- 1Ludwig-Maximilians-Universität München, Munich, Germany
- 2Chair of Physics Education, Ludwig-Maximilians-Universität München, Munich, Germany
Although multimedia applications can undeniably have a positive impact on the learning success of students, they are not used by all physics teachers. The study presented in this paper examines the influence of technological knowledge of physics teachers on their acceptance behavior by adding TPACK of a physics teacher, as an additional variable to an adapted technology acceptance model (TAM). In addition, the TAM has been adapted to study the acceptance of multimedia applications of physics teachers in physics education. For this purpose, both, the design features were adapted and items were reworded to adjust them to the usage of multimedia applications in school and teaching context. While the first part of the study evaluates the changes of the TAM, the second part of the study deals with the extension of the TAM by the factor TPACK. TPACK acts as a superordinate moderator variable, which has a highly significant influence on the adapted TAM's design features “perceived ease of use,” “perceived usefulness for pupils” and the “personal job relevance assessment.” Interestingly, the results of the study show that TPACK has no significant influence on the “perceived personal usefulness” of multimedia applications in physics teaching. Nevertheless, the prediction of the acceptance behavior of multimedia applications in physics teaching can be improved by extending the adapted TAM2/UTAUT model by TPACK.
Introduction
Hattie (2008) discovered in his meta-study that teachers' professional knowledge in designing appropriate lessons have a major impact on their students' performance. The current conceptualizations of teachers' professional knowledge are based on Shulman's taxonomy from 1987. Shulman distinguished mainly between the content knowledge and the pedagogical knowledge and worked out the pedagogical content knowledge as “the special amalgam of content and pedagogy” (Shulman, 1987, p. 8).
Since then, many conditions have changed. Today we live in the information age, where smartphones and other devices provide access to information anytime, anywhere. In recent years, a wide variety of information technologies has found its way into everyday life of students and teachers. To face this rapid social progress, schools have to adapt. This development can also be determined by the change in the classroom itself (Behrens and Rathgeb, 2017). Teaching is evolving from a teacher-centered lesson to a more student-centered lesson (Lee et al., 2009), which mostly requires a significantly higher level of technology use. While teachers worked almost exclusively with chalkboard, books and overhead projectors a few years ago, today they have to be able to confidently deal with tablets, smartphones, document cameras and projectors. Additionally there are innumerable software products for desktop computers and handhelds. Especially in physics, teachers need electronic measuring systems, simulations and animations to perform experiments and explain connections. For some years, smartphones and tablets, which require even more skills from a teacher, have been added as further innovative experimenting devices (Kuhn and Vogt, 2013; Vogt and Kuhn, 2013; González and González, 2016; Crompton et al., 2017; Strzys et al., 2017; Thees et al., 2017; Bano et al., 2018). All these technologies require additional skills for purposeful application in the classroom, which in turn requires knowledge of the technologies to be used.
Technological, Pedagogical and Content Knowledge (TPACK)
Koehler et al. (2014) discuss that topic by further developing the PCK theory into TPACK framework (also: Mishra and Koehler, 2006) in order to articulate and improve teachers' use of technology for teaching and learning. Since introducing the framework, researchers, and teacher educators have adopted TPACK as a tool for understanding and advancing preservice and in-service teachers' abilities to integrate technology into their instruction (Graham et al., 2009b; Baran et al., 2011; Mouza et al., 2014; Crompton et al., 2017; Claro et al., 2018).
TPACK consists of 7 different knowledge areas:
The pedagogical knowledge (PK) and the content knowledge (CK) as well as the pedagogical content knowledge (PCK) are already known from Shulman (1986). Adding the technology knowledge (TK) results in new intersections, the technology-specific content knowledge (TCK), the technological-pedagogical knowledge (TPK) and the technological pedagogical and content knowledge (TPACK). The technology knowledge (TK) in the context of integrating technologies in school includes digital technologies such as desktop PCs, laptops, tablet PCs, the Internet, and software applications. The TK also includes knowledge that enables the user to change the purpose of an existing technology to fit into a learning environment enhanced by this technology (Mishra and Koehler, 2006; Koehler and Mishra, 2009; Koehler et al., 2014). The technology-specific content knowledge (TCK) refers to knowledge about how technology can be used and what it offers to deliver new content. For example, digital animations allow students to get an idea of what is going on inside a dipole and what meaning electrons have for it (Mishra and Koehler, 2006; Koehler and Mishra, 2009; Koehler et al., 2014). The technological pedagogical knowledge (TPK) refers to the needs and constraints of technology as the enabler of different teaching methods (Mishra and Koehler, 2006; Koehler and Mishra, 2009; Koehler et al., 2014). Finally, the technological pedagogical and content knowledge (TPACK) refers to the knowledge and understanding of the interaction between CK, PK and TK when using technology in the teaching-learning process. It provides an understanding of the complexity of relationships between students, teachers, content, methods, and technologies (Mishra and Koehler, 2006; Koehler and Mishra, 2009; Koehler et al., 2014).
Although or perhaps because of the fact that there are also critical voices about TPACK (Graham, 2011), TPACK framework has evolved. Work on TPACK framework initially focused on developing a basic definition (Mishra and Koehler, 2006), explaining and interpreting the construct, and discussing the properties of TPACK and its use in different content areas. Meanwhile, TPACK has reached a second generation, focusing on using the construct in research and development projects (Thompson, 2013; Bibi and Khan, 2015; Kiray, 2016; Claro et al., 2018). In this sense, TPACK is used in this study as a tool for describing attitudes and skills of teachers. Furthermore, because of the enormous bandwidth of new technologies, this study only deals with the field of multimedia applications in physics teaching at schoolüü, manage and cross-link multimedia content, like simulations and animations of physical content, movies of physical experiments, merge content of different webpages and simple methods to check the learning success. Examples of such applications include Learning Management System (LMS) such as Moodle, Schoology, Canvas LMS or the LMS PUMA@LMU developed at the LMU Munich.
Technology Acceptance Model (TAM, TAM2/UTAUT)
One goal of modern education must be to prepare students for life in a digital world. Of course, this requires a suitable use of digital media in the classroom. The use of digital media, though, depends especially on how much teacher accept it. One way to examine the teachers' acceptance of multimedia applications is the Davis Technology Acceptance Model (TAM) (Davis, 1986, 1989; Davis et al., 1989) and its extension to the TAM2/UTAUT model (see Figure 1) (Venkatesh and Davis, 2000; Venkatesh et al., 2003). The TAM is an adaptation of the Theory of Reasoned Action (TRA) (Fishbein and Ajzen, 1975, 1977), which in turn is a theory developed in the field of social psychology that explains a person's behavior through their intentions. Therein, the intention is determined by two constructs: first, the individual attitudes toward the behavior and second the social norms describing the belief that specific individuals or a specific group would approve or disapprove of the behavior.
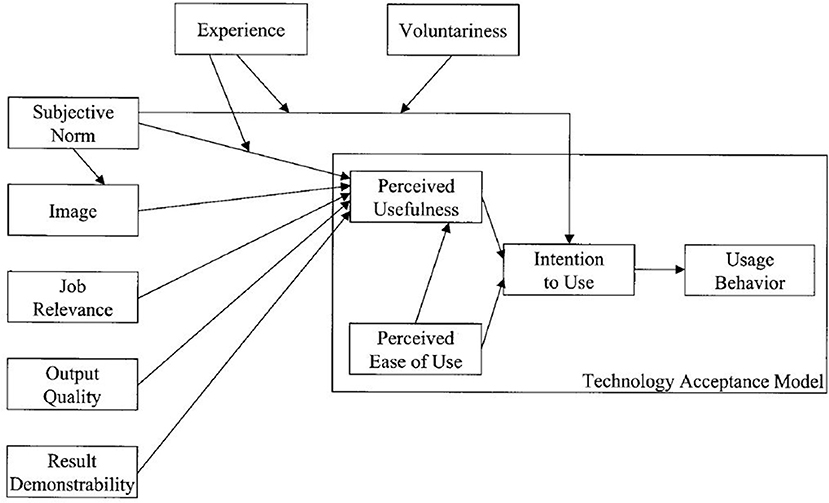
Figure 1. Original technology acceptance model (TAM2/UTAUT) (Venkatesh and Davis, 2000).
While TRA was theorized to explain general human behavior, TAM specifically explains the determinants of computer acceptance that are general and capable of explaining user behavior across a broad range of end-user computing technologies and the user population (Davis et al., 1989). The TAM originally came from the business world and was intended to be used, among other things, for researching buying behavior online as well as offline (Lin and Wang, 2005; Kulviwat et al., 2007; Thong et al., 2011) and optimizing marketing strategies as well as analyse the acceptance of systems among employees in companies until the 1990s. Meanwhile, the TAM is also used in the analysis of the acceptance of cryptocurrencies (Kumpajaya and Dhewanto, 2015), mobile or virtual payment systems (Schierz, 2008), personal computers or tablets (Venkatesh and Brown, 2001; El-Gayar et al., 2011), or the acceptance of Online Games (Hsu and Lu, 2004) and e-Learning in companies (Olbrecht, 2010; Wu et al., 2012).
In acceptance research, TAM is considered to be the best empirically evaluated and operationalized predictive model for explaining the acceptance of technical systems when describing relationships between attitudes and behavior (Legris et al., 2003; King and He, 2006; Schepers and Wetzels, 2007; Venkatesh and Bala, 2008). Davis (1989, p. 320) uses the behavioral intention as an immediate predictor of behavior. He describes that the behavioral intention (see TRA: Attitude Toward Using, ATU) expresses the intention of a person to use a relevant and possible technical system in the future. Furthermore, he assumes that the intention is influenced by two subjectively perceived and considered system dependent components, the “perceived usefulness” (PU; Davis:U) and the “perceived ease of use” (PEOU; Davis: E). These components are influenced by other variables, which Davis first calls “Design Features” (Davis, 1986, p. 24) and in his further elaborations describes them as “External Variables” (Davis, 1989; Davis et al., 1989, p. 985). External variables are interpreted differently from study to study. For example, according to Venkatesh and Davis (2000), the “perceived job relevance,” the “subjective norm,” the “output quality” or even the “result demonstrability” have to be mentioned. While the output quality describes the quality of the results of using technologies, the result demonstrability describes how tangible the results of using technologies are. The Subjective norm indicates “the person's perception that most people who are important to him think he should or should not perform the behavior in question” (Fishbein and Ajzen, 1975, p. 302). Later several different, business specific factors were added to the external variable by Venkatesh and Bala (2008).
However, according to Davis (1989), Davis et al. (1989) and Olbrecht (2010), in certain cases no influence of the subjective norm can be demonstrated. Davis explains this because the subjective norm has to be considered as a factor only if the use of technology goes along with social interactions, such as in social networks or collaborative applications. The same applies to the factor image, because “individuals often respond to social normative influences to establish or maintain a favorable image within a reference group” (Venkatesh and Davis, 2000). However, if there is no reference group, as the teacher mostly teaches alone, then this factor can be neglected, too.
The TAM and its extensions have so far not been used, in essence, to investigate the acceptance of the use of technologies in school education. The aim of this study is to investigate the acceptance behavior of teachers toward multimedia applications in physics teaching and to identify school-specific influencing factors on the acceptance behavior. So far, the TAM and its extensions examined the acceptance behavior of a specific actual user. In physics lessons, the actual user of a multimedia application is not necessarily the teacher, but it can also be the student. In the context of school, it has to be clarified whether the TAM can also be used if the actual user is often a third person and the teacher makes an assessment for the actual user.
Methods and Materials
The Participants
Idcgatekiva and Lindner (2015) showed that teachers do not fully exploit the opportunities that multimedia offers in the classroom. Instead, teachers continue to use traditional presentation methods. Among other things, Idcgatekiva and Lindner (2015) attribute this to a lack of knowledge about the possibilities of using multimedia applications in the classroom and their pedagogical impact on an individual student. In addition, the acceptance of new technologies on the part of in service teachers is also an essential prerequisite for actually using technologies in the classroom. In the different subject areas, there are often very different scenarios of how multimedia content can be used purposefully. A study designed to cover this broad spectrum naturally becomes extremely complex. To avoid this, this study was restricted to physics teachers. On the one hand, this teacher group has the advantage that there is a large number of sources of multimedia teaching materials for physics teaching worldwide, that physics teacher can use (e.g., research institutes, chairs, commercial offers, teacher content) and, secondly, there are numerous empirically examined application scenarios for a targeted use of multimedia applications (e.g., Garofalakis et al., 2013; Opfermann et al., 2017).
The adapted instrument developed for this study was applied to a total of 174 active physics teachers at high schools in the area of Munich in Germany as part of a teacher training. The resulting sample consists of 58% (101) male and 39% (68) female teachers. 5 teachers gave no indication of gender. The difference between male and female teachers roughly corresponds to the sex composition of physics teachers working in Bavaria (Germany). The teachers were aged between 24 and 65 years and had an average age of 42.4 years (SD: 11,4a). About 35% were younger than 35 years, about 48% were between 36 and 55 years old and about 17% were older than 55 years old. The average age also corresponds to the average age of the physics teachers in Bavaria (Germany). Each of the participants has completed at least nine semesters of physics studies and thus have a correspondingly good physical-technical background. The working experience of the participants is 12,9 years (SD: 10,4a).
Adaption of the TAM/UTAUT Model
The context of school therefore requires some changes to the TAM/UTAUT model. For this, the “External Variables” are adapted to the needs of the context of school and teaching. First of all, in this study the factor “subjective norm” is dispensed with, as no social interactions, such as data exchange or cooperation between the users, are intended. Other influencing factors, such as “personal job relevance assessment”, “output quality” or “result demonstrability”, on the other hand, will be used in this study. However, with regard to the context of school, the factors “output quality” and “result demonstrability” are summarized and interpreted as a new factor. This is necessary because it is extremely difficult to measure the “output quality” of students. Since it is not clear what is actually the output of a school (learning success, good grades, personal development, preparation for working life) this factor cannot be measured directly. The same applies to the verifiability of the results. Therefore, for this specific context, we bring these two factors together into a new factor, which we call “perceived usefulness for students.” This factor should be subjectively assessed by the teacher, because only the teacher is able to evaluate the success of different objectives of the lesson.
As another external factor, we propose the previously described TPACK, because in the increasingly digitized world the technology knowledge of a teacher has an increasing importance and therefore should not be neglected in the assessment of the acceptance. We assume that TPACK has a direct influence on the perceived personal usefulness. Although the TAM actually prohibits a direct effect of an external variable on another external variable, we still assume that TPACK of a teacher has a direct influence on the PEOU, the “perceived job relevance assessment,” and the “perceived usefulness to students.” This assumption is supported by the fact that TPACK, which includes technology knowledge (TK), should have a positive influence on the “perceived ease of use.” In this case, a teacher with a high TK should rate an application as easier to use than a person with a lower TK. A teacher who has a high TPACK also has a comprehensive knowledge about how to use an application specifically in the classroom. Thus a direct influence on the perceived job relevance assessment is to be expected. The same applies to the perceived usefulness to students.
The Instrument
The items for TPACK scale were adapted from different surveys of Archambault and Crippen (2009), Graham et al. (2009a) and An and Reigeluth (2014). The items for the TAM scales were adapted from a version of Venkatesh and Davis (2000) questionnaire, which were partly translated into German by Olbrecht (2010). All items have been adapted to the context of multimedia applications usage in physics teaching in schools. The survey consists of 74 items. In addition, there were 10 sociodemographic items. Each item of the survey had the following response categories: (1) I Totally agree about, (2) I agree about, (3) neither nor, (4) I do not agree (5) I do not agree at all. To test the reliability of the scales, the internal reliability coefficient Cronbach's alpha was calculated by using SPSS 25.0 software. The scale characteristics can be seen in Table 1.
Study—Part 1
Research Question and Hypotheses
It has already been shown that the TAM can be successfully applied to the usage of different technologies (e.g., Olbrecht, 2010; Evans et al., 2014; Fathema et al., 2015; Kim and Woo, 2016). In the majority of the studies using the TAM, the theoretical assumptions could be sufficiently confirmed. Nevertheless, there are no empirical findings in the literature concerning the applicability of TAM using multimedia applications in physics education or the associated effects.
To adapt the TAM to multimedia applications, all items were reworded in such a way that they are as close as possible to the original item, but the relation to multimedia applications is clearly recognizable. Because of the extensive modifications of the items and the scales, an initial test of the questionnaire is essential. This results in research question 1:
Are the model parameters as well as the model fit parameters of the modified TAM2/UTAUT model comparable to the corresponding parameters of the original TAM2/UTAUT model?
The question can only be answered, if the following hypotheses can be verified.
The perceived usefulness of multimedia applications for students have a direct impact on the perceived personal usefulness that a teacher sees in a multimedia application. High scores on the scale of the teacher's expected utility of a multimedia application result in a teacher rating the personal value of a multimedia application higher.
Results
The path model was tested using AMOS 25 with the Maximum Likelihood (ML) method. The condition for the ML method, the multivariate normal distribution, was tested and given. There was also no correlation with the age of participants or their gender.
While Figure 2 shows the adapted technology acceptance model used, as a conceptual model with the help of which the hypotheses belonging to research question 1 will be statistically tested, Figure 3 shows the result of the statistical model calculation. The appropriate fit parameters, show that the model has a good fit (CMIN/DF = 2.106; RMSEA = 0.08;CFI = 0.80) (Bentler, 1990; Browne and Cudeck, 1993; Hu and Bentler, 1999).
It could be shown that the “perceived personal usefulness” is a strong predictor of the behavioral intention (H5, β = 0.781, C.R. = 7.901, p < 0.001). The “expected personal usefulness,” is strongly influenced by the “personal job relevance assessment” (H4, β = 0.659, C.R. = 7.036, p < 0.001) and the “expected usefulness for students” (H3, β = 0.380, C.R. = 5.010, p < 0.001). It is also possible to confirm the influence of the “perceived personal usefulness” by the “perceived ease of use” (H2, β = 0.328, C.R. = 3.648, p < 0.001). The predictive effect of the behavioral intention on the acceptance behavior predicted in the UTAUT model can also be confirmed as expected (H6, β = 0.410, C.R. = 4.620, p < 0.001). Overall, the explained amount of variance in the “acceptance behavior” (AB) is R2 = 0.168 (C.R. = 5.038 p < 0.001) and in the “behavioral intention” is R2 = 0.559 (C.R. = 4.837 p < 0.001). For the “perceived personal usefulness” the explained amount of variance is R2 = 0.686 (C.R. = 3.823, p < 0.001).
However, the study showed a very interesting result in terms of the mediator property of the variable “behavioral intention.” According to Urban and Mayerl (2018) a mediator test was carried out. Similar to other studies, no mediator effect of the variable “behavior intention” can be found (see also Olbrecht, 2010; Wu and Zhang, 2014). This suggests that the variable “perceived personal usefulness” is by far the most important factor for predicting the acceptance behavior.
Study—Part 2
Framework
The modified technology acceptance model is extended by additional factors which are to be considered in school context. Politicians for example in Germany (KMK, 2016), the UK (e.g., The Scottish Government, 2016) or the US (South and Stevens, 2017) have therefore paved the way for schools by providing guidelines for education in a digital world that teachers will need to take even more into account in the future. But this also increases the demands on a teacher, especially in the technical field. At the same time, numerous studies show reasons why modern information technologies are not or only rarely used in teaching: These studies include, for example, institutional frameworks (curriculum) (Mumtaz, 2006; Keengwe et al., 2008), technical frameworks (equipment) (Mumtaz, 2006; Panda and Mishra, 2007; Keengwe et al., 2008), perceived lack of success through the use of technologies (Haßler et al., 2016), or insufficient dissemination and continuing education of teachers (Koc and Bakir, 2010), which may adversely affect the acceptance of such technologies on the part of teachers. The mentioned factors at the beginning can only be influenced marginally from a pedagogical point of view and from the teachers perspective.
However, a teacher also needs a broad understanding of technologies and how these technologies can be used in teaching (Mishra and Koehler, 2006; Koehler and Mishra, 2009; Koehler et al., 2014). Thus, a teacher's TPACK plays a key role in the integration of new technologies, and multimedia applications in particular. However, this aspect is not taken into account in the current research on the technology acceptance model, which is why the modified technology acceptance model (basic model) has been expanded by the factor TPACK within this study.
Research Question and Hypotheses
With the accompanying changes to the basic model, another research question can now be deduced:
Can the model parameters of the Technology Acceptance Model (TAM2/UTAUT) be improved in its predictive power for the usage of multimedia applications in physics teaching by adding TPACK and adapting it to the school context?
This question can only be answered with yes if the following hypotheses can be verified (see Figure 4).
Results
The extended TAM2/UTAUT model continues to take into account the prevailing conditions according to which the factors “perceived ease of use” and “perceived personal usefulness” have no direct influence on the acceptance behavior, so that according to Davis (1989) the “acceptance behavior” is directly predicted (mediated) by the “behavioral intention.”
Figure 5 shows the result of the statistical model calculation and the appropriate fit parameters show that the model has a good fit (CMIN/DF = 1.92; RMSEA = 0.073;CFI = 0.79) (Bentler, 1990; Browne and Cudeck, 1993; Hu and Bentler, 1999).
Overall, it can be shown that TPACK has a very strong influence on the “perceived ease of use” of multimedia applications (H10, β = 0.761, C.R. = 3.962, p < 0.001). This means that if TPACK increases by one standard deviation, the “perceived personal usefulness” increases by 76% of the standard deviation. Likewise, TPACK has a strong impact on the teacher's “perceived usefulness of multimedia applications for students” (H8, β = 0.471, C.R. = 3.780, p < 0.001). The influence of TPACK on “personal job relevance assessment” is also highly significant (H9, β = 0.612, C.R. = 4, 263, p < 0.001). Only the influence of TPACK on the “perceived personal usefulness” (H7, β = 0.142, C.R. = 1.019) is not significant and has a low charge as well.
It can also be stated that the “perceived personal usefulness” is a very strong predictor of the behavioral intention (β = 0.845, C.R. = 7.923, p < 0.001). The “perceived personal usefulness” is strongly influenced (β = 0.552, C.R. = 5.839, p < 0.001) by the “personal job relevance assessment” and the “perceived usefulness to students” (β = 0.306, C.R. = 4.214, p < 0.001). Finally the “behavioral intention” strongly influences the “Acceptance Behavior” (β = 0.443, C.R. = 5.118, p < 0.001).
The variance explanation of the acceptance behavior lies at R2 = 0.20 (p < 0.001) and for the “perceived personal usefulness” at R2 = 0.78 (p < 0.001). While the variance analysis of the “acceptance behavior” is about the value of the adapted TAM2/UTAUT model (R2 = 0.17), it increases in “perceived personal usefulness” by 9 to 78%. Since there are no essential changes compared to study 1, this means that hypothesis 12 can also be accepted.
However, a mediator test showed that the factor “behavioral intention” cannot be considered as a mediator in the sense of Davis (1989) as required in hypothesis 11. Because the path BI → AB decreases to a not significant β value while the additional path PPU → AB have a significant regression weight (β = 0.864, C.R. = 5.018, p < 0.001). However, the second additional path PEOU→AB is not significant.
Discussion
In this study, the hypotheses of the research questions on the modified UTAUT model and its extension by the influence factor TPACK were investigated.
Study 1 was dedicated to test the adaption of the UTAUT model to multimedia applications. The hypotheses describe the individual interactions in order to adapt the technology acceptance model to multimedia applications in schools and to conceptualize them as a structural model. The assumptions were verified by a set of structural equation models computed using IBM AMOS 25 with the Maximum Likelihood (ML) method.
With this adapted technology acceptance model, the theoretically expected causal relationships can be shown. The correlations of the TAM2/UTAUT model (PPU→BI:β = 0.78 cf. β = 0.55; PEOU→BI:β = 0.14 cf. β = 0.17 BI→AB:β = 0.41 cf. β = 0.52) are similar to Venkatesh and Davis (2000, pp. 196–197). Also the R2 coincides well with this previous studies (PPU:R2 = 69% cf. R2 = 60%; BI:R2 = 56% cf 37% < R2 < 52%). Only the R2 of the Acceptance Behavior (AB) is outside the range (R2 = 17% cf 44% < R2 < 57%) of Venkatesh and Davis (2000, pp. 196–197). All in all the measurements indicate that the adapted model fits well with the survey instrument and can be used for further investigation. Also, the question of the applicability of the TAM can be answered positively if a third person (teacher) has made an assessment for the direct user (student). However, it should be noted that the “acceptance behavior” must have other influencing factors that have not been taken into account in study 1. These aspects have to be considered in future studies. The results also matches with numerous previous studies (e.g., Davis, 1989; Venkatesh and Davis, 2000; Venkatesh et al., 2007; Wu and Zhang, 2014). Furthermore these results are consistent with the results of the study by Joo et al. (2018). Thus among preservice teachers, TPACK has an impact on the “perceived usefulness” of multimedia applications.
The one exception is the influence of the “perceived ease of use” of a multimedia application on the “behavioral intention,” as intended by hypothesis 1. As in other studies, no significant correlation could be found (see also Davis et al., 1992; Horton et al., 2001; Olbrecht, 2010). This initially appears as a strange result. But when looking at the participants, some reasons can be mentioned. The participants of the study are only physics teachers, and it should be noted that physics teachers are a special group of teachers. Because of their physical-technical studies, physics teachers have a deeper understanding of modern technologies and are able to understand the different possibilities modern technologies can provide them with. Furthermore, physics teachers often have to work with less intuitive but partly excellent software packages for school purposes, such as “Cassy Lab” by Leybold Didactic in Europe or by Klinger Educational Products in the US. It seems that the considered population of teachers do not attribute too much importance to the usability of an application. Instead, physics teachers also take advantage of applications that seem to be less easy to use. This can explain the insignificant relationship between the “perceived ease of use” and the “behavioral intention.”
Study 2 shows that the adaptation and extension of the UTAUT model by the factor TPACK is suitable for investigating the “acceptance behavior” of multimedia applications.
Unlike the suggestion of Davis et al. (1992), the results show that the variable TPACK as an external variable has no direct influence on the “perceived usefulness.” Instead, the results show, that TPACK stands outside Davis's suggested core frame as a superordinate moderator variable of the external variables “perceived ease of use,” “personal job relevance assessment” and “perceived usefulness for students.” Teachers with a strong TPACK have pronounced knowledge of technology and a strong pedagogical knowledge concerning the use of technology, they also have an in-depth knowledge of how to use multimedia applications in the classroom. In addition, they know which possibilities multimedia applications offer and which misconceptions can be counteracted by targeted use. Therefore, they see the benefits that students can gain from using multimedia applications in the classroom. As mentioned earlier, this study has shown that teachers who have a high TPACK rate multimedia applications as more relevant to their teaching. One possible reason for this is a better understanding of the possibilities that multimedia applications offer for targeted and, in part, student-centered instruction. So, if a teacher sees more opportunities to use a multimedia application in the classroom, this teacher will of course assess a multimedia application as more relevant to his teaching. This is further reinforced by the knowledge of how multimedia applications and the associated teaching methods can specifically counteract physic specific beliefs. The role of TPACK as a superordinate moderator variable, on the other hand, is likely to be applicable to other groups of teachers.
This model can therefore be used to investigate the effectiveness of teacher training events with regard to increasing the acceptance of multimedia applications.
However, since no mediator effect of the “behavior intention” could be shown in either of the two studies, the question arises, which meaning the “behavior intention” actually has for the acceptance of multimedia applications in the context of school. Although this result is consistent with other studies (Horton et al., 2001; Olbrecht, 2010), it is in contrast to Davis et al. (1992), who have been able to demonstrate a mediator effect. Furthermore, an interesting finding is, that when removing the BI from the model (see Figure 6), the models fit-parameters become better and about 39% of the variance of the AB can be explained. One reason for that finding could be that the items that are supposed to measure this construct were not precise enough. Another possibility would be that in the sample examined, the BI actually has by far a smaller influence on the “acceptance behavior” than expected. The second possibility should also be considered in the context of the result of the mediator test, which could serve as an indication of this possibility. Because the failed mediator test indicates, that the BI can be neglected in the context considered. However, this finding is unsatisfactory and requires further investigation of the relationship.
This study limited itself to providing evidence that the TAM could be adapted to the context of physics education in schools, to reduce the complexity of the study and to reduce the size of the survey instrument, since both would have meant that the sample of teachers would have been much smaller. Of course, this means that some influential factors, like constitutional conditions of the individual school, curricula, technical conditions of the individual schools and the available lesson time, could not be investigated. However, as these influencing factors were frequently mentioned in the commentary section, a strong influence on the “perceived personal usefulness” and the “acceptance behavior” can be expected by these factors. Therefore, in further studies, the model should be extended by these factors, although this will significantly increase the complexity of the model and the whole study.
Data Availability
The datasets generated for this study are available on request to the corresponding author.
Ethics Statement
An approval by an ethics committee was not required for this study as per applicable institutional and national guidelines and regulations and the informed consent of the participants was implied through survey completion. At this point we will refer to the submission of the Ethics Committee of the German Psychological Society. It describes general information for participants that was fully followed during the preparation and execution of this study. All the participants were informed about the following regulations in detail. The participation in the study is voluntary. Participants may terminate participation in this study at any time without giving cause or the danger of suffering from any consequences. Even if the participants cancel the study prematurely, they can participate in the lecture and the practical part. The data and personal information collected in this study will be kept confidential. The staff who have personal data through direct contact with the participants are subject to confidentiality. Furthermore, the results of the study will be published anonymously without revealing the personal data of participants. The collection of the personal data of the participants is completely anonymised, i. e., at no point a name is requested. The answers and results are stored under an anonymous token, so that personal data cannot be associated with any specific participant. The anonymous data will be stored for at least 10 years. However, subscribers can request the deletion of the data, whenever they want to. For this, the participants do not have to tell their name, but only give their token. In accordance with current practice, participants were explicitly advised that they automatically agree to these terms and conditions when participating in the study.
Author Contributions
PM carried out the study and its evaluation and wrote the essay. RG was involved in the development, execution, and evaluation of the study.
Funding
The study was conducted in the project “Lehre @ LMU”, funded by the Federal Ministry of Education and Research of Germany (BMBF).
Conflict of Interest Statement
The authors declare that the research was conducted in the absence of any commercial or financial relationships that could be construed as a potential conflict of interest.
References
An, Y. J., and Reigeluth, C. (2014). Creating technology-enhanced, learner-centered classrooms: K−12 teachers' beliefs, perceptions, barriers, and support need. J. Digit. Learn. Teach. Edu. 28, 54–62. doi: 10.1080/21532974.2011.10784681
Archambault, L., and Crippen, K. (2009). Examining TPACK among K-12 online distance educators in the United States. Technol. Teach. Edu. 9, 71–88.
Bano, M., Zowghi, D., Kearney, M., Schuck, S., and Aubusson, P. (2018). Mobile learning for science and mathematics school education: a systematic review of empirical evidence. Comput. Educ. 121, 30–58. doi: 10.1016/j.compedu.2018.02.006
Baran, E., Chuang, H.-H., and Thompson, A. (2011). TPACK: an emerging research and development tool for teacher educators. Turkish Online J. Education. Technol. 10, 370–377. Available online at: http://www.tojet.net/articles/v10i4/10437.pdf
Behrens, P., and Rathgeb, T. (2017). JIM Studie. Medienpädagogischer Forschungsverbund Südwest (mpfs) (Stuttgart).
Bentler, P. M. (1990). Comparative fit indexes in structural models. Psychol. Bull. 107:238. doi: 10.1037/0033-2909.107.2.238
Bibi, S., and Khan, S. H. (2015). TPACK in action: a study of a teacher educator's thoughts when planning to use ICT. Austral. J. Edu. Technol. 33, 70–87. doi: 10.14742/ajet.3071
Browne, M. W., and Cudeck, R. (1993). Alternative ways of assessing model fit. Sage Focus Ed. 154, 136.
Claro, M., Salinas, A., Cabello-Hutt, T., San Martín, E., Preiss, D. D., Valenzuela, S., and Jara, I. (2018). Teaching in a digital environment (TIDE): defining and measuring teachers' capacity to develop students' digital information and communication skills. Compt. Educ. 121, 162–174. doi: 10.1016/j.compedu.2018.03.001
Crompton, H., Burke, D., and Gregory, K. H. (2017). The use of mobile learning in PK-12 education: a systematic review. Comp. Educ. 110, 51–63. doi: 10.1016/j.compedu.2017.03.013
Davis, F., Bagozzi, R., and Warshaw, P. (1992). Extrinsic and intrinsic motivation to use computers in the workplace1. J. Appl. Soc. Psychol. 22, 1111–1132. doi: 10.1111/j.1559-1816.1992.tb00945.x
Davis, F. D. (1986). Technology acceptance model for empirically testing new end-user information systems: theory and results (Dissertation). Massachusetts Institute of Technology, Sloan School of Cambridge, Cambridge, MA, United States.
Davis, F. D. (1989). Perceived usefulness, perceived ease of use and user acceptance of information technology. MIS Q. 13, 319–339. doi: 10.2307/249008
Davis, F. D., Bagozzi, R. P., and Warshaw, P. R. (1989). User acceptance of computer technology: a comparison of two theoretical models. Manag. Sci. 35, 982–1003. doi: 10.1287/mnsc.35.8.982
El-Gayar, O., Moran, M., and Hawkes, M. (2011). Students' acceptance of tablet PCs and implications for educational institutions. J. Edu. Technol. Soc. 14, 58–70.
Evans, C., Raymond, H., Rauniar, R., Rawski, G., Yang, J., and Johnson, B. (2014). Technology acceptance model (TAM) and social media usage: an empirical study on Facebook. J. Enter. Informat. Manag. 27, 6–30. doi: 10.1108/JEIM-04-2012-0011
Fathema, N., Shannon, D., and Ross, M. (2015). Expanding the technology acceptance model (TAM) to examine faculty use of learning management systems (LMSs) in higher education institutions. J. Online Learn. Teach. 11, 210–232.
Fishbein, M., and Ajzen, I. (1977). Belief, Attitude, Intention, and Behavior: An Introduction to Theory and Research.
Fishbein, M., and Ajzen, I. (1975). Belief, Attitude, Intention, and Behaviour: An Introduction to Theory and Research. Reading, MA: Addison-Wesley.
Garofalakis, J. D., Lagiou, E. V., and Plessas, A. P. (2013). Use of web 2.0 tools for teaching physics in secondary education. Int. J. Informat. Edu. Technol. 3, 6–9. doi: 10.7763/IJIET.2013.V3.224
González, M., and González, M. (2016). Smartphones as experimental tools to measure acoustical and mechanical properties of vibrating rods. Eur. J. Phys. 37:45701. doi: 10.1088/0143-0807/37/4/045701
Graham, C. R. (2011). Theoretical considerations for understanding technological pedagogical content knowledge (TPACK). Compt. Edu. 57, 1953–1960. doi: 10.1016/j.compedu.2011.04.010
Graham, C. R., Burgoyne, N., Cantrell, P., Smith, L., St. Clair, L., and Harris, R. (2009a). TPACK development in science teaching: measuring the TPACK confidence of inservice science teachers measuring the TPACK confide of inservice science teachers. TeachTrends 53, 70–79. doi: 10.1007/s11528-009-0328-0
Graham, C. R., Cox, S., and Velasquez, A. (2009b). “Teaching and measuring TPACK development in two preservice teacher preparation programs,” in Proceedings of Society for Information Technology and Teacher Education International Conference 2009, eds I. Gibson, R. Weber, K. McFerrin, R. Carlsen, and D. A. Willis. (Charleston, SC: Association for the Advancement of Computing in Education (AACE), 4081–4086.
Haßler, B., Major, L., and Hennessy, S. (2016). Tablet use in schools: a critical review of the evidence for learning outcomes. J. Comp. Assist. Learn. 32, 139–156. doi: 10.1111/jcal.12123
Hattie, J. (2008). Visible Learning: A Synthesis of Over 800 Meta-Analyses Relating to Achievement. London: Routledge.
Horton, R. P., Buck, T., Waterson, P. E., and Clegg, C. W. (2001). Explaining intranet use with the technology acceptance model. J. Informat. Technol. 16, 237–249. doi: 10.1080/02683960110102407
Hsu, C. L., and Lu, H.-P. (2004). Why do people play on-line games?: an extended TAM with social influences and flow experience. Informat. Manag. 41, 853–868. doi: 10.1016/j.im.2003.08.014
Hu, L., and Bentler, P. M. (1999). Cutoff criteria for fit indexes in covariance structure analysis: Conventional criteria versus new alternatives. Struct. Eq. Model. 6, 1–55. doi: 10.1080/10705519909540118
Idcgatekiva, T., and Lindner, M. (2015). “Multimedia: a suitable tool for project-based education: A survey among czech, slovakian and german biology teachers,” in Project-Based Education in Science Education, eds M. Rusek, W. F. McComas (Rotterdam: SensePublishers), 81–87.
Joo, Y. J., Park, S., and Lim, E. (2018). Factors influencing preservice teachers' intention to use technology: TPACK, teacher self-efficacy, and technology acceptance model. Edu. Technol. Soc. 21, 48–59.
Keengwe, J., Onchwari, G., and Wachira, P. (2008). Computer technology integration and student learning: barriers and promise. J. Sci. Educ. Technol. 17:560–565. doi: 10.1007/s10956-008-9123-5
Kim, Y. G., and Woo, E. (2016). Consumer acceptance of a quick response (QR) code for the food traceability system: application of an extended technology acceptance model (TAM). Food Res. Int. 85, 266–272. doi: 10.1016/j.foodres.2016.05.002
King, W. R., and He, J. (2006). A meta-analysis of the technology acceptance model. Informat. Manag. 43, 740–755. doi: 10.1016/j.im.2006.05.003
Kiray, S. A. (2016). Development of a TPACK self-efficacy scale for preservice science teachers. Int. J. Res. Edu. Sci. 2:527. doi: 10.21890/ijres.64750
KMK (2016). Bildung in Der Digitalen Welt: Strategie der Kultusministerkonferenz. Available online at: https://www.kmk.org/fileadmin/Dateien/pdf/PresseUndAktuelles/2016/Bildung__digitale__Welt__Webversion.pdf (accessed March 15, 2019).
Koc, M., and Bakir, N. (2010). A needs assessment survey to investigate pre-service teachers' knowledge, experiences and perceptions about preparation to using educational technologies. Turk. Online J. Educ. Technol. 9, 13–22. Available online at: https://eric.ed.gov/?id=EJ875758
Koehler, J., and Mishra, P. (2009). What is technological pedagogical content knowledge? Contem. Issues Technol. Teacher Educ. 9, 60–70. Available online at: https://citejournal.s3.amazonaws.com/wp-content/uploads/2016/04/v9i1general1.pdf
Koehler, M., Mishra, P., Kereluik, K., Shin, T. S., and Graham, C. R. (2014). “The technological pedagogical content knowledge framework,”in Handbook of Research on Educational Communications and Technology, 4th ed, eds J. M. Spector, M. D. Merrill, J. Elen, and M. J. Bishop. (Dordrecht: Springer), 101–111. doi: 10.1007/978-1-4614-3185-5_9
Kuhn, J., and Vogt, P. (2013). Analyzing acoustic phenomena with a smartphone microphone. Phys. Teach. 51:118. doi: 10.1119/1.4775539
Kulviwat, S., Bruner, I. I. G. C., Kumar, A., Nasco, S. A., and Clark, T. (2007). Toward a unified theory of consumer acceptance technology. Psychol. Market. 24, 1059–1084. doi: 10.1002/mar.20196
Kumpajaya, A., and Dhewanto, W. (2015). The acceptance of bitcoin in indonesia: extended TAM with IDT. J. Bus. Manag. 4, 28–38. Available online at: http://journal.sbm.itb.ac.id/index.php/jbm/article/download/1385/821
Lee, B.-C., Yoon, J.-O., and Lee, I. (2009). Learners' acceptance of e-learning in South Korea: theories and results. Comput. Edu. 53, 1320–1329. doi: 10.1016/j.compedu.2009.06.014
Legris, P., Ingham, J., and Collerette, P. (2003). Why do people use information technology?: a critical review of the technology acceptance model. Informat. Manag. 40, 191–204. doi: 10.1016/S0378-7206(01)00143-4
Lin, H.-H., and Wang, Y.-S. (2005). “Predicting consumer intention to use mobile commerce in taiwan,” in International Conference on Mobile Business, 2005: ICMB 2005; 11 - 13 July 2005, [Crowne Plaza Darling Harbour, Sydney, Australia], ed W. Brookes (Los Alamitos, Calif.: IEEE Computer Society, 406–412.
Mishra, P., and Koehler, J. (2006). Technological pedagogical content knowledge: a framework for teacher knowledge. Teach. Coll. Record 108, 1017–1054 doi: 10.1111/j.1467-9620.2006.00684.x
Mouza, C., Karchmer-Klein, R., Nandakumar, R., Ozden, S. Y., and Hu, L. (2014). Investigating the impact of an integrated approach to the development of preservice teachers' technological pedagogical content knowledge (TPACK). Compt. Educat. 71, 206–221. doi: 10.1016/j.compedu.2013.09.020
Mumtaz, S. (2006). Factors affecting teachers' use of information and communications technology: a review of the literature. J. Informat. Technol. Teach. Edu. 9, 319–342. doi: 10.1080/14759390000200096
Olbrecht, T. (2010). Akzeptanz von E-Learning: Eine Auseinandersetzung mit dem Technologieakzeptanzmodell zur Analyse individueller und sozialer Einflussfaktoren. Available online at: http://www.db-thueringen.de/servlets/DerivateServlet/Derivate-21996/Olbrecht/Dissertation.pdf (accessed February 10, 2019).
Opfermann, M., Schmeck, A., and Fischer, H. E. (2017). “Multiple representations in physics and science education-why should we use them?,” in Multiple Representations in Physics Education, eds D. Treagust, R. Duit, and H. Fischer (Cham: Springer), 1–22. doi: 10.1007/978-3-319-58914-5_1
Panda, S., and Mishra, S. (2007). E-learning in a mega open university: faculty attitude, barriers and motivators. Edu. Media Int. 44, 323–338. doi: 10.1080/09523980701680854
Schepers, J., and Wetzels, M. (2007). A meta-analysis of the technology acceptance model: investigating subjective norm and moderation effects. Informat. Manag. 44, 90–103. doi: 10.1016/j.im.2006.10.007
Schierz, P. G. (2008). Akzeptanz von Mobilen Zahlungssystemen: Eine Empirische Analyse Basierend auf dem Technologieakzeptanzmodell. Kovač.
Shulman, L. (1987). Knowledge and teaching: foundations of the new reform. Harvard Educat. Rev. 57, 1–23. doi: 10.17763/haer.57.1.j463w79r56455411
Shulman, L. S. (1986). Those who understand: knowledge growth in teaching. Edu. Res. 15, 4–14. doi: 10.3102/0013189X015002004
South, J., and Stevens, K. (2017). Reimaging the Role of Technology in Education: 2017 National Eduaction Technology Plan Update. Washington, DC: U.S. Department of Education - Office of Educational Technology.
Strzys, M. P., Kapp, S., Thees, M., Kuhn, J., Lukowicz, P., Knierim, P., and Schmidt, A. (2017). Augmenting the thermal flux experiment: a mixed reality approach with the HoloLens. Phys. Teach. 55, 376–377. doi: 10.1119/1.4999739
The Scottish Government (2016). Enhancing Learning and Teaching Through the Use of Digital Technology: A Digital Learning and Teaching Strategy for Scotland (Edinburgh).
Thees, M., Hochberg, K., Kuhn, J., and Aeschlimann, M. (2017). Adaptation of acoustic model experiments of STM via smartphones and tablets. Phys. Teach. 55, 436–437. doi: 10.1119/1.5003749
Thompson, P. (2013). The digital natives as learners: Technology use patterns and approaches to learning. Comput. Edu. 65, 12–33. doi: 10.1016/j.compedu.2012.12.022
Thong, J. Y. L., Venkatesh, V., Xu, X., Hong, S.-J., and Tam, K. Y. (2011). Consumer acceptance of personal information and communication technology services. IEEE Trans. Eng. Manag. 58, 613–625. doi: 10.1109/TEM.2010.2058851
Urban, D., and Mayerl, J. (2018). Angewandte Regressions Analyse: Theorie, Technik und Praxis. Wiesbaden: Springer Fachmedien Wiesbaden.
Venkatesh, V., and Bala, H. (2008). Technology acceptance model 3 and a research agenda on interventions. Decision Sci. 39, 273–315. doi: 10.1111/j.1540-5915.2008.00192.x
Venkatesh, V., and Brown, S. A. (2001). A longitudinal investigation of personal computers in homes: Adoption determinants and emerging challenges. MIS Q. 25, 71–102. doi: 10.2307/3250959
Venkatesh, V., and Davis, F. D. (2000). A theoretical extension of the technology acceptance model: four longitudinal field studies. Manag. Sci. 46, 186–204. doi: 10.1287/mnsc.46.2.186.11926
Venkatesh, V., Davis, F. D., and Morris, M. G. (2007). Dead or alive? The development, trajectory and future of technology adoption research. J. Assoc. Inform. Syst. 8:267. doi: 10.17705/1jais.00120
Venkatesh, V., Morris, M. G., Davis, G., and Davis, F. D. (2003). User acceptance of information technology: toward a unified view. MIS Q. 27, 425–478. doi: 10.2307/30036540
Vogt, P., and Kuhn, J. (2013). Analyzing radial acceleration with a smartphone acceleration sensor. Phy. Teach. 51:182. doi: 10.1119/1.4792021
Wu, B., and Zhang, C. (2014). Empirical study on continuance intentions towards E-Learning 2.0 systems. Behav. Informat. Technol. 33, 1027–1038. doi: 10.1080/0144929X.2014.934291
Keywords: TPACK, technology-acceptance-model (TAM), multimedia applications, physics teaching, teacher education
Citation: Mayer P and Girwidz R (2019) Physics Teachers' Acceptance of Multimedia Applications—Adaptation of the Technology Acceptance Model to Investigate the Influence of TPACK on Physics Teachers' Acceptance Behavior of Multimedia Applications. Front. Educ. 4:73. doi: 10.3389/feduc.2019.00073
Received: 20 April 2019; Accepted: 08 July 2019;
Published: 31 July 2019.
Edited by:
Gary James Harfitt, The University of Hong Kong, Hong KongReviewed by:
Tracy X. P. Zou, The University of Hong Kong, Hong KongKaterina Plakitsi, University of Ioannina, Greece
Copyright © 2019 Mayer and Girwidz. This is an open-access article distributed under the terms of the Creative Commons Attribution License (CC BY). The use, distribution or reproduction in other forums is permitted, provided the original author(s) and the copyright owner(s) are credited and that the original publication in this journal is cited, in accordance with accepted academic practice. No use, distribution or reproduction is permitted which does not comply with these terms.
*Correspondence: Peter Mayer, cGUubWF5ZXJAbG11LmRl