- 1CIPER, Faculdade de Motricidade Humana, Universidade de Lisboa, Lisbon, Portugal
- 2Centre for Research and Technology Hellas, Information Technologies Institute, Thessaloniki, Greece
- 3Laboratory of Medical Physics, Aristotle University of Thessaloniki, Thessaloniki, Greece
- 4Department NetMedia, Fraunhofer Institute IAIS, Sankt Augustin, Germany
- 5PLUX, Wireless Biosignals, Lisbon, Portugal
- 6Department of Biosciences and Nutrition, Karolinska Institutet, Huddinge, Sweden
- 7Elliniko Kentro Kenotomias Microsoft, Athens, Greece
- 8AGE Platform Europe, Brussels, Belgium
- 9COSMOTE Kinites Tilepekoinonies AE, Athens, Greece
- 10Multimedia Understanding Group, Information Processing Laboratory, Deptartment of Electrical and Computer Engineering, Aristotle University of Thessaloniki, Thessaloniki, Greece
- 11International Parkinson Excellence Research Centre, King's College Hospital NHS Foundation Trust, London, United Kingdom
- 12Department of Neurology, Technical University Dresden, Dresden, Germany
- 13Third Neurological Clinic, G. Papanikolaou Hospital, Thessaloniki, Greece
- 14Department of Electrical and Computer Engineering, Aristotle University of Thessaloniki, Thessaloniki, Greece
- 15Department of Electrical and Computer Engineering, Khalifa University of Science and Technology, Abu Dhabi, United Arab Emirates
Being the second most common neurodegenerative disease, Parkinson's disease (PD) can be symptomatically treated, although, unfortunately, it cannot be cured yet. Moreover, diagnosing and assessing PD patients is a complex process, requiring continuous monitoring. In this vein, the design, development, and validation of innovative assessment tools may be helpful in the management of patients with PD, in particular. Based on intelligent ICT interventions, the i-PROGNOSIS project intends to mitigate PD's specific symptoms, such as neurological movement disorders of gait, balance, coordination, and posture, already characterized in the early phase of the disease. From this perspective, an innovative iPrognosis motor assessment tool is presented here, taking into consideration the Unified Parkinson Disease Rating Scale (UPDRS) Part III motor skills testing items, for evaluating the motor skills status. The efficiency of the proposed Assessment Tests to reflect the motor skills status, similarly to the UPDRS Part III items, was validated via 27 participants (18 males; mean age = 62 years, SD = 10.36 years; range, 43–79 years) with early (n = 10) and moderate (n = 17) PD who performed the Assessment Tests. Features from the latter were then correlated with the corresponding clinically assessed UPDRS Part III items, and statistically significant negative correlations (range, −0.364 to −0.802) were identified between the median values of the Assessment Tests and the UPDRS Part III items. In this vein, the iPrognosis Assessment Tests were integrated within the personalized interventions of the i-PROGNOSIS project, providing alternative means of assessing their effect on the PD patient's motor skills enhancement. The promising results presented here elaborate on the concept of using ICT-based assessment means to achieve comparable outcomes with the clinical standards in motor skills assessment.
Introduction
Parkinson's disease (PD) is one of the commonest neurodegenerative diseases, affecting ~1% of individuals older than 60 years and 2–4% over than 75 years, causing progressive disability that results in a burden of ~2.2 million disability-adjusted life years (DALYs), exhibiting the greatest loss of quality-adjusted life years (QALYs) among 29 major chronic conditions (Dorsey et al., 2007; Tanner et al., 2008). For the management of the PD, physiotherapists and/or occupational therapists have had an important role in improving functional activity of the patients (Keus et al., 2006). In addition, physical therapy can be oriented to address loss of mobility, falls, difficulties in reaching and manipulating objects, as well as self-care activities, such as eating and dressing (Morris, 2000; Keus et al., 2006). On the other hand, as part of the (motor) assessment process, clinicians usually gather information about impairments and physical activity limitations to inform treatment decisions based on standardized measurement tools. These are disease-specific measures, such as the Unified Parkinson's Disease Rating Scale (UPDRS) (Martinez-Martin and Forjaz, 2006) and the Parkinson's Disease Questionnaire-39 (PDQ-39) (Peto et al., 1995), which include test items as part of a total composite score, reflecting overall disability (UPDRS) or quality of life (PDQ-39) of the patients. However, a combination of standardized and non-standardized methods can be used to holistically collect this information (Bernhardt and Hill, 2005; Fawcett, 2007).
In clinical practice, the assessment of PD severity mostly depends on clinicians' judgement, supported by common rating scales, such as UPDRS; nevertheless, the strong dependence on clinicians' experience makes the assessment essentially subjective. In the case where objective measurements exhibit high correlation with the commonly accepted “gold standard”—UPDRS overall or subscores, it is reasonable to accept that an objective measuring system can be seen a useful alternative to the existing rating scales in the progression monitoring of PD (Yang et al., 2016). Nowadays, assessing the impact of medication and behavioral changes in the motor condition of the PD patients mainly depends on the diaries and memory of the PD patients, which are neither objective nor reliable. Additionally, Utsumi et al. (2012) concluded that subjective assessment of PD patients does not necessarily match the findings of quantitative objective assessment in PD with gait disorders, suggesting that objective long-term monitoring system would be helpful. In fact, many low-cost, body-tracking systems have been employed in the health care environments; for instance, the Microsoft Kinect® sensor has been used for neurological rehabilitation (Knippenberg et al., 2017), for assessing body balance and preventing falls (Yang et al., 2014; Stone and Skubic, 2015), for clinical measurement of motor functions (Otte et al., 2016), for monitoring people with PD (Galna et al., 2014), for PD gait assessment (Rocha et al., 2015), for PD hand tracking (Ferraris et al., 2014), and for analyzing PD posture and lower limb tasks (Ferraris et al., 2019). However, in the fall of 2017, the manufacture of the Kinect sensor was discontinued1. As an alternative solution to the Kinect device, since its creation in 2015, the use of the MentorAge® sensor tracking system has proven its capabilities and potentialities in real-life scenarios (e.g., Anzivino et al., 2019; Petsani et al., 2019).
In this vein, and motivated by the aforementioned perspectives, an innovative and personalized motor assessment tool capable of monitoring and tracking the behavioral change of PD patients (mostly related to posture, walking/gait, agility, balance, and coordination impairments) is presented here, within the context of the i-PROGNOSIS framework (www.i-prognosis.eu) that aims to develop early and unobtrusive PD detection and intervention based on the interaction of the users with intelligent devices.
Background
Motor Assessment in PD
Clinimetrics, seen as a set of rules behind indexes (Fava et al., 2012), can result in a difficult and complex task, since the assessment of PD patients is mainly subjective. Overall, the basic motor symptoms of PD (e.g., bradykinesia, posture instability, lack of arms co-movements, handwriting difficulties, rigidity in the muscles, tremor appearance) can hardly be assessed. Moreover, the symptoms variability due to collateral effects from drugs, such as Levodopa, sets an extra difficulty. Hoehn and Yahr (1967) proposed the first PD scale using observational data from 856 patients. In fact, they distinguished five stages of injury/disability corresponding to five disease progression stages from Stage I: as unilateral damage without disorders, to Stage V: patient is confined in a wheelchair or bed. Later, Fahn and Elton (1987) proposed the most often used Unified Parkinson's Disease Rating Scale (UPDRS), with six integral different parts, i.e., (1) the state of intellectual and mood disorders (Part I); (2) activities of daily living (separately for phase “on” and “off”) (Part II); (3) motor examination (Part III); (4) complications of treatment (Part IV); (5) stages of the disease (Part V); and (6) self-assessment of independence using the Schwab-England Scale (Part VI). The evaluation is based on a Likert scale (0–4), in terms of increasing severity in disorder from “No involvement” (0) up to “No function or Severe disorders” (4). UPDRS Part III relates with the motor skills and assesses the speech, faces, tremor, rigidity, rapid movements of the fingers, rapid hand movements, alternating movements, leg movements, getting up from a chair, posture, stability of posture, starting walking, and bradykinesia. In fact, the motor examination part is the only component of the UPDRS where items are scored by the physician instead by patient self-report. In Goetz et al. (2008), presented a modified UPDRS, termed as revised MDS-UPDRS, consisting of 65 items, containing 48 items ranging from 0 to 4, and 7 options with answers “yes” or “no”; however, the old versions of the Hoehn and Yahr scale and UPDRS are still in usage. Furthermore, the motor assessment and corresponding tests of balance and posture, arm and hand function, and gait/walking for PD have been extensively explored, as described below:
• Balance/posture in PD: Statistical data reveal that falls affect more than 50% of PD patients (Bloem et al., 2001; Canning et al., 2014; Opara et al., 2017). To assess the balance and control of posture, the Timed Up and Go Test (TUG) is frequently used (Podsiadło and Richardson, 1989) to measure the time that is needed for the patient to get up from a chair, walking to a line on the floor 10 feet, turn, walk back to the start point, and then sit down. Morris et al. (2001) assessed the appropriateness of TUG for identifying changes in mobility of PD patients and its usefulness was confirmed. In addition, posturometric and stabilometric evaluation has been used for assessing balance training (van der Burg et al., 2006). Song et al. (2012) concluded that compared to healthy controls, people with early-stage PD altered their postural control strategies (i.e., shorter distance between the center of pressure and the extrapolated center of mass) during the step turn and seem to decrease their general movement amplitude, suggesting that dynamic postural control during turning is altered, even in the early stages of PD. Overall, the most commonly used tests for assessment of balance and posture in PD are the following: UPDRS part III (Fahn and Elton, 1987), the TUG (Podsiadło and Richardson, 1989), the Berg Balance scale (Berg et al., 1992), the Tinetti Balance and Gait Assessment Tool (Tinetti, 1986), Brunel Balance Assessment (Tyson and DeSouza, 2002), Functional Reach Test (Behrman et al., 2002), Activity Specific Balance Confidence (Powell and Myers, 1995), Balance Evaluation Systems Test (Horak et al., 2009), and Balance Evaluation Systems ib32Mini Test (Franchignoni et al., 2010).
• Arm/hand function in PD: Arm and hand dysfunction are common findings in patients with neurological movement disorders; overall, motor dysfunction compromises the efficiency of grip force scaling during object handling among patients with PD and focal hand dystonia (Agostino et al., 2003; Uitti et al., 2005; Bleton et al., 2014). Motor dysfunctions/disorders of the hand, in particular, is expressed via upper extremity clumsiness, fine motor problems, poor manual dexterity, incapacity to control grip force output, as well as difficulty in reach to grasp movements regarding amplitude, speed, and coordination (Pradhan et al., 2015). The most commonly used tests for assessment arm and hand movements in PD are the following: the UPDRS Part II and III (Fahn and Elton, 1987), Purdue Pegboard Test (Tiffin and Asher, 1948), Nine-Hole Peg Test (Kellor et al., 1971), Jebsen and Taylor test (Jebsen et al., 1969), Frenchay Arm Test (Wade et al., 1983), Action Research Arm Test (Lyle, 1981; van der Lee et al., 2002), Wolf Motor Function Test (Wolf et al., 1989), Fugl-Meyer Motor Assessment Scale (Fugl-Meyer et al., 1975), Södring Motor Evaluation (Sødring, 1994), and the Finger-Tapping Test (Shimoyama et al., 1990).
• Walking in PD: PD patients have a significantly slower speed of walking and reduced step length in comparison to controls (Rochester et al., 2004). Including a total of 153 PD patients, Elbers et al. (2013) conducted a study to investigate the predictive value of gait speed for community walking in PD and to develop a multivariate prediction model for community walking. The authors concluded that timed walking tests are valid measurements to predict community walking in PD; however, the evaluation of community walking must consider an assessment of fear of falling. In another study, King et al. (2013) studied which outcome measures are sensitive to exercise intervention and to explore the effects of different exercise programs (i.e., Agility Boot Camp and Treadmill training; 4 × /week for 4 weeks) in order to enhance mobility in PD patients. The results suggest that future randomized clinical trials of mobility intervention must include objective measures of balance and gait at the body structure/function level of the International Classification of Functioning (ICF) to discriminate between two types of physical therapy intervention with reasonable size groups. More recently, Schlachetzki et al. (2017) developed a wearable sensor-based gait analysis system, which consists of inertial sensor units attached laterally to both shoes, as a diagnostic tool that could objectively assesses gait in PD. The results revealed that wearable sensor-based gait analysis reaches clinical applicability and can provide a high biomechanical resolution for gait impairment in PD. Moreover, the most commonly used tests for assessment of walking in PD are the following: UPDRS Part II and III (Fahn and Elton, 1987), Time 10-Meter Walk Test (Bohannon et al., 1996), Time 20-Meter Walk Test (Cunningham et al., 1982), 2-min Walk Test (Butland et al., 1982), 6-min Walk Test (Balke, 1963), Functional Ambulation Category (Holden et al., 1984), and Emory Functional Ambulation Profile (Wolf et al., 1999).
• Leg Agility in PD: Several works have been focusing on the evaluation of the performance of specific motor tasks, such as gait analysis and tremor; yet, limited attention has been devoted to the evaluation of the leg agility in PD, with specific focus on the lower limbs (Giuberti et al., 2014). Leg agility is an item in the UPDRS; however, just a visual detection of the related features is commonly used, leading once again to subjectivity (Ornelas-Vences et al., 2019). Based on the recommendations of the Movement Disorder Society (MDS), the leg agility task should be assessed by observing different parameters, namely, amplitude, slowing, hesitations, interruptions, and freezing. The parameter related to the amplitude of movements directly corresponds to a physical measure, and the quantitative evaluation of the other parameters normally depends on the experience of the neurologists; as a result, inter-neurologist score variations cannot be excluded (Giuberti et al., 2014).
• Visuo-motor coordination (VMC) in PD: Due to the inability of the body to coordinate movements in PD, it progressively becomes more demanding to maintain adequate posture and coordination during walking. Moreover, the intact capability to initiate grip and load forces simultaneously and to adapt the force level during precision grip is as interesting as the impairments, since it has been reported that patients with PD have difficulties coordinating two movements simultaneously (Schwab et al., 1954; Benecke et al., 1986; Ingvarsson et al., 1997). By definition, VMC in particular requires normal cognitive executive functionality, a capability to transform visual inputs into movement plans and motor-execution skills (Inzelberg et al., 2008). Moreover, it is well-reported that VMC is deficient in patients with PD (Flowers, 1978; Stern et al., 1983; Johnson et al., 1996), in early PD patients (Cooper et al., 1991; Hocherman and Giladi, 1998), as well as in moderate (Hocherman and Aharon-Peretz, 1994) and advanced PD patients, and it appears to pertain to a high level linkage between perception and action (Fucetola and Smith, 1997). Additionally, strong correlations were found between the UPDRS gait/posture items and the measures of directional control in VMC (Inzelberg et al., 2008).
To sum up, motor assessment of PD patients, in particular, can be divided into different types of clinimetrics indexes, e.g., assessment of balance/posture, arm/hand function, and gait/walking; however, it is clear that the most used in the clinical practice are the H&Y stages of progression of the disease and the UPDRS.
Innovative Technologies for Motor Assessment in PD
An exponential increase in the use of innovative technology in the healthcare sector has been reported in recent years. More specifically, in the area of neurological disease, research is being conducted into novel assessment and treatment technologies based on motion analysis, robotics, virtual reality, and telerehabilitation fields. However, these innovative and intelligent solutions are often very costly, limiting their use in clinical practice, regardless of their effectiveness. In PD, possible uses of innovative devices and solutions include (i) increase in the collaboration between multidisciplinary teams, in order to reduce time and distance limitations in communication between patients and healthcare professionals, and (ii) the development of systems for tracking and monitoring patient's status progression.
Furthermore, in the last decades, researchers have developed several non-invasive, objective methods for detecting early symptoms of PD by using physiological biomarkers, including electromyography (EMG) (Ruonala et al., 2013) and electroencephalogram (EEG) (Handojoseno et al., 2012) signals, techniques based on 3D motion analysis or imaging modalities [computed tomography (CT) scans, magnetic resonance imaging (MRI), and depth sensors] (Burrus et al., 1997; Long et al., 2012; Stawarz et al., 2012; Procházka et al., 2015), and techniques to examine motion signals by using unimodal wearable sensors (Patel et al., 2009; Cancela et al., 2011). Moreover, an ideal home-based monitoring intelligent system should (i) record movement data continuously over a long-term period, (ii) include enough portability to avoid interfering with the daily activities of the PD patients, (iii) be able to test PD patients in a home-based and unsupervised environment, (iv) reveal the real motor condition of the PD patients (for instance, to work as a remote UPDRS assessment), and (v) have the ability to capture the occurrence of PD patients' motor fluctuations (Yang et al., 2016).
Overall, this diversity of objective assessments can be seen as favorable tools/techniques that allow long-term home-based monitoring, having the possibility of improving the medical standards, delivering healthcare and, probably, becoming an effective and cost-saving procedure in PD progression. In the succeeding section, an unobtrusive ICT-based PD early detection and related risk-reduction intervention, namely, i-PROGNOSIS approach, is presented in detail.
The i-prognosis Paradigm
Overall Concept
The cardinal objective of the i-PROGNOSIS ecosystem (www.i-prognosis.eu) is the development of (i) an ICT-based behavioral analysis approach for capturing, as early as possible, the PD symptoms appearance, and (ii) the application of ICT-based interventions countering identified risks. To achieve this, awareness initiatives have been employed, resulting in i-PROGNOSIS community, targeting older individuals, in order to unobtrusively sense large-scale behavioral data from its members, acquired from their natural use of mobile devices (smartphone/smartwatch). Ensuring anonymization and secure Cloud archiving, i-PROGNOSIS developed and employed advanced big data analytics and AI-based techniques, in a distributed and privacy aware fashion, instantiating a PD behavioral model and constructing reliable early PD symptoms detection alarms. The data handling includes pseudo-anonymization, secure transmission, and storage of data. The user ID and the actual data are stored in separate places via secure methods, so that collected data cannot be traced back to a single individual without the act of the authorized data administrator and/or authenticated medical doctor. i-PROGNOSIS project was based on the privacy-by-design approach following the European General Data Protection Regulation (EU GDPR) restrictions (https://gdpr-info.eu/). The selected Microsoft Azure Cloud environment has full GDPR compliance.
To those identified and clinically validated as early-stage PD patients, ICT-based interventions are provided via the i-PROGNOSIS Intervention Platform, including: (i) a Personalized Game Suite (ExerGames, DietaryGames, EmoGames, and Handwriting/VoiceGames) for physical/emotional support, (ii) targeted nocturnal intervention to increase relaxation/sleep quality, and (iii) assistive interventions for voice enhancement and gait rhythm guidance. In this way, i-PROGNOSIS constructively uses the AI in order to contribute to active and healthy aging.
Furthermore, the main innovative elements of i-PROGNOSIS that make it unique compared to the competition are (i) the introduction of new diagnostic tests for early PD symptoms based on features extracted from securely Cloud-stored behavioral and sensorial data, collected by smart devices (e.g., smartphone, smartwatch), wearable biosensors, and IoT-based everyday living sensorial artifacts, and processed by advanced big data analytics and AI-based techniques; (ii) design and implementation of novel ICT-based adaptive, gamified, and personalized interventions, along with assistive interventions, taking into account older adults' physical and psychological status, promoting his/her health self-management at the family setting by providing dynamic feedback toward the improvement of older adult's skills and functionalities for reduction of the PD-related risks of frailty, depression, and falls; and (iii) fostering of social awareness for volunteerism in early PD detection and construction of socio-economic and informed behavioral models for new cost-effective ICT-based PD early detection and related risk-reduction intervention practices and policies for the sustainability of health and care systems and the benefit of the older adults (see Figure 1). i-PROGNOSIS leverages and extends the state of the art in a number of different areas, such as behavioral, physiological and lifestyle monitoring, motion capture, physical activity evaluation, personalized gaming, home-based human–computer interfaces, multi-parametric data modeling, and decision support systems, ensuring valuable intellectual property. For the present study, part of the i-PROGNOSIS ecosystem will be presented and discussed, namely, the iPrognosis Assessment Tests included in the Personalized Game Suite (see Figure 1), also referred to as iPrognosis Games.
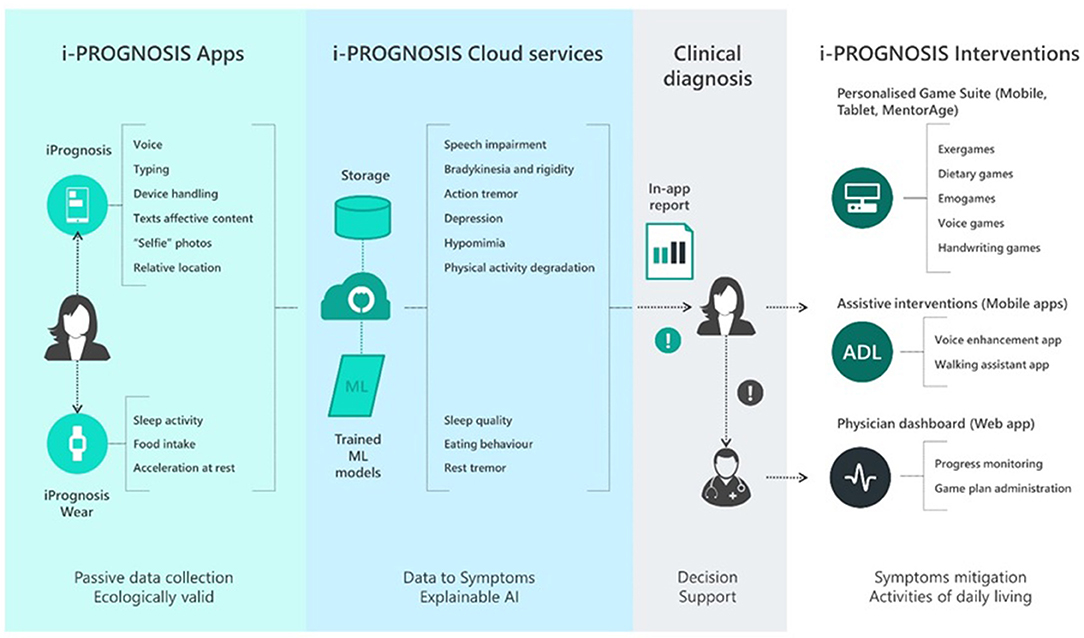
Figure 1. The i-PROGNOSIS ecosystem. AI, Artificial Intelligence; ML, Machine Leaning; ADL, Activities of Daily Living.
The iPrognosis Games
The iPrognosis Games application, an Android mobile application, already available on Google Play Store, consists of 14 different games, taking into account the PD symptoms (i.e., Exergames, Dietarygames, Emogames, Handwriting, and Voicegames), constituting the Personalized Game Suite (PGS), along with the Warming up game and motor Assessment Tests.
The developed battery of 14 interventional and quality-of-life improving games are based on the following PD-targeted symptomatology: (1) Motor improvement (six games): posture, gait, postural instability, fine motor skills impairment, and tremor; (2) Non-motor improvement (five games): facial expression/depression and constipation; and (3) Speech-improvement (three games): voice and speech difficulties. More detailed information about the design and development of the games can be found in Dias et al. (2016, 2017a,b, 2018), Savvidis et al. (2018, 2019), and Grammatikopoulou et al. (2019). The iPrognosis Games differ in the required equipment for playing them, which also defines different target uses; more specifically, (i) games requiring the MentorAge®2 sensor device (four games) are targeted to be used inside specialized PD clinical centers or at home if the user purchases the sensor device (~€700), and (ii) games requiring a smartwatch or tablet (with microphone and frontal camera) (10 games) can be played either at a home environment or under supervision in clinical settings.
Tackling the most often appearing PD symptoms via the gamification concept, iPrognosis Games can provide a user-friendly environment with bilateral functionality, i.e., both engaging the user into long-lasting supportive activities, which could retard the evolution of the symptoms, and providing the physician with necessary information for monitoring the PD patient's status in a quantitative and detailed way.
The iPrognosis Motor Assessment Tests
In order to evaluate the effect of the iPrognosis Games on the PD patient's status, a set of Assessment Tests that accompany the iPrognosis Games has been constructed. It is important to note that all motor aspects related to these Assessment Tests are reflected in multiple ways in the general design and development of the different games included in the iPrognosis Games. In this way, the Assessment Tests and the iPrognosis Games can be seen as a holistic alternative to the PD patient's conventional status monitoring and intervention processes, aiming at optimizing the improvement of his/her quality of life by increasing his/her QALYs.
The design of the proposed motor Assessment Test was informed by expert neurologists coming from three European medical centers (Greece, UK, and Germany). Six different motor Assessments Tests (i.e., Test 1–Test 6) integrated in the iPrognosis Games were designed and developed based on the UPDRS Part III examination (mostly related to motor postural, balance, agility, coordination, and hand movements), namely, items 18, 23, 24, 25, 26, and 28; Table 1 provides an analytical description of each Assessment Test.
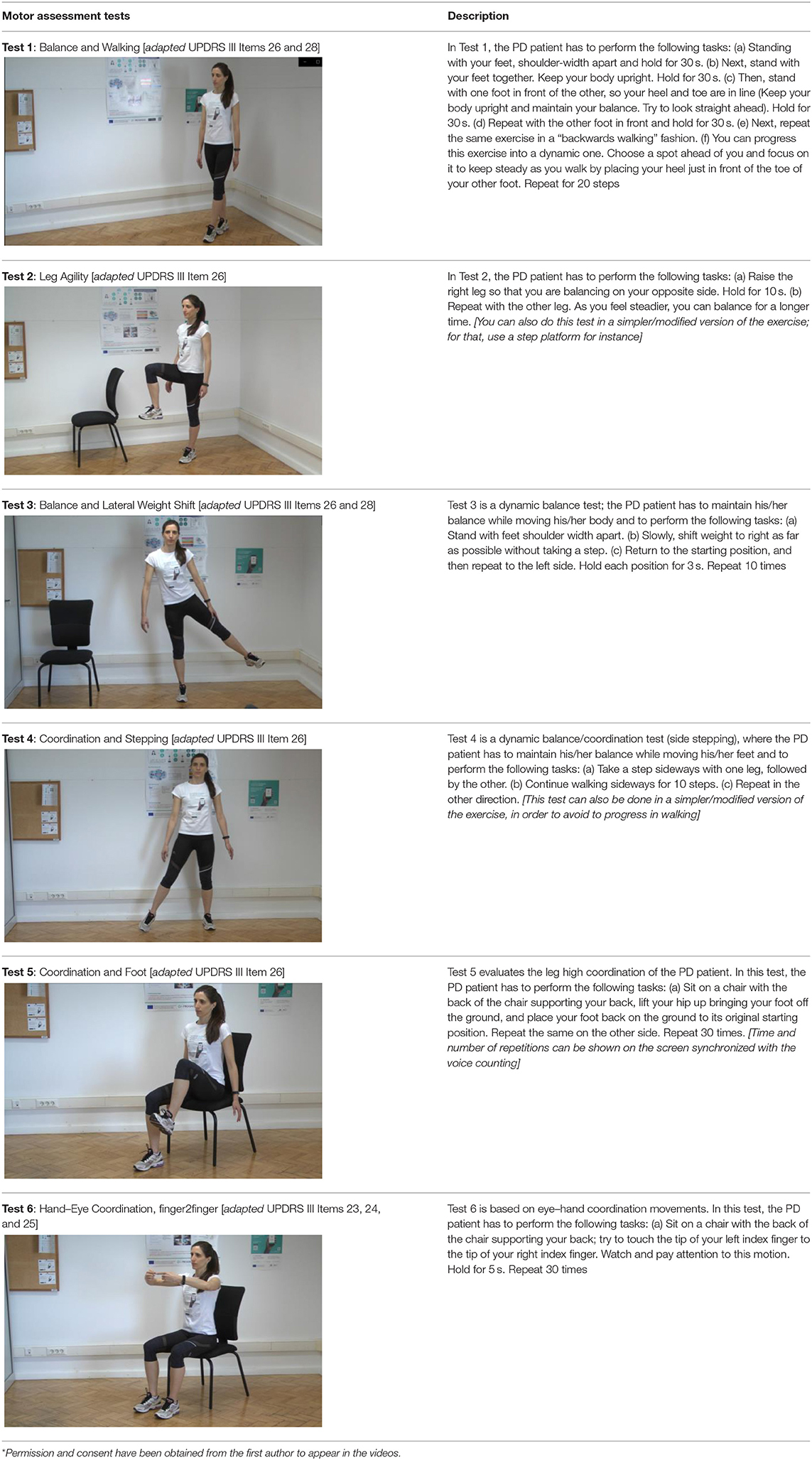
Table 1. Description of the Assessment Tests (Test 1–Test 6) of the iPrognosis Games based on the UPDRS Part III motor items.
User Interface of the Assessment Tests
The setting of the user interface (UI) of the Assessment Tests of the iPrognosis Games environment is depicted in Figure 2. As it is clear from the latter, it is possible to select one of the six aforementioned motor Assessment Tests out of a dropdown menu and push play button (top left), in order to view the corresponding tutorial video. Moreover, while PD patients watch the videos, they can synchronously perform the selected Assessment Test and receive an instant, real-time, evaluation after its completion (see Figure 2). The UI of the motor Assessment Test presents the user's performance on the right side and the expert's performance on the left, so the players can easily synchronize their movement along with the expert's and detect misfires (see Figure 2).
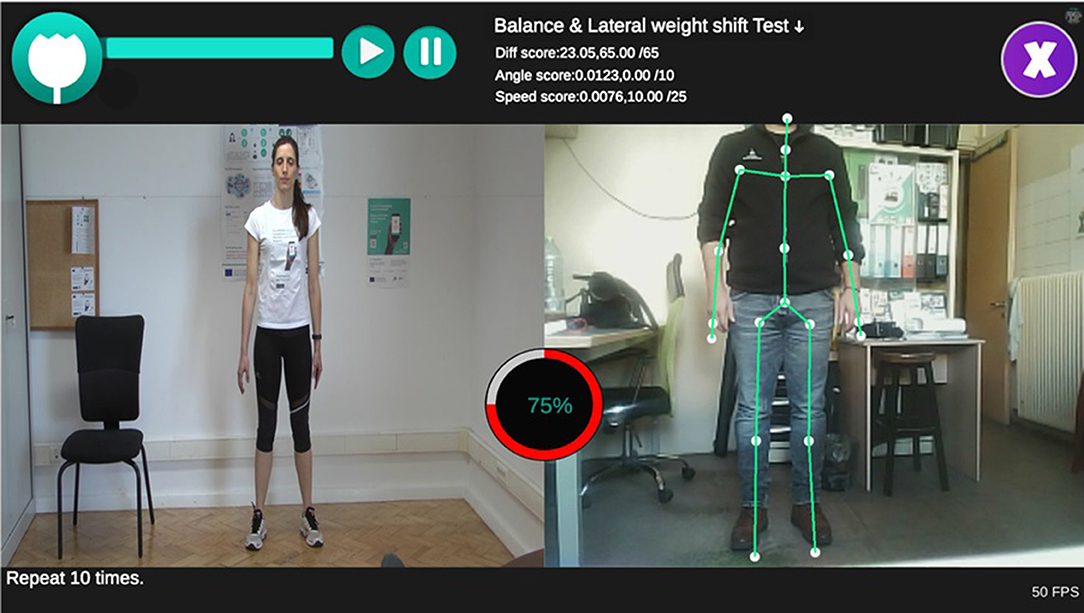
Figure 2. User Interface of the Assessment Test 1(Balance and Walking) of the iPrognosis Games. The user's performance (on the right side) and the expert's performance (on the left side), so the players easily synchronize their movement along with the expert's movement. The general score of the user (75%) (represented by red circle on the center of the panel) is displayed in real-time, as well as intermediate metrics (i.e., Diff score, Angle score and Speed score) (on the top). The MentorAge® tracking system sensor allows to display the skeleton of the user and corresponding joints (right panel). [Permission and consent have been obtained from the first author to appear on the image (on the left)].
During the realization of each motor Assessment Test, PD patients can receive helpful guidelines and instructions to execute the tests in a successful manner, and they can also follow its progress via the slider on the top left of the screen, informing them about the time left to finish the selected Assessment Test (see Figure 2).
Assessment Tests Motor Score Calculation
For the evaluation of the patient's performance, the iPrognosis Assessment Tests environment analyzes the movement/action of the PD patient and estimates an assessment Motor Score (MS) related to the metrics/features of each Assessment Test. More specifically, the skeletal data (i.e., the 3D coordinates of each joint of the skeleton) of the user (PD patient) are initially estimated and then normalized to be independent of the user's height and/or his/her position from the MentorAge® sensor. For each out of six Assessment Tests of the iPrognosis Games, two types of joints (or more) are selected in order to perform a comparison between the performance of the PD patient and the recorded experts' performance (MentorAge® motion data were received during the performance of the Assessment Tests). For each Assessment Test performance, three parameters of movement are taken into consideration regarding the selected joints, namely, Accuracy, Speed, and Angle. These were selected so as to extract clinically interpretable indices based on the clinical routine followed by the clinicians during the UPDRS Part III items marking; they actually are focused on speed, halts, hesitations, and amplitude variations of each movement. All the latter are reflected in the way accuracy, speed, and angle are used and incorporated in the final score, as explained below. Moreover, the selection of only those specific parameters offered to the patients substantial indications on how to improve themselves in future efforts, and at the same time, not overwhelm them with information.
For the Accuracy parameter, the movement of the following selected joints is verified for every received skeleton frame, namely:
• Assessment Test 1 (Balance and Walking): Comparison of the Euclidian distance between the right (AR) and left (AL) ankle joint movement in the x and z vectors for both the patient's and the physician's movement:
• Assessment Test 2 (Leg Agility): Comparison of the Euclidian distance between the right and left ankle joint in the y vector for both the patient's and the physician's movement:
• Assessment Test 3 (Balance and Lateral Weight Shift): Comparison of the Euclidian distance between the right and left ankle joint in x, y, and z vectors for both the patient's and the physician's movement:
• Assessment Test 4 (Coordination and Stepping): Comparison of the Euclidian distance between the right and left ankle joint in the x and z vectors for the patient's and the physician's movement:
• Assessment Test 5 (Coordination and Foot): Comparison of the Euclidian distance between the right (KR) and left knee (KL) joint in the y vector for both the patient's and the physician's movement:
• Assessment Test 6 (Hand–Eye Coordination): Comparison of the Euclidian distance between the right and left wrist joint and the neck (NC) joint in the y vector for both the patient's and the physician's movement:
Taking into consideration the possibility of frame rate fluctuations, the timestamp of each received skeleton frame is also recorded. In addition, the aforementioned Euclidian metrics are being calculated for each received skeleton frame for both the patients and physician's movements and the distance metrics are being added for each second of each Assessment Test. In this way, the patient's and physician movement difference is being compared per sec, which is being added to the final difference score.
For the Speed parameter, the average speed value of selected joints was calculated per 2 s and compared to physician's corresponding average speed values. Specifically:
• Test 1 (Balance and Walking Test): Comparison of the average speed of left and right ankle in the z vector,
• Test 2 (Leg Agility): Comparison of the average speed of left and right ankle in the y vector,
• Test 3 (Balance and Lateral Weight Shift): Comparison of the average speed of left and right ankle in the y vector,
• Test 4 (Coordination and Stepping): Comparison of the average speed of left and right ankle in the x vector,
• Test 5 (Coordination and Foot): Comparison of the average speed of left and right knee in the y vector, and
• Test 6 (Hand–Eye Coordination): Comparison of the average speed of left and right wrist in the y vector.
Similarly, regarding the Angle parameter, the average angle values for the aforementioned selected joints were calculated per 2 s and compared to the physician's corresponding average angle values.
Finally, for each Assessment Test of the iPrognosis Games, a series of testing took place before a lower and upper threshold were established regarding the final accuracy, speed, and angle subscores for evaluating the success of the patient's performed test. In this vein, the three generated metrics, namely, accuracy, speed, and angle score, formed the final score outcome according to the following equation:
where wi, i = 1, 2, 3, denote the weight factors used for each subscore in the calculation of the FinalMotorScore. Here, the weighting set of [65, 25, 10] was adopted, as the AccuracyScore was considered most relevant to measure the overall degree of success of the patient's performance. Then, the SpeedScore was weighted more than the AngleScore, as, for the specific Assessment Tests, improving the synchronization with the expert's movements was considered as more important than matching joint angles with those of the expert.
An example of the AccuracyScore estimation of the Assessment Test 1 (Table 1: Balance and Walking) is depicted in Figure 3. In the latter, the results from the expert and three users are presented, and for each one, the distance between user's ankles is estimated [see Equation (1)] and the produced one-dimensional time series is compared against that of the expert user.
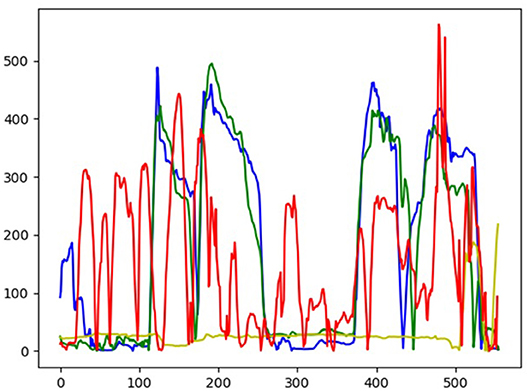
Figure 3. User output from motor Assessment Test 1 (Balance & Walking) related with the distance between user's ankles [see (1)]. The blue color indicates the motion of the expert user, while the lines with the other colors belong to three different users (green: good performance, red: wrong execution, yellow: no execution).
The time series from each user and the expert are of the same size, meaning that the user has to complete the Assessment Test 1 in a specific time interval. Hence, any deviations from the predefined motion of the expert create a measurable difference between the two signals. The mean square error between the two time series is calculated and normalized, providing the AccuracyScore to be inputted in Equation (7). The similar procedure is followed for the estimation of the SpeedScore and AngleScore to be used in Equation (7), so as to produce the FinalMotorScore that is shown to the user via the UI (see Figure 2).
Spiral Development of the iPrognosis Assessment Tests
The Assessment Tests of the iPrognosis Games followed a spiral development model. Initially, they were mainly performed by the i-PROGNOSIS technical partners, who were also developers of the iPrognosis Games. Then, a testing group of five PD patients and five controls performed the Assessment Tests at the North Greece Parkinson's Disease Association (Greece), in order to refine the ongoing technical process and involve the users as “co-creators” of the Assessment Tests. In practice, the co-creation concept adopted here took the form of a collaborative work in which PD patients, exercise professionals, clinicians, researchers, designers, and developers explored and improved the solution together, taking into account their (different) approaches, needs, and perspectives. From this perspective, major and minor enhancements were considered; for instance, from a technical and accessibility point of view, based on user and medical feedback, different languages (i.e., English, Greek and German) were included in the iPrognosis Assessment Tests, as well as the integration of the option of audio captions in the instruction panel of the UI of the Assessment Tests.
Validation of the Iprognosis Assessment Tests
Study Protocol, Participants' Selection, and Clinical Assessments
Twenty-seven patients with PD were successfully enrolled in the validation study of the i-Prognosis Assessment Tests and recruited from three different medical centers [i.e., The Third Neurological Clinic of the Papanikolaou Hospital of Thessaloniki (Thessaloniki, Greece); Department of Neurology of the Technical University Dresden (Dresden, Germany); and Department of Basic and Clinical Neuroscience of the King's College Hospital (London, United Kingdom)], from September 2019 to January 2020. The protocol included periodic sessions (one per month) of the iPrognosis Assessment Tests under the controlled environment at the three medical centers. The PD patients could perform each Assessment Test up to four times per session. At the beginning of each session of the Assessment Tests, an exercise game (ExerGame) was first performed by the PD patients, serving as a warm-up game, mainly to provide the PD patient with an initial contact with the sensor device and related environment and to gradually increase their heart rate and body temperature, including general coordination activities to prepare the PD patients for the preceding Assessment Tests.
The PD patients were selected according to the following inclusion criteria:
1) Diagnosis of idiopathic PD based on the neurologist/movement disorders expert opinion in compliance with the UK brain bank criteria as diagnostic standard criteria for PD;
2) Hoehn and Yahr stages 1 to 3 in the ON state and stages 1 to 4 in the OFF state, and
3) No relevant cognitive impairment (MOCA ≥ 22 points).
Moreover, the exclusion criteria considered for recruitment purposes were the following:
1) Use of a smartphone other than an android smartphone;
2) Not willing to provide an informed consent;
3) Age <40 years or older than 90 years;
4) Relevant cognitive impairment (MOCA < 22 points);
5) Severely disabled persons with expected physical interference when engaging in the intervention study based on the opinion of the principal investigator (e.g., orthopedic diseases, relevant heart insufficiency with reduced capacity for exercise); and
6) Language barrier (meaning that English, German, or Greek language cannot be spoken or understood in an adequate manner).
The majority of the recruited 27 PD patients were male (n = 18, 66.7%). The mean age was 62 years (SD = 10.36 years), ranging from 43 to 79 years. All but one PD patients were under PD medication.
For clinical assessment, the impairment and severity of PD-related symptoms were initially assessed by neurologist/movement disorders experts in the corresponding medical center (in Greece, Germany, and United Kingdom) using the UPDRS part III and H&Y clinical rating scales. In particular, the scores of the UPDRS Part III Item 23 (Finger Taps), Item 24 (Hand Movements), Item 25 (Rapid Alternating Movements of Hands), Item 26 (Leg Agility), and Item 28 (Posture) were assessed based on interview and clinical observation, aiming to compare these values with the relevant results (FinalMotorScore) derived from the Assessment Tests of the iPrognosis Games data [see Equation (7)]. Overall, due to the early-mid stage of the disease, PD patients presented values mostly between 0 and 2 points and rarely values of 3 and 4 points (note that 4 points is the max of the UPDRS symptom severity scale, meaning that higher values represent more severe symptomatology) (see Table 2). Moreover, in order to measure how Parkinson's symptoms progress and the level of disability of the patients, the H&Y scale was used. Most of the PD patients were rated in H&Y mid-stage 3 (n = 17, 63%) (meaning that the PD patient has balance impairment, mild to moderate disease, physically independent), seven patients were rated in H&Y early-stage 1 [meaning that the symptoms were observed on one side only (unilateral)], two patients were rated in H&Y early-stage 2 (meaning that the symptoms were observed on both sides but no impairment of balance), and one patient was rated in H&Y early to mid-stage 2.5 (meaning that mild symptoms on both sides were observed, with recovery when the “pull” test3 was given).
Apparently, when there is new media to use, there are always some transitional effects until the user gets acquainted with the process involved. Here, there were introductory demonstrations to the users and trials until they felt confident that they have understood the process and could follow the guidelines with no any performance issue related to miscomprehension.
The demographics along with their clinical assessment test of the selected participants are tabulated in detail in Table 2.
Data Acquisition Setup
Developed in Unity 3D (https://unity.com/), the i-Prognosis Assessment Tests were applied at the premises of the three medical centers involved in the study to monitor the motor symptoms of the PD patients by acquiring data that are mostly related to posture, walking/gait, agility, balance, and coordination impairments. For that purpose, each medical center involved in the present study was equipped with the depth sensor tracking system Mentorage® and assisted with technical support. The proper position of the PD patient facing the MentorAge® was verified by the neurologists, in order to secure the availability of the whole MentorAge® skeleton and its correct positioning. Overall, the PD patients were able to perform the iPrognosis Assessment Tests by moving in front of the MentorAge® sensor, placed at least 1.5 m in front of it, as it can be seen in Figure 4. MentorAge® is a commercially available depth sensor; it is a plug-and-play Android device, connected via HDMI with a monitor (e.g., TV, projector, computer screen), that is easy to be configured. In this study, the configuration setup was performed by the technical support of each medical center; yet, the whole operational interface and functionality can easily be perceived and followed, after a simple training, by the PD patients. This was verified by the users themselves, who did not report any negative user experience during their interaction with it. The acquired data were de-identified and securely stored in the backend database of the i-PROGNOSIS.
Validation Data Analysis
The FinalMotorScore [see Equation (7)] acquired from the multiple use of the Assessment Tests of the iPrognosis Games by the PD patients were subjected to feature extraction, in order to be compared with the corresponding clinical feature set, i.e., UPDRS Part III item scores [23, 24, 25, 26, 28, their average denoted as Motor Score (MS)] and the Hoehn and Yahr (1967) stage level. According to the structure and definition of the Assessment Tests (see Table 1), the correlation analysis considered the pairs of (T1:T5, {26, 28, MS, HY}) and (T6, {23, 24, 25, MS, HY}), where T1:T6 corresponds to Test 1:Test 6. The Assessment Test feature vector was constructed using the Max, Min, Average, Std, and Median values of the Assessment Tests FinalMotorScore. It was then subjected to Pearson correlation analysis with the corresponding clinical feature set, in order to identify those features that have statistically significant correlation index r and corresponding coefficient of determination (r2). The level of statistical significance for the probability of false alarm p was initially set to a = 0.05, i.e., p < a; then, it was corrected, accordingly, using the Holm–Bonferroni correction method (Holm, 1976). Moreover, linear regression equations were estimated and superimposed on the corresponding scatter plots for those features that exhibit statistically significant r values across multiple comparisons between the FinalMotorScore from the Assessment Tests and clinical feature set. The whole analysis was implemented using Matlab (The MathWorks, Inc., ver. 2020a).
Results and Discussion
Correlation Analysis Results
In Figure 5, the results from the correlation analysis between the data from the Assessment Test of the iPrognosis Games and the data from the medical evaluation are presented, in the form of inactive (p > a; r = 0) and active (p < a; r ∈ [−1, 1]r≠0) elements of the r matrix. Clearly, a different correlation distribution among the features of the Assessment Tests FinalMotorScore and the clinical feature set is noticed. In particular, Figures 5A–E illustrate the significant correlations between the Max, Min, Average, Std, and Median FinalMotorScore values of the Assessment Tests and the clinical feature set, respectively. From Figure 5E, it is apparent that the Median of the FinalMotorScore values of the Assessment Tests is the feature that exhibits the highest number of statistically significant correlations with the clinical feature set, when compared to the correlation analysis results of the other four features (Figures 5A–D). In this vein, Table 3 tabulates the estimated correlation r and coefficient of determination (r2) between the Median of the FinalMotorScore values of the Assessment Tests (T1–T6) and the clinical feature set. The enhanced performance, in terms of higher number of significant correlations, of the Median final score values is due to its insusceptibility to outliers (unlike the average) and ability to efficiently capture the underlying trends in the final score data. From Figure 5E and Table 3, it is apparent that all estimated correlations based on the Median FinalMotorScore values are negative, reflecting the expected relation between the low (high) final score values with the high (low) clinical feature set, as the high (low) values of the clinical features reflect the advanced (early) stage of the PD pathology. Moreover, the highest correlation value was found between T6med and HY [r(T6med,HY) = −0.802], whereas the lowest one was found between T3med and UPDRS Part III Item 27 [r(T3med,27) = −0.329]. In addition, T1med and T3med showed significant correlation with both UPDRS Part III Items 26 and 28; T2med and T4med showed significant correlation with UPDRS Part III Item 28; T5med showed significant correlation with UPDRS Part III Item 26, and T6med showed significant correlation with all related UPDRS Part III Items 23, 24, 25, MS, and HY. From all assessments, T4med results in the weakest correlation with the MS and HY clinical features, as the PD patients exhibited quite high final score T4med values, although their MS and HY scores were medium and/or high, revealing feasibility of PD patients in performing the T4 assessment test.
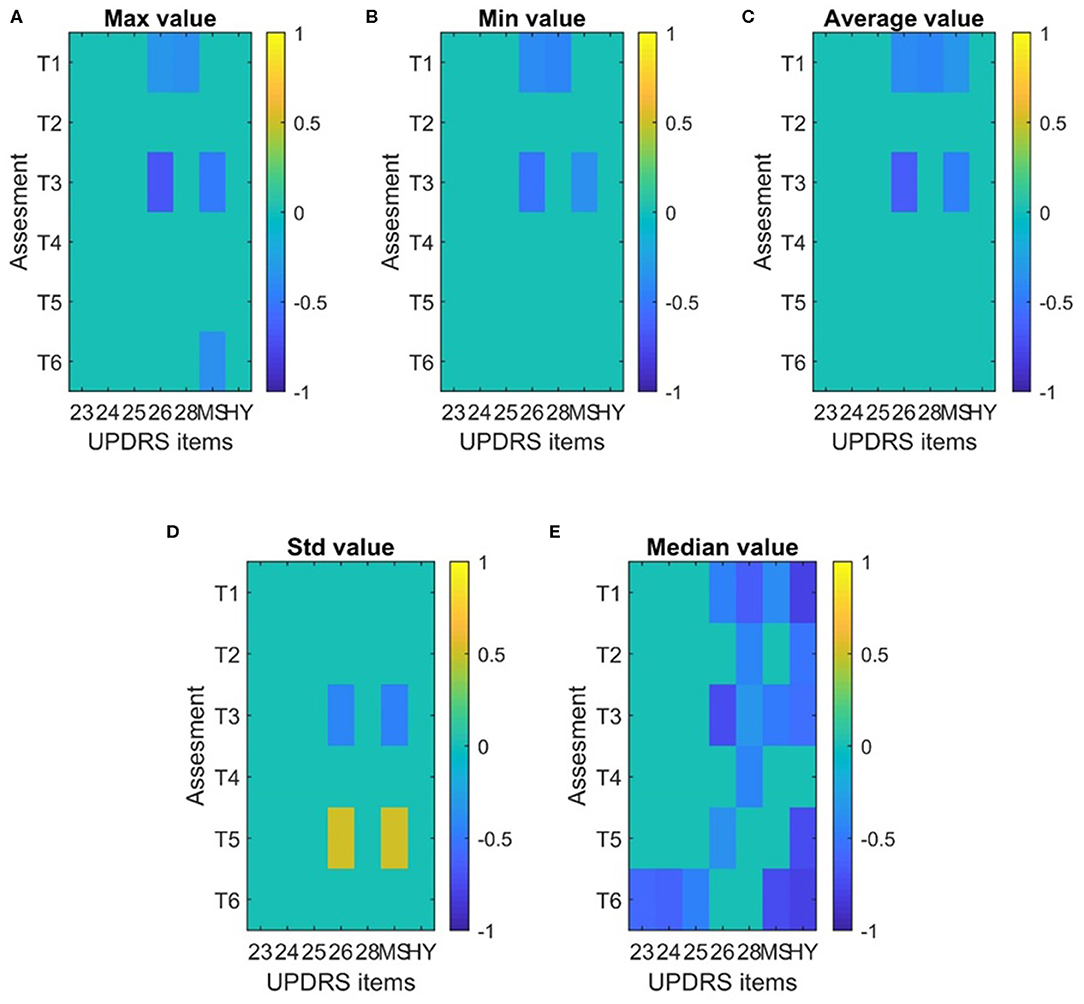
Figure 5. Correlation analysis results (r matrix) between the UPDRS Part III items and the features extracted from the Assessment Tests FinalMotorScore data, i.e., (A) Max value, (B) Min value, (C) Average value, (D) Std value, and (E) Median value. Inactive and active r matrix elements are defined as (p > a; r = 0) and (p < a; r ∈ [-1,l]r≠o), accordingly.
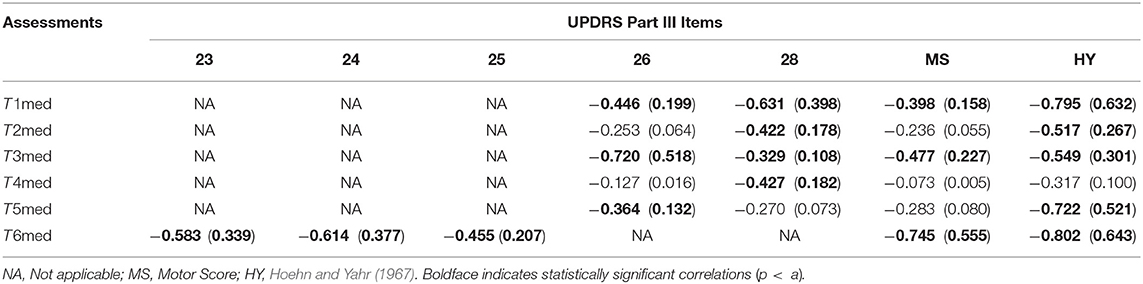
Table 3. Estimated correlation r and coefficient of determination (r2) between the median values of the Final Motor Score for (T1 − T6) and the UPDRS Part III Items.
Figure 6 illustrates the scatter plots of the Median FinalMotorScore values with all correlated pairs of the clinical feature set, i.e., (T1med:T5med, {26, 28, MS, HY}) and (T6med, {23, 24, 25, MS, HY}); the corresponding estimated r, r2, and linear regression lines and equations are also superimposed on the scatter plots, accordingly. The scatter plots and the regression lines in Figure 6 facilitate the graphical expression of the correlation degree between the examined data pairs, showing tight (high degree) or loose (low degree) data distribution around the regression line.
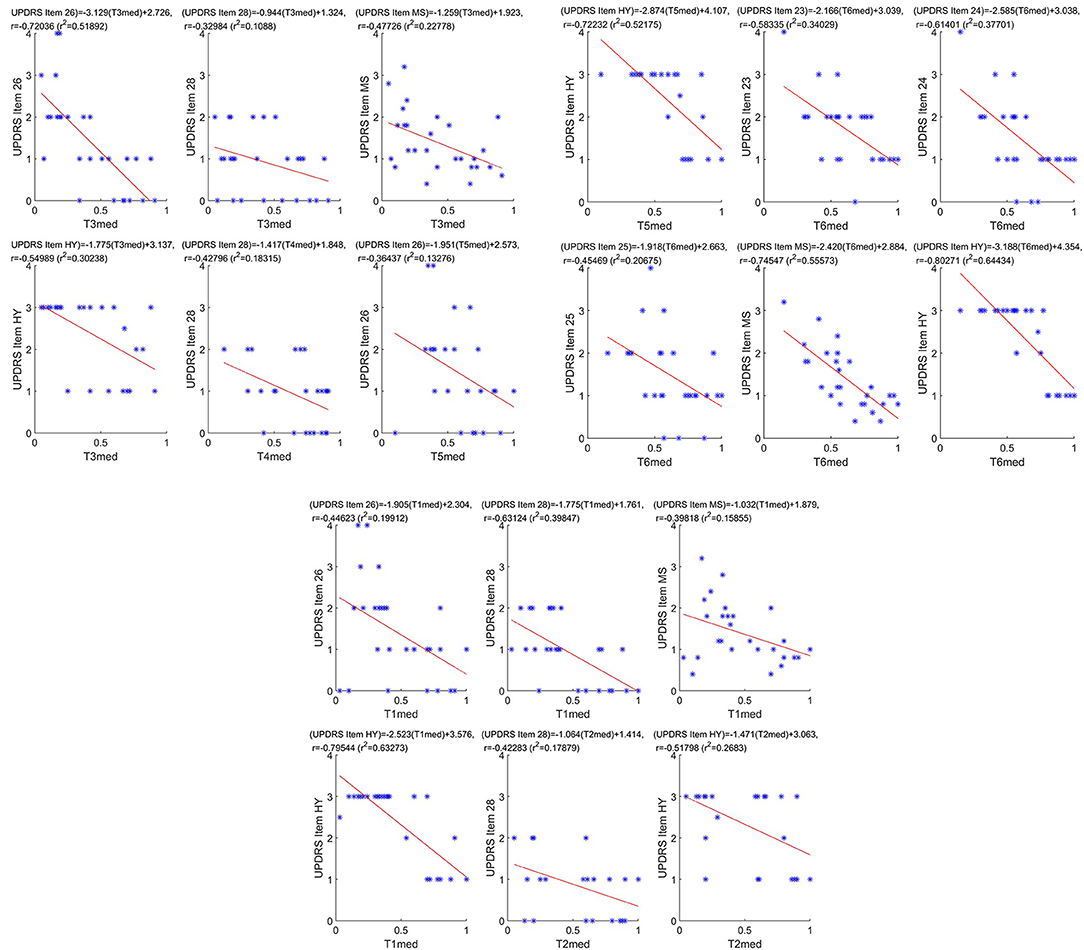
Figure 6. Scatter plots of the Median FinalMotorScore values with all correlated pairs of the clinical feature set, i.e., (T1med:T5med, {26,28, MS, HY}) and (T6med,{23,24,25, MS, HY}); the corresponding estimated r, (r2) and linear regression lines and equations are also superimposed on the scatter plots, accordingly.
The lowest correlation (absolute) value seen between T3med and UPDRS Part III Item 28 (Table 3) can be explained by the fact that T3 test relates with the standing balance in one foot that should be sustained aside for 3 s across the test (see Table 1). According to the estimated UPDRS Part III Item 28 (Table 2), there is a dispersion in the Item 28 values across the PD patients, and a dispersion in the T2med values (Figure 6, middle panel, first row, middle subfigure) caused by the nature of the T3. In fact, the latter targets the assessment of postural instability, which is one of the four primary motor symptoms of PD. This viability, though, is explained by the fact that not all patients with PD experience issues with balance in the same way. Actually, each patient with PD eventually will get postural instability; yet, the severity of each type of balance impairments may be very different (Park et al., 2015). Apparently, this dispersed behavior is reflected in the relevant scatter plot of Figure 6, justifying the low values of the relevant correlation between T3med and UPDRS Part III Item 28. On the other hand, the highest correlation (absolute) value is seen between T6med and HY (Table 3), with T6 being based on eye–hand coordination movements (Table 2). In particular, the VMC that is involved in T6 requires normal cognitive executive functionality. This ability that transforms visual inputs into movement plans, along with motor-execution skills, is known to be impaired in PD patients (Inzelberg et al., 2008). This is evident in even early-stage PD patients and are deteriorated further as the PD progresses (Muilwijk et al., 2013). As HY expresses the severity of the PD, reduction in the related T6med values is expected, justifying the high correlation between them tabulated in Table 3 and illustrated in the increased negative inclination of the regression line seen in Figure 6 (last subfigure).
Age and Gender Effect
In order to examine if age and gender had any effect on the FinalScore, a linear regression analysis was performed between the {age, gender} and the Timed (i = 1 : 6), since these metrics have exhibited the highest absolute correlation with the corresponding UPDRS Part III Items (see Table 3). The linear regression analysis has resulted, for all cases, in p > 0.05 values, i.e., [; ; ; ; ; ], [; ; ; ; ; ]. These results clearly indicate that the proposed metrics are not affected by the age and gender of the PD patient.
Practical Implications
Early interventions via the use of objective assessments have a significant benefit to the older adults with PD, as from one hand, it is widely accepted that patients with PD who remain untreated, or insufficiently treated, will experience ongoing and substantial symptomatic deterioration and negative effects on their quality of life, whereas early intervention could slow disease progression, delayed and diminished symptoms, coping with related risks of frailty, falls, and depression, limiting deterioration of patient's quality of life and achieving long-term cost savings.
The Assessment Tests of the iPrognosis Games can be used as an important component of self-management, by bringing PD symptoms handling to patients at their own home, to be used and accessed at their convenience 24-7. Since the Assessment Tests of the iPrognosis Games have been designed to reflect the motor status of the PD patient and, as shown by the experimental results, the median score value exhibits a high negative correlation with the severity of the PD patient's motor symptoms, it can be used as a measure to monitor the evolution of the pathology and identify changes in their physical performance. For example, as shown in Figures 5, 6 and Table 3, T6med has statistically significant negative correlation with the UPDRS Part III Items 23, 24, and 25 that relate to hand skills. Clearly, early PD patients perform fast and smooth movements of their hands, exhibiting a good ability to move their hands toward the expert's hand target; this results in high T6med values. On the other hand, advanced-stage PD patients face significant challenges when moving their hands, subjected to the expert's video targets, resulting in low T6med values. Such results verify the connection of the T6med feature to the symptom of the exaggeration of long-latency reflexes, a major contributor to rigidity in PD (Tatton and Lee, 1975; Berardelli et al., 1983; Rothwell et al., 1983). PD patients experience them more intensely and they can occur even if the stretch is applied when muscles are not contracting. A motor control theory, known as “optimal feedback control” (Todorov and Jordan, 2002; Diedrichsen et al., 2010) states that for every mobility task, the motor system sets how easily the movement can be changed. Optimal feedback control theory suggests that the brain sets “feedback gains,” which determine how powerfully the hand resists alterations in trajectory (Liu et al., 2013). Taking into account the hypothesis that muscle tone, mediated by long-latency reflexes, normally sets the amount of limb stability, the interpretation of Parkinsonian rigidity at the level of motor control is feasible (Mazzoni et al., 2012). In this vein, the experimental results suggest that the iPrognosis Game Assessment Tests could contribute toward such direction, as they can be used as a tool that can serve as a PD motor impairment status evaluator. As the PD progresses, motor disorder symptoms are expected to become more severe; hence, monitoring of this deterioration via the proposed motor Assessment Tests is feasible, as the difficulties in following the expert's performance by the PD patient at more severe stages will be reflected in the gradual reduction of his/her subscores and final score [see Equation (7)]. Apparently, these deviations from the expert would signal profound motor symptom deterioration that can trigger an alarm to the caring physician, evoking more intensive interventions.
Moreover, the Assessment Tests of the iPrognosis Games are beyond the current state of the art, by individualizing the information based on automatically sensed and processed data from the smart devices, social interaction, and behavioral change information sources, enabling PD patients to understand their own health and its challenges, helping them to identify ambiguity to behavioral change and to develop their own appropriate action plans and have the self-confidence to improve their own health. Furthermore, the Assessment Tests of the iPrognosis Games have the potential to be adapted (easily and with low cost) in other diseases/conditions that would equally benefit from a behavioral change program, centered around physical activity (e.g., obesity/anorexia/diabetes type 2/osteoporosis/cancer/high blood pressure) and musculoskeletal injuries (e.g., osteoarthritis/back pain/soft tissue ruptures). This offers a significant potential for accessing new markets and provides the potential for a sustainable plan beyond the initial targeting of PD, which itself is a very sizeable market. Additionally, within the i-PROGNOSIS consortium, some initial contacts have been established with representatives from relevant stakeholders (e.g., national heath executive, health service regulators, healthy aging centers, insurance, and PD patient advocacy), forming a holistic strategy to adopt the iPrognosis Games and the Assessment Tests within the health system.
The monitoring of the motor feasibility offered by the proposed iPrognosis Assessment Tests could also be transferred to the assessment of the prodromal PD phase. For example, people that have higher propensity toward PD, such as PD relatives, could adopt it as a predictive assessment tool, to validate their motor skills and feasibility. It is estimated that 15% of people with PD have a family history of the condition (Jankovic and Tolosa, 2007). This motivates the use of ICT-based tools, such as the proposed iPrognosis Assessment Tests, to shed light upon motor skills dynamic alteration across time, setting the proposed approach at the center of efficient assessment, both at the pre- and post-PD phases.
As it was presented in Section Background, motor assessment of PD patients involves different types of clinimetrics indexes, with the most often used in the clinical practice being the H&Y stages of progression of the disease and the UPDRS. Hence, these indices are the gold standard for the evaluation of any ICT-based motor skills assessment approach. This was clearly followed in the proposed approach, as described in the correlation analysis (Section Correlation Analysis Results). As it is well-documented, most of the proposed ICT-based motor skills assessment tools are based on wearables, such as accelerometers placed on the user's body (i.e., on feet, wrist), that try to capture the user's movement parameters (Suppa et al., 2018). The proposed approach here, unlike the other approaches, adopts the concept of natural interfacing; hence, the user is free of any embedded sensor and his/her body becomes the information interface. This allows for more naturalistic expression of the motor gestures and fosters the objective observation of the user's motor skills status. Clearly, the proposed approach normalizes the technology to the user's needs and not vice versa, sustaining, at the same time, the high compatibility with the clinical evaluation scores.
Limitations
Clearly, the number of participants in the current study sets a limitation in the generalization of the findings; nevertheless, the controlled environment where the data were captured and clinically validated secures the validity of the findings, which establish a solid bed-set for the expansion and validation to large-scale pilots. Efforts toward such direction are already on the way, via the extension of the i-PROGNOSIS project for more than 4 years, reaching a wider PD community. Moreover, the proposed Assessment Tests target the motor skill impairment of the PD patients. Focus to fine motor skills assessment by handwriting testing is also considered to be integrated at the current technical setting, as a paperless handwriting assessment test (e.g., by touchless finger movements at predefined letter-/spiral drawing-driven paths seen on the screen). Furthermore, non-motor skills degradation is also evident in PD patients and additional Assessment Tests could be proposed. In this vein, a new Assessment Test has been designed that targets voice degradation; yet, it has not been validated in the clinical setting. Finally, at a step further toward such direction, a sleep assessment test is also explored, though this will demand a different technical setting, such as a smartwatch or sleep-dedicated hardware for sleep quality estimation, such as the Oura Ring (www.ouraring.com).
Conclusions
An early, innovative, and personalized intervention iPrognosis Assessment tool capable of monitoring and tracking the behavioral change of PD patients (mostly related to posture, walking/gait, agility, balance, and coordination impairments) was presented here. Twenty-seven early PD patients were involved in the present study performing six motor Assessment Tests-based experimental metrics, integrated in the iPrognosis Games, revealing high correlation with the medical validation indices and justifying the validity of the Assessment Tests in evaluating the progress of the PD state and symptoms. Future work includes the connection of the iPrognosis Game scores with the Assessment Tests scores and the clinical scores, so as to further evaluate the contribution of the iPrognosis Games interventions in the PD patient's motor skills enhancement. This will offer an integrated solution to the PD patient in confronting his/her motor skills degradation, providing him/her with a holistic tool that includes both reliable intervention and assessment means for his/her motor skills monitoring.
Data Availability Statement
All data generated and analyzed during the current study are available from the corresponding author on a reasonable request.
Ethics Statement
All the experimental and ethical protocols were approved by Bioethics Committee of the Aristotle University of Thessaloniki (AUTH) Medical School, Thessaloniki, Greece (401/31.01.2018), Ethik-Kommission an der Technischen Universität Dresden, Dresden, Germany (EK 451112017), and United Kingdom London – Surrey Borders Research Ethics Committee (18/LO/0074). Written informed consent was obtained from all PD patients participated in the study, for the publication of any potentially identifiable images or data included in this article.
Author Contributions
SD, JD, AG, KD, TS, PB, and LH conceived, designed, and developed the iPrognosis Assessment Test. VZ, ZK, SB, LK, and DT handled with the corresponding ethical approvals and conducted/performed the clinical evaluations and experiments. LH analyzed the data. All authors contributed for the conceptual design of the iPrognosis Assessment Test, discussed the results, and contributed to the manuscript.
Funding
The research leading to these results has received funding from the European Union's Horizon 2020 Research and Innovation Programme under grant agreement no. 690494—i-PROGNOSIS: Intelligent Parkinson early detection guiding novel supportive interventions.
Conflict of Interest
HS and GT were employed by the company PLUX, Wireless Biosignals. Moreover, KF, ET, and GL were employed by the company COSMOTE Kinites Tilepekoinonies AE.
The remaining authors declare that the research was conducted in the absence of any commercial or financial relationships that could be construed as a potential conflict of interest.
Acknowledgments
The authors would like to thank all PD patients who volunteered to participate in this study for their time, commitment, and contribution.
Footnotes
1. ^https://developer.microsoft.com/en-us/windows/kinect
2. ^https://www.nively.com/en/
3. ^In the “pull” test, the doctor stands behind the patient and asks to maintain his/her balance when pulled backwards.
References
Agostino, R., Currà, A., Giovannelli, M., Modugno, N., Manfredi, M., and Berardelli, A. (2003). Impairment of individual finger movements in Parkinson's disease. Mov. Disord. 18, 560–565. doi: 10.1002/mds.10313
Anzivino, S., Nollo, G., Conotter, V., Guandalini, G. M. A., Conti, G., and Tessarolo, F. (2019). “Designing multidimensional assessment of ICTs for elderly people: the UNCAP clinical study protocol,” in Ambient Assisted Living. ForItAAL 2018. Lecture Notes in Electrical Engineering, Vol. 544, eds A. Leone, A. Caroppo, G. Rescio, G. Diraco, and P. Siciliano (Cham: Springer), 47–69.
Balke, B. (1963). A Simple Field Test For The Assessment Of Physical Fitness. Oklahoma: Civil Aeromedical Research Institute, 1–8.
Behrman, A. L., Light, K. E., Flynn, S. M., and Thigpen, M. T. (2002). Is the functional reach test useful for identifying falls risk among individuals with Parkinson's disease? Arch. Phys. Med. Rehabil. 83, 538–542. doi: 10.1053/apmr.2002.30934
Benecke, R., Rothwell, J. C., Dick, J. P. R., Day, B. L., and Marsden, C. D. (1986). Performance of simultaneous movements inpatients with Parkinson's disease. Brain 109, 739–757. doi: 10.1093/brain/109.4.739
Berardelli, A., Sabra, A. F., and Hallett, M. (1983). Physiological mechanisms of rigidity in Parkinson's disease. J. Neurol. Neurosurg. Psychiatr. 46, 45–53. doi: 10.1136/jnnp.46.1.45
Berg, K. O., Wood-Dauphinee, S. L., Williams, J. I., and Maki, B. (1992). Measuring balance in the elderly: validation of an instrument. Canad. J. Public Health 83(Suppl 2), S7–S11.
Bernhardt, J., and Hill, K. (2005). “We only treat what it occurs to us to assess: the importance of knowledge-based assessment,” in Science-Based Rehabilitation: Theories into Practice, eds. K. Refshauge, L. Ada, E. Ellis (London: Butterworth Heinemann), 15–48.
Bleton, J. P., Teremetz, M., Vidailhet, M., Mesure, S., Maier, M. A., and Lindberg, P. G. (2014). Impaired force control in writer's cramp showing a bilateral deficit in sensorimotor integration. Mov. Disord. 29, 130–134. doi: 10.1002/mds.25690
Bloem, B. R., Grimbergen, Y. A., Cramer, M., Willemsen, M., and Zwinderman, A. H. (2001). Prospective assessment of falls in Parkinson's disease. J. Neurol. 248, 950–958. doi: 10.1007/s004150170047
Bohannon, R. W., Andrews, A. W., and Thomas, M. W. (1996). Walking speed: reference values and correlates for older adults. J. Orthop. Sports Phys. Ther. 24, 86–90. doi: 10.2519/jospt.1996.24.2.86
Burrus, C. S., Gopinath, R. A., and Guo, H. (1997). Introduction to Wavelets and Wavelet Transforms: A Primer. (Englewood Cliffs, NJ: Prentice-Hall).
Butland, R. J., Pang, J. A. C. K., Gross, E. R., Woodcock, A. A., and Geddes, D. M. (1982). Two-, six, and 12-minute walking tests in respiratory disease. Br. Med. J. 284:1607. doi: 10.1136/bmj.284.6329.1607
Cancela, J., Pastorino, M., Arredondo, M., Pansera, M., Pastor-Sanz, L., Villagra, F., et al. (2011). “Gait assessment in Parkinson's disease patients through a network of wearable accelerometers in unsupervised environments,” in Proceedings of the 2011 Annual International Conference of the Engineering in Medicine and Biology Society. (Boston, MA). 2233–2236.
Canning, C. G., Paul, S. S., and Nieuwboer, A. (2014). Prevention of falls in Parkinson's disease: a review of fall risk factors and the role of physical interventions. Neurodegener. Dis. Manage. 4, 203–221. doi: 10.2217/nmt.14.22
Cooper, J. A., Sagar, H. J., Jordan, N., Harvey, N. S., and Sullivan, E. V. (1991). Cognitive impairment in early, untreated Parkinson's disease and its relationship to motor disability. Brain 114, 2095–2122. doi: 10.1093/brain/114.5.2095
Cunningham, D. A., Rechnitzer, P. A., Pearce, M. E., and Donner, A. P. (1982). Determinants of self-selected walking pace across ages 19 to 66. J. Gerontol. 37, 560–564. doi: 10.1093/geronj/37.5.560
Dias, S. B., Diniz, J. A., Hadjidimitriou, S., Charisis, V., Konstantinidis, E., Bamidis, P. D., et al. (2016). Personalized game suite: a unified platform to sustain and improve the quality of life of parkinson's disease patients. Front. Human Neurosci. 10:23. doi: 10.3389/conf.fnhum.2016.220.00023
Dias, S. B., Diniz, J. A., Konstantinidis, E., Bamidis, P., Charisis, V., Hadjidimitriou, S., et al. (2017a). “On supporting Parkinson's disease patients: the i-PROGNOSIS personalized game suite design approach,” in Proceedings of the IEEE International Symposium on Computer-Based Medical Systems - CBMS 2017 (Thessaloniki, Greece).
Dias, S. B., Diniz, J. A., Konstantinidis, E., Savvidis, T., Bamidis, P., Jaeger, H., et al. (2018). “On exploring design elements in assistive serious games for parkinson's disease patients: the i-PROGNOSIS exergames paradigm,” in Proceedings of the 2nd International Conference on Technology and Innovation in Sports, Health and Wellbeing (TISHW 2018) (IEEE).
Dias, S. B., Konstantinidis, E., Diniz, J. A., Bamidis, P., Charisis, V., Hadjidimitriou, S., et al. (2017b). “Serious games as a means for holistically supporting Parkinson's Disease patients: The i-PROGNOSIS personalized game suite framework,” in Proceedings of the 9th International Conference on Virtual Worlds and Games for Serious Applications (VS-Games 2017), Sept 6-8, 2017. (Athens, Greece).
Diedrichsen, J., Shadmehr, R., and Ivry, R. B. (2010). The coordination of movement: optimal feedback control and beyond. Trends Cogn. Sci. 14, 31–39. doi: 10.1016/j.tics.2009.11.004
Dorsey, E. R., Constantinescu, R., Thompson, J. P., Biglan, K. M., Holloway, R. G., Kieburtz, K., et al. (2007). Projected number of people with Parkinson disease in the most populous nations, 2005 through 2030. Neurology 68, 384–386. doi: 10.1212/01.wnl.0000247740.47667.03
Elbers, R. G., van Wegen, E. E. H., Verhoef, J., and Kwakkel, G. (2013). Is gait speed a valid measure to predict community ambulation in patients with Parkinson's disease? J. Rehab. Med. 45, 370–375. doi: 10.2340/16501977-1123
Fahn, S., and Elton, R. L. (1987). “Members of the UPDRS development committee. unified parkinson's disease rating scale,” in Recent Developments in Parkinson's Disease, eds. S. Fahn, C.D. Marsden, D. Calne, and N. Holstein (Plorhan Park, NJ: Macmillian Healthcare Information), 153–163.
Fava, G. A., Tomba, E., and Sonino, N. (2012). Clinimetrics: the science of clinical measurements. Int. J. Clin. Practice 66.1, 11–15. doi: 10.1111/j.1742-1241.2011.02825.x
Fawcett, A. L. (2007). Principles of Assessment and Outcome Measurement for Occupational Therapists and Physiotherapists: Theory, Skills and Application. (Chichester: Wiley).
Ferraris, C., Nerino, R., Chimienti, A., Pettiti, G., Cau, N., Cimolin, V., et al. (2019). Feasibility of home-based automated assessment of postural instability and lower limb impairments in Parkinson's disease. Sensors 19:1129. doi: 10.3390/s19051129
Ferraris, C., Nerino, R., Chimienti, A., Pettiti, G., Pianu, D., Albani, G., et al. (2014). “Remote monitoring and rehabilitation for patients with neurological diseases,” in Proceedings of the 10th International Conference on Body Area Networks (BODYNETS 2014) (London). 76–82.
Flowers, K. (1978). Lack of prediction in the motor behaviour of Parkinsonism Brain 101, 35–52. doi: 10.1093/brain/101.1.35
Franchignoni, F., Horak, F., Godi, M., Nardone, A., and Giordano, A. (2010). Using psychometric techniques to improve the balance evaluation systems test: the mini-BEStest. J Rehabil. Med. 42, 323–331. doi: 10.2340/16501977-0537
Fucetola, R., and Smith, M. C. (1997). Distorted visual feedback effects on drawing in Parkinson's disease. Acta Psychol. 95, 255–266. doi: 10.1016/S0001-6918(96)00043-1
Fugl-Meyer, A. R., Jääskö, L., Leyman, I., Olsson, S., and Steglind, S. (1975). The post-stroke hemiplegic patient. 1. a method for evaluation of physical performance. Scand. J. Rehabil. Med. 7, 13–31.
Galna, B., Barry, G., Jackson, D., Mhiripiri, D., Olivier, P., and Rochester, L. (2014). Accuracy of the Microsoft Kinect sensor for measuring movement in people with Parkinson's disease. Gait Posture 39, 1062–1068. doi: 10.1016/j.gaitpost.2014.01.008
Giuberti, M., Ferrari, G., Contin, L., Cimolin, V., Azzaro, C., Albani, G., et al. (2014). “Linking UPDRS scores and kinematic variables in the leg agility task of Parkinsonians,” in Proceedings of 11th International Conference on Wearable and Implantable Body Sensor Networks (BSN) (Zurich, Switzerland). 1–6.
Goetz, C. G., Tilley, B. C., Shaftman, S. R., Stebbins, G. T., Fahn, S., Martinez-Martin, P., et al. (2008). Movement disorder society UPDRS revision task force. Movement disorder society-sponsored revision of the unified Parkinson's Disease rating scale (MDS-UPDRS): scale presentation and clinimetric testing results. Mov. Disord. 23, 2129–2170. doi: 10.1002/mds.22340
Grammatikopoulou, A., Dimitropoulos, K., Bostantjopoulou, S., Katsarou, Z., and Grammalidis, N. (2019). “Motion analysis of Parkinson diseased patients using a video game approach,” in Proceedings of the 12th ACM International Conference on Pervasive Technologies Related to Assistive Environments (Rhodes), 523–527.
Handojoseno, A. A., Shine, J. M., Nguyen, T. N., Tran, Y., Lewis, S. J., and Nguyen, H. T. (2012). “The detection of freezing of gait in Parkinson's disease patients using EEG signals based on wavelet decomposition,” in Proceedings of the 2012 Annual International Conference of the Engineering in Medicine and Biology Society (EMBC) (San Diego, CA), 69–72.
Hocherman, S., and Aharon-Peretz, J. (1994). Two-dimensional tracing and tracking in patients with Parkinson's disease. Neurology 44, 111–116. doi: 10.1212/WNL.44.1.111
Hocherman, S., and Giladi, N. (1998). Visuomotor control abnormalities in patients with unilateral Parkinsonism. Neurology 50, 1648–1654. doi: 10.1212/WNL.50.6.1648
Hoehn, M. M., and Yahr, M. D. (1967). Parkinsonism: onset, progression and mortality. Neurology 17, 427–442. doi: 10.1212/WNL.17.5.427
Holden, M. K., Gill, K. M., Magliozzi, M. R., Nathan, J., and Piehl-Baker, L. (1984). Clinical gait assessment in the neurologically impaired: Reliability and meaningfulness. Phys. Therapy 64, 35–40. doi: 10.1093/ptj/64.1.35
Horak, F. B., Wrisley, D. M., and Frank, J. (2009). The Balance Evaluation Systems Test (BESTest) to differentiate balance deficits. Phys. Ther. 89, 484–498. doi: 10.2522/ptj.20080071
Ingvarsson, P. E., Gordon, A. M., and Forssberg, H. (1997). Coordination of manipulative forces in Parkinson's disease. Exp. Neurol. 145, 489–501. doi: 10.1006/exnr.1997.6480
Inzelberg, R., Schechtman, E., and Hocherman, S. (2008). Visuo-motor coordination deficits and motor impairments in Parkinson's disease. PLoS ONE 3:e3663. doi: 10.1371/journal.pone.0003663
Jankovic, J., and Tolosa, E. (eds.). (2007). Parkinson's Disease and Movement Disorders. (Lippincott Williams & Wilkins).
Jebsen, R. H., Taylor, N. E. A. L., Trieschmann, R. B., Trotter, M. J., and Howard, L. A. (1969). An objective and standardized test of hand function. Arch. Phys. Med. Rehabil. 50, 311–319.
Johnson, M. T., Kipnis, A. N., Coltz, J. D., Gupta, A., Silverstein, P., et al. (1996). Effects of levodopa and viscosity on the velocity and accuracy of visually guided tracking in Parkinson's disease. Brain 119, 801–813. doi: 10.1093/brain/119.3.801
Kellor, M., Frost, J., Silberberg, N., Iversen, I., and Cummings, R. (1971). Hand strength and dexterity. Am. J. Occup. Ther. 25, 77–83.
Keus, S. H. J., Hendriks, H. J. M., and Bloem, B. R. (2006). KNGF guidelines for physical therapy in patients with Parkinson's disease. Amersfoort: Royal Dutch Society for Physical Therapy. Available online at: http://www.appde.eu/pdfs/Dutch%20Parkinson%27s%20Physiotherapy%20Guidelines.pdf (accessed December, 2019).
King, L. A., Salarian, A., Mancini, M., Priest, K. C., Nutt, J., Serdar, A., et al. (2013). Exploring outcome measures for exercise intervention in people with Parkinson's disease. Parkinsons Dis. 2013:572134. doi: 10.1155/2013/572134
Knippenberg, E., Verbrugghe, J., Lamers, I., Palmaers, S., Timmermans, A., and Spooren, A. (2017). Markerless motion capture systems as training device in neurological rehabilitation: A systematic review of their use, application, target population and efficacy. J. Neuroeng. Rehabil. 14:61. doi: 10.1186/s12984-017-0270-x
Liu, S., Shen, Z., Mei, J., Ji, J., and Miao, C. (2013). Parkinson's disease predictive analytics through a pad game based on personal data. Int. J. Inf. Technol. 19, 1–17. Available online at: http://www.ntulily.org/wp-content/uploads/journal/Parkinsons_Disease_Predictive_Analytics_through_a_Pad_Game_Based_on_Personal_Data_accepted.pdf
Long, D., Wang, J., Xuan, M., Gu, Q., Xu, X., Kong, D., et al. (2012). Automatic classification of early Parkinson's disease with multi-modal MR imaging. PLoS ONE 7:e47714. doi: 10.1371/journal.pone.0047714
Lyle, R. C. (1981). A performance test for assessment of upper limb function in physical rehabilitation treatment and research. Int. J. Rehabil. Res. 4, 483–492. doi: 10.1097/00004356-198112000-00001
Martinez-Martin, P., and Forjaz, M. J. (2006). Metric attributes of the unified Parkinson's disease rating scale 3.0 battery: Part I, feasibility, scaling assumptions, reliability, and precision. Mov. Disord. 21, 1182–1188. doi: 10.1002/mds.20916
Mazzoni, P., Shabbott, B., and Cortés, J. C. (2012). Motor control abnormalities in Parkinson's disease. Cold Spring Harb. Perspect. Med. 2:a009282. doi: 10.1101/cshperspect.a009282
Morris, M. E. (2000). Movement disorders in people with Parkinson disease: a model for physical therapy. Phys. Ther. 80, 578–597. doi: 10.1093/ptj/80.6.578
Morris, S., Morris, M. E., and Iansek, R. (2001). Reliability of measurements obtained with the Timed “Up & Go” test in people with Parkinson disease. Phys. Ther. 81, 810–818. doi: 10.1093/ptj/81.2.810
Muilwijk, D., Verheij, S., Pel, J. J., Boon, A. J., and van der Steen, J. (2013). Changes in Timing and kinematics of goal directed eye-hand movements in early-stage Parkinson's disease. Transl. Neurodegener. 2:1. doi: 10.1186/2047-9158-2-1
Opara, J., Malecki, A., Malecka, E., and Socha, T. (2017). Motor assessment in Parkinson's disease. Ann. Agric. Environ. Med. 24, 411–415. doi: 10.5604/12321966.1232774
Ornelas-Vences, C., Sánchez-Fernández, L. P., Sánchez-Pérez, L. A., and Martínez-Hernández, J. M. (2019). Computer model for leg agility quantification and assessment for Parkinson's disease patients. Med. Biol. Eng. Comp. 57, 463–476. doi: 10.1007/s11517-018-1894-0
Otte, K., Kayser, B., Mansow-Model, S., Verrel, J., Paul, F., Brandt, A. U., et al. (2016). Accuracy and reliability of the kinect version 2 for clinical measurement of motor functions. PLoS ONE 11:e0166532. doi: 10.1371/journal.pone.0166532
Park, J. H., Kang, Y. J., and Horak, F. B. (2015). What is wrong with balance in Parkinson's disease? J. Move. Disord. 8, 109–114. doi: 10.14802/jmd.15018
Patel, S., Lorincz, K., Hughes, R., Huggins, N., Growdon, J., Standaert, D., et al. (2009). Monitoring motor fluctuations in patients with Parkinson's disease using wearable sensors. IEEE Trans. Inf. Technol. Biomed. 13, 864–873. doi: 10.1109/TITB.2009.2033471
Peto, V., Jenkinson, C., Fitzpatrick, R., et al. (1995). The development and validation of a short measure of functioning and well being for individuals with Parkinson's disease. Qual Life Res. 4, 241–248. doi: 10.1007/BF02260863
Petsani, D., Mantziari, D., Zilidou, V., Konstantinidis, E. I., Billis, A., Timoleon, M., et al. (2019). “Co-design the future CAPTAIN system with older adults: focusing on the e-coaching dimensions,” in Proceedings of the 12th ACM International Conference on Pervasive Technologies Related to Assistive Environments (Rhodes), 639–644.
Podsiadło, D., and Richardson, S. (1989). The timed “Up and Go” test. Arch. Phys. Med. Rehabil. 67, 387–389.
Powell, L. E., and Myers, A. M. (1995). The activities-specific balance confidence (ABC) scale. J. Gerontol. A Biol. Sci. Med. Sci. 50, M28–M34. doi: 10.1093/gerona/50A.1.M28
Pradhan, S., Scherer, R., Matsuoka, Y., and Kelly, V. E. (2015). Grip force modulation characteristics as a marker for clinical disease progression in individuals with Parkinson disease: case control study. Phys Ther 95, 369–379. doi: 10.2522/ptj.20130570
Procházka, A., Vyšata, O., Vališ, M., Tupa, O., Schätz, M., and Marík, V. (2015). Bayesian classification and analysis of gait disorders using image and depth sensors of Microsoft Kinect. Digit. Signal Process. 47, 169–177. doi: 10.1016/j.dsp.2015.05.011
Rocha, A. P., Choupina, H., Fernandes, J. M., Rosas, M. J., Vaz, R., and Silva Cunha, J. P. (2015). “Kinect v2 based system for Parkinson's disease assessment,” in Proceedings of the IEEE 37th Annual International Conference on Engineering in Medicine and Biology Society (EMBC) (Milan, Italy). 1279–1282.
Rochester, L., Hetherington, V., Jones, D., Nieuwboer, A., Willems, A. M., Kwakkel, G., et al. (2004). Attending to the task: Interference effects of functional tasks on walking in Parkinson's disease and the roles of cognition, depression, fatigue, and balance. Arch. Phys. Med. Rehabil. 85, 1578–1585. doi: 10.1016/j.apmr.2004.01.025
Rothwell, J. C., Obeso, J. A., Traub, M. M., and Marsden, C. D. (1983). The behaviour of the long-latency stretch reflex in patients with Parkinson's disease. J. Neurol. Neurosurg. Psychiatr. 46, 35–44. doi: 10.1136/jnnp.46.1.35
Ruonala, V., Meigal, A., Rissanen, S., Airaksinen, O., Kankaanpaa, M., and Karjalainen, P. (2013). “EMG signal morphology in essential tremor and parkinson's disease,” in Proceedings of the 35th Annual International Conference of the Engineering in Medicine and Biology Society (EMBC), (Osaka), 5765–5768.
Savvidis, T., Konstantinidis, E., Dias, S. B., Diniz, J. A., Hadjileontiadis, L. J., and Bamidis, P. (2018). Exergames for Parkinson's Disease patients: how participatory design led to technology adaptation. Stud. Health Technol. Inform. 251, 78–81. doi: 10.3233/978-1-61499-880-8-78
Savvidis, T., Konstantinidis, E., Dias, S. B., Zilidou, V., Romanopoulou, E., Hadjileontiadis, L. J., et al. (2019). “Co-creating Exergames with Parkinson's Disease Patients,” in Proceedings of the 8th Pan-Hellenic Conference on Biomedical Technology (ELEVIT 2019) (Athens).
Schlachetzki, J. C., Barth, J., Marxreiter, F., Gossler, J., Kohl, Z., Reinfelder, S., et al. (2017). Wearable sensors objectively measure gait parameters in Parkinson's disease. PLoS ONE 12:e0183989. doi: 10.1371/journal.pone.0183989
Schwab, R. S., Chafez, M. E., and Walker, S. (1954). Control of two simultaneous voluntary motor acts in normals and in Parkinsonism. Arch. Neurol. Psych. 72, 591–598. doi: 10.1001/archneurpsyc.1954.02330050061010
Shimoyama, I., Ninchoji, T., and Uemura, K. (1990). The finger-tapping test. a quantitative analysis. Arch. Neurol. 47, 681–684. doi: 10.1001/archneur.1990.00530060095025
Sødring, K. M. (1994). The Sødring Motor Evaluation of Stroke Patients Manual. Oslo: Clinic for Geriatrics and Rehabilitation Medicine, Ullevål University Hospital.
Song, J., Sigward, S., Fisher, B., and Salem, G. J. (2012). Altered dynamic postural control during step turning in persons with early-stage parkinson's disease. Parkinson's Dis. 2012:386962. doi: 10.1155/2012/386962
Stawarz, M., Polański, A., Kwiek, S., Boczarska-Jedynak, M., Janik, L., Przybyszewski, A., et al. (2012). “A system for analysis of tremor in patients with Parkinson's disease based on motion capture technique,” in Computer Vision and Graphics. ICCVG 2012. Lecture Notes in Computer Science, Vol. 7594, eds L. Bolc, R. Tadeusiewicz, L. J. Chmielewski, and K. Wojciechowski (Berlin; Heidelberg: Springer), 618–625.
Stern, Y., Mayeux, R., Rosen, J., and Ilson, J. (1983). Perceptual motor dysfunction in Parkinson's disease: a deficit in sequential and predictive voluntary movement. J. Neurol. Neurosurg. Psychiatr. 46, 145–151. doi: 10.1136/jnnp.46.2.145
Stone, E. E., and Skubic, M. (2015). Fall detection in homes of older adults using the Microsoft Kinectics. IEEE J. Biomed. Health Inf. 19, 290–301. doi: 10.1109/JBHI.2014.2312180
Suppa, A., Irrera, F., and Cabestany, J. (2018). New advanced wireless technologies for objective monitoring of motor symptoms in Parkinson's Disease. Front. Neurol. 9:216. doi: 10.3389/978-2-88945-486-0
Tanner, C. M., Brandabur, M., and Dorse, E. R. (2008). Research Reports-Parkinson's Disease: A Global View. Parkinson Report, 9–11.
Tatton, W. G., and Lee, R. G. (1975). Evidence for abnormal long-loop reflexes in rigid Parkinsonian patients. Brain Res. 100, 671–676. doi: 10.1016/0006-8993(75)90167-5
Tiffin, J., and Asher, E. J. (1948). The purdue pegboard: norms and studies of reliability and validity. J. Appl. Psychol. 32:234. doi: 10.1037/h0061266
Tinetti, M. E. (1986). Performance-oriented assessment of mobility problems in elderly patients. J. Am. Geriatr. Soc. 34, 119–126. doi: 10.1111/j.1532-5415.1986.tb05480.x
Todorov, E., and Jordan, M. I. (2002). Optimal feedback control as a theory of motor coordination. Nat. Neurosci. 5, 1226–1235. doi: 10.1038/nn963
Tyson, S. F., and DeSouza, L. H. (2002). The Brunel balance assessment: a new measure of balance disability post-stroke. Physiotherapy 88:700. doi: 10.1016/S0031-9406(05)60114-9
Uitti, R. J., Baba, Y., Wszolek, Z. K., and Putzke, D. J. (2005). Defining the Parkinson's disease phenotype: Initial symptoms and baseline characteristics in a clinical cohort. Parkinsonism Relat. Disord. 11, 139–145. doi: 10.1016/j.parkreldis.2004.10.007
Utsumi, H., Terashi, H., Ishimura, Y., Takazawa, T., Okuma, Y., Yoneyama, M., et al. (2012). How far do the complaints of patients with Parkinson's disease reflect motor fluctuation? Quantitative analysis using a portable gait rhythmogram. ISRN Neurol. 2012:372030. doi: 10.5402/2012/372030
van der Burg, J. C., van Wegen, E. E., Rietberg, M. B., Kwakkel, G., van Dieën, J. H., et al. (2006). Postural control of the trunk during unstable sitting in Parkinson's disease. Parkinsonism Relat. Disord. 12, 492–498. doi: 10.1016/j.parkreldis.2006.06.007
van der Lee, J. H., Roorda, L. D., Beckerman, H., Lankhorst, G. J., and Bouter, L. M. (2002). Improving the action research arm test: a unidimensional hierarchical scale. Clin. Rehabil. 16, 646–653. doi: 10.1191/0269215502cr534oa
Wade, D. T., Langton-Hewer, R., Wood, V. A., Skilbeck, C. E., and Ismail, H. M. (1983). The hemiplegic arm after stroke: measurement and recovery. J. Neurol. Neurosurg. Psychiatr. 46, 521–524. doi: 10.1136/jnnp.46.6.521
Wolf, S. L., Catlin, P. A., Gage, K., Gurucharri, K., Robertson, R., and Stephen, K. (1999). Establishing the reliability and validity of measurements of walking time using the emory functional ambulation profile. Phys. Ther. 79, 1122–1133. doi: 10.1093/ptj/79.12.1122
Wolf, S. L., Lecraw, D. E., Barton, L. A., and Jann, B. B. (1989). Forced use of hemiplegic upper extremities to reverse the effect of learned nonuse among chronic stroke and head-injured patients. Exp. Neurol. 104, 125–132. doi: 10.1016/S0014-4886(89)80005-6
Yang, K., Xiong, W. X., Liu, F. T., Sun, Y. M., Luo, S., Ding, Z. T., et al. (2016). Objective and quantitative assessment of motor function in Parkinson's disease—from the perspective of practical applications. Ann. Transl. Med. 4:90. doi: 10.21037/atm.2016.03.09
Keywords: i-PROGNOSIS, parkinson's disease (PD), motor assessment tests, unified parkinson disease rating scale (UPDRS) part III, motor skills decline
Citation: Dias SB, Grammatikopoulou A, Diniz JA, Dimitropoulos K, Grammalidis N, Zilidou V, Savvidis T, Konstantinidis E, Bamidis PD, Jaeger H, Stadtschnitzer M, Silva H, Telo G, Ioakeimidis I, Ntakakis G, Karayiannis F, Huchet E, Hoermann V, Filis K, Theodoropoulou E, Lyberopoulos G, Kyritsis K, Papadopoulos A, Delopoulos A, Trivedi D, Chaudhuri KR, Klingelhoefer L, Reichmann H, Bostantzopoulou S, Katsarou Z, Iakovakis D, Hadjidimitriou S, Charisis V, Apostolidis G and Hadjileontiadis LJ (2020) Innovative Parkinson's Disease Patients' Motor Skills Assessment: The i-PROGNOSIS Paradigm. Front. Comput. Sci. 2:20. doi: 10.3389/fcomp.2020.00020
Received: 21 February 2020; Accepted: 27 May 2020;
Published: 21 July 2020.
Edited by:
Gualtiero Volpe, University of Genoa, ItalyReviewed by:
Jan Mucha, Brno University of Technology, CzechiaYih-Ru Wu, Chang Gung Memorial Hospital, Taiwan
Copyright © 2020 Dias, Grammatikopoulou, Diniz, Dimitropoulos, Grammalidis, Zilidou, Savvidis, Konstantinidis, Bamidis, Jaeger, Stadtschnitzer, Silva, Telo, Ioakeimidis, Ntakakis, Karayiannis, Huchet, Hoermann, Filis, Theodoropoulou, Lyberopoulos, Kyritsis, Papadopoulos, Delopoulos, Trivedi, Chaudhuri, Klingelhoefer, Reichmann, Bostantzopoulou, Katsarou, Iakovakis, Hadjidimitriou, Charisis, Apostolidis and Hadjileontiadis. This is an open-access article distributed under the terms of the Creative Commons Attribution License (CC BY). The use, distribution or reproduction in other forums is permitted, provided the original author(s) and the copyright owner(s) are credited and that the original publication in this journal is cited, in accordance with accepted academic practice. No use, distribution or reproduction is permitted which does not comply with these terms.
*Correspondence: Leontios J. Hadjileontiadis, leontios.hadjileontiadis@ku.ac.ae