- 1Irstea, UR MALY Laboratoire D'écotoxicologie, Centre de Lyon-Villeurbanne, Villeurbanne, France
- 2Laboratoire Innovations Technologiques pour la Détection et le Diagnostic, Service de Pharmacologie et Immunoanalyse, Commissariat à l'Energie Atomique et aux Energies Alternatives, Institut National de la Recherche Agronomique, Bagnols-sur-Cèze, France
Taxon-specific proteins from sentinel species have been shown to be among the most relevant proteins to monitor the biological effects of anthropogenic contaminations. Shotgun proteomics allows high-throughput analysis of animal proteomes, but comprehensive coverage remains difficult to achieve due to the wide dynamic range. Here, the advantages of a new generation of mass analyser for environmental toxicoproteomics are documented through the identification of candidate biomarkers in a sentinel animal species for which the genome is as yet unsequenced. A label-free comparative proteomic study was performed on testis from the freshwater amphipod Gammarus fossarum challenged with 0.5 μg or 50 μg/L pyriproxyfen, a juvenile hormone analog used as an insecticide. A total of 4,031 proteins could be monitored with a Q Exactive HF mass spectrometer incorporating an ultra-high-field Orbitrap analyser. Five times more MS/MS spectra and 4-fold more peptides and proteins were identified with this instrument compared to a LTQ Orbitrap XL spectrometer. Comparison of protein abundance between control and organisms exposed to 0.5 μg or 50 μg/L pyriproxyfen indicated that 32 and 21 proteins, respectively, were significantly modulated between test and control conditions. Functional annotation of these novel candidate biomarkers and their specificities are discussed in light of their potential applications in freshwater quality monitoring. The potential contribution of next-generation mass spectrometers to environmental toxicoproteomics is also touched on.
Introduction
Ecotoxicological assessment is based on expert knowledge of the physiological response of sentinel species and the use of biomarkers to monitor toxic effects of contaminants on these organisms. Clearly, today we need to better understand the molecular mechanisms of homeostasis and response to environmental chemical stressors in numerous models, and global omics approaches are contributing to this understanding (Monsinjon and Knigge, 2007; Garcia-Reyero and Perkins, 2011). Proteomics is increasingly frequently used in environmental toxicology since, compared to gene expression studies, protein modulation is more likely to be linked to phenotypic changes (Sellami et al., 2015; Campos et al., 2016; Ilyas et al., 2016; Rajapakse et al., 2016). Proteomics allows exhaustive comparison of proteins from controls and environmentally challenged organisms following laboratory or field exposure. These comparisons can be used to determine how chemical contaminants work and to propose new biomarkers for environmental risk assessment. Up to now, most proteomics studies in ecotoxicology have reported alterations to levels of housekeeping and abundant proteins, such as those involved in ATP supply and maintenance of the cytoskeletal structure, and therefore tended to list known proteins as differentially expressed biomarker candidates (Petrak et al., 2008). The main reasons for these results are: (i) the use of 2D-PAGE, which favors the detection of highly abundant proteins, and (ii) a lack of species-specific protein sequence databases, which limits interpretation of mass spectrometry data to species currently used in environmental monitoring (Trapp et al., 2014a). Compared to 2D-PAGE, shotgun proteomics allows deeper proteome characterisation and increases the number of proteins identified, therefore allowing detection of under-represented proteins (Armengaud, 2016). This technique consists in the identification of proteins from complex mixtures by high-throughput tandem mass spectrometry without requiring prior protein separation on 2D-PAGE. To do so, the whole-protein extract is proteolysed with trypsin; the resulting peptides are resolved by high-performance liquid chromatography (HPLC) and identified using high-throughput tandem mass spectrometry. Once identified, comparative protein amounts can be readily determined by counting the number of MS/MS spectra. Although not an absolute quantification method, this approach makes it possible to compare the relative quantities of each protein detected in different conditions if equivalent samples are analyzed.
Approaches combining genomics and proteomics data known as proteogenomics are a promising alternative to homology-driven proteomics when working with species or taxa that are underrepresented in genomics databases (Armengaud et al., 2014). Indeed, proteogenomics allows higher rates of protein identification than were possible a decade ago (Nesvizhskii, 2014). To rapidly identify protein sequences in eukaryotes through a proteogenomics approach, mRNAs are sequenced by next-generation sequencing technology and the resulting sequences are translated into all possible reading frames to produce a specific protein database. Although most sequences in this database will be erroneous, it nevertheless contains the relevant protein sequences. This customized draft predicted protein sequence database can then be used to interpret MS/MS data, allowing the identification of sequences corresponding to real proteins (Armengaud et al., 2014).
Recently, we applied a proteogenomics strategy to discover key reproductive proteins from the freshwater crustacean Gammarus fossarum, a sentinel species used in ecotoxicology. The transcriptome from the reproductive organs was established by RNAseq and the proteome was studied using shotgun proteomics (Trapp et al., 2014b). This initial study identified 1,873 proteins certified by mass spectrometry, including 218 orphans. To reveal new candidates involved in G. fossarum's reproductive process, we compared the abundance of proteins in male and female reproductive tissues. This analysis identified 204 sexually-dimorphic proteins. In addition, comparative proteomics of testicular extracts at seven different stages during spermatogenesis identified proteins potentially involved in male reproduction. We also performed a proteomics analysis in testis from G. fossarum exposed to three different xenobiotics in order to propose specific biomarker candidates for toxicity assays (Trapp et al., 2015). Interestingly, a substantial proportion of the candidates identified in these experiments were orphans or highly divergent proteins, thus they would not have been accessible by traditional homology-driven proteomics as few crustacean genome sequences have been obtained and annotated to date. As a result of sexual selection, reproductive proteins are highly diversified in the tree of life, and they consequently tend to be highly species-specific (Swanson and Vacquier, 2002). Furthermore, as first revealed by transcriptomic analysis on Daphnia pulex, orphan genes are among the most eco-responsive to environmental challenges (Colbourne et al., 2011). We firmly believe that highly divergent proteins are essential to environmental toxicology (Trapp et al., 2014a), and thus proteogenomics approaches with environmental organisms should flourished in the near future (Armengaud et al., 2014). Monitoring specific proteins on environmental samples using multiplexed targeted proteomics is an attractive method to validate candidate biomarkers discovered by proteogenomics and to perform environmental monitoring (Charnot et al., 2017; Gouveia et al., 2017a,b).
Without adequate proteome fractionation, shotgun strategies, like 2D-PAGE, preferentially detect abundant proteins, whereas species-specific proteins are generally only present in low-abundance. Increasing mass spectrometry efforts by multiplying the number of fractions and extending nanoLC-MS/MS runs are time-consuming and thus limits the number of samples that can be treated and compared. Novel generations of high-resolution mass spectrometers are periodically released, with enhanced resolution and scan speed that improve detection rates for low-abundance ions in complex matrices. These next-generation mass spectrometers are therefore attractive as they should make possible to obtain more data from the same sample. Indeed, the depth of proteome coverage is increasingly high, and one of the most recently released instruments, the Q Exactive HF tandem mass spectrometer (Thermo), allowed the detection of a set of 8,526 proteins from human tissue (Wei et al., 2016).
Two major distinct hormone classes constitute the endocrine system of arthropods: ecdysteroids, known as the molting hormone; and juvenoid hormones. The juvenoid analog pyriproxyfen is used in agriculture as a growth regulator to inhibit the larval development and maturation of many arachnid and insect pests: ticks, mosquitos, ants, houseflies, and fleas (Sullivan and Goh, 2008). This molecule also affects crustaceans, inducing morphological abnormalities and limiting growth rates in decapods (LeBlanc, 2007), decreasing female fecundity and altering the sex ratio in branchiopods (Olmstead and LeBlanc, 2003; Ginjupalli and Baldwin, 2013), and reducing sperm production in amphipods (Trapp et al., 2015).
The objective of this study was to document the performance of the latest Orbitrap detector, named ultra-high-field Orbitrap, in an environmental ecotoxicology context using proteogenomics approaches. Two mass spectrometers currently contain this type of detector: the Fusion/Lumos tribrid mass spectrometer and the Q Exactive HF/HF-X hybrid tandem mass spectrometer, both of which are produced by Thermo (Scheltema et al., 2014). For this study, we analyzed the testis proteome of the amphipod G. fossarum to identify a larger set of candidate biomarkers of male reproductive impairment induced by exposure to an endocrine disruptor. To do so, we analyzed the proteomes of control organisms and organisms exposed to the insecticide pyriproxyfen. Using an LTQ Orbitrap XL tandem mass spectrometer, 935 proteins were validated, whereas 4,031 proteins were validated when using the Q Exactive HF instrument. A comparison of protein abundance between control and exposed organisms revealed significant modulation of 17 and 53 proteins between the contaminated condition and the control when using the LTQ Orbitrap XL and Q Exactive HF, respectively. These novel proteins were detected at altered abundance in pyriproxyfen-exposed animals and could be relevant for environmental toxicology monitoring while also potentially representing novel biomarkers of reproductive disorders in G. fossarum.
Materials and Methods
Exposing Gammarids to Pyriproxyfen and Protein Extraction
G. fossarum amphipods were collected from the Bourbre river in mid-eastern France (45°34′10″N; 5°27′33″E) by kick sampling, and acclimatized to laboratory conditions in 30-L tanks. Male organisms were exposed to 0.5 μg/L or 50 μg/L pyriproxyfen diluted in acetone (0.005% final concentration) over two consecutive spermatogenesis cycles (15 days), as previously described (Trapp et al., 2015). For each condition, 35 males were placed into five 500-mL polyethylene beakers (seven animals per beaker), under constant oxygenation. A piece of netting (6 × 5 cm) was added to each beaker as a resting surface. Control conditions were exposure to 0.005% acetone (solvent) or no exposure. Organisms were fed ad libitum with conditioned alder leaves (Alnus glutinosa). Throughout the experiment, exposure media and food source were renewed every 2 days. After 1 week in these conditions, mature females were placed with the males to allow fertilization, thus triggering a second spermatogenesis cycle. Survival was checked daily. The experiment was ended 7 days after the second fertilization. Male gonads were collected from amphipods under stereomicroscopic magnification using fine forceps. The reproductive organs from five animals were analyzed by shotgun proteomics for each condition. Each pair of testes was directly dissolved in 40 μL of LDS sample buffer (Invitrogen). After sonication for 1 min (transonic 780H sonicator), samples were boiled for 5 min at 95°C. A 35-μL aliquot of each sample was then subjected to SDS-PAGE for a short electrophoretic migration, as described previously (Trapp et al., 2015). The whole-protein content from each well was extracted as a single polyacrylamide band, processed as described (Hartmann et al., 2014), and submitted to proteolysis with trypsin (Roche) using 0.01% ProteaseMAX surfactant (Promega).
Q Exactive HF and LTQ Orbitrap XL Mass Spectrometry Settings
The peptide mixtures were analyzed in data-dependent mode on two tandem mass spectrometers. The parameters used with the LTQ Orbitrap XL hybrid mass spectrometer (ThermoFisher) combined with an UltiMate 3000 LC system (Dionex-LC Packings) were as previously described (Trapp et al., 2016). Briefly, a volume of 2 μl of peptide samples was loaded by the LC system. Peptides were desalted online on a reverse-phase precolumn, C18 PepMap 100 precolumn (LC Packings), and then resolved on a nanoscale C18 PepMapTM 100 capillary column (LC Packings) at a flow rate of 0.3 μl/min with a gradient of CH3CN, 0.1% formic acid prior to injection into the ion trap mass spectrometer. Peptides were resolved upon their hydrophobicity using a 90 min gradient from 5 to 60% solvent B (0.1% HCOOH, 80% CH3CN). Solvent A was 0.1% HCOOH, 100% H2O. The following tune method was used: a full scan spectral range from m/z 350 to 1800, an Automatic Gain Control (AGC) target value set at 5 × 105, and a mass resolving power of 30,000. MS/MS were analyzed in data-dependent mode with a minimum signal required set to 15,000 and a Top5 method. The Q Exactive HF mass spectrometer (Thermofisher), also associated with an UltiMate 3000 LC system (Dionex-LC Packings), was operated essentially as described (Klein et al., 2016). Briefly, peptides were first desalted on-line on a reverse-phase C18 PepMap 100 precolumn (LC Packings), before being resolved onto a nanoscale C18 PepMapTM 100 capillary column (LC Packings) with a 90-min gradient of CH3CN, 0.1% formic acid, at a flow rate of 0.2 μL/min. Peptides were analyzed by applying a data-dependent Top15 method consisting in a scan cycle initiated by a full scan of peptide ions in the Orbitrap analyser, followed by high-energy collisional dissociation and MS/MS scans on the 15 most abundant precursor ions. Full scan mass spectra were acquired from m/z 350 to 1800 with an AGC target set at 3 × 106 ions and a resolution of 60,000. MS/MS scan was initiated when the ACG target reached 105 ions with a threshold intensity of 83,000 and potential charge states of 2+ and 3+. Ion selection was performed applying a dynamic exclusion window of 10 sec.
Protein Identification and Spectral Count Quantification
MS/MS spectra were assigned to peptide sequences by the MASCOT Daemon 2.3.2 search engine (Matrix Science) searching against the customized RNAseq-derived database GFOSS, which is described by Trapp et al. (2014b). This database contains 1,311,444 putative protein sequences totalling 289,084,257 amino acids. The following parameters were used for MS/MS spectra assignment: full-trypsin specificity, maximum of two missed cleavages, mass tolerances of 5 ppm on the parent ion and 0.5 Da on the MS/MS acquired with the LTQ Obitrap XL and 0.02 Da on the MS/MS acquired with the Q Exactive HF, carboxyamidomethylated cysteine (+57.0215) as a fixed modification, and oxidized methionine (+15.9949) and deamidation of asparagine and glutamine as variable modifications. All peptide matches presenting a MASCOT peptide score with a p-value of < 0.05 were filtered and assigned to a protein according to the parsimony principle. At least two different peptide sequences were required for protein validation. The false discovery rate for protein identification was estimated to be < 0.1% based on MASCOT's (Matrix Science) decoy search option. All mass spectrometry proteomics data have been uploaded to the ProteomeXchange Consortium (Vizcaíno et al., 2013) via the PRIDE partner repository under dataset identifier PXD008622 and Project DOI 10.6019/PXD008622 [This dataset can be accessed by reviewers using “reviewer83847@ebi.ac.uk” as username and “tbmMV2he” as password].
Differential Shotgun Proteomics Data Analysis
Four protein datasets were produced: Control without solvent consisting in 0.005% acetone (T); Control with solvent consisting in 0.005% acetone (TS); 0.5 μg/L pyriproxyfen treatment; and 50 μg/L pyriproxyfen treatment. Each of these conditions were analyzed in five separate biological replicates. Spectral counts were extracted for each protein and each condition by counting the number of MS/MS spectra assigned to a given protein accession. The normalized spectral abundance factor (NSAF) was calculated by aggregating the spectral counts of a given polypeptide from each replicate divided by its molecular weight, and expressed in percentage for direct comparison of the whole dataset. The Tfold module of the PatternLab programme for proteomics [version 4.0.0.59; (Carvalho et al., 2016)] was used to normalize data based on the total signal and to compare proteomics datasets based on spectral count, as previously described (Hartmann and Armengaud, 2014). Selection of proteins were done only considering proteins detected in at least three of the different biological replicates. The L-stringency parameter was set at 0.6 and the F-stringency parameter was optimized as recommended (Carvalho et al., 2016). To determine the set of proteins for which expression levels were modulated, the fold-change was calculated by comparing spectral counts for each protein dataset to those for the control. The p values were calculated by the PatternLab software based on a Student t-test taking into account the five biological replicates. Proteins satisfying both the fold-change and statistical criteria were considered potential protein signatures of pyriproxyfen treatment.
Protein Annotation and Gene Ontology Classification
Functional annotation was performed using the DIAMOND open source programme (Buchfink et al., 2015) targeting the SWISSPROT database (http://www.uniprot.org/; downloaded on 2016/09/30) to extract the most similar proteins. Only alignments with an expected value of ≤ 0.001 were considered. The report presents those with a bit score >40. Gene Ontology (GO) annotation was then performed using data provided by the GO Consortium through the GO website and the web application Amigo (http://amigo.geneontology.org). Two levels (1 and 2) of GO annotation were extracted.
Graph Drawing and Hierarchical Clustering
Graphs were drawn with the Excel software (Microsoft). Heatmap was produced using the function heatplot from the software package MADE4 (version 1.52.0) proposed by Culhane et al. (Culhane et al., 2005) under R (version 3.4.3) environment (https://bioconductor.org/packages/relea se/bioc/html/made4.html). Heatplot draws dendrograms of the cases and variables using correlation similarity metric and average linkage clustering.
Results and Discussion
Numbers of Proteins Identified by Each Mass Spectrometer
Organisms were exposed over two consecutive spermatogenetic cycles (i.e., 2 weeks) to 0.5 or 50 μg/L of pyriproxyfen, and data were compared to those for a solvent control. No significant mortality was observed during exposure, with survival rates in all conditions exceeding 85%. To assess the effects of pyriproxyfen in terms of protein content, the testes from five individuals were dissected for each condition. Label-free shotgun proteomics analysis was performed on the testes extracted from individual organisms using two different high-resolution instruments: the LTQ Orbitrap XL and the Q Exactive HF hybrid tandem mass spectrometers. Their performances are different in terms of scan speed. This level of performance can only be achieved if the reverse-phase column delivers sufficient peptide ions throughout the applied gradient, thus performance should be measured with real proteomics samples. A total of 1,106,968 MS/MS spectra were recorded with the Q Exactive HF instrument when analyzing the 20 samples, whereas a total of 222,436 MS/MS spectra were recorded with the LTQ Orbitrap XL instrument. Thus the most recent tandem mass spectrometer offers 5-fold increase in spectrum acquisition capacity compared to the previous generation. The proteins identified from the LTQ Orbitrap XL and the Q Exactive HF data are listed in Supplementary data Table S1 and Table S2, respectively. Table 1 lists the mean numbers of MS/MS spectra recorded, assigned spectra, unique peptide sequences and proteins identified for the four experimental conditions from data produced by the two instruments. The number of MS/MS spectra per run produced by the LTQ Orbitrap XL instrument or the Q Exactive HF was relatively similar across the samples analyzed (relative standard deviation <20%), thus both analytical platforms are relatively stable. The spectra recorded with the two instruments were assigned to peptide sequences from the same proteogenomics database (GFOSS) derived from RNAseq information (Trapp et al., 2014b). The MS/MS spectrum attribution rate was considerably improved with the Q Exactive HF data, from which 22% of the total spectra (241,135 MS/MS spectra) were assigned, compared to only 13% from the LTQ Orbitrap XL data (28,275 MS/MS spectra). This higher rate of MS/MS spectrum attribution directly correlated with the higher quality of the MS/MS spectra. The faster acquisition rate and quadrupole filtering properties of the Q Exactive HF resulted in lower spectrum contamination. Because of the increase in MS/MS quality and number of MS/MS spectra acquired, the total number of assigned MS/MS spectra with the Q Exactive HF was 8.5-fold greater than with the LTQ Orbitrap XL instrument with these samples. In terms of the number of unique peptide sequences, a greater difference was observed, with a total of 3,103 non-redundant peptide sequences identified with the LTQ Orbitrap XL, compared to 14,818 with the Q Exactive HF. As shown in Figure 1A, a total of 2,961 peptides were common to both datasets. The resulting proteome sequence coverage was much higher with the Q Exactive HF instrument compared to the LTQ Orbitrap XL platform (x4.8). In terms of proteins identified, a total of 935 proteins were validated with at least two unique peptides from the LTQ Orbitrap XL data, whereas 4,031 were confirmed using the Q Exactive HF. Thus, at the protein level, the Q Exactive HF allowed the discovery of 3,325 additional proteins, as 706 overlapped with the LTQ Orbitrap XL analysis. Consequently, 229 proteins were only detected by the LTQ Orbitrap XL (Figure 1B). Obviously, these 229 proteins are among the lowest detected proteins in this dataset, as they represent 13% of the NSAF, so a mean contribution of 0.05% each. These data demonstrate that the Q Exactive HF identified almost 5-fold more peptides and allowed validation of five times more proteins than the LTQ Orbitrap XL when operated with the same gradient length. This performance enhancement can be explained by the faster scan rate (20 Hz with the Q Exactive HF, compared to 4 Hz with the LTQ Orbitrap XL) possible thanks to the ultra-high-field Orbitrap analyser and the instrument configuration incorporating a quadrupole rather than a linear trap (Michalski et al., 2011).
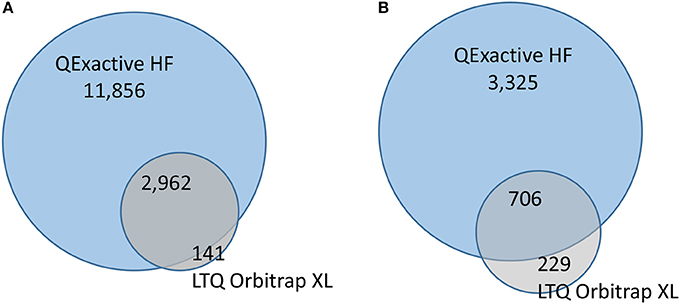
Figure 1. Peptides and proteins identified by the two mass spectrometry platforms. (A). Numbers of peptide sequence-spectrum matches. (B) Numbers of proteins identified by at least 2 peptide sequences.
Proteome Features Specific to Each Mass Spectrometer
For the two mass spectrometers, Table 2 lists the most abundant proteins identified in the G. fossarum testis proteome in the combined data from the 20 individual proteomes. The abundances of these 14 proteins were estimated based on their NSAF. Most of these proteins perform general housekeeping functions such as cytoskeletal structure maintenance (actin and myosin) and energy or metabolic homeostasis (arginine kinases for maintenance of ATP levels). The preponderance of histones, arginine kinase, and immune proteins (hemocyanin-like) in the G. fossarum testis proteome has previously been reported (Trapp et al., 2015). Reassuringly, all the components of this short-list of most abundant proteins were identified with both analytical systems. Moreover, their relative quantities were roughly estimated to be the same range (Table 2). However, the 14 most abundant proteins together represent almost 29% of the total proteome in terms of abundance when measured with the LTQ Orbitrap XL, but only 13% when proteins were quantified by the Q Exactive HF instrument. This difference is directly linked to the increased proteome coverage possible with the Q Exactive HF instrument, which allows analysis of a higher dynamic range. When considering only the 14 most abundant proteins when calculating the NSAF factors (NSAF-14), the ratio of each protein was quite similar with the two analytical systems as the mean standard deviation is 23% (Table 2).
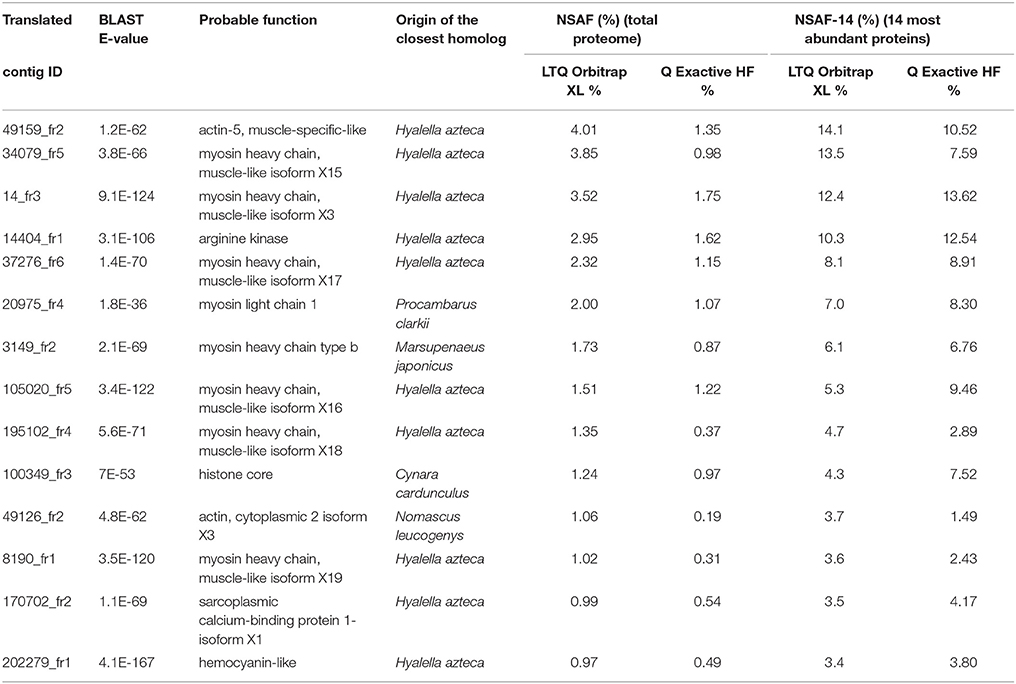
Table 2. List of the most abundant proteins in the total proteome identified by the LTQ Orbitrap XL and by the Q Exactive HF instruments.
Figure 2 shows the distribution of the number of non-redundant peptides as a function of protein abundance. To produce this graph, all detected proteins were ranked by quintile according to abundance, as measured by their NSAF. As expected, the most abundant proteins were detected based on numerous different peptide sequences, while low-abundance proteins were mostly detected based on just a few peptides. This classification was done for both datasets. As indicated in Figure 2, the mean ratio of peptide sequences was roughly comparable for each of the five classes of proteins identified from data produced by the two analytical platforms. However, the numbers of proteins detected were quite different. Interestingly, the distribution of the number of peptides per protein was slightly more variable for the Q Exactive HF. This phenomenon is also illustrated by the outliers, represented by the empty circles in Figure 2. This observation is especially true for low-abundance proteins and could explain the higher variability of data recorded with this instrument. It has long been known that proteins at low concentrations produce more variable results in terms of protein confidence and coverage (Pereira-Medrano et al., 2007). Indeed, even with exhaustive MS-based proteomics discovery experiments, proteins can be inconsistently observed across samples due to stochastic sampling of complex proteomes (Gillette and Carr, 2013). This effect is exacerbated with low-abundance and/or small proteins, since the detection of an additional peptide considerably increases the protein validation threshold and coverage, as well as its quantification based on spectral count.
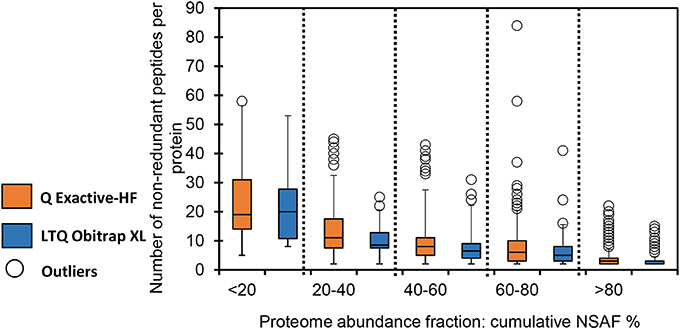
Figure 2. Distribution of the number of non-redundant peptides per protein based on their abundance in the whole proteome. Boxes extend from the first to the third quartile; the bold segment indicates the median value; the whiskers extend to the most extreme data points, no more than 1.5 times the interquartile range. Data points at more than 1.5 times the interquartile range are represented by empty circles (outliers). Orange: Q-Exactive HF dataset; blue: LTQ Orbitrap XL dataset.
A total of 4,261 different translated contigs/polypeptides were identified when all the data were merged. Figure 3 shows the protein-detection saturation curve for the proteins ranked by relative abundance. The cumulated NSAF percentage was calculated for all proteins ranked from the most abundant to the least abundant. From this analysis, we determined that 50% of the proteome content corresponded to the 53 most abundant proteins identified in the LTQ Orbitrap XL data, and the 159 most abundant proteins identified from the Q Exactive HF data; 80% of the proteome corresponded to 225 proteins with the LTQ Orbitrap XL platform compared to 692 proteins with the Q Exactive HF. As shown in Figure 3, the Q Exactive HF instrument also outperformed the LTQ Orbitrap XL platform when detecting low-abundance proteins. The 20% of the proteome corresponding to the least abundant proteins represented only 281 proteins with the LTQ Orbitrap instrument, compared to 3,341 proteins identified from the Q Exactive HF data. It is thus clear that the new generation of mass spectrometer improves identification of low-abundance proteins with enhanced discovery capacities, without incurring major reductions in protein sequence coverage.
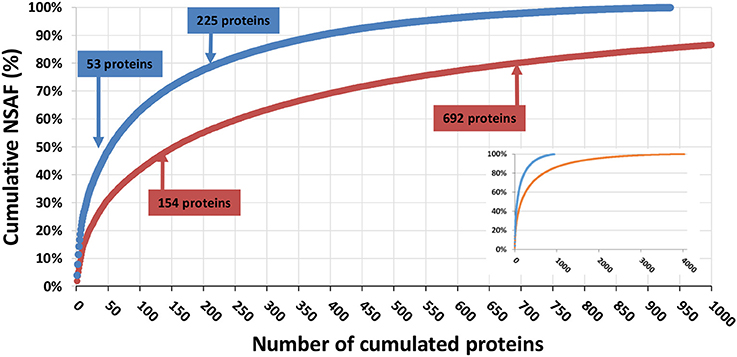
Figure 3. Saturation curve for number of proteins identified. Proteins detected by the Q Exactive HF (orange) or LTQ Orbitrap XL (blue) were ranked from the most to the least abundant (x axis). Their cumulated abundance, as determined based on their NSAF, is represented in terms of percentage of the overall proteome (y axis). The inset presents the same figure with a fully extended x axis.
Systematic Functional Annotation of the Proteins Identified
Functional annotation of the whole dataset of 4,261 proteins identified by the shotgun approach was performed by means of cross-species prediction based on sequence similarity searches. A total of 3,606 proteins were functionally annotated thanks to identification of relatively similar sequences (threshold E-value set at 1E−10). Table S1 and Table S2 list the 809 and 3,430 annotated proteins that were recorded with the LTQ Orbitrap XL and the Q Exactive HF instruments, respectively, and their corresponding GO terms. Although the proteomics analysis was performed on testis extracts, perhaps due to a lack of molecular knowledge with regard to crustacean proteins, the GO analysis only revealed a small number of biological processes or molecular functions more specifically related to reproductive functions. Thus, only four proteins in the testis proteome were identified by GO terms reflecting the biological process of reproduction: Contig_Gammarus_90_85071_fr3 which encodes a Laminin-like protein epi-1 with strong similarities to Caenorhabditis elegans Q21313.1 protein homologs, the Contig_Gammarus_90_179325_fr3 (serine protease inhibitor 42Dd), the Contig_Gammarus_90_12
305_fr5 (also a serine protease) and the Contig_Gammarus_90_98198_fr6 (Papilin homolog). These four proteins were estimated to be present at low-abundance. This result highlights the difficulties associated with automatic functional prediction based on sequence similarities when studying non-model species which are only distantly related to well-characterized genomes. This is especially true for amphipods because of the lack of publicly-available genomic sequences for these animals and above all, because of a lack of knowledge of the molecular functions of most arthropod gene products despite the diversity and abundance of this ancient lifeform. Recently, a first tentative annotated amphipod RefSeq genome was released based on currently unpublished Hyalella azteca transcriptomics data (GCF_000764305.1). Unfortunately, as this tentative genome comprises 18,000 scaffolds it only affords a partial and dispersed view of Hyalella's background. Despite this novel information, up to 20% of the proteins identified in the reproductive organs from G. fossarum males could not be annotated by GO terms. This lack of knowledge is especially problematic for reproductive proteins which display a trend to be species-specific as a result of sexual selection (Swanson and Vacquier, 2002). Our first proteogenomics survey of G. fossarum had already revealed a larger number of orphan proteins in reproductive organs than in other body parts (Trapp et al., 2014b).
Identification of Molecular Markers Linked to Pyriproxyfen Exposure
To identify molecular markers linked to pyriproxyfen exposure, we first compared the different proteome datasets acquired with the Q Exactive HF instrument. Supplementary Table S2 presents the protein abundance fold-changes and their associated p-value confidence when comparing (i) the control with solvent to the control without solvent, (ii) the pyriproxyfen 0.5 μg/L to the control with solvent, and (iii) the pyriproxyfen 50 μg/L to the control with solvent.
Applying a stringent Tfold parameter of at least two, a significant effect was observed for the solvent which induced increased expression for 28 proteins and decreased expression for 4 proteins. Among the proteins up-regulated, most play a direct (Myosin, Collagen, Papilin, Gelsolin, Plastin) or indirect (Glucosylceramidase by its hydrolase activity) role in maintaining cell structure. A significant number of the proteins with altered expression levels are involved in oxidative stress response (Chromate reductase, Aldose reductase, Catalase, Glutathione S-transferase 1, Hydroxysteroid dehydrogenase-like protein 2). Among the lower-abundance proteins, proteins known to circulate in haemolymph, such as Hemocyanins and Haemolymph clottable proteins, were identified. Altogether, these data demonstrated an effect of the solvent (acetone) on protein profiles in the G. fossarum testis, even at a concentration which is considered very low (0.005% v/v).
To assess the effects of Pyr on protein expression, taking the presence of the solvent into account, the low dose and the high dose groups were compared to the solvent control using the same fold-change threshold. Compared to the solvent control, at the low pyriproxyfen dose (0.5 μg/L) a set of 6 proteins were identified as more abundant, and 26 were less abundant. At the high pyriproxyfen dose (50 μg/L), 6 proteins were also detected in higher amounts, and 15 in lower amounts. A clustering analysis of these results highlights the differences between the two pyriproxyfen doses and the control (Figure 4). Notably, in our experimental conditions pyriproxyfen exposure was associated with a molecular signature characterized by few modifications of the proteomic profile in Gammarus testes. However, among these proteins, five were modulated to the same extent at both doses (Supplementary Table S2). One of these was up-regulated and annotated as haemocyanin, whereas the other four were downregulated, two of which have sequence similarities with as yet uncharacterised Hyallela proteins. Overall, proteins over-expressed in the solvent control tended to be under-expressed in samples treated with pyriproxyfen, and vice versa. For example, Hemocyanin, a protein circulating in haemolymph and responsible for oxygen transport which is also an important immune effector in invertebrates, was detected at lower amounts in the control with solvent, but strongly induced at the two pyriproxyfen doses. This result indicates that pyriproxyfen may induce a stress response at both doses tested. With the treatment at 0.5 μg/L, among the 32 proteins modulated, several metabolic enzymes were found to be induced (Glycogen phosphorylase, Succinate dehydrogenase, L-lactate dehydrogenase). These proteins are closely related in terms of function, i.e., glycogenesis and cellular respiration. Glycogen phosphorylase (Contig_Gammarus_90_ 147423_fr4) was also up-regulated (from 5.3- to 7.5-fold, p-value ≤ 0.05) after treatment with 0.5 μg/L of pyriproxyfen. Like in higher eukaryotes, glycogen has been suggested to be an important fuel reserve in G. fossarum and other crustaceans (Hervant, 1996). Interestingly, in Artemica sinica, another crustacean, glycogen phosphorylase expression was reported to be stress-related (Zhao et al., 2014). Among the decreased proteins, the identification of pyruvate carboxylase hinted at reduced pyruvate cycling. This effect would be expected based on the upregulation of the previously discussed metabolic enzymes. Furthermore, extensive cellular and organ cytoskeleton re-organization could be hypothesized based on the strong decrease in abundance of several proteins involved in these processes (Ubiquitin-like modifier-activating enzyme, Myosin, Ezrin, Zasp-like isoform, Collagen alpha-2 chain, and Basement membrane-specific heparan sulfate proteoglycan core protein) or potentially involved in remodeling (Gelsolin, Carboxypeptidase B, Metallocarboxypeptidase ECM14). Gelsolin is an actin-binding protein which regulates actin filament assembly (Sun et al., 1999), helps actin nucleation before polymerisation, and cleaves actin filaments, resulting in actin mobilization (Laine et al., 1998). Its decreased expression is an indicator of cytoskeleton disturbance, reflecting loss of actin polymerisation (Khaitlina and Hinssen, 1997). Ezrin is also an actin-binding protein involved in cell proliferation, survival, and migration, its regulation depends on its phosphorylation state (Bosk et al., 2011). The high dose of pyriproxyfen caused modulation of the 21 proteins. These proteins had the same proteomic signatures (metabolic effects and cytoskeleton disturbance) as those identified at the low dose, but with a marked effect on mitochondria shown by the significant decrease (8.5-fold, p-value ≤ 0.05) of the Contig_Gammarus_90_111327_fr6 which was annotated as MICOS complex subunit MIC60. This large protein complex associated with the mitochondrial inner membrane plays a crucial role in maintenance of the crista junction, the inner membrane architecture and formation of contact sites with the outer membrane, all of which are required for efficient respiration and ATP generation (Eydt et al., 2017). Mitochondrial dysfunction associated with lower abundance of the Contig_Gammarus_90_204722, annotated as a mitochondrial dihydrolipoyl dehydrogenase, hints at impaired spermatogenesis, as this enzyme is involved in hyperactivation of spermatozoa during capacitation and in the spermatozoal acrosome reaction (Mitra et al., 2005). Among the proteins with high dynamics, a set of uncharacterised proteins (LOC108676008, LOC108679669, LOC108664518, LOC108679669, hypothetical protein MGE_01014) were also identified, mostly at the high pyriproxyfen concentration.
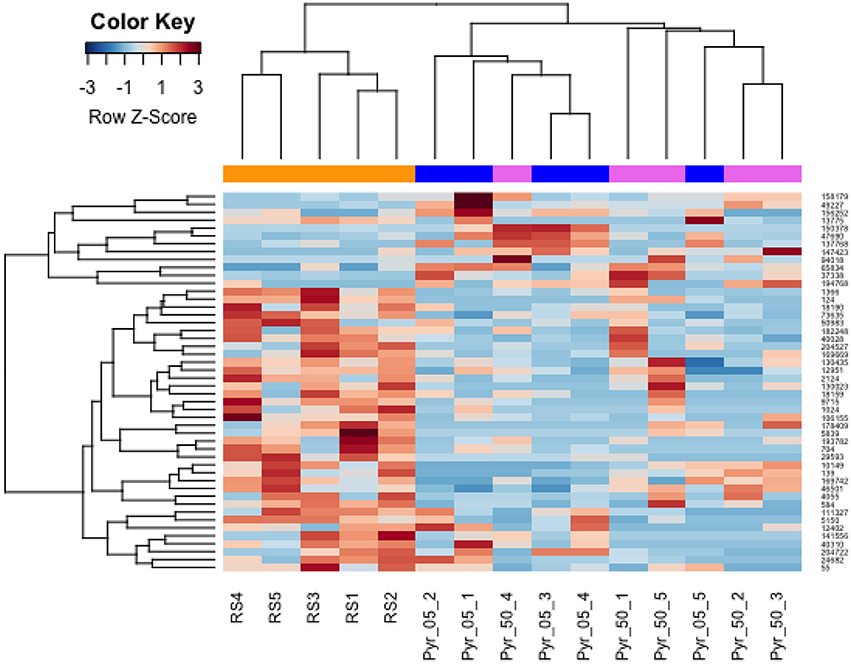
Figure 4. Hierarchical clustering of the 48 proteins modulated in response to pyriproxyfen stress. Orange: controls with solvent; blue: samples treated with 0.5 μg/L pyriproxyfen; pink: samples treated with 50 μg/L pyriproxyfen.
Conclusion
Through this case study documenting the testes proteome of G. fossarum organisms exposed to pyriproxyfen, we compared the performance of two different generations of tandem mass spectrometers based on the Orbitrap analyser technology: the LTQ Orbitrap XL and the Q Exactive HF. Due to its faster scan rate, quadrupole filtering properties, and higher resolution on MS/MS secondary ions, the Q Exactive HF instrument recorded almost 5 times more MS/MS spectra from the same G. fossarum proteome samples. In addition, and more importantly, it also increased the intrinsic quality of these spectra. Data acquired on the Q Exactive HF machine allowed the identification of a 4-fold more peptides and proteins than data from the LTQ Orbitrap XL instrument. Protein coverage was also enhanced, with a slight increase in the average number of peptides recorded for each protein, thus sequence coverage was improved even for less abundant proteins. The quality of data in terms of quantification was also improved thanks to the higher spectral counts available for each protein. Importantly, the list of potential biomarkers reported based on LTQ Orbitrap XL data (Trapp et al., 2015) was further extended with the Q Exactive HF data. Here, we report some metabolic proteins as potential protein markers of pyriproxyfen treatment. These results confirm the trend for perturbed cellular homeostasis triggered by pyriproxyfen stress. We recently reported that pyriproxyfen exposure was linked to a reduced sperm count, and this toxic effect correlated with modulation of a limited number of testes-specific proteins following exposure (Trapp et al., 2015). This observation suggests that a sub-population of testicular cells, such as presursors of mature spermatozoa, could be the main target of pyriproxyfen exposure. Within a proteogenomics framework for non-model animals used in ecotoxicology, we recommend the use of tandem mass spectrometers with performances such as those of the Q Exactive HF instrument as they provide a more comprehensive view of the proteome dynamics following stress, and thus allow a greater depth of analysis when seeking to determine the effects of a given xenobiotic. Our experimental data speak in favor of the use of the Q Exactive HF instruments to improve proteome coverage for any biological sample, even though the previous generation of tandem mass spectrometers also provided sufficient information to obtain preliminary insights into stress response. Large numbers of biological replicates and technical replicates are usually recommended when assessing biological mechanisms or validating biomarkers as part of routine ecotoxicological risk assessment (Trapp et al., 2014a). In addition, next-generation instruments based on the ultra-high-field Orbitrap analyser significantly outperform previous generations, allowing achieving comparative proteomics studies with numerous biological and technical replicates, and conditions to be completed more quickly. However, despite these advances in mass spectrometry technology, the high dynamic range of the proteome of complex organisms continues to hinder the analysis of very low-abundance proteins. These proteins may be permanently or transiently present in the proteome, and generally comprise proteins with a high diagnostic potential, normally associated with transcription and translation mechanisms, membrane receptors, allergens, polypeptides, and post-translationally modified proteins. Their detection by mass spectrometry remains a key technical challenge in shotgun proteomics and biomarker discovery. Nevertheless, as demonstrated in this study, the continuous progresses in mass spectrometry technology should lead to the complete proteome age within a few years.
The ecotoxicology results presented here show that proteins involved in cytoskeleton organization are perturbed in arthropods tested following exposure to pesticides. The activity of several of these proteins is controlled by post-translational modifications. Examining the phosphorylation pattern of these cytoskeletal proteins by targeted proteomics approaches could help to increase our understanding of how pyriproxyfen works and better disentangle the differences between solvent and pyriproxyfen effects. Among the proteins for which abundance was dramatically increased by pyriproxyfen, the Contig_Gammarus_90_150378 - annotated as a glycogen phosphorylase - could be a promising biomarker of pyriproxyfen-induced stress. Moreover, the increase in protein expression was dose-dependent. The Contig_Gammarus_90_204722, identified as mitochondrial dihydrolipoyl dehydrogenase, would also be interesting to monitor as it is modulated in response to pyriproxyfen and is also involved in spermatogenesis.
Author Contributions
JT, DG, CA, AC, OG, and JA designed the study. JT, DG, CA, DD, and JA analyzed the data, with input from OP, AC, OG. JT, DG, CA, and J-CG performed protein extraction and tandem mass spectrometry. JT, DG, CA, and JA drafted the manuscript. All authors approved the final version of the manuscript. JT and DG contributed equally and should be considered both as first co-author.
Conflict of Interest Statement
The authors declare that the research was conducted in the absence of any commercial or financial relationships that could be construed as a potential conflict of interest.
Acknowledgments
We thank the Institut National de Recherche en Sciences et Technologies pour l'Environnement et l'Agriculture (France), the Commissariat à l'Energie Atomique et aux Energies Alternatives (France) through the transversal toxicology program (PPTOX), and the Agence Nationale de la Recherche program ProteoGam (ANR-14-CE21-0006-02) for financial support. We are also grateful to Laurent Guillet-Revol, Khedidja Abbaci, Hervé Quéau, and Renaud Tutundjian for technical assistance during exposure experiments.
Supplementary Material
The Supplementary Material for this article can be found online at: https://www.frontiersin.org/articles/10.3389/fenvs.2018.00054/full#supplementary-material
References
Armengaud, J. (2016). Next-generation proteomics faces new challenges in environmental biotechnology. Curr. Opin. Biotechnol. 38, 174–182. doi: 10.1016/j.copbio.2016.02.025
Armengaud, J., Trapp, J., Pible, O., Geffard, O., Chaumot, A., and Hartmann, E. M. (2014). Non-model organisms, a species endangered by proteogenomics. J. Proteomics 105, 5–18. doi: 10.1016/j.jprot.2014.01.007
Bosk, S., Braunger, J. A., Gerke, V., and Steinem, C. (2011). Activation of F-actin binding capacity of ezrin: synergism of PIP(2) interaction and phosphorylation. Biophys. J. 100, 1708–1717. doi: 10.1016/j.bpj.2011.02.039
Buchfink, B., Xie, C., and Huson, D. H. (2015). Fast and sensitive protein alignment using DIAMOND. Nat. Methods 12, 59–60. doi: 10.1038/nmeth.3176
Campos, A., Danielsson, G., Farinha, A. P., Kuruvilla, J., Warholm, P., and Cristobal, S. (2016). Shotgun proteomics to unravel marine mussel (Mytilus edulis) response to long-term exposure to low salinity and propranolol in a Baltic Sea microcosm. J. Proteomics 137, 97–106. doi: 10.1016/j.jprot.2016.01.010
Carvalho, P. C., Lima, D. B., Leprevost, F. V., Santos, M. D., Fischer, J. S., Aquino, P. F., et al. (2016). Integrated analysis of shotgun proteomic data with PatternLab for proteomics 4.0. Nat. Protoc. 11, 102–117. doi: 10.1038/nprot.2015.133
Charnot, A., Gouveia, D., Armengaud, J., Almunia, C., Chaumot, A., Lemoine, J., et al. (2017). Multiplexed assay for protein quantitation in the invertebrate Gammarus fossarum by liquid chromatography coupled to tandem mass spectrometry. Anal. Bioanal. Chem. 409, 3969–3991. doi: 10.1007/s00216-017-0348-0
Colbourne, J. K., Pfrender, M. E., Gilbert, D., Thomas, W. K., Tucker, A., Oakley, T. H., et al. (2011). The ecoresponsive genome of Daphnia pulex. Science 331, 555–561. doi: 10.1126/science.1197761
Culhane, A. C., Thoulouse, J., Perrière, G., and Higgins, D. G. (2005). MADE4: an R package for multivariate analysis of gene expression data. Bioinformatics 21, 2789–2790. doi: 10.1093/bioinformatics/bti394
Eydt, K., Davies, K. M., Behrendt, C., Wittig, I., and Reichert, A. S. (2017). Cristae architecture is determined by an interplay of the MICOS complex and the F1FO ATP synthase via Mic27 and Mic10. Microb. Cell 4, 259–272. doi: 10.15698/mic2017.08.585
Garcia-Reyero, N., and Perkins, E. J. (2011). Systems biology: leading the revolution in ecotoxicology. Environ. Toxicol. Chem. 30, 265–273. doi: 10.1002/etc.401
Gillette, M. A., and Carr, S. A. (2013). Quantitative analysis of peptides and proteins in biomedicine by targeted mass spectrometry. Nat. Methods 10, 28–34. doi: 10.1038/nmeth.2309
Ginjupalli, G. K., and Baldwin, W. S. (2013). The time- and age-dependent effects of the juvenile hormone analog pesticide, pyriproxyfen on Daphnia magna reproduction. Chemosphere 92, 1260–1266. doi: 10.1016/j.chemosphere.2013.04.061
Gouveia, D., Chaumot, A., Charnot, A., Almunia, C., François, A., Navarro, L., et al. (2017a). Ecotoxico-proteomics for aquatic environmental monitoring: first in situ application of a new proteomics-based multibiomarker assay using caged amphipods. Environ. Sci. Technol. 51, 13417–13426. doi: 10.1021/acs.est.7b03736
Gouveia, D., Chaumot, A., Charnot, A., Queau, H., Armengaud, J., Almunia, C., et al. (2017b). Assessing the relevance of a multiplexed methodology for proteomic biomarker measurement in the invertebrate species Gammarus fossarum: A physiological and ecotoxicological study. Aquat. Toxicol. 190, 199–209. doi: 10.1016/j.aquatox.2017.07.007
Hartmann, E. M., Allain, F., Gaillard, J. C., Pible, O., and Armengaud, J. (2014). Taking the shortcut for high-throughput shotgun proteomic analysis of bacteria. Methods Mol. Biol. 1197, 275–285. doi: 10.1007/978-1-4939-1261-2_16
Hartmann, E. M., and Armengaud, J. (2014). Shotgun proteomics suggests involvement of additional enzymes in dioxin degradation by Sphingomonas wittichii RW1. Environ. Microbiol. 16, 162–176. doi: 10.1111/1462-2920.12264
Hervant, F. (1996). The activities of enzymes associated with the intermediary and energy metabolism in hypogean and epigean crustaceans. C. R. Acad. Sci. III 319, 1071–1077.
Ilyas, S., Rehman, A., Coelho, A. V., and Sheehan, D. (2016). Proteomic analysis of an environmental isolate of Rhodotorula mucilaginosa after arsenic and cadmium challenge: identification of a protein expression signature for heavy metal exposure. J. Proteomics 141, 47–56. doi: 10.1016/j.jprot.2016.04.012
Khaitlina, S., and Hinssen, H. (1997). Conformational changes in actin induced by its interaction with gelsolin. Biophys. J. 73, 929–937. doi: 10.1016/S0006-3495(97)78125-6
Klein, G., Mathé, C., Biola-Clier, M., Devineau, S., Drouineau, E., Hatem, E., et al. (2016). RNA-binding proteins are a major target of silica nanoparticles in cell extracts. Nanotoxicology 10, 1555–1564. doi: 10.1080/17435390.2016.1244299
Laine, R. O., Phaneuf, K. L., Cunningham, C. C., Kwiatkowski, D., Azuma, T., and Southwick, F. S. (1998). Gelsolin, a protein that caps the barbed ends and severs actin filaments, enhances the actin-based motility of Listeria monocytogenes in host cells. Infect. Immun. 66, 3775–3782.
LeBlanc, G. A. (2007). Crustacean endocrine toxicology: a review. Ecotoxicology 16, 61–81. doi: 10.1007/s10646-006-0115-z
Michalski, A., Damoc, E., Hauschild, J. P., Lange, O., Wieghaus, A., Makarov, A., et al. (2011). Mass spectrometry-based proteomics using Q Exactive, a high-performance benchtop quadrupole Orbitrap mass spectrometer. Mol. Cell. Proteomics 10:M111 011015. doi: 10.1074/mcp.M111.011015
Mitra, K., Rangaraj, N., and Shivaji, S. (2005). Novelty of the pyruvate metabolic enzyme dihydrolipoamide dehydrogenase in spermatozoa: correlation of its localization, tyrosine phosphorylation, and activity during sperm capacitation. J. Biol. Chem. 280, 25743–25753. doi: 10.1074/jbc.M500310200
Monsinjon, T., and Knigge, T. (2007). Proteomic applications in ecotoxicology. Proteomics 7, 2997–3009. doi: 10.1002/pmic.200700101
Nesvizhskii, A. I. (2014). Proteogenomics: concepts, applications and computational strategies. Nat. Methods 11, 1114–1125. doi: 10.1038/nmeth.3144
Olmstead, A. W., and LeBlanc, G. A. (2003). Insecticidal juvenile hormone analogs stimulate the production of male offspring in the crustacean Daphnia magna. Environ. Health Perspect. 111, 919–924. doi: 10.1289/ehp.5982
Pereira-Medrano, A. G., Sterling, A., Snijders, A. P., Reardon, K. F., and Wright, P. C. (2007). A systematic evaluation of chip-based nanoelectrospray parameters for rapid identification of proteins from a complex mixture. J. Am. Soc. Mass Spectrom. 18, 1714–1725. doi: 10.1016/j.jasms.2007.06.011
Petrak, J., Ivanek, R., Toman, O., Cmejla, R., Cmejlova, J., Vyoral, D., et al. (2008). Deja vu in proteomics. A hit parade of repeatedly identified differentially expressed proteins. Proteomics 8, 1744–1749. doi: 10.1002/pmic.200700919
Rajapakse, K., Drobne, D., Kastelec, D., Kogej, K., Makovec, D., Gallampois, C., et al. (2016). Proteomic analyses of early response of unicellular eukaryotic microorganism Tetrahymena thermophila exposed to TiO2 particles. Nanotoxicology 10, 542–556. doi: 10.3109/17435390.2015.1091107
Scheltema, R. A., Hauschild, J. P., Lange, O., Hornburg, D., Denisov, E., Damoc, E., et al. (2014). The Q Exactive HF, a Benchtop mass spectrometer with a pre-filter, high-performance quadrupole and an ultra-high-field Orbitrap analyzer. Mol. Cell. Proteomics 13, 3698–3708. doi: 10.1074/mcp.M114.043489
Sellami, B., Khazri, A., Louati, H., Dellali, M., Driss, M. R., Aïssa, P., et al. (2015). Effects of anthracene on filtration rates, antioxidant defense system, and redox proteomics in the Mediterranean clam Ruditapes decussatus (Mollusca: Bivalvia). Environ. Sci. Pollut. Res. Int. 22, 10956–10968. doi: 10.1007/s11356-015-4328-7
Sullivan, J. J., and Goh, K. S. (2008). Environmental fate and properties of pyriproxyfen. J. Pest. Sci. 33, 339–350. doi: 10.1584/jpestics.R08-02
Sun, H. Q., Yamamoto, M., Mejillano, M., and Yin, H. L. (1999). Gelsolin, a multifunctional actin regulatory protein. J. Biol. Chem. 274, 33179–33182. doi: 10.1074/jbc.274.47.33179
Swanson, W. J., and Vacquier, V. D. (2002). The rapid evolution of reproductive proteins. Nat. Rev. Genet. 3, 137–144. doi: 10.1038/nrg733
Trapp, J., Almunia, C., Gaillard, J. C., Pible, O., Chaumot, A., Geffard, O., et al. (2016). Proteogenomic insights into the core-proteome of female reproductive tissues from crustacean amphipods. J. Proteomics 135, 51–61. doi: 10.1016/j.jprot.2015.06.017
Trapp, J., Armengaud, J., Pible, O., Gaillard, J. C., Abbaci, K., Habtoul, Y., et al. (2015). Proteomic investigation of male Gammarus fossarum, a freshwater crustacean, in response to endocrine disruptors. J. Proteome Res. 14, 292–303. doi: 10.1021/pr500984z
Trapp, J., Armengaud, J., Salvador, A., Chaumot, A., and Geffard, O. (2014a). Next-generation proteomics: toward customized biomarkers for environmental biomonitoring. Environ. Sci. Technol. 48, 13560–13572. doi: 10.1021/es501673s
Trapp, J., Geffard, O., Imbert, G., Gaillard, J. C., Davin, A. H., Chaumot, A., et al. (2014b). Proteogenomics of Gammarus fossarum to document the reproductive system of amphipods. Mol. Cell. Proteomics 13, 3612–3625. doi: 10.1074/mcp.M114.038851
Vizcaíno, J. A., Côté, R. G., Csordas, A., Dianes, J. A., Fabregat, A., Foster, J. M., et al. (2013). The PRoteomics IDEntifications (PRIDE) database and associated tools: status in 2013. Nucleic Acids Res. 41, D1063–D1069. doi: 10.1093/nar/gks1262
Wei, W., Luo, W., Wu, F., Peng, X., Zhang, Y., Zhang, M., et al. (2016). Deep coverage proteomics identifies more low-abundance missing proteins in human testis tissue with Q-Exactive HF mass spectrometer. J. Proteome Res. 15, 3988–3997. doi: 10.1021/acs.jproteome.6b00390
Keywords: proteomics, high-resolution tandem mass spectrometer, endocrine disruptor, environmental toxicoproteomics, insecticide, Gammarus fossarum
Citation: Trapp J, Gouveia D, Almunia C, Pible O, Degli Esposti D, Gaillard J-C, Chaumot A, Geffard O and Armengaud J (2018) Digging Deeper Into the Pyriproxyfen-Response of the Amphipod Gammarus fossarum With a Next-Generation Ultra-High-Field Orbitrap Analyser: New Perspectives for Environmental Toxicoproteomics. Front. Environ. Sci. 6:54. doi: 10.3389/fenvs.2018.00054
Received: 06 March 2018; Accepted: 25 May 2018;
Published: 13 June 2018.
Edited by:
Harminder Pal Singh, Panjab University, IndiaReviewed by:
Naresh Singhal, University of Auckland, New ZealandSonia Garcia Alcega, Cranfield University, United Kingdom
Copyright © 2018 Trapp, Gouveia, Almunia, Pible, Degli Esposti, Gaillard, Chaumot, Geffard and Armengaud. This is an open-access article distributed under the terms of the Creative Commons Attribution License (CC BY). The use, distribution or reproduction in other forums is permitted, provided the original author(s) and the copyright owner are credited and that the original publication in this journal is cited, in accordance with accepted academic practice. No use, distribution or reproduction is permitted which does not comply with these terms.
*Correspondence: Jean Armengaud, jean.armengaud@cea.fr
†These authors have contributed equally to this work and are co first-author.