calculation of VitroF was based on the staining of Ki67 and TUNEL of tissue treated with control- DMSO (Ct) and treatment (Tr),
Calculation of VivoF was based on the rate of tumor growth on day 6 and end day of the experiment: Control Tumor Volume (CTV) and Treatment Tumor Volume (TTV).
Statistical Analysis
Graphical and statistical analyses were performed using GraphPad Prism 5.0 software. Statistical analysis of the data was performed using t-test, one way and two way ANOVA and including Bonferroni multiple comparison test as a post-hoc analysis after the ANOVA (with p-values of * < 0.05, ** < 0.01 or *** < 0.001 as indicated on the figures).
Results
Optimization of Culture Timings For TEVA
To identify the appropriate culture timings for TEVA, we studied tumor tissue from PDXs of 3 patients; SE-Pt#1, SE-Pt#2, and SE-Pt#3. After cutting the PDXs to 2 × 2 × 2 mm3 explants, they were cultured till 72 h in DMEM with 20% FBS culture media, and pathological analysis was performed. The work flow is shown schematically (Figure 1A). The tumor tissue structure of the explants was examined by hematoxylin and eosin (H&E) staining and tumor marker of cytokeratin 14 expression. Representative images from SE-Pt#2 showed that the tissue structure is well preserved after 24 h of culture, while longer culture for 48 and 72 h resulted in tissue degradation (Figure 1B, first and second panel lines). Further analysis showed no significant changes in the tumor microenvironment after 24 h of culturing as the level of vimentin and α-SMA, fibroblast markers remained unaltered (Figure 1B, third and fourth panel lines).
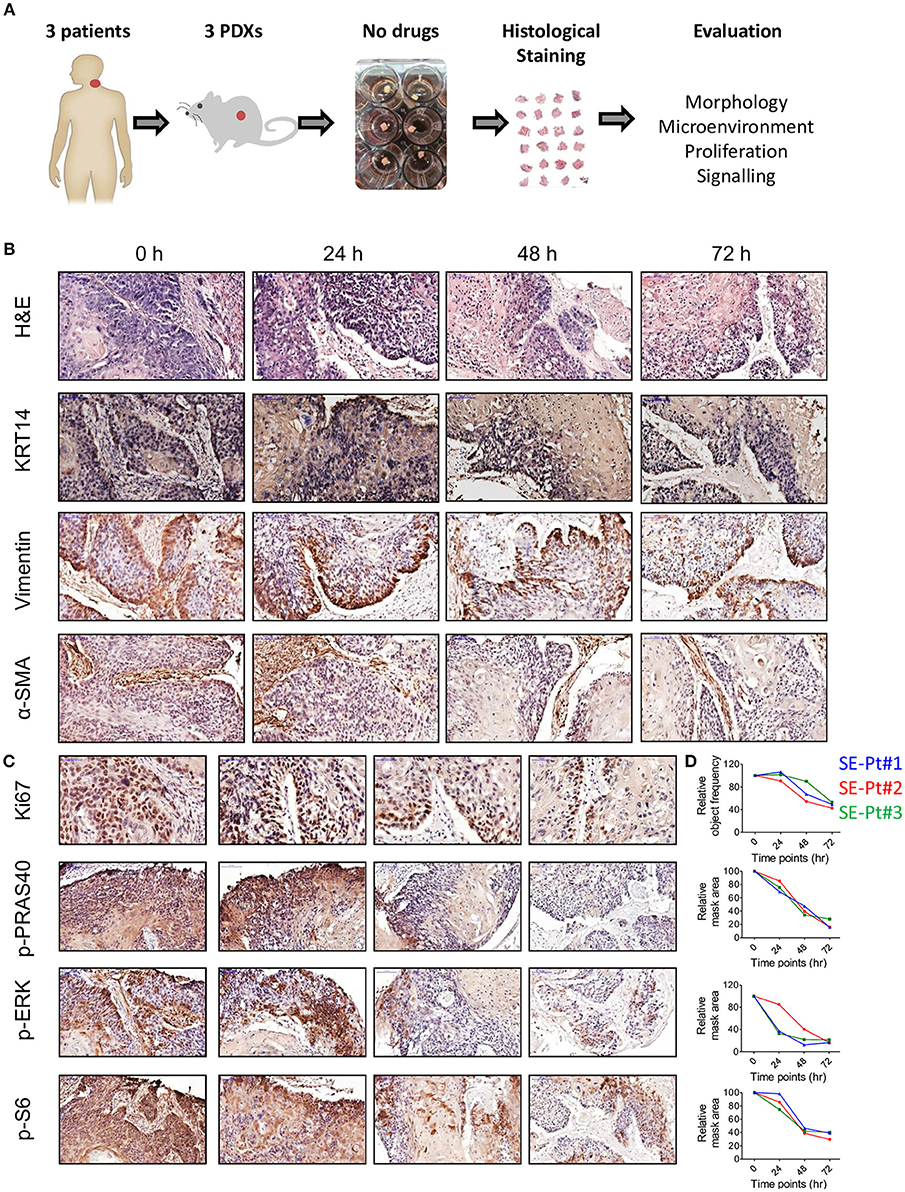
Figure 1. Ex vivo 2 × 2 × 2 mm3 tissue explants retain tissue morphology, tissue microenvironment, cell proliferation and cell signaling pathways. (A) Schematic diagram of the work flow. 2 × 2 × 2 mm3 tissue explants cut from the xenograft tumors of 3 HNC patients (SE-Pt#1, SE-Pt#2, and SE-Pt#3) were cultured for 24, 48, and 72 h in 400 μl DMEM media. At 0, 24, 48, and 72 h, explants were fixed, embedded and stained. (B) 2 × 2 × 2 mm3 tissue explants retain tumor morphology and tumor microenvironment. The tissue explants were stained for hematoxylin and eosin (H&E) and IHC staining was done for epithelial tumor cell marker, cytokeratin 14, fibroblast cell marker, vimentin and α-SMA (Magnification = 20×, bars = 100 μm). (C) 2 × 2 × 2 mm3 tissue explants retain cell proliferation and cell signaling pathways. The tissue explants were stained for cell proliferation marker Ki67 and cell signaling molecules p-PRAS40, p-ERK and p-S6 (For p-PRAS40, p-ERK and p-S6, magnification = 20×, bars = 100 μm and for Ki67, magnification = 40×, bars = 50 μm). (D) Graphs showing quantification of Ki67 positive cells; p-PRAS40, p-ERK and p-S6 positive areas in all three patient xenograft tumors at 0, 24, 48, and 72 h, respectively. Images were captured by pannoramic scanner and analysis was done by HistoQuant™ software.
Cell proliferation status was evaluated by Ki67 expression. Representative images from SE-Pt#2 showed no significant changes in Ki67 levels after 24 h of culture, while decrease in cell proliferation was observed after 48 and 72 h (Figure 1C, first panel line). Signaling activation of two key survival pathways was checked; the AKT/mTOR and MAPK. Quantification of p-PRAS40/p-S6 levels (indicators for AKT and mTOR, respectively), and of p-ERK (indicator for MAPK pathway), confirmed that after 24 h of culturing tumor tissue remain viable and active. To reinforce our observation, we validated our results in two other independent PDXs (SE-Pt#1 and SE-Pt#3) (Figure 1D). Overall the results showed that within the first 24 h of culture, cells in the tissue explants were able to maintain their viability, which allow us to conduct drug test.
Signaling Pathways Can Be Targeted in TEVA
To investigate if tumor tissue explants from PDXs can be used for ex vivo drug efficacy, effects of chemotherapy and two targeted therapies on signaling pathway inhibition was performed on SE-Pt#1. The 2 × 2 × 2 mm3 explants were cultured with a chemotherapeutic agent, cisplatin, an EGFR inhibitor, erlotinib, and a PI3K inhibitor, BYL719 for 24 h followed up by western blot and pathological analysis (Figure 2A). Western blotting analysis indicates that erlotinib inhibited MAPK pathway, indicated by reduction of p-ERK, while BYL719 inhibited the AKT pathway shown by reduction of the phosphorylation of AKT. IHC analysis of the tumors confirmed that the drug penetrated into the tumor, as the signaling pathways of tumors were inhibited with these agents. As expected, cisplatin exhibited no effect on AKT and MAPK pathways as the levels of p-AKT/p-PRAS40 and p-ERK levels sustained (Figure 2B). These pathway inhibition results were significantly evidenced by image analysis of the western blot and IHC results (Figures 2C–E, respectively).
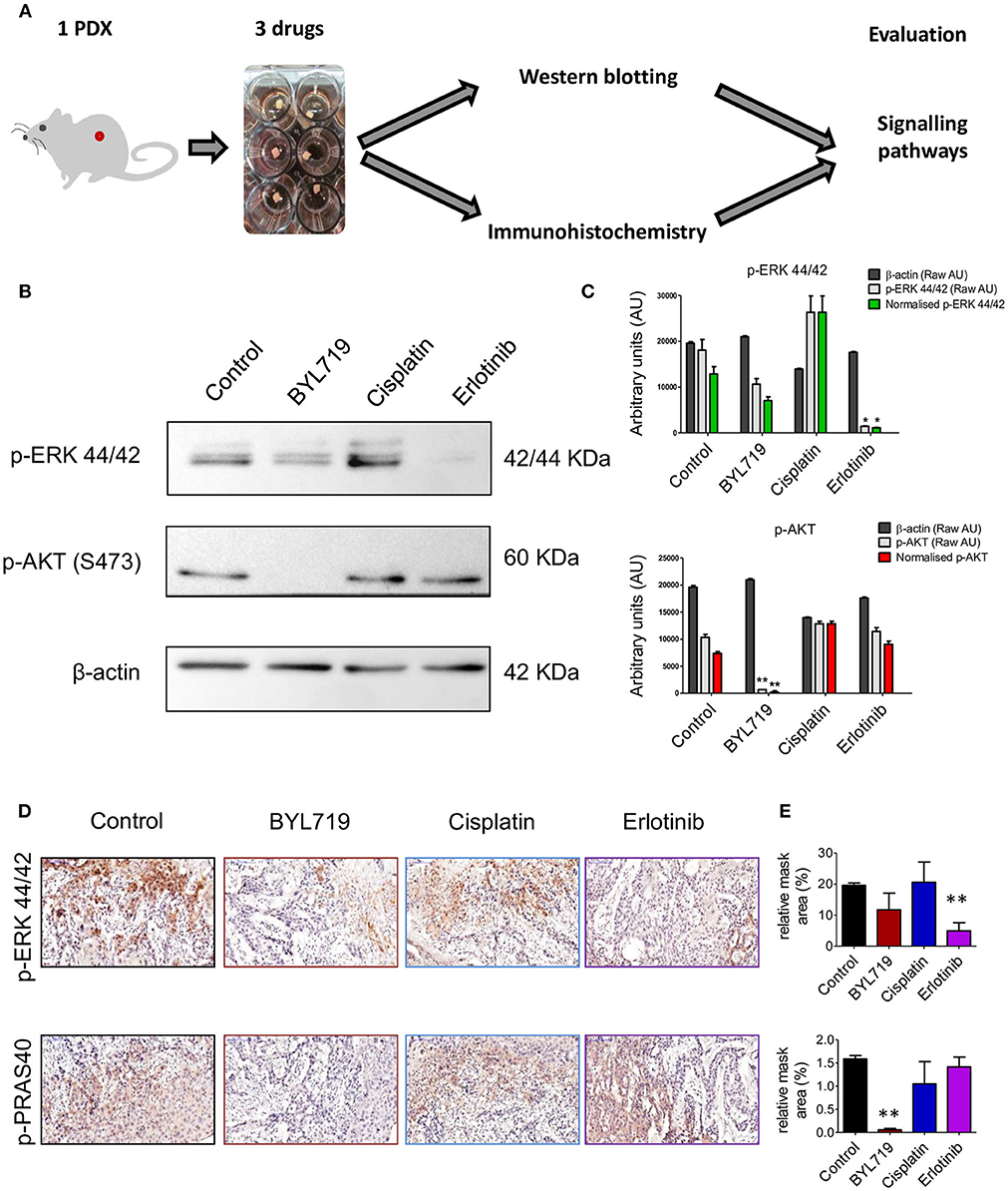
Figure 2. Signaling pathways can be targeted in ex vivo 2 × 2 × 2 mm3 tissue explants culture. (A) Schematic diagram of the workflow. 2 × 2 × 2 mm3 tissue explants from the xenograft tumor of SE-Pt#1 were cultured with EGFR inhibitor, erlotinib, PI3Kα inhibitor, BYL719 and chemotherapeutic drug, cisplatin for 24 h. Western blotting and immunohistochemistry were done to check the expression levels of the signaling proteins. (B) Western blotting was done with the protein lysates extracted from the tissue explants with protein lysis buffer to check the expression levels of p-ERK 44/42 and p-AKT. Representative images of the immunoblots were shown. (C) Representative graphs of densitometry analysis of the depicted immunoblots. Results are shown as arbitrary units (AU) of control β-actin, p-AKT, and p-ERK 44/42, obtained by densitometry analysis; Results are given both in raw AU (gray-shaded columns) and in β-actin-normalized AU (red/green color-shaded columns). (D) IHC staining was done from FFPE tissue explants to check the expression levels of p-ERK and p-PRAS40. Representative images were shown (magnification = 20×, bars = 100 μm). (E) Representative graphs showing quantification of p-ERK and p-PRAS40 positive areas of IHC images. Graphs representing mean ± SEM. *p < 0.05, **p < 0.01, Student's t-test, significantly different from control.
Correlation of Drug Testing Results From ex vivo and in vivo Assays
After establishing our explant culture system, we sought to explore if 24 h of ex vivo treatment will provide an indication on the potency of therapy in vivo. In order to do that, we tested side by side the effect of five different therapeutic agents (cetuximab, erlotinib, BYL719, cisplatin, and olaparib) on tumor cell viability ex vivo and tumor volume in vivo (Figure 3A). For this purpose we used a PDX (SE-Pt#4). For ex vivo cell viability staining, IHC of Ki67 (proliferation), and TUNEL (apoptosis marker) were performed. Figure 3B showed representative images of Ki67 and TUNEL positive cells of untreated control and treatment groups. Quantification of the Ki67 and TUNEL positive cells was performed by HistoQuant™ software. Note that between different FFPE cuts of the same explant and among different explants, the ratio of tumor vs. stroma could be different. Therefore, quantitative results for Ki67 and TUNEL staining are expressed as object frequency (pcs/mm2), which normalizes the results only for the tumor area in the scan (see Materials and Methods). We observed that of the 5 drugs tested, cetuximab treatment ex vivo showed the best effect in Ki67 assay and second-best in TUNEL, while erlotinib treatment showed the best effect in TUNEL assay and the worst effect in Ki67 (Figure 3C). These differences could represent a different balance in the drugs' effectiveness on proliferation vs. cytotoxicity. Therefore, we devised a formula that take into account both assays and defined a TEVA score (VitroF, see Materials and Methods) to assess the ex vivo results.
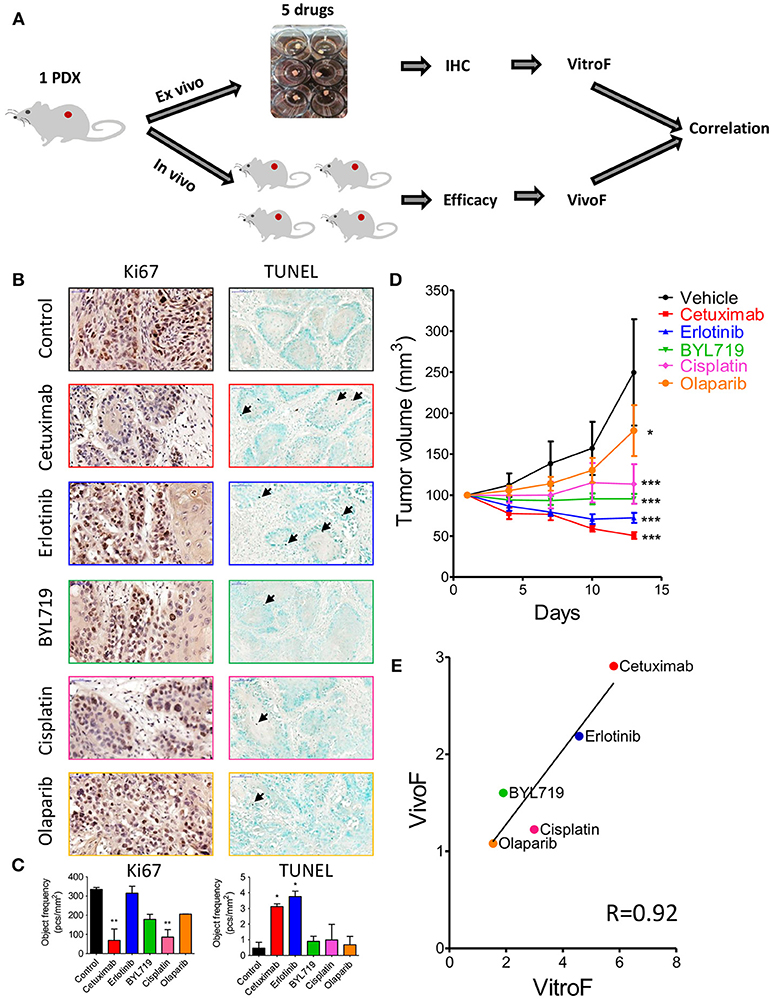
Figure 3. Response of 2 × 2 × 2 mm3 tissue explants of SE-Pt#4 to multiple therapeutic drugs correlates with the in vivo results. (A) Schematic diagram of the work flow. 2 × 2 × 2 mm3 tissue explants from the xenograft tumor of SE-Pt#4 were treated with cetuximab, erlotinib, BYL719, cisplatin, and olaparib for 24 h. TEVA score (VitroF) was derived based on IHC staining and analysis of Ki67 and TUNEL. In vivo score (VivoF) was derived based on efficacy studies on the xenograft bearing mice. (B) Representative IHC images of control and treated explants showing cell proliferation marker, Ki67 (magnification = 40×, bars = 50 μm) and apoptotic marker, TUNEL (magnification = 20×, bars = 100 μm). (C) Graphs showing quantification of Ki67 and TUNEL positive cells of control and treated explants, expressed as object frequency (pcs/mm2). Graphs representing mean ± SEM. *p < 0.05, **p < 0.01, One-way ANOVA with Bonferroni's post-hoc test, significantly different from control. (D) SE-Pt#4 xenografts bearing NOD/SCID mice were treated with vehicle, cetuximab, erlotinib, BYL719, cisplatin, and olaparib for 13 days. Tumor volumes (mm3) of vehicle and drug treated mice were shown in the tumor growth curve. Graphs representing mean ± SEM. *p < 0.05, ***p < 0.001, Two-way ANOVA with Bonferroni's post-hoc test, significantly different from vehicle. (E) Correlation data between VitroF and VivoF for each drug in SE-Pt#4 (GraphPad 5.0). The statistical significance of the correlation was determined using the correlation coefficient (R = 0.92). A linear regression line is shown in the plot.
To validate the drug screening results of TEVA in vivo, efficacy studies in NOD/SCID mice were performed. The PDX-bearing mice were allocated into 6 groups and treated with vehicle, cetuximab, erlotinib, BYL719, cisplatin, and olaparib for 13 days. Tumor volume was measured every 3 days (Figure 3D). To assess the in vivo effect and better compare it to the VitroF score of the ex vivo assay, we formulated an in vivo effect score termed as VivoF. Basically, the first and last measurements for each PDX were normalized to the average of vehicle-treated group and formulated together to give the VivoF (see Materials and Methods). We then compared VitroF and VivoF (Figure 3E). Cetuximab treatment was the most potent treatment as assessed by both VitroF and VivoF and regression curve showed a significant correlation between VitroF and VivoF (R = 0.92) for all drugs. These results of SE-Pt#4 suggest that TEVA can successfully predict multiple drug responses.
To validate the ex vivo–in vivo correlation for multiple drugs observed for SE-Pt#4, we tested PDXs from 4 other patients (SE-Pt#2, SE-Pt#3, SE-Pt#5, and SE-Pt#6) with parallel treatment of 3 therapeutic drugs (Cetuximab, erlotinib, and BYL719). Figure 4A shows schematic diagram of the work flow. As described earlier, Ki67 and TUNEL were chosen to predict the drug responses in TEVA. To validate the drug screening results in TEVA, in vivo studies were done with respective xenografts bearing NOD/SCID mice. The mice were allocated in each experiment into four groups and treated with vehicle, cetuximab, erlotinib, and BYL719 for 15 days. Tumor volume was measured every 3 days. VitroF and VivoF were derived for each drug as described earlier. Overall the results showed a correlation between VitroF and VivoF (R = 0.47) for three drugs in four PDXs (Figure 4B). For erlotinib and BYL719, ex vivo–in vivo results are highly correlated whereas for cetuximab the level of correlation is not high. Results also showed that for erlotinib, SE-Pt#2 was most sensitive whereas SE-Pt#3 and SE-Pt#5 were less sensitive. On the other hand for BYL719, SE-Pt#5 was most sensitive whereas SE-Pt#2 was least sensitive (Figure 4C). These suggest that TEVA results are in concordance with the patient derived xenografts responses.
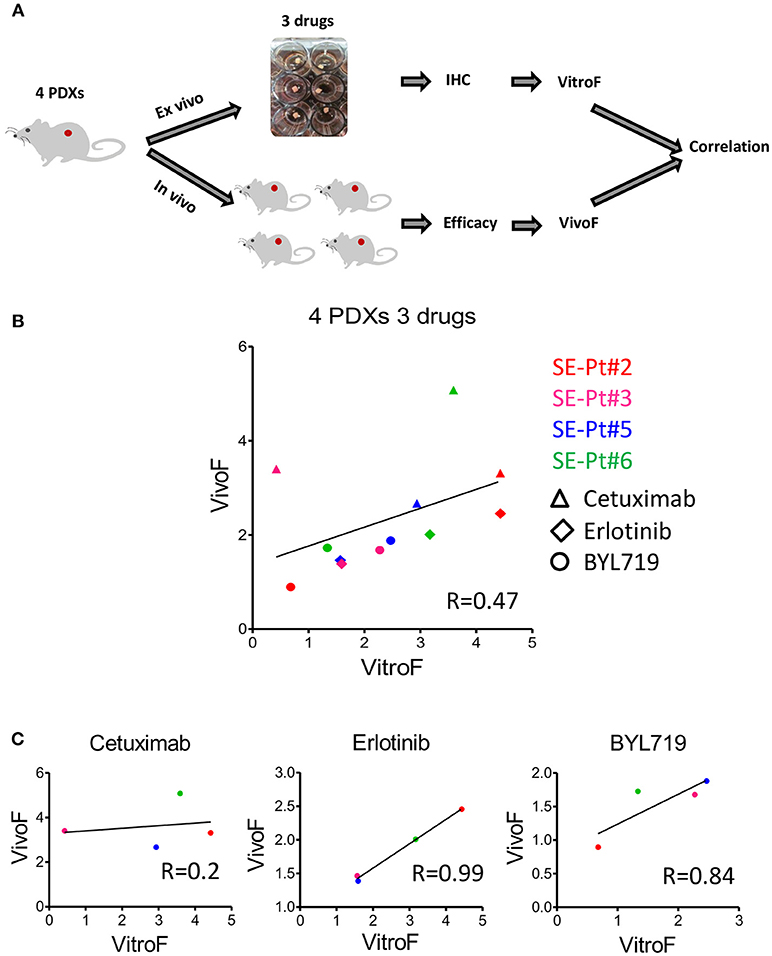
Figure 4. TEVA score correlates with the in vivo score for multiple drugs in multiple PDXs. (A) Schematic diagram of the work flow. 2 × 2 × 2 mm3 tissue explants from the xenograft tumors of four patients (SE-Pt#2, SE-Pt#3, SE-Pt#5, and SE-Pt#6) were treated with cetuximab, erlotinib, and BYL719 for 24 h. TEVA score (VitroF) was derived based on IHC staining and analysis of Ki67 and TUNEL. In vivo score (VivoF) was derived based on efficacy studies on the xenografts bearing mice of the four patients in different xenograft specific experiments. (B) Correlation data between VitroF and VivoF among three drugs in four patients (GraphPad 5.0). The statistical significance of the correlation was determined using the correlation coefficient (R = 0.47). A linear regression line is shown in the plot. (C) Correlation data between VitroF and VivoF in cetuximab (R = 0.2), erlotinib (R = 0.99), and BYL719 (R = 0.84) in four patients were shown (GraphPad 5.0). The statistical significance of the correlation was determined using the correlation coefficient. A linear regression line is shown in each plot.
Drug Combinations Can be Screened in TEVA
Finally we sought to test TEVA responses to combination of targeted and chemotherapeutic drugs. For this study, we chose SE-Pt#3 that displayed a modest response to erlotinib (Figure 4). To enhance the anti-tumor activity of erlotinib, it was combined with the chemotherapeutic drug, 5FU (Figure 5A). Figure 5B showed representative images of Ki67 and TUNEL positive cells of untreated control and treatment groups. Quantification of the Ki67 and TUNEL positive cells showed that the combination of erlotinib and 5FU has significantly high number of TUNEL positive cells than the control although no significant effect on Ki67 levels (Figure 5C). To validate the drug screening results in TEVA, in vivo studies were done with SE-Pt#3 xenografts bearing NOD/SCID mice. The mice were allocated into four groups and treated with vehicle, erlotinib, 5FU and combination of erlotinib and 5FU for 14 days. Tumor volume was measured every 3 days and represented in a tumor growth curve where the combination of erlotinib and 5FU showed significant decrease in the tumor volume with respect to vehicle (Figure 5D). VitroF and VivoF were derived for each drug as described earlier. Regression curve showed a significant correlation between VitroF and VivoF (R = 0.99) for each drug in SE-Pt#3 (Figure 5E), suggesting that TEVA can successfully predict responses of drug combinations.
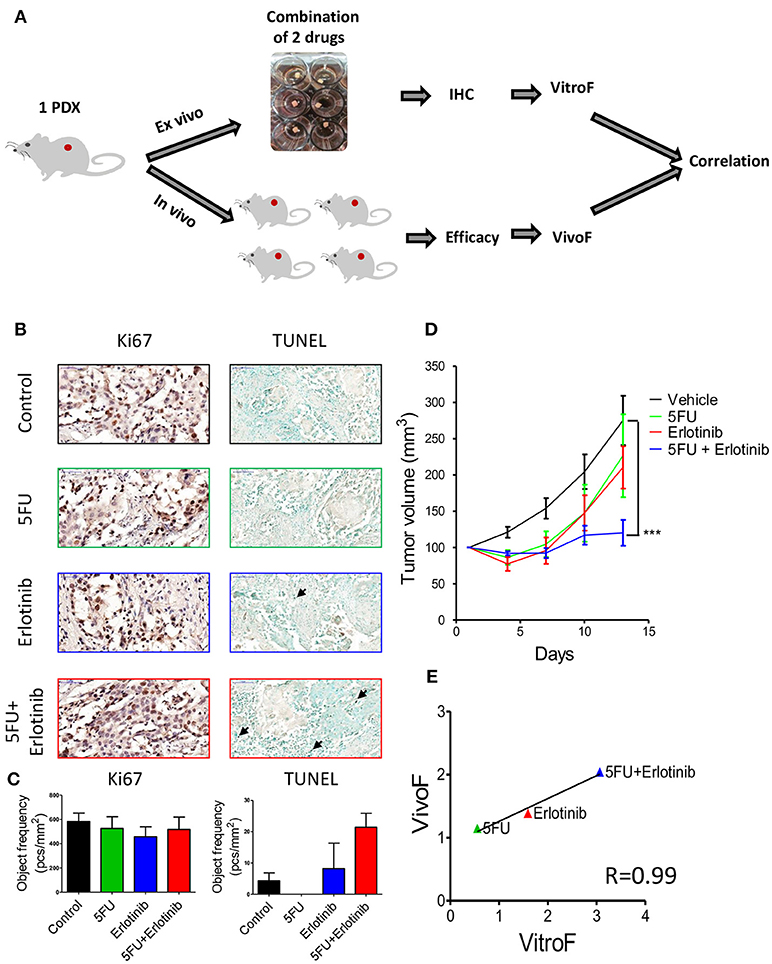
Figure 5. Response of 2 × 2 × 2 mm3 tissue explants of SE-Pt#3 to combination of two drugs correlates with the in vivo results. (A) Schematic diagram of the work flow. 2 × 2 × 2 mm3 tissue explants from the xenograft tumor of SE-Pt#3 were treated with 5FU, erlotinib and combination of 5FU and erlotinib for 24 h. TEVA score (VitroF) was derived based on IHC staining and analysis of Ki67 and TUNEL. In vivo score (VivoF) was derived based on efficacy studies on the xenograft bearing mice. (B) Representative IHC images of control and treated explants showing cell proliferation marker, Ki67 (magnification = 40×, bars = 50 μm) and apoptotic marker, TUNEL (magnification = 20×, bars = 100 μm). (C) Graphs showing quantification of Ki67 and TUNEL positive cells of control and treated explants, expressed as object frequency (pcs/mm2). (D) SE-Pt#3 xenografts bearing NOD/SCID mice were treated with vehicle, 5FU, erlotinib and combination of 5FU and erlotinib for 14 days. Tumor volumes (mm3) of vehicle and drug treated mice were shown in the tumor growth curve. Graphs representing mean ± SEM. ***p < 0.001, Two-way ANOVA with Bonferroni's post-hoc test, significantly different from vehicle. (E) Correlation data between VitroF and VivoF for each drug in SE-Pt#3 (GraphPad 5.0). The statistical significance of the correlation was determined using the correlation coefficient (R = 0.99). A linear regression line is shown in the plot.
Discussion
Tailoring therapy based on omics has a significant impact in medical oncology that enlarges the number of therapeutic strategies (34–36). Precision medicine using targeted therapy is subjected to two major challenges: biomarker of response (beyond genomic alteration), and acquisition of resistance [reviewed in (37)]. Taking together the recent development of cancer genomic and precision medicine, oncologists face a daily problem to select the optimal and most potent drug to each cancer patient. Hence, a functional platform that will help in decision making is urgently needed. Here, we suggest such a platform that potentially can be used to screen many drugs efficiently. We showed that a rapid and a low-cost ex vivo system, termed as TEVA, which is based on patient's PDX material, can mimic the efficacy of therapy assessment in mice. Since TEVA can rapidly evaluate the efficacy of numerous single drugs or combination based on material harvested from only one PDX (e.g., first generation PDX), TEVA potentially can assist in guiding personalized treatment, in relatively short time and from an extended list of omics-predicted targeted chemotherapy as well as off-label drugs.
Recently many studies has been done by employing patient derived xenografts (PDXs) from HNC, breast cancer, ovarian cancer, lung cancer to urological cancer patients for preclinical studies (5, 13–17). PDXs are successful in drawing the attention of the cancer biologists as it maintains more similarities in terms of structure, genetic profile and heterogeneity to the parental tumor than 2D monolayers (38). Despite all the ingenious advantages of PDX models in oncology research, these models also have major drawbacks to predict patient outcome alone (39). To fulfill the need of ex vivo drug screening to predict patient outcome, many studies have been done with patient derived tumor spheroids (20, 21), 3D organoids (22, 23), tumor tissue slices (24–27) and tumor tissue explants. These models have obviously advanced our understanding of the tumor behavior and bridge the gap between cell line/xenograft studies and actual efficacy in patients (18). Moreover, drug screening in tumor tissue explants hold the promise to maintain intact tumor microenvironment. Recently an ovarian cancer study using cryopreserved tumor tissue explants showed evidence of intact tumor microenvironment. The group successfully predicted carboplatin treatment and combination of carboplatin and 4-MU response in chemo sensitive vs. chemo resistant tissues (29). Another study of lung carcinoma using fresh tumor tissue explants from patients also showed that the tissue architecture is maintained. They showed cisplatin treatment response in the explants to be correlated with the survival of the patients and their outcome (28). However, this platform needs to be more perfected for multiple therapeutic drug screening.
In our study, we have perfected the ex vivo tumor explant culture platform using PDXs. In HNC, expansion of tumor mass as a PDX is required due to the limited tissue volume available from the surgery. The cultivation effect on the explants showed that the most favorable time point to see the optimal drug response in our system was 24 h. To prolong the viability of the tumor explants ex vivo, the culture media was supplemented with different growth factors. However, for the purpose of this study, exposure of tumors to therapeutic drugs for 24 h was found to be sufficient to detect drug efficacy. Specifically, we showed a potent signaling pathway inhibition after 24 h of treatment with anti-PI3K and EGFR drugs in all parts of the 2 × 2 × 2 mm3 explants.
Our data also showed that TEVA can patient-specifically predict multiple drug responses as well as drug combination effects and mimics in vivo results. For predicting drug response in TEVA, we used the concept of tumor microarray and generated TMA blocks capable of containing up to 24 whole explants. Panoramic scanner enabled us to scan the whole TMA images, reducing the chance of biasness of taking pictures of specific areas of interest in other microscopes and quantification of the TMA images was done by HistoQuant™ software. These all techniques enabled us to compare explant responses to different therapeutic drugs along with untreated solvent control (DMSO) on one single slide. Thus, differential drug responses can be predicted more accurately by TEVA within just few days, surpassing the need of PDXs in near future. However, it will be important to evaluate the explant response in a larger cohort of patients and it will be interesting to see the explant response to resistant drugs in the patients who failed first line therapy.
Note that for head and neck cancer resected samples, the time taken from getting the resected sample to having first generation PDX model in appropriate size, varies from 2 to 4 months and from PDX model to do TEVA to get personalized therapy data usually takes 3–4 days including analysis. This timeframe might not suffice for adjuvant therapy of head and neck cancer that usually begin 6–8 weeks after surgery. However, in the context of other malignancies, this time frame allows TEVA to be feasible in (i) guiding personalized treatment in the context of adjuvant therapy given 2–4 months after surgery (when TEVA is performed from first generation PDX), (ii) following relapse (including head and neck cancers), and as aforementioned (iii) patients with advanced tumors who failed first line therapy (including head and neck cancers).
Among all the drugs tested, we found that for cetuximab alone, TEVA results not always mimics in vivo results. Cetuximab is an anti-EGFR human-mouse chimeric IgG1 antibody. The Fc region of cetuximab can bind to the Fc-receptors on different immune cells, including NK cells and thus to induce antibody-dependent cell-mediated cytotoxicity (ADCC) (40). Thus, cetuximab can enhance the ADCC of NK cells and studies proved that NOD/SCID mice do have 25.2% NK cells (41). This could be a possible reason of the enhanced activity of cetuximab in vivo irrespective of its direct effect on the tumor cells. Therefore, for chemotherapy and small molecules-based targeted therapy, we expect TEVA to fully mimic in vivo results. For evaluating mAb-based targeted therapy in PDX-mice vs. TEVA, we should be somewhat cautious. Human IgG1, and to a lesser extent human IgG4, can bind effectively to murine CD64 (FcγRI) (42), expressed by murine macrophages and neutrophils. Thus, we should bear in mind that for some humanized mAbs, assessed for potency of targeted therapy in PDX mice vs. TEVA, and recognizing moieties on the cancer cells, ADCC could be more efficient in the PDX-bearing mice as compared to the TEVA.
In conclusion, we developed and optimized a rapid, low-cost, reliable and reproducible PDX-based tumor ex vivo culture system (TEVA) which can predict multiple drug responses. It can also be used for large scale drug screening as well as a platform for preclinical evaluation of new anti-cancer drugs. Based on the availability and size, this method can be done also directly on patient samples, allowing much lesser time in predicting drug response. Thus, in future this method can be used in conjunction with genomics data or independently as a personalized system to predict patient specific drug responses.
Author Contributions
AP and ME were behind the concept of the study. SG, AP, and ME did the study design. Data acquisition was done by SG, KK, MP, JZ, LC, KY, B-ZJ, BL, OD, AB-D, and AM. Data analysis and interpretation was done by SG, KK, LC, VL, ME, and AP. Manuscript preparation and editing was done by SG, KK, ME, and AP. Manuscript review was done by ME and AP.
Funding
This work was supported by the Israel Science Foundation grant 1188/16 (AP), the US/Israel Binational Science Foundation grant 2015344 (AP), the Israeli Ministry of Science and Technology/German Cancer Research Center program Grant CA172 (AP), the Israeli Ministry of Science and Technology grant 54180 (AP). This work was also funded by the Israel Cancer Association (ICA, 20170024) (to ME), the Israeli Cancer Research Foundation (ICRF, 17-1693-RCDA) (to ME), Israel Science Foundation (ISF, 700/16) (to ME), United State—Israel Binational Science Foundation (BSF, 2017323) (to ME). ME is supported by an Alon Fellowship for outstanding young researchers.
Conflict of Interest Statement
The authors declare that the research was conducted in the absence of any commercial or financial relationships that could be construed as a potential conflict of interest.
References
1. Link W. Anti-cancer drugs: discovery, development and therapy. In: de Mello RA, Tavares Á, Mountzios G, editors. International Manual of Oncology Practice: (iMOP)–Principles of Medical Oncology. Cham: Springer International Publishing (2015). p. 81–94.
2. Ibrahim R, Pasic M, Yousef GM. Omics for personalized medicine: defining the current we swim in. Expert Rev Mol Diagn. (2016) 16:719–22. doi: 10.1586/14737159.2016.1164601
3. Aberle MR, Burkhart RA, Tiriac H, Olde Damink SWM, Dejong CHC, Tuveson DA, et al. Patient-derived organoid models help define personalized management of gastrointestinal cancer. Br J Surg. (2018) 105:e48–60. doi: 10.1002/bjs.10726
4. Kodack DP, Farago AF, Dastur A, Held MA, Dardaei L, Friboulet L, et al. Primary patient-derived cancer cells and their potential for personalized cancer patient care. Cell Rep. (2017) 21:3298–309. doi: 10.1016/j.celrep.2017.11.051
5. Byrne AT, Alférez DG, Amant F, Annibali D, Arribas J, Biankin AV, et al. Interrogating open issues in cancer precision medicine with patient-derived xenografts. Nat Rev Cancer (2017) 17:254–68. doi: 10.1038/nrc.2016.140
6. Cognetti DM, Weber RS, Lai SY. Head and neck cancer. Cancer (2008) 113:1911–32. doi: 10.1002/cncr.23654
7. Marur S, Forastiere AA. Head and neck squamous cell carcinoma: update on epidemiology, diagnosis, and treatment. Mayo Clin Proc. (2016) 91:386–96. doi: 10.1016/j.mayocp.2015.12.017
8. Aung KL, Siu LL. Genomically personalized therapy in head and neck cancer. Cancers Head Neck (2016) 1:2. doi: 10.1186/s41199-016-0004-y
9. Tejani MA, Cohen RB, Mehra R. The contribution of cetuximab in the treatment of recurrent and/or metastatic head and neck cancer. Biologics (2010) 4:173–85. doi: 10.2147/BTT.S3050
10. Vermorken JB, Mesia R, Rivera F, Remenar E, Kawecki A, Rottey S, et al. Platinum-based chemotherapy plus cetuximab in head and neck cancer. N Engl J Med. (2008) 359:1116–27. doi: 10.1056/NEJMoa0802656
11. Licitra L, Störkel S, Kerr KM, Van Cutsem E, Pirker R, Hirsch FR, et al. Predictive value of epidermal growth factor receptor expression for first-line chemotherapy plus cetuximab in patients with head and neck and colorectal cancer: analysis of data from the EXTREME and CRYSTAL studies. Eur J Cancer (2013) 49:1161–8. doi: 10.1016/j.ejca.2012.11.018
12. Licitra L, Mesia R, Rivera F, Remenár É, Hitt R, Erfán J, et al. Evaluation of EGFR gene copy number as a predictive biomarker for the efficacy of cetuximab in combination with chemotherapy in the first-line treatment of recurrent and/or metastatic squamous cell carcinoma of the head and neck: EXTREME study. Ann Oncol. (2011) 22:1078–87. doi: 10.1093/annonc/mdq588
13. Owonikoko TK, Zhang G, Kim HS, Stinson RM, Bechara R, Zhang C, et al. Patient-derived xenografts faithfully replicated clinical outcome in a phase II co-clinical trial of arsenic trioxide in relapsed small cell lung cancer. J Transl Med. (2016) 14:111. doi: 10.1186/s12967-016-0861-5
14. Inoue T, Terada N, Kobayashi T, Ogawa O. Patient-derived xenografts as in vivo models for research in urological malignancies. Nat Rev Urol. (2017) 14:267. doi: 10.1038/nrurol.2017.19
15. Chen K, Ahmed S, Adeyi O, Dick JE, Ghanekar A. Human solid tumor xenografts in immunodeficient mice are vulnerable to lymphomagenesis associated with Epstein-Barr virus. PLoS ONE (2012) 7:e39294. doi: 10.1371/journal.pone.0039294
16. Hidalgo M, Amant F, Biankin AV, Budinská E, Byrne AT, Caldas C, et al. Patient-derived xenograft models: an emerging platform for translational cancer research. Cancer Discov. (2014) 4:998–1013. doi: 10.1158/2159-8290.CD-14-0001
17. Gao H, Korn JM, Ferretti S, Monahan JE, Wang Y, Singh M, et al. High-throughput screening using patient-derived tumor xenografts to predict clinical trial drug response. Nat Med. (2015) 21:1318. doi: 10.1038/nm.3954
18. Meijer TG, Naipal KAT, Jager A, van Gent DC. Ex vivo tumor culture systems for functional drug testing and therapy response prediction. Fut Sci OA. (2017) 3:FSO190. doi: 10.4155/fsoa-2017-0003
19. Blom K, Nygren P, Alvarsson J, Larsson R, Andersson CR. Ex vivo assessment of drug activity in patient tumor cells as a basis for tailored cancer therapy. J Lab Autom. (2015) 21:178–87. doi: 10.1177/2211068215598117
20. Zhang Z, Wang H, Ding Q, Xing Y, Xu Z, Lu C, et al. Establishment of patient-derived tumor spheroids for non-small cell lung cancer. PLoS ONE (2018) 13:e0194016. doi: 10.1371/journal.pone.0194016
21. Lazzari G, Nicolas V, Matsusaki M, Akashi M, Couvreur P, Mura S. Multicellular spheroid based on a triple co-culture: a novel 3D model to mimic pancreatic tumor complexity. Acta Biomater. (2018) 78:296–307. doi: 10.1016/j.actbio.2018.08.008
22. Boj SF, Hwang CI, Baker LA, Chio II, Engle DD, Corbo V, et al. Organoid models of human and mouse ductal pancreatic cancer. Cell (2015) 160:324–38. doi: 10.1016/j.cell.2014.12.021
23. Pauli C, Hopkins BD, Prandi D, Shaw R, Fedrizzi T, Sboner A, et al. Personalized in vitro and in vivo cancer models to guide precision medicine. Cancer Discov. (2017) 7:462–77. doi: 10.1158/2159-8290.CD-16-1154
24. Majumder B, Baraneedharan U, Thiyagarajan S, Radhakrishnan P, Narasimhan H, Dhandapani M, et al. Predicting clinical response to anticancer drugs using an ex vivo platform that captures tumour heterogeneity. Nat Commun. (2015) 6:6169. doi: 10.1038/ncomms7169
25. Gerlach MM, Merz F, Wichmann G, Kubick C, Wittekind C, Lordick F, et al. Slice cultures from head and neck squamous cell carcinoma: a novel test system for drug susceptibility and mechanisms of resistance. Br J Cancer (2013) 110:479. doi: 10.1038/bjc.2013.700
26. Grinshpun A, Gavert N, Granit RZ, Masuri H, Ben-Porath I, Breuer S, et al. Ex vivo organ culture as potential prioritization tool for breast cancer targeted therapy. Cancer Biol Ther. (2018) 19:645–8. doi: 10.1080/15384047.2018.1450114
27. Roife D, Dai B, Kang Ya, Perez MVR, Pratt M, Li X, et al. Ex vivo testing of patient-derived xenografts mirrors the clinical outcome of patients with pancreatic ductal adenocarcinoma. Clin Cancer Res. (2016) 22:6021–30. doi: 10.1158/1078-0432.CCR-15-2936
28. Karekla E, Liao W-J, Sharp B, Pugh J, Reid H, Le Quesne JP, et al. Ex vivo explant cultures of non-small cell lung carcinoma enable evaluation of primary tumor responses to anticancer therapy. Cancer Res. (2017) 77:2029–39. doi: 10.1158/0008-5472.CAN-16-1121
29. Ricciardelli C, Lokman NA, Sabit I, Gunasegaran K, Bonner WM, Pyragius CE, et al. Novel ex vivo ovarian cancer tissue explant assay for prediction of chemosensitivity and response to novel therapeutics. Cancer Lett. (2018) 421:51–8. doi: 10.1016/j.canlet.2018.02.006
30. Yossef R, Gur C, Shemesh A, Guttman O, Hadad U, Nedvetzki S, et al. Targeting natural killer cell reactivity by employing antibody to NKp46: implications for type 1 diabetes. PLoS ONE (2015) 10:e0118936. doi: 10.1371/journal.pone.0118936
31. Elhaik Goldman S, Moshkovits I, Shemesh A, Filiba A, Tsirulsky Y, Vronov E, et al. Natural killer receptor 1 dampens the development of allergic eosinophilic airway inflammation. PLoS ONE (2016) 11:e0160779. doi: 10.1371/journal.pone.0160779
32. Bersudsky M, Luski L, Fishman D, White RM, Ziv-Sokolovskaya N, Dotan S, et al. Non-redundant properties of IL-1α and IL-1β during acute colon inflammation in mice. Gut (2014) 63:598. doi: 10.1136/gutjnl-2012-303329
33. Kundu K, Dutta K, Nazmi A, Basu A. Japanese encephalitis virus infection modulates the expression of suppressors of cytokine signaling (SOCS) in macrophages: implications for the hosts' innate immune response. Cell Immunol. (2013) 285:100–10. doi: 10.1016/j.cellimm.2013.09.005
34. Xue Y, Wilcox WR. Changing paradigm of cancer therapy: precision medicine by next-generation sequencing. Cancer Biol Med. (2016) 13:12–8. doi: 10.20892/j.issn.2095-3941.2016.0003
35. Kou T, Kanai M, Matsumoto S, Okuno Y, Muto M. The possibility of clinical sequencing in the management of cancer. Jpn J Clin Oncol. (2016) 46:399–406. doi: 10.1093/jjco/hyw018
36. Hyman DM, Taylor BS, Baselga J. Implementing genome-driven oncology. Cell (2017) 168:584–99. doi: 10.1016/j.cell.2016.12.015
37. Tyson DR, Quaranta V. Beyond genetics in personalized cancer treatment: assessing dynamics and heterogeneity of tumor responses. Per Med. (2013) 10:221–5. doi: 10.2217/pme.13.6
38. Pompili L, Porru M, Caruso C, Biroccio A, Leonetti C. Patient-derived xenografts: a relevant preclinical model for drug development. J Exp Clin Cancer Res. (2016) 35:189. doi: 10.1186/s13046-016-0462-4
39. Jung J, Seol HS, Chang S. The generation and application of patient-derived xenograft model for cancer research. Cancer Res Treat. (2018) 50:1–10. doi: 10.4143/crt.2017.307
40. Taylor RJ, Saloura V, Jain A, Goloubeva O, Wong S, Kronsberg S, et al. Ex vivo antibody-dependent cellular cytotoxicity inducibility predicts efdicacy of cetuximab. Cancer Immunol Res. (2015) 3:567–74. doi: 10.1158/2326-6066.CIR-14-0188
41. Ito M, Hiramatsu H, Kobayashi K, Suzue K, Kawahata M, Hioki K, et al. NOD/SCID/γ mouse: an excellent recipient mouse model for engraftment of human cells. Blood (2002) 100:3175–82. doi: 10.1182/blood-2001-12-0207
Keywords: head and neck cancer, patient derived xenografts, ex vivo, explant culture, targeted therapy
Citation: Ghosh S, Prasad M, Kundu K, Cohen L, Yegodayev KM, Zorea J, Joshua B-Z, Lasry B, Dimitstein O, Bahat-Dinur A, Mizrachi A, Lazar V, Elkabets M and Porgador A (2019) Tumor Tissue Explant Culture of Patient-Derived Xenograft as Potential Prioritization Tool for Targeted Therapy. Front. Oncol. 9:17. doi: 10.3389/fonc.2019.00017
Received: 18 October 2018; Accepted: 07 January 2019;
Published: 22 January 2019.
Edited by:
Giovanna Schiavoni, Istituto Superiore di Sanità (ISS), ItalyReviewed by:
Natalie Julie Serkova, University of Colorado, United StatesSilviya Petrova Zustiak, Saint Louis University, United States
Copyright © 2019 Ghosh, Prasad, Kundu, Cohen, Yegodayev, Zorea, Joshua, Lasry, Dimitstein, Bahat-Dinur, Mizrachi, Lazar, Elkabets and Porgador. This is an open-access article distributed under the terms of the Creative Commons Attribution License (CC BY). The use, distribution or reproduction in other forums is permitted, provided the original author(s) and the copyright owner(s) are credited and that the original publication in this journal is cited, in accordance with accepted academic practice. No use, distribution or reproduction is permitted which does not comply with these terms.
*Correspondence: Moshe Elkabets, moshee@bgu.ac.il
Angel Porgador, angel@bgu.ac.il
†These authors have contributed equally to this work
‡These authors have contributed equally to this work