ORIGINAL RESEARCH
Published on 28 Nov 2022
Single-cell transcriptomic profiling reveals specific maturation signatures in human cardiomyocytes derived from LMNB2-inactivated induced pluripotent stem cells
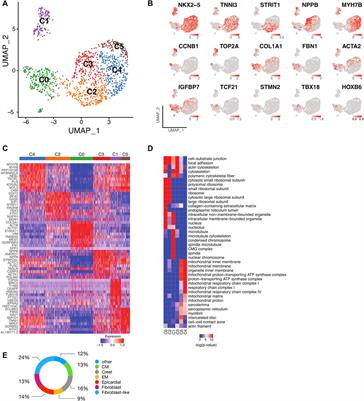
doi 10.3389/fcell.2022.895162
- 4,095 views
- 4 citations