- 1Department of Forest and Rangeland Stewardship, Colorado State University, Fort Collins, CO, United States
- 2School of Natural Resources and the Environment, University of Arizona, Tucson, AZ, United States
- 3Department of Biological Sciences, Merriam-Powell Center for Environmental Research, Northern Arizona University, Flagstaff, AZ, United States
- 4Department of Ecology and Evolutionary Biology, University of Arizona, Tucson, AZ, United States
- 5Department of Earth & Environment, Boston University, Boston, MA, United States
Ecologists are improving predictive capability using near-term ecological forecasts, in which predictions are made iteratively and publically to increase transparency, rate of learning, and maximize utility. Ongoing ecological forecasting efforts focus mostly on long-term datasets of continuous variables, such as CO2 fluxes, or more abrupt variables, such as phenological events or algal blooms. Generally lacking from these forecasting efforts is the integration of short-term, opportunistic data concurrent with developing climate extremes such as drought. We posit that incorporating targeted experiments and regional surveys, implemented rapidly during developing extreme events, into current forecasting efforts will ultimately enhance our ability to forecast ecological responses to climate extremes, which are projected to increase in both frequency and intensity. We highlight a project, “chasing tree die-off,” in which we coupled an experiment with regional-scale observational field surveys during a developing severe drought to test and improve forecasts of tree die-off. General insights to consider in incorporating this approach include: (1) tracking developing climate extremes in near-real time to efficiently ramp up measurements rapidly and, if feasible, initiate an experiment quickly—including funding and site selection challenges; (2) accepting uncertainty in projected extreme climatic events and adjusting sampling design over-time as needed, especially given the spatially heterogeneous nature of many ecological disturbances; and (3) producing timely and iterative output. In summary, targeted experiments and regional surveys implemented rapidly during developing extreme climatic events offer promise to efficiently (both financially and logistically) improve our ability to forecast ecological responses to climate extremes.
Introduction
The frequency and severity of climate extremes such as drought, floods, and heat waves are projected to increase with global climate change (de Coninck et al., 2018; Hayhoe et al., 2018). These climate extremes can trigger rapid ecosystem responses (i.e., extreme climatic events; Smith, 2011), including widespread tree die-off (Allen et al., 2010, 2015), algal blooms (Havens et al., 2016), wildfires (Moritz et al., 2010), plant invasions (Sheppard et al., 2012), and extensive soil erosion (Coppus and Imeson, 2002). This has created a need for rapid anticipatory science and management to increase ecosystem resistance to and/or recovery following extreme climate events (Ummenhofer and Meehl, 2017; Bradford et al., 2018). Yet forecasting not only the climate extremes, but also the ecological responses (Smith, 2011), is a key prerequisite toward conducting anticipatory science and management in the face of climate change (Dietze et al., 2018). Near-term ecological forecasting has been developed to make iterative predictions of ecological responses to inform management action and has the potential to transform our ability to rapidly manage natural resources during and following extreme climatic events (Clark et al., 2001; Dietze et al., 2018). In this Perspective, we propose that near-term ecological forecasting can be enhanced by targeted experiments and regional surveys implemented rapidly during developing extreme climatic events to improve our ability to forecast, and ultimately manage, ecological responses to climate extremes.
Near-term ecological forecasting has already been used to iteratively forecast wildfires (Chen et al., 2011), influenza outbreaks (Shaman and Karspeck, 2012), algal blooms (Stumpf et al., 2009), and more (see also Dietze, 2017; Dietze et al., 2018) and can be coupled with adaptive management to further improve predictions and guide anticipatory management (Bradford et al., 2018). Successful near-term ecological forecasting can be achieved by continually making predictions, taking measurements, observing results of those predictions, and integrating data with models (Kalnay, 2002; Dietze, 2017)—in essence “learning by doing” (Shuman, 1989). These steps can be further refined using the scientific method to propose alternative models that test appropriate hypotheses by comparing observations to specific, quantitative predictions rather than the conventional null hypothesis. These improved models are then used to forecast, observe, analyze, and refine hypotheses, and the iterative forecast cycle continues (Dietze et al., 2018). Coupled synchronously with this ongoing iterative forecast cycle is the adaptive management cycle, wherein monitoring data can be used not only to assess previous management decisions but can also be assimilated into forecasts that explore alternative management scenarios moving forward (Gregory et al., 2012; Ketz et al., 2016). Performed in tandem, these two cycles will accelerate our capacity to predict and respond to extreme climatic events.
We see an opportunity to complement the near-term ecological forecasting framework to more effectively address rapidly developing, ecologically significant extreme climatic events that are projected to increase with global climate change. At present, most examples of near-term ecological forecasting have generally relied on long-term data from a single site (e.g., Hobbs et al., 2015), a network of sites (e.g., Kuikka et al., 2014; Thomas et al., 2017), or near-real time data through satellites (e.g., Stumpf et al., 2009). Yet speedily implemented natural and manipulative experiments provide a way to target transient and progressive spatially-heterogeneous extreme climatic events (e.g., algal blooms, exotic species invasions, disease and insect outbreaks, drought-induced tree mortality and dieback) while simultaneously increasing understanding of these events and harnessing the predictive power of long-term datasets. As highlighted by recent efforts to increase adaptive monitoring whereby monitoring efforts adjust overtime to more efficiently capture spatiotemporal dynamic ecological processes (e.g., Hooten et al., 2009; Krause et al., 2015), we posit that targeting the event as it develops is an informative way to study these phenomena. Manipulative experiments, a core tool in ecology (Hairston, 1989; Peters, 1991; Sala et al., 2000; Scheiner and Gurevitch, 2001; Weltzin and McPherson, 2003), can identify the mechanisms underpinning responses and the potential thresholds that are difficult to identify post-hoc, and have successfully been used to improve ecological forecasting (Jiang et al., 2018). Additionally, regional surveys and associated summaries are effective for change detection associated with extreme climatic events (Hughes et al., 2018; Ruthrof et al., 2018; Fettig et al., 2019; Flake and Weisberg, 2019), and are especially useful given the often spatially heterogeneous nature of many ecological disturbances (e.g., Lybrand et al., 2018). There are a range of remote sensing technologies that can be used to augment regional field surveys and forecast changes at a broader spatial scale. These include an array of satellite imagery, including not only traditional multispectral imagery (Landsat, Sentinel-2, MODIS, VIIRS), but also lidar (GEDI), thermal (ECOSTRESS), radar (PALSAR, NISAR), microwave soil moisture (SMAP), gravimetric (GRACE), high temporal resolution geostationary imagery (GOES), and commercial cubesat constellations (e.g., Planet Labs). There are also emerging opportunities to leverage airborne and drone technologies to augment field data and/or satellite imagery and ultimately improve near-term ecological forecasting. We propose that adding rapidly implemented experimental and regional studies during developing extreme climate events can complement existing near-term ecological forecasting efforts to ultimately improve our capacity to forecast ecological responses to climate extremes.
Below we: (1) provide a framework for expanding near-term ecological forecasting by incorporating targeted experiments and regional surveys implemented rapidly during developing extreme climatic events; (2) illustrate our points with a case study, “chasing tree die-off”; and (3) provide examples of where this approach can be used to improve our ability to forecast other critical ecological processes.
Expanding Near-Term Ecological Forecasting to Opportunistically Exploit Extreme Events as they are Developing
A key aspect of near-term ecological forecasting to date is that it is generally based on ongoing long-term measurements at a single site or across a network of sites at regional or larger scales (Figure 1B). This is practical because there is substantial effort needed to launch an iterative and highly automated near-term ecological forecasting framework. The advantages of such a framework are sound and can aid management decisions (Dietze, 2017; Dietze et al., 2018). However, ecological changes are increasingly being driven by climate extremes, which are infrequent in time, variable in space, and therefore require a rapid-response capacity to capture observations or implement experiments. Some of these ecological changes, such as vegetation and biogeochemical responses to extreme drought (e.g., Ciais et al., 2005; Schlesinger et al., 2016) can potentially be anticipated during the development of the extreme event. Given the importance of extreme climatic events, we propose that rapidly implemented studies at local and regional scales can be a useful complement to other approaches based on ongoing long-term data collection (Figure 1A). A developing extreme climatic event can become the focus of an experimental study implemented rapidly within the impact location to increase return on investment, such as by implementing water additions as treatments during a developing drought (Jentsch et al., 2007). This type of effort is most likely to provide rapid useful information on the impacts of climate extremes, particularly concerning ecological thresholds. Similarly, opportunistic surveys at a regional scale can provide added insight on extreme event impacts. An excellent example of this is the rapid monitoring of an emerging influenza outbreak in 2009 to document and ultimately improve forecasting of influenza spread (Ong et al., 2010). These targeted regional surveys are especially useful in documenting spatially heterogeneous disturbances. The rapid approach and long-term approach are highly complementary: long-term monitoring sites can be used to generate forecasts during developing extreme events (e.g., Diffenbaugh et al., 2017) and insights learned from the rapid approach can subsequently be used to improve forecasts at these long-term monitoring sites.
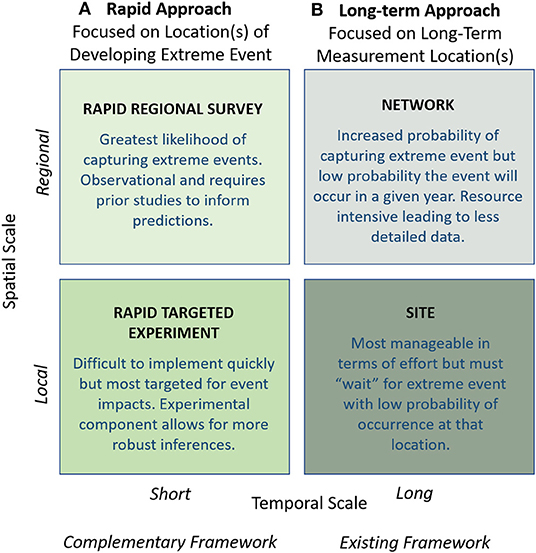
Figure 1. Approaches used for near-term ecological forecasting that vary in spatial and temporal scale. Rapid approaches (A) complement existing long-term studies (B) to improve near-term ecological forecasting of ecological events driven by extreme climate events. Blue text details the pros and cons of each spatiotemporal quadrant for near-term ecological forecasting.
Within this proposed expanded framework of near-term ecological forecasting (Figure 1), there are pros and cons to each spatiotemporal quadrant. In the simplest type of near-term ecological forecast, ongoing long-term data at a site are used to iteratively update predictions (Figure 1B, lower). This type of approach is most feasible in terms of effort because it is focused on a single site, allowing for intensive, detailed measurements, and access to long-term time series, which makes the specific timing of initiating the forecasting perhaps less critical (Dietze, 2017; Dietze et al., 2018). However, in this type of forecast, the study site has a high probability of being located outside the area impacted by an extreme climatic event and much data may need to be collected before the timing and location of an extreme event impact that site. Moving to a regional scale, with a network of long-term ongoing data (Figure 1B, upper) the probability of capturing an extreme climatic event within the network increases, although this depends on network density. Networks of sites are beneficial in that they are more spatially extensive relative to a single-site but, in general, have less detailed data due to the greater expense of maintaining multiple sites. Further, there is still the issue, albeit of less concern relative to a single site, of potentially waiting a long time for an extreme climatic event to occur at one of the sites within a network, particularly if there is a low density of sites. By observing progressive conditions for climate extremes such as a drought, efforts can potentially be rapidly deployed at a specific location within the area forecasted to be impacted by the extreme event (rapid targeted experiment; Figure 1A, lower). Similarly, at regional scales, a survey following an extreme climate event can document its impact (rapid regional survey; Figure 1A, upper), but this type of approach often relies on having preliminary data at a regional scale that can be built upon. This survey can be done using observations from on the ground field surveys and/or with remote sensing technologies to document ecological responses (e.g., Miller et al., 2006; Schepaschenko et al., 2019) and to also develop forecasts (e.g., Liu et al., 2019). These two types of efforts, can strongly enhance our understanding of the ecological consequences of extreme climate events and require less financial costs over-time, but can be challenging to implement because funding, site selection, experimental treatments (Figure 1A, lower only), and monitoring all need to occur quickly. Importantly, the rapid approach discussed here is most effective in climate extremes like drought that develop on slower time-scales. In summary, each of these four quadrats (Figure 1) can provide useful information for near-term ecological forecasting, and each has constraints. Furthermore, advances in statistical methods for iterative forecasting, and data fusion approaches for informing models with multiple data constraints, play a critical role when trying to combine information from these four quadrats (Dietze, 2017). We next illustrate how rapidly implemented local and regional scale studies provide useful insights into the left-hand column of the framework.
Chasing Tree Die-Off: A Case Study
We began tracking predictive maps provided by the US Drought Monitor (The National Drought Mitigation Center, 2019) and local weather stations during the winter of 2017/2018. The cumulative evidence predicted 2018 to be an exceptionally dry year that could lead to regional tree mortality comparable to 2002-2003 (Breshears et al., 2005). We received funding from the NSF-RAPID program to implement a two-part study integrating an experiment and regional surveys into near-term ecological forecasting to evaluate piñon pine (Pinus edulis) mortality during a developing drought in the US Southwest during 2018. We coupled a watering experiment at two sites (i.e., Figure 1A, lower) with a regional survey (i.e., Figure 1A, upper) to forecast mortality based on over a dozen published equations to predict mortality at varying spatiotemporal scales (Breshears et al., 2018).
Rapid Target Experiment
In April 2018, two sites were established in a piñon-juniper woodland separated by approximately 300 m in elevation. To test previously published hypotheses on how tree size and age affect mortality (e.g., Floyd et al., 2009; Meddens et al., 2015), we identified 24 clusters of trees at each site that consisted of one reproductively mature individual in close proximity to a sapling and a seedling. We randomly assigned each cluster of trees to one of three treatments: ambient (drought), small watering, and large watering. Watering treatments were done on May 21, 2018 by imposing an artificial rain event of two magnitudes by slowly saturating either the top 10 or 30 cm of soil. This was done in an effort to vary soil moisture across trees to refine mortality thresholds. We tracked soil moisture using a combination of handheld and permanent soil moisture probes through the duration of the experiment. We sampled pre-dawn water potential, stomatal conductance, and canopy percent brown from late May to mid-September at ~2-week intervals to assess water stress and tree mortality, and continued to sample canopy percent brown monthly through mid-November, 2018 to obtain final estimates of tree mortality. Mortality forecasts were updated ~every two weeks during the duration of the experiment based on our water stress measurements and compared to mortality data.
Rapid Regional Survey
The regional survey of piñon pine mortality was stratified by elevation and soil available water capacity across a 700 km region of Colorado and New Mexico to target sites that were anticipated to have variable levels of mortality. In October, 2018, we measured the size, vigor, and survival of all trees at 32 sites. Further, we recorded microsite conditions of all juvenile trees to refine predictions of nursing effects on juvenile survival under varying climate conditions (e.g., Redmond et al., 2015). The landscape to regional-scale mortality predictions for piñon pine focused on here can only be updated at an annual time-step (Breshears et al., 2018) and thus forecasts were done at the end of the study once all input climate data were available. Due to the short duration of this study (1 year), we were only able to perform iterative predictions for the finer spatiotemporal scale predictors assessed in the experimental survey and we were unable to assess whether mortality continued into the following growing season. Collectively, these two approaches (manipulative experiment and regional survey) allowed us to test previously published equations used to predict piñon pine mortality at varying spatial scales and refine future predictions.
Watering treatments in the rapid targeted experiment successfully increased soil moisture levels initially, with the small and large watering treatments resulting in a 4.7% and 11.6% increase in volumetric water content, respectively. This subsequently resulted in a trend of decreased tree water stress (i.e., less negative water potentials) at the low elevation site only. Yet the effect of watering on soil moisture rapidly declined—by 2 weeks soil moisture levels were equivalent between the treatments and there were no differences in mortality between treatments. The rapid target experiment was challenged by a 1,000-year rainfall event that occurred in July 2018 and resulted in very little (<3%) subsequent overstory mortality, despite predictions of high (>30%) mortality based on prior plant water potential thresholds identified in Adams et al. (2017). As a result, we documented the recovery of a population affected by drought following a substantial rain event despite exceeding previously established thresholds that closely linked extreme plant water potentials to mortality. Forecasting mortality based on data collected during the developing drought in the rapid target experiment (i.e., Figure 1A, lower) allowed us to refine previous predictions by providing data of where mortality was expected to occur, but ultimately did not occur. This highlights the importance of forecasting mortality during droughts rather than post-hoc investigations following known die-off events. Notably, the rapid regional survey (Figure 1A, upper) allowed us to sample areas where the developing drought continued to persist and ultimately lead to tree mortality (Wion et al., unpublished). Given uncertainties of forecasting climate extremes and the spatially heterogeneous nature of ecological disturbances, this two-part study reveals the benefit of integrating targeted experiments with regional surveys during an emerging drought to further improve ecological forecasting.
From our project, some of the more general insights that apply toward conducting rapid studies during developing extreme climate events (e.g., Figure 1A) include:
(1) The need to track developing events and their potential timeline. This is more challenging for extreme climate events that occur and end rapidly, such as heat waves, relative to extreme climate events that develop over longer time periods, such as drought.
(2) The ability to ramp up measurements rapidly and if appropriate install and initiate an experiment quickly. This includes challenges associated with quickly obtaining or redirecting funding and selecting sites on a tight timeline.
(3) Accepting uncertainty in projected climate extremes and, when possible, altering the sampling design (i.e., adaptive monitoring) as the event progresses, especially given the spatially heterogeneous nature of many ecological disturbances.
(4) Producing timely and iterative output under a short time period.
Example Opportunities that can Rapidly Exploit Developing Disturbances
We highlight five disparate examples of how coupling rapid experiments and/or regional surveys with near-term ecological forecasting can allow for efficient and effective investigation of extreme events (Table 1). These examples represent both aquatic and terrestrial responses to shifting climate drivers and are not an exhaustive list. We summarize some of the major challenges related to predicting and understanding these ecological events, while cataloging some of the opportunities available for future study using our expanded framework (see Table 1).
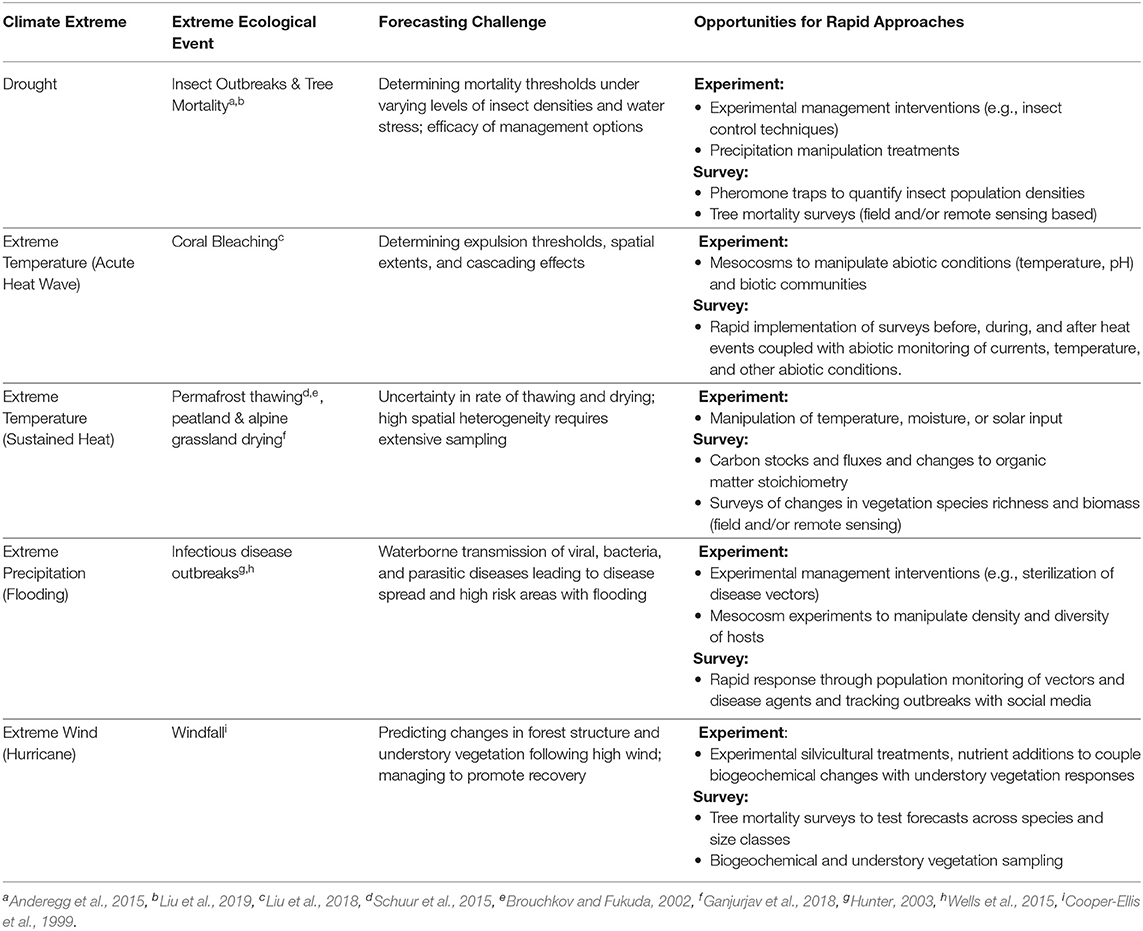
Table 1. Examples of where near-term ecological forecasting can be enhanced by experiments and regional surveys implemented rapidly during emerging climate extremes to improve our ability to forecast extreme ecological events and mitigate risk through early detection.
Conclusions
Climate change is occurring at such a rapid pace that the Anthropocene falls outside of the typical range of natural variability (Smith et al., 2009), creating a need for iterative near-term ecological forecasting (Dietze et al., 2018). Generally lacking from these near-term ecological forecasting efforts is the integration of shorter-term, opportunistic data associated with developing extreme events such as targeted experiments or regional surveys. In this Perspective, we propose that studies implemented rapidly during developing extreme events such as drought can provide useful complements to near-term ecological forecasting. Implementing targeted experiments and regional-scale surveys increase the likelihood of capturing highly spatially heterogeneous ecological disturbances and ultimately improve our ability to forecast the ecological responses to climate extremes. By drawing on advancements in adaptive monitoring whereby monitoring efforts adjust overtime to more efficiently capture spatiotemporal dynamic ecological processes, we posit that studies implemented rapidly during a developing extreme climate event can ultimately enhance our ability to forecast extreme ecological events. This approach has already been successfully used to study influenza outbreaks (Ong et al., 2010) and tree die-off in response to drought. Notably, this framework can be further expanded as near-term ecological forecasting models continue to be developed to not only target developing extreme climate events but to also use ecological forecasting predictions to target sampling efforts to test alternative hypotheses and ultimately refine hypotheses and predictions.
Author Contributions
DB conceived the idea with input from AW, CC, DL, JF, MR, NM, and NC. MR lead the writing of the paper, and AW, CC, DB, DL, and MR all wrote sections of the first draft of the paper. All authors contributed substantially to refinement of the ideas, examples provided in the text and in the table, and editing of the text.
Funding
This work was made possible by a collaborative NSF RAPID grant: NSF DEB-1833529 to Colorado State University, DEB-1833502 to University of Arizona, and DEB-1833505 to Northern Arizona University. Additional support was provided by EF-1340624, EF-1550756, EF-1638577, EAR-1331408, and EAR-1659546, and the Arizona Agricultural Research Station, and OISE-1748275 and OISE-1748204 for Near-Term Ecological Forecasting workshop participation.
Conflict of Interest
The authors declare that the research was conducted in the absence of any commercial or financial relationships that could be construed as a potential conflict of interest.
Acknowledgments
We are grateful to Chad Hoffman for providing feedback on examples of other extreme climatic events discussed in this article.
References
Adams, H. D., Zeppel, M. J. B., Anderegg, W. R. L., Hartmann, H., Landhäusser, S. M., and Tissue, D. T. (2017). A multi-species synthesis of physiological mechanisms in drought-induced tree mortality. Nat. Ecol. Evol. 1, 1285–1291. doi: 10.1038/s41559-017-0248-x
Allen, C. D., Breshears, D. D., and McDowell, N. G. (2015). On underestimation of global vulnerability to tree mortality and forest die-off from hotter drought in the Anthropocene. Ecosphere 6, 1–55. doi: 10.1890/ES15-00203.1
Allen, C. D., Macaladay, A. K., Chenchouni, H., Bachelet, D., McDowell, N., Vennetier, M., et al. (2010). A global overview of drought and heat-induced tree mortality reveals emerging climate change risks for forests. Forest Ecol. Manage. 259, 660–684. doi: 10.1016/j.foreco.2009.09.001
Anderegg, W. R. L., Hicke, J. A., Fisher, R. A., Allen, C. D., Aukema, J., Bentz, B., et al. (2015). Tree mortality from drought, insects, and their interactions in a changing climate. New Phytologist 208, 674–683. doi: 10.1111/nph.13477
Bradford, J. B., Betancourt, J. L., Butterfield, B. J., Munson, S. M., and Wood, T. E. (2018). Anticipatory natural resource science and management for a changing future. Front. Ecol. Environ. 16, 295–303. doi: 10.1002/fee.1806
Breshears, D. D., Carroll, C. J. W., Redmond, M. D., Wion, A., Allen, C. D., Cobb, N. S., et al. (2018). A dirty dozen ways to die: metrics and modifiers of mortality driven by drought and warming for a tree species. Front. For. Glob. Change 1:4. doi: 10.3389/ffgc.2018.00004
Breshears, D. D., Cobb, N. S., Rich, P. M., Price, K. P., Allen, C. D., Balice, R. G., et al. (2005). Regional vegetation die-off in response to global-change-type drought. Proc. Natl. Acad. Sci. 102:15144. doi: 10.1073/pnas.0505734102
Brouchkov, A., and Fukuda, M. (2002). “Preliminary measurements on methane content in permafrost, Central Yakutia, and some experimental data.” Permafr. Periglac. Process. 13, 187–197. doi: 10.1002/ppp.422
Chen, I.-C., Hill, J. K., Ohlemuller, R., Roy, D. B., and Thomas, C. D. (2011). Rapid range shifts of species associated with high levels of climate warming. Science 333, 1024–1026. doi: 10.1126/science.1206432
Ciais, P., Reichstein, M., Viovy, N., Granier, A., Ogée, J., Allard, V., et al. (2005). Europe-wide reduction in primary productivity caused by the heat and drought in 2003. Nature 437, 529–533. doi: 10.1038/nature03972
Clark, J. S., Carpenter, S. R., Barber, M., Collins, S., Dobson, A., Foley, J. A., et al. (2001). Ecological forecasts: an emerging imperative. Science 293, 657–660. doi: 10.1126/science.293.5530.657
Cooper-Ellis, S., Foster, D. R., Carlton, G., and Lezberg, A. (1999). Forest response to catastrophic wind: results from an experimental hurricane. Ecology 80, 2683–2696. doi: 10.1890/0012-9658(1999)080[2683:FRTCWR]2.0.CO;2
Coppus, R., and Imeson, A. C. (2002). Extreme events controlling erosion sediment transport in a semi-arid sub-Andean valley. Earth Surf. Process Landforms 27, 1365–1375. doi: 10.1002/esp.435
de Coninck, H., Revi, A., Babiker, M., Bertoldi, P., Buckeridge, M., Cartwright, A., et al. (2018). “Strengthening and implementing the global response.,” in: Global Warming of 1.5°C. An IPCC Special Report on the Impacts of Global Warming of 1.5°C Above Pre-industrial Levels and Related Global Greenhouse Gas Emission Pathways, in the Context of Strengthening the Global Response to the Threat of Climate Change, Sustainable Development, and Efforts to Eradicate Poverty, eds V. Masson-Delmotte, P. Zhai, H. O. Pörtner, D. Roberts, J. Skea, P.R. Shukla, et al. (Geneva), 313–443.
Dietze, M. C., Fox, A., Beck-Johnson, L. M., Betancourt, J. L., Hooten, M. B., Jarnevich, C. S., et al. (2018). Iterative near-term ecological forecasting: nNeeds, opportunities, and challenges. Proc. Natl. Acad. Sci. U.S.A. 115, 1424–1432. doi: 10.1073/pnas.1710231115
Diffenbaugh, N. S., Singh, D., Mankin, J. S., Horton, D. E., Swain, D. L., Touma, D., et al. (2017). Quantifying the influence of global warming on unprecedented extreme climate events. Proc. Natl. Acad. Sci. U.S.A. 114, 4881–4886. doi: 10.1073/pnas.1618082114
Fettig, C. J., Mortenson, L. A., Bulaon, B. M., and Foulk, P. B. (2019). Tree mortality following drought in the central and southern Sierra Nevada, California, U.S. Forest For. Ecol. Manage. 432, 164–178. doi: 10.1016/j.foreco.2018.09.006
Flake, S. W., and Weisberg, P. J. (2019). Fine-scale stand structure mediates drought-induced tree mortality in pinyon-juniper woodlands. Ecol. Appl. 29:e01831. doi: 10.1002/eap.1831
Floyd, M. L., Clifford, M., Cobb, N. S., Hanna, D., Delph, R., Ford, P., et al. (2009). Relationship of stand characteristics to drought-induced mortality in three southwestern piñon-juniper woodlands. Ecol. Appl. 19, 1223–1230. doi: 10.1890/08-1265.1
Ganjurjav, H., Hu, G., Wan, Y., Li, Y., Danjiu, L., and Gao, Q. (2018). Different responses of ecosystem carbon exchange to warming in three types of alpine grassland on the central Qinghai–Tibetan Plateau. Ecol. Evol. 8, 1507–1520. doi: 10.1002/ece3.3741
Gregory, R., Failing, L., Harstone, M., Long, G., McDaniels, T., and Ohlson, D. (2012). Structured Decision Making: A Practical Guide to Environmental Management Choices. New York, NY: Wiley. doi: 10.1002/9781444398557
Hairston, N. G. (1989). Ecological Experiments: Purpose, Design and Execution. Cambridge, UK: Cambridge University Press. doi: 10.1017/CBO9780511608513
Havens, K., Paerl, H., Phlips, E., Zhu, M., Beaver, J., and Srifa, A. (2016). Extreme weather events and climate variability provide a lens to how shallow lakes may respond to climate change. Water 8:229. doi: 10.3390/w8060229
Hayhoe, K., Wuebbles, D. J., Easterling, D. R., Fahey, D. W., Doherty, S, Kossin, J., et al. (2018). “Our Changing Climate,” in Impacts, Risks, and Adaptation in the United States: Fourth National Climate Assessment, Vol. II, eds D. R. Reidmiller, C. W. Avery, D. R. Easterling, K. E. Kunkel, K. L. M. Lewis, T. K. Maycock, and B. C. Stewart (Washington, DC: U.S. Global Change Research Program), 72–144. doi: 10.7930/NCA4.2018.CH2
Hobbs, N. T., Germia, C., Treanor, J., Wallen, R., White, P. J., Hooten, M. B., et al. (2015). State-space modeling to support management of brucellosis in the Yellowstone bison population. Ecol. Monogr. 85, 525–556. doi: 10.1890/14-1413.1
Hooten, M. B., Wikle, C. K., Sheriff, S. L., and Rushin, J. W. (2009). Optimal spatio-temporal hybrid sampling designs for ecological monitoring. J. Veg. Sci. 20, 639–649. doi: 10.1111/j.1654-1103.2009.01040.x
Hughes, T. P., Kerry, J. T., Baird, A. H., Connolly, S. R., Dietzel, A., Eakin, C. M., et al. (2018). Global warming transforms coral reef assemblages. Nature 556, 492–496. doi: 10.1038/s41586-018-0041-2
Hunter, P. R. (2003). Climate change and waterborne and vector-borne disease. J. Appl. Microbiol. 94, 37–46. doi: 10.1046/j.1365-2672.94.s1.5.x
Jentsch, A., Kreyling, J., and Beierkuhnlein, C. (2007). A new generation of climate-change experiments: events, not trends. Front. Ecol. Environ. 5, 365–374. doi: 10.1890/1540-9295(2007)5[365:ANGOCE]2.0.CO;2
Jiang, J. Y., Huang, S., Ma, M., Stacy, Z., Shi, D. M., Ricciuto, P. J., et al. (2018). Forecasting responses of a northern peatland carbon cycle to elevated co2 and a gradient of experimental warming. J. Geophys. Res: Biogeosciences Biogeosci. 123, 1057–1071. doi: 10.1002/2017JG004040
Kalnay, E. (2002). Atmospheric Modeling, Data Assimilation, and Predictability. Cambridge: Cambridge University Press. doi: 10.1017/CBO9780511802270
Ketz, A. C., Johnson, T. L., Monello, R. J., and Hobbs, N. T. (2016). Informing management with monitoring data: the value of Bayesian forecasting. Ecosphere 7:e01587. doi: 10.1002/ecs2.1587
Krause, S., Lewandowski, J., Dahm, C. N., and Tockner, K. (2015). Frontiers in real-time ecohydrology? A paradigm shift in understanding complex environmental systems. Ecohydrology 8, 529–537. doi: 10.1002/eco.1646
Kuikka, S., Vanhatalo, J., and Pulkkinen, H. (2014). Experiences in Bayesian inference in Baltic salmon management. Stat. Sci. 29, 42–49. doi: 10.1214/13-STS431
Liu, G., Eakin, C. M., Chen, M., Kumar, A., De La Cour, J. L., Heron, S. F., et al. (2018). Predicting heat stress to inform reef management: NOAA coral reef watch's 4-month coral bleaching outlook. Front. Mar. Sci. 5:57. doi: 10.3389/fmars.2018.00057
Liu, Y., Kumar, M., Katul, G. G., and Porporato, A. (2019). Reduced resilience as an early warning signal of forest mortality. Nature Climmate. Change. 9, 880–885: doi: 10.1038/s41558-019-0583-9
Lybrand, R., Gallery, R. E., Trahan, N., and Moore, D. J. P. (2018). Disturbance alters the relative importance of topographic and biogeochemical controls on microbial activity in temperate montane forests. Forests 9:97. doi: 10.3390/f9020097
Meddens, A. J. H., Hicke, J. A., Macalady, A. K., Buotte, P. C., Cowles, T. R., and Allen, C. D. (2015). Patterns and causes of observed piñon pine mortality in the southwestern United States. New Phytol. 206, 91–97. doi: 10.1111/nph.13193
Miller, D. W., Harding, L. W. Jr., and Afolf, J. E. (2006). Hurricane Isabel generated an unusual fall bloom in Chesapeake Bay. Geophys. Res. Lett. 33:L06612. doi: 10.1029/2005GL025658
Moritz, M. A., Moody, T. J., Krawchuk, M. A., Hughes, M., and Hall, A. (2010). Spatial variation in extreme winds predicts large wildfire locations in chaparral ecosystems. Geophys. Res. Lett. 37:L04801. doi: 10.1029/2009GL041735
Ong, J. B. S., Chen, M. I.-C., Cook, A. R., Lee, H. C., Lee, V. J., Lin, R. T. P., et al. (2010). Real-time epidemic monitoring and forecasting of H1N1-2009 using influenza-like illness from general practice and family doctor clinics in Singapore. PLOS ONE 5:e10036. doi: 10.1371/journal.pone.0010036
Redmond, M. D., Cobb, N. S., Clifford, M. J., and Barger, N. N. (2015). Woodland recovery following drought-induced tree mortality across an environmental stress gradient. Global Change Biology 21, 3685–3695. doi: 10.1111/gcb.12976
Ruthrof, K. X., Breshears, D. D., Fontaine, J. B., Froend, R. H., Matusick, G., Kala, J., et al. (2018). Subcontinental heat wave triggers terrestrial and marine, multi-taxa responses. Sci. Rep. 8:13094. doi: 10.1038/s41598-018-31236-5
Sala, O. E., Jackson, R. B., Mooney, H. A., and Howarth, R. W., (eds.). (2000). “Chapters 19-24: Part 3. Manipulative ecosystem experiments,” in Methods in Ecosystem Science (New York, NY: Springer-Verlag), 289-369.
Scheiner, S. M., and Gurevitch, J. (2001). Design and Analysis of Ecological Experiments. Oxford: Oxford University Press.
Schepaschenko, D. J., Chave, O. L., Phillips, S. L., Lewis, S. J., Davies, M., Réjou-Méchain, M., et al. (2019). The forest observation sSystem, building a global reference dataset for remote sensing of forest biomass. Sci. Data 6, 1–11. doi: 10.1038/s41597-019-0196-1
Schlesinger, W. H., Dietze, M. C., Jackson, R. B., Phillips, R. P., Rhoades, C. C., Rustad, L. E., et al. (2016). Forest biogeochemistry in response to drought. Glob. Chang. Biol. 22, 2318–2328. doi: 10.1111/gcb.13105
Schuur, E. A. G., McGuire, A. D., Schädel, C., Grosse, G., Harden, J. W., Hayes, D. J., et al. (2015). Climate change and the permafrost carbon feedback. Nature 520, 171–179. doi: 10.1038/nature14338
Shaman, J., and Karspeck, A. (2012). Forecasting seasonal outbreaks of influenza. Proc. Natl. Acad. Sci. 109, 20425–20430. doi: 10.1073/pnas.1208772109
Sheppard, C. S., Alexander, J. M., and Billeter, R. (2012). The invasion of plant communities following extreme weather events under ambient and elevated temperature. Plant Ecol. 213, 1289–1301. doi: 10.1007/s11258-012-0086-5
Shuman, F. (1989). History of numerical weather prediction at the National Meteorological Center. Weather Forecast. 4, 286–296. doi: 10.1175/1520-0434(1989)004<0286:HONWPA>2.0.CO;2
Smith, M. D. (2011). An ecological perspective on extreme climatic events: a synthetic definition and framework to guide future research. J. Ecol. 99, 656–663. doi: 10.1111/j.1365-2745.2011.01798.x
Smith, M. D., Knapp, A. K., and Collin, S. L. (2009). A framework for assessing ecosystem dynamics in response to chronic resource alterations induced by global change. Ecology 90, 3279–3289. doi: 10.1890/08-1815.1
Stumpf, R. P., Tomlinson, M. C., Calkins, J. A., Kirkpatrick, B., Fisher, K., Nierenberg, K., et al. (2009). Skill assessment for an operational algal bloom forecast system. J. Marine Systems 76, 151–161. doi: 10.1016/j.jmarsys.2008.05.016
The National Drought Mitigation Center (2019). The U.S. Drought Monitor. Available online at: https://droughtmonitor.unl.edu/ (accessed November 2017 – October 2018).
Thomas, R. Q., Brooks, E. B., Jersild, A. L., Ward, E. J., Wynne, R. H., Albaugh, T. J., et al. (2017). Leveraging 35 years of Pinus taeda research in the southeastern US to constrain forest carbon cycle predictions: regional data assimilation using ecosystem experiments. Biogeosciences 14, 3525–3547. doi: 10.5194/bg-14-3525-2017
Ummenhofer, C. C., and Meehl, G. A. (2017). Extreme weather and climate events with ecological relevance: a review. Philos. Trans. Royal Soc. B Biol. Sci. 372:20160135. doi: 10.1098/rstb.2016.0135
Wells, M. L., Trainer, V. L., Smayda, T. J., Karlson, B. S. O., Trick, C. G., Kudela, R. M., et al. (2015). Harmful algal blooms and climate change: learning from the past and present to forecast the future. Harmful Algae 49, 68–93. doi: 10.1016/j.hal.2015.07.009
Weltzin, J. F., and McPherson, G. R., (eds.). (2003). “Assessing response of terrestrial populations, communities, and ecosystems to changes in precipitation regimes. Progress to date and future directions,” in Changing Precipitation Regimes and Terrestrial Ecosystems (Tuscon, AZ: University of Arizona Press), 180–188.
Keywords: ecological forecasting, adaptive monitoring, anticipatory science, disturbance, climate change, climate extremes, extreme climatic event, drought
Citation: Redmond MD, Law DJ, Field JP, Meneses N, Carroll CJW, Wion AP, Breshears DD, Cobb NS, Dietze MC and Gallery RE (2019) Targeting Extreme Events: Complementing Near-Term Ecological Forecasting With Rapid Experiments and Regional Surveys. Front. Environ. Sci. 7:183. doi: 10.3389/fenvs.2019.00183
Received: 25 February 2019; Accepted: 31 October 2019;
Published: 27 November 2019.
Edited by:
Jesús Julio Camarero, Spanish National Research Council (CSIC), SpainReviewed by:
Nathaniel K. Newlands, Agriculture and Agri-Food Canada (AAFC), CanadaCate Macinnis-Ng, The University of Auckland, New Zealand
Copyright © 2019 Redmond, Law, Field, Meneses, Carroll, Wion, Breshears, Cobb, Dietze and Gallery. This is an open-access article distributed under the terms of the Creative Commons Attribution License (CC BY). The use, distribution or reproduction in other forums is permitted, provided the original author(s) and the copyright owner(s) are credited and that the original publication in this journal is cited, in accordance with accepted academic practice. No use, distribution or reproduction is permitted which does not comply with these terms.
*Correspondence: Miranda D. Redmond, bWlyYW5kYS5yZWRtb25kQGNvbG9zdGF0ZS5lZHU=