ORIGINAL RESEARCH
Published on 17 Aug 2023
Improved prediction of MHC-peptide binding using protein language models
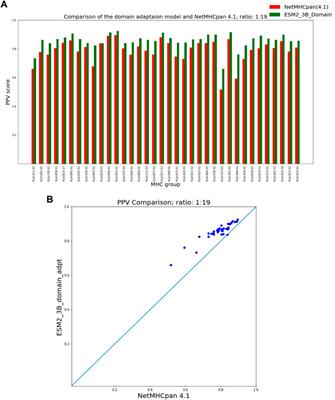
doi 10.3389/fbinf.2023.1207380
- 4,612 views
- 10 citations
2,869
Total downloads
15k
Total views and downloads
You will be redirected to our submission process.
ORIGINAL RESEARCH
Published on 17 Aug 2023
ORIGINAL RESEARCH
Published on 06 Apr 2023
ORIGINAL RESEARCH
Published on 17 Nov 2022
ORIGINAL RESEARCH
Published on 10 Oct 2022