ORIGINAL RESEARCH
Published on 08 Sep 2022
Low-power flexible organic memristor based on PEDOT:PSS/pentacene heterojunction for artificial synapse
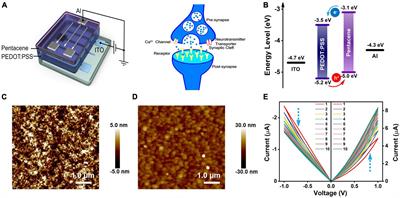
doi 10.3389/fnins.2022.1016026
- 5,079 views
- 26 citations
3,949
Total downloads
23k
Total views and downloads
ORIGINAL RESEARCH
Published on 08 Sep 2022
ORIGINAL RESEARCH
Published on 15 Aug 2022
ORIGINAL RESEARCH
Published on 27 Apr 2022
ORIGINAL RESEARCH
Published on 31 Jan 2022
ORIGINAL RESEARCH
Published on 05 Jan 2022