- 1Educational Excellence Team, University of Nottingham, Nottingham, United Kingdom
- 2School of Psychology, University of Nottingham, Nottingham, United Kingdom
- 3School of Psychology and N/LAB, University of Nottingham, Nottingham, United Kingdom
- 4School of Mathematical Sciences, University of Nottingham, Nottingham, United Kingdom
Analytic and holistic marking are typically researched as opposites, generating a mixed and inconclusive evidence base. Holistic marking is low on content validity but efficient. Analytic approaches are praised for transparency and detailed feedback. Capturing complex criteria interactions, when deciding marks, is claimed to be better suited to holistic approaches whilst analytic rules are thought to be limited. Both guidance and evidence in this area remain limited to date. Drawing from the known complementary strengths of these approaches, a university department enhanced its customary holistic marking practices by introducing analytic rubrics for feedback and as ancillary during marking. The customary holistic approach to deciding marks was retained in the absence of a clear rationale from the literature. Exploring the relationship between the analytic criteria and holistic marks became the focus of an exploratory study during a trial year that would use two perspectives. Following guidance from the literature, practitioners formulated analytic rules drawing on their understanding of the role of criteria, to explain output marks by allocating weightings. Secondly, data derived throughout the year consisting of holistic marks and analytic judgements (criteria) data were analyzed using machine learning techniques (random forests). This study reports on data from essay-based questions (exams) for years 2 and 3 of study (n = 3,436). Random forests provided a ranking of the variable importance of criteria relative to holistic marks, which was used to create criterion weightings (data-derived). Moreover, illustrative decision trees provide insights into non-linear roles of criteria for different levels of achievement. Criterion weightings, expected by practitioners and data-derived (from holistic marks), reveal contrasts in the ranking of top criteria within and across years. Our exploratory study confirms that holistic and analytic approaches, combined, offer promising and productive ways forward both in research and practice to gain insight into the nature of overall marks and relations with criteria. Rather than opposites, these approaches offer complementary insights to help substantiate claims made in favor of holistic marking. Our findings show that analytic may offer insights into the extent to which holistic marking really aligns with assumptions made. Limitations and further investigations are discussed.
Introduction: Divergent Assessments, Marking, and Rubrics
Most assessment methods used in higher education elicit student-constructed responses to an open question (e.g., essay, reports, projects, presentations). These are divergent assessments in that a broad range of student individual responses can meet the desired criteria and outcomes. The implementation of divergent assessments presents challenges in marking and also ensuring students understand expectations (Brown et al., 1997; Biggs and Tang, 2011).
One of the most salient challenges is marking, attracting much public attention and debate. Concerns range from consistency across markers within and across institutions (Bloxham et al., 2011, 2016a), grade inflation and even the very nature of marks (Elton, 1998; Elton and Johnston, 2002; Yorke, 2011; Boud, 2018). External examination and moderation have also been questioned (Hay and Macdonald, 2008; Bloxham and Price, 2013; Bloxham et al., 2016b).
In particular the UK university sector has been urged to enhance transparency (Woolf, 2004; Bloxham et al., 2016a). Transforming assessment practices requires systemic and cultural changes (Macdonald and Joughin, 2009; Yorke, 2011) which can only be gradual and require clear rationales and guidance to overcome cultural barriers. Limitations to the existing evidence base and the intrinsic challenges of transforming marking cultures, coalesce to hinder the resolution in practice of the complex challenge of increasing transparency. Public scrutiny, practitioners and research grapple with the intricacies of achieving transparency for a complex set of stakeholders. At the basis of all these concerns is marking and decisions about rubric design and uses by practitioners. This fundamental part of the complex challenge of transparency is the focus of the present study. Theory, evidence and practice perspectives are explored in shaping the aims of the study and its contributions.
Reviews on rubric research converge on key benefits of rubric use. Firstly, rubrics are powerful allies for instruction and learning (Andrade, 2005; Andrade and Du, 2005; Jönsson and Svingby, 2007; Reddy and Andrade, 2010; Panadero and Jönsson, 2013; Panadero and Romero, 2014; Brookhart and Chen, 2015; Suskie, 2017; Brookhart, 2018; Panadero and Broadbent, 2018; Jönsson and Prins, 2019). Secondly, rubric use for marking shows positive impacts on enhancing reliability and validity. However, research on validity has mainly been limited to considering linguistic features (Reddy and Andrade, 2010) and mostly investigated from the angle of user views (Brookhart, 2018). Most reviews conclude on the limited understanding of the relationship between validity and rubric use (Jönsson and Svingby, 2007; Reddy and Andrade, 2010). These reviews highlight the limits of the existing evidence base. The design and use of holistic and analytic rubrics for marking and student engagement is less conclusive (Jönsson and Svingby, 2007; Reddy and Andrade, 2010; Brookhart, 2018). Advancing our understanding of the role that analytic and holistic approaches to rubric design and uses is highly necessary.
The Continuum of Analytic and Holistic Rubric Design and Uses: Research and Principles
Literature reviews indicate inconsistent uses of the labels holistic and analytic and they are better conceptualized as a continuum (Hunter et al., 1996; Harsch and Martin, 2013). A spectrum of options range from impressionistic scoring (holistic with no reference to any standards) through to analytic scoring using specific rules to individual ratings of criteria and a composite score being derived and even to atomistic scoring (e.g., focus on in narrow features such as counting number of errors) (Hunter et al., 1996). Our study focuses on holistic and analytic marking, since impressionistic scoring is largely discredited and atomistic scoring rarely applies to divergent assessments.
Choosing between features of holistic and analytic marking presents challenges in practice. Holistic marking consists of forming overall judgements on student work where criteria are considered simultaneously. Links to standards may be achieved by virtue of reference to written descriptors (rubrics) and exemplars (Sadler, 2009b, 2014). Analytic rubrics display pre-set criteria and defined levels of performance typically in a matrix. In analytic marking, judgements on individual criteria provide a basis for deriving marks using explicit rules.
Use of holistic and analytic approaches, in particular when marks are required, polarizes opinion both in the literature and practice. Dominant discourses and research perspectives have emphasized their seemingly opposite natures. Below inconsistencies between evidence and some arguments used in the advocacy of holistic approaches are explored to highlight the less explored combined potential of these approaches. In order to advance existing understanding, it will be argued, new perspectives on the matter are required.
The existing body of work has mainly focused on comparative approaches in research so far generating an inconclusive evidence base and no clear rationale for the use of either approach (Jönsson and Svingby, 2007; Sadler, 2009a, 2014; Reddy and Andrade, 2010; Brookhart, 2018). Table 1 below presents a summary of several reference sources and research on the multiple arguments that exist in favor and against both approaches. Table 1 is adapted with permission on the work by Brookhart and Nitko (2019, p. 280) but has been expanded to include additional convergent findings/arguments from other research and reference materials (Sadler, 1987, 2009a; Perlman, 2002; Kuo, 2007; Hay and Macdonald, 2008; Harsch and Martin, 2013; Jones and Alcock, 2014). Descriptive commentaries and theoretical discussions providing arguments in support of either approach are summarized (Sadler, 1987, 2009a; Kuo, 2007; Suskie, 2014, 2017). Research reviews and their convergent conclusions are also reflected (Jönsson and Svingby, 2007; Reddy and Andrade, 2010; Brookhart and Chen, 2015; Brookhart, 2018). Lastly, empirical studies on rubrics and marking are also summarized (Perlman, 2002; Hay and Macdonald, 2008; Harsch and Martin, 2013; Jones and Alcock, 2014; Björklund et al., 2015). The summary list of mixed advantages and disadvantages across the literature is convergent, also echoing discussions in practice.
In essence, holistic marking offers greater reliability (cross-marker agreement) (Jones and Alcock, 2014; Björklund et al., 2015), but this effect has also been attributed to analytic marking (Jönsson and Svingby, 2007). Fewer studies investigate intra-rater consistency (consistency with self) according to Jönsson and Svingby (2007). Generally, intra-rater consistency is aided by the use of a rubric during marking (Jönsson and Svingby, 2007; Harsch and Martin, 2013) and a few studies report this can be enhanced with analytic rubrics (Kuo, 2007; Harsch and Martin, 2013). Regarding the learning aspect, guides on good practice argue for the use of analytic rubrics with students on the grounds of the detail being beneficial (Perlman, 2002); however, no studies have substantiated this aspect. Whilst analytic rubrics offer more feedback, they are reportedly less efficient for markers than holistic marking.
The most recent review on rubric related research (Brookhart, 2018) concludes, echoing previous reviews (Jönsson and Svingby, 2007; Reddy and Andrade, 2010), that neither holistic nor analytic rubrics can be deemed better on account of enhancing reliability, validity or their impact on learning. Much of the evidence relies on descriptive accounts or analyses (Sadler, 1987, 2009a; Kuo, 2007). Evidence from empirical studies is not only scarce but also contradictory in key areas such as inter and intra-marker agreement with different studies reporting opposite findings. Research on the impact of these approaches on students and learning is scarce.
The remainder of our review considers claims made in favor of either approach that remain unsubstantiated, in particular, in relation to content and structural validity. Advocacy in favor of holistic scoring and its underlying fuzzy logic, is mostly based on arguments against the systematic and linear nature of analytic approaches. The main challenges against analytic approaches are that not every aspect of a performance can be described and that linear formulae cannot capture the complex ways in which criteria interact (Sadler, 2009a, 2014). Yet, whilst the limitations inherent to analytic approaches have been extensively discussed, an evidence based rationale for the use of holistic approaches is also absent.
Content validity (Messick, 1994, 1996) relates to ensuring that an assessment, indeed, assesses what it intends to assess. Holistic marking is repeatedly associated with the masking of different uses of criteria (Huot, 1990; Hay and Macdonald, 2008; Suto and Nadas, 2008; Harsch and Martin, 2013; Bloxham et al., 2016a). This finding is recurrent in the literature and suggests that content validity with holistic marking is low. Despite the evidence of threats to content validity, associated with holistic marking, proponents of this approach argue that its intuitive nature favors validity (Sadler, 1987, 2009a) which remains largely unsubstantiated.
The second main concern raised in the literature on analytic approaches concerns structural validity which considers the relationship between outcomes (marks) and these being interpretable according to more important criteria and learning outcomes (Messick, 1994, 1996). Claims on the purported intrinsic structural validity of holistic marking rest on theoretical descriptions (Sadler, 1987, 2009a) and remain unsubstantiated to date. With the use of analytic approaches practitioners decide weightings for criteria based on their understanding of instructional goals and performance in a task. This approach also has advocates on the grounds of transparency and communication (Suskie, 2014, 2017). Yet, the nature of rules and approaches in deciding them is an area where little investigation is to be found. Allocating weightings to criteria, a commonly recommended approach, promotes compensation and may fail to represent complex interactions amongst criteria and levels (e.g., threshold levels and criteria) cannot be captured in linear ways (Sadler, 1987, 2009a). In practice it would be really hard for practitioners to hypothesize over non-linear relations and therefore, broad ranking of criteria and allocation of weightings is commonly used.
It is on this particular area where, beyond these claims and very generic guidance to practitioners, there is little understanding for example on rules that underlie holistic marking and the complexity of criteria interactions. Equally, understanding how these stand in comparison to the analytic, expert derived weightings (or rules), would really advance our understanding of the limitations and advantages of both approaches. A very scarce evidence base with studies using mathematical modeling (Principal Component Analysis, PCA) on tutors' ranking of theses and their reasons, in a holistic manner, suggest the use of idiosyncratic weightings applied to criteria (Björklund et al., 2015). Whilst a small-scale study, this study illustrates how holistic judgement may be represented in analytic terms potentially helping to inform analytic rules. This exploratory study warrants further exploration of the holistic approach and indeed, how it relates to outputs may be explored with non-linear methods of analysis. Whether linear formulas are the right approach or not, can only be answered by examining holistic judgement and modeling it so we can better understand significance of criteria implicit in the judgements and relationships amongst criteria.
Moreover, structural validity also considers communication and maintaining standards, that is, staff and students should also share understanding of expectations of quality and criteria (Messick, 1996; Dochy, 2009). Again the literature presents many claims to date without a clear rationale. Holistic approaches are advocated on the basis that using rubrics (verbal descriptors) and exemplars and sharing this with staff and students are effective mechanisms to address this important aspect of validity (Sadler, 1987, 2009b). Perceived perils of greater detail in analytic criteria potentially leading to mechanistic learning and possibly with counterproductive effects have also been used to argue against analytic approaches (Torrance, 2007). Promoting students' understanding of what is important in assessment, including varying degrees of quality, can happen in discussions with markers and by using rubrics and exemplars (ibid.). These advocacy claims remain unsubstantiated with some studies also indicating the need for more transparent links where holistic approaches to marking exist (Grainger et al., 2008).
Lastly, the complementary strengths and natures of analytic and holistic approaches are less well-understood and have been suggested as a productive avenue of research (Hunter et al., 1996; Harsch and Martin, 2013). Harsch and Martin (2013) offer suggestions for the combination of both analytic and holistic scoring strategies and use of quantitative and qualitative methods in the design of rubrics, descriptors and during training. For example, in the context of large-scale evaluation of writing, a conclusion reached was that holistic decisions may be retained (face validity, cost-effectiveness) but analytic rating may be a complement for feedback and reducing error (Hunter et al., 1996).
In sum, both analytic and holistic approaches in marking offer strengths. The review highlights areas where the role of analytic and holistic approaches in particular in relation to structural validity rests on advocacy and research is needed. We draw particular attention to the largely unexplored area of the nature of underlying rules, of both holistic and analytic approaches to marking that need to be better understood. Much advocacy for holistic approaches rests on shortcomings of analytic approaches but no evidence of their strengths. The review establishes the need to focus on understanding better the underlying nature of the rules of these approaches. Holistic and analytic marking, treated as opposites, in research and the literature leave many unresolved questions in practice. Emphasizing their combined strengths has been less well-explored and is suggested as a way forward. A practice perspective on these literature discussions is provided below.
Analytic and Holistic Rubric Design and Uses: Applying Evidence in Practice
A university department in the United Kingdom (UK) allows the exploration of highlighted areas where research is limited and ways in which these cause tensions in practice. The literature review above provided a basis for the formulation of a project to gradually transform existing customary holistic marking practices. Rubric design and uses (marking, feedback) would be the starting point. Developing students' evaluative competence (Sadler, 2009b; Boud et al., 2018) was also in scope. Implementing department-wide change in real settings requires gradual approaches and has placed constraints on the pace and number of changes that can be introduced at a given time. The case illustrates how unresolved questions in the literature are addressed in practice.
The transition toward greater transparency considered the inclusion of elements from analytic approaches, on the basis of the review above, but holistic elements were retained in the absence of clear rationales. The resulting model of practice combines elements from both analytic and holistic approaches where a clear rationale existed.
• Rubric design and display of criteria and descriptors: holistic (statements of overall quality lumped together) or analytic (matrix style display with criteria and levels). Our study introduced the analytic display for the known greater detail and better feedback replacing customary practice which had consisted of holistic rubrics.
• During marking reference and use of criteria. Markers would be required to indicate, during marking, levels of performance against criteria using the newly introduced analytic rubrics, which is known, as discussed above, to reduce the use of irrelevant criteria. In the previous practice, it was unknown whether markers referred to existing holistic rubrics.
• Deciding marks: Holistic marking refers to approaches where the mark is decided by simultaneously considering all criteria. Analytic marking relies on combination rules to derive marks following judgements on individual criteria. Rules for analytic marking are derived from experts' understanding of significant criteria and expressed typically as weightings or threshold levels. As discussed in the literature, the absence of a clear rationale led to the customary holistic decisions on marks remaining unchanged.
• Feedback stage: this element considers including rubric use for marking with marker indications of level of performance per criterion. Analytic rubrics are known for offering greater detail and better feedback and, after completion during marking, they would be included in the feedback to students and this was also a new element in practice.
A stepped approach to the cultural transformation to marking and rubric use in practice was adopted both considering the literature and nature of changing marking practice. Table 2 below sums up the traditional practice across the department and the modified aspects during the trial year.
Aims of the Study
Rubrics are recognized to have a positive impact in instruction and learning. The review has drawn attention to questions on holistic and analytic approaches to marking that, both in the literature and practice, polarize opinion with little conclusive guidance or rationale as is repeatedly echoed in reviews of the field (Jönsson and Prins, 2019). A real case illustrates a situation in practice and is a product of the limited evidence and guidance available to practitioners in addition to the difficulty of changing marking culture. Our case is situated in the UK university sector where holistic practices are customary and enhancing transparency a reported challenge. With a view to enhancing transparency, strengths of both analytic and holistic approaches are combined in our case.
The case provides an opportunity to examine claims in the literature favoring holistic approaches for their natural capacity to capture complex judgements in contrast with analytic approaches (e.g., too linear, compensatory and relying on expert understanding). Advancing understanding in the literature and practice on this contentious aspect is the focus of our study. We aim to explore how holistic marking and its outputs align with assumptions made about the uses of criteria and their relative importance. The objective is to inform decisions in practice on this matter whilst providing exploratory insights to advance our understanding of how output marks relate to significant criteria and the capacity of analytic and holistic approaches to represent that. The research questions are:
1. What are the expectations by practitioners of the importance and contribution of different criteria in marking?
2. What is the contribution of criteria associated with holistic marks?
3. How do practitioner expected and data derived weightings in holistic marking relate?
A Case Study: Data Collection in the Process of Design, Marking and Review
The study is part of a university department-wide (science subject) enhancement initiative to modify its marking practices in relation to rubric design and uses. The overall aim was to strengthen the congruence between rubrics, marking, and feedback with the intended instructional learning outcomes of the undergraduate programs of study. Understanding the importance of criteria in relation to holistic marks was the focus of investigation during a trial year.
The overall framework chosen is a case study (Yin, 2002) given the close blurred boundaries between the context and the phenomenon we wanted to investigate. Also, case studies provide a flexible framework in particular for real life phenomena where multiple approaches can be integrated (Yin, 2002). The case study, whilst mainly framed within qualitative approaches of enquiry allows for a variety of information sources and types (e.g., quantitative and qualitative) throughout the process.
Exploration of holistic marks in context and in relation to analytic criteria was approached in a multifaceted manner. Design, marking and rubric review stages in the course of the transformation project and trial year offered opportunities to record expectations of staff on the role of criteria and marking data to offer ways of exploring how overall marks (holistic) related to analytic criteria.
Rubric Design: Elicitation of Criteria, Descriptors, and Expectations of Criteria Weightings
The department consists of ~700 undergraduate students and 55 academic staff. A department team of three experienced lecturers from the same science discipline in the department and an assessment adviser was set up to work collaboratively on the design of analytic rubrics and lead the implementation of changes in the trial year. The assessment adviser works across the university with all faculty on projects to enhance practice. University-wide objectives inform the work but departments lead on the implementation (e.g., pace of change, scale) given the known sensitivities of cultural transformation. In the initial stages a professor, formerly head of the department, also joined to steer and approve the direction of the work. The steps followed are informed by guidance literature (Boston, 2002; Stevens and Levi, 2005; Dawson, 2015; Suskie, 2017) adapted to the circumstances of the project and, as described, the sensitivities of cultural transformation across the department.
Criteria and Level Descriptors
Five experienced markers from the same department were invited to individual interviews with the assessment adviser on how they made holistic decisions during marking. The markers were invited to bring a sample of student written coursework covering a range of qualities (poor, good, and excellent) in their view. This would enable contrasts and elicitation of criteria providing a basis for their articulation of aspects that are deemed more important, of a higher level, in the eyes of markers when deciding marks, which is the custom. Semi-structured interviews were planned to elicit key criteria considered in different levels of quality:
• From the best example of work, through to the lower quality, markers were prompted to explain the salient features that distinguished them: “So this is a first class quality, what were the reasons or what was particularly good, that it received such a high mark”
• Prompts for further probing were used: “in the case of this excellent work, what was it missing that it did not receive a higher mark?”
This initial exercise was followed with a thematic analysis in relation to key learning outcomes. The coursework was laboratory reports which have overlapping learning outcomes with both essays and exams. This initial exercise provided a basis to describe criteria and level descriptors for all assessments which were then amended accordingly to fit different assessment methods. The pre-existing holistic marking guides were also reviewed in the process and integrated as part of the new analytic, more descriptive, rubrics. All key assessment types in the department were covered: laboratory reports, essays (coursework); essay-based exams, and projects. The study reports on essay-based questions in exams given the in-depth case study and modeling. Future publications will report on results for other assessment types. An extract of the rubric for exam marking of essay questions is shown in Table 3 below.
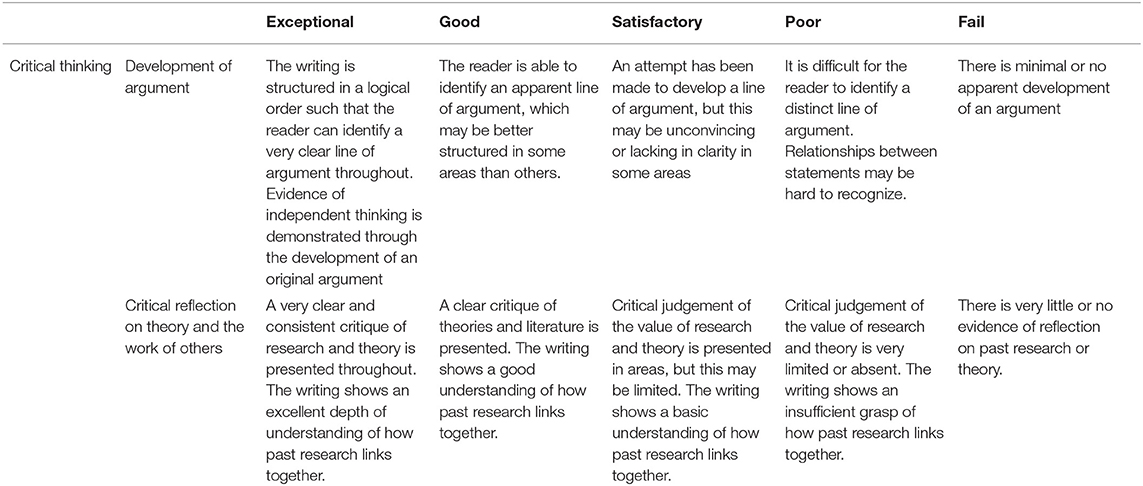
Table 3. Extract of the rubric designed for marking—example of criteria related to the learning outcome of critical thinking in essay questions in exams.
The department design team concluded that since the assessment types were consistent across the program, the same criteria (and associated learning outcomes) were relevant across all years. This is also recommended in the literature to enhance a clear message to the students in their progression through the years of study (Brookhart, 2018).
Expected Weightings of Criteria and Numerical Conversions
Allocation of weightings to analytic rubrics is best done intuitively drawing from expert insights of practitioners into the learning outcomes and progression (Dawson, 2015; Suskie, 2017). As discussed in the review, this is also optional both according to the literature and most guidance in practice. Weightings expressed as percentages is one accepted approach to allocating credit to learning outcomes. Rather than as an exact measure, they are used as a way to rank importance and signal to students what criteria contribute more to marks.
Whilst the rubric that would be published during the trial year would only be used for feedback during marking, the department team also captured the weightings to each criterion considering, in their view, the important aspects of performance in line with instructional learning outcomes. The department leads on this project met and discussed how they thought criteria should be weighted for both the year 2 and the year 3 analytic rubrics. They were asked to consider their own experience, understanding of the department practice and expectations from the undergraduate programs of study. Marker feedback from the interviews also provided additional insights from five colleagues. Starting with expert views is the first step in building a validity argument (Messick, 1994, 1995; Dochy, 2009;Shaw and Crisp, 2012).
The use of a small leading team of three, at this stage, was deliberate for being congruent with the common accepted practices in our context. Typically, module leads make design decisions such as the ones described. Consultation with other markers may happen but that is up to module leads. The whole body of practitioners was not surveyed on their expectations as the team and the interviews offered sufficient insights into assumptions made about the relative importance of criteria in the context of the curriculum objectives. As indicated, introducing too many changes at this point could have been counterproductive.
Lastly, at this point, these weightings were not publicized since they were not relevant to the changes introduced to practice during the trial year. These were relevant to contrast, for the purpose of our investigation in the trial year, how practitioners understood relevance of criteria. Post-marking moderation of assessments in the department had been the main mechanism to check on consistency in marking. In the context of customary holistic marking being the accepted practice, it was counterintuitive to introduce discussions about weightings of criteria at this early stage in introducing changes to the marking culture and practice. Discussions in the wider marking team were planned after the trial year to consider expected relevance of criteria and the analysis of marking data.
Preparation for the Implementation of Analytic Rubrics for Marking and Feedback
At the start of the academic year, all new analytic rubrics were published to staff and students. Activities to engage students in understanding rubrics, quality and expectations from assessment were also implemented (see Boud et al., 2018) to promote understanding of the use and meaning of criteria. However, the current study focuses on the marking aspect.
For the duration of an entire academic year, all rubrics were rolled out across all assessments in the department. Markers were instructed to:
• Mark in the traditional holistic manner as they had always done.
• Use the analytic rubrics to indicate performance levels against criteria during marking.
In a meeting, staff were informed of the rubrics that were to be used across the department in undergraduate assessment. All staff were invited to attend a talk in which a member of the department team outlined the evidence behind rubrics discussed in the literature review and the reasons why analytic rubrics for feedback were being introduced. The specifics of the construction of rubrics were shared (e.g., consultation, interviews with markers), and the criteria and definitions outlined. This discussion served to air any concerns with the provided rubrics. It was important to highlight the evidence-based approach taken to the decision to use rubrics and the creation of the rubrics themselves as this helped to increase staff confidence and trust of the rubric. Staff were shown how the rubric would be used in during marking coursework (on Turnitin). The plan for implementation was outlined, along with tips for marking. These tips encouraged focusing on quality descriptors, avoiding comparisons between students and making quicker judgements on criteria (Brown, 2001).
Decision on marks during marking would remain holistic (i.e., by considering all criteria simultaneously) as was the custom. Assessment types used were the same and decisions on marks (holistic) remained unchanged, criteria were simply made more explicit, that is, they had not changed, they were existing already. It was therefore assumed that decision making, now including a more detailed analytic rubric, would serve the purpose of enhancing validity by possibly reducing the use of irrelevant criteria which is a known risk in the previous approach to marking. Markers were marking to the same standards that were already established in the department and had been maintained through post-marking moderation. Moderation in the department provides a check on all assessments with the module convenors controlling for consistency in marking across marking teams. Whilst greater enhancement of shared standards is in the university's agenda (e.g., introducing pre-marking marker training exercises), in the context of the changes this would be considered in the future once the new rubrics were embedded.
Initially, the introduction of analytic rubrics, as part of the marking process, aimed at providing more detailed and qualitative feedback in line with relevant criteria for the tasks therefore enhancing transparency of marking. Colleagues were informed that marks and analytic rubric judgements, during the academic year, would be analyzed with the aim to gain insights into the relationship between holistic marks and assessment criteria. It was agreed that transitioning from holistic approaches to decision making (marks) to analytic, at such a scale, would require greater clarity about why analytic rules may be needed and the nature of those rules based not only on experts' understanding of criteria but actually by exploring how the customary holistic marking operated. It is noteworthy that these decisions in the implementation of the trial year were in response to sensitivities and the existing perceptions that the traditional holistic marking and moderation processes had established the standards. Introducing analytic rubrics was already a major shift for the department and, in our experience, introducing multiple new ideas simultaneously could be counterproductive.
Rubrics were implemented across the department using diverse modes (Excel, online marking tools) depending on assessment type. For example, in the case of exam marking, markers were given Excel spreadsheets where they would record judgements against set criteria for essay questions in exams during the marking process.
Marking: Holistic Marks and Analytic Rubrics for Feedback
The modified marking procedures served as the data collection mechanism. Marking data from the entire academic trial year generated, for each piece of work (e.g., essay):
• Markers' overall judgements (holistic), summative and expressed in the customary percentage scale. Holistic marking in the department uses peg marking with percentage marks ending in 2, 5, and 8. For example, a maker could not give the grade a 63, they would have to choose between a 62 and a 65. This is typically recommended in the literature (Suskie, 2017).
• Markers in the process of marking recorded their judgements on individual criteria and levels using the analytic rubrics alongside the holistic mark. The completed rubrics were provided for each piece of work. These resulted in a record of associated levels of performance (Fail, Poor, Satisfactory, Good, and Excellent) against each criterion in the rubric (see Table 3).
As a result, the data set consisted of marks with associated judgements of criteria and different levels of performance that had all been formulated during marking. Whilst an extensive data set was gathered for all assessments across the department, this case study focuses on essay-based exam questions in years 2 and 3, during the same academic year. Year 1 exams do not include essay based questions and were not relevant.
A description of the data collected during marking and used in this analysis is below (Table 4). A summary of the total number of exams completed in year 2 and 3 is presented below. In study year 2 all students complete one essay-based question per exam (5 exams in total). In study year 3 students complete two essay questions per exam and take a varying number of exams depending on their module choices. The total number of essay questions assessed is shown in Table 4. Each question was marked with both an overall mark and individual criterion judgements. Marking was completed by a number of staff in the department as the mean number of markers per student in each year. Teams are allocated to mark each exam, depending on their subject specialization. Many staff marked exams across both year groups.
Taking into account that the criteria were common according to assessment type, each year's sample was considered for analysis. All marks in each year have been treated as one big data set (i.e., not as nested variables), despite there being multiple observations per students and a group of markers and across different modules. The total sample contained marks and judgements that belonged to multiple markers and students repeated times. As marking was anonymous, each marker treated each piece of work as an individual case, and made their judgment accordingly and our goal was to model the markers' holistic judgment as they made it. As multiple different markers graded different exams by one student, marker biases would be distributed across different students and different essays, preventing marker bias being modeled as an overall effect. Additionally, the basis of holistic judgement is that it incorporates an individual's opinion, and we want to try to understand that judgement, not control for the subjectivity. Moreover, individual student characteristics were not relevant to our model. The data analysis section fully explains how the sampling method of our non-linear approach to analysis would distribute marker effects to prevent these being modeled.
Data Analysis
Staff-Derived Expected Weightings of Criteria
General guidance on construction of analytic rules advises that experts allocate weightings or other rules (e.g., threshold criteria) based on their understanding and experience (Suskie, 2017). As part of the design procedures, practitioners' expectations of relative contributions of different criteria at different years of study was already captured. The views of academic staff that were deemed representative having consulted with five colleagues and also summing up the views of a department design team of three members. Also, to keep in line with common practice, this was deemed sufficient for the purposes of the investigation at this stage. The team, following customary practice, used percentage weightings to indicate the relative significance of each criterion which did not require any further processing.
Modeling Marking Data (Analytic Criteria and Holistic Marks): Random Forests and Decision Trees
The aim of the study is to provide initial insights into the significance of criteria when formulating holistic judgements (Sadler, 2009a). Ours is an initial exploration by identifying the relative importance of individual criteria to predict different student overall marks (holistic). The marking output, across the department, generated information on student performance captured during marking: holistic marks and levels of performance for each criterion. Machine learning methods were deemed suitable to provide insights into the variable importance of criteria, initial insights into their interactions associated with holistic marking, so that design follow up discussions in practice could follow.
Meta data (e.g., course, marker and student variables) were not input into the model as studying marker and student level effects were not in the scope of this study. In addition, if they were modeled the random forest would have treated them as predictors and they would have interfered with the interpretation of the variable importance, destabilizing the insights into the different criteria.
The reliability of marks was assumed to be established via the existing departmental procedures for post-marking moderation. Usually, a proportion of work, decided by module convenors, graded by each marker is reviewed per module. Our study is primarily concerned with understanding the relationship between criteria and marks. The departmental checks for inter-marker agreement, whilst limited, was deemed sufficient for the purpose of our analysis. The marks were treated as true marks and this is something that future studies may address by collecting multiple judgements on each piece of work to arrive at a true mark. This is a limitation of conducting a study in a real setting.
The analytic information gathered per criterion during marking with the analytic rubric, were transformed to the numerical values associated with each level of quality (Excellent through to Fail) as discussed with the design team (see results). We obtained weights for each criterion from fitting a prediction model to the data and extracting the variable importance marks (i.e. how useful each criterion was at helping the model to make the prediction—see below for more detail). The model predicted the overall holistic mark using the numerical marks applied to each criterion (e.g., Excellent = 85). The prediction model we used was a random forest algorithm (Breiman et al., 1984). We chose this algorithm as random forests deal well with missing data, are non-linear, and high correlations between the criteria (independent variables) would not affect the result. The latter was particularly important as five correlations between the independent variables were over 0.7 for both groups' data1.
A random forest is an algorithm that produces many decision trees using different samples of the data. Decision trees make predictions by splitting the data using binary decisions to reduce the most variance within the data or subsample (Breiman et al., 1984). The splitting process is repeated on each new subsample, creating the tree structure. To choose where to split the data the algorithm iterates through all the input variables, and points on the variables, to find the split-point which gives the greatest decrease in variance. The chosen point is the split which minimizes the sum of the within-group sum of squares (SS) of the two new subsamples. Random Forests are more powerful than individual decision trees as they build multiple trees using different random samples of the data and independent variables, which prevents the model from being sculpted to one dataset, and therefore reflect more generalized relationships. The prediction produced by the forest is an average across all of the trees, and usually more accurate than a single decision tree. For more information on decision trees or random forests see Breiman et al. (1984).
Random forests allow us to quantify how useful each of the different criteria are at making predictions using an inherent process called variable importance (Grömping, 2009). Each split's effectiveness is determined by how much that split decreases node impurity which, for a regression tree, is the error (Friedman, 2001). Here we used the residual sum of squares. To assess how useful a variable is over the whole tree, the incremental node purity is calculated as the sum of the decrease in node impurity for each time the variable is used to split the data. For the incremental node impurity of a variable over the whole forest, the average incremental node purity across all the trees in the forest is taken. To enable us to compare the importance rankings across different models (i.e., comparing year 2–3) the incremental node purity needed to be transformed to the same scale. The total incremental node purity within a tree varies depending on how much error can be reduced within a tree, which will depend on what variables the tree can select from, what sample of data the tree is modeling, and the length and accuracy of the tree. Thus, we converted the incremental node impurity into a percentage (Grömping, 2009) in order to make relative variable comparisons. We used these percentages as the weights for our data-derived algorithm. Therefore, the resulting variable importance of each criterion is reported as a percentage weighting which is used to rank the criterion. This rank allows us to compare how strongly each criterion relates to the overall holistic mark.
Because of the nature of random forests, each time the algorithm is run the prediction error can vary slightly, and each tree will have a slightly different path it took to get to the prediction, depending on what data it is modeling and what variables are available for it to select. To combat this potential instability of the model, we used 300 trees in the random forest. Three hundred trees were selected as it is the point at which error does not decrease any further when more trees are added to the forest (see Figure 1). Figure 1 shows the decrease in mean square error (MSE) plotted against the number of trees in our random forest. After 300 trees the error does not decrease. As the variable importance results were extracted from an average of 300 iterations we expected these results to be suitably stable. The stability of the variable importance rankings was also explicitly tested using 10 iterations with 10 different random seeds. As the random forest will be affected by the data samples randomly selected for the individual trees and the sample of variables randomly selected at each split, each of the 10 iterations will have slightly differing information for the random forest algorithm to use. We found that for 9 out of the 10 iterations the rankings of the variables in importance were consistent with the rankings found in our analysis, indicating that our results are indeed stable and reliable.
Post-hoc exploratory analyses were used to fit a single decision tree to year 2 and year 3 data, enabling us to view the tree structure, and the information associated with each split decision. These decision trees will be included to provide additional insights about the complex logic of holistic judgements. Decision trees can allow for potential interactions to be discovered, as well as uncover non-linearities in the data. This can help to counter the limitation of using weightings as a linear and analytic formula.
Ethical Consent
Whilst marking data was gathered as part of marking, the proposed extra analyses were conducted only with anonymized data. An ethical committee considered anonymization procedures prior to analyses being conducted. No ethical threats were posed by the proposal and therefore procedures were compliant with ethical conduct.
Results: Expected and Data-Derived Criterion Weightings
This section provides the results of our exploration of the contribution of criteria according, first, to the interpretation of practitioners (experts). Secondly, the results of the machine learning analyses of marking data gathered throughout the year on exam essay-based questions provide a data-derived insight into how individual criteria relate to holistic marks.
Staff-Derived Expected Criterion Weightings
The department design team assigned weightings according to their views of what should attract marks for different criteria, at different stages, in the study program (see Table 5). This study reports on results from essay-based exams in years 2 and 3 of study.
The expectations of the department design team were that markers would alter the way in which they valued criteria across different years. In earlier years, it was perceived that markers are looking out for knowledge and the ability to articulate that knowledge. Later in the degree (year 3), it is expected that students have that skill and so markers then put more value on other criteria, such as critical reflection. These thoughts were guided by the structure of the observed learning outcome (SOLO) taxonomy (Biggs and Collis, 1982), which classes analysis and evaluation as a more complex skill compared to knowledge and comprehension. Weightings were allocated based on these reflections. When discussing these ratings, the team were aware that writing style criteria were no longer going to be considered as a formal learning outcome in exams, which is why they were weighted zero.
In addition, the design team also provided numerical values for the defined levels of quality for each criterion which appeared as: Fail, Poor, Satisfactory, Good, and Excellent. The department design team used grade boundaries from degree classifications as guidance. These were: Excellent = 85, Good = 65, Satisfactory = 55, Poor = 45, and Fail = 35.
Data-Derived Criterion Weightings and Decision Trees of Holistic Decision Making (Holistic Marks and Criteria)
The random forest analysis generated insights into the variable importance of different criteria in relation to holistic marks. In our analysis we have converted the variable importance of individual criteria to weightings associated with individual criteria. This has been done bearing in mind our practitioner context and the need to offer the results of this ranking to be compared with the practitioner recommended weightings. The main result, however, is the resulting ranking of the criteria, rather than the exact weightings. The resulting weightings associated with each criterion are below (Table 6).
Decisions about students' overall marks were influenced by knowledge and understanding related criteria (i.e., descriptions and explanations; relevance and range of literature), those criteria were more highly weighted by markers (Table 6). Critical thinking ranked lower in its contribution to overall marks. Stylistic and writing related skills contributed very little. It is also noteworthy that, overall, the ranking of importance of different criteria associated with decisions made holistically (marks) is consistent across years of study.
In recognition that the conversion to percentage weightings of criteria is limited in revealing the complex interactions of criteria we also fit a single decision tree to year 2 and 3 data to better understand and break down the holistic judgments. The tree for year 2 that gave the most accurate prediction (measured using cross validation error) was a tree with 60 splits. However, after 15 splits, error did not dramatically decrease (Figure 2). For visualization purposes we have included the diagram of the tree with 9 splits in order to demonstrate the most important criteria and split points (Figure 4).
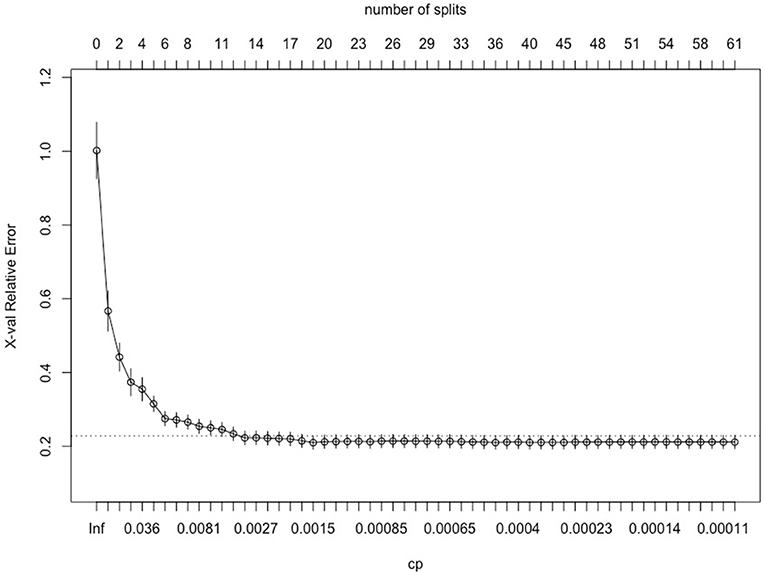
Figure 2. Effect of the tree size (number of splits) along the top compared with the relative cross validated error. Cp along the bottom refers to the control parameter used in the R package rpart to determine the size of the tree (Year 2).
A single decision tree was also fit to the year 3 data to try to better understand and break down the average holistic judgment being made. The tree which was the most accurate (measured using cross validation error) was a tree with 66 splits. However, after around 15 splits, error did not decrease (Figure 3). For visualization purposes Figure 5 shows a tree with 10 splits.
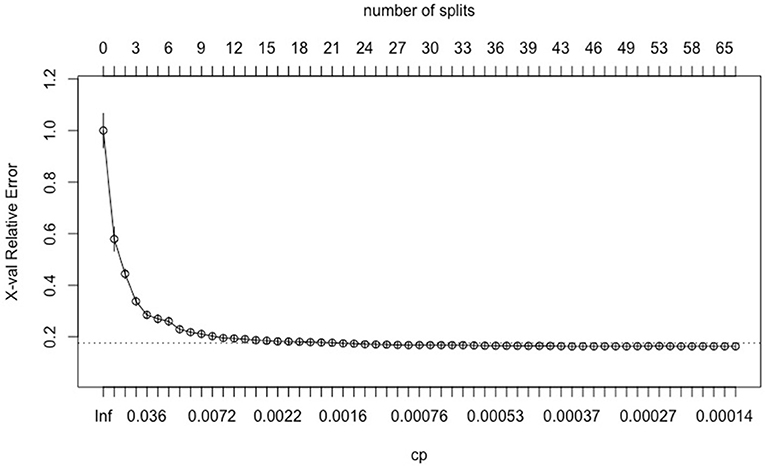
Figure 3. Effect of the tree size (number of splits) along the top compared with the relative cross validated error. Cp along the bottom refers to the control parameter used in the R package rpart to determine the size of the tree (Year 3).
Decision trees are included here to illustrate the non-linear relations between criteria and holistic marks, the important criteria and points to predict the resulting holistic marks. Decision trees illustrate how most important criteria split the data and related to higher or lower marks. Decision trees show average marks and the proportion of the sample and the criterion cut-off point. This tree has been pruned to include the first set of binary decisions and predicts 10 different marks (in the range of 23–74%). Each decision point in the tree shows the tree's prediction (mark) at that point in the structure (i.e., 62) and the percentage is how many people from the sample is in each group or “node” (i.e., 100% of the sample at the top).
The illustrative decision tree for year 2 data (Figure 4) shows that only four criteria (critical reflection, relevance and range of the literature, development of the argument, descriptions and explanations of concepts) are used in the top 10 decisions, showing that these are the most important, corroborating our variable importance results from the random forest. Structure of the sentences and paragraphs, use of scientific language, and in text citations were not used in the first 10 splits of the decision tree prediction, showing these are less important predictors. The most important binary predictor of overall grade was whether or not the relevance and range of the literature was greater or less than a 60 or a “Good.” For example, the year 2 tree shows an average mark of 62 (out of 100), with 100% of the data in the top node. The first decision to split the data is whether the relevance and range of the literature is above or below a 60 (out of 100).
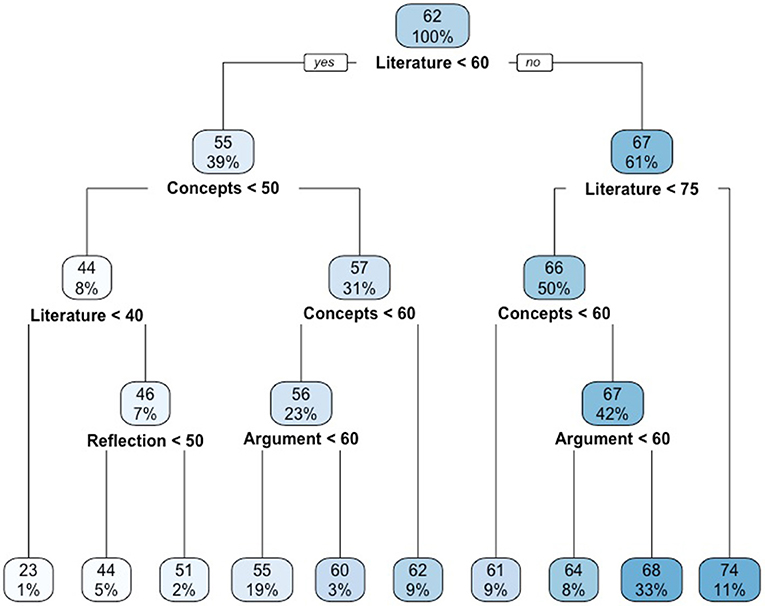
Figure 4. Simplified decision tree for year 2 exams, pruned after 9 splits for illustrative purposes. The top number in each node is the percentage mark prediction at that point in the tree, and the bottom number is the percentage of the sample contained within that node. Literature: Relevance and range of the literature; Concepts: Descriptions and explanations of concepts and relation between them; Argument: Development of the argument; Reflection: Critical reflection on the work of others.
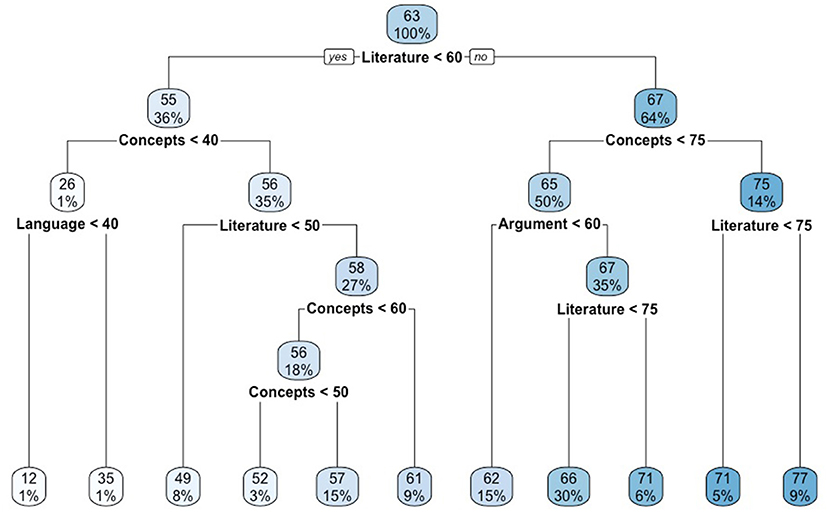
Figure 5. Simplified decision tree for year 3 exams, pruned after 10 splits for illustrative purposes. The top number in each node is the percentage mark prediction at that point in the tree, and the bottom number is the percentage of the sample contained within that node. Literature: Relevance and range of the literature; Concepts: Descriptions and explanations of concepts and relation between them; Argument: Development of the argument; Language: Use of scientific language and style.
Both trees (year 2 and 3) use the same first split (Literature > 60?), then if YES whether the descriptions and explanations of concepts >50 or not. The tree for year 3 does not use critical reflection on theory and the work of others in the top 10 splits. In both trees relevance and range of the literature predicts highest and lowest marks. The decision trees are not complete but illustrate how the random forest algorithm uses criteria to split the data and make predictions of holistic marks.
Expected and Data-Derived Criterion Weightings: Contrasts
The graphs (Figures 6, 7) show the comparisons between expected weightings (expert derived) and data-derived weightings of criteria for essay-based questions in exams. Each graph shows the contrasts for a different year of study. The resulting rankings of importance, between expected (expert assumptions) and actual (data-derived) differ with critical thinking being perceived as the most significant aspect in instruction but ranks lower in the actual weightings received during holistic marking. The second contrast is that, from an instructional angle, a progression was expected from year to year reflected as a greater weighting of critical thinking in year 3. This assumption was not reflected in the weightings derived from the modeling of tutors' overall marks and analytic criteria.
Summary and Discussion
A department-wide project sought to implement rubrics and enhance their alignment with intended learning outcomes. The ultimate goal was to ensure transparency in the communication of expectations and uses of rubrics in marking with feedback. A review of the literature informed several decisions on rubric design: use of analytic design of rubrics for greater detail in feedback and more transparent use of criteria. The review also highlighted gaps in research. Holistic or analytic approaches to deriving marks are left to practitioners' choice. Whilst holistic marking was the custom in the particular context of the department, questions over its alignment and nature in relation to analytic rubrics were raised. The literature on the matter offered a complex set of perspectives with a limited evidence base mainly drawn from comparative studies which remains inconclusive presenting somewhat contradictory findings, often from small scale studies.
Research to date warning of challenges to validity associated with holistic approaches (e.g., use of irrelevant criteria, idiosyncratic rules) seemed to contradict some claims in favor of holistic approaches. Most guidance on the use of holistic or analytic approaches to deriving marks, leave the decision to practitioners as optional. A sense of an absence of a clear rationale (Dawson, 2015; Suskie, 2017) provided the basis for our exploratory study.
With view to advancing our understanding of the rationale for either approach, the role of these approaches for the wider promulgation of standards in a collective (multiple markers and students) remains under-investigated. For example, proponents of holistic approaches make claims about the intrinsic validity of holistic judgement to capture complex relations amongst criteria as well as to promulgate standards in communities of practice involving students (Sadler, 1987, 2009b). Ensuring consistency between messages and expectations and actual outcomes (marks) is an essential aspect of validity (structural) and plays a significant role for student learning. However, many of the claims made remain unsubstantiated.
The present study has provided some initial insights into the workings of holistic marking that may support further examination of claims made about the extent to which holistic approaches are adequate vehicles for the definition and promulgation of standards in departments. The study provided some insights into the purported alignment of holistic marking with instructional intended learning outcomes. In order to elicit insights into the cohesion between these aspects, analytic and holistic approaches in decision-making, marking and feedback have been combined. The case study has collected multiple sources of information. Firstly, assumptions about relevant criteria and expected weightings by a department design team were captured. Secondly, marking data were collected using an analytic rubric during an entire academic year alongside the customary marking (holistic marks). Machine learning techniques have enabled the exploration of relevant desired instructional qualities (criteria and levels) related to tutor decisions on overall marks.
The study provided initial insights into how holistic marks relate to relevant task criteria and, by extension, with learning outcomes. Secondly, contrasts with assumptions made by a department design team provide an initial basis for discussions about alignment between marks and relevant criteria. Whilst the project encompassed all assessments across a department, the report has focused on marking of essay-based questions in exams in two years of study (year 2 and 3).
Q1 What are the expectations by practitioners of the importance and contribution of different criteria in marking?
In the design of the analytic rubrics, a department team of three members was asked to allocate weightings based on their experience and understanding of the instructional learning outcomes. In other words, this is expert judgement which is a valid approach discussed in the reference literature to deciding combination rules associated with analytic approaches (Messick, 1994, 1995, 1996; Suskie, 2017). Also, this was conducted in a way consistent with practice in the context of the institution. One or a few colleagues typically might decide weightings of criteria estimating their value according to their understanding of important criteria (related to learning outcomes).
The department team expressed their views of a ranking of importance of the criteria in the form of percentage weightings which is customary practice. The department team made assumptions about the greater importance of critical thinking overall. Also, further assumptions were made about progression across years of study. More advanced years of study would see the increased difficulty also reflected in the increased weighting by awarding a higher proportion of marks according to performing better on criteria such as critical thinking.
Q2 What is the contribution of different criteria associated with holistic marks?
The study has explored holistic judgement by deploying analytic rubrics as ancillary during marking. These have provided a basis for quantifying holistic marks, narrowing down the breadth of criteria used and retaining holistic formulation of marks, both in line with recommendations from the literature. Percentage weightings, elicited using random forests analyses, indicate the existence of a ranking of importance and contribution of different criteria to overall marks. The percentage weightings provide insights into the ranking of the contributions by different criteria deemed more important when deciding marks holistically. Decision trees illustrate the non-linear relations further evidencing the ranking of criterion contributions.
The nature of the rules underlying marks, reveals that criteria relating to knowledge and understanding (i.e., descriptions and explanations of concepts; relevance and range of the literature) contributed more highly toward the overall mark than critical thinking related criteria (i.e., development of argument, reflection on theory). Style and writing related criteria contributed much less. Also, year 2 and 3 results were quite similar in terms of the contribution of different criteria toward the final mark.
The study illustrates how analytic criteria and information elicited during marking, may be used to gain insights into the implicit weightings associated with holistic marks.
Q3 How do practitioner expected and data derived weightings in holistic marking relate?
Percentage weightings are a common way to indicate rankings of importance for relevant assessment criteria. Rather than the exact weightings, our main interest is in the different rankings of criteria elicited by using these two different approaches. The second main contrast relates to assumptions made about progression between years and increasing the reward for more difficult outcomes, according to practitioners' (expert) views.
Critical thinking was expected to attract more marks by the department team. In the final year of study, an increase in its weighting would account for a higher demand in the performance. The study reveals misalignments between the assumptions and expectations made about the contribution of certain criteria and how these were weighted, in effect, during marking holistically. The first important comparison is that generally, what the department team deemed to be of greater importance (i.e., critical thinking), indeed ranked lower to criteria that were considered easier (e.g., describing concepts, explanations, selecting appropriate literature) when compared to the statistical analysis.
The year 2 and 3 data analysis also showed that the rankings of the criteria according to the data-derived weightings remain stable across years of study. The assumption that more important criteria attract more marks, expected by staff, is not really substantiated by the analysis of marking data in relation to holistic marks. Secondly, how progression occurs would need to be further explored as the criteria weightings in different years is stable.
Implications for Practice
The study aimed primarily to provide a basis for discussions in practice concerning the status of combination rules in marking. The findings from this study provide a complementary perspective on the role and rationale to use both holistic or analytic approaches to marking. The findings, in an authentic marking setting and based on a large marking data sample, give a new perspective. Previous smaller scale studies alerted to the threats posed to content validity derived from the lack of transparency associated with holistic approaches to marking.
The study builds from previous research showing that irrelevant criteria may be used during marking holistically therefore weakening the validity of holistic marking (content) (Harsch and Martin, 2013; Björklund et al., 2015; Bloxham et al., 2016a). Our study flags the existence of additional misalignments between expectations of criterion weightings with de facto rules. This further contributes to potentially obscuring messages or producing contradictory ones. The large-scale analysis of marking gives a sense of scale and impact of the misalignment between expected instructional learning outcomes and the implicit rules of holistic marking. This finding bears important implications for practitioners, students and learning. Implications for practice are discussed below both in a general context and more concretely in the follow-ups in the particular context of our departmental project.
Firstly, the study has shown how in an authentic marking situation, marking data from teams may be exploited to gain insights into holistic judgement through the use of ancillary analytic criteria. In the specific setting of the study, the findings have provided a basis for revisions of their assumptions and more detailed discussions about the nature and purpose of the assessments. More widely, extending to other contexts, the study has exemplified a promising avenue for both further enquiry and to enable faculty teams to develop understanding of the rules underlying holistic marking practices.
Secondly, expected (practitioners) and de facto (data derived) combination rules were misaligned. In lay terms, this means that what practitioners might have been communicating to students, or assuming, whether discussed or not discussed, were indeed misaligned with the actual decision making in holistic marking. Holistic marks may be misaligned with assumptions made by teams about what is more important. Rules, unchecked and implicit, render interpretation of the output marks, both for students and staff, invalid or at least unclear. Interpretation of marks using rubrics, without explicit allocation of weightings, may be invalid. Learning and validity of marks therefore are at risk simply by the absence of a clear guideline. As a result, it would follow that publishing weightings associated with criteria would be important to enhance transparency and reduce the misalignments shown. This would be the case regardless of whether holistic or analytic approaches are in place since, even when holistic judgements are made it appears that we can uncover which criteria play a more significant role.
Thirdly, beyond the particular focus of the study, the results enable a different perspective that transcends the dominant perception of holistic and analytic as opposites and revealing the potential to use them as complementary tools as has been highlighted in some literature (e.g., Hunter et al., 1996; Harsch and Martin, 2013). Practitioners might gain important insights from the combination of both approaches to understand how marking is in effect aligning with desired outcomes in a collective. Implementation decisions regarding analytic and holistic approaches to marking may consider different options:
a) Retain holistic marking practice but publish the “tacit” relationship with marks, that is, retain holistic marking but declaring the verified rules (i.e., what is awarded more marks).
b) Introduction of analytic marking, applying explicit formulae that are also published to the community, in line with expected learning outcomes.
Lastly, in the context of the department in which the study was conducted, the review led to the replacement of holistic marking with a fully analytic method (marking and feedback). Analytic rubrics have now been introduced not only as a feedback tool but also to derive marks introducing a given formula. The analysis presented here provided the basis for a discussion with markers who trusted the introduction of analytic rules based on the de facto weightings. The enhancement project is not concluded, and many areas of marking practice still need addressing, the trial and investigation alleviated fears of the introduction of rules that were not in line with the holistic practice.
Limitations and Further Research
The case study, set deliberately in an authentic marking context, provides insights that may transfer to other contexts and conveys a sense of the combined potential of holistic and analytic approaches in marking. Despite the contributions, the case study also has limitations to be considered in future investigations. The study is part of a project that followed a stepped approach given sensitivities in the change of the assessment and marking culture in a real setting. The study has provided valuable initial exploratory insights but future studies can expand and further challenge the findings from this study.
Future studies may consider whether marker training, more intensive use of exemplars and discussing explicitly criteria, may have affected holistic marking and use of criteria as reflected in the analysis of marking data. The present study used a small team of three members keeping in line with a natural approach in context. This could be addressed in future studies. Explicit training discussing relevant weightings of criteria was not seen to be appropriate in the context of the present study given that holistic marking had been the tradition and that was left unchanged during the trial. However, future studies might introduce explicit discussions about expected weightings and perhaps training to explore the influence of such measures on marking outputs to identify whether more intense training might have achieved greater alignment between expectations and de facto criterion contributions to holistic marks, in holistic marking. This is important as it might validate one of the claims in favor of holistic approaches that use of exemplars and discussions are sufficient to promulgate standards (Sadler, 2009b).
The reliability of marks was established with existing departmental moderation mechanisms. As explained, we were interested in the relationship between criteria and overall marks, and reliability in this context was not central. Other ways of strengthening reliability and use of more robust true marks could have been achieved by having multiple markers judging the same piece of work.
Future analyses, also drawing from our case, will address aspects of assessment type and context. For example, exam and coursework settings may impact on the role of different criteria and holistic marks. The exam conditions under which students wrote essay-based questions might play a role. Contrasts with coursework conditions for writing essay-based questions will be reported in follow up publications.
Additional important questions for practitioners remain unanswered. Our study has explored how the combined use of analytic and holistic approaches can offer new perspectives to uncover the underlying nature of holistic marking. Whilst we have, in the context of use, opted to use a conversion to weightings, many more combination rules should be explored.
Further investigation of different combination rules and their implications for overall marks are significant aspects that future research should take up. Our exploratory study, attempting to model holistic marking, has elicited rankings of importance of criteria, translated these into criterion weightings and used illustrative decision trees. Many more perspectives are yet to be understood and brought to bear on this subject. For example, our next analysis will consider Rasch measurements which provide insights into the discriminating power of different criteria (Suskie, 2009; Utaberta and Hassanpour, 2012). This would offer complementary perspectives in understanding and developing effective combination rules.
Furthermore, the criteria that were selected by the random forests as being the most important predictors were the criteria that were best for dividing up the data over the whole span of different marks (i.e. for fail to excellent), and the relevance and range of the literature criterion was the most successful at this. However, some criteria may be better at predicting a pass from a fail, but not be good at predicting a fail from excellent. Therefore, it is important to recognize this as a limitation. For example, it could be that critical reflection can predict a good from an excellent, but is not as useful at predicting lower grades. Further analyses are planned to address which criteria are best at predicting between different grade boundaries, investigating the possible non-linearity and interdependencies of the rubric criteria. Further investigation of decision trees may offer insights into which criteria could be interpreted as threshold criteria at different levels. Threshold criteria and complex rules would be difficult for markers to define without a basis. Our decision trees offer an initial exploration of how interdependencies may be explored in future studies. Future analyses plan to pick apart the dependencies between the rubric criteria, and to evaluate whether hierarchical rules may be more appropriate. For example, questions such as whether it is valid to assess critical reflection when knowledge and understanding does not meet a certain level should be addressed.
Lastly, analyses are being re-run with similar data from new cohorts to correct from possible biases. The study highlights the existence of rules underlying holistic marking and provides evidence for a potential misalignment between assumptions made by practitioners and actual rules underlying collective holistic marking. Important aspects of validity come under threat (structural validity) if these underlying rules and assumptions are not made visible. As highlighted above, future studies should further explore how training might moderate the findings from our study. Moreover, uncovering further non-linear interactions amongst criteria might be further explored in the future.
Conclusions
In sum, the case study has provided complementary insights into the nature of holistic marking. The potential for misalignment with expectations, from an instructional point of view, warrant further consideration of the implications for validity in its widest sense (structural, consequential). The study concludes that holistic marking and analytic criteria can offer a productive perspective on marking. Rather than arguing that either is the better option, we argue that their combination, to investigate the nature of criteria in relation to overall marks, can be enlightening for practitioners. The results should encourage practitioners to check such underlying rules in their own contexts to ensure clarity and alignment with the communication of expectations involving both markers and students. Further pointers for research have also been discussed to productively advance understanding to date.
Data Availability
The datasets for this study will not be made publicly available because this is sensitive marking data gathered from a department in a university which cannot be published.
Ethics Statement
The study and collection of data procedures were approved by the Ethics Committee of the University of Nottingham. The Head of Department granted permission to the analyses of marking data which were provided to the authors in an anonymized format.
Author Contributions
CT and EW have led the conception and design of the study, data gathering, data analysis, and interpretation. CT has led the writing of the manuscript with section contributions by EW, RL-H, and KS. RL-H and KS have led the data analysis. All authors contributed to manuscript revision, read, and approved the submitted version.
Conflict of Interest Statement
The authors declare that the research was conducted in the absence of any commercial or financial relationships that could be construed as a potential conflict of interest.
Acknowledgments
We would like to thank Dr. Chris Brignell (School of Mathematical Sciences, University of Nottingham) for his support and advice on the analyses in this study.
Footnotes
1. ^This also makes the use of linear models unfit for our analysis since highly related variables will explain a large percentage of the same variance in the data and this would interfere with interpretation of importance of the variables (Allison, 2012). This issue of multicollinearity is usually dealt with either by removing or combining features (Mini Tab, 2013). As we wanted to know how important all of the seven variables were in their raw form (without transforming or engineering them) the random forest algorithm was used.
References
Allison, P. (2012). When Can You Safely Ignore Multicollinearity? Statistical Horizons. Available online at: https://statisticalhorizons.com/multicollinearity.
Andrade, H. (2005). Teaching with rubrics: the good, the bad, and the ugly. Coll. Teach. 53, 27–31. doi: 10.3200/CTCH.53.1.27-31
Andrade, H., and Du, Y. (2005). Student perspectives on rubric-referenced assessment. Pract. Assess. Res. Eval. 10, 1–11. Available online at: http://PAREonline.net/getvn.asp?v=10&n=3
Biggs, J., and Tang, C. (2011). Teaching For Quality Learning At University, 4th Edn. Buckingham: The Society for Research into Higher Education and Open University Press.
Biggs, J. B., and Collis, K. F. (1982). Evaluating The Quality Of Learning: The SOLO Taxonomy (Structure of the observed learning outcome). London: Academic Press.
Björklund, L., Stolpe, K., Lundström, M., and Åström, M. (2015). “To measure the unmeasurable: using the repertory grid technique to elicit tacit criteria used by examiners,” in 5th International Assessment in Higher Education Conference (Birmingham).
Bloxham, S., Boyd, P., and Orr, S. (2011) Mark my words: the role of assessment criteria in UK higher education grading practices. Stud. Higher Educ. 36, 655–670. doi: 10.1080/03075071003777716
Bloxham, S., den-Outer, B., Hudson, J., and Price, M. (2016a). Let's stop the pretence of consistent marking: exploring the multiple limitations of assessment criteria. Assess. Eval. High. Educ. 41, 466–481. doi: 10.1080/02602938.2015.1024607
Bloxham, S., Hughes, C., and Adie, L. (2016b). What's the point of moderation? A discussion of the purposes achieved through contemporary moderation practices. Assess. Eval. High. Educ. 41, 638–653. doi: 10.1080/02602938.2015.1039932
Bloxham, S., and Price, M. (2013). External examining: fit for purpose?. Stud. High. Educ. 40, 195–211. doi: 10.1080/03075079.2013.823931
Boston, C. (2002). Understanding Scoring Rubrics: A Guide for Teachers. College Park, MD: ERIC Clearinghouse on Assessment and Evaluation.
Boud, D. (2018). Assessment could demonstrate learning gains, but what is required to do so? High. Educ. Pedagogies 3, 4–6. doi: 10.1080/23752696.2017.1413671
Boud, D., Ajjawi, R., Dawson, P., and Tai, J. (2018). Developing Evaluative Judgement in Higher Education. Abingdon: Routledge. doi: 10.4324/9781315109251
Breiman, L., Friedman, J. H., Olshen, R. A., and Stone, C. J. (1984). Classification and Regression Trees. Boca Raton, FL: Chapman and Hall/CRC. Available online at: https://www.crcpress.com/Classification-and-Regression-Trees/Breiman-Friedman-Stone-Olshen/p/book/9780412048418#googlePreviewContainer
Brookhart, S. M. (2018). Appropriate criteria: key to effective rubrics. Front. Educ. 3:22. doi: 10.3389/feduc.2018.00022
Brookhart, S. M., and Chen, F. (2015). The quality and effectiveness of descriptive rubrics. Educ. Rev. 67, 343–368. doi: 10.1080/00131911.2014.929565
Brookhart, S. M., and Nitko, A. J. (2019). Educational Assessment Of Students, 8th Edn. Boston, MA: Pearson Education
Brown, G., Bull, J., and Pendlebury, M. (1997). Assessing Student Learning in Higher Education. London: Routledge.
Brown, George. (2001). Assessment: A Guide For Lecturers. York: LTSN Generic Centre: Assessment Series 3.
Dawson, P. (2015). Assessment rubrics: towards clearer and more replicable design, research and practice. Assess. Eval. High. Educ. 42, 347–360. doi: 10.1080/02602938.2015.1111294
Dochy, F. (2009). “The edumetric quality of new modes of assessment: some issues and prospects,” in Assessment, Learning and Judgement in Higher Education, ed G. Joughin (Dordrecht: Springer Netherlands), 1–30. doi: 10.1007/978-1-4020-8905-3_6
Elton, L. (1998). Are UK degree standards going up, down or sideways?. Stud. High. Educ. 23, 35–42. doi: 10.1080/03075079812331380472
Elton, L., and Johnston, B. (2002). Assessment in Universities: a critical review of research. Learning and Teaching Support Network. Available online at: https://eprints.soton.ac.uk/59244/1/59244.pdf.
Friedman, J. (2001). Greedy function approximation: a gradient boosting machine. Ann. Statist. 29, 1189–1232. doi: 10.1214/aos/1013203451
Grainger, P., Purnell, K., and Zipf, R. (2008) Judging quality through substantive conversations between markers. Assess. Eval. High. Educ. 33, 133–142. doi: 10.1080/02602930601125681
Grömping, U. (2009). Variable importance assessment in regression: linear regression versus random forest. Am. Statist. 63, 308–319. doi: 10.1198/tast.2009.08199
Harsch, C., and Martin, G. (2013). Comparing holistic and analytic scoring methods: issues of validity and reliability. Assess. Educ. 20, 281–307. doi: 10.1080/0969594X.2012.742422
Hay, P., and Macdonald, D. (2008). (Mis)appropriations of criteria and standards-referenced assessment in a performance-based subject. Assess. Educ. 15, 153–168. doi: 10.1080/09695940802164184
Hunter, D. M., Jones, R. M., and Randhawa, B. S. (1996). The use of holistic versus analytic scoring for large-scale assessment of writing. Can. J. Prog. Eval. 11, 61–85.
Huot, B. (1990). Reliability, validity and holistic scoring: what we know and what we need to know. Coll. Composit. Commun. 41, 201–213. doi: 10.2307/358160
Jones, I., and Alcock, L. (2014). Peer assessment without assessment criteria. Stud. High. Educ. 39, 1774–1787. doi: 10.1080/03075079.2013.821974
Jönsson, A., and Prins, F. (2019). Editorial: transparency in assessment—Exploring the influence of explicit assessment criteria. Front. Education. 3:119. doi: 10.3389/feduc.2018.00119
Jönsson, A., and Svingby, G. (2007). The use of scoring rubrics: reliability, validity and educational consequences. Educ. Res. Rev. 22, 130–144. doi: 10.1016/j.edurev.2007.05.002
Kuo, S. (2007). Which rubric is more suitable for NSS liberal studies? Analytic or holistic? Educ. Res. J. 22, 179–199. Available online at: http://www.fed.cuhk.edu.hk/ceric/erj/200700220002/0179.htm
Macdonald, R., and Joughin, G. (2009). “Changing assessment in Higher Education: a model in support of institution-wide improvement,” in Assessment, Learning and Judgment in Higher Education, ed G. R. Joughin (Dordrecht: Springer), 193–213. doi: 10.1007/978-1-4020-8905-3_11
Messick, S. (1994). The interplay of evidence and consequences in the validation of performance assessments. Educ. Res. 23, 13–23. doi: 10.3102/0013189X023002013
Messick, S. (1995). Validity of psychological assessment: validation of inferences from persons' responses and performances as scientific inquiry into score meaning. Am. Psychol. 50, 741–749. doi: 10.1037/0003-066X.50.9.741
Messick, S. (1996). “Validity in performance assessments,” in Technical Issues in Large-scale Performance Assessment, ed G. W. Phillips (Washington DC: National Centre for Educational Statistics, 1–18.
Mini Tab (2013). Enough Is Enough! Handling Multicollinearity in Regression Analysis. The Minitab Blog. Available online at: https://blog.minitab.com/blog/understanding-statistics/handlingmulticollinearity-in-regression-analysis.
Panadero, E., and Broadbent, J. (2018). “Developing evaluative judgment: self-regulated learning perspective,” in Developing Evaluative Judgement in Higher Education. Assessment for Knowing and Producing Quality Work, eds D. Boud, R. Ajjwi, P. Dawson, and J. Tai (London: Routledge), 81–89. doi: 10.4324/9781315109251-9
Panadero, E., and Jönsson, A. (2013). The use of scoring rubrics for formative assessment purposes revisited: a review. Educ. Res. Rev. 9, 129–144. doi: 10.1016/j.edurev.2013.01.002
Panadero, E., and Romero, M. (2014). To rubric or not to rubric? The effects of self-assessment on self-regulation, performance and self-efficacy. Assess. Educ. 21, 133–148. doi: 10.1080/0969594X.2013.877872
Perlman, C. (2002). “An introduction to performance assessment scoring rubrics,” in Understanding Scoring Rubrics, ed C. Boston (College Park, MD: ERIC Clearinghouse on Assessment and Evaluation), 5–13.
Reddy, Y. M., and Andrade, H. (2010). A review of rubric use in higher education. Assess. Eval. High. Educ. 35, 435–488. doi: 10.1080/02602930902862859
Sadler, D. R. (1987). Specifying and promulgating achievement standards. Oxford Educ. Rev. 13:2. doi: 10.1080/0305498870130207
Sadler, D. R. (2009a). Indeterminacy in the use of preset criteria for assessment and grading in higher education. Assess. Eval. High. Educ. 34, 159–179. doi: 10.1080/02602930801956059
Sadler, D. R. (2009b). “Transforming holistic assessment and grading into a vehicle for complex learning,” in Assessment, Learning and Judgement in Higher Education, ed G. Joughin (Dordrecht: Springer, 45–63.
Sadler, R. D. (2014). The futility of attempting to codify academic achievement standards. High. Educ. 67, 273–288. doi: 10.1007/s10734-013-9649-1
Shaw, S., and Crisp, V. (2012). An Approach to Validation: Developing and Applying an Approach for the Validation of General Qualifications. Research Matters: A Cambridge Assessment Publication, Special Issue 3. Available online at: https://www.cambridgeassessment.org.uk/Images/110003-research-matters-special-issue-3-an-approach-to-validation.pdf
Stevens, D., and Levi, A. (2005). Introduction to Rubrics: An Assessment Tool to Save Grading Time, Convey Effective Feedback, and Promote Student Learning, 2nd Edn. Sterling: Stylus (VA). Available online at: https://www.amazon.es/Introduction-Rubrics-Assessment-Effective-Feedback/dp/1579225888
Suskie, L. (2009). “Using assessment results to inform teaching practice and promote lasting learning,” in Assessment, Learning and Judgement in Higher Education, ed G. Joughin (Dordrecht: Springer Netherlands), 133–152. doi: 10.1007/978-1-4020-8905-3_8
Suskie, L. (2014). Five Dimensions of Quality: A Common Sense Guide to Accreditation and Accountability. San Francisco, CA: Jossey-Bass.
Suskie, L. (2017). “Rubric development,” in Handbook On Measurement, Assessment and Evaluation in Higher Education, 2nd Edn., eds C. Secolsky and D. B. Denison, (London: Routledge), 545–559. doi: 10.4324/9781315709307-43
Suto, I., and Nadas, R. (2008). What determines GCSE marking accuracy? An exploration of expertise among maths and physics markers. Res. Papers Educ. 23, 477–497. doi: 10.1080/02671520701755499
Torrance, H. (2007). Assessment as learning? How the use of explicit learning objectives, assessment criteria and feedback in post-secondary education and training can come to dominate learning. Assess. Educ. 14, 281–294. doi: 10.1080/09695940701591867
Utaberta, N., and Hassanpour, B. (2012). Aligning assessment with learning outcomes. Soc. Behav. Sci. 60, 228–235. doi: 10.1016/j.sbspro.2012.09.372
Woolf, H. (2004). Assessment criteria: reflections on current practices. Assess. Eval. High. Educ. 29, 479–493. doi: 10.1080/02602930310001689046
Keywords: analytic, holistic, judgement, marks, rubrics, criteria, weightings, validity
Citation: Tomas C, Whitt E, Lavelle-Hill R and Severn K (2019) Modeling Holistic Marks With Analytic Rubrics. Front. Educ. 4:89. doi: 10.3389/feduc.2019.00089
Received: 17 February 2019; Accepted: 05 August 2019;
Published: 18 September 2019.
Edited by:
Anders Jönsson, Kristianstad University, SwedenReviewed by:
Susan M. Brookhart, Duquesne University, United StatesPhillip Dawson, Deakin University, Australia
Copyright © 2019 Tomas, Whitt, Lavelle-Hill and Severn. This is an open-access article distributed under the terms of the Creative Commons Attribution License (CC BY). The use, distribution or reproduction in other forums is permitted, provided the original author(s) and the copyright owner(s) are credited and that the original publication in this journal is cited, in accordance with accepted academic practice. No use, distribution or reproduction is permitted which does not comply with these terms.
*Correspondence: Carmen Tomas, Y2FybWVuLnRvbWFzQG5vdHRpbmdoYW0uYWMudWs=