- 1TUM School of Education, Teaching and Learning with Digital Media, Technical University of Munich, Munich, Germany
- 2Institute of Human Computer Media, Psychology of Communication and New Media, University of Würzburg, Würzburg, Germany
Desirable difficulties initiate learning processes that foster performance. Such a desirable difficulty is generation, e.g., filling in deleted letters in a deleted letter text. Likewise, letter deletion is a manipulation of processing fluency: A deleted letter text is more difficult to process than an intact text. Disfluency theory also supposes that disfluency initiates analytic processes and thus, improves performance. However, performance is often not affected but, rather, monitoring is affected. The aim of this study is to propose a specification of the effects of disfluency as a desirable difficulty: We suppose that mentally filling in deleted letters activates analytic monitoring but not necessarily analytic cognitive processing and improved performance. Moreover, once activated, analytic monitoring should remain for succeeding fluent text. To test our assumptions, half of the students (n = 32) first learned with a disfluent (deleted letter) text and then with a fluent (intact) text. Results show no differences in monitoring between the disfluent and the fluent text. This supports our assumption that disfluency activates analytic monitoring that remains for succeeding fluent text. When the other half of the students (n = 33) first learned with a fluent and then with a disfluent text, differences in monitoring between the disfluent and the fluent text were found. Performance was significantly affected by fluency but in favor of the fluent texts, and hence, disfluency did not activate analytic cognitive processing. Thus, difficulties can foster analytic monitoring that remains for succeeding fluent text, but they do not necessarily improve performance. Further research is required to investigate how analytic monitoring can lead to improved cognitive processing and performance.
Introduction
During learning students might face difficulties. However, facing difficulties does not necessarily result in low performance. Inversely, difficulties can be desirable because they can initiate effortful and analytic processes. Hence, difficulties during learning can be desirable in some cases because they can initiate processes that help students to learn better (e.g., Bjork et al., 2007; McDaniel and Butler, 2011). One important metacognitive process during learning in educational contexts is monitoring. Students can make different types of judgments during the learning process to monitor learning (e.g., Nelson and Narens, 1990). For example, they judge how difficult it is to learn a text, or they judge how much knowledge they have acquired during learning and how successful they will be in an upcoming test. However, students often use cues for metacognitive judgments that are not valid for their performance. For example, students often predict high performance for texts that are easily processed, although the ease of processing (fluency) does not improve performance (e.g., Rawson and Dunlosky, 2002; Experiment 4). Non-valid cues are problematic, because using valid cues for judgments is a prerequisite for effective learning and high performance (see theories of metacognition and self-regulated learning, e.g., Nelson and Narens, 1990; Zimmerman, 1990; Boekaerts, 1997; Winne and Hadwin, 1998; Dunlosky et al., 2005). Therefore, students‘ monitoring of the learning process should be analytic in order to use valid cues and consequently make accurate judgments (see Pieger, 2017). Desirable difficulties like disfluency seem to be a way to activate analytic processes (Alter et al., 2007) that are relevant for metacognition. Analytic monitoring enables students to no longer use fluency as a cue for their judgments. Moreover, once activated, more analytic monitoring should be found not only for disfluent but also for succeeding fluent material. The aim of this study is to investigate whether disfluency is a desirable difficulty and activates analytic monitoring, not only for a disfluent text itself, but also for a succeeding fluent text (see also Pieger, 2017). In the following, we will argue for this assumption and describe our theoretical framework in more detail.
Effects of Disfluency on Metacognition: A Specification
Fluency is defined as ease of processing (Schwarz, 2010). Material that is easy to process is fluent, whereas material that is difficult to process is disfluent. There are different types of fluency, like conceptual and perceptual fluency (Schwarz, 2010, see also Alter and Oppenheimer, 2009, for a more detailed taxonomy). Conceptual fluency includes the ease of identifying the meaning of words and knowledge structures and can be manipulated, e.g., by letter deletion. Perceptual fluency describes the ease of identifying words and can be manipulated, e.g., by font. Conceptual fluency is especially relevant when learning with texts because it affects processing on a higher level than perceptual fluency; when learning with text, students do not only have to decipher words (surface level), but they also have to construct a meaningful representation of the texts (textbase level and situation model, see e.g., Kintsch, 1994; De Bruin and van Gog, 2012; Redford et al., 2012).
The disfluency-theory supposes that disfluency initiates slow, deliberate and analytic processes (System 2 processes), whereas fluency initiates quick, intuitive, associative and surface processes (System 1 processes; see Alter et al., 2007). Further, they suggest that disfluency serves as a metacognitive cue resulting in more System 2 reasoning. Additionally, we distinguish between cognitive processing (i.e., coherence formation when reading a text) and metacognitive processing (i.e., monitoring and control) based on Nelson and Narens (1990) model of metamemory. We use the term analytic monitoring for a deliberate processing of cues when monitoring cognitive processing, and thus analytic monitoring enables students to no longer use fluency as a cue for their judgments. Additionally we use the term analytic control for deliberate control processes that may potentially result from analytic monitoring. For example, a reader may decide to re-read a passage of a text (i.e., control) based on a judgment of low retention (i.e., monitoring) that is based on a valid cue (i.e., a failed retention attempt). When reading a text, one may assume that analytic monitoring and analytic control leads to changes in cognitive processing (i.e., re-reading) that may result in better performances. Thus, learning with disfluent material is expected to lead to better performance compared to learning with fluent material. The difference between fluent and disfluent material, e.g., on performance, is called the fluency effect. Hence, finding a fluency effect on performance indicates that disfluency is a desirable difficulty. However, previous research has shown that fluency does often not affect performance as no fluency effect was found (e.g., Maki et al., 1990, Experiment 2; McDaniel et al., 1986, Experiment 1; Rawson and Dunlosky, 2002, Experiment 4, for conceptual fluency; see also Eitel et al., 2014; Eitel and Kühl, 2016, Experiment 2–4; Lehmann et al., 2016; Rummer et al., 2016; Strukelj et al., 2016, for perceptual fluency). Due to the inconsistent findings of fluency effects on performance, some conditions for fluency effects have been investigated (see McDaniel and Butler, 2011; Kühl and Eitel, 2016). Bjork and Yue (2016) further mention that disfluency is a difficulty, but whether it is a desirable difficulty depends from the processes that are activated by disfluency.
Because the empirical evidence does often not support the assumption that disfluency is a desirable difficulty, it is reasonable to more precisely specify, which processes are activated by disfluency (see Pieger, 2017). In accordance with Alter et al. (2007; see also Diemand-Yauman et al., 2011), we assume that disfluency leads to more analytic metacognitive processes. However, we further state (see also Pieger, 2017) that these metacognitive processes mainly refer to metacognitive monitoring and not necessarily to metacognitive control (i.e., the regulation of cognitive processes, e.g., Koriat et al., 2006; Dunlosky and Metcalfe, 2009). Moreover, we assume that analytic monitoring is not only slower but also less automatic and more elaborate. Theories of self-regulated learning and metacognition state that monitoring may affect control. Further, effective control is required to alter cognitive processes and therefore to improve performance (Nelson and Narens, 1990; Zimmerman, 1990; Boekaerts, 1997; Winne and Hadwin, 1998). Hence, by theory, there are different explanations how disfluency can improve performance. The first explanation is: disfluency leads to deep cognitive processing and thus, performance improves. However, as we have outlined above, often no fluency effects on performance are found. Hence, disfluency does not seem to activate deep cognitive processing but simply slows down processing of the learning material. This slowdown is due to processes that are irrelevant for text comprehension (i.e., deciphering words in a non-fluent font or mentally filling in letters). Therefore, longer reading-times for disfluent rather than for fluent texts (e.g., McDaniel, 1984; McDaniel et al., 1986, for conceptual fluency; see also Miele and Molden, 2010; Eitel and Kühl, 2016; Pieger et al., 2016, Experiment 3; Sanchez and Jaeger, 2015, Experiment 1 and 2, for perceptual fluency) might be due to less automatic processing but not necessarily due to deeper cognitive processing. In this case, disfluency seems to be not desirable as a difficulty as it does not activate analytic cognitive processes. A second explanation is that analytic cognitive processing is not directly activated by disfluency but the effect of disfluency is mediated by metacognitive processes (see Pieger, 2017). Analytic monitoring can activate analytic cognitive processing: As supposed by theories about metacognition (e.g., Nelson and Narens, 1990; Zimmerman, 1990; Boekaerts, 1997; Winne and Hadwin, 1998), monitoring affects control of cognitive processes. Hence, if analytic monitoring is used to control cognitive processing, this might result in deeper cognitive processes and, thus, in better performance. Hence, whether disfluency is a desirable difficulty that results in better performance for disfluent than for fluent texts, seems to depend on the fluency effect on monitoring. Although disfluency might not activate analytic cognitive processing directly, disfluency might activate analytic monitoring, i.e., it alters metacognitive judgments. If students use these metacognitive judgments to control cognitive processes, performance can improve, moderated by the fluency effect on monitoring.
Our assumption that disfluency activates analytic monitoring is supported by the studies by Alter et al. (2007). For example in their Experiment 2, they found that students processed arguments of persuasion in a more analytic way when the masthead (not the arguments per se) was presented in a disfluent way than in a fluent way. Only the masthead and not the arguments were disfluent, therefore, the processing of the arguments was not directly affected by disfluency. Nevertheless, performance improved for these arguments. Thus, one explanation for this finding is that disfluency has activated analytic monitoring. This analytic monitoring even remained during reading the fluently printed arguments and enabled analytic processing of the arguments. Our interpretation of the Alter et al. study is that disfluency activates analytic monitoring and that this analytic monitoring remains for the subsequent learning material, even if this material is fluent.
As a consequence of analytic monitoring for disfluent and succeeding fluent material, students might think about what they have learned instead of using fluency of a text as a cue for performance. Thus, they should not predict higher performance for the fluent text than for the disfluent text. Hence, presenting disfluent and, afterwards, fluent material (we will call this contrast disfluent-fluent) should reduce the fluency effect on monitoring. This reduced fluency effect is due to analytic monitoring of the disfluent and the fluent text. Under the condition of contrast disfluent-fluent, disfluency is a desirable difficulty that activates analytic metacognitive processes, and these analytic processes remain for succeeding fluent material. Inversely, when fluent material is presented before disfluent material (we will call this contrast fluent-disfluent), analytic monitoring is only activated for the disfluent but not for the previous fluent material. Consequently, a fluency effect on monitoring should be found: Students use fluency as a cue for their judgments (see Nelson and Narens, 1990, for different types of judgments), e.g., when judging how difficult it will be to learn a text (ease of learning judgment, EOL judgment), when predicting their own performance (judgments of learning, or more precisely predictions of performance, POPs) or judging the correctness of their answers in a knowledge test (retrospective confidence judgments, RC judgments). In previous research there is some evidence for fluency effects on judgments (e.g., Maki et al., 1990; Pieger et al., 2016, 2017, Experiment 1; Rawson and Dunlosky, 2002, Experiment 4), supporting our assumption of fluency being a cue for judgments. Most studies focus on one kind of judgment, predominantly POPs (e.g., Rawson and Dunlosky, 2002) or two kinds of judgments (e.g., POP and RC, Maki et al., 1990). In order to investigate the entire process of learning from texts some researcher included additionally other types of judgments like EOLs, familiarity judgments and comprehension judgments (Pieger et al., 2016, 2017). Using the latter approach one may test if fluency activates analytic monitoring not only after reading a disfluent text but also via a short presentation of the fluency manipulation (EOL, see Pieger et al., 2016), and if the fluency effect remains for RC judgments even though the performance test is presented in a fluent manner (Pieger et al., 2017). Moreover, Pieger et al. (2016) found that not only the type of judgment, but also the stage of the learning process seem to matter.
Summing up, the aim of this study is to test whether and under which conditions disfluency is a desirable difficulty. Thereby, the effects of disfluency are specified with respect to monitoring, control, cognitive processing, and performance.
Research Questions and Hypotheses
We assume (see also Pieger, 2017) that disfluency activates analytic monitoring and that analytic monitoring remains for succeeding fluent material. Hence, the sequence of presenting fluent and disfluent material (we will call this type of contrast) moderates fluency effects on judgments. When students learn with a disfluent text first and then with a fluent text (contrast disfluent-fluent), disfluency should activate analytic monitoring, which should then remain for the succeeding fluent text. Because of analytic monitoring for the disfluent and fluent texts, fluency should not be used as a cue for judgments. Thus, no fluency effects on EOL judgments, POPs, and RC judgments that are made at different learning stages are expected.
Inversely, when students learn with a fluent text first and afterwards with a disfluent text (contrast fluent-disfluent), monitoring of the fluent text is expected to be surface level instead of analytic. Thus, students should base all of their judgments on the experience of fluency: They should judge disfluent, compared to fluent texts, as more difficult (EOL judgments), they should predict lower performance (POPs) for disfluent than for fluent texts, and they should be less confident in the correctness of a retrieved answer for disfluent than for fluent texts (RC judgment).
Independent from the type of contrast in fluency, disfluency should lead to slower processing: Disfluency compared with fluency should lead to longer reading-times. Although disfluency might not lead to analytic cognitive processing and better performance, analytic monitoring can activate analytic control.
Method
Participants and Experimental Design
The experiment was part of a dissertation (see Pieger, 2017) and it was carried out at a university in Germany. In total N = 65 students participated in the study (age: M = 20.09, SD = 1.60 years, 78.46% female); n = 49 (75.38%) students studied media communication and n = 16 (24.62%) students studied human-computer-interaction (semester of studies: M = 2.68, SD = 1.58). No ethical approvement by an ethical committee was needed because the students were not exposed to any threat like medical or physical threat, and neither the university nor the institute human—computer—media requires an ethical approvement mandatory. The students voluntarily participated in the study and they gave informed, written consent to participate in the study and to use of their data for research purposes. The acquired sample size fulfills the required sample size of 67 students, computed with G*-Power (Faul et al., 2007) by setting the Type I error to 0.05, the power to 0.80, and assuming an effect size of f = 0.35 because we expected higher effects for conceptual than for perceptual fluency (see also Maki et al., 1990; Rawson and Dunlosky, 2002). Fluency was varied within persons whereas type of contrast was varied between persons. Students were assigned to one of two groups randomly. The experimental groups differed in the type of contrast in fluency: Students in contrast group disfluent-fluent (n = 32) read a disfluent text and afterwards a fluent text, whereas students in contrast group fluent-disfluent (n = 33) read a fluent text and afterwards a disfluent text. Monitoring (EOL judgments, POPs, and RC judgments), control (reading-time and termination of study), and performance were used as the dependent variables.
Material
Two expository texts about motivational psychology (Rudolph, 2009) were used as learning material. These texts had been used in a previous study and were classified as comparable in length and difficulty: Text A was about the rubicon model and consisted of 936 words (Flesch-Kincaid grade-level score = 18.94); text B explained causal dimensions in attribution theory and consisted of 929 words (Flesch-Kincaid grade-level score = 20.92). Scores were computed by a tool developed by Michalke (2012).
For disfluent texts, letters were deleted using an algorithm similar to the one used by McDaniel (1984). The algorithm was adapted for German texts in order to create disfluent texts that were comparable to the experiments by Maki et al. (1990) and by Rawson and Dunlosky 2002, Experiment 4). We first deleted all vowels in every noun, adjective and verb, except for initial letters, and replaced them by one underscore for each deleted letter. To guarantee that each word was recognizable as one word, the words were spaced by five blank spaces (see Figure 1 for an example of a disfluent text). Texts were piloted to ensure that the words could still be correctly read. Therefore, students in the pilot study (N = 10) had to fill in each deleted letter of the two texts. If <50% of these students were able to fill in the letters, all letters of this word were filled in for the experiment. This was the case for four technical terms.
The materials that have been used in this study will be made available by the authors, without undue reservation, to any qualified researcher.
Instruments
Reading-time was used as a manipulation-check because slow processing of disfluent texts directly affects reading-time when reading a text once. Judgments were captured by asking questions that students had to answer on a continuous visual analog scale from 0 to 100 by keyboarding integer numbers. For EOL judgments, we asked, “How easy or difficult is it to learn the text?” using a scale labeled from 0 = difficult (50 = middle) to 100 = easy. For POPs, we asked, “What percentage of questions about the text will you answer correctly?” using a scale labeled from 0 = none (50 = half) to 100 = all. For RC judgments, we asked “How confident are you that your answer is correct?” using a scale labeled from 0 = unconfident (50 = middle) to 100 = confident.
Performance was assessed by a knowledge test that consisted of 23 questions on text A, and of 24 questions on text B. The test included questions that asked for the recall of information, comprehension of the text (i.e., inference questions) and transfer to issues that were not mentioned in the text explicitly. Each question consisted of 6 statements. An example for a question with six statements on text A is presented in Figure 2. Each statement was sequentially presented on the screen, and students had to decide if it was true or false. For each statement that students correctly identified as true or false, they were awarded one point. Performance was computed as the mean of all statements. As the chance to guess the correct answer was 50%, this score was corrected for guessing using the algorithm 200· × −100. This algorithm transforms the value of guessing to zero (200·0.50 – 100 = 0), resulting in a performance scale from 0% up to 100%. Reliability of the performance test was Cronbach's α = 0.81 (M = 51.07%, SD = 10.38%).
Procedure
E-Prime-Software (E-Prime Professional 2.0) was used to present the materials and to collect the data. The procedure is shown in Figure 3. First, an extract of the first text was presented for 2 s on the screen, and afterwards, students made EOL1. Then, students were instructed to read the entire text only once on the screen (without rereading or skipping), and afterwards, they made EOL2 and POP1 on the next screens. In this phase, reading times were captured as a manipulation check. Moreover, when making judgments, students had more information about the text than after the short text presentation but they did not have the chance to elaborate the text. Therefore, the text was shown again, and this time, students were allowed to reread, to take notes, and to skip within the text for a maximum of 15 min. However, students were allowed to terminate their study before the time expired. Immediately afterwards, students made POP2 on the next screen. The same procedure was done with the second text. Finally, the knowledge test with questions about each text was presented. The order of the texts was randomized. If text A was the first, text questions on text A were presented first, and if text B was the first, text questions on text B were presented first, so there was a delay between learning the text and answering questions about this text. Each question statement of a question was separately presented on the screen, and students had to decide if this statement was true or false. Students received one point for each correct decision. Immediately after each statement, students made a RC judgment on the next screen. The experiment took approximately 2 h.
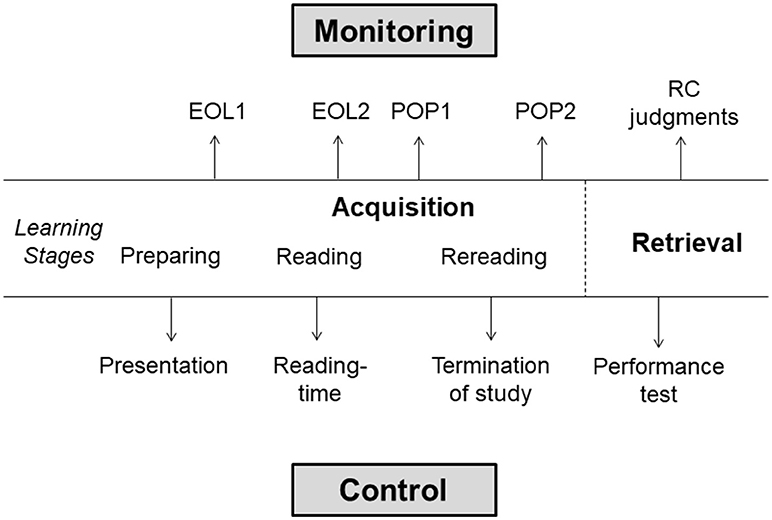
Figure 3. Procedure of the study. The procedure was the same for each of the two texts; performances were captured after acquisition of both texts.
Results
For all analyses the Type I error rate was set to 0.05. The raw data supporting the conclusions of this manuscript will be made available by the authors, without undue reservation, to any qualified researcher. Frequentist statistics were calculated using SPSS Version 24. For univariate analyses, we calculated Bayes-Factors using JASP Version 0.9.0.1. We used the default prior distribution that is implemented into JASP, that is, combinations of uninformative Couchy-priors (Rouder et al., 2012, for details). We report BF10, that is the likelihood in favor of the H1 compared to the null-model (i.e., the model that includes only the grand mean and the subjects), and for interaction effects we additionally compared the full-effects model to the main-effects model. We used the terms as suggested by Jeffreys (cited from Jarosz and Wiley, 2014) when describing the results.
Descriptive statistics of dependent variables (judgments, reading-time, termination of study, and performance) in contrast group disfluent-fluent and in contrast group fluent-disfluent are presented in Table 1. We computed a mixed MANOVA to test if there was a significant multivariate effect of the within-subject factor fluency (fluent vs. disfluent), and the between-subject factor type of contrast (disfluent-fluent vs. fluent-disfluent) on the dependent variables that are listed in Table 1 (correlations between dependent variables can be found in Table (A1). Results showed a significant main effect of fluency, V = 0.671, F(8, 52) = 13.23, p < 0.001, = 0.67, and a significant interaction between fluency and type of contrast, V = 0.383, F(8, 52) = 4.04, p = 0.001, = 0.38. The main effect of type of contrast was not significant, V = 0.147, F(8, 52) = 1.12, p = 0.363, = 0.15. Next, we report the significant effects for each dependent variable separately.
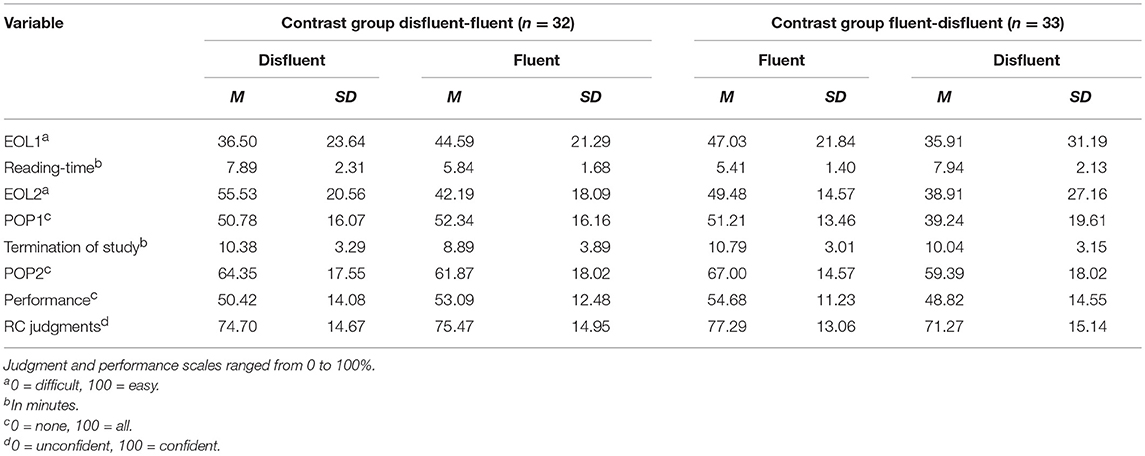
Table 1. Descriptive statistics of dependent variables in contrast group disfluent-fluent and in contrast group fluent-disfluent.
To test whether our fluency-manipulation was effective, reading-time was analyzed. We found a significant main effect of fluency on reading-time in the reading phase, F(1, 63) = 74.56, p < 0.001, = 0.54, BF10 = 1.99 × 1010. The fluency-manipulation worked equally effectively for both types of contrast; the interaction between fluency and type of contrast was not significant, F(1, 63) = 0.84, p = 0.364, = 0.01, BF10 = 2.05 × 109. Comparing the likelihood of the full model to the main-effects model, BF10 = 5.85 × 109, shows that the full model is 0.35 times less likely. Thus, fluent texts were read longer in both conditions.
Next, the judgments are analyzed in the order in which they were made throughout the experiment. We found a significant main effect of fluency on EOL1, F(1, 60) = 10.82, p = 0.002, = 0.15, BF10 = 19.43, but no significant interaction between fluency and type of contrast, F(1, 60) = 0.37, p = 0.547, = 0.01, BF10 = 1.82. Comparing the full model to the main-effect only model, BF10 = 6.06, shows that the full model is 0.30 times less likely. Therefore, the disfluent text was judged as more difficult than the fluent text for both types of contrast.
A significant interaction between fluency and type of contrast was found for EOL2, F(1, 63) = 14.33, p < 0.001, = 0.19, BF10 = 10.38. The full model is 139.19 times more likely than the main effects model, BF10 = 0.08, indicating that the fluency effect was moderated by the type of contrast. Students in contrast group disfluent-fluent judged the fluent text compared with the disfluent text as more difficult, F(1, 31) = 9.63, p = 0.004, = 0.24, BF10 = 16.64, whereas students in contrast group fluent-disfluent judged the disfluent text as more difficult than the fluent text, F(1, 32) = 5.24, p = 0.029, = 0.14, BF10 = 2.45, but this effect is merely anecdotal as the effect is only 2.45 more likely than the null-hypothesis.
For POP1, the fluency effect was moderated by the type of contrast because there was a significant interaction between fluency and type of contrast, F(1, 63) = 5.08, p = 0.028, = 0.08, BF10 = 16.52. However, the full model is only 2.08 times more likely compared to the main effects model, BF10 = 8.13. As expected, students in contrast group disfluent-fluent predicted equal performance for the fluent and the disfluent text, F(1, 31) = 0.29, p = 0.591, = 0.01, BF10 = 0.29, and students in contrast group fluent-disfluent predicted lower performance for the disfluent than for the fluent text, POP1, F(1, 32) = 11.13, p = 0.002, = 0.26, BF10 = 27.73. Whereas the evidence for the former non-fluency effect (i.e., fluency is 0.29 times less likely than non-fluency) is substantial, we have strong evidence (i.e., a fluency-effect is 27.73 times more likely than no effect) for the latter fluency effect.
For POP2, after rereading the text, we found a similar pattern: The interaction between fluency and type of contrast was significant, F(1, 62) = 4.47, p = 0.039, = 0.07, BF10 = 0.15, but comparing the bayes-factor of the full model with the bayes-factor of the main effects only model, BF10 = 0.09, shows, that the model including the interaction is only 1.71 times more likely than the model that does not include the interaction. Nevertheless, students in contrast group disfluent-fluent predicted equal performance for the fluent and the disfluent text, F(1, 30) = 0.62, p = 0.436, = 0.02, BF10 = 0.34, as the bayes-factor indicates substantial evidence for the null-model. The students in contrast group fluent-disfluent predicted lower performance for the disfluent compared to the fluent text, POP2, F(1, 32) = 4.56, p = 0.040, = 0.12, BF10 = 2.00, but again the bayes-factor shows that this is merely anecdotal evidence (i.e., the fluency-effect is two times more likely than no fluency-effect).
Moreover, we found a significant interaction between fluency and type of contrast on RC judgments, F(1, 63) = 4.42, p = 0.040, = 0.07, BF10 = 2.65. Nevertheless, the model including the interaction effect is only 1.58 times more likely than the main effects model, BF10 = 1.68. In contrast group disfluent-fluent, students made equal RC judgments for the fluent and the disfluent text, F(1, 31) = 0.23, p = 0.636, = 0.01, BF10 = 0.28. According to the bayes-factor we have substantial evidence for the null-hypothesis (i.e., the fluency-effect is 0.28 times likely than no fluency-effect). In contrast group fluent-disfluent, students were less confident about their performance for the disfluent than for the fluent text, F(1, 32) = 10.11, p = 0.003, = 0.24, BF10 = 10.68.
For termination of study in the rereading phase, a significant interaction between fluency and type of contrast indicates different fluency effects depending on the type of contrast, F(1, 63) = 7.65, p = 0.007, = 0.11, BF10 = 0.75. The comparison of the full model to the main effects only model, BF10 = 0.12, shows, that the former one is 6.43 times more likely than the latter one. Students in contrast group disfluent-fluent studied disfluent texts significantly longer than fluent texts, F(1, 31) = 5.67, p = 0.024, = 0.15, BF10 = 2.36, but the bayes-factor shows that this is only anecdotal evidence. Students in contrast group fluent-disfluent, however, did not terminate their study later for the disfluent than for the fluent text, F(1, 32) = 2.10, p = 0.157, = 0.06, BF10 = 0.59, but again the evidence for the null-effect hypothesis is anecdotal.
Finally, for performance, a significant main effect of fluency was found, F(1, 63) = 4.68, p = 0.034, = 0.07, BF10 = 1.70. The interaction between fluency and type of contrast showed no significant result, F(1, 63) = 0.65, p = 0.422, = 0.01, BF10 = 0.13. The full model is 0.31 times less likely than the main effects model, BF10 = 0.43. Thus, for both types of contrast, performance was significantly different between fluent and disfluent texts in favor of the fluent texts. However, as the bayes-factor renders only anecdotal evidence for this hypothesis, it remains open if fluency had an effect on performance.
To sum up, we found different fluency effects for the two types of contrast for all judgments that were made after reading, rereading, and during the test (EOL2, POP1, POP2, and RC judgments). Whereas, no fluency effect on these judgments was found in contrast group disfluent-fluent, a fluency effect on these judgments was found in contrast group fluent-disfluent. However, this conclusion is based on frequentistic statistics; using Bayesian statistics the results are not that convincing anymore as for some effects (i.e., POP1, POP2, RC judgments) the likelihood is only around two times higher in favor for the interaction between contrast type and fluency. Additionally, the fluency effect on EOL2 was inverted in contrast group disfluent-fluent. On EOL1, a fluency effect was found for both types of contrast.
Discussion
The aim of this study was to investigate whether disfluency is a desirable difficulty and activates analytic monitoring, not only for disfluent, but also for the succeeding fluent text. We expected that the type of contrast in fluency moderates the fluency effect on judgments: When a disfluent text is presented before a fluent text, disfluency should activate analytic monitoring, which remains for the succeeding fluent text. Therefore, fluency should not be used as a cue for judgments. Inversely, when students first learn with a fluent and then with a disfluent text, monitoring of the fluent text is expected to be surface, and thus, fluency should be a cue for judgments.
Results from the frequentist statistics widely support this assumption, and results from Bayesian statistics support this assumption at least partly: When a fluent text was presented before a disfluent text, fluency is used as a cue for all types of judgments during the entire learning process. These results are consistent with previous findings of fluency effects on judgments (e.g., Rawson and Dunlosky, 2002, Experiment 4). Moreover, these results go beyond previous findings because we found this effect on different types of judgments during the learning process, even on RC judgments during the test.
Inversely, when students first learned with a disfluent and then with a fluent text, fluency was no longer used as a cue for POP1, POP2, and RC judgments. This non-effect of fluency cannot be attributed to a failed manipulation of fluency because students in contrast group disfluent-fluent read and reread the disfluent text significantly longer than the fluent text. Therefore, students experienced fluency, but this experience was not used as a cue for POPs and RC judgments. This finding supports our hypothesis that disfluency activates analytic monitoring, and it remains activated for the succeeding fluent text.
Additionally, results concerning performance in both experimental groups further support our assumption that disfluency does not necessarily lead to more analytic or deeper processing of the learning material. Although students read the disfluent text significantly longer than the fluent text, they did not show better performance on the disfluent text. Therefore, processing of the text was slower, but not more analytic for the disfluent than for the fluent text. These findings are consistent to our specification of processes that are activated by disfluency: Disfluency-theory postulates that disfluency leads to slower and to more analytic processes (Alter et al., 2007). We suggested that (a) these processes seem to be metacognitive (see also Alter et al., 2007) and that (b) these processes refer to analytic metacognitive monitoring but not necessarily to analytic control of deeper cognitive processing of the text.
However, based on our results, we conclude that the effects of disfluency should not only be specified with respect to monitoring and cognitive effects. Moreover, the metacognitive effects of disfluency on monitoring seem to depend on the type of metacognitive judgment. We found that fluency was not used as a cue for POPs or for RC judgments in contrast group disfluent-fluent but fluency was used as a cue only for EOL1 judgments. This finding is consistent with the findings by Maki et al. (1990, Experiment 1): Fluency effects on judgments were found when students judged how difficult a text is to understand, but no fluency effects on judgments were found when students predicted performance. Whereas POPs and RC judgments ask students about their performance, EOL judgments ask students about the difficulty of the texts. Disfluent texts are indeed more difficult to read, as can be seen in the prolonged reading-times compared to fluent texts. Mentally filling in deleted letters requires more time compared to reading an intact text. This affects the surface level of text processing. Therefore, students judged the disfluent text as more difficult to learn than the fluent text (EOL1) even though the content was the same. Inversely, students made equal POPs and RC judgments for disfluent and fluent texts. Therefore, students did not use fluency as a cue for POPs and RC judgments because of analytic monitoring. However, we have found a significant effect of fluency on performance in favor of the fluent texts, even though the result from Bayesian statistics does not support this result much, and a recent meta-analysis reports a null-effect of perceptual disfuency on performances when reading texts (Xie et al., 2018).
Moreover, students in contrast group disfluent-fluent judged the fluent text as more difficult to learn than the disfluent text after reading the texts once (i.e., EOL2). This is somewhat surprising given the fact that they judged the disfluent text as being more difficult than the fluent text after a presentation for 2 s on the screen (EOL1). Therefore, not only the type of judgment but also the learning stage seems to play a role in fluency effects on EOL judgments. When reading a text once, students have much more information about the text than after a short text presentation. Hence, they can use different cues for EOL2 than for EOL1. For EOL1, disfluency might be salient as a cue, but after reading more cues are available such as e.g., the terms that are used in the text, the mental model that has been constructed during the first reading etc. As the participants in group disfluent-fluent have experienced disfluency and thus, they switched to more analytic monitoring, these cues might have been used instead of the fluency when judging the difficulty of the text for a second time. This is also true for POP1, which are also made after the first reading phase. The different finding on EOL2 and POP1 might result because EOL2 explicitly asked for the difficulty of the text, whereas POP1 asked for performance predictions. Hence, different cues might play a role for the judgments, dependent from the learning stage and the type of judgment. However, further research is required to investigate these post-hoc explanations. Further research should also investigate further learning stages, e.g., fluency effects on judgments and performance after a longer delay. In this study, there was a delay between learning and testing of a text: Students first sequentially learned the two texts and afterwards questions about the first and then about the second text followed. Further research should test whether results are the same for longer delays.
Summing up, disfluency can activate analytic monitoring. Moreover, this analytic monitoring is not only found for disfluent but also for succeeding fluent material. More analytic monitoring is activated by the disfluent text and remains for the succeeding fluent text when making POPs and RC judgments. Inversely, when presenting first a fluent and, afterwards, a disfluent text, monitoring of the fluent text is not analytic. Under the condition of contrast fluent-disfluent, students base their judgments on the experience of quick processing of the fluent texts and of slow processing of the disfluent text. Thus, the type of contrast in fluency affects the fluency effect on POPs and RC judgments.
Importantly, the Bayes-factor analyses show not such clear evidence. Models that include the interaction of fluency and type of contrast are barely around two times more probable than the main effects model (except for EOL2, showing clear evidence for the interaction effect). However, our results parallel the results of Pieger et al. (2017) for contrast effects of perceptual fluency manipulations, using different text material. Thus, we assume that stronger fluency effects on metacognitive judgments can be found when a fluent text is learned first and a disfluent text is learned second. Further, weaker or even no fluency effects can be found when a disfluent text is learned first and a fluent text is learned second. Nevertheless, these effects should be replicated in further studies that use larger samples to get clear evidence for or against the interaction effect of contrast type and fluency. Additionally, a variety of texts should be used in order to show the robustness of the effect over different materials.
Conclusion
In this study, we investigated if disfluency activates analytic monitoring not only for disfluent but also for a succeeding fluent text. Another goal was to give some empirical evidence for our specification of the effects of disfluency to explain inconsistent findings of fluency effects in previous research. Disfluency-theory supposes that disfluency leads to slow, analytic processes whereas fluency leads to quick, surface processes (Alter et al., 2007). However, these processes need to be specified as they can refer to metacognitive monitoring, metacognitive control, or processing of the learning material. We supposed that disfluency activates analytic monitoring but that it does not necessarily activate analytic control or deeper processing of the learning material, and these assumptions are in line with our results. Hence, when considering whether disfluency is a desirable difficulty, it is important to specify the processes, as difficulties might be desirable not only for control and for performance, but also for monitoring. Moreover, based on our results and on results from previous research, we suppose that further specifications are required (see Pieger, 2017): Fluency effects on judgments are affected by the interplay between the type of contrast in fluency, the type of judgment students are asked for, and the stage of the learning process. To derive guidelines for educational contexts, it is important to conduct systematic research that investigates this interplay (Pieger, 2017). These guidelines are needed because disfluency is used in textbooks (e.g., italic, bold, font types, fill-in-the-blank text) without understanding the effects of disfluency and its interactions with the learning stage of students' monitoring. Based on our findings, we conclude that disfluency might be more than just a cue for judgments, it might be a way to activate analytic monitoring.
Ethics Statement
We asked all participants for informed consent according to the ethical policy of the Deutsche Gesellschaft für Psychologie (German Psychological Association).
Author Contributions
EP developed the research, conceptualizing, preparation, management, data-analysis, discussion, presentation of the experiment, and publishing the paper. MB and CM involved in the conceptualization of the study. CM has written small parts of the paper and did a minor part of the data-analysis. MB and CM were discussion-partners and provided feedback on the paper.
Conflict of Interest Statement
The authors declare that the research was conducted in the absence of any commercial or financial relationships that could be construed as a potential conflict of interest.
The handling editor declared a shared affiliation, though no other collaboration, with one of the authors (CM) at time of review.
Acknowledgments
A previous version of this paper appeared in the following dissertation: Pieger (2017). Metacognition and disfluency—The effects of disfluency on monitoring and performance. [Dissertation]. [Germany]: Julius-Maximilans-University of Wuerzburg. The dissertation can be accessed online: urn:nbn:de:bvb:20-opus-155362. The publication is in line with universities policy.
References
Alter, A. L., and Oppenheimer, D. M. (2009). Uniting the tribes of fluency to form a metacognitive nation. Personal. Soc. Psychol. Rev. 13, 219–235. doi: 10.1177/1088868309341564
Alter, A. L., Oppenheimer, D. M., Epley, N., and Eyre, R. N. (2007). Overcoming intuition: metacognitive difficulty activates analytic reasoning. J. Exp. Psychol. Gen. 136, 569–576. doi: 10.1037/0096-3445.136.4.569
Bjork, E. L., DeWinstanley, P. A., and Storm, B. C. (2007). Learning how to learn: can experiencing the outcome of different encoding strategies enhance subsequent encoding? Psychon. Bull. Rev. 14, 207–211. doi: 10.3758/BF03194053
Bjork, R. A., and Yue, C. L. (2016). Commentary: is disfluency desirable? Meta. Learn. 11,133–137. doi: 10.1007/s11409-016-9156-8
Boekaerts, M. (1997). Self-regulated learning: a new concept embraced by researchers, policy makers, educators, teachers, and students. Learn. Instr. 7, 161–186. doi: 10.1016/S0959-4752(96)00015-1
De Bruin, A. B., and van Gog, T. (2012). Improving self-monitoring and self-regulation: from cognitive psychology to the classroom. Learn. Instr. 22, 245–252. doi: 10.1016/j.learninstruc.2012.01.003
Diemand-Yauman, C., Oppenheimer, D. M., and Vaughan, E. B. (2011). Fortune favors the bold (and the italicized): effects of disfluency on educational outcomes. Cognition 118, 114–118. doi: 10.1016/j.cognition.2010.09.012
Dunlosky, J., Hertzog, C., Kennedy, M. R. T., and Thiede, K. W. (2005). The self-monitoring approach for effective learning. Cognit. Technol. 9, 4–11.
Eitel, A., and Kühl, T. (2016). Effects of disfluency and text expectancy on learning with text. Meta. Learn. 11, 107–121. doi: 10.1007/s11409-015-9145-3
Eitel, A., Kühl, T., Scheiter, K., and Gerjets, P. (2014). Disfluency meets cognitive load in multimedia learning: does harder-to-read mean better-to-understand? Appl. Cogn. Psychol. 28, 488–501. doi: 10.1002/acp.3004
Faul, F., Erdfelder, E., Lang, A.-G., and Buchner, A. (2007). G*Power 3: a flexible statistical power analysis program for the social, behavioral, and biomedical sciences. Behav. Res. Methods 39, 175–191. doi: 10.3758/BF03193146
Jarosz, A., and Wiley, J. (2014). What are the odds? A practical guide to computing and reporting bayes factors. J. Probl. Solv. 7, 1–9. doi: 10.7771/1932-6246.1167
Kintsch, W. (1994). Text comprehension, memory, and learning. Am. Psychol. 49, 294–303. doi: 10.1037/0003-066X.49.4.294
Koriat, A., Ma'ayan, H., and Nussinson, R. (2006). The intricate relationships between monitoring and control in metacognition: lessons for the cause-and-effect relation between subjective experience and behavior. J. Exp. Psychol. Gen. 135, 36–69. doi: 10.1037/0096-3445.135.1.36
Kühl, T., and Eitel, A. (2016). Effects of disfluency on cognitive and metacognitive processes and outcomes. Meta. Learn. 11, 1–13. doi: 10.1007/s11409-016-9154-x
Lehmann, J., Goussios, C., and Seufert, T. (2016). Working memory capacity and disfluency effect: an aptitude-treatment-interaction study. Meta. Learn. 11, 89–105. doi: 10.1007/s11409-015-9149-z
Maki, R. H., Foley, J. M., Kajer, W. K., Thompson, R. C., and Willert, M. G. (1990). Increased processing enhances calibration of comprehension. J. Exp. Psychol. Learn. Mem. Cognit. 16, 609–616. doi: 10.1037/0278-7393.16.4.609
McDaniel, M. A. (1984). The role of elaborative and schema processes in story memory. Mem. Cognit. 12, 46–51. doi: 10.3758/BF03196996
McDaniel, M. A., and Butler, A. C. (2011). A contextual framework for understanding when difficulties are desirable, in Successful Remembering and Successful Forgetting: Essays in Honor of Robert A. Bjork, ed A. S. Benjamin (New York, NY: Psychology Press), 175–199.
McDaniel, M. A., Einstein, G. O., Dunay, P. K., and Cobb, R. E. (1986). Encoding difficulty and memory: toward a unifying theory. J. Mem. Lang. 25, 645–656. doi: 10.1016/0749-596X(86)90041-0
Michalke, M. (2012). koRpus: A R package for text analysis (version 0.04-36). Available online at: http://reaktanz.de/?c=hacking&s=koRpus (Accessed October 30, 2013).
Miele, D. B., and Molden, D. C. (2010). Naive theories of intelligence and the role of processing fluency in perceived comprehension. J. Exp. Psychol. Gen. 139, 535–557. doi: 10.1037/a0019745
Nelson, T. O., and Narens, L. (1990). Metamemory: a theoretical framework and new findings, in The Psychology of Learning and Motivation, ed G. H. Bower (New York, NY: Academic Press), 125–173.
Pieger, E. (2017). Metacognition and Disfluency – The Effects of Disfluency on Monitoring and Performance. [Dissertation]. Julius-Maximilans-University of Wuerzburg. urn:nbn:de:bvb:20-opus-155362.
Pieger, E., Mengelkamp, C., and Bannert, M. (2016). Metacognitive judgments and disfluency – Does disfluency lead to more accurate judgments, better control, and better performance? Learn. Instr. 44, 31–40. doi: 10.1016/j.learninstruc.2016.01.012
Pieger, E., Mengelkamp, C., and Bannert, M. (2017). Fostering analytic metacognitive processes and reducing overconfidence by disfluency: the role of contrast effects. Appl. Cogn. Psychol. 31, 291–301. doi: 10.1002/acp.3326
Rawson, K. A., and Dunlosky, J. (2002). Are performance predictions for text based on ease of processing? J. Exp. Psychol. Learn. Mem. Cogn. 28, 69–80. doi: 10.1037/0278-7393.28.1.69
Redford, J. S., Thiede, K. W., Wiley, J., and Griffin, T. D. (2012). Concept mapping improves metacomprehension accuracy among 7th graders. Learn. Instr. 22, 262–270. doi: 10.1016/j.learninstruc.2011.10.007
Rouder, J. N., Morey, R. D., Speckman, P. L., and Province, J. M. (2012). Default bayes factors for ANOVA designs. J. Math. Psychol. 56, 356–374. doi: 10.1016/j.jmp.2012.08.001
Rudolph, U. (2009). Motivationspsychologie kompakt [Motivational psychology compact], 2nd edn. Weinheim, Beltz PVU.
Rummer, R., Schweppe, J., and Schwede, A. (2016). Fortune is fickle: null-effects of disfluency on learning outcomes. Meta. Learn. 11, 57–70. doi: 10.1007/s11409-015-9151-5
Sanchez, C. A., and Jaeger, A. J. (2015). If it's hard to read, it changes how long you do it: reading time as an explanation for perceptual fluency effects on judgment. Psychon. Bull. Rev. 22, 206–211. doi: 10.3758/s13423-014-0658-6
Schwarz, N. (2010). Meaning in context: Metacognitive experiences, in The Mind in Context, eds B. Mesquita, L. F. Barrett, and E. R. Smith (New York, NY: Guilford), 105–125.
Strukelj, A., Scheiter, K., Nyström, M., and Holmqvist, K. (2016). Exploring the lack of a disfluency effect: evidence from eye movements. Meta. Learn. 11, 71–88. doi: 10.1007/s11409-015-9146-2
Winne, P. H., and Hadwin, A. F. (1998). Studying as self-regulated learning, in Metacognition in Educational Theory and Practice,eds D. J. Hacker, J. Dunlosky, and A. C. Graesser (Hillsdale, NJ: LEA), 277–304.
Xie, H., Zhou, Z., and Liu, Q. (2018). Null effects of perceptual disfluency on learning outcomes in a text-based educational context: a meta-analysis. Educ. Psychol. Rev. 30, 745–771. doi: 10.1007/s10648-018-9442-x
Zimmerman, B. J. (1990). Self-regulating academic learning and achievement: the emergence of a social cognitive perspective. Educ. Psychol. Rev. 2, 173–201. doi: 10.1007/BF01322178
Appendix
Keywords: metacomprehension, disfluency, metacognitive monitoring, metacognitive control, metacognitive judgments, desirable difficulties
Citation: Pieger E, Mengelkamp C and Bannert M (2018) Disfluency as a Desirable Difficulty—The Effects of Letter Deletion on Monitoring and Performance. Front. Educ. 3:101. doi: 10.3389/feduc.2018.00101
Received: 18 June 2018; Accepted: 29 October 2018;
Published: 22 November 2018.
Edited by:
Tobias Richter, Universität Würzburg, GermanyReviewed by:
Anique De Bruin, Maastricht University, NetherlandsAlexander Eitel, Albert-Ludwigs-Universität Freiburg, Germany
Copyright © 2018 Pieger, Mengelkamp and Bannert. This is an open-access article distributed under the terms of the Creative Commons Attribution License (CC BY). The use, distribution or reproduction in other forums is permitted, provided the original author(s) and the copyright owner(s) are credited and that the original publication in this journal is cited, in accordance with accepted academic practice. No use, distribution or reproduction is permitted which does not comply with these terms.
*Correspondence: Christoph Mengelkamp, Y2hyaXN0b3BoLm1lbmdlbGthbXBAdW5pLXd1ZXJ6YnVyZy5kZQ==