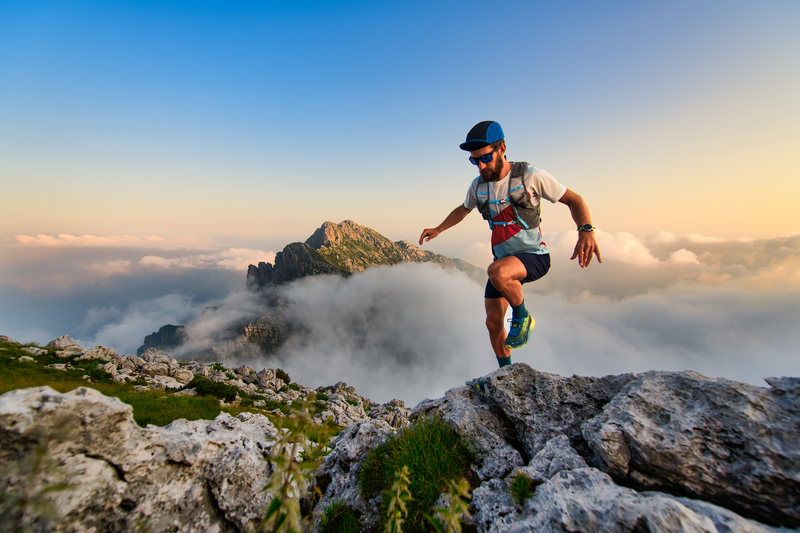
95% of researchers rate our articles as excellent or good
Learn more about the work of our research integrity team to safeguard the quality of each article we publish.
Find out more
REVIEW article
Front. Nutr. , 16 July 2019
Sec. Nutrition and Food Science Technology
Volume 6 - 2019 | https://doi.org/10.3389/fnut.2019.00107
This article is part of the Research Topic The Sustainability Challenge: New Perspectives on the use of Microbial Approaches and their Impact on Food and Feed View all 12 articles
The production of dairy, meat, and fiber by ruminant animals relies on the biological processes occurring in soils, forage plants, and the animals' rumens. Each of these components has an associated microbiome, and these have traditionally been viewed as distinct ecosystems. However, these microbiomes operate under similar ecological principles and are connected via water, energy flows, and the carbon and nitrogen nutrient cycles. Here, we summarize the microbiome research that has been done in each of these three environments (soils, forage plants, animals' rumen) and investigate what additional benefits may be possible through understanding the interactions between the various microbiomes. The challenge for future research is to enhance microbiome function by appropriate matching of plant and animal genotypes with the environment to improve the output and environmental sustainability of pastoral agriculture.
The arrival of the next generation sequencing (NGS) era has opened up new opportunities for understanding biological processes and implementing new strategies for improving these processes and monitoring the environmental impacts of agriculture (1–7). This has enabled the development of tools to implement genomic selection (GS) particularly in the livestock industry, and provide genome wide association studies (GWAS) to further elucidate genomic regions of importance in production traits. Genome assemblies, together with re-sequencing, have helped to establish SNP arrays for assessing genetic variation within and between genomes of individuals. This has become well-established for diverse breeds and species from around the globe in animal [e.g., (8–13)], and plant species [e.g., (14–18)]. In addition, genomic selection methods (19–29) used in conjunction with imputation strategies (30–33) that utilize various SNP densities in a cost effective manner, encourage the uptake of GEBVs by the breeding industry with the view to increase the rate of genetic gain in both animals (22) and forage plants (34). Furthermore, utilization of genotypes that are imputed to a whole genome sequence equivalent level for use in GWAS and GS are now a reality (35–39). Continued reductions in DNA sequencing costs together with an improvement in longer read technology has generated more refined genome assemblies that are being annotated at the functional level via assays designed to establish chromatin architecture, accessibility, modification and subsequent transcription and translation profiles (40–45). The human and mouse ENCODE projects (46–49) have paved the way for the international consortium FAANG [Functional Annotation of the Animal Genome; (50, 51)], which aims to identify all the functional elements in animal genomes. A significant challenge in the post-genomic era is connecting genotype to quantitative phenotype in basic and applied biology, represented as the genome to phenome challenge. Understanding the genotype to phenotype link is not only important from a genomic selection perspective but also assists in improving the fundamental understanding of the biology of the system.
The use of genomic tools in livestock research has had a substantial effect on genetic gain for the industry (22). Similar technologies are now being developed and implemented in forage species (34, 52). However, the animal and plant genomes constitute only two components of the “pasture to plate ecosystem.” They exist and function in association with their own microbiomes, and the microbiome of the soil on which pastures are grown and animals graze. The Human Microbiome Project has lead the way in characterizing the contributions that microbiomes make to host phenotypes, with an ever-increasing list of human attributes which are influenced by microbial activities [e.g., (53–69)]. Subsequently, NGS technology has advanced research in the characterization and understanding of the microbiome of the rumen of grazing animals (70), as well as the microbiome of forage plants and soils. Microbiome characterization initially involved sequencing of marker genes within microbial communities mainly targeting rRNA gene sequences but has grown to include deep metagenomic and metatranscriptomic sequencing. This has allowed global characterization of both culturable and unculturable microbial species within an environment coupled with quantification of their gene expression which enables functional profiling. In addition to understanding the contribution microbiomes make to production in grazing animals, genomic techniques can also be extended to monitoring sources of food or water contamination, thereby having important potential impacts for human health.
The integration of genomic information from host organisms with their microbiomes and with other environmental parameters of the target ecosystem has become an important challenge to research projects seeking to enhance agricultural output while reducing its environmental footprint. These host × microbiome × environment relationships in agricultural production systems involve extremely broad and complex interactions along the soil-plant and animal continuum, and its investigation needs to be divided into more specific research questions to enable detailed dissection and analysis. In this context, an “ecological genomics” approach is appropriate, whereby the microbiomes associated with soil, plants and animals are recognized as an integral part of an interconnected system that influence the functions of their hosts and thereby contribute significantly to productive processes in the pastoral sector (71). The key features of, and interactions between the soil-plant-animal microbiomes need to be identified so that their contributions to these agricultural processes and their impacts on the environment can be quantified.
In this paper, we consider the recent developments in genomics that provide new tools to understand the microbiome along the soil-plant-animal continuum within the pastoral production system. We summarize how these tools provide more precision in the identification and quantification of the structure of the microbial communities and how the emerging tools in metagenomics can be applied. Within the soil-plant-animal continuum we look at the animal and farm management opportunities arising from advanced understanding of microbial diversity and ecosystem function and how that can be used to improve soil processes, forage growth and pasture utilization and help withstand the challenges of diseases and climate change. These opportunities are summarized via three case studies involving: the microbiomes of the soil, the pasture, and the rumen of grazing animals. The potential for interdependencies, interplay and interactions between the microbiomes of the ecosystems along this continuum are considered along with other downstream impacts on ecosystems associated with water runoff. We finally propose how an “ecological genomics” approach can contribute to improved understanding of these microbiomes to improve the performance of the pastoral sector.
The biology of soils has long been recognized as being central to the productive capacity of natural and managed ecosystems (72, 73). While we cannot directly observe much of the soil microbiome, its function shapes the world around us. Soils microbiomes are highly diverse ecosystems, comprising complex assemblages of bacteria, archaea, and eukaryotic taxa, and are considered the most genetically diverse ecosystems on earth (74). Estimates of the total of life in soil vary widely; bacterial species alone, are present in the order of thousands to tens-of-thousands of species (inferred from 16S rRNA gene phylotypes) per gram of agricultural soil (74–76). Soils provide a reservoir of microbial species that may either support or inhibit the growth of plants and animals directly; as beneficial symbionts or as pathogens, respectively, or indirectly via actions which affect the biological availability of nutrients and toxins (77). Furthermore, functions supported by the soil microbiome provide a range of enabling and provisioning ecosystem services that support the natural environment, including interaction between above- and below-ground terrestrial biomes, aquatic ecosystems (rivers, lakes, groundwater), and the earth's atmosphere [e.g., (78)].
New soil management approaches are aimed at opportunities based on the understanding of soil microbiomes for improved processes and lowered environmental impact (79, 80). These management strategies increasingly use ecological genomics approaches (81) where soil is treated as an ecosystem hosting a rich diversity of species which harbor diverse “functional” genetic elements (e.g., genes conferring antibiotic resistance or nitrogen fixation). Assessing these at an ecosystem level is technologically challenging and requires the development of new bioinformatic and statistical tools for ecological analysis [e.g., (82, 83)]. Most importantly, an ecological genomics approach necessitates a shift in conceptual thinking from the organism or gene using ecosystem property/function interactions, to embracing the complexity of interactions among organisms, their genetic elements, and the biotic and abiotic factors that are expressed collectively to deliver ecosystem processes (84, 85). Ecological genomics offers a key opportunity to further advance the understanding of soil ecology and function and thereby help unravel the complexity of their ecosystems across spatial and temporal scales (86–88).
The application of molecular-based tools has become essential to characterize and understand soil ecosystems, because the soil is hyper-diverse and therefore genetically complex. A single gram of soil is estimated to contain up to 1,000 Gbp of metagenome DNA (80, 89) and current NGS platforms can only provide partial coverage of the metagenomic DNA in a soil sample. To date, most soil metagenome research has relied on the characterization of specific elements within the metagenome. Examples include the use of meta-barcoded primers to assess community composition [e.g., (90)], application of NGS or high-density environmental microarrays to determine functional status/composition of the community [e.g., (91, 92)], or functional screening of libraries of cloned DNA fragments for novel enzymes and bioactive compounds (93).
A good example illustrating metagenomics applications to study the soil microbiome involves soils suppressive to soil-borne plant diseases. These are defined as those in which the activity of the resident soil microbiota reduces the occurrence or severity of plant disease caused by soil-borne pathogens (94). Examples of such disease reduction in soils include suppression in wheat of take-all (Gaeumannomyces graminis var. tritici) and Rhizoctonia bare patch (Rhizoctonia solani AG-8) diseases, and the role Streptomyces spp. in the plant rhizosphere and endosphere play in promoting plant growth and the induction of resistance via antibiotic production and competitive exclusion (95). Given the high cost of soil-borne disease on agricultural production [e.g., estimated costs of 28–50% of pasture production in New Zealand; (96, 97)] and the lack of practicable and economic control options, the development of disease suppression in soil microbial communities represents an important soil service that serves to maintain agricultural activity and the food and fiber it produces (86). Disease suppression has been observed in a number of soils, with different disease-host interactions, and can develop naturally over time (94, 98). In instances where disease suppression develops, it is underpinned by alteration in the soil microbial community structure toward a greater number of disease suppressive taxa, or expression of potential (latent) disease suppressive activity (86, 99). Not surprisingly, the development of disease suppression in soils is highly desirable, and there have been considerable efforts to understand how this can be facilitated through changes in system management [e.g., via fertilizer use and plant residue management; or via reduced tillage and crop rotation; (94, 100)]. However, the characterization of the community and functions associated with general disease suppression has been very difficult, particularly as they potentially represent a small fraction of the total microbial diversity in soils (101). Furthermore, in the case of “general” disease suppression (102) underpinned by phylogenetically diverse consortia of microbiota, functions such as lytic enzyme production, antibiotic secretion, and elicitation of plant defense mechanisms, may be collectively responsible (94).
Advances in understanding changes within the soil community during development of disease suppression are being supported through application of ecosystem genomic tools [see Dignam et al. (86) review]. These include the application of high density oligonucleotide microarrays (88), tag-based NGS (103), and shotgun metagenomics (104, 105). In each case, phylogenetically diverse microbial consortia were associated with disease suppression. Resolving these taxa against the rich background of soil microbial diversity would not have been possible without an ecological genomics approach.
More recently, the focus on assessment of soil-borne disease suppression has been extended from approaches focused on identifying the taxa responsible, toward assessing soil ecosystems based on functional genes. This has followed recognition that, in many instances, a phylogenetic description of a microorganism can be a poor reflection of the metabolic (functional) ability outside of its base metabolism. This is particularly important where functions, such as antibiotic production, antibiotic resistance, host compatibility, and virulence, are borne on mobile/transferrable genetic elements such as plasmids (106). The acquisition or loss of a plasmid can change the biology and wider ecology of individuals of the same species in the soil. In these cases, the identification of a species only indicates the presence of a “potential host” that may or may not harbor the functional genes of interest [e.g., (107)]. As such, the detection of multiple functional genes associated with disease suppression is likely to provide a richer understanding of the ecosystem potential for this important ecosystem function (108). To achieve this, technology platforms such as functional environmental microarrays (91) are being constantly updated to include information on genes either directly or putatively associated with disease suppression. These include many antibiotic production genes, such as phzF and phzA (phenazine), bacA (bacilysin), pabA (chloramphenicol), phlD (DAPG), lgrD (gramicidin), lmbA, (lincomycin), prnD (pyrolnitrin), strR (streptomycin), spaR (subtilin), and pcbC (β-lactam) genes, alongside sub-sets of existing gene probes for detection of lytic enzyme production, e.g., hcnB (cyanide formation) (109). By assessing the abundance and distribution of these genes in soil environmental DNA (eDNA) samples, the functional ecology of disease suppressive communities may be determined. Impacts of farm management practices on disease suppression can then be interpreted through the lens of functional changes in the soil biology. Over time, this knowledge is expected to provide novel opportunities for on-farm management of soil biological resources toward enhanced disease suppression. Furthermore, molecular-based tools may enable the rapid identification of soils suppressive to specific diseases. These soils will represent important natural resources enabling the transmission of disease suppression from one soil to another by deliberate soil inoculation (94).
Functional properties of the soil microbial ecosystem are being inferred based on the taxa present. Using phylogenetic marker gene information (e.g., 16S rRNA or ITS gene sequences), bioinformatic tools such as PICRUSt [Phylogenetic Investigation of Communities by Reconstruction of Unobserved States; (110)] and FUNGuild (111) can predict the metagenome level functional content (e.g., C and N cycling genes) or functional guilds (pathogens, saprotrophs, symbionts, etc.) to provide deeper ecological insights into the functional ecology of the data sets. The expansion of these and similar tools, alongside better reference data (annotated genomes) which these tools reference, will provide further cost-effective approaches to describe the ecology and functioning of complex ecosystems such as soils.
The transfer of microbial species from one soil to another can confer new ecosystem phenotypes. This has been well-established for various microbial species such as mycorrhizal fungi, plant pathogens, and rhizobia. The movement of these taxa among soils has direct impact on the productive capacity and success of various plant species in the receiving environment (112, 113). This demonstrates the potential to manage soil biology for specific production-based and/or environmental outcomes.
Soil biology is the “engine room” that recycles plant material, either from direct inputs (leaf fall, root senescence), or secondary deposition (animal manure, urine) (114). The nutrients in these materials are either recycled within the biosphere, or mineralized into the geochemical matrix of the soil (114). In terrestrial systems, the soil microbiology provides an interface between the biological and abiotic worlds, affecting movement of essential major and minor elements between the geologic reserves and the biosphere. As such, there are a broad range of opportunities to harness the potential of soil ecosystems to optimize nutrient cycling. These include increasing the supply of many major and minor essential elements for plant use, stimulating the long-term storage of carbon in soils and promoting “closed” nutrient cycling within specific environments such that N within NO3 and N2O, for example, stay on-farm. Given our current lack of understanding of soil biology, we still have only a rudimentary knowledge of the extent of species interactions that may potentially affect critical rate regulating biogeochemical transformations in the mineralization, immobilization, and cycling of nutrients and the coupling of nutrient cycles. Indeed, it is highly likely that cryptic species and/or functional processes will have hitherto unrecognized importance in many aspects of soil nutrient cycling.
Grassland composition and forage production is finely balanced under the influence of interactions among many factors (115). These include the physical environment of soil, water, nutrient availability, temperature, extreme climatic events [e.g., (116)], pasture management of the grazing process (117), plant genetics (118), and the soil and plant microbiomes (see case study 1, above). Pasture-based livestock industries are primarily based on relatively simple mixtures of temperate grass and legume species as the main feed source for ruminant animals. Yet even these “simple” vegetation communities vary greatly in space and time (119), often for reasons that are not obvious using traditional scientific monitoring or analytical methods.
Well-studied components of the microbiome in grass-legume pastures involve the symbiotic association of Epichloë endophytes in grasses and Rhizobium nodules on legume roots. We illustrate ecological interactions involving these critically important microbiome components with an example based upon pasture dynamics under dairy cattle grazing in a warm-temperate region of New Zealand.
New knowledge of the ecology of pasture communities in northern New Zealand has revealed a clear instance where the microbiome drives change in community structure, with consequent feedback loops that engage other microbial communities. Sustained high densities of the root-feeding insect pest black beetle (Heteronychus arator) since 2007/08 in the Waikato and Bay of Plenty regions of New Zealand (120), combined with other stress factors, particularly increasing summer-autumn soil moisture deficit (121), has led to widespread but spatially disaggregated instances of near-complete failure of pastures based on perennial ryegrass (Lolium perenne). For example, when a ryegrass population contains a strain of the endophyte Epichloë festucae var. lolii [formerly Neotyphodium lolii (122)] that offers minimal protection against the insect pest black beetle, pasture collapse is observed within 2 years after sowing (123). In contrast, when the ryegrass population contains an Epichloë endophyte strain effective against black beetle, ryegrass populations are maintained (124).
A signature of ryegrass failure is the content of white clover (Trifolium repens) in the pasture. When white clover is sown together with ryegrass cultivars containing the endophyte strain AR1 (which does not protect against black beetle), the white clover content of the pasture increases rapidly. In contrast, in pastures sown with perennial ryegrass containing endophytes that are effective against black beetle such as wildtype endophyte or strain AR37 (125) the ryegrass/clover balance is stable. The plant genome does not explain this different survival pattern, although there can be subtle host genotype x endophyte strain interactions that mediate the speed and scale of change (126). The outcome of clover dominance, resulting from reduced competitive pressure from the grass (127) leads to Rhizobium symbiosis becoming a dominant process in the community. This illustrates the connectivity between the plant and soil microbiomes—mediated through ecological processes, in this case competition. Furthermore, clover dominance changes the nutritional composition of the feed eaten by livestock, reducing total fiber content and increasing soluble protein (128), which in turn creates a change in the rumen microbiome where the microbial composition changes in response to the altered substrate. This change results in a further interaction involving the microbiome impacting on the host animal. A direct outcome of this interaction is greater ammonia release in the rumen, flowing through to increased excretion of surplus nitrogen via higher urinary nitrogen concentrations (129) and heightened risk of nitrate leaching from beneath the urine patches returned to the grazed pasture (130).
This example illustrates the influence of, and in some case control by, the microbiome on the productivity and sustainability of a pastoral ecosystem. It highlights feedback loops, precipitated by a mis-match between the plant microbiome and the environment, led to the transformation of a grass-dominant and relatively nitrogen-efficient pasture to a legume dominant pasture with a leaky nitrogen cycle. The new pasture state with strong clover content may increase total herbage accumulation (compared with a ryegrass-dominant sward) in the short term. However, if clover contribution remains in the range of 15–30% of herbage mass (131), the consequences for the long-term yield potential of the system cannot be easily predicted. For instance, the new pasture composition may accentuate patch selection if grazing animals express partial preference for clover (132, 133). The resulting bimodal frequency of pasture mass observed in cattle grazed systems [e.g., (134)] will likely reduce the total production of the system (135). Questions that then arise include: Where and how should we intervene to manipulate the microbiome in an ecological system such as this? With what purpose and consequences? What benefit can we expect, relative to the manipulation of the plant or animal genome itself, from going down this pathway?
By virtue of converting human-indigestible plant polymers (cellulose and hemicellulose) into edible animal protein, ruminants enable high value food production from pasture plant resources. In the New Zealand context, ruminant animals are therefore an important part of the pastoral sector and produce a wide range of food and fiber products of considerable value to the economy. The digestion of plant material is achieved via the ruminant's specialized digestive systems, consisting of a multi-chambered stomach which supports the growth and fermentation activities of a diverse array of anaerobic microbes. The main digestive processes are carried out in the first two stomach compartments, called the reticulo-rumen, where microbes colonize and degrade forage plant material. The microbes ferment the released sugars into volatile fatty acids (VFA), which are absorbed from the reticulo-rumen and used by the animal to drive growth and formation of food and fiber products. The process is regulated so that only a partial fermentation occurs, allowing the ruminant host to absorb and utilize the intermediate fermentation products for its own metabolism and growth. The ruminant also benefits from the provision of vitamins and from the microbial cells flowing further down the digestive tract.
There has been a continual drive by livestock breeders and farmers to improve the efficiency of digestion in the rumen. Studies of the rumen microbiome have focused on understanding the contribution that the microbes make to the digestion and metabolism of particular feeds, or that are involved in production traits that are selected during animal breeding. However, microbiome analyses are increasingly being used to identify new ways to manipulate microbial metabolism, to enhance digestive capacity and drive greater output of food and fiber products by the host animal, while reducing waste or detrimental end products of the fermentation that have negative impacts on digestive efficiency, rumen function or the environment. There are many previous examples of microbial manipulations in ruminants to influence digestion, including additives such as buffers, antibiotics [ionophores and non-ionophores; (136–139)], methane inhibitors (140–148), vitamins, minerals, iso-acids, enzymes, and exogenous bacteria and/or yeast (149). These additives target different processes in the rumen and have varying degrees of effectiveness, depending on the ruminant species targeted and the diet fed to the animals. Many of these additives are non-selective or have unknown modes of action, and there is a need to have a better understanding of rumen microbiome responses so that these manipulations can be more precisely tailored for delivery of the desired improvements while removing, or minimizing, any unintended consequences.
Rapid advances in DNA and RNA sequencing, and new high throughput screening technologies for proteins and metabolites, are now making a complete description of the rumen microbiome an achievable goal (150). Combined with the ability to interpret the “omics” information using new bioinformatics approaches, this is transforming our understanding of the rumen microbial ecosystem (70, 151–154) and will inevitably lead to new ways of manipulating ruminal fermentation processes. Although these technologies are relatively new, they are being used to address recurring questions about the contribution the rumen microbiome makes to the nutritional functions of the ruminant. This will allow assessment of the types of microbes that are present, how many organisms are there, their relative quantities, and their functional role. Furthermore, as a better appreciation is gained of the importance of gastrointestinal microbes to their host, new questions around their protective, immunological, and developmental benefits to the host are being posed (155–157).
An example which illustrates the interactions between the host animal and its rumen microbiome involves methane yield differences in sheep that are related to expression of genes encoding the hydrogenotrophic methane formation pathway (158). Methane is produced in the rumen by the methanogenic archaea and is released from animals via eructation, or belching, and is also respired via the breath (159). Methane is an important agricultural greenhouse gas and has a global warming potential (GWP) of 28, meaning it is 28x the GWP of CO2. Agricultural methane emissions contribute ~14% of all anthropogenic emissions and therefore reducing emissions from ruminant animals is an important goal globally. While the main rumen methanogens are known, the process of methane formation is not clearly linked to either the number (160–162) or a particular community structure of methanogens (163, 164). However, it is known that the concentration of methanogenic substrates (mainly hydrogen and methyl compounds such as methanol and methylamines) and the interactions between methanogens and microbes producing and consuming hydrogen in the rumen (165, 166) are important factors contributing to methane emissions. To better understand methane formation, there has been a concerted effort to accurately measure methane emissions from ruminant animals, to examine the variation in methane yield (g methane/kg dry matter intake) between animals, and to assess the effects of different diets or dietary additives on methane output. Measurements made in sheep have shown methane yields vary considerably between individual animals within flocks (167–169), by as much as 34% between the low and high methane emission phenotypes. These variations in methane yield have been linked to differences in particle retention time in the rumen (167, 170, 171) and rumen volume (172). Furthermore, the variations were found to persist under different grazing conditions and to be a heritable trait in sheep (169). The genetic basis for the methane phenotype in sheep is indicative of a key interaction between the host animal and the rumen microbiome. Because methane is produced solely by the action of methanogenic archaea, rumen methanogens must make some contribution to the methane phenotype in sheep, either directly or via changes to the microbial community in the rumen.
To examine the contribution that the microbiome makes to methane yield, sheep with high or low emission status were rumen sampled and DNA and RNA were extracted to enable both metagenome and metatranscriptome analysis of their rumen microbiomes (158).
Surprisingly, these analyses showed no differences in the relative abundance of bacteria, archaea or eukaryotes between the low and high methane yield sheep (158). Even detailed genus-level analysis of methanogens showed only slightly elevated levels of Methanosphaera spp. in the low methane yield sheep and slightly higher Methanobrevibacter gottschalkii in the high methane yield sheep, however these were not sufficient to explain the differences in animal methane yield. An analysis of abundance of genes encoding the methanogenesis pathway also showed no significant differences, which confirmed the rRNA gene analyses. However, when the metatranscriptomic data were examined, there were clear increases in transcripts of genes encoding the methane metabolism pathway in high methane yield sheep. In particular, the genes encoding the hydrogenotrophic methanogenesis pathway (in which methane is formed from hydrogen and carbon dioxide) were significantly up-regulated compared to the methylotrophic methanogenesis pathway (where methane is formed from methyl compounds). Specifically, high methane yield sheep had high transcript levels of the methyl coenzyme M reductase enzyme (mcr, EC: 2.8.4.1) which catalyzes the final step in the methane formation pathway. A detailed comparison of these mcr genes found that they clustered into three distinct groups, called sheep rumen MCR groups 1, 2, and 3. The SRMR1 group of mcr genes were derived from a new group of rumen methanogens which belong to the order Methanomassiliicoccales. The SRMR2 group was identified as encoding an isozyme of methyl coenzyme M reductase (MCRII encoded by the mrt gene) and was found in both Methanobrevibacter spp. and Methanosphaera spp., while the SRMR3 was derived from Methanobrevibacter spp. only. The vast majority of methyl coenzyme M reductase transcripts were from the SRMR1 and SRMR3 groups and were 2.84- and 2.85-fold more abundant in high methane yield sheep, respectively, while SRMR2 transcripts were very low (158). These results showed that transcriptional up-regulation of the hydrogenotrophic methanogenesis pathway was an important microbial mechanism contributing to higher methane yield in sheep.
It makes biological sense that an up-regulation of methanogenesis genes in rumen methanogens results in more methane emissions from animals, but why does this happen in some sheep and not in other grazing animals? A possible mechanism has been proposed which incorporates differences in rumen size and feed particle retention time, leading to altered microbial growth kinetics and fermentation thermodynamics which affects ruminal dissolved hydrogen levels (165). It is proposed that low methane yield sheep have a smaller sized rumen, which causes increased particle passage rate that leads to higher rumen hydrogen concentrations (Figure 1). The higher hydrogen concentration causes a negative feedback that results in less hydrogen formation by fermentative microbes, leading to less methane formation. Conversely, high methane yield animals are predicted to have a larger rumen with slower particle passage, which results in lower hydrogen concentrations, enhanced hydrogen formation during fermentation, and more methane. Under ruminal conditions of slower particle passage rate and lower hydrogen concentrations, it is predicted that there is a higher turnover rate of a smaller hydrogen pool through the methanogenesis pathway to account for the elevated methane formed. The lower ruminal hydrogen concentration means that methanogens have to increase expression of methanogenesis genes to produce more enzymes to scavenge the hydrogen and maintain its turnover rate. This is because enzyme concentrations as well as substrate concentrations can limit the flux through a pathway, and increasing enzyme expression partially overcomes the limitation of lower substrate concentrations. Conversely, a high particle passage rate and high hydrogen conditions would require a lower level of expression of methanogenesis pathway genes to permit the same flux. More recent studies have shown that the dissolved hydrogen concentrations in the rumen are higher than predicted from calculations that assume equilibrium with the gas phase (173). The concentrations of hydrogen measured in vivo show that hydrogen is super-saturated in the rumen, and significantly affects the calculated ΔG of hydrogen-forming and hydrogen consuming reactions in the liquid phase of rumen (174).
The strong relationship between expression levels of the hydrogenotrophic methanogenesis pathways in rumen methanogens and methane yield in sheep, is the first example of rumen microbial gene expression being directly linked to an animal phenotype of relevance to environmental sustainability and production.
The case studies described above illustrate the complex nature of interactions within the microbiomes of the soil, plants and the rumen of grazing animals. The potential for further higher-level interdependencies and interactions between the microbiomes along the soil, plant and animal continuum dramatically increases the overall complexity in a wider ecological context. Associations between the microbiomes of soil, plants, above and below-ground animals, and the environment are massively complex. These involve soil “genotype” x plant genotype x animal genotype x rumen “genotype” × environment interactions. Thus, while there are many examples of the importance of management of individual and simple microbiomes, most ecosystem outcomes are supported by the activities of multiple consortia of microorganisms. These outcomes are the result of many microbial species and strains, with a collective functional capacity resulting in an altered ecosystem phenotype. The opportunities to harness these interactions are immense, and offer great potential if they can be understood, directed and actively managed.
Critically, the soil microbiome has a number of direct influences on plant performance. With the exception of seed-borne (vertically transmitted) endophytes, the soil biology provides the primary reservoir of microorganisms that colonize the root rhizoplane, rhizosphere, and ultimately the wider endophytic microbiome within the plant (175–179). The discovery of plant endophytes remains in its infancy, and estimates of 1 million endophytic species of higher plants may be reasonable (180). The consequences of the endophytic colonization of plants are profound, as the plant microbiome has wide ranging impacts on expression of plant phenotypes. Plant-associated microbiomes have been shown to confer drought tolerance (181), alter flowering phenology and timing (182, 183), influence plant shoot dry matter production (179), and induce systemic resistance to diseases (184). The interaction between the microbiome and plant genetics also affects aspects of plant quality via altering changes in the production of plant metabolites, or providing additional metabolic capacity via ancillary metabolic pathways encoded in the microbiome (185). An interesting example of interactions within the soil-plant microbiomes involves assessment of transgenic potato plants expressing an antimicrobial protein that is secreted into the apoplastic space between cells (186). While minor differences in the microbiomes were found in the rhizosphere of transgenic vs. non-transgenic plants, these changes were negligible compared to differences between non-transgenic plants of different potato cultivars. Another example is in flavor development in strawberries where the quantity and profile of their flavor is influenced by microbiome regulation of fuaranol synthesis (187, 188). Furthermore, the soil microbiome influences the microbial community on the grape berry and subsequent wine properties (189). It is also likely that microbiomes affect the quality of resins, fruit, honey, and essential oils (185). While the manipulation of plant traits through the microbiome have been vastly under-studied, this represents major opportunities for production of novel products or additional value of current products (190). Indeed, the microbiome background in which plants are grown can be seen to contribute to the wider terroir of the plant product, and may be used to add value to the provenance of products grown in different soils.
There are many direct and indirect interactions between below and above ground ecosystems, and these converge into terrestrial ecosystem function (191, 192). Collectively these express as an “ecotype,” or “functional status” to the soil. Across a multitude of functions, a “normal operating range” of soil ecosystems can be defined. These can be assessed by sampling across a range of sites to give a generalized understanding of the performance of a soil, allowing an assessment of measured vs. expected system function (81). This framework can be expanded to investigation of factors such as expression of plant-genotype effects, impacts on soil ecosystems due to disturbance (e.g., by humans or climate), and assessing ecosystem recovery. Future decisions about plant or cultivar selection for different farming systems (e.g., pastoral, arable, horticulture, and forestry) will include an understanding of the underlying soil biology. Furthermore, these decisions are likely to extend to precision use of fertilizers, agri-chemicals, and seed dressings (including biological ingredients), that consider the wider ecosystem parameters. These opportunities will need to find a balance between optimal output of products and sustainable environmental outcomes, which are not obtainable with the current laissez-faire approach.
Research is now striving to understand the interactions between the soil, plant, and animal microbiomes within different environmental situations (Figure 2). Such holistic, community-level approaches to assess complex, multi-trophic linkages and communication among microorganisms, plants, and animals, within a wide environmental spatiotemporal heterogeneity, will require application of a range of emerging tools and approaches such as those based on ecological genomics (71). These will need to deliberately embrace the inherent complexity of microbiomes as “meta-ecosystem” containing an assortment of biological elements (species, mobile genetic elements), with different functional potentials resulting in an overall ecosystem phenotype. An integrative ecological genomics approach, that explores interactions among and across these meta-ecosystems and their collective ecological control, will be required to translate the biology to useful applications in a control-analysis approach (81).
As indicated in the dairy pasture dynamics case study, animal production systems in New Zealand are based on year-round grazing of ruminants on pastures, which are dominated by perennial ryegrass-clover mixtures (125). There are clear connections between the plant and animal microbiomes via the ingestion and fermentation of plant material in the rumen. However, there is very little information on how the above ground plant microbiomes (i.e., endophytic and ectophytic microbes colonizing the internal and external parts of plant stems and leaves) affect digestive processes in the rumen. It is known that as soon as plant material enters the rumen, it is colonized by a succession of different rumen microbes which initiate digestion (193–195). There is also evidence that ingested plant material continues to metabolize and undergoes a cell death response which leads to DNA fragmentation and protein breakdown, independent of rumen bacterial activities (196). This autolytic plant protein breakdown contributes to the inefficient use of plant protein which can result in much of the ingested nitrogen being lost from the animal in the form of ammonia and urea, which can cause environmental problems when excreted from the animal. The types of endo- and ecto-phytic plant microbes entering the rumen, and their activities during the plant digestion process, are poorly understood. Knowing what type of microbes are carried into the rumen in, or on, plant forages may reveal opportunities for microbial manipulation of the plant autolytic processes, allowing for the enhancement of rumen microbial colonization of the plant material or improvement of the ruminal digestion process itself.
Investigation of plant microbiomes entering the rumen may also offer some new insights and perspectives into facial eczema (FE), a significant disease in ruminants caused by saprophytic fungi growing on the dead litter at the base of pastures. The fungus, Pithomyces chartarum, proliferates under the warm moist conditions typically found in late summer and early autumn, and produces large numbers of spores which are subsequently ingested by the animal. In the rumen, the spores release a mycotoxin, sporidesmin, which is absorbed and causes damage to the liver and bile ducts. The damaged liver is unable to breakdown chlorophyll normally and a toxin, phylloerythrin, builds up in the blood causing sensitivity to sunlight and skin inflammation, leading to the FE symptoms of skin irritation and peeling, lowered production and sometimes death of the animal. Current management practices for FE include treatment of stock with zinc sulfate (supplied via their drinking water, drenched as a liquid product or delivered via an intraruminal slow release capsule), or applying fungicide to pastures before the spore counts become too high. There are also animal breeding programmes underway to select for FE-resistant sheep and cattle. Non-toxigenic isolates of P. chartarum have also been investigated as potential competitive exclusion biocontrol agents under New Zealand conditions (197). While sporidesmin levels were reduced by the application of the non-toxigenic isolates to pastures, the percentage of such isolates declined from 90 to 54% of all P. chartarum isolates retrieved during the 19-week trial, and after 15 months represented only 4% of the isolates from treated plots. This indicates that non-sporidesmin-producing isolates did not persist in the environment, at least under the field conditions examined. These observations suggest that better understanding of the microbiomes associated with decaying plant material in pastures, and of the ingestion and subsequent degradation of spores in the animal gut, may lead to easier and more effective means of controlling this serious animal disease.
While the interactions between plant and ruminant microbiomes are obvious, it is less appreciated that ruminants also ingest significant quantities of soil. The amounts ingested by ruminants depends on the amount of soil that becomes attached to the portions of the plant grazed by the animal, which is influenced by the weather conditions and soil type. Soil ingestion is lowest in the summer months on soils with strong structure and is highest in the winter months on soils with poor structure, where there is greater opportunity for rainfall to mediate soil transfer to the above ground parts of the forage plants (198, 199). Soil ingestion was originally studied in relation to teeth wear in sheep, as particularly abrasive soil types contribute significantly to teeth wear, limiting the productive lifetime of ewes. These studies showed that ewes can ingest up to 400 g of soil per day (198), while similar studies carried out in dairy cows found they could ingest more than 2 kg of soil per day under certain conditions (199). Soil ingestion is also a major route of uptake of trace elements for ruminants (198). The best known example of this is the uptake of cobalt (Co) to alleviate the symptoms of “bush sickness” or “wasting disease.” Co is required in ruminant diets for the bacterial synthesis in the rumen of cobalamin, which is also known as vitamin B12. Cobalamin is an essential cofactor for the enzyme methylmalonyl-CoA mutase, involved in an important metabolic pathway of ruminants, converting propionic acid (one of the main VFA produced by the rumen fermentation) to glucose (200, 201).
Given that soil can contain 109-1010 organisms per g, the amount of soil ingested by ruminants reported above (198, 199) represents up to 4 ×1011 to 4 ×1012 soil microorganisms ingested by sheep per day, or 2 × 1012-2 × 1013 microorganisms per day by cattle. The potential impact of this ingested soil microbiome is estimated to be from ~8 to ~2.6% of the rumen microbiome of sheep and cattle, respectively. These estimates of soil-borne microbes entering the rumen are consistent with recent findings of a global rumen census, in which exogenous microbes (likely to be derived from ingested plant material, water or soil) on average made up ~3% of the rumen microbiome sequences (151). The changes in dairy pasture dominance by clover over ryegrass in Case Study 2 described above, has shown the indirect effects of soil microbes on ruminant production via altered forage species mix and elevated N fixation. However, there has been no examination of the direct influence that ingested soil microbes themselves might have in the rumen. The rumen and the soil environments have very different physico-chemical conditions which select for dissimilar microbiomes, therefore one would not expect soil organisms to survive, or be metabolically active, for long periods in the rumen. However, the effects of enhanced trace element supply to ruminants via ingestion of soils and the subsequent co-factor biosynthesis by bacteria in the rumen, suggest that some interactions of relevance to ruminant growth and health do occur at this interface, and are worthy of further characterization.
After digestion in the rumen, the remaining material is further fermented in the hind gut before being passed from the animal as dung. In the New Zealand dairy grazing system, dung is mainly deposited as “cow pats” onto the pasture, and eventually is broken down by a combination of microbial, insect and earthworm activity and incorporated into the soil or is volatized as ammonia. The amounts of manure produced by cattle varies considerably; beef cattle consuming 12 kg dry matter intake (DMI) per day produce about 5–6% of their body weight as manure each day (average ~27 kg wet weight), while dairy cattle with a 22 kg DMI produce closer to 70 kg per day. The microbial density in manure is roughly equivalent to the density in the rumen, but the phylogenetic distribution of microbes within the manure differs. While the rumen microbiome is usually dominated by Firmicutes and Bacteroidetes, manure can have altered Firmicutes to Bacteroidetes ratios (202) or elevated levels of Proteobacteria (203). The type of diet consumed by the animal also influences the composition of the fecal microbiome (204). Studies on the effects of manure deposited onto pastures of upland soils, indicate that dung deposition provides additional substrate for microbial growth and metabolism, and alters nutrient availability (205–207). The contribution of ruminant gut microbes in the manure, to these soil processes remains unknown, and represents a potential point of intervention to affect beneficial changes to the availability of nutrients from the soil.
While focusing on increasing the benefits of enhancing agricultural production through the microbiomes along the soil-plant-animal continuum, there is also a need to consider the potential effects of animal pathogens on human health. We know that changes in animal diets and/or farm systems can affect the zoonoses carried by farm animals (208–210). These zoonoses can impact on human health via multiple pathways. The first is direct animal contact which impacts predominantly on the people who work in the industry, as well as non-occupational contact (211). The second pathway is via contamination of the food products consumed (212, 213). The third major pathway is via water contamination (214) which in itself can exhibit three separate pathways such as drinking water (215), contact recreation (216) and irrigation of food crops (217). These outbreaks of zoonotic disease events can have considerable economic cost to the agricultural industries (218–220).
Genomic technologies present a major opportunity to have a transformational impact on environmental monitoring and food monitoring. This will result in increased use and adoption of genomics tools for diagnostic purposes associated with the monitoring of “risk microbes” involved with environmental health, food safety and well-being of people. Genomic techniques have already shown great potential in linking and understanding sources of food or water contamination (221–223). Highly sensitive targeted amplicon sequencing can readily detect specific pathogens and environmental metagenomics will generate huge data sets in which risk microbes can be identified.
Genomic technologies are extremely sensitive, therefore interpreting a positive signal for the presence of a DNA sequence in a sample becomes absolutely critical. There is an urgent need to establish “genomic thresholds” for water quality or food contamination to allow appropriate interpretation of genomic diagnostic data by environmental and food regulatory authorities. Similar issues are also posed for the implementation of genomic diagnostics in biosecurity decisions at border controls or clinician/veterinarian interpretations in human/animal health. A critical requirement will be the up-skilling of end-users in genomics to ensure that genomic-based diagnostic data can be effectively interpreted and appropriate actions implemented by stakeholders when enforcing policy decisions.
The principal advantage of using genomic tools to improve the understanding of microbiome interactions is the greater precision in identification and quantification of the structure of the microbial communities. Enhanced detection and characterization of the microbial members of each microbiome (the number of different species, the number of individuals within the species, and the detection of unculturable microbes), along with predictions of their metabolic capabilities from retrieved genomic information, will greatly enhance our understanding of microbiome community structure and function.
Assessment of species rank-abundance curves (RAC's) show that the soil microbiome contains many rare species (224, 225). This has particularly been brought to light with NGS-based community sequencing analysis; with increasing depth of sequencing, generally using SSU rRNA phylogeny, more species are discovered. That is, the tails of the RAC's generated for soil microbial ecosystems are very long. However, do these rare species matter in relation to soil-provided ecosystem function? In many cases, the rare biosphere is the reservoir of many novel lineages, colloquially referred to as “microbial dark matter” (226). Our understanding of these taxa is slight, particularly as many of these novel, and sometimes candidate phyla remain to be isolated in pure culture (227). As such, the ecological importance of the rare biosphere is unclear. Genomic analysis has shown that these taxa harbor unexpected metabolic features (226), and are therefore a potential source of novel enzymes and “stored ecosystem potential” (228). Furthermore, the recruitment of taxa, with unique ecophysiological adaptations, has been shown to be essential in recovery of soil ecosystem function after disturbance events, such as ammonia oxidation (229). Thus, the rare biosphere has wider impacts on ecosystem function than the total size of the community. It represents an important “seed bank” of organisms with which we may begin to have a functional role as opportunities arise, for example recruitment by a host plant or animal, or edaphic or environmental changes.
Numerous microbiome studies have been performed using rRNA gene targeted approaches. While this marker gene has worked well for many examples it is dependent on organisms within the sample having matches to the primer sequences used. New primer-independent, metagenomic shotgun sequencing approaches are rapidly increasing the volume of sequence data of microbiome samples across numerous environments along the soil-plant-grazing animal continuum. This is producing large databases of sequence information which is providing the science community with a significant resource for data mining to better understand these microbiomes, and will also act as a reference for characterization of future microbiomes. The continual increase in sequencing capacity at lower cost and access to constantly improving computational resources (e.g., more powerful data processing hardware and purpose-written, open source software) will allow new science questions to be asked about microbial functions in systems that were previously not possible. These advances will substantially improve the degree of replication and depth of sequencing required to cover the variables present in a given microbiome, or compensate for variation within samples that was not previously feasible (230).
The metagenomic shotgun sequencing of significant components of entire microbial communities is now becoming achievable at a reasonable cost. Coupled with improved computational power and bioinformatic analyses, this will dramatically improve investigation of microbiomes. Having the technologies to understand how the organisms within a microbiome interact to support ecosystem functions, such as nutrient cycling, is an exciting prospect and will undoubtedly lead to opportunities for discovery of novel microbiome features to improve ecosystem production and environmental outcomes. However, there are still significant hurdles to overcome (231, 232), and achieving a more comprehensive description of the soil-plant-grazing animal microbiome continuum would represent a remarkable advance in our ability to characterize and understand complex ecosystems.
Understanding of the various microbiomes that make up the soil-plant-grazing animal microbiome continuum involves a major effort. Integrating metagenomic data from multiple microbiomes to get a more holistic view of ecosystems is rare, but is beginning to be addressed. Trying to understand the soil-plant-grazing animal microbiome continuum requires a clear framework, informed by the answers to some key questions. These include: how do the different microbes within a microbiome contribute to the overall phenotype? how do the different microbiomes interact with each other? and how do we move to an ecosystem-wide approach to understand the (role of the) microbiomes across the ecosystem? While these questions are answered, the main metabolic pathways in each microbiome need to be characterized along with how the composition of the microbiome can predict the phenotype. Genomics has already allowed for a vast amount of data to be generated, but the knowledge on how to translate this genomic knowledge into a phenotype requires further attention.
With the ever increasing volume of data available for each microbiome sample, microorganisms present in minute quantities will be increasingly detected with greater accuracy. This will provide a greater understanding of the relationship between the quantity of microorganisms in a sample and the contribution (“quality”) or ecological “phenotype” of that organism. For example, do microorganisms present in high frequency (quantity) contribute more to the overall phenotype than microorganisms which are rare (quality)?
Improved understanding of the microbiome composition with respect to quantity and quality will raise potential options to manipulate the microbiome to our advantage. Examples include the potential of keeping nutrients in the soil through using diverse plant genotypes/ plant species to manipulate the microbiome. The challenge ahead is to use the expanding genomics knowledge not only to increase the resilience of pastoral systems (and pasture persistence) by manipulating the microbiome, but to achieve this with less environmental impact while maintaining or improving agricultural outputs.
All authors have contributed to the writing of this manuscript and read and approved the manuscript.
This work was supported by the National Science Challenge Our Land & Water New Zealand through contract A23168 to AgResearch.
The authors declare that the research was conducted in the absence of any commercial or financial relationships that could be construed as a potential conflict of interest.
We would like to thank the National Science Challenge Our Land & Water, New Zealand for funding for the writing of this review.
1. Brenner S, Johnson M, Bridgham J, Golda G, Lloyd DH, Johnson D, et al. Gene expression analysis by massively parallel signature sequencing (MPSS) on microbead arrays. Nat Biotechnol. (2000) 18:630–4. doi: 10.1038/76469
2. Liu L, Li Y, Li S, Hu N, He Y, Pong R, et al. Comparison of next-generation sequencing systems. J Biomed Biotechnol. (2012) 2012:251364. doi: 10.1155/2012/251364
3. Pettersson E, Lundeberg J, Ahmadian A. Generations of sequencing technologies. Genomics. (2009) 93:105–11. doi: 10.1016/j.ygeno.2008.10.003
4. Ronaghi M, Karamohamed S, Pettersson B, Uhlén M, Nyrén P. Real-time DNA sequencing using detection of pyrophosphate release. Anal Biochem. (1996) 242:84–9. doi: 10.1006/abio.1996.0432
5. Schuster SC. Next-generation sequencing transforms today's biology. Nat Methods. (2008) 5:16–8. doi: 10.1038/nmeth1156
6. van Vliet AH. Next generation sequencing of microbial transcriptomes: challenges and opportunities. FEMS Microbiol Lett. (2010) 302:1–7. doi: 10.1111/j.1574-6968.2009.01767.x
7. Voelkerding KV, Dames SA, Durtschi JD. Next generation sequencing: from basic research to diagnostics. Clin Chem. (2009) 55:641–58. doi: 10.1373/clinchem.2008.112789
8. Bovine Genome Sequencing and Analysis Consortium. The genome sequence of taurine cattle: a window to ruminant biology and evolution. Science. (2009) 324:522–8. doi: 10.1126/science.1169588
9. Jiang Y, Xie M, Chen W, Talbot R, Maddox JF, Faraut T, et al. The sheep genome illuminates biology of the rumen and lipid metabolism. Science. (2014) 344:1168–73. doi: 10.1126/science.1252806
10. Kijas JW, Townley D, Dalrymple BP, Heaton MP, Maddox JF, McGrath A, et al. A genome wide survey of SNP variation reveals the genetic structure of sheep breeds. PLoS ONE. (2009) 4:e4668. doi: 10.1371/journal.pone.0004668
11. Kijas JW, Lenstra JA, Hayes B, Boitard S, Porto Neto LR, San Cristobal M, et al. Genome-wide analysis of the world's sheep breeds reveals high levels of historic mixture and strong recent selection. PLoS Biol. (2012) 10:e1001258. doi: 10.1371/journal.pbio.1001258
13. The Bovine HapMap Consortium. Genome-wide survey of SNP variation uncovers the genetic structure of cattle breeds. Science. (2009) 324:528–32. doi: 10.1126/science.1167936
14. Byrne SL, Nagy I, Pfeifer M, Armstead I, Swain S, Studer B, et al. A synteny-based draft genome sequence of the forage grass Lolium perenne. Plant J. (2015) 84:816–26. doi: 10.1111/tpj.13037
15. Chagné D, Crowhurst RN, Troggio M, Davey MW, Gilmore B, Lawley C, et al. Genome-wide SNP detection, validation, and development of an 8K SNP array for apple. PLoS ONE. (2012) 7:e31745. doi: 10.1371/journal.pone.0031745
16. Hamilton JP, Hansey CN, Whitty BR, Stoffel K, Massa AN, Van Deynze A, et al. Single nucleotide polymorphism discovery in elite north American potato germplasm. BMC Genomics. (2011) 12:302. doi: 10.1186/1471-2164-12-302
17. The Potato Genome Sequencing Consortium. Genome sequence and analysis of the tuber crop potato. Nature. (2011) 475:189–95. doi: 10.1038/nature10158
18. Winfield MO, Allen AM, Burridge AJ, Barker GLA, Benbow HR, Wilkinson PA, et al. High-density SNP genotyping array for hexaploid wheat and its secondary and tertiary gene pool. Plant Biotechnol J. (2016) 14:1195–206. doi: 10.1111/pbi.12485
19. Auvray B, McEwan JC, Newman SA, Lee M, Dodds KG. Genomic prediction of breeding values in the New Zealand sheep industry using a 50K SNP chip. J Anim Sci. (2014) 92:4375–89. doi: 10.2527/jas.2014-7801
20. Daetwyler HD, Swan AA, van der Werf JH, Hayes BJ. Accuracy of pedigree and genomic predictions of carcass and novel meat quality traits in multi-breed sheep data assessed by cross-validation. Genetics Select Evol. (2012) 44:33. doi: 10.1186/1297-9686-44-33
21. Endelman JB, Schmitz Carley CA, Bethke PC, Coombs JJ, Clough ME, da Silva WL, et al. Genetic variance partitioning and genome-wide prediction with allele-dosage information in autotetraploid potato. Genetics. (2018) 209:77–87. doi: 10.1534/genetics.118.300685
22. Hayes BJ, Bowman PJ, Chamberlain AJ, Goddard ME. Invited review: genomic selection in dairy cattle: progress and challenges. J Dairy Sci. (2009) 92:433–43. doi: 10.3168/jds.2008-1646
23. Kemper KE, Reich CM, Bowman PJ, vander Jagt CJ, Chamberlain AJ, Mason BA, et al. Improved precision of QTL mapping using a nonlinear Bayesian method in a multi-breed population leads to greater accuracy of across-breed genomic predictions. Genetics Select Evol. (2015) 47:29. doi: 10.1186/s12711-014-0074-4
24. Meuwissen THE, Hayes BJ, Goddard ME. Prediction of total genetic value using genome-wide dense marker maps. Genetics. (2001) 157:1819–29.
25. Meuwissen T, Hayes B, Goddard M. Accelerating improvement of livestock with genomic selection. Annu Rev Anim Biosci. (2013) 1:221–37. doi: 10.1146/annurev-animal-031412-103705
26. Moser G, Lee SH, Hayes BJ, Goddard ME, Wray NR, Visscher PM. Simultaneous discovery, estimation and prediction analysis of complex traits using a Bayesian mixture model. PLoS Genet. (2015) 11:e1004969. doi: 10.1371/journal.pgen.1004969
27. VanRaden PM. Efficient methods to compute genomic predictions. J Dairy Sci. (2008) 91:4414–23. doi: 10.3168/jds.2007-0980
28. VanRaden PM, Van Tassell CP, Wiggans GR, Sonstegard TS, Schnabel RD, Taylor JF, et al. Invited review: reliability of genomic predictions for North American Holstein bulls. J Dairy Sci. (2009) 92:16–24. doi: 10.3168/jds.2008-1514
29. Weller JI, Ron M. Invited review: quantitative trait nucleotide determination in the era of genomic selection. J Dairy Sci. (2011) 94:1082–90. doi: 10.3168/jds.2010-3793
30. Boichard D, Chung H, Dassonneville R, David X, Eggen A, Fritz S, et al. Design of a bovine low-density SNP array optimized for imputation. PLoS ONE. (2012) 7:e34130. doi: 10.1371/journal.pone.0034130
31. Erbe M, Hayes BJ, Matukumalli LK, Goswami S, Bowman PJ, Reich CM, et al. Improving accuracy of genomic predictions within and between dairy cattle breeds with imputed high-density single nucleotide polymorphism panels. J Dairy Sci. (2012) 95:4114–29. doi: 10.3168/jds.2011-5019
32. Goddard ME, Kemper KE, MacLeod IM, Chamberlain AJ, Hayes BJ. Genetics of complex traits: prediction of phenotype, identification of causal polymorphisms and genetic architecture. Proc R Soc B. (2016) 283:20160569. doi: 10.1098/rspb.2016.0569
33. Rupp R, Mucha S, Larroque H, McEwan J, Conington J. Genomic application in sheep and goat breeding. Anim Front. (2016) 6:39–44. doi: 10.2527/af.2016-0006
34. Barrett BA, Faville MJ, Nichols SN, Simpson WR, Bryan GT, Conner AJ. Breaking through the feed barrier: options for improving forage genetics. Anim Prod Sci. (2015) 55:883–92. doi: 10.1071/AN14833
35. Calus MP, Bouwman AC, Schrooten C, Veerkamp RF. Efficient genomic prediction based on whole-genome sequence data using split-and-merge Bayesian variable selection. Genetics Select Evol. (2016) 48:49. doi: 10.1186/s12711-016-0225-x
36. Heidaritabar M, Calus MPL, Megens HJ, Vereijken A, Groenen MAM, Bastiaansen JWM. Accuracy of genomic prediction using imputed whole-genome sequence data in white layers. J Anim Breed Genetics. (2016) 133:167–79. doi: 10.1111/jbg.12199
37. MacLeod IM, Hayes BJ, Goddard ME. The effects of demography and long-term selection on the accuracy of genomic prediction with sequence data. Genetics. (2014) 198:1671–84. doi: 10.1534/genetics.114.168344
38. MacLeod IM, Bowman PJ, Vander Jagt CJ, Haile-Mariam M, Kemper KE, Chamberlain AJ, et al. Exploiting biological priors and sequence variants enhances QTL discovery and genomic prediction of complex traits. BMC Genomics. (2016) 17:144. doi: 10.1186/s12864-016-2443-6
39. Meuwissen T, Hayes B, Goddard M. Genomic selection: a paradigm shift in animal breeding. Anim Front. (2016) 6:6–14. doi: 10.2527/af.2016-0002
40. Barutcu AR, Fritz AJ, Zaidi SK, van Wijnen AJ, Lian JB, Stein JL, et al. C-ing the genome: a compendium of chromosome conformation capture methods to study higher-order chromatin organization. J Cell Physiol. (2016) 231:31–5. doi: 10.1002/jcp.25062
42. Lawrence M, Daujat S, Schneider R. Lateral thinking: how histone modifications regulate gene expression. Trends Genet. (2016) 32:42–56. doi: 10.1016/j.tig.2015.10.007
43. Murdoch BM, Murdoch GK, Greenwood S, McKay S. Nutritional influence on epigenetic marks and effect on livestock production. Front Genet. (2016) 7:182. doi: 10.3389/fgene.2016.00182
44. Sequeira-Mendes J, Gutierrez C. Genome architecture: from linear organisation of chromatin to the 3D assembly in the nucleus. Chromosoma. (2016) 125:455–69. doi: 10.1007/s00412-015-0538-5
45. Steele EJ. Commentary: past, present, and future of epigenetics applied to livestock breeding–Hard versus Soft Lamarckian inheritance mechanisms. Front Genet. (2016) 7:29. doi: 10.3389/fgene.2016.00029
46. ENCODE Project Consortium. The ENCODE (ENCyclopedia of DNA elements) project. Science. (2004) 306:636–40. doi: 10.1126/science.1105136
47. ENCODE Project Consortium. An integrated encyclopedia of DNA elements in the human genome. Nature. (2012) 489:57–74. doi: 10.1038/nature11247
48. Harrow J, Frankish A, Gonzalez JM, Tapanari E, Diekhans M, Kokocinski F, et al. GENCODE: the reference human genome annotation for The ENCODE Project. Genome Res. (2012) 22:1760–74. doi: 10.1101/gr.135350.111
49. Yue F, Cheng Y, Breschi A, Vierstra J, Wu W, Ryba T, et al. A comparative encyclopedia of DNA elements in the mouse genome. Nature. (2014) 515:355–64. doi: 10.1038/nature13992
50. Andersson L, Archibald AL, Bottema CD, Brauning R, Burgess SC, Burt DW, et al. Coordinated international action to accelerate genome-to-phenome with FAANG, the Functional Annotation of Animal Genomes project. Genome Biol. (2015) 16:57. doi: 10.1186/s13059-015-0622-4
51. Tuggle CK, Giuffra E, White SN, Clarke L, Zhou H, Ross PJ, et al. GO-FAANG meeting: a gathering on functional annotation of animal genomes. Anim Genet. (2016) 47:528–33. doi: 10.1111/age.12466
52. Faville MJ, Ganesh S, Cao MS, Jahufer MZZ, Bilton TP, Easton HS, et al. Predictive ability of genomic selection models in a multi-population perennial ryegrass training set using genotyping-by-sequencing. Theor Appl Genet. (2018) 131:703–20. doi: 10.1007/s00122-017-3030-1
53. Budden F, Gellatly SL, Wood DLA, Cooper MA, Morrison M, Hugenholtz P, et al. Emerging pathogenic links between microbiota and the gut–lung axis. Nat Rev Microbiol. (2016) 15:55–63. doi: 10.1038/nrmicro.2016.142
54. Clemente JC, Ursell LK, Parfrey LW, Knight R. The impact of the gut microbiota on human health: an integrative view. Cell. (2012) 148:1258–70. doi: 10.1016/j.cell.2012.01.035
55. Gao R, Gao Z, Huang L, Qin H. Gut microbiota and colorectal cancer. Eur J Clin Microbiol Infect Dis. (2017) 36:757–69. doi: 10.1007/s10096-016-2881-8
56. Hall AB, Tolonen AC, Xavier RJ. Human genetic variation and the gut microbiome in disease. Nat Rev Genet. (2017) 18:690–9. doi: 10.1038/nrg.2017.63
57. Hand W, Vujkovic-Cvijin I, Ridaura VK, Belkaid Y. Linking the microbiota, chronic disease, and the immune system. Trends Endocrinol Metab. (2016) 27:831–43. doi: 10.1016/j.tem.2016.08.003
58. Honda K, Littman DR. The microbiota in adaptive immune homeostasis and disease. Nature. (2016) 535:75–84. doi: 10.1038/nature18848
59. Ivanov I, Frutos Rde L, Manel N, Yoshinaga K, Rifkin DB, Sartor RB, et al. Specific microbiota direct the differentiation of IL-17-producing T-helper cells in the mucosa of the small intestine. Cell Host Microbe. (2008) 4:337–49. doi: 10.1016/j.chom.2008.09.009
60. Kau AL, Ahern PP, Griffin NW, Goodman AL, Gordon JI. Human nutrition, the gut microbiome and the immune system. Nature. (2011) 474:327–36. doi: 10.1038/nature10213
61. Koeth A, Wang Z, Levison BS, Buffa JA, Org E, Sheehy BT, et al. Intestinal microbiota metabolism of l-carnitine, a nutrient in red meat, promotes atherosclerosis. Nat Med. (2013) 19:576–85. doi: 10.1038/nm.3145
62. Le Chatelier E, Nielsen T, Qin J, Prifti E, Hildebrand F, Falony G, et al. Richness of human gut microbiome correlates with metabolic markers. Nature. (2013) 500:541–6. doi: 10.1038/nature12506
63. Qin J, Li Y, Cai Z, Li S, Zhu J, Zhang F, et al. A metagenome-wide association study of gut microbiota in type 2 diabetes. Nature. (2012) 490:55–60. doi: 10.1038/nature11450
64. Ridaura VK, Faith JJ, Rey FE, Cheng J, Duncan AE, Kau AL, et al. Gut microbiota from twins discordant for obesity modulate metabolism in mice. Science. (2013) 341:1241214. doi: 10.1126/science.1241214
65. Rothschild D, Weissbrod O, Barkan E, Kurilshikov A, Korem T, Zeevi D, et al. Environment dominates over host genetics in shaping human gut microbiota. Nature. (2018) 555:210–5. doi: 10.1038/nature25973
66. Turnbaugh PJ, Ley RE, Mahowald MA, Magrini V, Mardis ER, Gordon JI. An obesity-associated gut microbiome with increased capacity for energy harvest. Nature. (2006) 444:1027–131. doi: 10.1038/nature05414
67. Turnbaugh PJ, Hamady M, Yatsunenko T, Cantarel BL, Duncan A, Ley RE, et al. A core gut microbiome in obese and lean twins. Nature. (2009) 457:480–4. doi: 10.1038/nature07540
68. Zeevi D, Korem T, Zmora N, Israeli D, Rothschild D, Weinberger A, et al. Personalized nutrition by prediction of glycemic responses. Cell. (2015) 163:1079–94. doi: 10.1016/j.cell.2015.11.001
69. Zmora N, Zeevi D, Korem T, Segal E, Elinav E. Taking it personally: personalized utilization of the human microbiome in health and disease. Cell Host Microbe. (2016) 19:12–20. doi: 10.1016/j.chom.2015.12.016
70. Seshadri R, Leahy SC, Attwood GT, Teh KH, Lambie SC, Cookson AL, et al. Cultivation and sequencing of rumen microbiome members from the Hungate1000 collection. Nat Biotechnol. (2018) 36:359–67. doi: 10.1038/nbt.4110
71. Wakelin SA, Cave VM, Dignam BE, D'Ath C, Tourna M, Condron LM, et al. Analysis of soil eDNA functional genes: potential to increase profitability and sustainability of pastoral agriculture. N Z J Agric Res. (2016) 59:333–50. doi: 10.1080/00288233.2016.1209529
72. Coleman DC, Whitman WB. Linking species richness, biodiversity and ecosystem function in soil systems. Pedobiologia (Jena). (2005) 49:479–97. doi: 10.1016/j.pedobi.2005.05.006
74. Curtis TP, Sloan WT, Scannell JW. Estimating prokaryotic diversity and its limits. Proc Natl Acad Sci USA. (2002) 99:10494–9. doi: 10.1073/pnas.142680199
75. Schloss PD, Handelsman J. Towards a census of bacteria in soil. PLoS Comput Biol. (2006) 2:e92. doi: 10.1371/journal.pcbi.0020092
76. Torsvik V, Ovreas L, Thingstad TF. Prokaryotic diversity – magnitude, dynamics, and controlling factors. Science. (2002) 296:1064–6. doi: 10.1126/science.1071698
77. Roper MM, Gupta VVSR. Management practices and soil biota. Aust J Soil Res. (1995) 33:321–39. doi: 10.1071/SR9950321
78. Orwin KH, Stevenson BA, Smaill SJ, Kirschbaum MUF, Dickie IA, Clothier BE, et al. Effects of climate change on the delivery of soil-mediated ecosystem services within the primary sector in temperate ecosystems: a review and New Zealand case study. Glob Chang Biol. (2015) 21:2844–60. doi: 10.1111/gcb.12949
79. Bissett A, Fitzgerald A, Meintjes T, Mele PM, Reith F, Dennis PG, et al. Introducing BASE: the biomes of Australian soil environments soil microbial diversity database. Gigascience. (2016) 5:21. doi: 10.1186/s13742-016-0126-5
80. Vogel TM, Simonet P, Jansson JK, Hirsch PR, Tiedje JM, van Elsas JD, et al. TerraGenome: a consortium for the sequencing of a soil metagenome. Nat Rev Microbiol. (2009) 7:252. doi: 10.1038/nrmicro2119
81. Van Straalen NM, Roelofs D. An Introduction to Ecological Genomics. Oxford: Oxford University Press (2006).
82. Xu Z, Hansen MA, Hansen LH, Jacquoid S, Sørenson SJ. Bioinformatic approaches reveal metagenomic characterisation of soil microbial community. PLoS ONE. (2014) 9:e93445. doi: 10.1371/journal.pone.0093445
83. Deng Y, Jiang Y-H, Yang Y, He Z, Luo F, Zhou J. Molecular ecological network analyses. BMC Bioinformatics. (2012) 13:113. doi: 10.1186/1471-2105-13-113
84. Torsvik V, Daae FL, Sandaa R-A, Øveå L. Microbial diversity and function in soil: from genes to ecosystems. Curr Opin Microbiol. (2002) 5:240–5. doi: 10.1016/S1369-5274(02)00324-7
85. Myrold DD, Zeglin LH, Jansson JK. The potential of metagenomic approaches for understanding soil microbial processes. Soil Sci Soc Am J. (2013) 78:3–10. doi: 10.2136/sssaj2013.07.0287dgs
86. Dignam BEA, O'Callaghan M, Condron LM, Raaijmakers JM, Kowalchuk GA, Wakelin SA. Challenges and opportunities in harnessing soil disease suppressiveness for sustainable pasture production. Soil Biol Biochem. (2016) 95:100–11. doi: 10.1016/j.soilbio.2015.12.006
87. Konopka A. What is microbial community ecology? ISME J. (2009) 3:1223–30. doi: 10.1038/ismej.2009.88
88. Mendes R, Kruijt M, de Bruijn I, Dekkers E, van der Voort M, Schneiderraaij JHM, et al. Deciphering the rhizosphere microbiome for disease-suppressive bacteria. Science. (2011) 332:1097–100. doi: 10.1126/science.1203980
89. Frisli T, Haverkamp THA, Jakobsen KS, Stenseth NC, Rudi K. Estimation of the metagenomic size and structure in an experimental soil microbiota from low coverage next generation sequencing data. J Appl Microbiol. (2012) 114:141–51. doi: 10.1111/jam.12035
90. Fierer N, Leff JW, Adams BJ, Nielsen UN, Bates ST, Lauber CL, et al. Cross-biome metagenomic analyses of soil microbial communities and their functional attributes. Proc Natl Acad Sci USA. (2012) 109:21390–5. doi: 10.1073/pnas.1215210110
91. He Z, Gentry TJ, Schadt CW, Wu L, Liebich J, Chong SC, et al. GeoChip: a comprehensive microarray for investigating biogeochemical, ecological and environmental processes. ISME J. (2007) 1:67–77. doi: 10.1038/ismej.2007.2
92. Nelson MB, Martiny AC, Martiny JBH. Global biogeography of microbial nitrogen-cycling traits in soil. Proc Natl Acad Sci USA. (2016) 113:8033–40. doi: 10.1073/pnas.1601070113
93. Lee MH, Lee S-W. Bioprospecting potential of the soil metagenome: novel enzymes and bioactivities. Genomics Inform. (2013) 11:114–20. doi: 10.5808/GI.2013.11.3.114
94. Weller DM, Raaijmakers JM, McSpadden Gardener BB, Thomashow LS. Microbial populations responsible for specific soil suppressiveness to plant pathogens. Annu Rev Phytopathol. (2002) 40:309–48. doi: 10.1146/annurev.phyto.40.030402.110010
95. Schlatter D, Kinkel L, Thomashow L, Weller D, Paulitz T. Disease suppressive soils: new insights from the soil microbiome. Phytopathology. (2017) 107:1284–97. doi: 10.1094/PHYTO-03-17-0111-RVW
96. Skipp RA, Watson RN. Pot experiments with pasture soils to detect soilborne pathogens of white clover and Lucerne, and effects of field application with fungicides. N Z J Agric Res. (1987) 30:85–93. doi: 10.1080/00288233.1987.10430481
97. Wakelin SA, Eslami Y, Dake K, Dignam BEA, O'Callaghan M. Cost of root disease on white clover growth in New Zealand dairy pastures. Aust Plant Pathol. (2016) 45:289–96. doi: 10.1007/s13313-016-0411-x
98. Mazzola M. Mechanisms of natural soil suppressiveness to soilborne diseases. Antonie van Leeuwenhoek. (2002) 81:557–64. doi: 10.1023/A:1020557523557
99. Mazzola M. Assessment and management of soil microbial community structure for disease suppression. Annu Rev Phytopathol. (2004) 42:35–59. doi: 10.1146/annurev.phyto.42.040803.140408
100. Peters RD, Sturz AV, Carter MR, Sanderson JB. Developing disease-suppressive soils through crop rotation and tillage management practices. Soil Tillage Res. (2003) 72:181–92. doi: 10.1016/S0167-1987(03)00087-4
101. Raaijmakers JM, Paulitz TC, Steinberg C, Alabouvette C, Moënne-Loccoz Y. The rhizosphere: a playground and battlefield for soilborne pathogens and beneficial microorganisms. Plant Soil. (2009) 321:341–61. doi: 10.1007/s11104-008-9568-6
102. Hornby D. Suppressive soils. Annu Rev Phytopathol. (1983) 21:1–65. doi: 10.1146/annurev.py.21.090183.000433
103. Kyselková M, Kopecký J, Frapolli M, Défago G, Ságová-Marecková M, Grundmann GL, et al. Comparison of rhizobacterial community composition in soil suppressive or conducive to tobacco black root rot disease. ISME J. (2009) 3:1127–38. doi: 10.1038/ismej.2009.61
104. Chapelle E, Mendes R, Bakker PAHM, Raaijmakers JM. Fungal invasion of the rhizosphere. ISME J. (2016) 10:265–8. doi: 10.1038/ismej.2015.82
105. Penton CR, Gupta VVSR, Tiedje JM, Neate SM, Ophel-Keller K, Gillings M, et al. Fungal community structure in disease suppressive soils assessed by 28S LSU gene sequencing. PLoS ONE. (2014) 9:e93893. doi: 10.1371/journal.pone.0093893
106. Rankin DJ, Rocha EPC, Brown SP. What traits are carried on mobile genetic elements, and why? Heredity (Edinb). (2011) 106:1–10. doi: 10.1038/hdy.2010.24
107. Young JPW, Crossman LC, Johnston AW, Thomson NR, Ghazoui ZF, Hull KH, et al. The genome of Rhizobium leguminosarum has recognizable core and accessory components. Genome Biol. (2006) 7:R34. doi: 10.1186/gb-2006-7-4-r34
108. Raaijmakers JM, Mazzola M. Diversity and natural functions of antibiotics produced by beneficial and plant pathogenic bacteria. Annu Rev Phytopathol. (2012) 50:403–24. doi: 10.1146/annurev-phyto-081211-172908
109. Dignam BEA, O'Callaghan M, Condron LM, Kowalchuk GA, Van Nostrand JD, Zhou J, et al. Effect of land use and soil organic matter quality on the structure and function of microbial communities in pastoral soils: implications for disease suppression. PLoS ONE. (2018) 13:e0196581. doi: 10.1371/journal.pone.0196581
110. Langille MGI, Zaneveld J, Caporaso JG, McDonald D, Knights D, Reyes JA, et al. Predictive functional profiling of microbial communities using 16S rRNA marker gene sequences. Nat Biotechnol. (2013) 8:1–10. doi: 10.1038/nbt.2676
111. Nguyen NH, Song Z, Bates ST, Branco S, Tedersoo L, Menke J, et al. FUNGuild: an open annotation tool for parsing fungal community datasets by ecological guild. Fungal Ecol. (2015) 20:241–8. doi: 10.1016/j.funeco.2015.06.006
112. Lau JA, Suwa T. The changing nature of plant–microbe interactions during a biological invasion. Biol Invasions. (2016) 18:3527–34. doi: 10.1007/s10530-016-1245-8
113. Schnitzer SA, Klironomos JN, HilleRisLambers J, Kinkel LL, Reich PB, Xiao K, et al. Soil microbes drive the classic plant diversity–productivity pattern. Ecology. (2011) 92:296–303. doi: 10.1890/10-0773.1
114. Coleman DC, Crossley DA, Hendrix PF. Fundamentals of Soil Ecology. 2nd ed. Burlington, MA: Elsevier Academic Press (2004). 386p.
115. Hodgson J, Illius AW. The Ecology and Management of Grazing Systems. Wallingford: CAB International (1996).
116. Harrison MT, Cullen BR, Rawnsley RP. Modelling the sensitivity of agricultural systems to climate change and extreme climatic events. Agric Syst. (2016) 148:135–48. doi: 10.1016/j.agsy.2016.07.006
117. Sheath GW, Clark DA. Management of grazing systems: temperate pastures. In: Hodgson J, Illius AW, editors. The Ecology and Management of Grazing Systems. Wallingford: CAB International (1996). p. 301–24.
118. Casler MD, van Santen E. Breeding objectives in forages. In: Boller B, Posselt UK, Veronesi F, editors. Fodder Crops and Amenity Grasses. New York, NY: Springer (2010). p. 115–36.
119. Parsons AJ, Carrere P, Schwinning S. Dynamics of heterogeneity in a grazed sward. In: Lemaire G, Hodgson J, de Moraes A, de F Carvalho PC, Nabinger C, editors. Grassland Ecophysiology and Grazing Ecology. Wallingford: CAB International (2000). p. 289–316.
120. Bell NL, Townsend RJ, Popay AJ, Mercer CF, Jackson TA. Black beetle: lessons from the past and options for the future. In: Mercer CF, editor. Pasture Persistence Symposium, Grassland Research and Practice Series No. 15. Dunedin: New Zealand Grassland Association (2011). p. 119–24.
121. Glassey CB. Summer pasture yield variation in a central Waikato location from 1979 – 2010: implications for pasture persistence. In: Mercer CF, editor. Pasture Persistence Symposium. Grassland Research and Practice Series No. 15. Dunedin: New Zealand Grassland Association (2011). p. 15–20.
122. Leuchtmann A, Bacon CW, Schardl CL, White JF Jr, Tadych M. Nomenclatural realignment of Neotyphodium species with genus Epichloë. Mycologia. (2014) 106:202–15. doi: 10.3852/13-251
123. Popay AJ, Hume DE. Endophytes improve ryegrass persistence by controlling insects. In: Mercer CF, editor. Pasture Persistence Symposium. Grassland Research and Practice Series No. 15. Dunedin: New Zealand Grassland Association (2011). p. 149–56.
124. Thom ER, Popay AJ, Hume DE, Fletcher LR. Evaluating the performance of endophytes in farm systems to improve farm outcomes – a review. Crop Pasture Sci. (2012) 63:927–43. doi: 10.1071/CP12152
125. Thom ER, Waugh CD, Minneé EMK, Waghorn GC. Effects of novel and wild-type endophytes in perennial ryegrass on cow health and production. N Z Vet J. (2013) 61:87–97. doi: 10.1080/00480169.2012.715379
126. Popay AJ, Hume DE, Davis KL, Tapper BA. Interactions between endophyte (Neophytodium spp.) and ploidy in hybrid and perennial ryegrass cultivars and their effects on Argentine stem weevil (Listronotus bonariensis). N Z J Agric Res. (2003) 46:311–9.
127. Schwinning S, Parsons AJ. Analysis of the coexistence mechanisms for grasses and legumes in grazing systems. J Ecol. (1996) 84:799–814. doi: 10.2307/2960553
128. Nicol AM, Edwards GR. Why is clover better than ryegrass? Proc N Z Soc Anim Prod. (2011) 71:71–8.
129. Moorby JM. Relationship between the composition of fresh grass-based diets and the excretion of dietary nitrogen from dairy cows. In: Hopkins A, Collins RP, Fraser MD, King VR, Lloyd DC, Moorby JM, Robson PRH, editors. EGF at 50: The Future of European Grasslands, Vol. 19, Grassland Science in Europe. Aberystwyth: European Grassland Federation; Aberystwyth University (2014). p. 686–9.
130. Kebreab E, France J, Beever D, Castillo A. Nitrogen pollution by dairy cows and its mitigation by dietary manipulation. Nutr Cycling Agroecosyst. (2001) 60:275–85. doi: 10.1023/A:1012668109662
131. Chapman DF, Lee JM, Rossi L, Edwards GR, Pinxterhuis JB, Minnee EKM. White clover: the forgotten component of high producing pastures? Anim Prod Sci. (2017) 57:1269–76. doi: 10.1071/AN16453
132. Chapman DF, Parsons AJ, Cosgrove GP, Barker DJ, Marotti DM, Venning KJ, et al. Impacts of spatial patterns in pasture on animal grazing behviour, intake and performance. Crop Sci. (2007) 47:399–415. doi: 10.2135/cropsci2006.01.0036
133. Rutter SM. Diet preference for grass and legumes in free-ranging domestic sheep and cattle: current theory and future application. Appl Anim Behav Sci. (2006) 97:17–35. doi: 10.1016/j.applanim.2005.11.016
134. Gibb MJ, Huckle CA, Nuthall R, Rook AJ. Effect of sward surface height on intake and grazing behaviour by lactating Holstein Friesian cows. Grass Forage Sci. (1997) 52:309–21. doi: 10.1111/j.1365-2494.1997.tb02361.x
135. Schwinning S, Parsons AJ. The stability of grazing systems revisited: spatial models and the role of heterogeneity. Funct Ecol. (1999) 13:737–47. doi: 10.1046/j.1365-2435.1999.00382.x
136. Russell JB, Strobel HJ. Microbial energetics. In: Dijkstra J, Forbes JM, France J, editors. Quantitative Aspects of Ruminant Digestion and Metabolism, 2nd ed. Wallingford: CAB International (1993). p. 229–61.
137. Rumsey TS. Monensin in cattle: introduction. J Anim Sci. (1984) 58:1461–4. doi: 10.2527/jas1984.5861461x
138. Schelling GT. Monensin mode of action in the rumen. J Anim Sci. (1984) 58:1518–27. doi: 10.2527/jas1984.5861518x
139. Russell JB, Houlihan AJ. Ionophore resistance of ruminal bacteria and its potential impact on human health. FEMS Microbiol Rev. (2003) 27:65–74. doi: 10.1016/S0168-6445(03)00019-6
140. Knight T, Ronimus RS, Dey D, Tootill C, Naylor G, Evans P, et al. Chloroform decreases rumen methanogenesis and methanogen populations without altering rumen function in cattle. Anim Feed Sci Technol. (2011) 166–7:101–12. doi: 10.1016/j.anifeedsci.2011.04.059
141. Smith M. Reversal of 2-bromoethanesulfonate inhibition of methanogenesis in Methanosarcina sp. J Bacteriol. (1983) 156:516–23.
142. Balch WE, Wolfe RS. Transport of coenzyme M (2-mercaptoethanesulfonic acid) in Methanobacterium ruminantium. J Bacteriol. (1979) 137:264–73.
143. Ungerfeld E, Rust S, Boone D, Liu Y. Effects of several inhibitors on pure cultures of ruminal methanogens. J Appl Microbiol. (2004) 97:520–6. doi: 10.1111/j.1365-2672.2004.02330.x
144. Karnati SK, Sylvester JT, Ribeiro CV, Gilligan LE, Firkins JL. Investigating unsaturated fat, monensin, or bromoethanesulfonate in continuous cultures retaining ruminal protozoa. I Fermentation, biohydrogenation, and microbial protein synthesis. J Dairy Sci. (2009) 92:3849–60. doi: 10.3168/jds.2008-1436
145. Haisan J, Sun Y, Guan L, Beauchemin K, Iwaasa A, Duval S, et al. The effects of feeding 3-nitrooxypropanol on methane emissions and productivity of Holstein cows in mid lactation. J Dairy Sci. (2014) 97:3110–9. doi: 10.3168/jds.2013-7834
146. Romero-Perez A, Okine E, McGinn S, Guan L, Oba M, Duval S, et al. Sustained reduction in methane production from long-term addition of 3-nitrooxypropanol to a beef cattle diet. J Anim Sci. (2015) 93:1780–91. doi: 10.2527/jas.2014-8726
147. Haisan J, Sun Y, Guan L, Beauchemin KA, Iwaasa A, Duval S, et al. The effects of feeding 3-nitrooxypropanol at two doses on milk production, rumen fermentation, plasma metabolites, nutrient digestibility, and methane emissions in lactating Holstein cows. Anim Prod Sci. (2017) 57:282–9. doi: 10.1071/AN15219
148. Martinez-Fernandez G, Abecia L, Arco A, Cantalapiedra-Hijar G, Martín-García AI, Molina-Alcaide E, et al. Effects of ethyl-3-nitrooxy propionate and 3-nitrooxypropanol on ruminal fermentation, microbial abundance, and methane emissions in sheep. J Dairy Sci. (2014) 97:3790–9. doi: 10.3168/jds.2013-7398
149. Chaucheyras-Durand F, Walker ND, Bach A. Effects of active dry yeasts on the rumen microbial ecosystem: past, present and future. Anim Feed Sci Technol. (2008) 145:5–26. doi: 10.1016/j.anifeedsci.2007.04.019
150. Hess MK, Rowe SJ, Van Stijn TC, Brauning R, Hess AS, Kirk MR, et al. High-throughput rumen microbial profiling using genotyping-by-sequencing. In: Proceedings of the 11th World Congress on Genetics Applied to Livestock Production. Auckland (2018). 331 p.
151. Henderson G, Cox F, Ganesh S, Jonker A, Young W, Global Rumen Census Collaborators, et al. Rumen microbial community composition varies with diet and host, but a core microbiome is found across a wide geographical range. Sci Rep. (2015) 5:14567. doi: 10.1038/srep14567
152. Stewart RD, Auffret MD, Warr A, Wiser AH, Press MO, Langford KW, et al. Assembly of 913 microbial genomes from metagenomic sequencing of the cow rumen. Nat Commun. (2018) 9:870. doi: 10.1038/s41467-018-03317-6
153. Solden LM, Hoyt DW, Collins WB, Plank JE, Daly RA, Hildebrand E, et al. New roles in hemicellulosic sugar fermentation for the uncultivated Bacteroidetes family BS11. ISME J. (2017) 11:691–703. doi: 10.1038/ismej.2016.150
154. Ormerod KL, Wood DLA, Lachner NL, Gellatly SL, Daly JN, Parsons JD, et al. Genomic characterization of the uncultured Bacteroidales family S24-7 inhabiting the guts of homeothermic animals. Microbiome. (2016) 4:36. doi: 10.1186/s40168-016-0181-2
155. Egert M, de Graaff AA, Smidt H, de Vos WM, Venema K. Beyond diversity: functional microbiomes of the human colon. Trends Microbiol. (2006) 14:86–91. doi: 10.1016/j.tim.2005.12.007
156. Hooper LV. Bacterial contributions to mammalian gut development. Trends Microbiol. (2004) 12:129–34. doi: 10.1016/j.tim.2004.01.001
157. Xu J, Gordon JI. Honor thy symbionts. Proc Natl Acad Sci USA. (2003) 100:10452–9. doi: 10.1073/pnas.1734063100
158. Shi W, Moon CD, Leahy SC, Kang D, Froula J, Kittelmann S, et al. Methane yield phenotypes linked to differential gene expression in the sheep rumen microbiome. Genome Res. (2014) 24:1517–25. doi: 10.1101/gr.168245.113
159. Patra AK. Recent advances in measurement and dietary mitigation of enteric methane emissions in ruminants. Front Vet Sci. (2016) 3:39. doi: 10.3389/fvets.2016.00039
160. Mosoni P, Martin C, Forano E, Morgavi DP. Long-term defaunation increases the abundance of cellulolytic ruminococci and methanogens but does not affect the bacterial and methanogen diversity in the rumen of sheep. J Anim Sci. (2011) 89:783–91. doi: 10.2527/jas.2010-2947
161. Popova M, Martin C, Eugène M, Mialon MM, Doreau M, Morgavi DP. Effect of fibre- and starch-rich finishing diets on methanogenic Archaea diversity and activity in the rumen of feedlot bulls. Anim Feed Sci Technol. (2011) 166–7:113–21. doi: 10.1016/j.anifeedsci.2011.04.060
162. Yanez-Ruiz DR, Hart KJ, Martin-Garcia AI, Ramos S, Newbold CJ. Diet composition at weaning affects the rumen microbial population and methane emissions by lambs. Aust J Exp Agric. (2008) 48:186–8. doi: 10.1071/EA07237
163. Morgavi DP, Martin C, Jouany J-P, Ranilla MJ. Rumen protozoa and methanogenesis: not a simple cause–effect relationship. Br J Nutr. (2012) 107:388–97. doi: 10.1017/S0007114511002935
164. Zhou M, Hernandez-Sanabria E, Guan LL. Characterization of variation in rumen methanogenic communities under different dietary and host feed efficiency conditions, as determined by PCR-denaturing gradient gel electrophoresis analysis. Appl Environ Microbiol. (2010) 76:3776–86. doi: 10.1128/AEM.00010-10
165. Janssen PH. Influence of hydrogen on rumen methane formation and fermentation balances through microbial growth kinetics and fermentation thermodynamics. Anim Feed Sci Technol. (2010) 160:1–22. doi: 10.1016/j.anifeedsci.2010.07.002
166. Morgavi DP, Forano E, Martin C, Newbold CJ. Microbial ecosystem and methanogenesis in ruminants. Animal. (2010) 4:1024–36. doi: 10.1017/S1751731110000546
167. Pinares-Patiño CS, Ebrahimi SH, McEwan JC, Clark H, Luo D. Is rumen retention time implicated in sheep differences in methane emission? Proc N Z Soc Anim Prod. (2011) 71:219–22.
168. Pinares-Patiño CS, McEwan JC, Dodds KG, Cárdenas EA, Hegarty RS, Koolaard JP, et al. Repeatability of methane emissions from sheep. Anim Feed Sci Technol. (2011) 166:210–8. doi: 10.1016/j.anifeedsci.2011.04.068
169. Pinares-Patiño CS, Hickey SM, Young EA, Dodds KG, MacLean S, Molano G, et al. Heritability estimates of methane emissions from sheep. Animal. (2013) 7:316–21. doi: 10.1017/S1751731113000864
170. Benchaar C, Pomar C, Chiquette J. Evaluation of dietary strategies to reduce methane production in ruminants: a modelling approach. Can J Anim Sci. (2001) 81:478-563-74. doi: 10.4141/A00-119
171. Smuts M, Meissner HH, Cronjé PB. Retention time of digesta in the rumen: its repeatability and relationship with wool production of Merino rams. J Anim Sci. (1994) 73:206–10. doi: 10.2527/1995.731206x
172. Goopy JP, Donaldson A, Hegarty R, Vercoe PE, Haynes F, Barnett M, et al. Low-methane yield sheep have smaller rumens and shorter rumen retention time. Br J Nutr. (2013) 111:578–85. doi: 10.1017/S0007114513002936
173. Wang M, Sun XZ, Janssen PH, Tang SX, Tan ZL. Responses of methane production and fermentation pathways to the increased dissolved hydrogen concentration generated by eight substrates in in vitro ruminal cultures. Anim Feed Sci Technol. (2014) 194:1–11. doi: 10.1016/j.anifeedsci.2014.04.012
174. Wang M, Ungerfeld EM, Wang R, Zhou CS, Basang ZZ, Ao SM, et al. Supersaturation of dissolved hydrogen and methane in rumen of Tibetan sheep. Front Microbiol. (2016) 7:850. doi: 10.3389/fmicb.2016.00850
175. Gaiero JR, McCall CA, Thompson KA, Day NY, Best AS, Dunfield KE. Inside the root microbiome: bacterial root endophytes and plant growth promotion. Am J Bot. (2013) 100:1738–50. doi: 10.3732/ajb.1200572
176. Hacquard S, Garrido-Oter R, Gonzalez A, Spaepen S, Ackermann G, Lebeis S, et al. Microbiota and host nutrition across plant and animal kingdoms. Cell Host Microbe. (2015) 17:603–16. doi: 10.1016/j.chom.2015.04.009
177. Nallanchakravarthula S, Mahmood S, Alström S, Findlay RD. Influence of soil type, cultivar and Verticillium dahliae on the structure of the root and rhizosphere soil fungal microbiome of strawberry. PLoS ONE. (2014) 9:e111455. doi: 10.1371/journal.pone.0111455
178. Rascovan N, Carbonetto B, Perrig D, Diaz M, Canciani W, Abalo M, et al. Integrated analysis of root microbiomes of soybean and wheat from agricultural fields. Sci Rep. (2016) 6:28084. doi: 10.1038/srep28084
179. Schreiter S, Ding G-C, Heuer H, Neumann G, Sandmann M, Grosch R, et al. Effect of the soil type on the microbiome in the rhizosphere of field-grown lettuce. Front Microbiol. (2014) 5:144. doi: 10.3389/fmicb.2014.00144
180. Ganley RJ, Brunsfield SJ, Newcombe G. A community of unknown, endophytic fungi in western white pine. Proc Natl Acad Sci USA. (2004) 101:10107–12. doi: 10.1073/pnas.0401513101
181. Zolla G, Badri DV, Bakker MG, Manter DK, Vivanco JM. Soil microbiomes vary in their ability to confer drought tolerance to Arabidopsis. Appl Soil Ecol. (2013) 68:1–9. doi: 10.1016/j.apsoil.2013.03.007
182. Panke-Buisse K, Poole AC, Goodrich JK, Ley RE, Kao-Kniffin J. Selection on soil microbiomes reveals reproducible impacts on plant function. ISME J. (2014) 9:980–9. doi: 10.1038/ismej.2014.196
183. Wagner MR, Lundberg DS, Coleman-Derr D, Tringe SG, Dangl JL, Mitchell-Olds T. Natural soil microbes alter flowering phenology and the intensity on flowering time in a wild Arabidopsis relative. Ecol Lett. (2014) 17:717–26. doi: 10.1111/ele.12276
184. Babu S, Bidyarani N, Chopra P, Monga D, Kumar R, Prasanna R, et al. Evaluating microbe-plant interactions and varietal differences for enhancing biocontrol efficacy in root rot disease challenged cotton crop. Eur J Plant Pathol. (2015) 142:345–62. doi: 10.1007/s10658-015-0619-6
185. Brader G, Compant S, Mitter B, Trognitz F, Sessitsch A. Metabolic potential of endophytic bacteria. Curr Opin Biotechnol. (2014) 27:30–7. doi: 10.1016/j.copbio.2013.09.012
186. O'Callaghan M, Gerard EM, Bell NL, Waipara N, Aalders LT, Baird DB, et al. Microbial and nematode communities associated with potatoes genetically modified to express the antimicrobial peptide magainin and unmodified potato cultivars. Soil Biol Biochem. (2008) 40:1446–59. doi: 10.1016/j.soilbio.2007.12.028
187. Veringer M, Siegmund B, Cardinale M, Müller H, Choi Y, Migeuz CB, et al. Monitoring the plant epiphyte Methylobacterium extorquens DSM 21961 by real-time PCR and its influence on strawberry flavour. FEMS Microbiol Ecol. (2010) 74:136–45. doi: 10.1111/j.1574-6941.2010.00942.x
188. Zabetakis I. Enhancement of flavour biosynthesis from strawberry (Fragaria x ananassa) callus cultures by Methylobacterium species. Plant Cell Tissue Organ Cult. (1997) 50:179–218. doi: 10.1023/A:1005968913237
189. Zarraonaindia I, Owens SM, Weisenhorn P, West K, Hampton-Marcell J, Lax S, et al. The soil microbiome influences grapevine-associated microbiota. MBio. (2015) 6:e02527–e02514. doi: 10.1128/mBio.02527-14
190. Berendsen RL, Pieterse CMJ, Bakker PAHM. The rhizosphere microbiome and plant health. Trends Plant Sci. (2012) 17:478–86. doi: 10.1016/j.tplants.2012.04.001
191. Hooper DU, Bignell DE, Brown VK, Brussard L, Dangerfield MJ, Wall DH, et al. Interactions between aboveground and belowground biodiversity in terrestrial ecosystems: patterns, mechanisms, and feedbacks. Bioscience. (2000) 50:1049–61. doi: 10.1641/0006-3568(2000)050[1049:IBAABB]2.0.CO;2
192. Wardle DA, Bardgett RD, Klironomos JN, Setää H, van der Putten WH, Wall DH. Ecological linkages between aboveground and belowground biota. Science. (2004) 304:1629–33. doi: 10.1126/science.1094875
193. Belanche A, Newbold CJ, Lin W, Rees Stevewns P, Kingston-Smith A. A systems biology approach reveals differences in the dynamics of colonization and degradation of grass vs. hay by rumen microbes with minor effects of vitamin E supplementation. Front Microbiol. (2017) 8:1456. doi: 10.3389/fmicb.2017.01456
194. Huws SA, Edwards JE, Creevey CJ, Rees Stevens P, Lin W, Girdwood SE, et al. Temporal dynamics of the metabolically active rumen bacteria colonizing fresh perennial ryegrass. FEMS Microbiol Ecol. (2016) 92:fiv137. doi: 10.1093/femsec/fiv137
195. Mayorga OL, Kingston-Smith AH, Kim EJ, Allison GG, Wilkinson TJ, Hegarty MJ, et al. Temporal metagenomic and metabolomic characterization of fresh perennial ryegrass degradation by rumen bacteria. Front Microbiol. (2016) 7:1854. doi: 10.3389/fmicb.2016.01854
196. Kingston-Smith AH, Davies TE, Edwards JE, Theodorou MK. From plants to animals; the role of plant cell death in ruminant herbivores. J Exp Bot. (2008) 59:521–32. doi: 10.1093/jxb/erm326
197. Fitzgerald JM, Collin RG, Towers NR. Biological control of sporidesmin-producing strains of Pithomyces chartarum by biocompetitive exclusion. Lett Appl Microbiol. (1998) 26:17–21. doi: 10.1046/j.1472-765X.1998.00260.x
199. Healy WB. Ingestion of soil by dairy cows. N Z J Agric Res. (1968) 11:487–99. doi: 10.1080/00288233.1968.10431444
200. Flavin M, Ochoa S. Metabolism of propionic acid in animal tissues. I Enzymatic conversion of propionate to succinate. J Biol Chem. (1957) 229:965–79.
201. Le Grusse J, Watier B. Les Vitamines: Données Biochimiques, Nutritionnelles et Cliniques. Neuilly-sur-Seine: Centre d'Etude et d'Information sur les Vitamines (1993).
202. Callaway TR, Dowd SE, Edrington TS, Anderson RC, Krueger N, Bauer N, et al. Evaluation of bacterial diversity in the rumen and feces of cattle fed different levels of dried distillers grains plus solubles using bacterial tag-encoded FLX amplicon pyrosequencing. J Anim Sci. (2010) 88:3977–83. doi: 10.2527/jas.2010-2900
203. Liu JH, Zhang M-L, Zhang R-Y, Zhu W-Y, Mao S-Y. Comparative studies of the composition of bacterial microbiota associated with the ruminal content, ruminal epithelium and in the faeces of lactating dairy cows. Microb Biotechnol. (2016) 9:257–68. doi: 10.1111/1751-7915.12345
204. Shanks OC, Kelty CA, Archibeque S, Jenkins M, Newton RJ, McLellan SL, et al. Community structures of fecal bacteria in cattle from different animal feeding operations. Appl Environ Microbiol. (2011) 77:2992–3001. doi: 10.1128/AEM.02988-10
205. Bardgett RD, Keiller S, Cook R, Gilburn AS. Interactions between soil animals and microorganisms in upland grassland soils amended with sheep dung: a microcosm experiment. Soil Biol Biochem. (1998) 30:531–9. doi: 10.1016/S0038-0717(97)00146-6
206. Bardgett RD, Wardle DA, Yeates GW. Linking above-ground and below-ground interactions: how plant responses to foliar herbivory influence soil organisms. Soil Biol Biochem. (1998) 30:1867–78. doi: 10.1016/S0038-0717(98)00069-8
207. Ruess RW, Seagle SW. Landscape patterns in soil microbial processes in the Serengeti National park, Tanzania. Ecology. (1994) 75:892–904. doi: 10.2307/1939414
208. Callaway TR, Elder RO, Keen JE, Anderson RC, Nisbet DJ. Forage feeding to reduce preharvest Escherichia coli populations in cattle, a review. J Dairy Sci. (2003) 86:852–60. doi: 10.3168/jds.S0022-0302(03)73668-6
209. Rapp D, Ross C, Cave V, Muirhead RW. Prevalence, concentration and genotypes of Campylobacter jejuni in feces from dairy herds managed in farm systems with or without housing. J Appl Microbiol. (2014) 116:1035–43. doi: 10.1111/jam.12425
210. Vanselow BA, Krause DO, McSweeney CS. The Shiga toxin-producting Escherichia coli, their ruminant hoses, and potential on-farm interventions: a review. Aust J Agric Res. (2005) 56:219–44. doi: 10.1071/AR04129
211. Klous G, Huss A, Heederik DJJ, Coutinho RA. Human-livestock contacts and their relationship to transmission of zoonotic pathogens, a systematic review of literature. One Health. (2016) 2:65–76. doi: 10.1016/j.onehlt.2016.03.001
212. Hussein HS, Sakuma T. Shiga toxin-producing Escherichia coli: pre- and postharvest control measures to ensure safety of dairy cattle products. J Food Prot. (2005) 68:199–207. doi: 10.4315/0362-028X-68.1.199
213. Strachan NJC, Dunn GD, Locking ME, Reid TMS, Ogden ID. Escherichia coli O157: burger bug or environmental pathogen? Int J Food Microbiol. (2006) 112:129–37. doi: 10.1016/j.ijfoodmicro.2006.06.021
214. Dufour A, Bartram J, Bos R, Gannon V. Animal Waste, Water Quality and Human Health. London: IWA Publishing.
215. Hurdey SE, Payment P, Huck PM, Gillham RW, Hurdey EJ. A fatal waterborne disease epidemic in Walkerton, Ontario: comparison with other waterborne outbreaks in the developed world. Water Sci Technol. (2003) 47:7–14. doi: 10.2166/wst.2003.0146
216. Soller JA, Schoen ME, Bartrand T, Ravenscroft JE, Ashbolt NJ. Estimated human health risks from exposure to recreational waters impacted by human and non-human sources of faecal contamination. Water Res. (2010) 44:4674–91. doi: 10.1016/j.watres.2010.06.049
217. Pachepsky Y, Shelton DR, McLain JET, Patel J, Mandrell RE. Irrigation waters as a source of pathogenic microoganisms in produce. A Rev Adv Agronomy. (2011) 113:73–138. doi: 10.1016/B978-0-12-386473-4.00007-5
218. Bennett R, Christiansen K, Clifton-Hadley R. Preliminary estimates of the direct costs associated with endemic diseases of livestock in Great Britain. Prev Vet Med. (1999) 39:155–71. doi: 10.1016/S0167-5877(99)00003-3
219. Christou L. The global burden of bacterial and viral zoonotic infections. Clin Microbiol Infect. (2011) 17:326–30. doi: 10.1111/j.1469-0691.2010.03441.x
220. Torgerson PR, Macpherson CNL. The socioeconomic burden of parasitic zoonoses: global trends. Vet Parasitol. (2011) 182:79–95. doi: 10.1016/j.vetpar.2011.07.017
221. Christen R. Global sequencing: a review of current molecular data and new methods available to assess microbial diversity. Microbes Environ. (2008) 23:253–68. doi: 10.1264/jsme2.ME08525
222. Cornelius AJ, Vandenberg O, Robson B, Gilpin BJ, Brante SM, Scholes P, et al. Same-day subtyping of Campylobacter jejuni and C. coli isolates by use of multiplex ligation-dependent probe amplification-binary typing. J Clin Microbiol. (2014) 52:3345–50. doi: 10.1128/JCM.00815-14
223. Staley C, Sadowsky MJ. Application of metagenomics to assess microbial communities in water and other environmental matrices. J Marina Biol Assoc UK. (2016) 96:121–9. doi: 10.1017/S0025315415001496
224. Caporaso JG, Lauber CL, Walters WA, Berg-Lyons D, Lozupone CA, Turnbaugh PT, et al. Global patterns of 16S rRNA diversity at a depth of millions of sequences per sample. Proc Natl Acad Sci USA. (2011) 108:4516–22. doi: 10.1073/pnas.1000080107
225. Huse S, Welch D, Morrison H, Sogin M. Ironing out the wrinkles in the rare biosphere through improved OTU clustering. Environ Microbiol. (2010) 12:1889–98. doi: 10.1111/j.1462-2920.2010.02193.x
226. Rinke C, Schwientek P, Sczyrba A, Ivanova NN, Anderson IJ, Cheng J-F, et al. Insights into the phylogeny and coding potential of microbial dark matter. Nature. (2013) 499:431–7. doi: 10.1038/nature12352
227. Stewart EJ. Growing unculturable bacteria. J Bacteriol. (2012) 194:4151–60. doi: 10.1128/JB.00345-12
228. Lynch MDJ, Neufeld JD. Ecology and exploration of the rare biosphere. Nat Rev Microbiol. (2015) 13:217–29. doi: 10.1038/nrmicro3400
229. Mertens J, Broos K, Wakelin SA, Kowalchuk GA, Springael D, Smolders E. Bacteria, not archaea, restore nitrification in a zinc-contaminated soil. ISME J. (2009) 3:916–23. doi: 10.1038/ismej.2009.39
230. Alivisatos AP, Blaser MJ, Brodie EL, Chun M, Dangl JL, Donohue TJ, et al. A unified initiative to harness Earth's microbiomes. Transition from description to causality and engineering. Science. (2015) 350:507–8. doi: 10.1126/science.aac8480
231. Prosser JI. Dispersing misconceptions and identifying opportunities for the use of ‘omics' in soil microbial ecology. Nat Rev Microb. (2015) 13:439–46. doi: 10.1038/nrmicro3468
Keywords: genomics, metagenomics, pasture, ecosystems, food, soil
Citation: Attwood GT, Wakelin SA, Leahy SC, Rowe S, Clarke S, Chapman DF, Muirhead R and Jacobs JME (2019) Applications of the Soil, Plant and Rumen Microbiomes in Pastoral Agriculture. Front. Nutr. 6:107. doi: 10.3389/fnut.2019.00107
Received: 01 February 2019; Accepted: 27 June 2019;
Published: 16 July 2019.
Edited by:
Rossana Coda, University of Helsinki, FinlandReviewed by:
Jeffrey Firkins, The Ohio State University, United StatesCopyright © 2019 Attwood, Wakelin, Leahy, Rowe, Clarke, Chapman, Muirhead and Jacobs. This is an open-access article distributed under the terms of the Creative Commons Attribution License (CC BY). The use, distribution or reproduction in other forums is permitted, provided the original author(s) and the copyright owner(s) are credited and that the original publication in this journal is cited, in accordance with accepted academic practice. No use, distribution or reproduction is permitted which does not comply with these terms.
*Correspondence: Jeanne M. E. Jacobs, amVhbm5lLmphY29ic0BhZ3Jlc2VhcmNoLmNvLm56
†Present Address: Steve A. Wakelin, Scion Research, Christchurch, New Zealand
Disclaimer: All claims expressed in this article are solely those of the authors and do not necessarily represent those of their affiliated organizations, or those of the publisher, the editors and the reviewers. Any product that may be evaluated in this article or claim that may be made by its manufacturer is not guaranteed or endorsed by the publisher.
Research integrity at Frontiers
Learn more about the work of our research integrity team to safeguard the quality of each article we publish.