- 1Faculty of Education, Centre for Research in Applied Measurement and Evaluation, University of Alberta, Edmonton, AB, Canada
- 2Department of Educational Psychology, University of Alberta, Edmonton, AB, Canada
Personalized feedback generated via digital score reports is essential in higher education due to the important role of feedback in teaching and learning as well as in improving students' performance and motivation in such educational settings. This study introduces and evaluates ExamVis, a novel and engaging digital score reporting system that delivers personalized feedback to students after they complete their exams. It also presents the results of two empirical studies employing hierarchical linear regressions in which 776 pre-service teachers took two midterms and a final exam, receiving a digital score report with personalized feedback after each exam and having the option to review each of the score reports. Study 1 found that reviewing short reports (with visual feedback given immediately after an exam) and extended reports (with visual and written feedback given after all students completed the exam) significantly predicted students' final exam scores above and beyond their midterm exam scores. Study 2 found that students who were provided with only extended digital score reports outperformed on the final exam those who had access to both short and extended digital score reports. Implications for designing digital score reports with personalized feedback that have a positive impact on student performance are discussed.
Introduction
Feedback is one of the most important catalysts for student learning in higher education (Bailey and Garner, 2010; Hattie and Gan, 2011; Evans, 2013). The role of feedback is to inform learning by providing students with information that addresses the gap between their current and their desired performance (i.e., the fulfillment of the intended learning outcomes; Hattie and Timperley, 2007). More specifically, feedback represents timely and specific dialogic information about student learning for improving the effectiveness of instruction and promoting student learning (Shute, 2008; Goodwin and Miller, 2012; Zenisky and Hambleton, 2012). Feedback is often considered an essential component of both summative and formative assessments, with the aim to support and to monitor learning and to provide ongoing confirmatory or disconfirmatory information to learners, whilst measuring the effects of feedback. Feedback in these environments can also support instructors in dynamically adjusting and improving their teaching (Bennett, 2011). Several positive outcomes have been associated with feedback, including academic achievement, increased motivation, directing future learning, and identifying students who require support (Kluger and DeNisi, 1996; Van der Kleij et al., 2011).
Despite the benefits of formative feedback, researchers have continued to voice serious concerns about its actual impact in practice (Price et al., 2010; Carless et al., 2011). For example, one aspect that exacerbates the issue of providing timely and specific feedback to students is scalability. This occurs not only in traditional classrooms with large numbers of students, especially in higher education settings (Higgins et al., 2002), but also in increasingly prevalent massive open online courses (MOOCs). The necessity of providing all students with timely and specific feedback leads to a significant increase in the time and effort spent by instructors on creating and providing feedback tailored to the needs of hundreds or thousands of students. Therefore, it is essential to automate the process of creation and delivery of feedback to students, while balancing the amount of time and effort spent by instructors and other academic staff. A promising solution for this automation problem is employing automatic feedback tools, such as computer-based testing (CBT) and other types of digital assessments (Eom et al., 2006). CBT denotes a computerized delivery of an assessment where students respond to either selected-response items (e.g., multiple-choice items) or constructed-response items (e.g., short-answer items, essays) using a computer or a similar device (e.g., tablet). Research examining the impact of automatically-generated feedback in CBT environments found that the electronic type of delivery significantly improves students' perception of the constructiveness of the feedback (Hattie and Timperley, 2007; Crook et al., 2012; Bayerlein, 2014;Henderson and Phillips, 2014).
Despite the advantages of CBTs in delivering assessments effectively, designing effective CBTs with automatically-generated feedback still remains to be a challenge in practice. To date, several guidelines that inform the design of CBTs with feedback have been proposed, some for integrating informal tutoring feedback (Narciss and Huth, 2004) and some for integrating formative feedback (Shute, 2008). Although the relevant research literature points to general guidelines for designing effective score reports for students in K-12 educational contexts (Goodman and Hambleton, 2004; Ryan, 2006; Roberts and Gierl, 2010, 2011; Zenisky and Hambleton, 2012, 2013), there is a paucity of such guidelines for higher education. Moreover, there is a lack of empirical studies that address this gap adequately in the literature. Another important challenge in CBT-based score reporting is the communication of assessment results that facilitates meaningful interpretation with the aim to improve the validity of assessments (Cohen and Wollack, 2006, p. 380; Ryan, 2006; Huhta, 2013; AERA, 2014). Previous research has already shown that there is large variability in the delivery and communication of assessment results to students (Knupp and Ainsley, 2008; Zenisky and Hambleton, 2015). Finally, students' capacity for self-regulated learning, including their ability to use feedback information and to reflect on it to improve their behavior and performance as well as their ability to assess their own performance, can also influence the effectiveness of feedback (Butler and Winne, 1995; Chung and Yuen, 2011).
To address the issues highlighted above, this study aims to employ a 3-fold strategy: (1) characterizing feedback generated via digital score reports at a large scale in a university classroom, (2) designing a novel and engaging interactive way of delivering feedback integrated in digital score reports, and (3) evaluating the utility of the proposed feedback delivery on students' learning outcomes. This study addresses the following research questions: (1) Are students interested in reviewing the feedback presented in digital score reports? (2) Is reviewing the feedback in digital score reports associated with better achievement outcomes in the course? To address these research questions, two empirical studies were designed and implemented with a large sample of undergraduate students who received feedback on their exam performance via an interactive score reporting system. The following section describes the theoretical framework that guided the design and implementation of the aforementioned score reporting system.
Theoretical Framework
Feedback and Student Learning
Feedback is often considered one of the important catalysts for student learning (Hattie and Gan, 2011). Feedback has been associated with a series of positive learning and instructional outcomes. First, feedback is associated with higher academic achievement (Gikandi et al., 2011; Falchikov, 2013). Previous research reveals that feedback can improve students' course grades with gains ranging from one half to one full grade (Hwang and Chang, 2011; Popham, 2011) to some of the largest learning gains reported for educational interventions (Earl, 2013, p.25; Vonderwell and Boboc, 2013). Second, feedback is associated with increased levels of motivation among students (Shute, 2008; Harlen, 2013; Ellegaard et al., 2018; Dawson et al., 2019). Third, feedback has been linked to remedial activities, such as directing future learning and identifying students who require additional support (Tett et al., 2012; Merry et al., 2013).
However, in higher education, considerable variability in the quantity, quality, and timing of feedback still remains to be a major concern, especially for large-size undergraduate courses (Bailey and Garner, 2010; Van der Kleij et al., 2012; Tucker, 2015). Even after feedback has been carefully assembled, another concern is the development of student feedback literacy to facilitate uptake of the feedback students receive. Recent research on student feedback literacy has emphasized the importance of students' capacity to understand feedback information and their attitudes toward feedback to improve their learning (Goodman and Hambleton, 2004; Trout and Hyde, 2006; Carless and Boud, 2018). For example, Carless and Boud (2018) distinguished four features underlying students' feedback literacy: appreciating feedback, making judgments, managing affect, and taking action. Also, in an inductive thematic analysis study, both university students and academic staff perceived feedback as useful for improving academic outcomes (Dawson et al., 2019). Particularly, students valued high-quality feedback the most, including feedback that was specific, detailed, non-threatening, and personalized to their work.
Computer-Based Testing
Computer-based testing is one of the growing technological capabilities to improve the quality and effectiveness of educational assessments. In a CBT platform, students complete their assessments at workstations (e.g., desktop computers, laptops, or tablet devices) so that their answers can be automatically marked by a scoring engine set up for the CBT platform. Davey (2011) describes the three basic reasons for the necessity of testing on computers as improved testing capabilities for measuring complex constructs or skills (e.g., reasoning, creativity, and comprehension), higher measurement precision and test security, and operational convenience for students, instructors, and those who use test scores such as immediate scoring, flexible scheduling, and simultaneous testing across multiple locations.
As an assessment tool, CBT offers several other advantages over traditional (i.e., pen-and-pencil) assessments that make most researchers and practitioners consider it state-of-the-art (e.g., Parshall et al., 2002; Gierl et al., 2018). First, students often benefit significantly from increased access and flexibility of CBT by choosing their own exam times or locations that are most convenient to them. Second, CBT permits testing on-demand thereby allowing students to take the test at any time during instruction. Testing on-demand also ensures that students can take tests whenever they or their instructor believe feedback is required. Third, students' performance on CBT administrations can be archived easily, which would allow students to easily review previous test results and feedback. Fourth, CBT decreases the time instructors need to spend on proctoring and scoring their students' exams and can help instructors make more timely decisions about their students' performance and, perhaps, adjust their subsequent instruction.
In addition to the advantages highlighted above, CBT also provides a promising solution to enhance the quality and quantity of feedback delivered to students (Smits et al., 2008; Wang, 2011). CBT can be used for generating personalized score reports, which can be available to students immediately upon conclusion of their assessment (Lopez, 2009; Van der Kleij et al., 2012; Timmers, 2013) and thereby making an instant impact on students' learning (Davey, 2011). In traditional pen-and-pencil testing, score reports present students' performance summary as scores or grades sometimes accompanied by a cursory description of the test and of the meaning of the grades. However, score reports can be delivered in an electronic (i.e., digital) format, especially if they originate from CBT (Huhta, 2013). Such reports can include interactive elements (e.g., visualizations and tables) that could engage students in interpreting and understanding their feedback. The next section describes the characteristics of digital score reports generated from CBT.
Digital Score Reports
The relevant research literature indicates that digital score reports can enhance the effectiveness of educational assessment in several ways. First, digital score reports provide immediate score reporting and analysis to instructors for all the students taking the exam using a computer. Second, digital score reports can be easily customizable and automated. Previous research indicates that personalized feedback delivered via CBT score reports enhances learning outcomes and positive attitudes regarding feedback (Goodman, 2013; Van der Kleij, 2013). Third, an aspect that was highlighted in the related literature is the recommendation to employ multiple modes of presentation of score reports, as redundancy was found to be beneficial to reaching different audiences (Zenisky and Hambleton, 2015). Digital score reports can present the assessment information in multiple formats, depending on the instructor's preferences. They may include interactive visualizations and tables that present multiple advantages over static text, rendering the information conveyed more readable and interpretable (Huhta, 2013). For instance, presenting both text and graphical representations of the same text can increase understanding of the test results.
Moreover, graphical score summaries are usually more readily comprehensible than tables of numbers (Zenisky and Hambleton, 2013, 2015; Zwick et al., 2014). Interactivity will not only support the delivery of high quality and customized feedback to students but it will also engage students in the interpretation and better understanding of their own performance, with its strengths and weaknesses (Hattie and Brown, 2008; Hattie, 2009; O'Malley et al., 2013). This deeper understanding may lead to more concrete actions that students can take to address areas that they need to improve. Finally, digital score reports are in themselves valuable tools for self-reflection for both instructors and students. Specifically, students can use these reports as self-assessment tools that can identify strengths, weaknesses, and areas of improvement, which enhance students' self-directed learning. Moreover, researchers have pointed out that users, particularly teachers, do not always interpret correctly the results of these reports (Van der Kleij and Eggen, 2013) and that facilitating interpretation of assessment results is also important from the perspective of test score validity (Hattie, 2009; Van der Kleij et al., 2014).
The design of the novel digital score reporting system presented in this research study draws on similar frameworks suggested by recent studies on score reporting (e.g., Zapata-Rivera et al., 2012; Zenisky and Hambleton, 2015) and it is guided by the methodologies used in the following areas: assessment design (Wise and Plake, 2015), formative feedback in higher education (Evans, 2013), software engineering (Tchounikine, 2011), and human-computer interaction (Preece et al., 2015). The digital nature of score reports presents several advantages, including the generation of feedback that is immediate, ubiquitous, tailored to the level of the learner, confidentially assigned by a computer rather than a human, and automated for a large sample of learners. For instance, in an empirical study with 3,600 students enrolled in a MOOC, a digital assessment platform called PeerStudio was used to allow students to provide each other with rapid peer feedback on their in-progress work. The results showed that rapid peer feedback had a significant impact on students' final grades only when administered within 24 h of completing a task (Kulkarni et al., 2015). One study sampling 464 college students found that, although detailed feedback had a positive effect on students' performance, the perceived source of the feedback (i.e., computers vs. humans) had no impact on the results (Lipnevich and Smith, 2009).
Delivery of digital score reports also enables the collection of data traces of learners' interaction with the feedback provided or patterns of visualization of the results. Thus, the digital score report system employed in the present study also draws on research regarding formative feedback (Shute, 2008), informal tutoring feedback (Narciss and Huth, 2004), and automated feedback (Van der Kleij et al., 2013). One of the reasons for integrating feedback in the digital score reports sent to the learners after their exams is that feedback in formative computer-based assessments was found to have positive effects on learners' performance (Van der Kleij et al., 2011). Although feedback incorporated in digital environments does not equate instant uptake, there is evidence that feedback is more readily sought when it is administered by a computer (Aleven et al., 2003).
Methods
Sample
The sample of this study consisted of 776 pre-service teachers (64% female, 36% male), with an average age of 24.7 (SD = 5.3), from a western Canadian university. All of the students were pre-service teachers from the Elementary Education (n = 473) and Secondary Education (n = 303) programs who were enrolled in an educational assessment course during the 2017–2018 academic year (only Winter 2018; n = 523) and the 2018–2019 academic year (both Fall 2018 and Winter 2019; n = 253). There were five sections of the course in Winter 2018 and two sections in Fall 2018 and Winter 2019. The number of students enrolled in each section was very similar (roughly 100 students per section). Each instructor followed the same course materials, provided the same homework assignments, and administered the same midterm and final exams to the students across the three terms mentioned above. The students completed two midterm exams and a final exam at a CBT center on campus. Data collection for this study was carried out in accordance with the recommendations of the Research Ethics Office and the protocol (Pro00072612) was approved by the Research Ethics Board 2 at the University of Alberta. All participating students provided the research team with online informed consent in accordance with the Declaration of Helsinki.
Procedures
Score Reporting
To provide students with feedback on their performance, we created a score reporting system, called ExamVis, which is essentially a web application integrated with the TAO assessment delivery platform (Open Assessment Technologies, 2016). To produce a score report using ExamVis, the instructor needs to define a test blueprint in three steps. Figure 1 demonstrates an example of feedback phrases by lecture. First, the instructor defines the content categories based on the material covered in the lectures. Second, the instructor identifies the key concepts measured by each item on the test. The key concept refers to a specific content knowledge that students need to have in order to answer the item correctly. The purpose of identifying key concepts is to provide students with item-level feedback in their score reports, without unveiling the entire item content for test security purposes. Third, the instructor devises written feedback phrases for each content category based on a certain exam performance threshold. If a student's subscore is at or above 80% on the items related to Lecture 1, the student would receive positive feedback about mastering those concepts. If, however, the student's subscore on Lecture 1 is below 80%, then the student would receive constructive feedback that points out the key concepts that require further study. The instructor can set a different threshold for each content category depending on several factors, such as the number of items for each content category and the relative importance of the content categories for the exam.
Figure 2 demonstrates a flowchart of the ExamVis reporting system. ExamVis queries the TAO database that stores the items (i.e., the item bank) and sends the items to the ExamVis database where the test blueprint and items are combined. Table 1 shows a sample test blueprint that includes content categories for the items, key concepts assessed by the items, and cognitive complexity of the items based on Bloom's revised taxonomy of the cognitive domain (Anderson et al., 2001). After students finish writing the exam, ExamVis matches the test blueprint, and other exam information from its database with students' responses to the items and generates a digital score report. The report includes interactive tables and visualizations summarizing students' performance on the exam and written feedback to inform students about the concepts and content areas that they may need to study further.
Upon completion of the exam, students can see two versions of their score report: a short report immediately after they complete their exam and an extended report after all students complete the exam. The short report presents a series of tables and visualizations that summarize students' overall exam performance (i.e., raw scores and percent-correct scores) as well as their performance in each content category on the exam (i.e., subscores by content categories). The extended report presents not only the student's overall score and subscores by content categories but also the overall classroom average and the average subscores by content categories. Figure 3 demonstrates some of the visualizations and their explanations included in the extended report (see the Figure Appendix for additional visualizations). In addition to visualized feedback, extended score reports also include written feedback organized by content categories (see Figure 1) and a list of key concepts based on the items that students answered incorrectly on the exam.
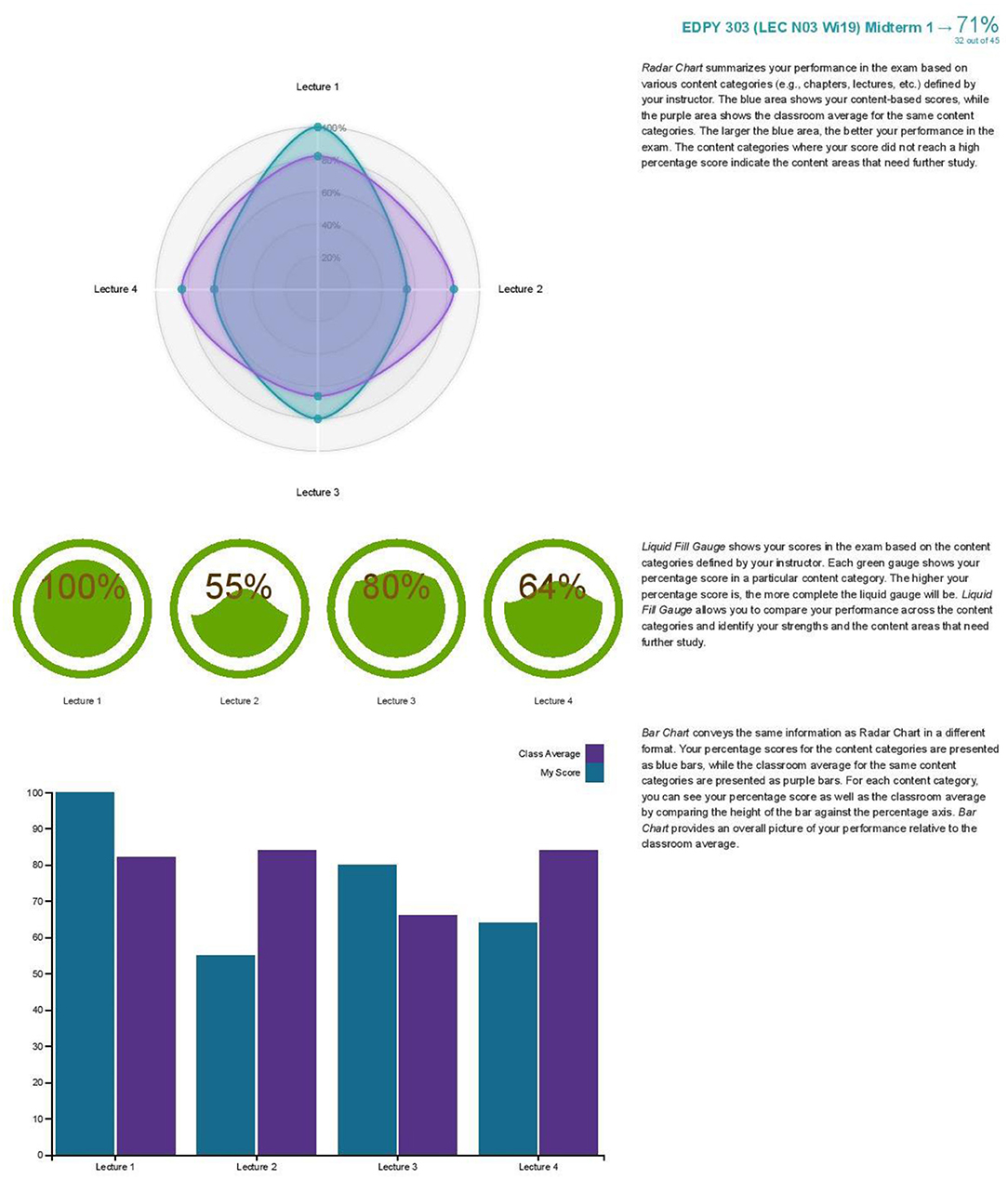
Figure 3. Examples of data visualizations generated for the extended version of the ExamVis score report.
The development of the ExamVis system was completed during the 2016–2017 academic year. The templates of short and extended score reports were created in consultation with the software development team, instructors, and students from the Faculty of Education. We received feedback from instructors regarding the usability of the ExamVis system (e.g., menu navigation on the ExamVis website, ease of setting up feedback statements for an exam). Additionally, we conducted a focus group with students to seek their feedback with regard to the interpretation of various visualizations and text in the score reports. Based on the feedback we received from instructors and students, we selected a set of interactive visualizations and finalized the templates of short and extended score reports.
ExamVis is a novel and engaging score reporting system in several ways. First, ExamVis utilizes the Data Driven Documents (D3) JavaScript library to present students' scores using a variety of visualizations. Using digital score reports generated with ExamVis, students can interpret their exam performance visually and explore the results further through interactive elements in the visualizations (e.g., enabling classroom average information on mouse click). Second, ExamVis aims to provide students with a holistic view of their performance rather than presenting item-by-item feedback that explains correct answers for each item. This approach follows the concept of mindfulness (Salomon and Globerson, 1987) that emphasizes the importance of giving feedback for the purpose of drawing new connections and constructing information structures. Learning from feedback can occur when students perceive the given information cognitively demanding. Visual and written feedback presented in ExamVis score reports does not explicitly explain students' mistakes in the items. Therefore, we believe that reviewing ExamVis score reports can encourage students to look for further details in their course materials. Third, generating score reports with ExamVis help instructors play a more active role in the design of their assessments. To utilize ExamVis for delivering visual and written feedback, instructors need to create a test blueprint where they categorize the items based on content categories and define key concepts for each item. In addition, instructors can use ExamVis to view students' overall class performance on the exams and identify content areas that require further instruction.
In sum, ExamVis is a promising tool for instructors who want to design and deliver effective feedback to students through digital score reports. To investigate the effects of the digital score reporting and feedback on student achievement, we conducted two empirical studies. The following sections describe the design and implementation of each study.
Study 1
Two primary objectives prompted Study 1. First, Study 1 aimed to helps us understand students' attitudes toward feedback and their preferences regarding the format, length, and frequency of feedback. Second, it aimed to investigate whether students' achievement would improve based on their access to feedback provided by ExamVis score reports. To address the first objective, an online survey at the beginning of the Winter 2018 academic term was administered to students. The survey consisted of several Likert-scale questions where the students were asked to indicate their attitudes or preferences regarding feedback. There were also a small number of open-ended questions where the students were asked to explain their personal views on feedback. Participation in the survey was voluntary. The overall response rate was around 50% based on valid responses to the survey questions.
To address the second objective of this study, the ExamVis system was used to generate digital score reports for students after they completed their course exams in the educational assessment course. The course instructors administered two midterm exams during the term and a final exam at the end of the term. The first midterm consisted of 45 multiple-choice questions over 90 min focusing on the content from the first six lectures of the course. The second midterm consisted of 45 multiple-choice items over 90 min focusing on the following four lectures in the course. The final exam consisted of 75 multiple-choice items over 2 h focusing on the entire course content (14 lectures in total). All of the exams were administered at a CBT center on campus. Using a flexible booking system, students were able to schedule and take the exams at the center within a 3-day exam period. All of the exams were scored using percent-correct scoring.
To organize written and visual feedback in score reports, the instructors used the lectures as content categories in the ExamVis system. That is, score reports from ExamVis included a total score based on students' overall performance on the exam and several subscores based on their performance on the content from each lecture. Furthermore, the instructors defined key concepts and written feedback statements based on the same lectures. Two methods were used for delivering score reports to students. First, upon completion of each midterm exam, all students immediately received a short score report on their screen summarizing their individual performance on the exam (i.e., percent-correct scores) via interactive visualizations. Second, once the exam period was over, students were given the option to view an extended score report that included a summary of their individual performance relative to the classroom average and personalized feedback depending on how well they performed on the items and content categories (i.e., lectures). To view extended score reports, students were asked to log in to the ExamVis website by using their username and passwords (i.e., the same username and password they use to view their university emails). Using the ExamVis website, students were able to revisit their extended score report as many times as they wanted until the end of the term.
To investigate the impact of accessing personalized feedback in the extended score reports on student achievement, we used total scores from the final exam as the dependent variable and the following variables as the independent variables: (1) students' total scores in Midterm 1; (2) students' total scores in Midterm 2; (3) whether students chose to view their extended score report for Midterm 1 (1 = Yes, 0 = No); and (4) whether students chose to view their extended score report for Midterm 2 (1 = Yes, 0 = No). We employed a hierarchical regression approach where we first used the total scores from Midterms 1 and 2 as the predictors (Model 1) and then added two additional predictors indicating whether students viewed their extended score reports for Midterm 1 and Midterm 2 (Model 2). We compared the two models to examine whether having access to the extended score reports predicted the final exam scores significantly and whether having access to the extended score reports explained additional variance in the final exam scores beyond what the scores from the two midterm exams could explain. The hierarchical regression analysis was completed using the R software program (R Core Team, 2019).
Study 2
The findings of Study 1 indicated that 30% of the students reviewed both score reports, 15% only reviewed the Midterm 1 report, and 20% only reviewed the Midterm 2 report. Despite the instructors strongly encouraging students to review the feedback in their extended score reports for Midterm 1 and Midterm 2, 35% of the students did not access either score report. The primary reason for students' low interest in accessing extended score reports was that students already had the opportunity to review their short score reports immediately after they completed each midterm. Thus, some students did not appear to be interested in reviewing their extended reports. A negative consequence of this avoidance behavior was that these students were not able to benefit from personalized and specific feedback in the extended reports, such as written feedback by lecture and a list of key concepts to study further.
To overcome this problem, Study 2 was conducted during Fall 2018 and Winter 2019, providing students only with extended score reports (i.e., eliminating the short score reports). Thus, in contrast with Study 1, in Study 2 students did not receive an immediate score report upon completing the midterm exams. Instead, once the exam period was complete, all students received their extended score reports as PDF and HTML documents via e-mail. With this application, students did not have to log in to the ExamVis website. Except for the change in score reporting, the rest of the course materials, instructional settings, and assessments were the same as those from Winter 2018. The main purpose of Study 2 was to compare students who only received extended score reports after each exam to students who had the opportunity to review both short and extended versions of score reports during Winter 2018. Therefore, we combined the exam scores from Study 2 with the exam scores from Study 1. As in the previous study, we followed a hierarchical linear regression approach where we used the percent-correct scores from Midterms 1 and 2 as the predictors of the percent-correct scores from the final exam (Model 1) and then included a new predictor identifying the reporting practice (1 = only extended report given, 0 = both short and extended reports given) in the next model (Model 2). We compared the two models to examine the impact of the reporting practice on student achievement in the course. The data analysis was again completed using the R software program (R Core Team, 2019).
Results
Study 1 Results and Discussion
The results of the survey administered at the beginning of the class indicated that over 95% of the students thought that, in general, feedback was beneficial. For example, almost all of the students either agreed or strongly agreed with feedback-related statements, such as “Feedback provides me with a sense of instructional support,” “Feedback helps me monitor my progress,” “Feedback helps me achieve the learning outcomes of the course,” and “Receiving feedback helps me learn more.” Furthermore, 97% of the students indicated that, if they were given feedback based on their performance on midterm exams, they would be willing to utilize it when studying for the final exam. One of the survey questions focused on the qualities that students think are the most important for creating effective feedback. Figure 4 shows that students consider specific feedback as the most important quality of effective feedback. In addition, students prefer to receive feedback that is future-performance oriented, positive, and detailed. This finding is aligned with previous research focusing on the qualities of feedback (e.g., Lipnevich and Smith, 2009; Wiggins, 2012; Bayerlein, 2014; Dawson et al., 2019). One question with a wide range of responses from students was about the type of feedback. The results showed that receiving feedback in the visual and table-based formats was not very appealing to students (6%). Most students preferred to receive either written feedback (30%) or feedback combining text, visualizations, and tables together (64%). An interesting finding was that half of the students seemed to prefer receiving feedback immediately after their own exam, whereas the other half preferred receiving feedback after the exam period was over for all students.
Table 2 shows the descriptive statistics for the three exams (Midterm 1, Midterm 2, and the Final Exam) by students' access to their extended score reports. The results in Table 2 indicate that 65% of the students viewed at least one of the two extended score reports, whereas 35% of the students viewed neither score report. Furthermore, students who either did not view any of their score reports or only saw the score report for Midterm 1 appeared to have lower scores in the exams than those who viewed either both score reports or only the score report for Midterm 2. This finding suggests that viewing personalized feedback in the extended report for Midterm 2 could be more effective than viewing the extended report for Midterm 1.

Table 2. Descriptive statistics for the percent-correct scores by students' access to score reports.
Table 3 shows the results of the hierarchical regression analyses where two nested regression models were compared with each other. As described earlier, Model 1 included two predictors, the percent-correct scores on Midterm 1 and Midterm 2, of the percent-correct scores on the final exam. Model 2 included the percent-correct scores from Midterm 1 and Midterm 2 as well as two additional predictors indicating whether students viewed their extended score reports for Midterm 1 and Midterm 2. In Model 1, results revealed that both Midterm 1 and Midterm 2 were statistically significant predictors of the final exam performance based on the significance level of α = 0.01 and the two predictors explained more than half (54.4%) of the total variance (R2 = 0.544) in the final exam scores. This finding is not necessarily surprising because all of the exams have been carefully designed by the course instructors over the past few years and thus scores from these exams tend to be strongly correlated with each other. Additionally, the Final Exam was cumulative, sampling all lectures, and thus, overlapping with both Midterm 1 and Midterm 2 in the concepts and content tested.
In Model 2, from the two additional predictors, only Report 2 (i.e., viewing the extended report for Midterm 2) was statistically significant with a positive regression coefficient, suggesting that viewing Report 2 had a positive impact on students' performance in the final exam. One reason for this finding is that the final exam included more items from the content areas covered in Report 2 (i.e., Lectures 5 to 8), compared to those in Report 1 (i.e., Lectures 1 to 4). In addition, we anticipate that students would become more familiar with the interpretation of the score reports after viewing the second report and thus utilize the received feedback more effectively.
Figure 5 shows the standardized regression coefficients from the two models, indicating that the effect of Report 1 was positive but quite small, whereas Report 2 had a positive and larger effect in the model. Furthermore, Model 2 was also able to explain more than half (56.3%) of the total variance (R2 = 0.563) in the final exam scores. The test of the R2 difference between the two models indicated that Model 2 with the two additional predictors accounted for significantly higher variance in the final exam scores, F(2, 518) = 11.1, p < 0.001, ΔR2 = 0.019.
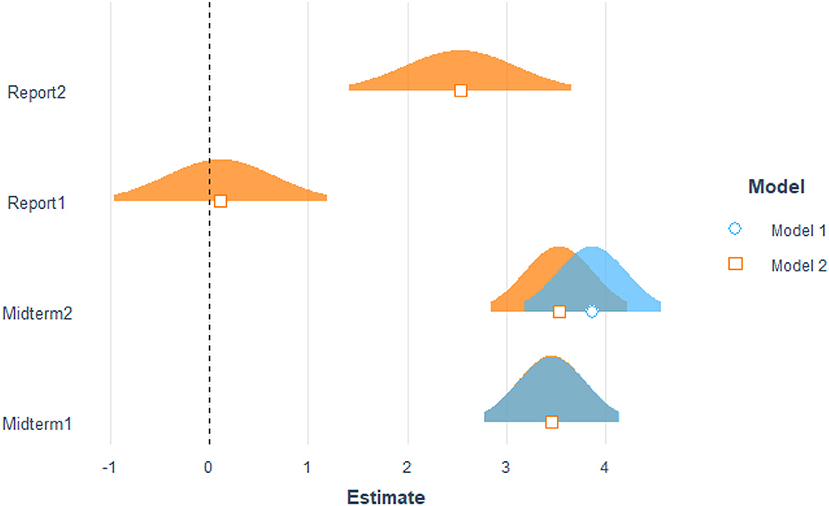
Figure 5. Standardized regression coefficients and their distributions for the four predictors across the two regression models.
The findings of the regression analyses suggest that providing students with personalized and specific feedback through the ExamVis system improved students' performance in the course. However, two problems arose with the implementation of the ExamVis system in Study 1. First, 35% of the students did not view their extended score reports, although most students had indicated in the survey that they would be willing to review feedback based on their performance on the midterm exams. Second, some students chose to view only one of the score reports, although both reports were available on the ExamVis website until the end of the term. Our discussions with the course instructors revealed that some students were not interested in viewing their extended score reports because they were able to view their results (i.e., short score report) immediately upon completion of the exams. This was an unanticipated finding because nearly all of the students who participated in the survey indicated that they would prefer written feedback or feedback that was a combination of text, visualizations, and tables. However, short score reports generated from ExamVis only included visual feedback, whereas extended score reports included both visual and written feedback summarizing students' performance on the exams.
In addition, the course instructors who participated in Study 1 suggested that providing students with a short score report immediately upon completion of the exams might have led to other unintended consequences, such as increased levels of pre-exam nervousness among some students. In both Study 1 and Study 2, the instructors utilized the flexible booking exam system where students were able to complete their exams at their chosen day and time during a 3-day exam period. However, students who completed their exams earlier during this period could theoretically share their results with other students, which in turn could negatively affect students who had not completed their exam yet. The instructors noted that, compared to previous years, they received more exam-related inquiries from students during the exam periods in Study 1, which could be a result of increased levels of pre-exam nervousness induced by students' conversations with each other regarding their exam results.
Study 2 Results and Discussion
The issues summarized above prompted a follow-up study where we could better motivate students to view their extended score reports and eliminate the negative effects of releasing exam scores immediately upon completion of the exams. Therefore, Study 2 was conducted in the same educational assessment course during the 2018–2019 academic year. As mentioned earlier, Study 2 focused on the comparison of the two different cohorts (i.e., Cohort 1: Winter 2018 vs. Cohort 2: Fall 2018 and Winter 2019). The first cohort of students (i.e., those who participated in Study 1) had access to both the short and the extended versions of their ExamVis reports, whereas the second cohort (i.e., those who participated in Study 2) had only access to the extended score reports generated by ExamVis at the end of the exam period, after all students had completed their exams. That is, students completed the midterm exams but could not view their percent-correct scores right after completing the exams. Instead, they had to wait until the end of the exam period to receive an extended score report generated by ExamVis. Furthermore, instead of asking students to log into the ExamVis website to view their reports, the score reports were automatically sent out to students' university e-mail addresses with a custom message indicating that their score reports along with additional feedback were available to review.
At the end of Study 2, we combined the results from Fall 2018 and Winter 2019 with the results of Study 1. Similar to Study 1, Study 2 also employed a hierarchical linear regression approach. Model 1 included the same predictors (i.e., total scores from Midterm 1 and 2). However, this time, Model 2 included a new binary predictor, Only Extended Report, which distinguishes students in Cohort 2 from those in Cohort 1 (i.e., Only Extended Report = 1 for Cohort 2 students; Only Extended Report = 0 for Cohort 1 students). Table 4 shows the results of the hierarchical linear regression analyses in Study 2. The percent-correct scores from Midterms 1 and 2 were statistically significant predictors of the percent-correct scores from the final exam, which confirms the corresponding Model 1 finding in Study 1. In Model 2, Only Extended Report had a significant, positive regression coefficient, indicating that students in Cohort 2 performed significantly better than those in Cohort 1. Model 2 explained 61.8% of the total variance (R2 = 0.618) in the final exam scores. The test of the R2 difference between Models 1 and 2 indicated that Model 2 accounted for a significantly higher variance in the final exam scores, F(1, 772) = 29.4, p < 0.001, ΔR2 = 0.014.
Overall, the results of Study 2 indicated that students who were provided with extended score reports with personalized feedback performed better than those who had access to both short and extended score reports. There are several possible explanations for this finding. First, students who participated in Study 2 had access to only extended score reports and they may have found it easier to access these reports sent via email rather than navigating through a web site and logging in to access their reports, if they were interested in receiving feedback on their exam performance. Second, delaying feedback until the end of the exam period prevented students from sharing their scores with other students, which might have reduced pre-exam nervousness among students, concomitantly increasing their motivation to study harder for the exam. This claim is, in part, warranted by the evidence that the instructors received no exam-related inquiries from students during the exam periods in Study 2, although they had experienced increasing numbers of exam-related inquiries in Study 1. In addition to the statistical analyses, we also had the opportunity to discuss the implications of Study 2 with the course instructors. The instructors mentioned that many students expressed, in the course evaluations at the end of the term, their appreciation of receiving detailed and personalized feedback in the extended score reports. Specifically, students appreciated personalized feedback on the content areas and key concepts and acknowledged that they benefited from the extended score reports as they studied for the final exam.
General Discussion
As universities and colleges strive to enhance student access, completion, and satisfaction, providing high-quality feedback has become an indispensable part of an effective teaching-learning environment in higher education. However, as the number of students continues to increase in university classrooms, much more staff time and effort seem necessary to produce high-quality feedback from assessments. These time constraints typically result in delayed feedback as well as student dissatisfaction about the effectiveness of assessment practices. Considering the growing technological capabilities in today's world, an important way to improve the quantity, quality, and timeliness of feedback for university students is the adoption of CBT. Score reports from computer-based tests can be used to deliver individualized, objective, and high-quality formative feedback in a timely manner.
Taken together, the results of these studies show that, when reviewed, feedback is beneficial to student performance and students generally recognize the benefits of feedback on their learning. The survey findings from Study 1 suggested that most students had a positive attitude toward feedback when they received elaborated feedback, which is aligned with the findings of previous feedback research (e.g., Van der Kleij et al., 2012). Furthermore, Study 1 indicated that students tend to pay more attention to immediate feedback than to delayed feedback, although this behavior yielded unintended consequences such as lower interest in viewing extended score reports. Clariana et al. (2000) argued that delayed feedback can yield more retention in learning than immediate feedback. The results of Study 2 appear to be aligned with this claim because students who received delayed feedback (i.e., extended score reports after the exam period was complete) outperformed students who were given both immediate feedback (i.e., short reports given immediately after the exams) and delayed feedback. Finally, the students did not seek further help from the instructors or teaching assistants when interpreting their score reports. However, almost 3% of the students still wanted to meet with their instructors and review the items that they answered incorrectly.
Our findings from both studies revealed that the score reports helped the students perform better on subsequent exams. However, a long-standing challenge in the feedback literature still remains. Even well-crafted feedback that is tailored to students' strengths and weaknesses, elaborates on deficient areas, and is administered via a computer to minimize possible harmful effects on students' self-esteem, does not translate in immediate adoption, processing, or feedback-seeking (Aleven et al., 2003; Timmers and Veldkamp, 2011). It is possible that this was a result of a complex interaction between student motivation and their experience with feedback presented in the ExamVis score reports. For instance, students may have felt demoralized by their own score on the immediate short score reports but also by the average class score information presented on the delayed score reports. Also, students were informed about the availability of their extended score reports during the week following the midterm exams. It is possible that some students may have missed the chance to view their reports right after they received these notification emails and then felt less motivated to see the reports at the end of the term. Therefore, follow-up studies are required to explore the relation between accessing the score report and student motivation.
Practical Implications
The findings of these studies have a number of practical implications for developing digital score reports with specific, customized feedback on a large scale. First, ExamVis constitutes a novel digital score reporting approach that provides feedback tailored to students' performance. It provides specific feedback linked not only to the overall threshold of performance per lecture, but also to the concepts linked with each lecture covered by the exam. The present studies show that this type of reporting has a beneficial impact on students' performance. Second, ExamVis provides both immediate and delayed feedback that is personalized at a large scale. Thus, such score reporting tools may reach thousands of students at the same time providing them with feedback customized to their performance, which would be impractical in a paper-based assessment environment. Third, as the feedback is both task-specific and administered by a computer and not a human, students can access it any time, without suffering from the stigma of a social interaction (e.g., ego threat) when negative feedback is administered. Fourth, ExamVis requires instructors to build a test blueprint that only includes individual items but also connects them to item-by-item feedback (i.e., key concepts) and domain-based feedback (i.e., content categories covered in the exam). This process helps instructors develop a better understanding of their assessments and prompts them to reflect on how students can be informed more effectively through feedback. Finally, practical applications of this study include providing item-by-item formative feedback with automatic item and rationale generation (Gierl and Bulut, 2017; Gierl and Lai, 2018).
Limitations and Future Research
The findings of this study may be somewhat limited due to several reasons. First, the sample of this study only included undergraduate students enrolled in an educational assessment course, which is a mandatory course for all pre-service teachers in the faculty of education. It could be argued that these students might have better feedback literacy than students from other faculties (e.g., engineering, nursing, or medicine) because future teachers are expected to know more about feedback and its benefits in order to be effective teachers themselves. Therefore, future research should include students from other faculties to investigate whether feedback literacy could be a mediator in explaining the association between the effects of feedback in digital score reports and student success. Second, the current study focused on generating digital score reports from three course examinations that only consisted of multiple-choice items. In the future, ExamVis will be extended to provide feedback on other item formats (e.g., short-answer items, completion items, and matching items). Third, we did not have a chance to identify whether students incorporated the feedback they received into their preparation for subsequent exams. In future studies, we will investigate this aspect. For instance, we will give students the opportunity to apply the feedback provided in the digital score reports to improve on the areas that require their utmost attention. Finally, we did not include any indirect measures, such as the students' GPA, level of motivation, or the type of mindset they endorsed, especially considering that this was a mandatory course for all students enrolled in the Faculty of Education. Future studies should consider these traits to identify whether feedback could be more effective for at-risk students, students with either low or high levels of motivation for learning, and students who endorse different types of mindset. For instance, a recent study found that pre-service students' growth mindset moderated the relation between feedback seeking and performance (Cutumisu, 2019). In spite of these limitations, this research adds to our understanding of score reporting and feedback in the context of higher education. It can also inform the development of digital score reports in higher education, with more general applications in large-scale MOOCs.
Conclusions
This study introduces ExamVis, a novel digital score reporting system that delivers immediate, specific, tailored feedback, scalable to hundreds of students. It also evaluates its effectiveness through two empirical studies. Study 1 found that providing students with immediate visual feedback and a combination of delayed visual and written feedback significantly predicted students' final exam scores above and beyond their midterm exam scores. Study 2 found that students who were provided with only delayed visual and written feedback performed better on their final exam than those who had access to both immediate visual feedback and delayed visual and written feedback. Additionally, ExamVis provides a user-friendly interface for the instructors to facilitate them in defining and linking concepts to larger categories that their students need to master in order to fulfill the goals of their class.
Data Availability
The datasets generated for this study are available on request to the corresponding author.
Author Contributions
OB designed the ExamVis system, implemented both studies, participated in data collection, conducted data analysis, and helped with manuscript preparation. MC actively participated in data collection and assisted with both data analysis and manuscript preparation. AA and DS assisted with the development of the survey instrument in Study 1, including question writing, piloting, and think-aloud protocols and helped with literature review.
Funding
This work was supported by a Social Sciences and Humanities Research Council of Canada (SSHRC) Insight Development Grant (430-2016-00039) to OB.
Conflict of Interest Statement
The authors declare that the research was conducted in the absence of any commercial or financial relationships that could be construed as a potential conflict of interest.
References
AERA APA, and NCME. (2014). Standards for Educational and Psychological Testing. Washington, DC: American Educational Research Association.
Aleven, V., Stahl, E., Schworm, S., Fischer, F., and Wallace, R. (2003). Help seeking and help design in interactive learning environments. Rev. Educ. Res. 73, 277–320. doi: 10.3102/00346543073003277
Anderson, L. W., Krathwohl, D. R., Airasian, P. W., Cruikshank, K. A., Mayer, R. E., Pintrich, P. R., et al. (2001). A Taxonomy for Learning, Teaching, and Assessing: A Revision of Bloom's Taxonomy of Educational Objectives. New York, NY: Pearson: Allyn & Bacon.
Bailey, R., and Garner, M. (2010). Is feedback in higher education assessment worth the paper it is written on? Teachers' reflections on their practices. Teach. High. Educ. 15, 187–198. doi: 10.1080/13562511003620019
Bayerlein, L. (2014). Students' feedback preferences: how do students react to timely and automatically generated assessment feedback? Assess. Eval. High. Educ. 39, 916–931. doi: 10.1080/02602938.2013.870531
Bennett, R. E. (2011). Formative assessment: a critical review. Assess. Educ. Princ. Policy Pract. 18, 5–25. doi: 10.1080/0969594X.2010.513678
Butler, D. L., and Winne, P. H. (1995). Feedback and self-regulated learning: a theoretical synthesis. Rev. Educ. Res. 65, 245–281. doi: 10.3102/00346543065003245
Carless, D., and Boud, D. (2018). The development of student feedback literacy: enabling uptake of feedback. Assess. Eval. High. Educ. 43, 1315–1325. doi: 10.1080/02602938.2018.1463354
Carless, D., Salter, D., Yang, M., and Lam, J. (2011). Developing sustainable feedback practices. Stud. High. Educ. 36, 395–407. doi: 10.1080/03075071003642449
Chung, Y. B., and Yuen, M. (2011). The role of feedback in enhancing students' self-regulation in inviting schools. J. Invit. Theory Pract. 17, 22–27.
Clariana, R. B., Wagner, D., and Murphy, L. C. R. (2000). Applying a connectionist description of feedback timing. Educ. Technol. Res. Dev. 48, 5–21. doi: 10.1007/BF02319855
Cohen, A., and Wollack, J. (2006). “Test administration, security, scoring, and reporting,” in Educational Measurement, 4th Edn, ed R. L. Brennan (Westport, CT: ACE, 355–386.
Crook, A., Mauchline, A., Mawc, S., Lawson, C., Drinkwater, R., Lundqvist, K., et al. (2012). The use of video technology for providing feedback to students: Can it enhance the feedback experience for staff and students? Comput Educ. 58, 386–396. doi: 10.1016/j.compedu.2011.08.025
Cutumisu, M. (2019). The association between critical feedback seeking and performance is moderated by growth mindset in a digital assessment game. Comput. Hum. Behav. 93, 267–278. doi: 10.1016/j.chb.2018.12.026
Davey, T. (2011). Practical Considerations in Computer-Based Testing. Retrieved from: https://www.ets.org/Media/Research/pdf/CBT-2011.pdf
Dawson, P., Henderson, M., Mahoney, P., Phillips, M., Ryan, T., Boud, D., et al. (2019). What makes for effective feedback: staff and student perspectives. Assess. Eval. High. Educ. 44, 25–36. doi: 10.1080/02602938.2018.1467877
Earl, L. M. (2013). Assessment as Learning: Using Classroom Assessment to Maximize Student Learning. Thousand Oaks, CA: Corwin Press.
Ellegaard, M., Damsgaard, L., Bruun, J., and Johannsen, B. F. (2018). Patterns in the form of formative feedback and student response. Assess. Eval. High. Educ. 43, 727–744. doi: 10.1080/02602938.2017.1403564
Eom, S. B., Wen, H. J., and Ashill, N. (2006). The determinants of students' perceived learning outcomes and satisfaction in university online education: An empirical investigation. J. Innov. Educ. 4, 215–235. doi: 10.1111/j.1540-4609.2006.00114.x
Evans, C. (2013). Making sense of assessment feedback in higher education. Rev. Educ. Res. 83, 70–120. doi: 10.3102/0034654312474350
Falchikov, N. (2013). Improving Assessment Through Student Involvement: Practical Solutions for Aiding Learning in Higher and Further Education. New York, NY: Routledge.
Gierl, M. J., and Bulut, O. (2017). “Generating rationales to support formative feedback in adaptive testing,” Paper presented at the International Association for Computerized Adaptive Testing Conference (Niigata, Japan).
Gierl, M. J., Bulut, O., and Zhang, X. (2018). “Using computerized formative testing to support personalized learning in higher education: an application of two assessment technologies,” in Digital Technologies and Instructional Design for Personalized Learning, ed R. Zheng (Hershey, PA: IGI Global), 99–119. doi: 10.4018/978-1-5225-3940-7.ch005
Gierl, M. J., and Lai, H. (2018). Using automatic item generation to create solutions and rationales for computerized formative testing. Appl. Psychol. Measurement. 42, 42–57. doi: 10.1177/0146621617726788
Gikandi, J. W., Morrow, D., and Davis, N. E. (2011). Online formative assessment in higher education: A review of the literature. Comput. Educ. 57, 2333–2351. doi: 10.1016/j.compedu.2011.06.004
Goodman, D. (2013). “Communicating student learning–one jurisdiction's efforts to change how student learning is reported,” Paper presented at the conference of the National Council on Measurement in Education (San Francisco, CA).
Goodman, D., and Hambleton, R. (2004). Student test score reports and interpretive guides: Review of current practices and suggestions for future research. Appl. Meas. Educ. 17, 145–221. doi: 10.1207/s15324818ame1702_3
Goodwin, B., and Miller, K. (2012). Research says/good feedback is targeted, specific, timely. Feedback Learn. 70, 82–83.
Harlen, W. (2013). Assessment & Inquiry-Based Science Education: Issues in Policy and Practice. Trieste: Global Network of Science Academies (IAP) Science Education Programme (SEP).
Hattie, J. (2009). Visibly Learning From Reports: The Validity of Score Reports. Available online at: http://community.dur.ac.uk/p.b.tymms/oerj/publications/4.pdf
Hattie, J., and Brown, G. T. (2008). Technology for school-based assessment and assessment for learning: development principles from New Zealand. J. Educ. Technol. Syst. 36, 189–201. doi: 10.2190/ET.36.2.g
Hattie, J., and Timperley, H. (2007). The power of feedback. Rev. Educ. Res. 77, 81–112. doi: 10.3102/003465430298487
Hattie, J. A. C., and Gan, M. (2011). “Instruction based on feedback,” in Handbook of Research on Learning and Instruction, eds R. Mayer and P. Alexander (New York, NY: Routledge), 249–271.
Henderson, M., and Phillips, M. (2014). “Technology enhanced feedback on assessment,” Paper presented at the Australian Computers in Education Conference (Adelaide, SA).
Higgins, R., Hartley, P., and Skelton, A. (2002). The conscientious consumer: reconsidering the role of assessment feedback in student learning. Stud. High. Educ. 27, 53–64. doi: 10.1080/03075070120099368
Huhta, A. (2013). “Administration, scoring, and reporting scores,” in The Companion to Language Assessment, ed A. J. Kunnan (Boston, MA: John Wiley & Sons, Inc.), 1–18. doi: 10.1002/9781118411360.wbcla035
Hwang, G.-J., and Chang, H.-F. (2011). A formative assessment-based mobile learning approach to improving the learning attitudes and achievements of students. Comput. Educ. 56, 1023–1031. doi: 10.1016/j.compedu.2010.12.002
Kluger, A. N., and DeNisi, A. (1996). The effects of feedback interventions on performance: a historical review, a meta-analysis, and a preliminary feedback intervention theory. Psychol. Bull. 119, 245–284. doi: 10.1037/0033-2909.119.2.254
Knupp, T., and Ainsley, T. (2008). “Online, state-specific assessment score reports and interpretive guides,” Paper presented at the meeting of the National Council on Measurement in Education (New York, NY).
Kulkarni, C. E., Bernstein, M. S., and Klemmer, S. R. (2015). ‘PeerStudio: rapid peer feedback emphasizes revision and improves performance,” in Proceedings of the Second (2015) ACM Conference on Learning at Scale, ed G. Kiczales (Vancouver, BC: ACM), 75–84. doi: 10.1145/2724660.2724670
Lipnevich, A. A., and Smith, J. K. (2009). Effects of differential feedback on students' examination performance. J. Exp. Psychol. Appl. 15, 319–333. doi: 10.1037/a0017841
Lopez, L. (2009). Effects of Delayed and Immediate Feedback in the Computer-Based Testing Environment (Unpublished doctoral dissertation). Terre Haute, IN: Indiana State University.
Merry, S., Price, M., Carless, D., and Taras, M. (2013). Reconceptualising Feedback in Higher Education: Developing Dialogue With Students. New York, NY: Routledge. doi: 10.4324/9780203522813
Narciss, S., and Huth, K. (2004). “How to design informative tutoring feedback for multi-media learning,” in Instructional Design for Multimedia Learning, eds H. M. Niegemann, D. Leutner, and R. Brunken (Munster, NY: Waxmann, 181–195.
O'Malley, K., Lai, E., McClarty, K., and Way, D. (2013). “Marrying formative, periodic, and summative assessments: I do,” in Informing the Practice of Teaching Using Formative and Interim Assessment: A Systems Approach, ed R. W. Lissitz (Charlotte, NC: Information Age, 145–164.
Open Assessment Technologies (2016). TAO – Testing Assisté Par Ordinateur [Computer software]. Available online at: https://userguide.taotesting.com (accessed June 17, 2019).
Parshall, C. G., Spray, J. A., Kalohn, J. C., and Davey, T. C. (2002). Practical Considerations in Computer-Based Testing. New York, NY: Springer. doi: 10.1007/978-1-4613-0083-0
Popham, W. J. (2011). Classroom Assessment: What Teachers Need to Know, 6th Edn. Boston, MA: Pearson Education, Inc.
Preece, J., Sharp, H., and Rogers, Y. (2015). Interaction Design: Beyond Human-Computer Interaction. New York, NY: John Wiley & Sons, Inc.
Price, M., Handley, K., Millar, J., and O'Donovan, B. (2010). Feedback: all that effort, but what is the effect? Assess. Eval. High. Educ. 35, 277–289. doi: 10.1080/02602930903541007
R Core Team (2019). R: A Language and Environment for Statistical Computing. Vienna: R Foundation for Statistical Computing.
Roberts, M. R., and Gierl, M. J. (2010). Developing score reports for cognitive diagnostic assessment. Educ. Meas. Issues Pract. 29, 25–38. doi: 10.1111/j.1745-3992.2010.00181.x
Roberts, M. R., and Gierl, M. J. (2011). “Developing and evaluating score reports for a diagnostic mathematics assessment,” Paper presented at the annual meeting of the American Educational Research Association (New Orleans, LA).
Ryan, J. (2006). “Practices, issues, and trends in student test score reporting,” in Handbook of Test Development, eds S. Downing and T. Haladyna (Mahwah, NJ: Erlbaum, 677–710.
Salomon, G., and Globerson, T. (1987). Skill may not be enough: the role of mindfulness in learning and transfer. Inter. J. Educ. Res. 11, 623–637. doi: 10.1016/0883-0355(87)90006-1
Shute, V. J. (2008). Focus on formative feedback. Rev. Educ. Res. 78, 153–189. doi: 10.3102/0034654307313795
Smits, M., Boon, J., Sluijsmans, D. M. A., and van Gog, T. (2008). Content and timing of feedback in a web-based learning environment: effects on learning as a function of prior knowledge. Interactive Learn. Environ. 16, 183–193. doi: 10.1080/10494820701365952
Tchounikine, P. (2011). Computer Science and Educational Software Design. New York, NY: Springer. doi: 10.1007/978-3-642-20003-8
Tett, L., Hounsell, J., Christie, H., Cree, V. E., and McCune, V. (2012). Learning from feedback? Mature students' experiences of assessment in higher education. Res. Post Compul Educ. 17, 247–260. doi: 10.1080/13596748.2011.627174
Timmers, C. F. (2013). Computer-Based Formative Assessment: Variables Influencing Feedback Behaviour (Unpublished doctoral dissertation). Enschede: University of Twente.
Timmers, C. F., and Veldkamp, B. P. (2011). Attention paid to feedback provided by a computer-based assessment for learning on information literacy. Comput. Educ. 56, 923–930. doi: 10.1016/j.compedu.2010.11.007
Trout, D. L., and Hyde, E. (2006). “Developing score reports for statewide assessments that are valued and used: Feedback from K-12 stakeholders,” Paper presented at the meeting of the American Educational Research Association (San Francisco, CA).
Tucker, B. M. (2015). The Student Voice: Using Student Feedback to Inform Quality in Higher Education (Unpublished doctoral dissertation). Perth, WA: Curtin University.
Van der Kleij, F. M. (2013). Computer-Based Feedback in Formative Assessment (Unpublished doctoral dissertation). Enschede: University of Twente,.
Van der Kleij, F. M., Eggen, T. J., and Engelen, R. J. (2014). Towards valid score reports in the Computer Program LOVS: A redesign study. Stud. Educ. Eval. 43, 24–39. doi: 10.1016/j.stueduc.2014.04.004
Van der Kleij, F. M., and Eggen, T. J. H. M. (2013). Interpretation of the score reports from the Computer Program LOVS by teachers, internal support teachers and principals. Stud. Educ. Eval. 39, 144–152. doi: 10.1016/j.stueduc.2013.04.002
Van der Kleij, F. M., Eggen, T. J. H. M., Timmers, C. F., and Veldkamp, B. P. (2012). Effects of feedback in a computer-based assessment for learning. Comput. Educ. 58, 263–272. doi: 10.1016/j.compedu.2011.07.020
Van der Kleij, F. M., Feskens, R., and Eggen, T. J. H. M. (2013). Effects of Feedback in Computer-Based Learning Environment on Students' Learning Outcomes: A Meta-Analysis. San Francisco, CA: NCME.
Van der Kleij, F. M., Timmers, C. F., and Eggen, T. J. H. M. (2011). The effectiveness of methods for providing written feedback through a computer-based assessment for learning: a systematic review. CADMO 19, 21–39. doi: 10.3280/CAD2011-001004
Vonderwell, S., and Boboc, M. (2013). Promoting formative assessment in online teaching and learning. TechTrends Link. Res. Pract. Improve Learn. 57, 22–27. doi: 10.1007/s11528-013-0673-x
Wang, T. (2011). Implementation of web-based dynamic assessment in facilitating junior high school students to learn mathematics. Comput. Educ. 56, 1062–1071. doi: 10.1016/j.compedu.2010.09.014
Wise, L. L., and Plake, B. S. (2015). ‘Test design and development following the standards for educational psychological testing,” in Handbook of Test Development, eds S. Lane, M. R. Raymond, and T. M. Haladyna (New York, NY: Routledge, 19–39.
Zapata-Rivera, D., VanWinkle, W., and Zwick, R. (2012). Applying Score Design Principles in the Design of Score Reports for CBAL™ Teachers. (ETS Research Memorandum RM−12–20). Princeton, NJ: ETS.
Zenisky, A. L., and Hambleton, R. K. (2012). Developing test score reports that work: the process and best practices for effective communication. Edu. Measur. Iss. Pract. 31, 21–26. doi: 10.1111/j.1745-3992.2012.00231.x
Zenisky, A. L., and Hambleton, R. K. (2013). “From “Here's the Story” to “You're in Charge”: Developing and maintaining large-scale online test and score reporting resources,” in Improving Large-Scale Assessment In Education, eds M. Simon, M. Rousseau, and K. Ercikan (New York, NY: Routledge), 175–185. doi: 10.4324/9780203154519-11
Zenisky, A. L., and Hambleton, R. K. (2015). “A model and good practices for score reporting,” in Handbook of Test Development, eds S. Lane, M. R. Raymond, and T. M. Haladyna (New York, NY: Routledge), 585–602.
Zwick, R., Zapata-Rivera, D., and Hegarty, M. (2014). Comparing graphical and verbal representations of measurement error in test score reports. Educ. Assess. 19, 116–138. doi: 10.1080/10627197.2014.903653
Appendix
Keywords: feedback, score reporting, computer-based testing, learning, higher education
Citation: Bulut O, Cutumisu M, Aquilina AM and Singh D (2019) Effects of Digital Score Reporting and Feedback on Students' Learning in Higher Education. Front. Educ. 4:65. doi: 10.3389/feduc.2019.00065
Received: 21 May 2019; Accepted: 17 June 2019;
Published: 28 June 2019.
Edited by:
Mustafa Asil, University of Otago, New ZealandReviewed by:
April Lynne Zenisky, University of Massachusetts Amherst, United StatesHui Yong Tay, Nanyang Technological University, Singapore
Copyright © 2019 Bulut, Cutumisu, Aquilina and Singh. This is an open-access article distributed under the terms of the Creative Commons Attribution License (CC BY). The use, distribution or reproduction in other forums is permitted, provided the original author(s) and the copyright owner(s) are credited and that the original publication in this journal is cited, in accordance with accepted academic practice. No use, distribution or reproduction is permitted which does not comply with these terms.
*Correspondence: Okan Bulut, YnVsdXRAdWFsYmVydGEuY2E=